Thank you for visiting nature.com. You are using a browser version with limited support for CSS. To obtain the best experience, we recommend you use a more up to date browser (or turn off compatibility mode in Internet Explorer). In the meantime, to ensure continued support, we are displaying the site without styles and JavaScript.
- View all journals
- Explore content
- About the journal
- Publish with us
- Sign up for alerts
- Review Article
- Published: 04 May 2021
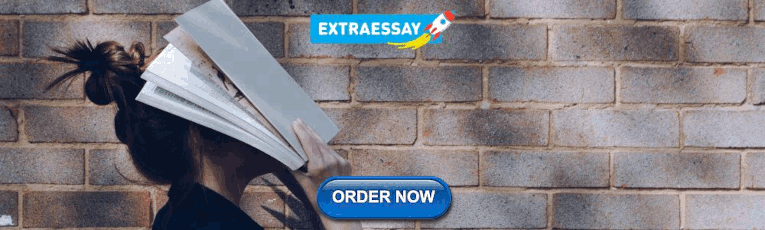
Testing at scale during the COVID-19 pandemic
- Tim R. Mercer 1 , 2 , 3 &
- Marc Salit 4 , 5
Nature Reviews Genetics volume 22 , pages 415–426 ( 2021 ) Cite this article
77k Accesses
210 Citations
949 Altmetric
Metrics details
- Genetic testing
- Genomic analysis
- Infectious diseases
Assembly and publication of the severe acute respiratory syndrome coronavirus 2 (SARS-CoV-2) genome in January 2020 enabled the immediate development of tests to detect the new virus. This began the largest global testing programme in history, in which hundreds of millions of individuals have been tested to date. The unprecedented scale of testing has driven innovation in the strategies, technologies and concepts that govern testing in public health. This Review describes the changing role of testing during the COVID-19 pandemic, including the use of genomic surveillance to track SARS-CoV-2 transmission around the world, the use of contact tracing to contain disease outbreaks and testing for the presence of the virus circulating in the environment. Despite these efforts, widespread community transmission has become entrenched in many countries and has required the testing of populations to identify and isolate infected individuals, many of whom are asymptomatic. The diagnostic and epidemiological principles that underpin such population-scale testing are also considered, as are the high-throughput and point-of-care technologies that make testing feasible on a massive scale.
Similar content being viewed by others
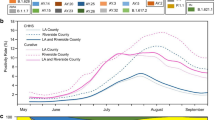
Local monitoring of SARS-CoV-2 variants in two large California counties in 2021
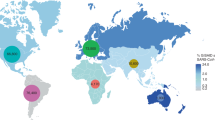
The next phase of SARS-CoV-2 surveillance: real-time molecular epidemiology
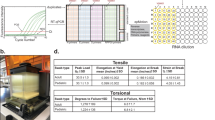
Development and implementation of a scalable and versatile test for COVID-19 diagnostics in rural communities
Introduction.
On 7 January 2020, a new coronavirus was detected by metatranscriptomic sequencing of lung fluid from a patient with pneumonia-like symptoms in Wuhan, China 1 , 2 . On 10 January 2020, the assembled reference genome of this new coronavirus, termed severe acute respiratory syndrome (SARS) coronavirus 2 (SARS-CoV-2), was published, and within 2 weeks the first diagnostic tests to detect the virus were issued 3 , 4 . To date, hundreds of millions of individuals have been tested for the presence of SARS-CoV-2 (ref. 5 ), leading to widespread public awareness of and debate regarding diagnostic concepts and technologies.
Testing for the presence of SARS-CoV-2 is typically performed for one of two reasons. First, a symptomatic patient might be tested to inform their clinical treatment. Such diagnostic testing is focused on therapeutic care and is typically performed in a well-controlled clinical setting, and the test results are usually interpreted alongside the patient’s history and symptoms. Second, testing might be performed to identify infectious individuals in a population, who are then isolated to prevent the onward infection of others. Such screening is focused on public health outcomes and aims to reduce viral transmission through a population. Individuals who are not symptomatic might be tested, and testing might need to be performed on a massive scale. These two different uses of testing have different requirements and priorities, and a test that is useful in clinical diagnosis can be ill-suited for population-scale screening.
The observation that countries with high testing rates were able to effectively control SARS-CoV-2 transmission during the initial stages of the pandemic 6 supported the proposal that screening could help to limit viral transmission. Accordingly, widespread testing rapidly gained traction as an intervention that might avoid both the immediate economic costs of lockdown and the societal costs of social distancing measures. Accordingly, many countries implemented population-scale testing to monitor and reduce viral transmission. However, despite the promise of this approach, obtaining reliable test results on such a massive scale is difficult and unprecedented, and has been achieved with differing outcomes and success 7 . Nonetheless, the COVID-19 pandemic has transformed the role of population-scale screening. With ongoing investment and innovation, large-scale testing is likely to become a common feature of public health.
This Review focuses on the use of testing as a tool to screen populations for individuals infected with SARS-CoV-2. We discuss the changing role of such testing in the COVID-19 pandemic: the initial use of genomic epidemiology to monitor the spread of viral strains around the world; the containment of disease outbreaks using contact tracing; and the massive screening programmes that aim to suppress community transmission (Table 1 ; Fig. 1 ). We also consider the testing strategies and technologies used to address the challenges of population-scale testing. We finally discuss findings from large-scale testing programmes that have measured the exposure of entire populations to SARS-CoV-2, and how these studies have evaluated and informed our response to the pandemic.
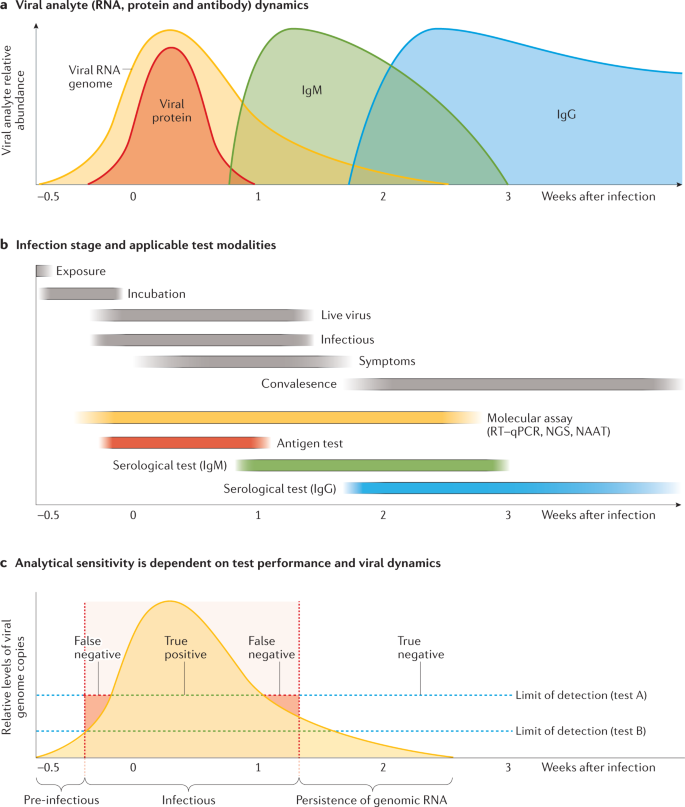
a | Shedding of the severe acute respiratory syndrome coronavirus 2 (SARS-CoV-2) RNA genome (yellow shading) typically increases rapidly following infection and peaks at the presentation of symptoms (although many patients can be asymptomatic) before gradually declining. Levels of viral proteins (red shading) also rapidly increase, albeit within a narrower window. In response to infection, the body produces IgM (green shading) and IgG (blue shading) antibodies, which persist for weeks to months. b | The SARS-CoV-2 RNA genome can be detected by molecular assays, such as reverse transcription–quantitative polymerase chain reaction (RT–qPCR), before the development of symptoms. Serological tests can detect reactive IgG and IgM, which indicate past infection with SARS-CoV-2. c | Assay sensitivity is dependent on both technical performance aspects and viral load. A test might not detect viral RNA even when an individual is infectious (false negative, red shaded area) or, alternatively, might detect persistent viral RNA after an individual is no longer infectious (false positive), which demonstrates that test positivity correlates poorly with infectivity. Owing to the rapid increase in viral shedding, only a narrow window exists wherein a more sensitive assay (test B) will outperform a less sensitive assay (test A). Note that this figure illustrates a generalized COVID-19 infectious course, and in practice the relative duration of detectability and analyte abundance differ considerably between individuals. NAAT, nucleic acid antigen testing; NGS, next-generation sequencing.
Genomic epidemiology of SARS-CoV-2
During replication and transmission, viral genomes accumulate mutations that can be used to define distinct variants. Whole-genome sequencing can identify these mutations and trace the transmission of variants between individuals, populations and countries around the world. This genomic epidemiology approach was used to monitor past outbreaks of Ebola virus and Zika virus infection 8 , 9 , 10 and has been widely used to reconstruct the spread of SARS-CoV-2. One of these SARS-CoV-2 strains, termed ‘B.1’, was initially transmitted to Italy, where it caused an outbreak in Lombardy before further circulating across Europe and onwards to the USA, where it seeded an outbreak in New York City 11 . This chain of transmission around the globe was reconstructed by the tracking of four mutations in the SARS-CoV-2 B.1 strain. The SARS-CoV-2 viral genome quickly diversified into different strains following the initial zoonotic transmission of the ancestral virus. During its initial spread, thousands of viral genomes were rapidly sequenced by research laboratories worldwide and shared in open-access databases such as the EpiCoV database from GISAID 12 and the Our World in Data COVID-19 dataset . These sequences, along with metadata (including location, date and method of sampling) and tools such as Nextstrain 13 , could then be used to track the spread of SARS-CoV-2 strains 14 .
Genomic epidemiology has also been widely used to reconstruct the routes by which SARS-CoV-2 is introduced into and spreads within countries 15 , 16 , 17 , 18 , 19 . These studies show that SARS-CoV-2 is typically introduced into a country several times 20 . Subsequent transmission is stochastic, wherein a small number of infected individuals are responsible for the majority of secondary infections in ‘ superspreader ’ events 21 , 22 . Genomic epidemiology studies also reveal the routes of transmission into and across a country, and their results have informed public health containment measures such as regional lockdowns, quarantines and travel restrictions 23 .
Despite the usefulness of genomic epidemiology, the data should be interpreted with caution. Although infection with different strains can rule out a chain of transmission between two individuals, infections by the same strain do not necessarily prove a direct link in the chain of transmission 24 . Moreover, compared with other pathogenic viruses, the SARS-CoV-2 genome accumulates mutations slowly and the amount of viral sampling and sequencing has differed widely between countries. Low strain diversity, rapid disease spread and non-representative sampling can confound the results of genomic epidemiology studies, and our understanding of SARS-CoV-2 spread and evolution was especially unclear during the early stages of the COVID-19 pandemic 11 , 25 .
Testing of individuals for SARS-CoV-2
The RNA genome of SARS-CoV-2 can be directly detected by nucleic acid assays such as reverse transcription–quantitative polymerase chain reaction (RT–qPCR) tests, whereas the presence of viral proteins can be detected by an antigen test (Box 1 ). The detection of these analytes requires that a sample contains sufficient viral genome copies or levels of viral proteins that exceed the limit of detection for a given assay (Fig. 2 ). However, the abundance of these viral analytes varies dramatically between different anatomical locations and different stages of infection 26 . Thus, the ability to detect SARS-CoV-2 is ultimately framed by the biology of the virus. Indeed, the sensitivity of tests for detecting SARS-CoV-2 can be as dependent on the time and site sampled as it is on the technical performance of the assay 27 .
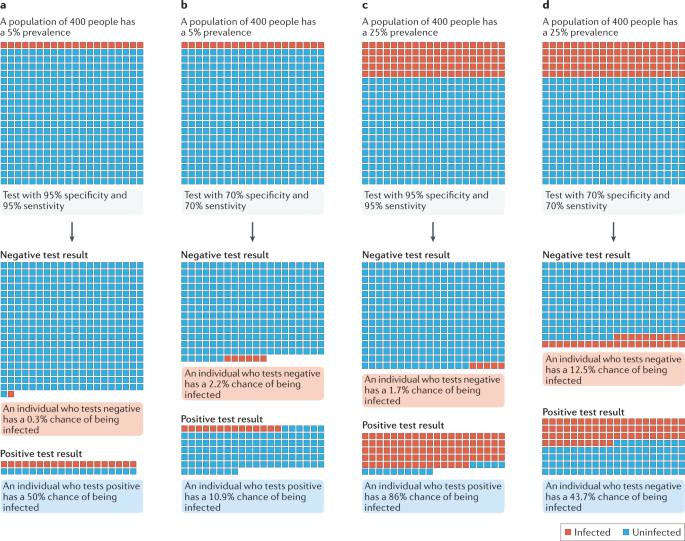
a , b | In a population with a low prevalence (5% here) of COVID-19 cases, even a highly sensitive and specific test (part a ) returns many false-positive results. c , d | By contrast, a high infection prevalence (25% here) increases the likelihood that a positive result is true, despite the test performance remaining unchanged. The positive predictive value (PPV) of a test describes the probability that an individual who tests positive is actually infected 35 , and thus depends on both the specificity of the test and the prevalence of infection. At low prevalence values, the proportion of false-positive results is increased and the PPV is reduced. Even a highly specific test returns mostly false-positive results (and therefore has a low PPV) when the prevalence of infection is low. Adapted from ref. 134 , Springer Nature Limited.
The viral load of SARS-CoV-2 changes dramatically during the course of infection 28 , 29 . Viral genome abundance rapidly increases following infection, and patients can become infectious and begin shedding viral particles 3–5 days before the development of COVID-19 symptoms 30 . During these early stages of infection, a nasopharyngeal sample (which requires a long swab to sample the back of the nasal cavity) generally provides the highest reported viral RNA abundance 31 , 32 . However, other, less invasive options, including oral swabs, nasal swabs or saliva tests, also have detectable levels of viral analytes and can reduce exposure of the health-care workers performing the sampling 33 .
Viral load typically declines gradually following the onset of symptoms. During the later stages of infection, samples from the lower respiratory tract (such as sputum, bronchoalveolar lavage fluid or tracheal secretions) can yield high viral titres. Even after the cessation of all symptoms, persistent viral RNA can be detected for several weeks. However, efforts to isolate replication-competent virus from this persistent RNA have been unsuccessful, and these recovered individuals are generally considered to be non-infectious 34 .
The response of an individual to the test result is a key factor in the effectiveness of testing programmes, and the interpretation of test results should consider both the probability of infection and any pre-existing risk factors 35 (Box 2 ; Fig. 2 ). Whereas a positive test result indicates the presence of infection and the need for isolation to prevent further transmission of the virus, a negative test result does not rule out the possibility of it being a false-negative finding 36 . If individuals are falsely reassured by receiving a negative test result, they might ignore other protection strategies and increase the risk of onward transmission of the virus 37 .
Following viral infection, the body mounts a humoral immune response that includes the production of antibodies that target SARS-CoV-2 antigens 38 , 39 , 40 . These antibodies directly bind to the spike protein and thereby neutralize viral action, as well as recruiting further immune responses to clear the virus. The levels of reactive (that is, anti-SARS-CoV-2) IgM antibodies typically increase within a few days of the viral infection and these antibodies persist for weeks, whereas reactive IgG antibodies appear in the middle and later stages of the infection and can remain circulating in the blood for several months 41 , 42 . Serological tests can detect reactive antibodies produced in response to viral exposure. As these tests do not directly detect the virus but instead detect the body’s response to the virus, they can indicate whether an individual was previously infected with SARS-CoV-2 (ref. 43 ). Serological tests can also indicate the strength and direction of an individual’s immune response. However, a positive serological test result does not necessarily indicate a protective immune response, and other anti-SARS-CoV-2 immunoglobulins (for example, IgA and IgE) are often not detected by available serological tests.
Box 1 Diagnostic technologies used in the COVID-19 pandemic
Reverse transcription–quantitative polymerase chain reaction
A method of severe acute respiratory syndrome coronavirus 2 (SARS-CoV-2) genome detection based on measuring the amplification of RdRP , E , N or S gene fragments using fluorescent probes 135 . Although reverse transcription–quantitative polymerase chain reaction (RT–qPCR) can provide a quantitative measure of viral abundance, it is most commonly used to detect viral presence above a quantitative cycle threshold chosen to minimize false positives. RT–qPCR is the current standard for population-scale testing 135 . Although this technique is theoretically capable of detecting very low quantities of the SARS-CoV-2 genome, its sensitivity in real-world clinical practice is only 50–70% 26 , 136 , 137 . The high specificity of RT–qPCR (~99%) can be further enhanced by targeting multiple loci. As RT–qPCR is typically conducted in large, centralized laboratories, efficient sample collection is critical to minimize reporting delays. RT–qPCR benefits from standardized operating procedures and a well-established supply chain.
Next-generation sequencing
Next-generation sequencing (NGS) was instrumental in the identification and assembly of the SARS-CoV-2 genome 1 , 2 and can also be used for high-throughput testing to identify mutations in the viral genome. As only a small amount of sequencing is required to detect amplified viral complementary DNA (cDNA), thousands of patient samples can be tested in a single sequencing run, which facilitates very large scale testing. Each sample is labelled with a unique molecular barcode that can be identified in the output library. Such barcoding can occur during initial reverse transcription, cDNA amplification or library preparation. The samples are then pooled and undergo multiplexed sequencing, an advance specific to large-scale testing 138 , 139 . Emerging findings indicate that NGS can distinguish between different SARS-CoV-2 variant strains and can also detect other respiratory viruses (such as influenza viruses) and synthetic RNA internal controls 140 .
Isothermal nucleic acid amplification assays
These assays use various nucleic acid amplification reactions that are conducted at a constant temperature. They generate large amounts of cDNA that can be detected by colorimetric or turbidimetric approaches. Examples include loop-mediated isothermal amplification 141 , 142 , nicking endonuclease amplification reaction, and recombinase polymerase amplification 143 . These approaches provide simple, rapid and cheap diagnostic tests that can be performed without specialized equipment. A preliminary report states that when reverse transcriptase is included in the reagent mix, this process can be done in a single step 144 ). However, the formation of non-specific products might require additional sequence-specific detection using NGS or CRISPR 145 .
Antigen tests
Antigen tests are based on antibodies that can bind to the SARS-CoV-2 spike protein or nucleocapsid protein. Bound antibodies are detected by use of a simple immunoassay, such as a lateral flow assay, which indicates the presence of viral protein 146 . These cost-effective tests can provide rapid results within 30 min at the point of care, and benefit from cheap and scalable manufacture. Despite these advantages, antigen tests tend to have lower specificity and sensitivity than corresponding nucleic acid-based assays 147 , 148 and are therefore usable across a narrower window of the SARS-CoV-2 infectious course.
Serological tests
These assays detect the presence of anti-SARS-CoV-2 IgM and/or IgG antibodies produced by the humoral immune response 38 . Serological assays can also indicate the extent and duration of immune protection offered by these antibodies, which is critical to understanding the success of vaccination programmes. The sensitivity of serological assays depends on both technical factors and antibody titres, which vary according to the duration and severity of infection, age and sex 149 , 150 . Serological tests for SARS-CoV-2 generally have reported specificities greater than 85%, although false positives can result from cross-reactivity with other coronaviruses 151 . Serological tests are particularly useful in epidemiological studies to determine the exposure of a population to SARS-CoV-2 (refs 64 , 65 , 66 ).
Box 2 Diagnostic and epidemiological statistics
Reproductive number
The reproductive number ( R ) is the average number of secondary individuals who are infected by a primary infected individual within a susceptible population 152 . R depends on the mode, duration and potency of viral infection, as well as the degree of contact between individuals, which is affected by population density, location, mobility and interventions such as social restrictions 153 , 154 , 155 . R is compounded with each infectious cycle, and models the compounded viral spread or collapse that is characteristic of pandemic waves over time. R > 1 indicates growing viral transmission, whereas R < 1 indicates decreasing viral transmission. For example, in the Italian municipality of Vo’, the SARS-CoV-2 R value fell from 2.49 before social lockdown to 0.41 afterwards 62 . Despite its advantages, the R value often does not capture the heterogeneous dynamics of viral transmission, wherein a small number of individuals cause the majority of secondary infections by ‘superspreader’ events 21 . This high stochasticity under low prevalence is characteristic of SARS-CoV-2 transmission, and is distinct from the lower stochasticity observed for other pathogens, such as influenza viruses.
Test positivity rate
The test positivity rate is the proportion of tests that return a positive result, which reflects the level of testing relative to the viral prevalence in a population. A low test positivity rate indicates low viral prevalence and adequate surveillance capacity of the testing scheme; a high test positivity rate indicates high viral prevalence or that testing is biased to symptomatic or selected individuals (positive results represent only a small fraction of the true number of infections). A rising test positivity rate suggests that viral transmission is faster than that seen in confirmed cases. Test positivity can be used with other metrics (such as R ) to inform intervention strategies. For example, the WHO recommends that test positivity remains less than 5% for at least 2 weeks before public health and social measures are changed 156 .
Sensitivity
Sensitivity is the fraction of individuals who are correctly identified as having the condition by a given test. A highly sensitive test returns few false-negative results because few infected individuals escape detection. The sensitivity of a test is often determined under controlled conditions and is solely dependent on test performance. In practice, variables such as sampling and processing errors decrease real-world sensitivity 157 . For example, ineffective swabbing has been cited as a major reason for decreased sensitivity, and some schemes require dual testing of nasopharyngeal and sputum or throat samples to improve performance.
Specificity
The specificity of a test refers to its ability to correctly identify a non-infected individual as not being infected 35 . Highly specific tests return few false-positive results because infection is erroneously diagnosed in few uninfected individuals. Tests with low specificity have the disadvantage that many uninfected individuals are erroneously identified as being infected and could potentially have to undergo unnecessary quarantine or receive unnecessary treatment. Low specificity is particularly problematic for large-scale testing schemes, as it can result in overwhelming absolute numbers of false positives.
Contact tracing
The introduction and initial outbreak of SARS-CoV-2 within a country can be contained by testing and contact tracing, wherein all infected individuals and their secondary contacts are identified and isolated. If these secondary exposed individuals are quarantined before they can infect others, further onward transmission of the virus is prevented 44 .
Large-scale contact tracing programmes have been instrumental in halting SARS-CoV-2 transmission in countries such as Vietnam, Taiwan and Japan 6 , 45 . Within days of detecting its first case on 20 January 2020, South Korea quickly scaled up the nation’s existing network of contact tracers, who had been used in the SARS-CoV outbreak in 2003. These contact tracers used patient interviews as well as medical, mobile phone and credit card transaction records to identify thousands of people linked to an outbreak in Itaewo, a popular nightclub district in Seoul. Subsequent testing identified and isolated hundreds of infected individuals, many of whom were separated by multiple cycles of transmission from the original Itaewo outbreak 46 .
Although simple in concept, contract tracing is often difficult to put into practice because the effectiveness of this approach depends on identifying contacts before they can infect others 47 , 48 . Given that individuals can become infectious within days of initial exposure to SARS-CoV-2 and before they develop symptoms, only a narrow window is available within which to identify individuals before they infect others. Therefore, rapid testing is of primary importance for effective contact tracing, and contacted individuals are recommended to pre-emptively isolate themselves while awaiting their test results.
Contact tracing is also laborious and time-consuming, and becomes increasingly difficult in the context of ongoing viral transmission. As more cases are diagnosed, the number of secondary contacts scales non-linearly to increasingly large numbers of people, who must in turn be identified, tested and isolated 49 . This increasing case burden results in delays that further decrease the effectiveness of contact tracing. The use of digital contact tracing using mobile apps and other technologies can help to automate contact tracing approaches and ease this burden, but requires widespread adoption and adherence 50 , 51 . Once the case burden exceeds a country’s contact tracing capacity, most individuals with secondary cases are contacted too late and tracing has little further effect on viral transmission.
Population-scale testing
Reducing community transmission.
Despite efforts to contain SARS-CoV-2, community transmission has become entrenched in many countries, which has required a shift from contact tracing to the population-scale testing required to detect the large fraction of presymptomatic, mildly symptomatic and asymptomatic individuals who can unwittingly transmit the virus 52 , 53 . On 31 October 2020, Slovakia became the first country to test its entire population in an attempt to reduce viral transmission 54 , 55 , 56 . This massive undertaking required thousands of health-care workers to test almost all citizens using a rapid antigen test, following which individuals who tested positive and their close contacts were advised to isolate themselves. Although the effects of this massive testing effort are difficult to distinguish from those of concurrent interventions, a marked reduction in SARS-CoV-2 infections was observed following the effort 57 . However, this reduction was limited to regions with high viral prevalence , and testing seemingly had little effect in regions with a lower viral prevalence 58 .
The success of population-scale testing can be precisely measured as a reduction in the reproductive number, which indicates the extent of viral transmission through a population (Box 2 ). However, the amount of testing that must be performed to markedly reduce the reproductive number is difficult to evaluate. The fraction of tests that return a positive result can provide some indication as to the adequacy of the testing programme: a low test positivity rate indicates both low viral prevalence and sufficient surveillance capacity, whereas a high test positivity rate suggests that testing is inadequate and that many infected individuals are going undetected 59 (Box 2 ).
For many countries, adequate surveillance mandates a massive amount of testing, requiring huge resources and a collaborative effort across a laboratory enterprise that includes commercial, clinical, government and research organizations 57 . Large coordinated systems are required for safe sample acquisition, transport and custody. In addition, test results must be returned sufficiently rapidly to enable individuals who test positive to isolate themselves before they infect others. Indeed, the speed and frequency of testing is considered as important as test sensitivity in effectively reducing viral transmission 58 . Such massive testing efforts can quickly strain supply chains and infrastructure, and many countries have faced shortages in key reagents 60 that left them unable to implement effective, widespread testing. As a result, testing of health-care workers and symptomatic individuals is often prioritized over testing of asymptomatic individuals. Competition for reagents has often reflected global health inequities, and wide disparities in testing rates are observed between countries 61 . However, containing SARS-CoV-2 transmission is a global challenge, and the global responsibility for sustainable testing must be recognized.
Monitoring of viral prevalence
Population-scale testing also provides critical viral prevalence data that have informed our response to the pandemic. On 21 February 2020, the town of Vo’ in Italy reported the first death from COVID-19 and quickly entered a complete lockdown for 2 weeks. Researchers were able to test almost every town resident and to quantify the effect of lockdown on reducing the viral reproduction number (Box 2 ), thereby providing early validation of the effectiveness of such interventions used to combat the pandemic 62 .
Population-scale testing enables the rate of viral spread through a population to be measured, aids in the identification of regional hotspots and high-risk subpopulations, and facilitates the calculation of incidence and mortality rates. However, as with any measurement process, such testing is subject to the errors and biases inherent in the assay and sampling processes. Low-sensitivity tests can underestimate viral prevalence, whereas low-specificity tests can overestimate prevalence. For example, an early study conducted in California, USA, was criticized for providing an inflated measure of SARS-CoV-2 prevalence owing to the use of low-specificity serological tests 63 .
Serological assays that detect reactive antibodies can indicate whether an individual was previously infected with SARS-CoV-2. These assays, which are sufficiently cheap to be widely deployed, have been used in some of the largest studies of SARS-CoV-2 spread and immunization 64 , 65 . Repeated rounds of serological sampling across large, representative populations in the UK conducted as part of the real-time assessment of community transmission (REACT) study provided ongoing estimates of viral exposure during successive pandemic waves 66 . The REACT investigators measured viral spread through subpopulations in the UK and their data were used to determine the need for regional lockdowns.
Iceland has proved ideal for the study of COVID-19 because more than half of its citizens have been tested for SARS-CoV-2 antibodies, and the clinical outcomes of individuals with a positive test result have been closely monitored 67 . However, despite extensive testing, almost half of infected people in Iceland were not detected, thereby illustrating the limitations of and gaps in even the most widespread population-scale testing schemes 64 . A similar seroprevalence study in Spain found that population-scale testing detected just 9% of infected people during the first pandemic wave, and almost one million infected people were estimated to have gone undetected 65 .
Strategies to scale up testing
Population-scale testing has relied heavily on RT–qPCR, which is performed in large, high-throughput, centralized laboratories with automated equipment by skilled personnel (Box 1 ). These centralized laboratories achieve sensitive and reliable results owing to validated and regulated oversight of testing procedures. However, samples need to be transported to the testing site, which can often extend the testing turnaround time to several days.
The pooling of several samples enables multiple individuals to be simultaneously tested. Chinese authorities tested more than seven million people in the city of Qingdao in 3 days using a pooling approach, wherein ten samples were combined and tested with a single reaction 68 . If the pool returns a negative test result, then all constituent individual samples are also considered to have a negative result. Only if the pooled test returns a positive result are the constituent samples tested individually to ultimately identify the positive sample or samples. A further innovation divides the samples among overlapping pools, such that any single sample is present in a unique combination of pools 69 , 70 . If the unique combination of pools returns a positive result, the individual sample can be identified without requiring an additional round of retesting 71 .
Pooling of patient samples, viral transport media or extracted RNA enables more individuals to be tested with use of fewer reagents. However, the advantages of pooling are limited in the context of a high test positivity rate. If positive samples are common, the majority of pools will return positive results, and most samples still need to be tested individually. In practice, this shortcoming can be mitigated by non-random pooling of samples from shared households or groups, which increases the likelihood that positive samples will be clustered within a small number of pools 72 . Nevertheless, the additional rounds of testing mandated by pooling can incur reporting delays, and the dilution of positive samples within large pools can reduce test sensitivity 73 .
Point-of-care (POC) tests can be performed on-site, such as within a local clinic, workplace or even a patient’s home. These tests are typically antigen-based lateral flow assays that can be easily shipped to the site and are sufficiently simple to be performed and interpreted without specialized training or equipment (Box 1 ). These features enable POC tests to be easily and widely distributed to the population without incurring the challenges and delays associated with sample collection and reporting 74 . Decentralization can improve access to testing, enable earlier and more frequent testing, and reduce exposure of health-care workers. Given these advantages, POC tests are widely considered an attractive approach to achieve a massive expansion in testing 75 , and a diverse range of POC tests have been developed that incorporate molecular, antigen-based and serological technologies 76 .
In November 2020, an initial pilot scheme aimed to screen up to half a million people in Liverpool, UK, using rapid, on-site antigen tests 74 . This scheme offered routine and repeated testing to all residents, regardless of symptoms, in a broad effort to achieve population-scale test coverage and reduce viral transmission. This community-led testing scheme identified more than one-third of the infected but mildly symptomatic or asymptomatic individuals in Liverpool 77 . However, despite this achievement, the sensitivity of the antigen tests used in this scheme was markedly lower than that reported in the original test validation studies, and the tests were thought to miss almost one-third of infectious individuals 78 .
Monitoring of emergent strains
The mutations that accumulate in the SARS-CoV-2 genome can alter the viral phenotype and confer a selective advantage that gives rise to new strains. Genomic epidemiology showed that one strain, distinguished by a non-synonymous D614G mutation in the spike protein, first emerged in Europe before expanding to become the predominant strain worldwide 79 owing to a selective fitness advantage conferred by the mutation that increased viral transmissibility 80 .
The integration of genome sequencing within population-scale testing can enable monitoring of the viral strains circulating within a population. Numerous countries have mandated that a proportion of positive samples is subjected to whole-genome sequencing, thereby providing ongoing surveillance of emerging and circulating variants. This sequencing information can identify emergent SARS-CoV-2 variants with differing transmission or pathogenicity, with resistance to antiviral treatment or that are at risk of vaccine escape 81 . In late December 2020, a new SARS-CoV-2 strain known as B.1.1.7 rapidly increased in prevalence throughout the UK, apparently outcompeting existing variants and prompting the rapid imposition of restrictions on travel to other countries 82 . Additional variants that might increase transmissibility and pathogenicity or reduce the efficacy of vaccines have similarly arisen in South Africa (B.1.351) 83 and Brazil (P.1) 84 . As the effect of these variants on the viral phenotype has become apparent, authorities have recognized that global testing will be increasingly needed to monitor the emergence and circulation of new variant SARS-CoV-2 strains.
Variant diversification identified by genomic surveillance is also important to assess the influence of new mutations on the ongoing performance of molecular diagnostic tests 85 , 86 . The emergent B.1.1.7 strain harbours a large number of mutations that might prevent the binding of some primers to the spike gene and thereby reduce the sensitivity of RT–qPCR tests 87 (Box 1 ). In response, numerous variant-specific primers have been developed, illustrating that strain diversification will require ongoing updates and validation of testing reagents.
Environmental testing
In the early stages of the COVID-19 pandemic, the routes of SARS-CoV-2 transmission were unclear, and viral particles that land on objects and surfaces ( fomites ) were considered to pose a risk of indirect transmission 88 . Testing of hospital and public surfaces close to infected patients detected the presence of viral RNA, and countries such as China implemented testing of frozen food imports 89 , 90 . However, ongoing environmental testing and epidemiological studies suggest that this mode of transmission is uncommon, and that viral transmission is instead primarily caused by contaminated respiratory droplets and aerosols 91 .
Nevertheless, although viral transmission via fomites is rare, the detection of SARS-CoV-2 in environmental samples can be used to infer its circulation within a community. SARS-CoV-2 can infect the gastrointestinal tract and can be shed at high concentrations in stool, and might be subsequently discharged into wastewater 92 . SARS-CoV-2 RNA can be sensitively detected in untreated municipal wastewater, including settled solids and sludge 93 , 94 , 95 . Accordingly, many municipalities have started to test sewage for the presence of SARS-CoV-2, similarly to the testing of primary sewage for poliovirus, which has been successfully used to monitor communities for decades 96 .
Retrospective studies show that increases in the concentration of SARS-CoV-2 RNA in raw wastewater are correlated with increases in reported COVID-19 cases 83 , 97 . For example, the detection of SARS-CoV-2 in wastewater from New Haven, Connecticut, USA, tracked rates of hospital admissions during early stages of the COVID-19 pandemic 98 . The detection of SARS-CoV-2 RNA in wastewater occurred days before the rise in the numbers of clinical COVID-19 cases at local hospitals and provided a valuable predictor of viral presence 98 .
Environmental testing has the advantage of detecting SARS-CoV-2 across a wide catchment area that encompasses many individuals. This cost-effective approach can provide community data even in the absence of large-scale testing and is unbiased with regard to people who lack access to health care. Environmental testing can also be used to closely monitor closed residential settings, such as colleges, universities, nursing homes and prisons 99 . Despite its potential, wastewater testing faces technical challenges in achieving sufficient sensitivity, in part because variables such as sampling location, type and volume can all affect detection. Rigorous validation and longitudinal monitoring is required for reliable, actionable wastewater testing 100 .
Ensuring the integrity of testing
The speed and scale of the COVID-19 pandemic has created unprecedented challenges to ensure the accuracy and reliability of testing. The implementation of population-scale screening is a massive undertaking that requires coordinated input from governmental, commercial and academic organizations. Numerous large programmes, such as the RADx initiative and the X-Prize Challenge, have been established to accelerate population-scale testing 101 , 102 .
The unique requirements of the pandemic have also called for modified regulatory regimes that encourage the development of rapid, cheap tests that are well suited to population-scale testing 26 . To expedite access to SARS-CoV-2 testing during the pandemic, many regulatory agencies have responded with expedited authorization of testing devices. For example, the FDA issues Emergency Use Authorizations (EUAs) if devices have a reasonable expectation of being effective (and various other criteria are met) 103 . Although EUAs provide welcome flexibility for commercial and laboratory developers, this approach also increases the risk that these tests could perform unexpectedly when deployed at scale in the field. For example, several poorly performing antigen tests were initially issued EUAs that were ultimately revoked, which required the FDA to independently evaluate these poor-quality tests and subsequently update its performance requirements and advice 104 , 105 .
Reference standards can improve quality and standardization across large and diverse testing schemes 106 . The rapid manufacture of synthetic SARS-CoV-2-positive controls has enabled molecular assays to be rapidly evaluated even in the context of limited access to patient samples. These positive controls also enable the early detection of defective reagents, such as faulty primers in RT–qPCR tests 107 , 108 . Inactivated viral fractions and serum samples have also been used to develop and evaluate the performance of serological tests 109 . Indeed, the proliferation of positive reference controls has been so widespread as to fuel concerns about contamination of testing and environmental surveillance schemes 110 , 111 .
The FDA has issued SARS-CoV-2 reference sample panels for the standardized evaluation of diagnostic assays 109 , and numerous other organizations, such as FIND, have launched standardized evaluation programmes 112 . Notably, findings from these standardized evaluations often differ markedly from test performance statistics published in the EUA 101 , 113 . The disparity might, in part, be due to the initial validation of test performance in hospitalized patients, who typically have high viral loads and undergo sampling in a well-controlled setting. This approach can render unreliable the estimation of test performance during population-scale screening, where many tested individuals might be presymptomatic or asymptomatic and have low viral or antibody loads 114 . This situation highlights that test validation is an ongoing process and that proficiency testing schemes are required to continually evaluate the performance of individual laboratories 115 .
Surveillance testing
SARS-CoV-2 will probably continue to circulate within human populations for many years, with ongoing regional or seasonal outbreaks 29 . Surveillance testing aims to identify these outbreaks early, often by the detection of a symptomatic index case, and to quickly contain their spread through contact tracing and isolation. Genomic epidemiology can distinguish between novel introductions and persistent local circulation, and can trace the source of outbreaks 116 , whereas environmental testing provides ongoing monitoring of large catchment areas.
Routine testing could also become more common in various aspects of life, with testing of closed communities and populations (such as prisons, schools and universities) increasingly common 115 , 117 , 118 . Given the role of cross-border transport in the rapid spread of SARS-CoV-2, testing might become a standard feature of international travel, with testing of incoming travellers mandatory in many countries that aim to limit reintroduction of SARS-CoV-2 or new variant strains 119 , 120 . Global surveillance of circulating SARS-CoV-2 variants using genome sequencing will also become increasingly important to match circulating variants with vaccines, and to monitor vaccine escape and the emergence of antiviral resistance 7 .
Clinical diagnostic testing for SARS-CoV-2 (alongside other respiratory pathogens, such as influenza viruses) is expected to become part of the standard evaluation of patients admitted to hospital with associated symptoms. Currently, the presence of SARS-CoV-2 is sufficient to direct clinical treatment; however, genome sequencing might be required to distinguish between different variant strains if they necessitate differing clinical or containment responses. Although the considerable innovation and investment in testing is likely to also benefit the detection of other infectious diseases and endemic diseases such as cancer, the disruption to national screening programmes as a result of the pandemic is anticipated to increase the case burden of these diseases for many years to come 121 .
Domestic and wild animal populations can also be infected with SARS-CoV-2 and could act as reservoirs for the virus. Spillover viral transmission between human and animal populations can result in changes in selection pressure that accelerate viral adaptation and evolution. Testing has found repeated, independent infections of domestic mink populations in the Netherlands and Denmark, where SARS-CoV-2 accumulated additional mutations before being reintroduced into human populations 122 , 123 , 124 . This observation has prompted culling of domestic mink populations as a result of fears that they could act as reservoirs for new SARS-CoV-2 strains. In addition, pressure is growing for increased testing of both wild and domestic animal populations to monitor viral prevalence and diversity.
Population-scale testing has assumed a central role in our efforts to combat the COVID-19 pandemic and will be similarly central to our preparation for future pandemics. Notably, countries such as South Korea and Taiwan that had been affected by SARS outbreaks were able to quickly scale up existing contact tracing and testing schemes, which proved key in containing COVID-19 outbreaks 45 , 125 . Many other countries are likely to establish similar national preparation plans that maintain their investment in testing infrastructure and supplies of reagents. The establishment of international genomic surveillance systems to monitor the emergence of novel SARS-CoV-2 strains and the zoonotic transmission of new viruses will enable prompt and appropriate responses, including the development and manufacture of diagnostic tests, and the rapid imposition of travel restrictions and testing requirements 7 . Although some of these preparations are specific to coronaviruses, which have been the source of multiple pandemics in the past two decades 126 , the lessons learned are also applicable to response planning for other viruses with different transmission dynamics, such as influenza viruses and Ebola virus 9 .
Conclusions
Population-scale testing has emerged as a major intervention strategy to manage the COVID-19 pandemic. As community transmission became entrenched within countries, the utility of population-scale testing has been intensely debated. Although widespread testing has been proposed as a solution to suppress and even stop the pandemic, the limitations of and gaps in population-scale testing have also become apparent, and both the cost and the effectiveness of mass testing on reducing viral transmission have been intensely debated.
In the early stages of the pandemic, the main limitations on widespread testing were technical, including insufficient reagents and an unprepared laboratory testing infrastructure. These practical obstacles to the implementation of testing on a massive scale favoured the use of established technologies, which explains why the vast majority of testing for SARS-CoV-2 has been performed by conventional RT–qPCR tests in centralized laboratories. These RT–qPCR tests were developed almost immediately after publication of the reference genome, were quickly scaled up through an existing infrastructure and have generally proved both accurate and reliable. However, with the pandemic ongoing, a new generation of rapid and convenient POC tests have been developed.
With sufficiently broad and frequent community testing, identification and isolation of most infectious individuals was considered possible, thereby arresting the pandemic 127 . However, even the most extensive testing schemes have been found to miss many infectious individuals and to provide only partial population coverage 64 , 65 . The rapid infectious course of SARS-CoV-2 and the possibility of its transmission by presymptomatic or asymptomatic individuals blunts the impact of population-scale testing, as many infectious individuals escape detection 128 . In France, for example, models based on health records suggest that most infected people were not tested or quarantined following the first pandemic wave in early 2020, despite the implementation of a nationwide surveillance programme 129 .
The benefits of widespread testing have been widely debated, with researchers arguing that screening of large populations with low infection rates is expensive and ineffective, as most positive results are false positives 130 . Furthermore, the effectiveness of population-scale testing becomes increasingly marginal as viral prevalence falls, and testing alone seems insufficient to eliminate viral transmission. Therefore, researchers have argued that testing should be reserved for symptomatic individuals, traced contacts, closed populations or high-risk groups with an increased probability of infection, where this approach has proven effective, and used in coordination with other interventions, such as targeted regional lockdowns or travel restrictions 131 , 132 , 133 .
Human populations have experienced many pandemics throughout history; however, this has been the first pandemic in which widespread and routine testing has been available. We have been able to monitor viral transmission and measure viral spread in successive pandemic waves of SARS-CoV-2 infection throughout human populations worldwide. The COVID-19 pandemic has already required unprecedented testing of millions of people, and this information has been critical to understanding viral transmission, informing effective responses and planning for future pandemics. Although population-scale testing has not provided a simple or single technical solution to the pandemic, it has served as an invaluable guide to our response.
Zhou, P. et al. A pneumonia outbreak associated with a new coronavirus of probable bat origin. Nature 579 , 270–273 (2020).
CAS PubMed PubMed Central Google Scholar
Wu, F. et al. A new coronavirus associated with human respiratory disease in China. Nature 579 , 265–269 (2020).
Sheridan, C. Coronavirus and the race to distribute reliable diagnostics. Nat. Biotechnol. 38 , 382–384 (2020).
CAS PubMed Google Scholar
World Health Organization. Molecular assays to diagnose COVID-19: summary table of available protocols. WHO https://www.who.int/publications/m/item/molecular-assays-to-diagnose-covid-19-summary-table-of-available-protocols (2020).
Hasell, J. et al. A cross-country database of COVID-19 testing. Sci. Data 7 , 345 (2020).
PubMed PubMed Central Google Scholar
Wang, C. J., Ng, C. Y. & Brook, R. H. Response to COVID-19 in Taiwan: big data analytics, new technology, and proactive testing. JAMA 323 , 1341–1342 (2020).
The COVID-19 testing debacle. Nat. Biotechnol . 38 , 653 (2020).
Gardy, J. L. & Loman, N. J. Towards a genomics-informed, real-time, global pathogen surveillance system. Nat. Rev. Genet. 19 , 9–20 (2018).
Gire, S. K. et al. Genomic surveillance elucidates Ebola virus origin and transmission during the 2014 outbreak. Science 345 , 1369–1372 (2014).
Faria, N. R. et al. Establishment and cryptic transmission of Zika virus in Brazil and the Americas. Nature 546 , 406–410 (2017).
Worobey, M. et al. The emergence of SARS-CoV-2 in Europe and North America. Science 370 , 564–570 (2020). This work uses genomic epidemiology to describe the initial international spread of SARS-CoV-2 variants .
Elbe, S. & Buckland-Merrett, G. Data, disease and diplomacy: GISAID’s innovative contribution to global health. Glob. Chall. 1 , 33–46 (2017).
Hadfield, J. et al. Nextstrain: real-time tracking of pathogen evolution. Bioinformatics 34 , 4121–4123 (2018).
Forster, P., Forster, L., Renfrew, C. & Forster, M. Phylogenetic network analysis of SARS-CoV-2 genomes. Proc. Natl Acad. Sci. USA 117 , 9241–9243 (2020).
Rockett, R. J. et al. Revealing COVID-19 transmission in Australia by SARS-CoV-2 genome sequencing and agent-based modeling. Nat. Med. 26 , 1398–1404 (2020).
Miller, D. et al. Full genome viral sequences inform patterns of SARS-CoV-2 spread into and within Israel. Nat. Commun. 11 , 5518 (2020).
Lu, J. et al. Genomic epidemiology of SARS-CoV-2 in Guangdong province, China. Cell 181 , 997–1003.e9 (2020).
Fauver, J. R. et al. Coast-to-coast spread of SARS-CoV-2 during the early epidemic in the United States. Cell 181 , 990–996.e5 (2020).
Seemann, T. et al. Tracking the COVID-19 pandemic in Australia using genomics. Nat. Commun. 11 , 4376 (2020).
Deng, X. et al. Genomic surveillance reveals multiple introductions of SARS-CoV-2 into northern California. Science 369 , 582–587 (2020).
Althouse, B. M. et al. Superspreading events in the transmission dynamics of SARS-CoV-2: opportunities for interventions and control. PLoS Biol. 18 , e3000897 (2020).
Lemieux, J. E. et al. Phylogenetic analysis of SARS-CoV-2 in Boston highlights the impact of superspreading events. Science 371 , eabe3261 (2020).
PubMed Google Scholar
Oude Munnink, B. B. et al. Rapid SARS-CoV-2 whole-genome sequencing and analysis for informed public health decision-making in the Netherlands. Nat. Med. 26 , 1405–1410 (2020).
Villabona-Arenas, C. J., Hanage, W. P. & Tully, D. C. Phylogenetic interpretation during outbreaks requires caution. Nat. Microbiol. 5 , 876–877 (2020).
Bedford, T. et al. Cryptic transmission of SARS-CoV-2 in Washington State. Science 370 , 571–575 (2020).
Wölfel, R. et al. Virological assessment of hospitalized patients with COVID-2019. Nature 581 , 465–469 (2020).
Article PubMed Google Scholar
Mina, M. J., Parker, R. & Larremore, D. B. Rethinking COVID-19 test sensitivity — a strategy for containment. N. Engl. J. Med. 383 , e120 (2020).
Li, Q. et al. Early transmission dynamics in Wuhan, China, of novel coronavirus–infected pneumonia. N. Engl. J. Med. 382 , 1199–1207 (2020). This report determines the initial SARS-CoV-2 transmission dynamics, including infectious and incubation periods and basic reproductive number .
Cevik, M. et al. SARS-CoV-2, SARS-CoV, and MERS-CoV viral load dynamics, duration of viral shedding, and infectiousness: a systematic review and meta-analysis. Lancet Microbe 2 , e13–e22 (2021).
Kissler, S. M., Tedijanto, C., Goldstein, E., Grad, Y. H. & Lipsitch, M. Projecting the transmission dynamics of SARS-CoV-2 through the postpandemic period. Science 368 , 860–868 (2020).
Pan, Y., Zhang, D., Yang, P., Poon, L. L. M. & Wang, Q. Viral load of SARS-CoV-2 in clinical samples. Lancet Infect. Dis. 20 , 411–412 (2020).
Wang, W. et al. Detection of SARS-CoV-2 in different types of clinical specimens. JAMA 323 , 1843–1844 (2020).
Vogels, C. B. F. et al. SalivaDirect: a simplified and flexible platform to enhance SARS-CoV-2 testing capacity. Med 2 , 263–280.e6 (2020).
van Kampen, J. J. A. et al. Duration and key determinants of infectious virus shedding in hospitalized patients with coronavirus disease-2019 (COVID-19). Nat. Commun. 12 , 1–6 (2021).
Google Scholar
Stites, E. C. & Wilen, C. B. The interpretation of SARS-CoV-2 diagnostic tests. Med 1 , 78–89 (2020).
Sethuraman, N., Jeremiah, S. S. & Ryo, A. Interpreting diagnostic tests for SARS-CoV-2. JAMA 323 , 2249–2251 (2020).
McCartney, M., Sullivan, F. & Heneghan, C. Information and rational decision-making: explanations to patients and citizens about personal risk of COVID-19. BMJ Evid. Based Med. https://doi.org/10.1136/bmjebm-2020-111541 (2020).
Article PubMed PubMed Central Google Scholar
Rodda, L. B. et al. Functional SARS-CoV-2-specific immune memory persists after mild COVID-19. Cell 184 , 169–183.e17 (2020).
Tay, M. Z., Poh, C. M., Rénia, L., MacAry, P. A. & Ng, L. F. P. The trinity of COVID-19: immunity, inflammation and intervention. Nat. Rev. Immunol. 20 , 363–374 (2020).
Wajnberg, A. et al. Humoral response and PCR positivity in patients with COVID-19 in the New York city region, USA: an observational study. Lancet Microbe 1 , e283–e289 (2020).
Long, Q. X. et al. Antibody responses to SARS-CoV-2 in patients with COVID-19. Nat. Med. 26 , 845–848 (2020).
Ng, D. L. et al. SARS-CoV-2 seroprevalence and neutralizing activity in donor and patient blood. Nat. Commun. 11 , 4698 (2020).
Amanat, F. et al. A serological assay to detect SARS-CoV-2 seroconversion in humans. Nat. Med. 26 , 1033–1036 (2020).
Kraemer, M. U. G. et al. The effect of human mobility and control measures on the COVID-19 epidemic in China. Science 368 , 493–497 (2020).
Wu, W. K., Liou, J. M., Hsu, C. C., Lin, Y. H. & Wu, M. S. Pandemic preparedness in Taiwan. Nat. Biotechnol. 38 , 932–933 (2020).
Kang, C. R. et al. Coronavirus disease exposure and spread from nightclubs, South Korea. Emerg. Infect. Dis. 26 , 2499–2501 (2020).
Kretzschmar, M. E. et al. Impact of delays on effectiveness of contact tracing strategies for COVID-19: a modelling study. Lancet Public Health 5 , e452–e459 (2020).
Kucharski, A. J. et al. Effectiveness of isolation, testing, contact tracing, and physical distancing on reducing transmission of SARS-CoV-2 in different settings: a mathematical modelling study. Lancet Infect. Dis. 20 , 1151–1160 (2020).
Hellewell, J. et al. Feasibility of controlling COVID-19 outbreaks by isolation of cases and contacts. Lancet Glob. Health 8 , e488–e496 (2020).
Ferretti, L. et al. Quantifying SARS-CoV-2 transmission suggests epidemic control with digital contact tracing. Science 368 , eabb6936 (2020).
The app credibility gap. Nat. Biotechnol. 38 , 768 (2020).
Long, Q. X. et al. Clinical and immunological assessment of asymptomatic SARS-CoV-2 infections. Nat. Med. 26 , 1200–1204 (2020).
Li, R. et al. Substantial undocumented infection facilitates the rapid dissemination of novel coronavirus (SARS-CoV-2). Science 368 , 489–493 (2020).
Holt, E. Slovakia to test all adults for SARS-CoV-2. Lancet 396 , 1386–1387 (2020).
Pavelka, M. et al. The impact of population-wide rapid antigen testing on SARS-CoV-2 prevalence in Slovakia. Science https://doi.org/10.1126/science.abf9648 (2021).
Frnda, J. & Durica, M. On pilot massive COVID-19 testing by antigen tests in Europe. case study: Slovakia. Infect. Dis. Rep. 13 , 45–57 (2021).
Vandenberg, O., Martiny, D., Rochas, O., van Belkum, A. & Kozlakidis, Z. Considerations for diagnostic COVID-19 tests. Nat. Rev. Microbiol. 19 , 171–183 (2020).
Larremore, D. B. et al. Test sensitivity is secondary to frequency and turnaround time for COVID-19 surveillance. Sci. Adv. 7 , eabd5393 (2021).
Russell, T. W. et al. Reconstructing the early global dynamics of under-ascertained COVID-19 cases and infections. BMC Med. 18 , 332 (2020).
Guan, D. et al. Global supply-chain effects of COVID-19 control measures. Nat. Hum. Behav. 4 , 577–587 (2020).
Songok, E. A locally sustainable approach to COVID-19 testing in Africa. Lancet Microbe 1 , e197 (2020).
Lavezzo, E. et al. Suppression of a SARS-CoV-2 outbreak in the Italian municipality of Vo’. Nature 584 , 425–429 (2020). Large-scale testing enabled the measurement of SARS-CoV-2 transmission within an entire town and allowed the impact of interventionists’ lockdown measures on the reproductive number to be determined .
Vogel, G. Antibody surveys suggesting vast undercount of coronavirus infections may be unreliable. Science https://doi.org/10.1126/science.abc3831 (2020).
Gudbjartsson, D. F. et al. Humoral immune response to SARS-CoV-2 in Iceland. N. Engl. J. Med. 383 , 1724–1734 (2020). This population-scale serological study measured the exposure of the Icelandic population to SARS-CoV-2 during the first pandemic wave and assessed the effectiveness of large-scale testing .
Pollán, M. et al. Prevalence of SARS-CoV-2 in Spain (ENE-COVID): a nationwide, population-based seroepidemiological study. Lancet 396 , 535–544 (2020).
Ward, H. et al. Antibody prevalence for SARS-CoV-2 in England following the first peak of the pandemic. Nat. Commun. 12 , 905 (2021).
Gudbjartsson, D. F. et al. Spread of SARS-CoV-2 in the Icelandic population. N. Engl. J. Med. 382 , 2302–2315 (2020).
Xing, Y., Wong, G. W. K., Ni, W., Hu, X. & Xing, Q. Rapid response to an outbreak in Qingdao, China. N. Engl. J. Med. 383 , e129 (2020).
Ghosh, S. et al. Tapestry: a single-round smart pooling technique for COVID-19 testing. Preprint at medRxiv https://doi.org/10.1101/2020.04.23.20077727 (2020).
Mutesa, L. et al. A pooled testing strategy for identifying SARS-CoV-2 at low prevalence. Nature 589 , 276–280 (2020).
Shental, N. et al. Efficient high-throughput SARS-CoV-2 testing to detect asymptomatic carriers. Sci. Adv. 6 , 5961–5972 (2020).
Barak, N. et al. Lessons from applied large-scale pooling of 133,816 SARS-CoV-2 RT-PCR tests. Sci. Transl Med. https://doi.org/10.1126/scitranslmed.abf2823 (2021).
Bateman, A. C., Mueller, S., Guenther, K. & Shult, P. Assessing the dilution effect of specimen pooling on the sensitivity of SARS-CoV-2 PCR tests. J. Med. Virol. 93 , 1568–1572 (2020).
Iacobucci, G. Covid-19: Mass population testing is rolled out in Liverpool. BMJ 371 , m4268 (2020).
Peeling, R. W., Olliaro, P. L., Boeras, D. I. & Fongwen, N. Scaling up COVID-19 rapid antigen tests: promises and challenges. Lancet Infect. Dis. https://doi.org/10.1016/S1473-3099(21)00048-7 (2021).
Kevadiya, B. D. et al. Diagnostics for SARS-CoV-2 infections. Nat. Mater. https://doi.org/10.1038/s41563-020-00906-z (2021).
GOV.UK. Research and analysis. Liverpool COVID-19 community testing pilot: interim evaluation report summary. gov.uk https://www.gov.uk/government/publications/liverpool-covid-19-community-testing-pilot-interim-evaluation-report-summary/liverpool-covid-19-community-testing-pilot-interim-evaluation-report-summary (2021).
Wise, J. Covid-19: Lateral flow tests miss over half of cases, Liverpool pilot data show. BMJ 371 , m4848 (2020).
Korber, B. et al. Tracking changes in SARS-CoV-2 Spike: evidence that D614G increases infectivity of the COVID-19 virus. Cell 182 , 812–827.e19 (2020).
Plante, J. A. et al. Spike mutation D614G alters SARS-CoV-2 fitness. Nature https://doi.org/10.1038/s41586-020-2895-3 (2020).
Thomson, E. C. et al. Circulating SARS-CoV-2 spike N439K variants maintain fitness while evading antibody-mediated immunity. Cell 184 , 1171–1187.e20 (2021).
Davies, N. G. et al. Estimated transmissibility and impact of SARS-CoV-2 lineage B.1.1.7 in England. Science 372 , eabg3055 (2021). This study describes the emergence of SARS-CoV-2 variants with higher estimated reproductive numbers than other variants .
Tegally, H. et al. Emergence and rapid spread of a new severe acute respiratory syndrome-related coronavirus 2 (SARS-CoV-2) lineage with multiple spike mutations in South Africa. Nature https://doi.org/10.1038/s41586-021-03402-9 (2021).
Voloch, C. M. et al. Genomic characterization of a novel SARS-CoV-2 lineage from Rio de Janeiro, Brazil. J. Virol. https://doi.org/10.1128/JVI.00119-21 (2021).
Artesi, M. et al. A recurrent mutation at position 26340 of SARS-CoV-2 is associated with failure of the E Gene quantitative reverse transcription-PCR utilized in a commercial dual-target diagnostic assay. J. Clin. Microbiol. 58 , e01598–20 (2020).
Ziegler, K. et al. SARS-CoV-2 samples may escape detection because of a single point mutation in the N gene. Eur. Surveill. 25 , 2001650 (2020).
Rambaut, A. et al. Preliminary genomic characterisation of an emergent SARS-CoV-2 lineage in the UK defined by a novel set of spike mutations. Virological https://virological.org/t/preliminary-genomic-characterisation-of-an-emergent-sars-cov-2-lineage-in-the-uk-defined-by-a-novel-set-of-spike-mutations/563 (2020).
van Doremalen, N. et al. Aerosol and surface stability of SARS-CoV-2 as compared with SARS-CoV-1. N. Engl. J. Med. 382 , 1564–1567 (2020).
Piana, A. et al. Monitoring COVID-19 transmission risks by quantitative real-time PCR tracing of droplets in hospital and living environments. mSphere 6 , e01070-20 (2021).
Pang, X. et al. Cold-chain food contamination as the possible origin of COVID-19 resurgence in Beijing. Natl Sci. Rev. https://doi.org/10.1093/nsr/nwaa264 (2020).
Article PubMed Central PubMed Google Scholar
Zhang, R., Li, Y., Zhang, A. L., Wang, Y. & Molina, M. J. Identifying airborne transmission as the dominant route for the spread of COVID-19. Proc. Natl Acad. Sci. USA 117 , 14857–14863 (2020).
Wu, Y. et al. Prolonged presence of SARS-CoV-2 viral RNA in faecal samples. Lancet Gastroenterol. Hepatol. 5 , 434–435 (2020).
Lodder, W. & de Roda Husman, A. M. SARS-CoV-2 in wastewater: potential health risk, but also data source. Lancet Gastroenterol. Hepatol. 5 , 533–534 (2020).
Graham, K. E. et al. SARS-CoV-2 RNA in wastewater settled solids is associated with COVID-19 cases in a large urban sewershed. Environ. Sci. Technol. 55 , 488–498 (2020).
Ahmed, W. et al. First confirmed detection of SARS-CoV-2 in untreated wastewater in Australia: a proof of concept for the wastewater surveillance of COVID-19 in the community. Sci. Total Environ. 728 , 138764 (2020).
Berchenko, Y. et al. Estimation of polio infection prevalence from environmental surveillance data. Sci. Transl Med. 9 , eaaf6786 (2017).
Medema, G., Heijnen, L., Elsinga, G., Italiaander, R. & Brouwer, A. Presence of SARS-coronavirus-2 RNA in sewage and correlation with reported COVID-19 prevalence in the early stage of the epidemic in the Netherlands. Environ. Sci. Technol. Lett. 7 , 511–516 (2020).
CAS Google Scholar
Peccia, J. et al. Measurement of SARS-CoV-2 RNA in wastewater tracks community infection dynamics. Nat. Biotechnol. 38 , 1164–1167 (2020). This group shows that detection of the presence of SARS-CoV-2 RNA in wastewater correlated with hospital COVID-19 admissions, thereby demonstrating that this approach can be used to monitor the presence of SARS-CoV-2 within a community catchment area .
Betancourt, W. Q. et al. COVID-19 containment on a college campus via wastewater-based epidemiology, targeted clinical testing and an intervention. Sci. Total Environ. 779 , 146408 (2021).
O’Reilly, K. M., Allen, D. J., Fine, P. & Asghar, H. The challenges of informative wastewater sampling for SARS-CoV-2 must be met: lessons from polio eradication. Lancet Microbe 1 , E189–E190 (2020).
MacKay, M. J. et al. The COVID-19 XPRIZE and the need for scalable, fast, and widespread testing. Nat. Biotechnol. 38 , 1021–1024 (2020).
Tromberg, B. J. et al. Rapid scaling up of COVID-19 diagnostic testing in the US — the NIH RADx initiative. N. Engl. J. Med. 383 , 1071–1077 (2020).
Shuren, J. & Stenzel, T. COVID-19 molecular diagnostic testing — lessons learned. N. Engl. J. Med. 383 , e97 (2020).
Kalokairinou, L., Zettler, P. J., Nagappan, A., Kyweluk, M. A. & Wexler, A. The promise of direct-to-consumer COVID-19 testing: ethical and regulatory issues. J. Law Biosci. 7 , lsaa069 (2020).
Abbasi, J. The promise and peril of antibody testing for COVID-19. JAMA 323 , 1881–1883 (2020).
Page, M., Almond, N., Rose, N. J. & Schneider, C. K. Diagnostics and the coronavirus: don’t let the standards slip. Nat. Biotechnol. 38 , 673–674 (2020).
Nalla, A. K. et al. Comparative performance of SARS-CoV-2 detection assays using seven different primer-probe sets and one assay kit. J. Clin. Microbiol. 58 , e00557-20 (2020).
Vogels, C. B. F. et al. Analytical sensitivity and efficiency comparisons of SARS-CoV-2 RT–qPCR primer–probe sets. Nat. Microbiol. 5 , 1299–1305 (2020).
US Food & Drug Administration. SARS-CoV-2 reference panel comparative data. FDA https://www.fda.gov/medical-devices/coronavirus-covid-19-and-medical-devices/sars-cov-2-reference-panel-comparative-data (2020).
Wang, C. Y. T., Buckley, C., Bletchly, C., Harris, P. & Whiley, D. Contamination of SARS-CoV-2 RT-PCR probes at the oligonucleotide manufacturer. Pathology 52 , 814–816 (2020).
Mögling, R. et al. Delayed laboratory response to COVID-19 caused by molecular diagnostic contamination. Emerg. Infect. Dis. 26 , 1944–1946 (2020).
FIND. SARS-CoV-2 diagnostic pipeline. FIND https://www.finddx.org/covid-19/pipeline/ (2021).
Fitzpatrick, M. C., Pandey, A., Wells, C. R., Sah, P. & Galvani, A. P. Buyer beware: inflated claims of sensitivity for rapid COVID-19 tests. Lancet 397 , 24–25 (2021).
Takahashi, S., Greenhouse, B. & Rodríguez-Barraquer, I. Are seroprevalence estimates for severe acute respiratory syndrome coronavirus 2 biased? J. Infect. Dis. 222 , 1772–1775 (2020).
Zeichhardt, H. & Kammel, M. INSTAND report on extra external quality assessment scheme group no. 340 virus genome detection SARS-CoV-2. INSTAND https://www.instand-ev.de/System/rv-files/340%20EN%20SARS-CoV-2%20Genome%20EQAS%20April%202020%2020200502j.pdf (2020).
Meredith, L. W. et al. Rapid implementation of SARS-CoV-2 sequencing to investigate cases of health-care associated COVID-19: a prospective genomic surveillance study. Lancet Infect. Dis. 20 , 1263–1272 (2020).
Rafiei, Y. & Mello, M. M. The missing piece — SARS-CoV-2 testing and school reopening. N. Engl. J. Med. 383 , e126 (2020).
Paltiel, A. D., Zheng, A. & Walensky, R. P. Assessment of SARS-CoV-2 screening strategies to permit the safe reopening of college campuses in the US. JAMA Netw. Open 3 , e2016818 (2020).
Chinazzi, M. et al. The effect of travel restrictions on the spread of the 2019 novel coronavirus (COVID-19) outbreak. Science 368 , 395–400 (2020).
Wells, C. R. et al. Impact of international travel and border control measures on the global spread of the novel 2019 coronavirus outbreak. Proc. Natl Acad. Sci. USA 117 , 7504–7509 (2020).
Maringe, C. et al. The impact of the COVID-19 pandemic on cancer deaths due to delays in diagnosis in England, UK: a national, population-based, modelling study. Lancet Oncol. 21 , 1023–1034 (2020).
Oude Munnink, B. B. et al. Transmission of SARS-CoV-2 on mink farms between humans and mink and back to humans. Science 371 , 172–177 (2020). This group uses whole-genome sequencing to show SARS-CoV-2 transmission between minks and humans .
Oreshkova, N. et al. SARS-CoV-2 infection in farmed minks, the Netherlands, April and May 2020. Euro Surveill. 25 , 2001005 (2020).
PubMed Central Google Scholar
van Dorp, L. et al. No evidence for increased transmissibility from recurrent mutations in SARS-CoV-2. Nat. Commun. 11 , 5986 (2020).
Dighe, A. et al. Response to COVID-19 in South Korea and implications for lifting stringent interventions. BMC Med. 18 , 321 (2020).
Cui, J., Li, F. & Shi, Z. L. Origin and evolution of pathogenic coronaviruses. Nat. Rev. Microbiol. 17 , 181–192 (2019).
Taipale, J., Romer, P. & Linnarsson, S. Population-scale testing can suppress the spread of COVID-19. Preprint at medRxiv https://doi.org/10.1101/2020.04.27.20078329 (2020).
Article Google Scholar
Gandhi, M., Yokoe, D. S. & Havlir, D. V. Asymptomatic transmission, the Achilles’ heel of current strategies to control COVID-19. N. Engl. J. Med. 382 , 2158–2160 (2020).
Pullano, G. et al. Underdetection of COVID-19 cases in France threatens epidemic control. Nature 590 , 134–139 (2020).
Raffle, A. E., Pollock, A. M. & Harding-Edgar, L. COVID-19 mass testing programmes. BMJ 370 , m3262 (2020).
Pollock, A. M. & Lancaster, J. Asymptomatic transmission of COVID-19. BMJ 371 , m4851 (2020).
Skittrall, J. P. et al. Diagnostic tool or screening programme? Asymptomatic testing for SARS-CoV-2 needs clear goals and protocols. Lancet Region. HealthEur. 1 , 100002 (2021).
Flaxman, S. et al. Estimating the effects of non-pharmaceutical interventions on COVID-19 in Europe. Nature 584 , 257–261 (2020).
Frasier, S. L. Coronavirus antibody tests have a mathematical pitfall. Sci. Am . 323 , 12–13 (2020).
Corman, V. M. et al. Detection of 2019 novel coronavirus (2019-nCoV) by real-time RT-PCR. Eur. Surveill. 25 , 2000045 (2020).
Liu, R. et al. Positive rate of RT-PCR detection of SARS-CoV-2 infection in 4880 cases from one hospital in Wuhan, China, from Jan to Feb 2020. Clin. Chim. Acta 505 , 172–175 (2020).
Wikramaratna, P., Paton, R., Ghafari, M. & Lourenco, J. Estimating false-negative detection rate of SARS-CoV-2 by RT-PCR. Euro Surveill. 25 , 2000568 (2020).
CAS PubMed Central Google Scholar
Schmid-Burgk, J. et al. LAMP-Seq: population-scale COVID-19 diagnostics using combinatorial barcoding. Preprint at bioRxiv https://doi.org/10.1101/2020.04.06.025635 (2020).
Yángüez, E. et al. HiDRA-seq: high-throughput SARS-CoV-2 detection by RNA barcoding and amplicon sequencing. Preprint at bioRxiv https://doi.org/10.1101/2020.06.02.130484 (2020).
Bloom, J. S. et al. Swab-Seq: a high-throughput platform for massively scaled up SARS-CoV-2 testing. Preprint at medRxiv https://doi.org/10.1101/2020.08.04.20167874 (2021).
Notomi, T. et al. Loop-mediated isothermal amplification of DNA. Nucleic Acids Res. 28 , e63 (2000).
Dao Thi, V. L. et al. A colorimetric RT-LAMP assay and LAMP-sequencing for detecting SARS-CoV-2 RNA in clinical samples. Sci. Transl Med. 12 , eabc7075 (2020).
Piepenburg, O., Williams, C. H., Stemple, D. L. & Armes, N. A. DNA detection using recombination proteins. PLoS Biol. 4 , 1115–1121 (2006).
El-Tholoth, M., Bau, H. H. & Song, J. A single and two-stage, closed-tube, molecular test for the 2019 novel coronavirus (COVID-19) at home, clinic, and points of entry. Preprint at https://doi.org/10.26434/chemrxiv.11860137.v1 (2020).
Broughton, J. P. et al. CRISPR–Cas12-based detection of SARS-CoV-2. Nat. Biotechnol. 38 , 870–874 (2020).
Lambert-Niclot, S. et al. Evaluation of a rapid diagnostic assay for detection of SARS-CoV-2 antigen in nasopharyngeal swabs. J. Clin. Microbiol. 58 , e00977-20 (2020).
Scohy, A. et al. Low performance of rapid antigen detection test as frontline testing for COVID-19 diagnosis. J. Clin. Virol. 129 , 104455 (2020).
Mak, G. C. et al. Evaluation of rapid antigen test for detection of SARS-CoV-2 virus. J. Clin. Virol. 129 , 104500 (2020).
Whitman, J. D. et al. Evaluation of SARS-CoV-2 serology assays reveals a range of test performance. Nat. Biotechnol. 38 , 1174–1183 (2020).
Petherick, A. Developing antibody tests for SARS-CoV-2. Lancet 395 , 1101–1102 (2020).
Tang, M. S. et al. Clinical performance of two SARS-CoV-2 serologic assays. Clin. Chem. 66 , 1055–1062 (2020).
Delamater, P. L., Street, E. J., Leslie, T. F., Yang, Y. T. & Jacobsen, K. H. Complexity of the basic reproduction number (R0). Emerg. Infect. Dis. 25 , 1–4 (2019).
Li, Y. et al. The temporal association of introducing and lifting non-pharmaceutical interventions with the time-varying reproduction number (R) of SARS-CoV-2: a modelling study across 131 countries. Lancet Infect. Dis. 21 , 193–202 (2020).
Zhang, J. et al. Changes in contact patterns shape the dynamics of the COVID-19 outbreak in China. Science 368 , 1481–1486 (2020).
Kucharski, A. J. et al. Early dynamics of transmission and control of COVID-19: a mathematical modelling study. Lancet Infect. Dis. 20 , 553–558 (2020).
World Health Organization. Considerations for implementing and adjusting public health and social measures in the context of COVID-19. WHO https://www.who.int/publications/i/item/considerations-in-adjusting-public-health-and-social-measures-in-the-context-of-covid-19-interim-guidance (2020).
Arnaout, R. et al. SARS-CoV2 testing: the limit of detection matters. Preprint at bioRxiv https://doi.org/10.1101/2020.06.02.131144 (2020).
Download references
Author information
Authors and affiliations.
Australian Institute for Bioengineering and Nanotechnology, University of Queensland, Brisbane, QLD, Australia
Tim R. Mercer
Garvan Institute of Medical Research, Sydney, NSW, Australia
St Vincent’s Clinical School, University of New South Wales, Sydney, NSW, Australia
Departments of Pathology and Bioengineering, Stanford University, Stanford, CA, USA
Joint Initiative for Metrology in Biology, SLAC National Accelerator Laboratory, Menlo Park, CA, USA
You can also search for this author in PubMed Google Scholar
Contributions
The authors contributed equally to all aspects of the article.
Corresponding author
Correspondence to Tim R. Mercer .
Ethics declarations
Competing interests.
The authors declare no competing interests.
Additional information
Peer review information.
Nature Reviews Genetics thanks Z. Kozlakidis, R. S. Vincenzo, J. Hay and the other, anonymous, reviewer(s) for their contribution to the peer review of this work.
Publisher’s note
Springer Nature remains neutral with regard to jurisdictional claims in published maps and institutional affiliations.
Related links
GISAID: https://www.gisaid.org/
Nextstrain: https://nextstrain.org/
Our World in Data COVID-19 dataset: https://ourworldindata.org/coronavirus-testing
The closure of non-essential businesses and activities, along with requiring individuals to remain within their homes, in an effort to reduce population mobility and contact and thereby reduce viral transmission.
The maintenance of a sufficient distance between individuals to prevent airborne transmission of a virus.
A single individual who infects many other individuals.
The fraction of individuals within a population who are affected. This value can indicate the a priori probability that a randomly selected individual from a given population is infected, and is a key determinant of the utility of a screening test.
Objects (such as clothes, utensils and furniture) that are likely to be contaminated with infectious material.
Rights and permissions
Reprints and permissions
About this article
Cite this article.
Mercer, T.R., Salit, M. Testing at scale during the COVID-19 pandemic. Nat Rev Genet 22 , 415–426 (2021). https://doi.org/10.1038/s41576-021-00360-w
Download citation
Accepted : 31 March 2021
Published : 04 May 2021
Issue Date : July 2021
DOI : https://doi.org/10.1038/s41576-021-00360-w
Share this article
Anyone you share the following link with will be able to read this content:
Sorry, a shareable link is not currently available for this article.
Provided by the Springer Nature SharedIt content-sharing initiative
This article is cited by
Implementing a pilot study of covid-19 self-testing in high-risk populations and remote locations: results and lessons learnt.
- Elena Marbán-Castro
- Vladimer Getia
- Sonjelle Shilton
BMC Public Health (2024)
Evaluations of modes of pooling specimens for COVID-19 screened by quantitative PCR and droplet digital PCR
- Daitao Zhang
- Lingyu Shen
- Shujuan Cui
Scientific Reports (2024)
Biporous silica nanostructure-induced nanovortex in microfluidics for nucleic acid enrichment, isolation, and PCR-free detection
- Eunyoung Jeon
- Joonseok Lee
Nature Communications (2024)
HaploCoV: unsupervised classification and rapid detection of novel emerging variants of SARS-CoV-2
- Matteo Chiara
- David S. Horner
- Graziano Pesole
Communications Biology (2023)
Effects of public-health measures for zeroing out different SARS-CoV-2 variants
- Shengjie Lai
Nature Communications (2023)
Quick links
- Explore articles by subject
- Guide to authors
- Editorial policies
Sign up for the Nature Briefing newsletter — what matters in science, free to your inbox daily.

COVID-19: impact on Public Health and hypothesis-driven investigations on genetic susceptibility and severity
Affiliations.
- 1 Departamento de Genética Humana, Instituto Nacional de Saúde Doutor Ricardo Jorge (INSA,IP), Lisboa, Portugal. [email protected].
- 2 Instituto de Investigação do Medicamento (iMed.ULisboa), Faculdade de Farmácia, Universidade de Lisboa, Lisboa, Portugal. [email protected].
- 3 Atlántida Centro de Investigación y Desarrollo de Estudios Profesionales (CIDEP), Granada, Spain.
- 4 MED-Instituto Mediterrâneo para a Agricultura, Ambiente e Desenvolvimento, Escola de Ciências e Tecnologia, Universidade de Évora, Évora, Portugal.
- 5 Université Claude Bernard Lyon 1, Lyon, France.
- 6 Departamento de Doenças Infeciosas, INSA, IP, Lisboa, Portugal.
- 7 Host-Pathogen Interaction Unit, Instituto de Investigação do Medicamento (iMed.ULisboa), Faculdade de Farmácia, Universidade de Lisboa, Lisboa, Portugal.
- 8 Hospital Egas Moniz, Centro Hospitalar Lisboa Ocidental, Lisboa, Portugal.
- PMID: 35348847
- PMCID: PMC8961091
- DOI: 10.1007/s00251-022-01261-w
COVID-19 is a new complex multisystem disease caused by the novel coronavirus SARS-CoV-2. In slightly over 2 years, it infected nearly 500 million and killed 6 million human beings worldwide, causing an unprecedented coronavirus pandemic. Currently, the international scientific community is engaged in elucidating the molecular mechanisms of the pathophysiology of SARS-CoV-2 infection as a basis of scientific developments for the future control of COVID-19. Global exome and genome analysis efforts work to define the human genetics of protective immunity to SARS-CoV-2 infection. Here, we review the current knowledge regarding the SARS-CoV-2 infection, the implications of COVID-19 to Public Health and discuss genotype to phenotype association approaches that could be exploited through the selection of candidate genes to identify the genetic determinants of severe COVID-19.
Keywords: COVID-19; Candidate gene association studies (CGAS); Genetic determinants of severe disease; Genetic susceptibility to infection; Genome-wide association studies (GWAS); Public Health.
© 2022. The Author(s), under exclusive licence to Springer-Verlag GmbH Germany, part of Springer Nature.
Publication types
- COVID-19* / genetics
- Genetic Predisposition to Disease
- Public Health
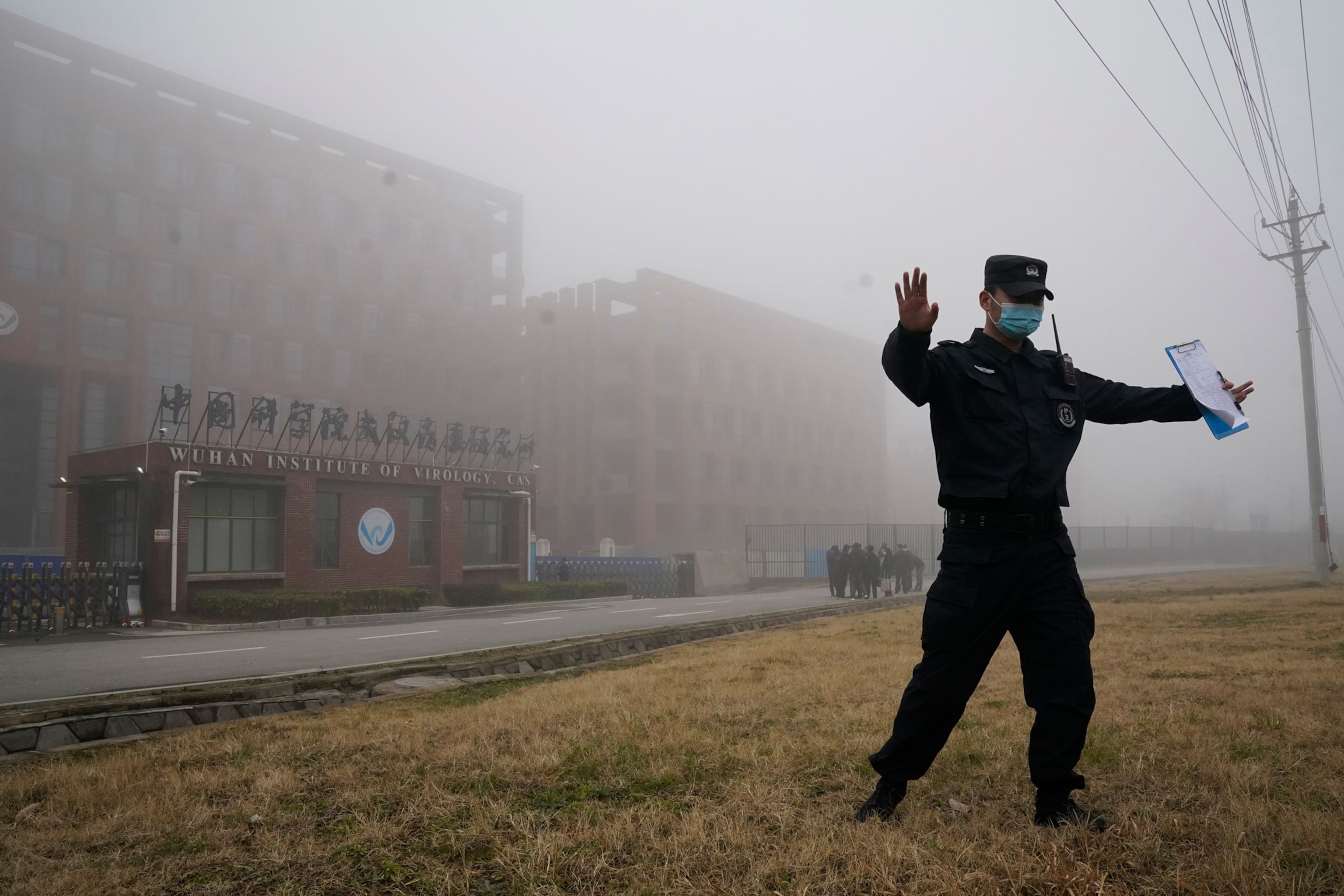
- CORONAVIRUS COVERAGE
What you need to know about the COVID-19 lab-leak hypothesis
Newly reported information has revived scrutiny of this possible origin for the coronavirus, which experts still call unlikely though worth investigating.
Months after a World Health Organization investigation deemed it “extremely unlikely” that the novel coronavirus escaped accidentally from a laboratory in Wuhan, China, the idea is back in the news, giving new momentum to a hypothesis that many scientists believe is unlikely, and some have dismissed as a conspiracy theory .
The renewed attention comes on the heels of President Joe Biden’s ordering U.S. intelligence agencies on May 26 to “ redouble their efforts ” to investigate the origins of the coronavirus. On May 11, Biden’s chief medical adviser, Anthony Fauci, acknowledged he’s now “ not convinced ” the virus developed naturally—an apparent pivot from what he told National Geographic in an interview last year.
Also last month, more than a dozen scientists—top epidemiologists, immunologists, and biologists—wrote a letter published in the journal Science calling for a thorough investigation into two viable origin stories: natural spillover from animal to human, or an accident in which a wild laboratory sample containing SARS-CoV-2 was accidentally released. They urged that both hypotheses “be taken seriously until we have sufficient data,” writing that a proper investigation would be “transparent, objective, data-driven, inclusive of broad expertise, subject to independent oversight,” with conflicts of interest minimized, if possible.
“Anytime there is an infectious disease outbreak it is important to investigate its origin,” says Amesh Adalja, an infectious disease physician and senior scholar at the Johns Hopkins University Center for Health Security who did not contribute to the letter in Science . “The lab-leak hypothesis is possible—as is an animal spillover,” he says, “and I think that a thorough, independent investigation of its origins should be conducted.”
Unanswered questions
The origins of SARS-CoV-2, the virus that causes COVID-19 and has infected more than 171 million people, killing close to 3.7 million worldwide as of June 4, remain unclear. Many scientists, including those that participated in the WHO’s months-long investigation, believe the most likely explanation is that that it jumped from an animal to a person—potentially from a bat directly to a human, or through an intermediate host. Animal-to-human transmission is a common route for many viruses; at least two other coronaviruses, SARS and MERS , were spread through such zoonotic spillover.
Other scientists insist it’s worth investigating whether SARS-CoV-2 escaped from the Wuhan Institute of Virology, a laboratory that has studied coronaviruses in bats for more than a decade.
For Hungry Minds
The WHO investigation —a joint effort between WHO-appointed scientists and Chinese officials—concluded it was “extremely unlikely” the highly transmissible virus escaped from a laboratory. But the WHO team suffered roadblocks that led some to question its conclusions; the scientists were not permitted to conduct an independent investigation and were denied access to any raw data. ( We still don’t know the origins of the coronavirus. Here are 4 scenarios .)
On March 30, when the WHO released its report, its director-general, Tedros Adhanom Ghebreyesus, called for further studies . “All hypotheses remain on the table,” he said at the time.
Then on May 11, Fauci told PolitiFact that while the virus most likely emerged via animal-to-human transmission, “it could have been something else, and we need to find that out.”
Recently disclosed evidence, first reported by the Wall Street Journal , has added fuel to the fire: Three researchers from the Wuhan Institute of Virology fell sick in November 2019 and sought hospital care, according to a U.S. intelligence report. In the final days of the Trump administration, the State Department released a statement that researchers at the institute had become ill with “symptoms consistent with both COVID-19 and common seasonal illness.”
You May Also Like
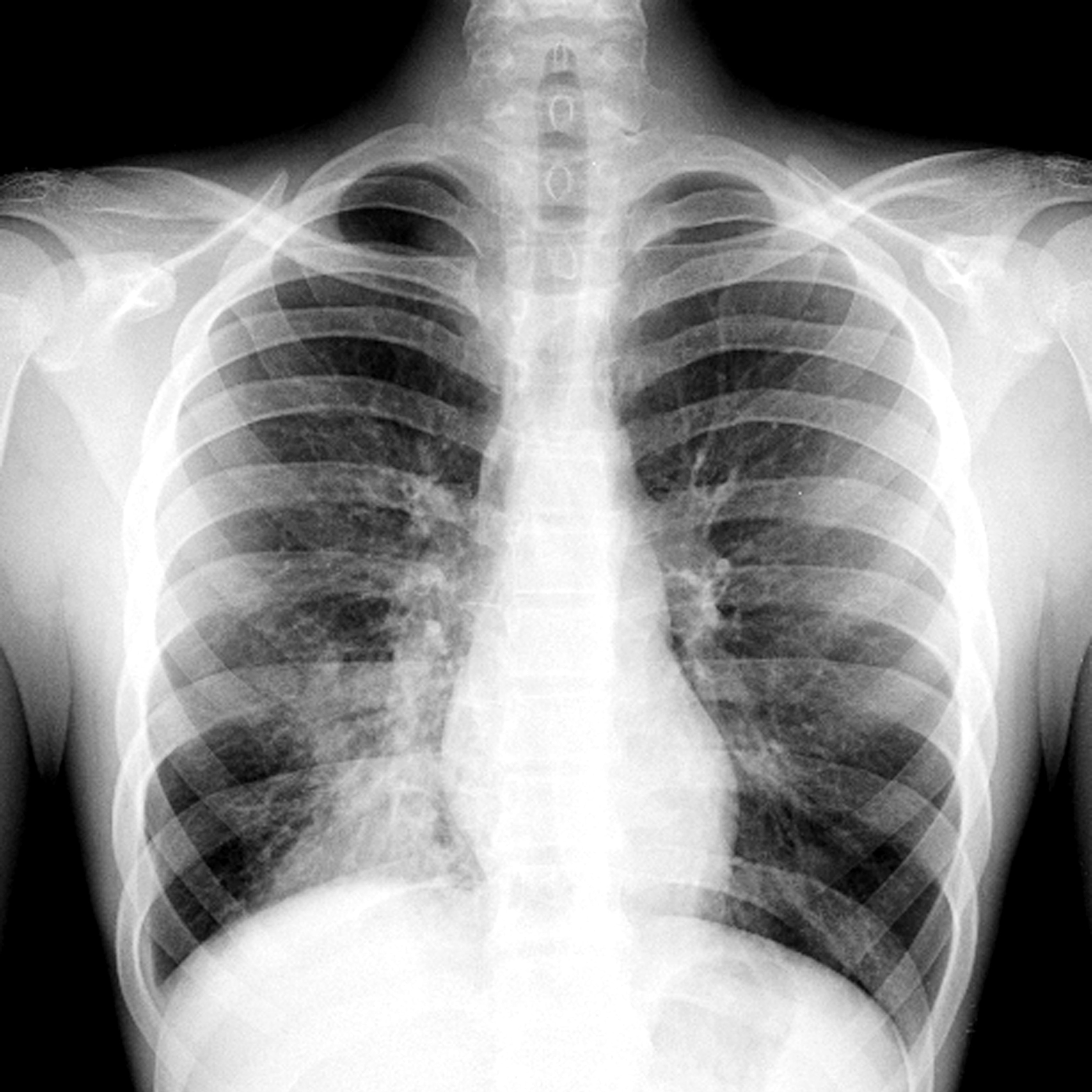
What is white lung syndrome? Here's what to know about pneumonia
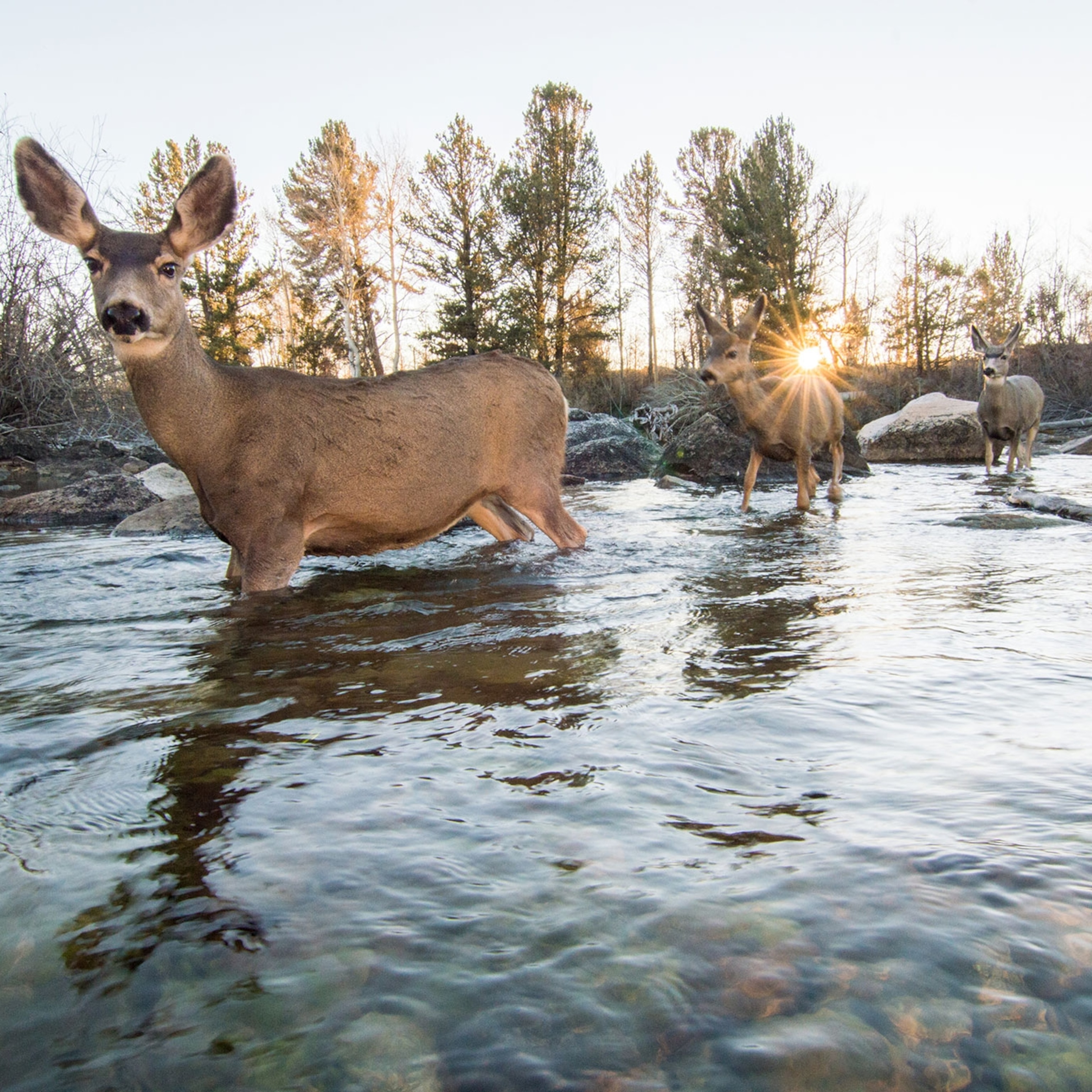
COVID-19 is more widespread in animals than we thought
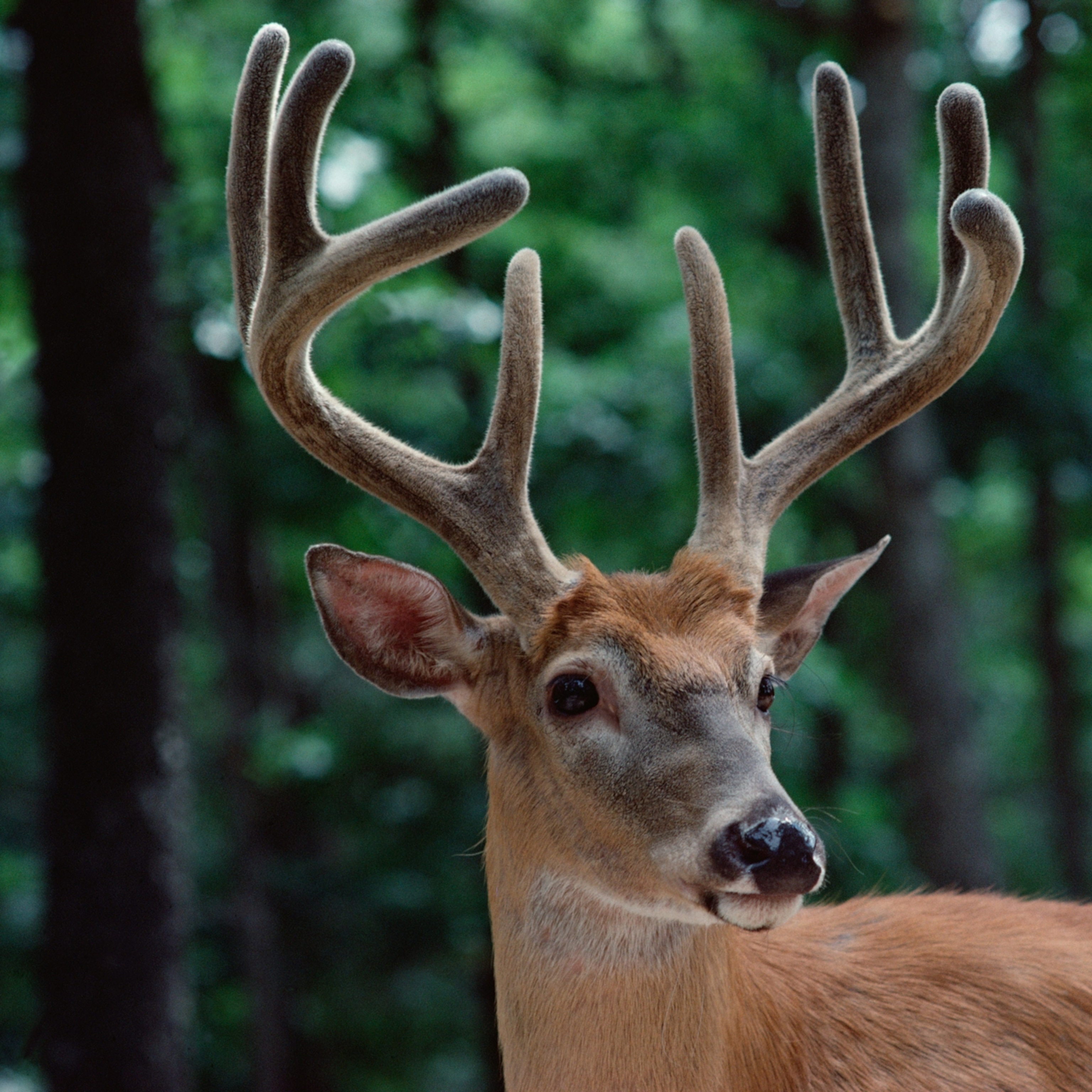
A deer may have passed COVID-19 to a person, study suggests
Most epidemiologists and virologists who have studied the novel coronavirus believe that it began spreading in November 2019. China says the first confirmed case was on December 8, 2019. During a briefing in Beijing this week, China’s foreign ministry spokesperson, Zhao Lijian, accused the U.S. of “ hyping up the theory of a lab leak ,” and asked, “does it really care about the study of origin tracing, or is it trying to divert attention?” Zhao also denied the Wall Street Journal report that three people had gotten sick.
Lab leak still ‘unlikely’
Some conservative politicians and commentators have embraced the lab-leak theory, while liberals more readily rejected it, especially early in the pandemic. The speculation has also heightened ongoing tensions between the U.S. and China.
On May 26, as the U.S. Senate passed a bill to declassify intelligence related to potential links between the Wuhan laboratory and COVID-19, Missouri Senator Josh Hawley, a Republican who sponsored the bill, said, “the world needs to know if this pandemic was the product of negligence at the Wuhan lab,” and lamented that “for over a year, anyone asking questions about the Wuhan Institute of Virology has been branded as a conspiracy theorist.”
Peter Navarro, Donald Trump’s former trade adviser, asserted in April 2020 that SARS-CoV-2 could have been engineered as a bioweapon, without citing any evidence.
The theory that SARS-CoV-2 was created as a bioweapon is “completely unlikely,” says William Schaffner, a professor of infectious diseases at Vanderbilt University Medical Center. For one thing, he explains, for a bioweapon to be successful, it must target an adversarial population without affecting one’s own. In contrast, SARS-CoV-2 “cannot be controlled,” he says. “It will spread, including back on your own population,” making it an extremely “counterproductive biowarfare agent.”
The more plausible lab-leak hypothesis, scientists say, is that the Wuhan laboratory isolated the novel coronavirus from an animal and was studying it when it accidentally escaped. “Not knowing the extent of its virulence and transmissibility, a lack of protective measures [could have] resulted in laboratory workers becoming infected,” initiating the transmission chain that ultimately resulted in the pandemic, says Rossi Hassad, an epidemiologist at Mercy College.
But Hassad adds he believes that this lab-leak theory is on the “extreme low end” of possibilities, and it “will quite likely remain only theoretical following any proper scientific investigation,” he says.
Biden ordered U.S. intelligence agencies to report back with their findings in 90 days, which would be August 26.
Based on the available information, Eyal Oren, an epidemiologist at San Diego State University, says it’s apparent why the most accepted hypothesis is that this virus originated in an animal and jumped to a human: “What is clear is that the genetic sequence of the COVID-19 virus is similar to other coronaviruses found in bats,” he says.
Some scientists remain skeptical that concrete conclusions can be drawn. “At the end, I anticipate that the question” of SARS-CoV-2’s origins “will remain unresolved,” Schaffner says.
In the meantime, science “moves much more slowly than the media and news cycles,” Oren says.
Related Topics
- CORONAVIRUS
- PUBLIC HEALTH
- WILDLIFE WATCHING
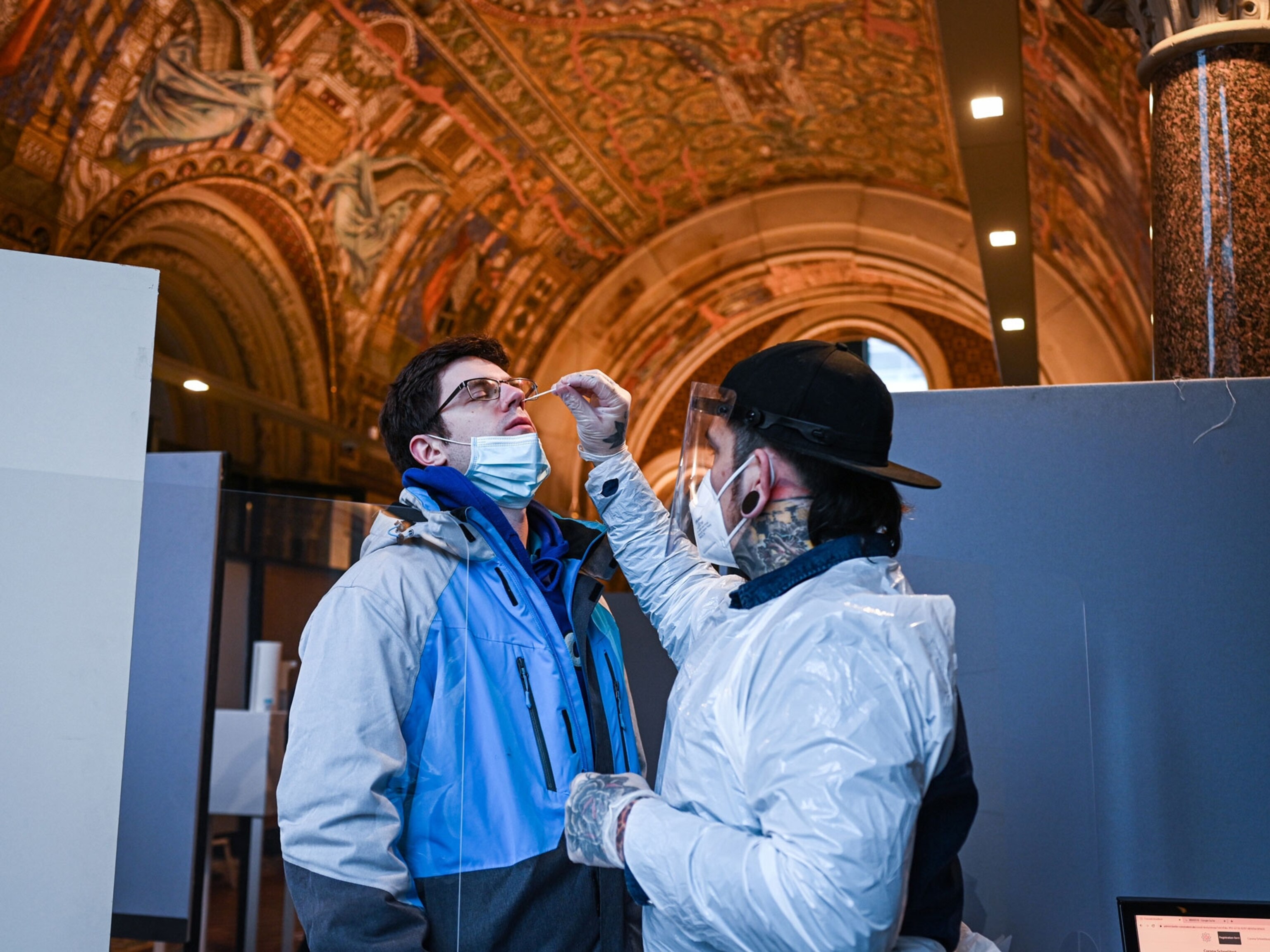
5 things to know about COVID-19 tests in the age of Omicron
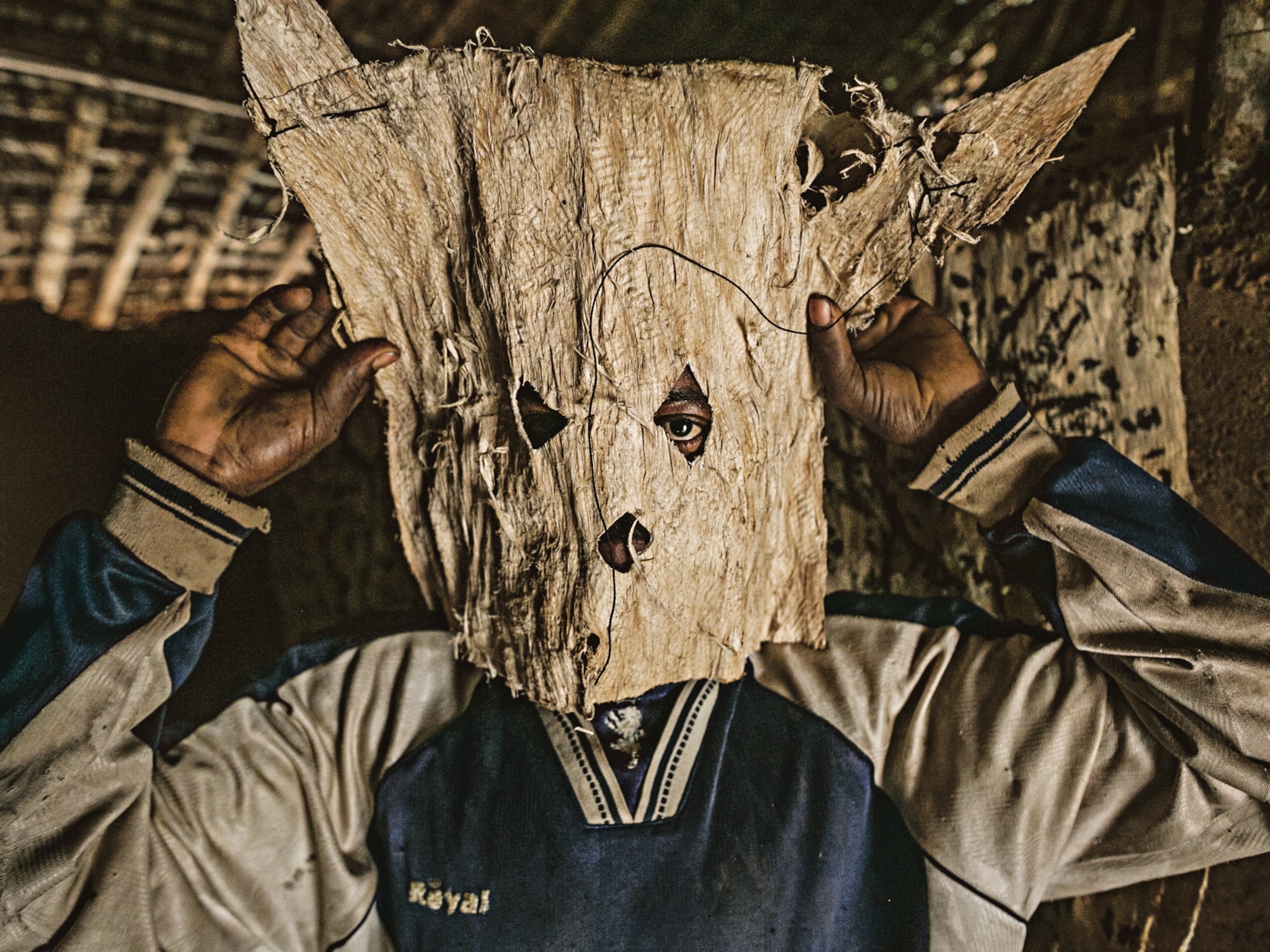
Seeking the Source of Ebola
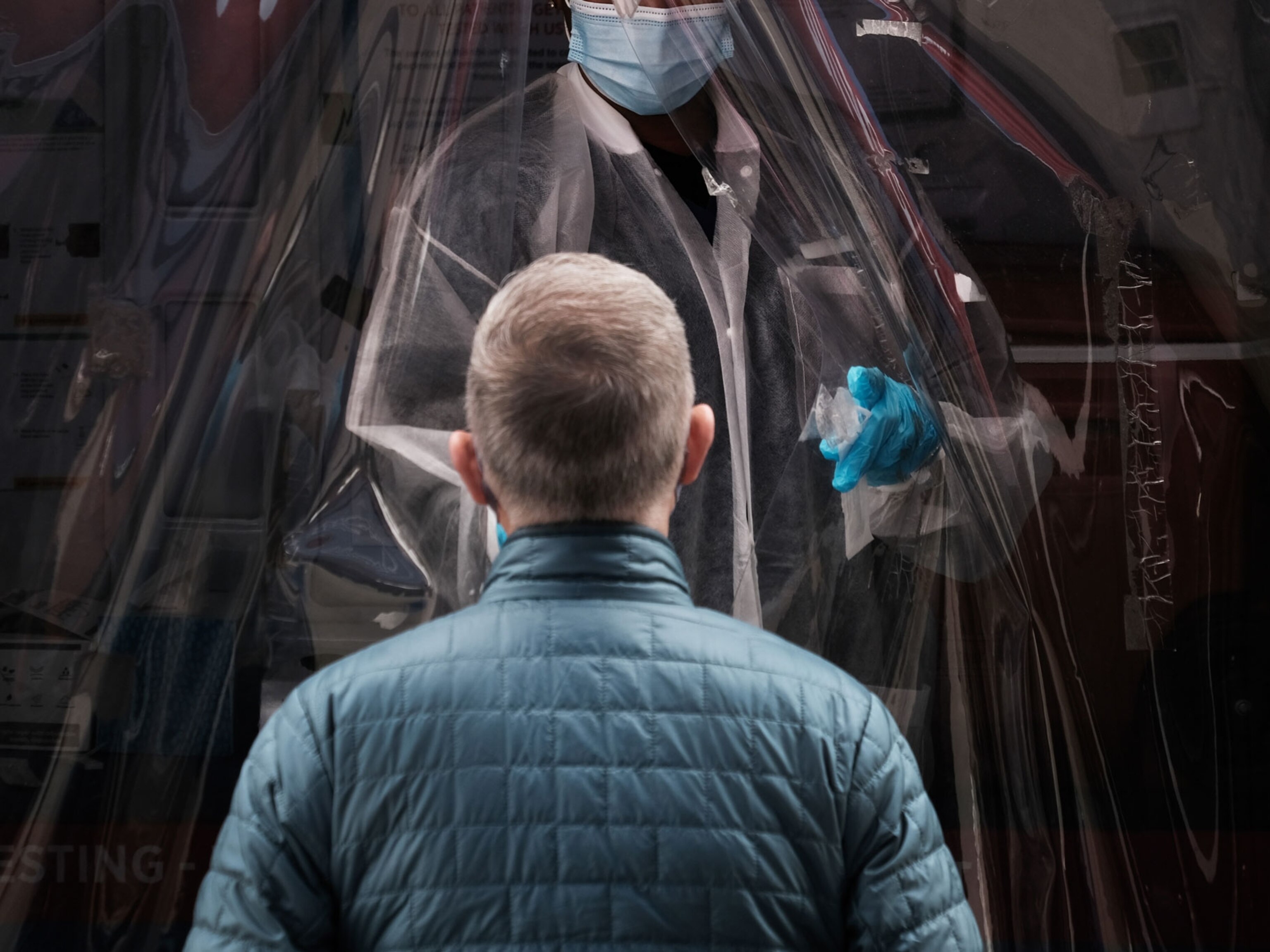
Here's what we know about the BA.2 Omicron subvariant driving a new COVID-19 wave
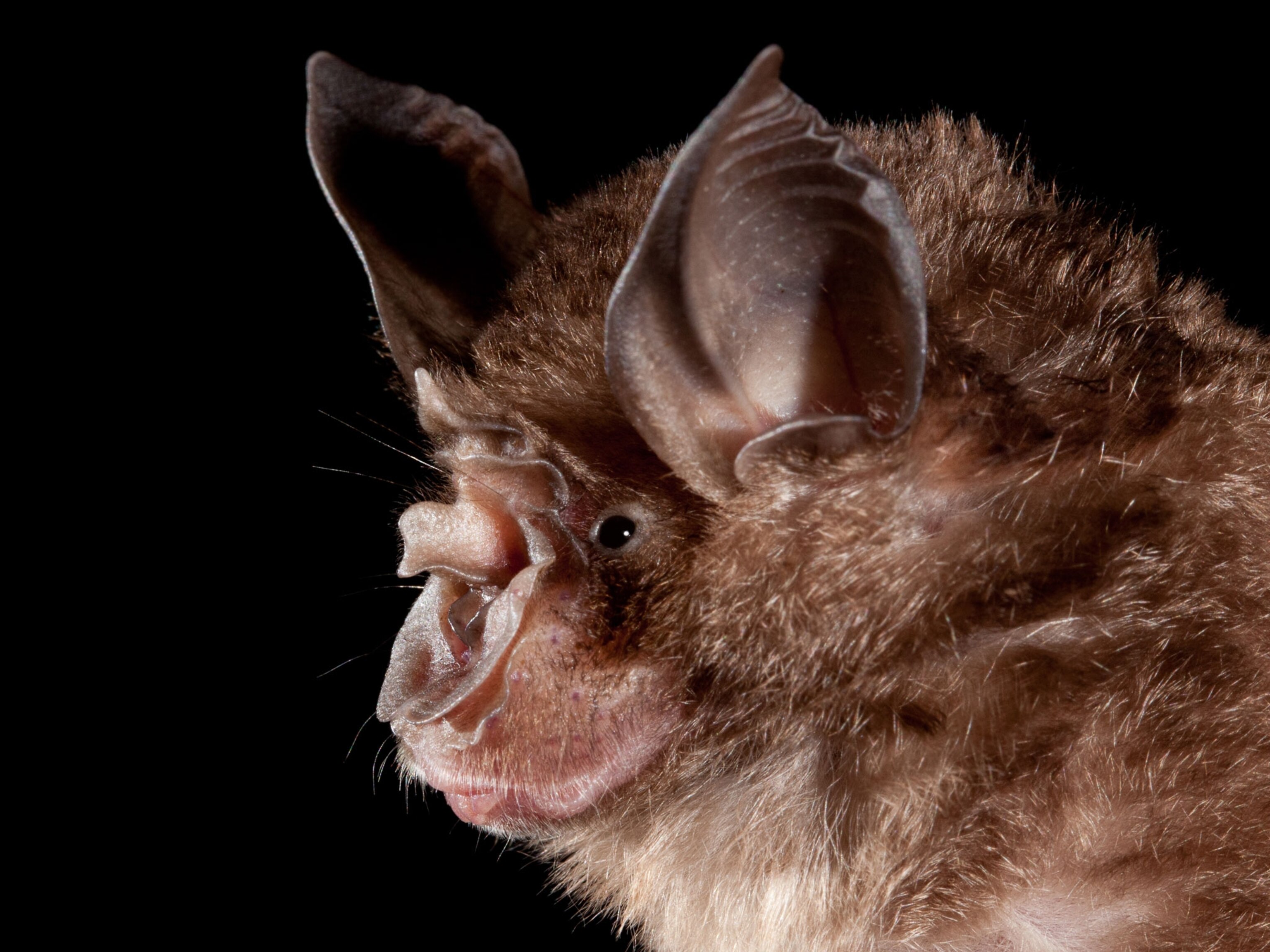
Humans are creating hot spots where bats could transmit zoonotic diseases
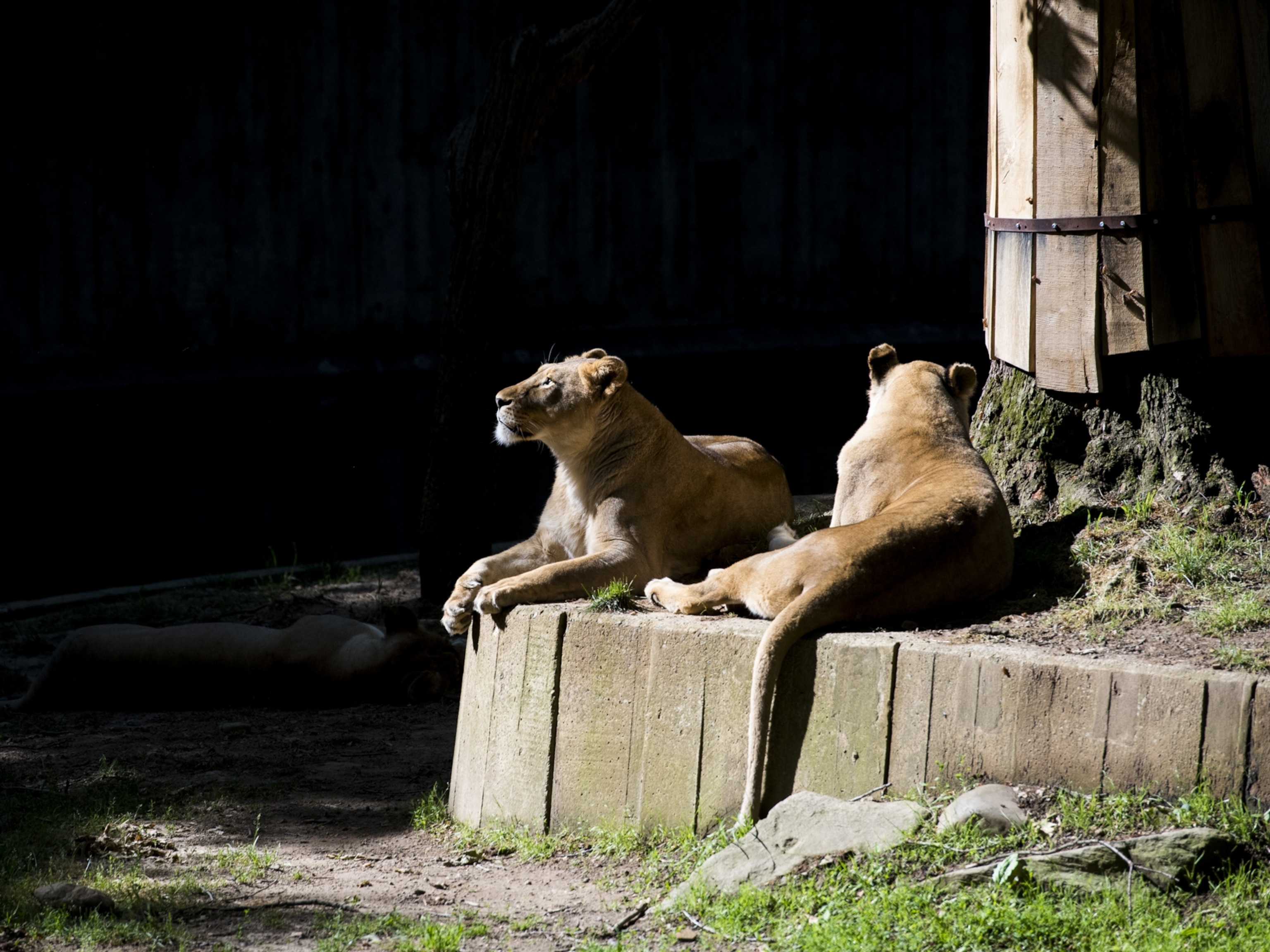
Hippos, hyenas, and other animals are contracting COVID-19
- Environment
- Perpetual Planet
History & Culture
- History & Culture
- History Magazine
- Mind, Body, Wonder
- Paid Content
- Terms of Use
- Privacy Policy
- Your US State Privacy Rights
- Children's Online Privacy Policy
- Interest-Based Ads
- About Nielsen Measurement
- Do Not Sell or Share My Personal Information
- Nat Geo Home
- Attend a Live Event
- Book a Trip
- Inspire Your Kids
- Shop Nat Geo
- Visit the D.C. Museum
- Learn About Our Impact
- Support Our Mission
- Advertise With Us
- Customer Service
- Renew Subscription
- Manage Your Subscription
- Work at Nat Geo
- Sign Up for Our Newsletters
- Contribute to Protect the Planet
Copyright © 1996-2015 National Geographic Society Copyright © 2015-2024 National Geographic Partners, LLC. All rights reserved
- About This IR
- Scholar Commons
Advancing Education in Quantitative Literacy
Home > Open Access Journals > NUMERACY > Vol. 14 (2021) > Iss. 1
Using COVID-19 Vaccine Efficacy Data to Teach One-Sample Hypothesis Testing
Frank Wang , LaGuardia Community College, CUNY Follow
COVID-19, hypothesis testing, Bayes’s rule, quantitative reasoning
In late November 2020, there was a flurry of media coverage of two companies’ claims of 95% efficacy rates of newly developed COVID-19 vaccines, but information about the confidence interval was not reported. This paper presents a way of teaching the concept of hypothesis testing and the construction of confidence intervals using numbers announced by the drug makers Pfizer and Moderna publicized by the media. Instead of a two-sample test or more complicated statistical models, we use the elementary one-proportion z -test to analyze the data. The method is designed to be accessible for students who have only taken a one-semester elementary statistics course. We will justify the use of a z -distribution as an approximation for the confidence interval of the efficacy rate. Bayes’s rule will be applied to relate the probability of being in the vaccine group among the volunteers who were infected by COVID-19 to the more consequential probability of being infected by COVID-19 given that the person is vaccinated.
https://doi.org/10.5038/1936-4660.14.1.1383
Recommended Citation
Wang, Frank. "Using COVID-19 Vaccine Efficacy Data to Teach One-Sample Hypothesis Testing." Numeracy 14, Iss. 1 (2021): Article 7. DOI: https://doi.org/10.5038/1936-4660.14.1.1383
Creative Commons License
Since January 11, 2021
Included in
Clinical Trials Commons , Higher Education and Teaching Commons
- Journal Home
- About This Journal
- Editorial Board
- Instructions to Authors
- Ethics and Malpractice Statement
- Submit Article
- Most Popular Papers
- Receive Email Notices or RSS
Advanced Search
ISSN: 1936-4660 (Online)
Scholar Commons | About This IR | FAQ | My Account | Accessibility Statement
Privacy Copyright
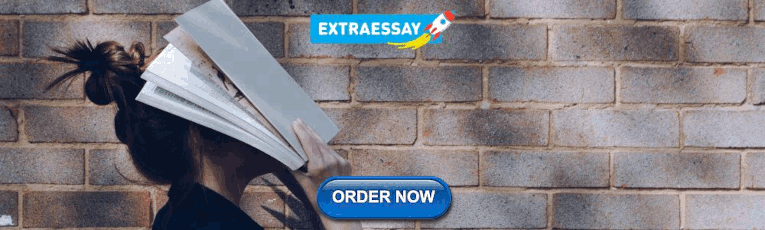
ORIGINAL RESEARCH article
The anxiety-buffer hypothesis in the time of covid-19: when self-esteem protects from the impact of loneliness and fear on anxiety and depression.
- 1 Section of Applied Psychology, Department of Philosophy, Sociology, Education, and Applied Psychology, University of Padua, Padua, Italy
- 2 Interdepartmental Center for Family Research, University of Padua, Padua, Italy
- 3 Unit of Psychology and Neuropsychology, Maugeri Scientific Institutes IRCCS, Novara, Italy
- 4 Psychology Research Laboratory, Ospedale San Giuseppe, IRCCS, Istituto Auxologico Italiano, Verbania, Italy
- 5 Department of Psychology, Catholic University of Milan, Milan, Italy
- 6 Department of Psychology, eCampus University, Novedrate, Italy
Introduction: The coronavirus (COVID-19) disease has spread worldwide, generating intense fear of infection and death that may lead to enduring anxiety. At the same time, quarantine and physical isolation can intensify feelings of dispositional loneliness that, by focusing on thoughts of disconnection from others, can trigger intense anxiety. Anxiety, generated by both fear of COVID-19 and dispositional loneliness, can activate negative expectations and thoughts of death, potentially generating alarming depressive symptoms. However, the anxiety-buffer hypothesis suggests that self-esteem acts as a shield (buffer) against mental health threats – fear and loneliness – thus hampering anxiety and depressive symptoms.
Objective: This study aims to test the process – triggered by COVID-19 fear and loneliness – in which self-esteem should buffer the path leading to anxiety symptoms, then to depression.
Methods: An observational research design with structural equation models was used. A sample of 1200 participants enrolled from the general population answered an online survey comprising: the fear of COVID-19 scale, the UCLA loneliness scale, the Rosenberg self-esteem scale, and the anxiety and depression scales of the Symptom Checklist-90-Revised.
Results: Structural equation models showed the link between anxiety symptoms ( mediator ) with both the fear of COVID-19 and dispositional loneliness ( predictors ), as well as its association with consequent depressive symptomatology ( outcome ). In line with the anxiety-buffer hypothesis, self-esteem mediated the relationship between the predictors and their adverse psychological consequences.
Conclusion: Self-esteem represents a protective factor from the antecedents of depression. Targeted psychological interventions should be implemented to minimize the psychological burden of the disease whilst promoting adaptation and positive psychological health outcomes.
Introduction
The novel coronavirus (COVID-19) is a new severe and potentially mortal disease threatening to infect the entire human population given that there is no prior immunity and not even a well-established cure or vaccine yet ( Baud et al., 2020 ).
COVID-19 displays a variety of clinical features ranging from asymptomatic presentations (20–50%), fever (>90%), cough (75%), shortness of breath (50%), up to acute respiratory distress syndrome, and death ( Byambasuren et al., 2020 ; Center for Disease Control and Prevention, 2020 ; Jiang et al., 2020 ). Categories of people at higher risk of developing severe complications of COVID-19 are older adults and people with previous underlying medical conditions, such as hypertension, cardiovascular disease, respiratory disease, and cancer ( Liu et al., 2020 ; Armitage and Nellums, 2020 ; Zheng et al., 2020 ). The contagion occurs from an infected person, even without obvious symptom manifestation, via respiratory droplets that can be inhaled or can land on surfaces which are later in contact with other people.
Due to its high transmissibility, since December 2019 COVID-19 has been rapidly spreading worldwide causing the current pandemic ( World Health Organization [WHO], 2020 ). Across the world, strict preventive policies were adopted to contain the outbreak of COVID-19 – including social distancing and social isolation. Nevertheless, the magnitude of this pandemic has generated serious concerns about its social and economic consequences both in the short and long-term ( Cerami et al., 2020 ). Thus, COVID-19 represents an epochal economic, physical, and biological threat to everyone’s lives.
Therefore, beyond threatening people’s physical conditions, COVID-19 is accompanied by remarkable psychological burdens heavily affecting people’s mental health ( Brooks et al., 2020 ; Torales et al., 2020 ; Wang et al., 2020 ). Similar to other physical diseases, COVID-19 represents a specific dangerous trigger activating a “fight or flight” reaction of (functional) fear focused on illness and death ( Schaller et al., 2015 ; Harper et al., 2020 ). The COVID-19 pandemic-related fear also led to counterproductive and detrimental behaviors for the whole society (i.e., demanding unnecessary medical care, excessively protecting against the virus, and overstocking certain supplies) ( Lin, 2020 ).
Moreover, fear of illness and death commonly lead to chronic vigilance for potential threats, thus contributing to the development of anxiety (i.e., the anticipation of a feared threat without a stimulus) that is future-oriented, unfocused, diffused, and extended to non-threatening situations ( Barlow, 2002 ; Harding et al., 2008 ).
In turn, anxiety might trigger and catalyze depressive symptoms via the activation of processes including persistent preoccupations, negative expectations, thoughts about death (of themselves or significant others), and pervasive pessimism ( Thompson et al., 2005 ; Starr and Davila, 2012 ). Depressive symptoms include feelings of sadness and loss, a negative view of the self, of the world, and of the future, thought and behavior are slowed down, and positive emotions are absent ( Beck, 1979 ). Noteworthy, depressive symptoms spread widely during the COVID-19 pandemic, representing an alarming predictor of suicide-behaviors ( McIntyre and Lee, 2020 ; Thakur and Jain, 2020 ).
At the same time, quarantine and physical distancing generated widespread feelings of isolation and loneliness – despite that fact that human connections were facilitated and granted by the use of communication technology ( Russell, 1996 ; Usher et al., 2020 ). Indeed, the dispositional trait of loneliness may have a crucial role in perceiving and amplifying feelings of isolation, thus exacerbating the adverse psychological impact of the outbreak ( Boffo et al., 2012 ). Indeed, dispositional loneliness is characterized by perceived disconnection from others and unpleasant feelings of isolation. Dispositional loneliness activates distressing thinking processes focusing on comparisons between the actual and the desired socio-relational situation. This contributes to the increase of unpleasant feelings and leads to the development of symptoms of anxiety that – in turn – lead to depressive symptomatology ( Cacioppo et al., 2006 , 2014 ; Santini et al., 2020 ). In other words, by activating (maladaptive) mechanisms and by influencing the brain and behavior, loneliness makes people more susceptible to the onset of anxious and depressive symptoms – thus representing an important risk factor for poor mental health ( Fiese et al., 2002 ; Heinrich and Gullone, 2006 ; Hossain et al., 2020 ; Lunn et al., 2020 ; Zhou et al., 2020 ), long-term morbidity (i.e., cardiovascular), and mortality ( Cacioppo et al., 2014 ; Leigh-Hunt et al., 2017 ; Rico-Uribe et al., 2018 ).
Consequently, both a fear of COVID-19 and dispositional loneliness could be considered as predictors of severe psychological symptoms of anxiety and depression, potentially leading to dismal effects, including extreme life-threatening behaviors ( Santini et al., 2020 ; Thakur and Jain, 2020 ).
However, self-esteem – that is the individuals’ attitudes, beliefs, and evaluations toward the self – may buffer these adverse patterns. According to Becker (1971 , 1973) , self-esteem is built on deep-rooted personal values derived from a given social, relational, and cultural context, and it is reinforced by social validation and the feeling of being a valuable human being with a meaningful role in society given by meeting the standards of a given culture and worldview ( Pyszczynski et al., 2004 ). More recently, the terror management theory (TMT) ( Greenberg et al., 1986 ) postulated that individuals’ awareness of mortality – in this case elicited by COVID-19 – conflicts with the human intrinsic desire for life and tendency to survive, thus generating terrifying fears of death and then anxiety. In this framework, the anxiety-buffer hypothesis (ABH; Greenberg et al., 1992 ) theorizes that, by reconnecting the individual with an enlarged universe of meanings and values, self-esteem could act as a protecting shield (buffer) against the detrimental psychological effects of life-threats and stressors.
Aims and Hypotheses
Considering this background, the present study aimed at testing the anxiety-buffer hypothesis during the COVID-19 pandemic. More in detail, self-esteem should buffer the relationships from both a fear of COVID-19 and dispositional loneliness to anxiety symptoms – that in turn lead to depressive symptoms. Moreover, specific hypotheses about each path (relationship) between variables were formulated:
H1: fear of COVID-19 and dispositional loneliness are positively associated with depressive symptomatology;
H2a: fear of COVID-19 predicts depressive symptomatology through anxiety symptoms (simple mediation) – without considering the buffering effect of self-esteem;
H2b: dispositional loneliness predicts depressive symptomatology through anxiety (simple mediation) – without considering the buffering effect of self-esteem;
H3: fear of COVID-19 and dispositional loneliness predict depressive symptomatology through anxiety symptoms (mediation) – without considering the buffering effect of self-esteem;
H4: fear of COVID-19 and dispositional loneliness predict depressive symptoms through self-esteem (buffering effect) and anxiety symptoms (multiple mediation).
In other words, it was hypothesized that a fear of COVID-19 and loneliness are associated with depressive symptomatology, but this relationship should be mediated by both anxiety and self-esteem. In particular, self-esteem should play a buffering role.
Materials and Methods
An online survey was developed and disseminated using the Qualtrics software for data collection.
Firstly, the survey was administered to 20 participants – not included into analysis (A) to ensure whether the items were understandable by the general population and (B) to estimate an acceptable time for its fulfillment (8’–20’), so as to deal adequately with potentially biased responses: too fast – random answers – or too slow –in which the subject could have been interrupted during the completion.
Then, the snowball sampling method ( Fricker, 2008 ) was used to recruit participants from the general population through personal invitations or materials advertised via social media platforms (i.e., Facebook, Twitter).
The recruitment materials provided details of what was required for participation in the study and a weblink to access the online questionnaire. The weblink directed potential participants first to further information on the research project in order to make an informed decision about study participation. Participants were informed that their responses were anonymous as well as that no economic payment was offered for their voluntary participation. Those who provided their consent online proceeded to the online questionnaire.
Inclusion criteria for the participants into the study were: (A) being a native Italian speaker; (B) being over 18 years old; and (C) providing informed consent. We excluded participants from the study who: (D) did not answer all the questions in the survey and (E) spent less than 8 min or more than 20 min completing the survey.
Data were collected in their entirety in a single week interval during the Italian quarantine to avoid confounding effects due to pandemic fluctuations. The study was approved by the Ethic Committee of the University of Padua in accordance with the Ethical standards of the Declaration of Helsinki.
Sample Size Determination
Considering the statistical analyses used in this study (see designated section), the sample size was calculated a priori according to the “ n:q criterion”: where n is the number of participants and q is the number of (free) model parameters to be estimated ( Hu and Bentler, 1999 ; Muthén and Asparouhov, 2002 ; Yu, 2002 ). Consequently, ten subject per free parameter (10:73; n minimum = 730) were guaranteed ( Bentler and Chou, 1987 ; Marsh et al., 1988 ; Hu and Bentler, 1999 ; Boomsma and Hoogland, 2001 ; Muthén and Asparouhov, 2002 ; Yu, 2002 ; Flora and Curran, 2004 ; Tomarken and Waller, 2005 ).
Participants
According to the inclusion criteria, 62 respondents were excluded from the study due to incomplete surveys ( n = 35) and inappropriate completion times ( n = 27).
The final sample was composed by 1200 participants [217 males (23.3%) and 713 females (76.7%), aged from 18 to 81 years ( mean = 39.59, SD = 12.334)], the average time competing the survey was 11’0.27” (SD = 3’0.02”). A total of 965 respondents were from Northern Italy (80.4%), 165 were from central Italy (13.8%), and 70 participants were from Southern Italy and the islands (5.8%). Descriptive statistics of this sample are reported in Table 1 .
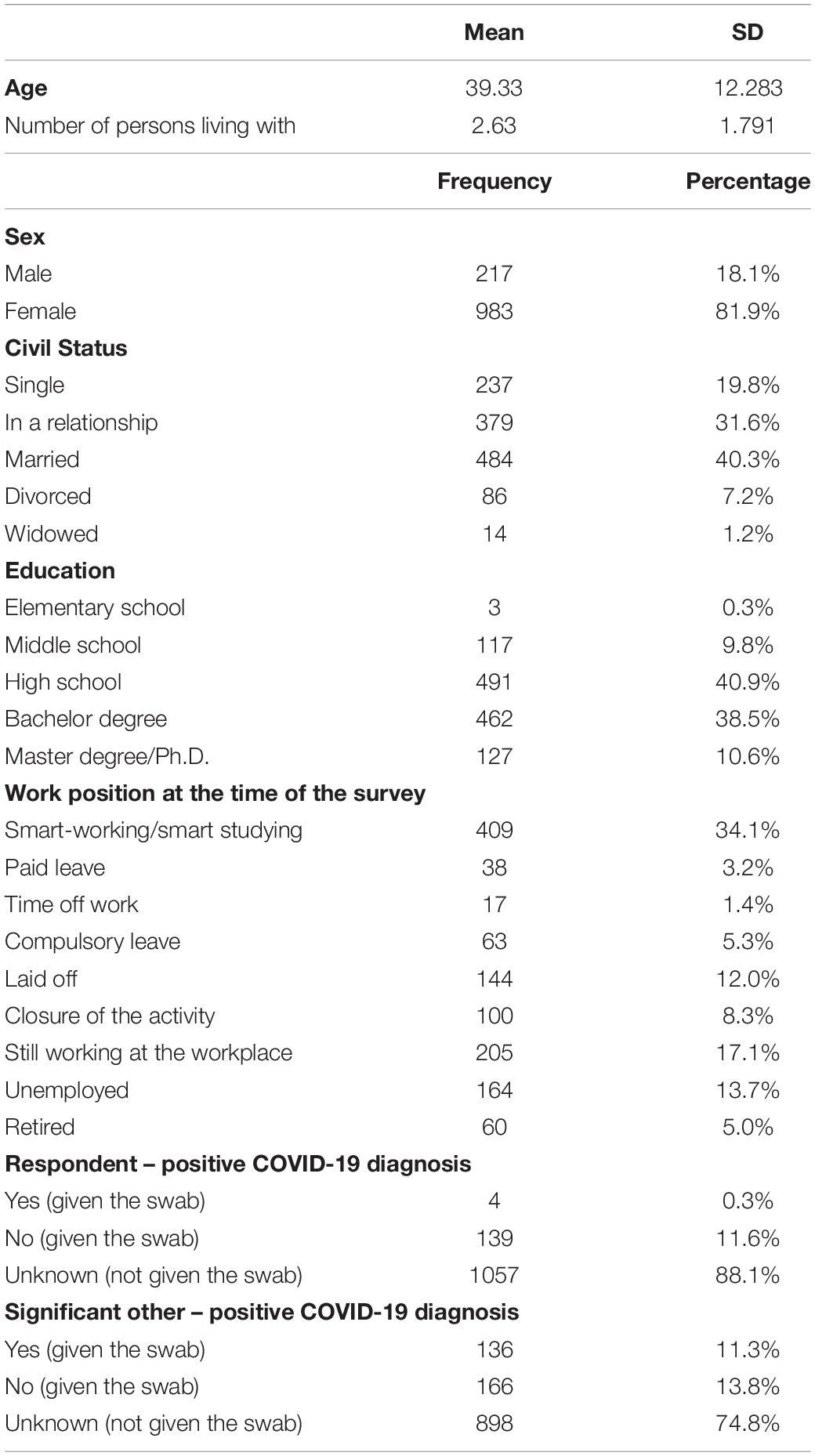
Table 1. Socio-demographic characteristics of the sample.
Socio-demographic information included sex, age, education, employment, Italian region of residence, number of persons living with, and confirmed positive COVID-19 diagnosis of the respondent and of his/her significant others. Table 1 reports the sample characteristics.
In addition, the following self-report measures were administered.
Fear of COVID-19 Scale – (FCV-19S)
The FCV-19S ( Ahorsu et al., 2020 ; Soraci et al., 2020 ) is a 7-item self-report questionnaire aimed at assessing emotional, cognitive, physiological, and behavioral manifestations of COVID-19-related fear in the general population. Respondents are asked to indicate their degree of agreement to each statement on a 5-point Likert-type scale (ranging from 1 = “strongly disagree” to 5 = “strongly agree” ) that provides a single-factor structure. Higher values indicate greater fear of COVID-19. In this study, the FCV-19S showed a high internal consistency (Cronbach’s alpha = 0.881).
University of California, Los Angeles, Loneliness Scale-Version 3 (UCLA-LS3)
The UCLA-LS3 ( Russell, 1996 ; Boffo et al., 2012 ) is a 20-item self-report scale that evaluates the individuals’ global and prolonged (dispositional) perceived sense of loneliness through three dimensions: (A) sense “habitual” isolation, (B) perception of being socially isolated, and (C) “traits” and dispositional factors of loneliness ( Boffo et al., 2012 ). In addition, a general dimension of “dispositional” loneliness is assumed. Respondents are asked to rate how often they feel the way described by each sentence on a 4-point Likert-type scale (ranging from 1 = “never” to 4 = “always” ). Higher values indicate the presence of a greater feeling of loneliness. In this study, the UCLA-LS3 showed a high internal consistency for each dimension (A – Isolation: Cronbach’s alpha = 0.805; B – Relational connectedness: Cronbach’s alpha = 0.822; C – Trait loneliness: Cronbach’s alpha = 0.869) and for the general dimension (Cronbach’s alpha = 0.913).
Rosenberg Self-Esteem Scale (RSE)
The RSE ( Rosenberg, 1965 ; Prezza et al., 1997 ) is one the most widely used self-report scales assessing global self-esteem in both clinical settings and in the general population. It consists of 10 positively and negatively worded statements evaluating feelings about one’s self. Respondents are asked to express their degree of agreement to each statement on a 4-point Likert-type scale (ranging from 1 = “not at all” to 4 = “always” ), and it provides a single-factor structure. Higher values indicate a greater sense of global self-esteem. In the present sample, the RSE showed a high internal consistency (Cronbach’s alpha = 0.869).
Anxiety Subscale of the Symptom Checklist-90 Revised (SCL-90R – ANX)
The SCL-90R ANX subscale ( Derogatis and Unger, 2010 ) is a 10-item self-report tool evaluating psychological, cognitive, and physical manifestations of anxiety during the previous week. For each statement, respondents are asked to rate the severity of their symptoms on a 5-point Likert-type scale (ranging from 1 = “not at all” to 5 = “always” ). The ANX subscale provides a single factor structure. Higher values indicate a greater anxiety symptomatology. In this study, the ANX subscale showed a high internal consistency (Cronbach’s alpha = 0.932).
Depression Subscale of the Symptom Checklist-90 Revised (SCL-90R – DEP)
The SCL-90R DEP scale of Derogatis and Unger (2010) is a 13-item self-report tool evaluating emotive, cognitive, and somatic manifestations of depression during the previous week. Respondents are asked to rate the severity of their symptoms on a 5-point Likert-type scale (ranging from 1 = “not at all” to 5 = “always” ). Also the DEP subscale provides a single factor structure. Higher values indicate a greater depressive symptomatology. In the present sample, the DEP subscale showed a high internal consistency (Cronbach’s alpha = 0.907).
Statistical Analyses
All analyses were performed with the R statistical software system (v. 3.5.3) [R-core project ( R Core Team, 2014 , 2017 )]. The following packages were used: psych (v. 1.8.12; Revelle, 2018 ), lavaan (v. 0.6-6; Rosseel, 2012 ; Rosseel et al., 2015 ), and semTools (v. 0.5-2; Jorgensen et al., 2019 ). Graphical representations were performed with graphViz in DiagrammeR (v.1.0.6.1; Iannone, 2018 ).
Preliminarily, a multivariate multiple regression analysis was performed to exclude the potential confounding effects of the following variables (covariates) on the aforementioned psychological constructs: (A) Italian region where respondents lived – as COVID-19 played out differently in Italy, (B) number of persons respondents lived with, (C) confirmed positive COVID-19 diagnosis of the respondents, and (D) confirmed positive COVID-19 diagnosis of the respondents’ significant other. Thus, external variables were simultaneously regressed on all the psychological constructs.
A Pearson correlation coefficient ( r ) was computed to evaluate the relationships between variables ( Tabachnick and Fidell, 2014 ).
A structural equation modeling (SEM) approach with latent variables was followed ( McDonald and Ho, 2002 ; Frazier et al., 2004 ; Weston and Gore, 2006 ; Iacobucci, 2008 ; Wiedermann and von Eye, 2015 ). A two-related separated predictors with a sequential multiple mediation model was specified ( MacKinnon, 2012 ; VanderWeele and Vansteelandt, 2014 ; Daniel et al., 2015 ; Hayes, 2017 ). The following procedure was performed.
Before examining the hypothesized model, the structural validity of each scale used in this study was tested by means of confirmatory factor analysis (CFAs). Considering the response scale of each of the questions administered in the study, the diagonally weighted least square (DWLS) estimator was used to perform each CFA separately ( Hoyle, 2012 ; Brown, 2015 ; Kline, 2016 ; Lionetti et al., 2016 ). Model fit was assessed by means of the following fit indices – and recommended cutoff values ( Bollen, 1989 ; Yu, 2002 ; Iacobucci, 2009 ; Hoyle, 2012 ; van de Schoot et al., 2012 ; Kline, 2016 ): (A) the Chi-square statistics (χ 2 ), preferably non-statistically significant ( p > 0.05) ( Bentler and Bonett, 1980 ; Muthén and Muthén, 1998-2017 ; Barrett, 2007 ); (B) the root-mean-square error of approximation (RMSEA), with values below 0.08 indicating an “acceptable” model fit and values below 0.05 indicating a “good” model fit ( Steiger and Lind, 1980 ; Steiger, 1990 ; Hu and Bentler, 1999 ; Barrett, 2007 ; van de Schoot et al., 2012 ); (C) the comparative fit index (CFI), with values between 0.90 and 0.95 for an “acceptable” fit ( Browne and Cudeck, 1989 ; Bentler, 1990 ; van de Schoot et al., 2012 ; Brown, 2015 ) and higher than 0.95 to indicate a “good” fit ( Bentler, 1990 ; Browne and Cudeck, 1992 ; Hu and Bentler, 1999 ), and (D) the standard root mean square residual (SRMR), with values lower than 0.08 considered a good model fit ( Hu and Bentler, 1999 ; Hoyle, 2012 ).
The Harman’s single-factor test was performed to check the potential “common method bias” ( Harman, 1976 ; Podsakoff et al., 2003 ; Brown, 2015 ). Firstly, a correlated factors model was specified: according to the measurement model, seven correlated factors (FCV19 – single factor, UCLA-LS3– three factors, RSE – single factor, ANX – single factor, and DEP – single factor) were specified – each item was specified to load onto its specific factor. Secondly, an alternative model was specified: a first-order single factor model was specified – all the items of the abovementioned scales were loaded onto a single latent dimension. Models were sequentially specified and compared using the test differences in goodness-of-fit indices (Δχ 2 : p > 0.050; ΔCFI: >0.010; ΔRMSEA: >0.015). Model comparisons were based on typical interpretation guidelines: for example, a statistically significant chi-square difference (Δχ 2 ; p < 0.050) and a ΔCFI greater than 0.010 suggest the absence of the bias ( Meredith, 1993 ; Vandenberg and Lance, 2000 ; Cheung and Rensvold, 2002 ; Chen, 2007 ; Millsap, 2012 ; Brown, 2015 ).
Latent factors were defined by using a partially disaggregated parcel approach in which latent constructs were defined by using parcels as indicators ( Bandalos and Finney, 2001 ; Coffman and MacCallum, 2005 ; Little et al., 2013 ). More in detail, since four scales were unidimensional (FCV-19S, RSE, ANX, and the DEP), item parcels were created using the “item-to-construct balance strategy” ( Bandalos and Finney, 2001 ; Little et al., 2002 ; Yang et al., 2010 ) – by inspecting factor loadings resulting from each measurement model ( Little et al., 2002 , 2013 ). However, since the UCLA-LS3 showed a hierarchical second-order structure, item parcels were created by using the “domain-representative strategy” ( Kishton and Widaman, 1994 ; Graham et al., 2000 ; Little et al., 2002 , 2013 ; Graham, 2004 ) – for each dimension, items were aggregated together. For each scale, at least a 3-item-parcel per latent variable were created – allowing each factor to be at least “just identified” – with factor loadings higher than |0.5| on the related construct ( Hoyle, 2012 ; Little et al., 2013 ; Brown, 2015 ; Kline, 2016 ). Once item parcels were created, descriptive statistics were examined: an almost normal distribution was found for the large majority of parcels. Thus, the maximum likelihood (ML) estimator was used for each SEM described in the following step (“Step 4”) ( Muthén and Muthén, 1998-2017 ; Hoyle, 2012 ; Kline, 2016 ). In addition, a 10,000 bootstrap resampling procedure was applied to each tested model ( MacKinnon, 2012 ).
The two-related separated predictors multiple mediation model was tested using a four-step approach ( MacKinnon et al., 2007 ; Iacobucci, 2009 ; Rucker et al., 2011 ; MacKinnon, 2012 ). Firstly , a predictors-only model was specified: fear of COVID-19 (X 1 ) and dispositional loneliness (X 2 ) predict depressive symptomatology (Y) – Figure 1 , Model 1. Secondly , a model was specified by excluding the effect of self-esteem (buffering variable) and dispositional loneliness: fear of COVID-19 (X 1 ) predict depressive symptomatology (Y) through anxiety symptoms (M) – Figure 1 , Model 2a. Thirdly , a parallel model was specified by excluding the effect of self-esteem (buffering variable) and fear of COVID-19: dispositional loneliness (X 2 ) predicts depressive symptomatology (Y) through anxiety symptoms (M) – Figure 1 , Model 2b. Fourthly , a semi-completed model was specified by only excluding the effect of self-esteem (buffering variable): fear of COVID-19 (X 1 ) and dispositional loneliness (X 2 ) predicts depressive symptomatology (Y) through anxiety symptoms (M) – Figure 1 , Model 3. Fifthly , the final model was specified by including the buffering effect of self-esteem: fear of COVID-19 (X 1 ) and dispositional loneliness (X 2 ) predict depressive symptoms (Y) through self-esteem (M 1 ) and anxiety symptoms (M 2 ) – Model 4, Figure 2 .
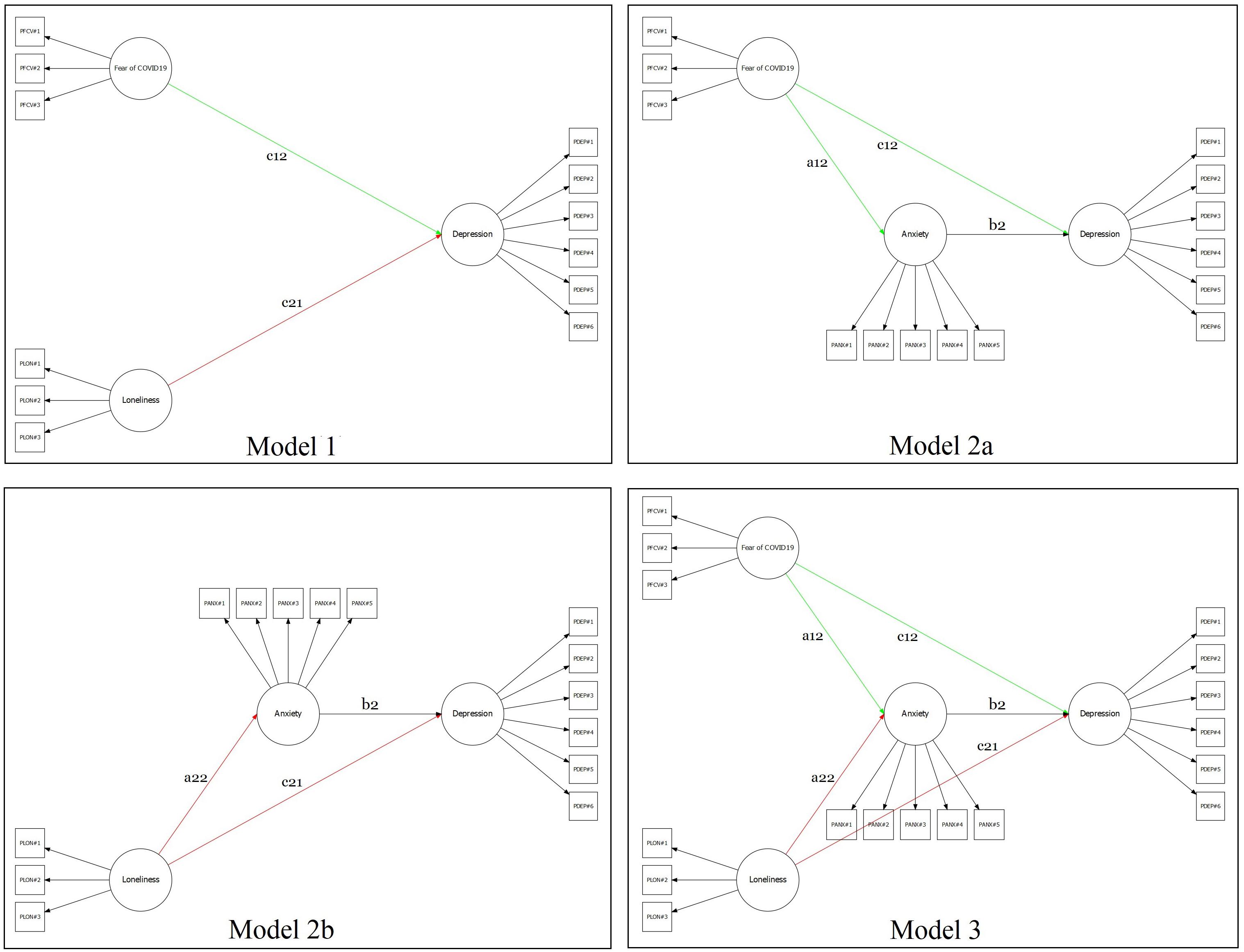
Figure 1. Graphical representation of the several mediation models tested.
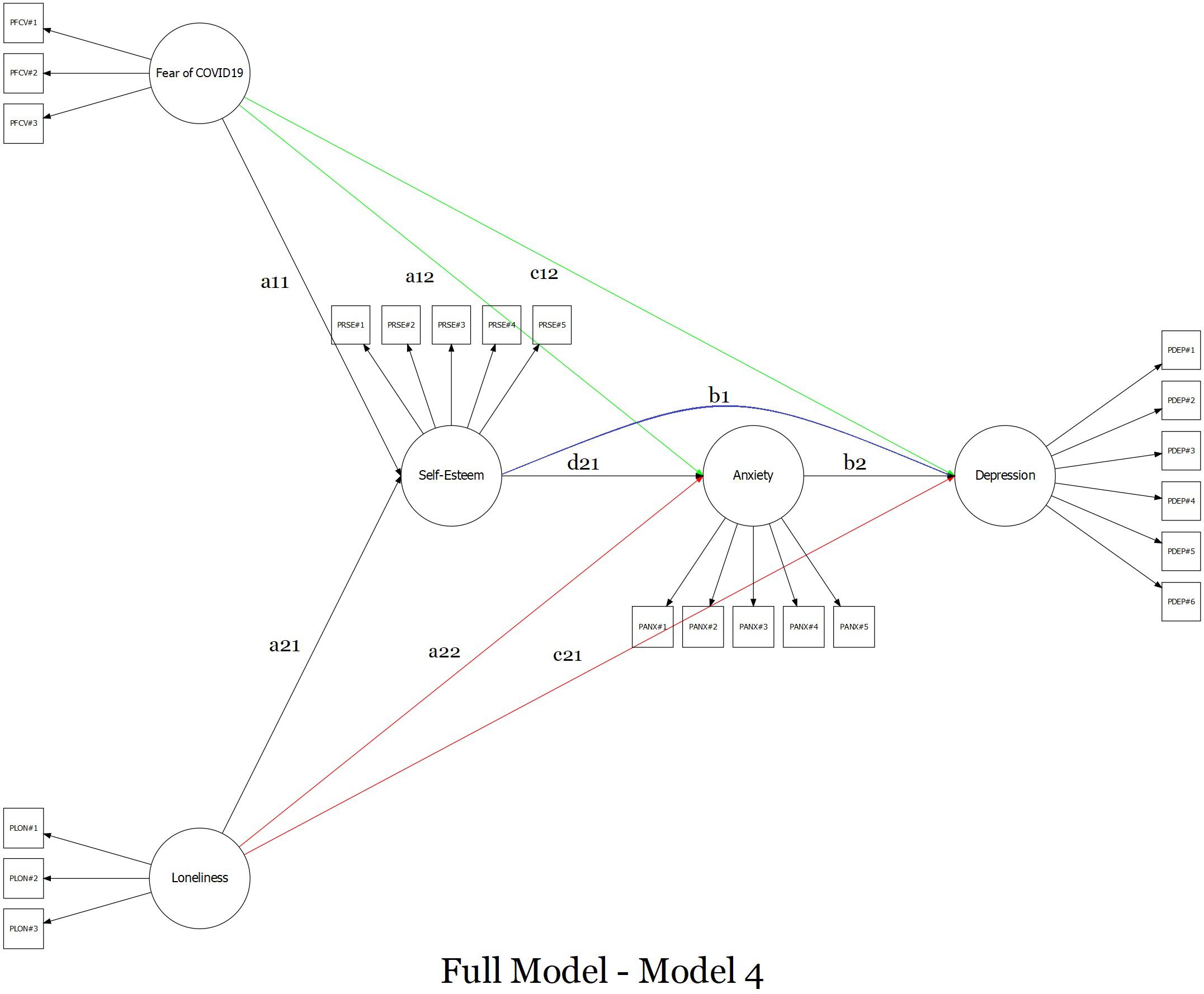
Figure 2. Graphical representation of the full sequential multiple mediation model with two-related different predictors.
Each of the five models described in “Step 4” was evaluated using the abovementioned “goodness-of-fit” indices (χ 2 , RMSEA, CFI, SRMR) and their cutoffs values – and each tested model had to provide good fit indices ( Frazier et al., 2004 ; Iacobucci, 2010 ). In addition, to avoid possible biases related to the scaling method (by default, the first factor loading of each latent variable was fixed to 1), an alternative model was specified by fixing the variance of each latent variable to unity ( Gonzalez and Griffin, 2001 ). This procedure was repeated for each of the five models described above. Finally, all regression coefficients (β) reported in the text were unstandardized.
Preliminary Analysis
The multivariate multiple regression analysis showed no statistically significant effects of external variables on psychological constructs. More in detail, controlling for other external variables, no statistically significant effect of (A) Italian region of residence was found on FCV-19S (β = 0.515, SE = 0.289, z = 1.786, p = 0.074), UCLA-LS3 (β = 0.290, SE = 0.518, z = 0.561, p = 0.575), RSE (β = −0.191, SE = 0.255, z = −0.751, p = 0.453), and DEP (β = 0.073, SE = 0.041, z = 1.800, p = 0.072). A negligible effect was found on ANX (β = 0.095, SE = 0.043, z = 2.207, p = 0.027). Moreover, controlling for other external variables, no statistically significant effect of the (B) number of persons living with was found on UCLA-LS3 (β = −0.377, SE = 0.236, z = −1.599, p = 0.110), RSE (β = 0.089, SE = 0.119, z = 0.747, p = 0.455), ANX (β = 0.029, SE = 0.020, z = 1.444, p = 0.149), and DEP (β = −0.017, SE = 0.019, z = −0.910, p = 0.363). A small effect was found on FCV-19S (β = 0.483, SE = 0.140, z = 3.443, p = 0.001). Moreover, controlling for other external variables, no statistically significant effect of (C) confirmed positive COVID-19 diagnosis of the respondent was found on FCV-19S (β = 0.556, SE = 0.544, z = 1.022, p = 0.307), UCLA-LS3 (β = −0.067, SE = 0.863, z = −0.077, p = 0.939), RSE (β = 0.508, SE = 0.394, z = 1.290, p = 0.197), ANX (β = 0.026, SE = 0.087, z = 0.302, p = 0.763), and DEP (β = −0.059, SE = 0.074, z = −0.800, p = 0.424). Finally, controlling for other external variables, no statistically significant effect of the presence of (D) confirmed positive COVID-19 diagnosis of the respondents’ significant other was found on FCV-19S (β = 0.100, SE = 0.268, z = 0.372, p = 0.710), UCLA-LS3 (β = 0.502, SE = 0.413, z = 1.217, p = 0.223), RSE (β = 0.086, SE = 0.205, z = 0.419, p = 0.675), ANX (β = −0.021, SE = 0.040, z = −0.511, p = 0.609), and DEP (β = −0.022, SE = 0.034, z = −0.667, p = 0.505).
In addition, correlation analyses suggested small-to-large associations between the variables involved in the multiple mediation model ( Table 2 ).
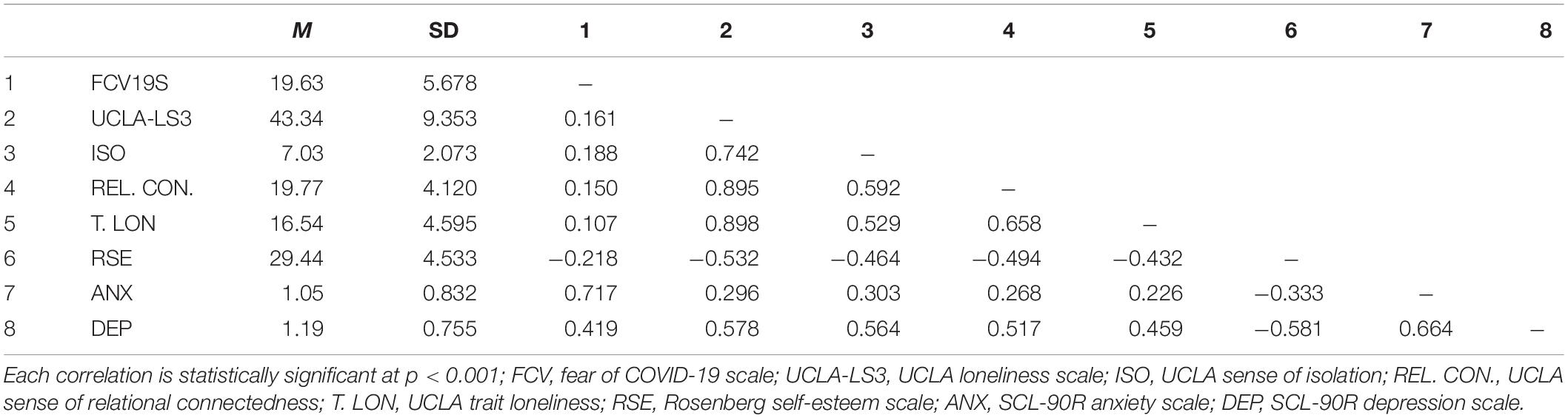
Table 2. Mean, standard deviation, and correlations between observed variables.
Structural Models
The FCV19S showed adequate goodness-of-fit indices: χ 2 (14) = 88.338; p < 0.001; RMSEA = 0.067; 90%CI 0.054–0.080; p (RMSEA < 0.05) = 0.018, CFI = 0.996, SRMR = 0.038. Factor loadings of the items ranged from 0.705 (item#2) to 0.872 (item#5) ( mean = 0.778; SD = 0.065).
The UCLA-LS3 showed adequate goodness-of-fit indices: χ 2 (167) = 1261.908; p < 0.001; RMSEA = 0.074; 90%CI 0.070–0.078; p (RMSEA < 0.05) < 0.001, CFI = 0.985, SRMR = 0.059. Factor loadings of the first-order items ranged from 0.555 (item#7 – Relational connectedness) to 0.892 (item#14 – Relational connectedness) ( mean = 0.719; SD = 0.157). Factor loadings of the second-order items ranged from 0.785 (Isolation) to 0.939 (Relational connectedness) ( mean = 0.851; SD = 0.079).
Also the RSE revealed good results: χ 2 (35) = 249.239; p < 0.001; RMSEA = 0.071; 90%CI 0.063–0.080; p (RMSEA < 0.05) < 0.001, CFI = 0.990, SRMR = 0.052. Factor loadings of the items ranged from 0.541 (item#4) to 0.817 (item#2) ( mean = 0.704; SD = 0.105).
Even the ANX showed good fit indices: χ 2 (35) = 208.462; p < 0.001; RMSEA = 0.064; 90%CI 0.056–0.073; p (RMSEA < 0.05) = 0.003, CFI = 0.997, SRMR = 0.036. Factor loadings of the items ranged from 0.768 (item#2) to 0.887 (item#3) ( mean = 0.830; SD = 0.043).
Finally, also the DEP revealed good fit indices: χ 2 (65) = 310.064; p < 0.001; RMSEA = 0.056; 90%CI 0.050–0.062; p (RMSEA < 0.05) = 0.053, CFI = 0.994, SRMR = 0.046. Factor loadings of the items ranged from 0.448 (item#1) to 0.896 (item#8) ( mean = 0.724; SD = 0.110).
Harman’s Single-Factor Test
The Harman’s single-factor test showed the absence of the “common method bias.” Indeed, the CFA with seven correlated factors (FCV19 – single factor, UCLA-LS3– three factors, RSE – single factor, ANX – single factor, and DEP – single factor) provided good fit indices [χ 2 (1689) = 8434.991; p < 0.001; RMSEA = 0.058; 90%CI 0.056–0.059; p (RMSEA < 0.05) < 0.001, CFI = 0.983, SRMR = 0.060]. Contrarily, the CFA with a single latent factor provided poor fit indices [χ 2 (1710) = 54429.649; p < 0.001; RMSEA = 0.160; 90%CI 0.159–0.162; p (RMSEA < 0.05) < 0.001, CFI = 0.866, SRMR = 0.147]. Model comparison suggested the absence of the “common method bias”: Δχ 2 (21) = 45995, p < 0.001; |ΔRMSEA| = 0.103, and |ΔCFI| = 0.117.
Multiple Mediation Model
The first model ( Figure 1 , model 1) provided adequate goodness-of-fit indices: χ 2 (51) = 377.938; p < 0.001; RMSEA = 0.073; 90%CI 0.066–0.080; p (RMSEA < 0.05) < 0.001, CFI = 0.964, SRMR = 0.043 ( Table 3 ). The fear of COVID-19 (X 1 ) was positively associated with depressive symptomatology (Y): β = 0.537 (SE = 0.047) [95%CI: 0.452; 0.632], z = 11.551, p < 0.001. At the same time, the dispositional loneliness (X 2 ) was positively associated with depressive symptomatology (Y): β = 0.932 (SE = 0.060) [95%CI: 0.822; 1.057], z = 15.484, p < 0.001. Moreover, fear of COVID-19 and loneliness were statistically significantly associated: β = 0.199 (SE = 0.035) [95%CI: 0.129; 0.267], z = 5.601, p < 0.001.
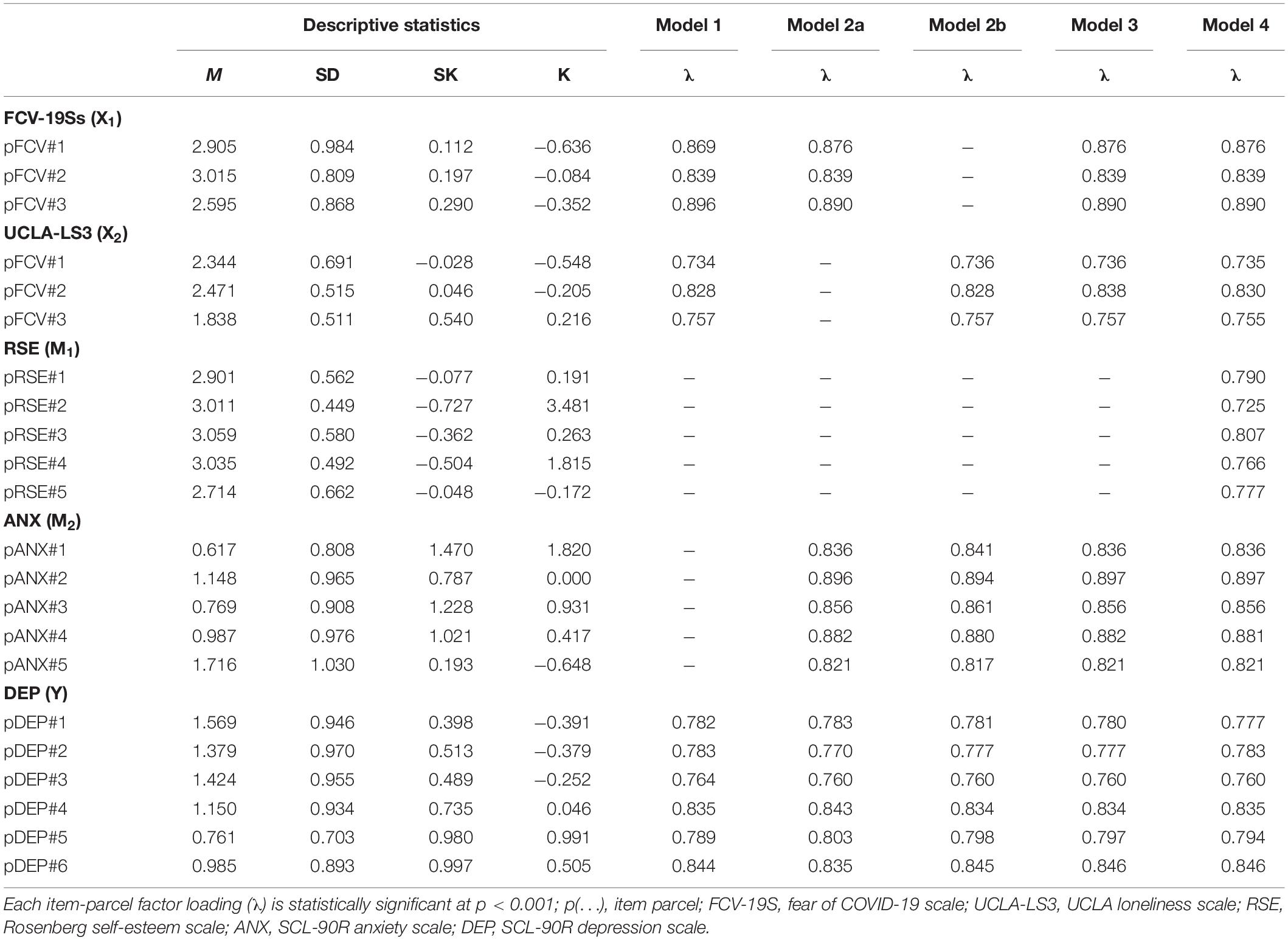
Table 3. Parcel descriptive statistics and standardized factor loadings (λ).
The second model ( Figure 1 , model 2a) provided adequate goodness-of-fit indices: χ 2 (74) = 505.982; p < 0.001; RMSEA = 0.070; 90%CI 0.064–0.076; p (RMSEA < 0.05) < 0.001, CFI = 0.968, SRMR = 0.039 ( Table 3 ). The fear of COVID-19 (X 1 ) was positively associated with anxiety symptomatology (M): β = 1.257 (SE = 0.064) [95%CI: 1.140; 1.390], z = 19.566, p < 0.001. Moreover, anxiety symptomatology (M) predicted depressive symptoms (Y): β = 0.827 (SE = 0.054) [95%CI: 0.724; 0.937], z = 15.321, p < 0.001. Also, fear of COVID-19 was negatively associated with depressive symptomatology: β = −0.338 (SE = 0.069) [95%CI: −0.476; −0.205], z = −4.865, p < 0.001. Furthermore, the total indirect effect was statistically significant [β = 1.039 (SE = 0.072) [95%CI: 0.908; 1.188], z = 14.372, p < 0.001] as well as the total model effect [β = 0.701 (SE = 0.058) [95%CI: 0.590; 0.821], z = 11.986, p < 0.001] – thus suggesting a partially mediated path.
The third model ( Figure 1 , model 2b) provided adequate goodness-of-fit indices: χ 2 (74) = 583.259; p < 0.001; RMSEA = 0.076; 90%CI 0.070–0.082; p (RMSEA < 0.05) < 0.001, CFI = 0.958, SRMR = 0.043 ( Table 3 ). Dispositional loneliness (X 2 ) was positively associated with anxiety symptomatology (M): β = 0.366 (SE = 0.038) [95%CI: 0.293; 0.442], z = 9.631, p < 0.001. Moreover, anxiety symptomatology (M) predicted depressive symptomatology (Y): β = 0.988 (SE = 0.063) [95%CI: 0.874; 1.121], z = 15.752, p < 0.001. In this case, dispositional loneliness was positively associated with depressive symptomatology: β = 0.931 (SE = 0.066) [95%CI: 0.806; 1.065], z = 14.025, p < 0.001. The total indirect effect was statistically significant [β = 0.361 (SE = 0.042) [95%CI: 0.285; 0.449], z = 8.660, p < 0.001] as well as the total model effect [β = 1.292 (SE = 0.080) [95%CI: 1.147; 1.459], z = 16.074, p < 0.001] – thus suggesting, a partially meditated model.
The fourth model ( Figure 1 , model 3) provided adequate goodness-of-fit indices: χ 2 (113) = 703.306; p < 0.001; RMSEA = 0.066; 90%CI 0.061–0.071; p (RMSEA < 0.05) < 0.001, CFI = 0.962, SRMR = 0.043 ( Table 3 ). As shown for “Model 1,” fear of COVID-19 (X 1 ) and dispositional loneliness (X 2 ) were positively associated: β = 0.199 (SE = 0.036) [95%CI: 0.128; 0.270], z = 5.523, p < 0.001. Fear of COVID-19 (X 1 ) was also positively associated with anxiety symptomatology (M): β = 1.256 (SE = 0.064) [95%CI: 1.136; 1.389], z = 19.713, p < 0.001. At the same time, dispositional loneliness (X 2 ) was positively associated with anxiety symptomatology (M): β = 0.330 (SE = 0.040) [95%CI: 0.251; 0.410], z = 8.179, p < 0.001. Moreover, anxiety symptomatology (M) predicted depressive symptomatology (Y): β = 0.722 (SE = 0.060) [95%CI: 0.661; 0.896], z = 12.938, p < 0.001. Also, as shown in “Model 2a” fear of COVID-19 was negatively associated with depressive symptomatology [β = −0.288 (SE = 0.079) [95%CI: −0.451; −0.138], z = −3.639, p < 0.001] and, as for “Model 2b,” dispositional loneliness was positively associated with depressive symptomatology: β = 0.924 (SE = 0.067) [95%CI: 0.801; 1.064], z = 13.852, p < 0.001.
The first total indirect effect (fear of COVID-19 → anxiety symptomatology → depressive symptomatology) was statistically significant [β = 0.970 (SE = 0.082) [95%CI: 0.822; 1.145], z = 11.785, p < 0.001] as well as the total model effect [β = 0.682 (SE = 0.060) [95%CI: 0.579; 0.806], z = 11.306, p < 0.001] – thus suggesting a partially mediated model. In addition, the second total indirect effect (dispositional loneliness → anxiety symptomatology → depressive symptomatology) was statistically significant [β = 0.255 (SE = 0.034) [95%CI: 0.191; 0.326], z = 7.427, p < 0.001] as well as the total model effect [β = 1.179 (SE = 0.078) [95%CI: 1.187; 1.714], z = 15.102, p < 0.001] – thus suggesting a partially mediated model.
The final model ( Figure 2 ) provided satisfying goodness-of-fit indices: χ 2 (199) = 918.943; p < 0.001; RMSEA = 0.055; 90%CI 0.051–0.059; p (RMSEA < 0.05) = 0.012, CFI = 0.962, SRMR = 0.039 ( Table 3 ). As shown for “Model 1,” fear of COVID-19 (X 1 ) and dispositional loneliness (X 2 ) were positively associated: β = 0.199 (SE = 0.036) [95%CI: 0.126; 0.269], z = 5.484, p < 0.001. According to the ABH, fear of COVID-19 (X 1 ) was negatively associated with self-esteem (M 1 ): β = −0.160 (SE = 0.040) [95%CI: −0.237; −0.082], z = −4.015, p < 0.001, and self-esteem – in turn – negatively predicted anxiety symptomatology (M 2 ): β = −0.127 (SE = 0.045) [95%CI: −0.216; −0.039], z = −2.797, p = 0.005 – thus revealing the buffering effect of self-esteem. Finally, anxiety symptomatology (M 2 ) positively predicted depressive symptomatology (Y): β = 0.769 (SE = 0.060) [95%CI: 0.657; 0.894], z = 12.775, p < 0.001. In addition, in line with the ABH, self-esteem (M 1 ) was negatively associated with depressive symptomatology (Y): β = −0.371 (SE = 0.052) [95%CI: −0.474; −0.269], z = −7.095, p < 0.001 – further suggesting the buffering effect of self-esteem. Furthermore, fear of COVID-19 (X 1 ) was positively associated with anxiety symptomatology (M 2 ) [β = 1.245 (SE = 0.065) [95%CI: 1.128; 1.380], z = 19.283, p < 0.001] and in line with “Model 2a” and “Model 3” fear of COVID-19 (X 1 ) was negatively associated with depressive symptomatology (Y) [β = −0.309 (SE = 0.079) [95%CI: −0.471; −0.159], z = −3.924, p < 0.001].
At the same time, according to the ABH, dispositional loneliness (X 2 ) was negatively associated with self-esteem (M 1 ): β = −0.798 (SE = 0.055) [95%CI: −0.913; −0.695], z = −14.403, p < 0.001 – revealing the buffering effect of self-esteem. Furthermore, dispositional loneliness (X 2 ) was positively associated with anxiety symptomatology (M 2 ) [β = 0.231 (SE = 0.055) [95%CI: 0.125; 0.341], z = 4.211, p < 0.001] and, in line with “Model 2b” and “Model 3,” also positively associated with depressive symptomatology (Y) [β = 0.703 (SE = 0.072) [95%CI: 0.570; 0.854], z = 9.700, p < 0.001].
The first total indirect effect (fear of COVID-19 → self-esteem → anxiety symptomatology → depressive symptomatology) was statistically significant [β = 0.016 (SE = 0.007) [95%CI: 0.004; 0.030], z = 2.324, p = 0.020] as well as the total model effect [β = 0.724 (SE = 0.064) [95%CI: 0.604; 0.858], z = 11.252, p < 0.001] – suggesting a partially mediated model that highlighted the buffering effect of self-esteem.
In addition, the second total indirect effect (dispositional loneliness → self-esteem → anxiety symptomatology → depressive symptomatology) was statistically significant [β = 0.078 (SE = 0.030) [95%CI: 0.023; 0.140], z = 2.634, p = 0.008] as well as the total model effect [β = 1.154 (SE = 0.083) [95%CI: 1.008; 1.332], z = 13.967, p < 0.001] – thus suggesting a partially mediated model with the buffering effect of self-esteem ( Table 4 ).
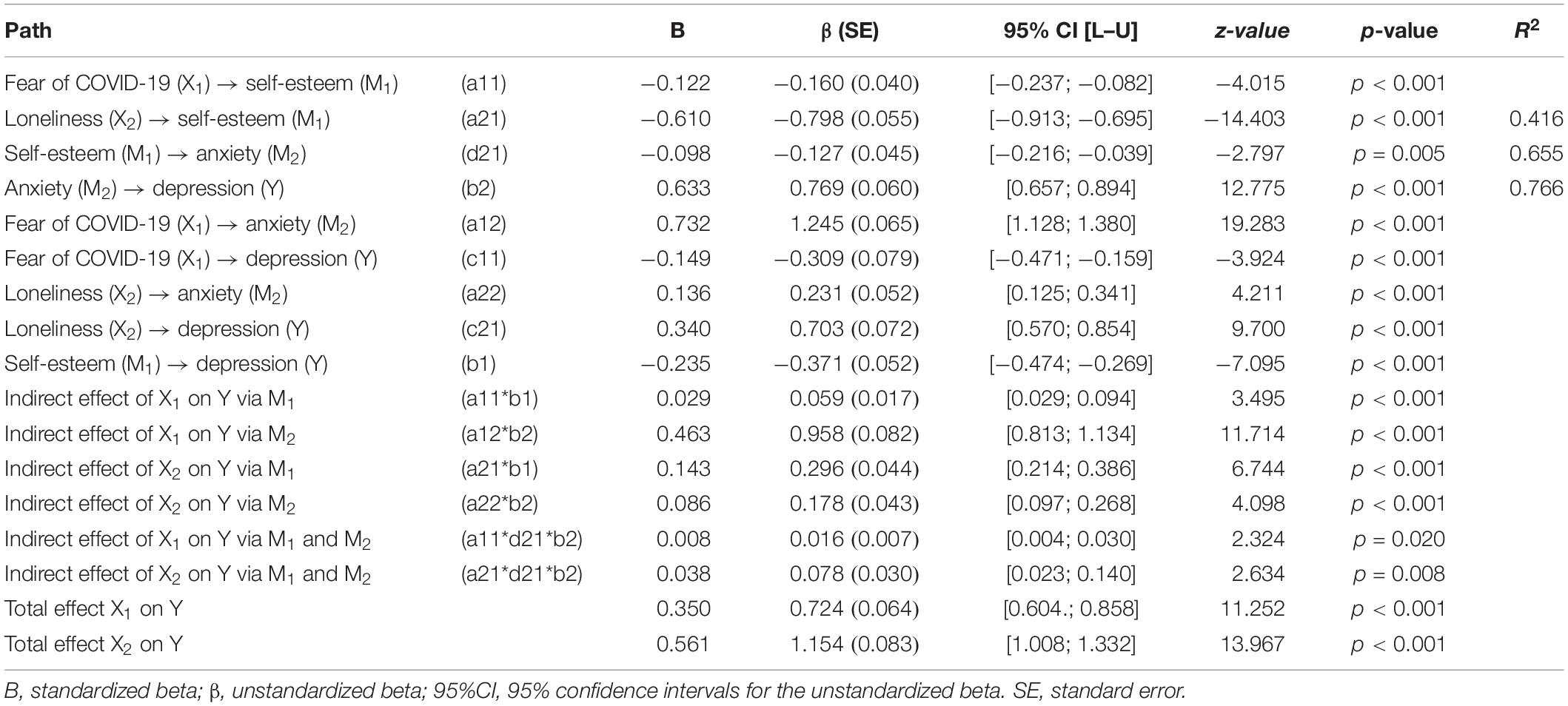
Table 4. Summary of parameter estimates (beta) with 95% confidence intervals for key pathways tested full model, Model 4 – Figure 2 .
Recently, the potential negative impact that the adverse psychological consequences of COVID-19 further had on the disease itself have been highlighted in the literature ( Center for Disease Control and Prevention, 2020 ; Lima et al., 2020 ; Parola, 2020 ; Parola et al., 2020 ; Thakur and Jain, 2020 ; Wind et al., 2020 ). Indeed, the advent of COVID-19 generated intense fear and anxiety about contagion, disease, and thoughts of death in the general population. At the same time, the sense of isolation was amplified by dispositional loneliness during the COVID-19 lockdown, with a consequent increase of anxiety symptoms. Therefore, both a fear of COVID-19 and dispositional loneliness represent major risk factors for the development of symptoms of anxiety and following symptoms of depression.
This study highlighted the buffering-effect of self-esteem on the relationships between negative psychological constructs, such as a fear of COVID-19 and dispositional loneliness feelings (predictors), and their consequent adverse psychological correlates – anxiety and depression (outcomes) during the COVID-19 pandemic.
In line with the scientific literature showing that (prolonged) fear can lead to depression ( Bowman, 2001 ), this study revealed that a fear of COVID-19 and loneliness might lead to depressive symptoms ( Santini et al., 2020 ; Thakur and Jain, 2020 ). Indeed, the first model that has been tested (Model 1 – predictors only) showed a positive relationship between a fear of COVID-19 and depressive symptomatology, with higher fear predicting higher depressive symptomatology. Indeed, when controlling for loneliness, an increase of 1 point in fear of COVID-19 was associated with an increase of 0.537 points in depression. At the same time, loneliness was positively associated with depressive symptoms: an increase in 1 point in dispositional loneliness was associated with an increase of 0.932 points in depression. These results suggest that a prolonged state of fear and dispositional feelings of loneliness might lead to the development of adverse psychological symptoms – thus representing major risk factors for the onset of symptoms of depression.
However, when controlling for anxiety activation (Model 3), fear (of COVID-19) and depression showed a negative association, probably due to the different nature of these emotional states. Indeed, on one hand, fear represents an activating emotion prompting the organism to react with the well-known “fight or flight” response. On the other hand, depression is characterized by a generalized de-activation, reflected in slowed-down behavior and thinking as well as flattened affectivity and pleasure ( Beck, 1979 ; Harper et al., 2020 ). At the same time, fear was positively strongly associated with anxiety symptoms ( Barlow, 2002 ; Harding et al., 2008 ), which might lead to depression ( Bowman, 2001 ) – thus suggesting a partially mediated model starting from fear up to depression through anxiety.
Simultaneously – when controlling for anxiety – dispositional loneliness was positively associated with depressive symptoms, further highlighting the existence of a strong relationship between these two constructs ( Cacioppo et al., 2014 ; Santini et al., 2020 ). Also, dispositional loneliness was positively associated with anxiety symptoms leading to depression ( Thompson et al., 2005 ; Starr and Davila, 2012 ) – suggesting, a partially mediated model (Model 3).
However, in line with the hypotheses, the final model (Model 4) highlighted the buffering role of self-esteem: despite positive associations held between fear of COVID-19, dispositional loneliness, and anxiety, the effect of self-esteem slowed down these negative adverse paths. Indeed – in line with the ABH and the TMT ( Greenberg et al., 1986 , 1992 ) – self-esteem had negative relationships with all the other psychological constructs (negative β values) due to its buffering effect hampering the relationships between adverse psychological variables. A partial mediation model was, therefore, suggested given that the relationship between fear of COVID-19, dispositional loneliness, anxiety, and depression held even when their paths were buffered by self-esteem.
Summarizing, results showed that self-esteem had a buffer effect protecting against anxiety symptoms triggered by a fear of COVID-19 and dispositional loneliness. Thus, these findings confirmed the validity of the ABH in the context of the COVID-19 pandemic.
Results also highlighted that both a fear of COVID-19 and dispositional loneliness were able to trigger unbearable feelings of anxiety that, in turn, were strongly linked to depressive symptomatology.
The strict interconnection between self-esteem and loneliness was probably due to the fact that loneliness is often related to negative self-evaluations, and feelings of being worthless, inferior, or unlovable ( Heinrich and Gullone, 2006 ). Previous studies suggested that self-esteem may impact on loneliness as a reinforcer or a buffer, as instances of influencing the relational competences ( Olmstead et al., 1991 ; Brage and Meredith, 1994 ; Heinrich and Gullone, 2006 ).
These results are in line with previous scientific literature highlighting that self-esteem can be a mediator in the relationship between loneliness, anxiety, and depression ( Brage and Meredith, 1994 ; Heinrich and Gullone, 2006 ; Çivitci and Çivitci, 2009 ).
Regarding the clinical implications of this study, its findings suggest a possible intervention strategy to provide psychological support to people suffering from the emotional consequences of COVID-19 and other COVID-19-related issues in order to alleviate the psychological outbreak of the pandemic. Indeed, according to the ABH, if self-esteem provides protection against stressors, such sources of stress should increase the need for self-esteem to relieve psychological burden ( Harmon-Jones et al., 1997 ). Consequently, increased self-esteem should function as a buffer toward anxiety, reducing the adverse psychological issues in response to threats or stressors. Thus, psychological interventions targeting self-esteem can represent an effective strategy to attenuate the distressing psychological responses to COVID-19 fear and dispositional feelings of loneliness – particularly among populations most susceptible and vulnerable to the negative psychological effects of the COVID-19 pandemic, including people with psychiatric disorders, those at risk of domestic violence, elderly people, and health-care practitioners ( Lai et al., 2020 ; Armitage and Nellums, 2020 ; Yao et al., 2020 ).
Moreover, given that loneliness derives from the perceived discrepancy between the actual and desired quality of relationships ( Peplau and Perlman, 1982 ), these results highlight the importance of perceived social support and positive relationships for people ( Ratti et al., 2017 ; Panzeri et al., 2019 ; Duan and Zhu, 2020 ). Individuals should, therefore, be guided and educated in strengthening their relationships and social support resources when physical contact is not possible (i.e., quarantine, hospitalization) by adopting tele-communication tools, such as smartphones. In line with the debated internet-paradox, proper technology use should be promoted to prevent distressing feelings ( Moody, 2001 ; Enez Darcin et al., 2016 ; Király et al., 2020 ).
Some noteworthy limitations of this study need to be acknowledged. Due to the observational/correlational nature of the research design, it was not possible to establish a causal relationship among variables, but only predictive relationships – still in line with the study purpose ( Fiedler et al., 2011 ). Moreover, the self-report nature of the online survey may convey intrinsic biases related to social desirability and other well-known issues ( Vidotto et al., 2018 ). Other limitations of this study were the prevalence of females in the sample and that the fact that geographical areas in Italy were not equally represented – although preliminary analyses showed no associated effects. Likewise, no differences emerged from sociodemographic characteristics, but future studies should deepen their possible role as protective/risk factors (i.e., presence vs. absence of social support) ( Mannarini et al., 2017a ). In addition, multi-group analyses assessing tested models across sex (males vs. females) were not performed. However, due to the small male sample size, multi-group mediation analyses would not be able to provide an accurate estimation of model parameters ( Hoyle, 2012 ; Kline, 2016 ). Future studies should, therefore, further test potential effects of sex on the suggested models. Moreover, all participants were Italian and possible effects of cross-cultural differences cannot be considered. Even though the ABH was successfully replicated in various countries as well as in different contexts ( Pyszczynski et al., 2004 ), future studies specifically examining the impact of COVID-19 on people’s lives should compare these results among different countries thus increasing the generalizability of these findings.
Finally, a mediation model was preferred to a moderation one for both theoretical and statistical reasons. Indeed, from a theoretical perspective a mediation-based approach is closer and more related to the original ABH and the TMT ( Greenberg et al., 1986 , 1992 ), conceptualizing self-esteem as an intermediating buffer between life-threatening stressors and anxiety ( Pyszczynski et al., 2004 ). In fact, self-esteem not only is able to influence individuals’ levels of anxiety and depression, but it is itself influenced by negative psychological experiences – such as fear and loneliness – activating negative cognitions and emotions that significantly affect the idea of oneself ( Greenberg et al., 1986 ; Heinrich and Gullone, 2006 ; Sowislo and Orth, 2013 ). Research show that fear can threaten self-evaluation ( Greenberg et al., 1986 ), and that people experiencing higher feelings of loneliness also have a worse self-evaluation ( Heinrich and Gullone, 2006 ). More in detail, negative experiences can activate both negative cognitions and emotions that significantly affect the idea of oneself (i.e., “I am a failure”, “I am worthless”) ( Beck, 1979 ) – thus, leaving scars in the self-concept, as well as persistently threatening and reducing self-esteem and self-efficacy ( Mannarini, 2010 ; Sowislo and Orth, 2013 ). Thus, a moderation approach would not suit the theoretical background of this study, and would not allow us to properly take into account the complexity of relationships among the considered psychological constructs. Regarding the strengths of the present study, it relies on a well-grounded theoretical basis supported by several experimental and longitudinal studies ( Greenberg et al., 1992 ; Brage and Meredith, 1994 ; Pyszczynski et al., 2004 ; Heinrich and Gullone, 2006 ). A wide sample of individuals from the general population was analyzed with strong statistical methodologies ( Iacobucci et al., 2007 ; MacKinnon, 2012 ; MacKinnon et al., 2012 , 2013 ) providing good results ( McDonald and Ho, 2002 ; Hayes, 2009 ; Iacobucci, 2010 ; Preacher, 2015 ). Moreover, the hypothesized models resulted in having a good fit, even if other solutions would have been possible but with lower fit indexes.
Given that individuals faced similar problems during past epidemics, findings from this study could also be generalized and applied to support people still coping with the negative consequences that previous disease outbreaks had on their mood (i.e., Ebola, SARS, MERS, and tuberculosis) ( Brown and Lees-Haley, 1992 ; Betancourt et al., 2016 ; Huremović, 2019 ; Chew et al., 2020 ). In a broader sense, these results could be extended to relieve the psychological burden of dysfunctional psychological reactions in response to physical and/or psychological illnesses ( Rossi Ferrario and Panzeri, 2020 ).
Overall, this study contributes to the current debate about the psychological implications of the COVID-19 pandemic, a prolonged and distressing situation triggering or worsening psychological issues. These findings may also be useful to help clinicians develop efficient and tailored interventions for increasing individuals’ mental health – with particular attention to the more fragile categories, such as young people and elderly people ( Parola and Donsì, 2018 , 2019 ; Balestroni et al., 2020 ).
Although, a considerable number of individuals may avoid seeking professional psychological help ( Rossi and Mannarini, 2019 ) due to the associated stigma ( Mannarini et al., 2017b , 2018 , 2020 ; Faccio et al., 2019 ; Mannarini and Rossi, 2019 ) or because of defensive denial reactions toward their psychological difficulties ( Sareen et al., 2007 ; Rossi Ferrario et al., 2019 ), thus choosing to manage the psychological issues on their own ( Wilson and Deane, 2012 ).
Future research will provide further insight about the evolution over time of the psychological issues related to COVID-19. Future studies might examine the role of social support as well as the changes in the dynamics of social and family relationships ( Mannarini et al., 2013 , 2017a ; Balottin et al., 2017 ).
Still, the role of other psychological constructs that may act as protective or risk factors, such as anger, post-traumatic symptoms, hopelessness, and denial should be further explored in future research in order to find effective treatment strategies to adopt in order to deal with consequences of both the COVID-19 and future pandemics.
The present research offers further support for the anxiety-buffer role of self-esteem, confirming TMT to be a well-grounded theoretical framework offering interesting and useful clinical insights in the context of the COVID-19 pandemic. Targeted psychological interventions should be implemented to properly support individuals suffering from COVID-19-related issues in order to minimize the psychological burden of the disease whilst promoting adaptation and positive psychological health outcomes.
Data Availability Statement
The raw data supporting the conclusions of this article will be made available by the authors, on reasonable requests.
Ethics Statement
The studies involving human participants were reviewed and approved by the Ethic Committee of the University of Padua. The patients/participants provided their written informed consent to participate in this study.
Author Contributions
AR conceived the study, performed the statistical analyses, and wrote the first draft. AP wrote a large part of the first draft and collected the data. GP helped with data collection and wrote part of the first draft. GM, GC, and SM provided important intellectual revisions. All authors contributed to the article and approved the submitted version.
Conflict of Interest
The authors declare that the research was conducted in the absence of any commercial or financial relationships that could be construed as a potential conflict of interest.
Ahorsu, D. K., Lin, C. Y., Imani, V., Saffari, M., Griffiths, M. D., and Pakpour, A. H. (2020). The Fear of COVID-19 scale: development and initial validation. Int. J. Ment. Health Addict. [Epub ahead of print]. doi: 10.1007/s11469-020-00270-8
CrossRef Full Text | Google Scholar
Armitage, R., and Nellums, L. B. (2020). COVID-19 and the consequences of isolating the elderly. Lancet. Public Health 5, e256–e256. doi: 10.1016/s2468-2667(20)30061-x
Balestroni, G., Panzeri, A., Omarini, P., Cerutti, P., Sacco, D., Giordano, A., et al. (2020). Psychophysical health of great elder inpatients in cardiac rehabilitation: a retrospective cohort study. Eur. J. Phys. Rehabil. Med. 56, 197–205. doi: 10.23736/S1973-9087.20.05970-5
PubMed Abstract | CrossRef Full Text | Google Scholar
Balottin, L., Mannarini, S., Mensi, M. M., Chiappedi, M., and Gatta, M. (2017). Triadic interactions in families of adolescents with anorexia nervosa and families of adolescents with internalizing disorders. Front. Psychol. 7:2046. doi: 10.3389/fpsyg.2016.02046
Bandalos, D. L., and Finney, S. J. (2001). “Item parceling issues in structural equation modeling,” in New Developments and Techniques in Structural Equation Modeling , eds G. Marcoulides and R. Schumacker (New York, NY: Psychology Press).
Google Scholar
Barlow, D. (2002). Anxiety and Its Disorders: The Nature and Treatment of Anxiety and Panic , 2nd Edn. New York, NY: Guilford Press.
Barrett, P. (2007). Structural equation modelling: adjudging model fit. Pers. Individ. Diff. 42, 815–824. doi: 10.1016/j.paid.2006.09.018
Baud, D., Qi, X., Nielsen-Saines, K., Musso, D., Pomar, L., and Favre, G. (2020). Real estimates of mortality following COVID-19 infection. Lancet Infect. Dis. 20:773. doi: 10.1016/S1473-3099(20)30195-X
Beck, A. T. (1979). Cognitive Therapy of Depression. New York, NY: Guilford press.
Becker, E. (1971). Birth and Death of Meaning: An Interdisciplinary Perspective on the Problem of Man. New York, NY: Free Press.
Becker, E. (1973). The Denial of Death. New York, NY: Simon & Schuster.
Bentler, P. M. (1990). Comparative fit indexes in structural models. Psychol. Bull. 107, 238–246. doi: 10.1037/0033-2909.107.2.238
Bentler, P. M., and Bonett, D. G. (1980). Significance tests and goodness of fit in the analysis of covariance structures. Psychol. Bull. 88, 588–606. doi: 10.1037/0033-2909.88.3.588
Bentler, P. M., and Chou, C. H. (1987). Practical issues in structural modeling. Sociol. Methods Res. 16, 78–117. doi: 10.1177/0049124187016001004
Betancourt, T. S., Brennan, R. T., Vinck, P., VanderWeele, T. J., Spencer-Walters, D., Jeong, J., et al. (2016). Associations between mental health and ebola-related health behaviors: a regionally representative cross-sectional survey in post-conflict sierra leone. PLoS Med. 13:e1002073. doi: 10.1371/journal.pmed.1002073
Boffo, M., Mannarini, S., and Munari, C. (2012). Exploratory structure equation modeling of the UCLA loneliness scale: a contribution to the Italian adaptation. TPM Test. Psychometr. Methodol. Appl. Psychol. 19, 345–363. doi: 10.4473/TPM19.4.7
Bollen, K. A. (1989). Structural Equations with Latent Variables. New York, NY: JohnWiley & Sons, Inc.
Boomsma, A., and Hoogland, J. J. (2001). “The robustness of LISREL modeling revisited. A Festschrift in honor of Karl Jöreskog,” in Structural Equation Models: Present and Future , eds R. Cudeck, S. du Toit, and D. Sorbom (Lincolnwood: Scientific Software International), 139–168.
Bowman, G. S. (2001). Emotions and illness. J. Adv. Nurs. 34, 256–263.
Brage, D., and Meredith, W. (1994). A causal model of adolescent depression. J. Psychol. 128, 455–468. doi: 10.1080/00223980.1994.9712752
Brooks, S. K., Webster, R. K., Smith, L. E., Woodland, L., Wessely, S., Greenberg, N., et al. (2020). The psychological impact of quarantine and how to reduce it: rapid review of the evidence. Lancet 395, 912–920. doi: 10.1016/s0140-6736(20)30460-8
Brown, R. S., and Lees-Haley, P. R. (1992). Fear of Future Illness, Chemical AIDS, and Cancerphobia: A Review. Psychological Reports. Los Angeles, CA: SAGE Publications.
Brown, T. A. (2015). Confirmatory Factor Analysis for Applied Research (Second ed.). New York: The Guilford Press.
Browne, M. W., and Cudeck, R. (1989). Single sample cross-validation indices for covariance structures. Multivariate Behav. Res. 24, 445–455. doi: 10.1207/s15327906mbr2404_4
Browne, M. W., and Cudeck, R. (1992). Alternative ways of assessing model fit. Sociol. Methods Res. 21, 230–258. doi: 10.1177/0049124192021002005
Byambasuren, O., Cardona, M., Bell, K., Clark, J., McLaws, M.-L., and Glasziou, P. (2020). Estimating the extent of true asymptomatic COVID-19 and its potential for community transmission: systematic review and meta-analysis. MedRxiv [Preprint]. doi: 10.1101/2020.05.10.20097543
Cacioppo, J. T., Hughes, M. E., Waite, L. J., Hawkley, L. C., and Thisted, R. A. (2006). Loneliness as a specific risk factor for depressive symptoms: cross-sectional and longitudinal analyses. Psychol. Aging 21, 140–151. doi: 10.1037/0882-7974.21.1.140
Cacioppo, S., Capitanio, J. P., and Cacioppo, J. T. (2014). Toward a neurology of loneliness HHS public access. Psychol. Bull. 140, 1464–1504. doi: 10.1037/a0037618
Center for Disease Control and Prevention (2020). Coronavirus Disease 2019 (COVID-19): Manage Anxiety & Stress. Atlanta: CDC.
Cerami, C., Santi, G. C., Galandra, C., Dodich, A., Cappa, S. F., Vecchi, T., et al. (2020). COVID-19 outbreak in Italy: are we ready for the psychosocial and economic crisis? baseline findings from the longitudinal psycovid study. SSRN Electron. J. 1–29. doi: 10.2139/ssrn.3569868
Chen, F. F. (2007). Sensitivity of goodness of fit indexes to lack of measurement invariance. Struct. Equ. Model. Multidiscip. J. 14, 464–504. doi: 10.1080/10705510701301834
Cheung, G. W., and Rensvold, R. B. (2002). Evaluating goodness-of-fit indexes for testing measurement invariance. Struct. Equ. Model. 9, 233–255. doi: 10.1207/S15328007SEM0902_5
Chew, Q., Wei, K., Vasoo, S., Chua, H., and Sim, K. (2020). Narrative synthesis of psychological and coping responses towards emerging infectious disease outbreaks in the general population: practical considerations for the COVID-19 pandemic. Singap. Med. J. 61, 350–356. doi: 10.11622/smedj.2020046
Çivitci, N., and Çivitci, A. (2009). Self-esteem as mediator and moderator of the relationship between loneliness and life satisfaction in adolescents. Pers. Individ. Diff. 47, 954–958. doi: 10.1016/j.paid.2009.07.022
Coffman, D. L., and MacCallum, R. C. (2005). Using parcels to convert path analysis models into latent variable models. Multivariate Behav. Res. 40, 235–259. doi: 10.1207/s15327906mbr4002_4
Daniel, R. M., De Stavola, B. L., Cousens, S. N., and Vansteelandt, S. (2015). Causal mediation analysis with multiple mediators. Biometrics 71, 1–14. doi: 10.1111/biom.12248
Derogatis, L. R., and Unger, R. (2010). “Symptom checklist-90-revised,” in Corsini Encyclopedia of Psychology , eds C. Nemeroff and W. E. Craighead (Hoboken, NJ: John Wiley & Sons, Inc). doi: 10.1007/978-3-319-56782-2_2012-2
Duan, L., and Zhu, G. (2020). Psychological interventions for people affected by the COVID-19 epidemic. Lancet Psychiatry 7, 300–302. doi: 10.1016/s2215-0366(20)30073-0
Enez Darcin, A., Kose, S., Noyan, C. O., Nurmedov, S., Yılmaz, O., and Dilbaz, N. (2016). Smartphone addiction and its relationship with social anxiety and loneliness. Behav. Inform. Technol. 35, 520–525. doi: 10.1080/0144929X.2016.1158319
Faccio, E., Belloni, E., Cipolletta, S., Iudici, A., Castiglioni, M., and Mannarini, S. (2019). The power of weight and the weight of power in adolescence: a comparison between young and adult women. J. Fam. Stud. 25, 46–60. doi: 10.1080/13229400.2016.1187660
Fiedler, K., Schott, M., and Meiser, T. (2011). What mediation analysis can (not) do. J. Exp. Soc. Psychol. 47, 1231–1236. doi: 10.1016/j.jesp.2011.05.007
Fiese, B. H., Tomcho, T. J., Douglas, M., Josephs, K.-B., Poltrock, S., and Baker, T. (2002). A review of 50 years of research on naturally occurring family routines and rituals: cause for celebration? J. Fam. Psychol. 16, 381–390. doi: 10.1037/0893-3200.16.4.381
Flora, D. B., and Curran, P. J. (2004). An empirical evaluation of alternative methods of estimation for confirmatory factor analysis with ordinal data. Psychol. Methods 9, 466–491. doi: 10.1037/1082-989X.9.4.466
Frazier, P. A., Tix, A. P., and Barron, K. E. (2004). Testing moderator and mediator effects in counseling psychology research. J. Counsel. Psychol. 51, 115–134. doi: 10.1037/0022-0167.51.1.115
Fricker, R. D. (2008). “Sampling methods for web and e-mail surveys,” in The SAGE Handbook of Online Research Methods , eds N. Fielding, G. Blank, and R. M. Lee (Thousand Oaks, CA: SAGE), 195–216. doi: 10.4135/9780857020055.n11
Gonzalez, R., and Griffin, D. (2001). Testing parameters in structural equation modeling: every “one” matters. Psychol. Methods 6, 258–269. doi: 10.1037/1082-989x.6.3.258
Graham, J. W. (2004). “Creating parcels for multi-dimensional constructs in structural equation modeling,” in Proceedings of the Biennal Meeting of the Society for Multivariate Analysis in the Behavioral Sciences , Jena.
Graham, J. W., Tatterson, J. W., and Widaman, K. F. (2000). “Creating parcels for multidimensional constructs in structural equation modeling,” in Proceedings of the Annual Meeting of the Society of Multivariate Experimental Psychology , Saratoga Springs, NY.
Greenberg, J., Pyszczynski, T., and Solomon, S. (1986). “The causes and consequences of a need for self-esteem: a terror management theory,” in Public Self and Private Self , ed. R. Baumeister (New York: Springer), 189–212. doi: 10.1007/978-1-4613-9564-5_10
Greenberg, J., Solomon, S., Pyszczynski, T., Rosenblatt, A., Burling, J., Lyon, D., et al. (1992). Why do people need self-esteem? Converging evidence that self-esteem serves an anxiety-buffering function. J. Pers. Soc. Psychol. 63, 913–922. doi: 10.1037/0022-3514.63.6.913
Harding, K. J., Skritskaya, N., Doherty, E., and Fallon, B. A. (2008). Advances in understanding illness anxiety. Curr. Psychiatry Rep. 10, 311–317. doi: 10.1007/s11920-008-0050-1
Harman, H. H. (1976). Modern Factor Analysis. Chicago, IL: University of Chicago Press.
Harmon-Jones, E., Simon, L., Greenberg, J., Solomon, S., Pyszczynski, T., and McGregor, H. (1997). Terror management theory and self-esteem: evidence that increased self-esteem reduces mortality salience effects. J. Pers. Soc. Psychol. 72, 24–36. doi: 10.1037/0022-3514.72.1.24
Harper, C. A., Satchell, L. P., Fido, D., and Latzman, R. D. (2020). Functional fear predicts public health compliance in the COVID-19 pandemic. Int. J. Ment. Health Addict. [Epub ahead of print]. doi: 10.1007/s11469-020-00281-5
Hayes, A. F. (2009). Beyond baron and kenny: statistical mediation analysis in the new millennium. Commun. Monogr. 76, 408–420. doi: 10.1080/03637750903310360
Hayes, A. F. (2017). Introduction to Mediation, Moderation, and Conditional Process Analysis: A Regression-Based Approach , 2nd Edn. New York, NY: Guilford publications.
Heinrich, L. M., and Gullone, E. (2006). The clinical significance of loneliness: a literature review. Clin. Psychol. Rev 26, 695–718. doi: 10.1016/j.cpr.2006.04.002
Hossain, M. M., Sultana, A., and Purohit, N. (2020). Mental health outcomes of quarantine and isolation for infection prevention: a systematic umbrella review of the global evidence. SSRN Electron. J. [Epub ahead of print]. doi: 10.2139/ssrn.3561265
Hoyle, R. H. (2012). Handbook of Strucural Equation Modeling. New York, NY: The Guilford Press.
Hu, L. T., and Bentler, P. M. (1999). Cutoff criteria for fit indexes in covariance structure analysis: conventional criteria versus new alternatives. Struct. Equ. Model. 6, 1–55. doi: 10.1080/10705519909540118
Huremović, D. (2019). “Psychiatry of pandemics. a mental health response to infection outbreak,” in Psychiatry of Pandemics , ed. D. Huremović (Cham: Springer International Publishing). doi: 10.1007/978-3-030-15346-5_1
Iacobucci, D. (2008). Mediation Analysis. Thousand Oaks, CA: Sage Publications, Inc.
Iacobucci, D. (2009). Everything you always wanted to know about SEM (structural equations modeling) but were afraid to ask. J. Consum. Psychol. 19, 673–680. doi: 10.1016/j.j.2009.09.002
Iacobucci, D. (2010). Structural equations modeling: fit indices, sample size, and advanced topics. J. Consum. Psychol. 20, 90–98. doi: 10.1016/j.jcps.2009.09.003
Iacobucci, D., Saldanha, N., and Deng, X. (2007). A meditation on mediation: evidence that structural equations models perform better than regressions. J. Consum. Psychol. 17, 139–153. doi: 10.1016/s1057-7408(07)70020-7
Iannone, R. (2018). DiagrammeR: Graph/Network Visualization. 1.0.0.
Jiang, F., Deng, L., Zhang, L., Cai, Y., Cheung, C. W., and Xia, Z. (2020). Review of the clinical characteristics of coronavirus disease 2019 (COVID-19). J. Gen. Intern. Med . 35, 1545–1549.
Jorgensen, T. D., Pornprasertmanit, S., Schoemann, A. M., and Rosseel, Y. (2019). semTools: Useful tools for structural equation modeling (Version 0.5–2).
Király, O., Potenza, M. N., Stein, D. J., King, D. L., Hodgins, D. C., Saunders, J. B., et al. (2020). Preventing problematic internet use during the COVID-19 pandemic: consensus guidance. Compr. Psychiatry 100:152180. doi: 10.1016/j.comppsych.2020.152180
Kishton, J. M., and Widaman, K. F. (1994). Unidimensional versus domain representative parceling of questionnaire items: an empirical example. Educ. Psychol. Meas. 54, 757–765. doi: 10.1177/0013164494054003022
Kline, R. B. (2016). Principles and Practice of Structural Equation Modeling. New York, NY: The Guilford Press.
Lai, J., Ma, S., Wang, Y., Cai, Z., Hu, J., Wei, N., et al. (2020). Factors associated with mental health outcomes among health care workers exposed to coronavirus disease 2019. JAMA Netw. Open 3:e203976. doi: 10.1001/jamanetworkopen.2020.3976
Leigh-Hunt, N., Bagguley, D., Bash, K., Turner, V., Turnbull, S., Valtorta, N., et al. (2017). An overview of systematic reviews on the public health consequences of social isolation and loneliness. Public Health 152, 157–171. doi: 10.1016/j.puhe.2017.07.035
Lima, C. K. T., Carvalho, P. M. M., Lima, I. A. A. S., Nunes, J. V. A., Saraiva, J. S., de Souza, R. I., et al. (2020). The emotional impact of coronavirus 2019-Ncov (New coronavirus disease). Psychiatry Res. 287:112915. doi: 10.1016/j.psychres.2020.112915
Lin, C.-Y. (2020). Social reaction toward the 2019 novel coronavirus (COVID-19). Soc. Health Behav. 3:1. doi: 10.4103/shb.shb_11_20
Lionetti, F., Keijsers, L., Dellagiulia, A., and Pastore, M. (2016). Evidence of factorial validity of parental knowledge, control and solicitation, and adolescent disclosure scales: when the ordered nature of Likert scales matters. Front. Psychol. 7:941. doi: 10.3389/fpsyg.2016.00941
Little, T. D., Cunningham, W. A., Shahar, G., and Widaman, K. F. (2002). To parcel or not to parcel: exploring the question, weighing the merits. Struct. Equ. Model. A Multidiscip. J. 9, 151–173. doi: 10.1207/s15328007sem0902_1
Little, T. D., Rhemtulla, M., Gibson, K., and Schoemann, A. M. (2013). Why the items versus parcels controversy needn’t be one. Psychol. Methods 18, 285–300. doi: 10.1037/a0033266
Liu, K., Chen, Y., Lin, R., and Han, K. (2020). Clinical features of COVID-19 in elderly patients: a comparison with young and middle-aged patients. J. Infect. 80, e14–e18.
Lunn, P., Belton, C., Lavin, C., Mcgowan, F., Timmons, S., and Robertson, D. (2020). Using behavioural science to help fight the coronavirus. Behav. Res. Unit 656, 1–24.
MacKinnon, D. P. (2012). Introduction to Statistical Mediation Analysis . New York, NY: Routledge
MacKinnon, D. P., Cheong, J., and Pirlott, A. G. (2012). “An introduction to statistical mediation analys,” in APA Handbook of Research Methods in Psychology. Research Designs: Quantitative, Qualitative, Neuropsychological, and Biological , eds H. Cooper, P. M. Camic, D. L. Long, A. T. Panter, D. Rindskopf, and K. J. Sher (Washington, DC: American Psychological Association), 313–331.
MacKinnon, D. P., Fairchild, A. J., and Fritz, M. S. (2007). Mediation analysis. Annu. Rev. Psychol. 58, 593–614. doi: 10.1146/annurev.psych.58.110405.085542
MacKinnon, D. P., Kisbu-Sakarya, Y., and Gottschall, A. C. (2013). “Developments in mediation analysis,” in The Oxford Handbook of Quantitative Methods: Statistical Analysis , Vol. 2 (New York, NY, US: Oxford University Press), 338–360.
Mannarini, S. (2010). Assessing the Rosenberg Self-esteem Scale dimensionality and items functioning in relation to self-efficacy and attachment styles. TPM Test Psychom. Methodol. Appl. Psychol. 4, 229–242.
Mannarini, S., Boffo, M., Bertucci, V., Andrisani, A., and Ambrosini, G. (2013). A Rasch-based dimension of delivery experience: spontaneous vs. medically assisted conception. J. Clin. Nurs. 22, 2404–2416. doi: 10.1111/jocn.12264
Mannarini, S., Boffo, M., Rossi, A., and Balottin, L. (2018). Etiological beliefs, treatments, stigmatizing attitudes toward schizophrenia. What do Italians and Israelis think? Front. Psychol. 8:2289. doi: 10.3389/fpsyg.2017.02289
Mannarini, S., Balottin, L., Munari, C., and Gatta, M. (2017a). Assessing conflict management in the couple: the definition of a latent dimension. Fam. J. 25, 13–22. doi: 10.1177/1066480716666066
Mannarini, S., Reikher, A., Shani, S., and Shani-Zinovich, I. (2017b). The role of secure attachment, empathic self-efficacy, and stress perception in causal beliefs related to mental illness - a cross-cultural study: Italy versus Israel. Psychol. Res. Behav. Manag. 10, 313–321. doi: 10.2147/PRBM.S138683
Mannarini, S., and Rossi, A. (2019). Assessing mental illness stigma: a complex issue. Front. Psychol. 9:2722. doi: 10.3389/fpsyg.2018.02722
Mannarini, S., Rossi, A., and Munari, C. (2020). How do education and experience with mental illness interact with causal beliefs, eligible treatments and stigmatising attitudes towards schizophrenia? A comparison between mental health professionals, psychology students, relatives and patients. BMC Psychiatry 20:167. doi: 10.1186/s12888-020-02580-6
Marsh, H. W., Balla, J. R., and McDonald, R. P. (1988). Goodness-of-fit indexes in confirmatory factor analysis: the effect of sample size. Psychol. Bull. 103, 391–410. doi: 10.1037/0033-2909.103.3.391
McDonald, R. P., and Ho, M.-H. R. (2002). Principles and practice in reporting structural equation analyses. Psychol. Methods 7, 64–82. doi: 10.1037/1082-989x.7.1.64
McIntyre, R. S., and Lee, Y. (2020). Preventing suicide in the context of the COVID-19 pandemic. World Psychiatry Off. J. World Psychiatr. Assoc. 19, 250–251. doi: 10.1002/wps.20767
Meredith, W. (1993). Measurement invariance, factor analysis and factorial invariance. Psychometrika 58, 525–543. doi: 10.1007/bf02294825
Millsap, R. E. (2012). Statistical Approaches to Measurement Invariance. New York, NY: Routledge.
Moody, E. J. (2001). Internet use and its relationship to loneliness. Cyberpsychol. Behav. 4, 393–401. doi: 10.1089/109493101300210303
Muthén, B., and Asparouhov, T. (2002). Latent variable analysis with categorical outcomes: multiple-group and growth modeling in Mplus. Mplus Web Notes 9, 1–22.
Muthén, L. K. Muthén, B. O (1998-2017). Mplus User’s Guide , 8th Edn. Los Angeles, CA: Muthén & Muthén.
Olmstead, R. E., Guy, S. M., O’Malley, P. M., and Bentler, P. M. (1991). Longitudinal assessment of the relationship between self-esteem, fatalism, loneliness, and substance use. J. Soc. Behav. Pers. 6, 749–770. doi: 10.1017/CBO9781107415324.004
Panzeri, A., Rossi Ferrario, S., and Vidotto, G. (2019). Interventions for psychological health of stroke caregivers: a systematic review. Front. Psychol. 10:2045. doi: 10.3389/fpsyg.2019.02045
Parola, A. (2020). Novel coronavirus outbreak and career development: a narrative approach into the meaning for Italian University Graduates. Front. Psychol . 11:2255. doi: 10.3389/fpsyg.2020.02255
Parola, A., and Donsì, L. (2018). Suspended in time. Inactivity and perceived malaise in NEET young adults. Psicol. Della Salute 3, 44–73. doi: 10.3280/PDS2018-003003
Parola, A., and Donsì, L. (2019). Time perspective and employment status: NEET categories as negative predictor of future. Mediterranean J. Clin. Psychol. 7, 1–20. doi: 10.6092/2282-1619/2019.7.2093
Parola, A., Rossi, A., Tessitore, F., Troisi, G., and Mannarini, S. (2020). Mental health through the COVID-19 quarantine: a growth curve analysis on Italian young adults. Front. Psychol. 11. doi: 10.3389/fpsyg.2020.567484
Peplau, L. A., and Perlman, D. (1982). Loneliness: A Sourcebook of Current Theory, Research, and Therapy. Hoboken, NJ: Wiley.
Podsakoff, P. M., MacKenzie, S. B., Lee, J. Y., and Podsakoff, N. P. (2003). Common method biases in behavioral research: a critical review of the literature and recommended remedies. J. Appl. Psychol. 88, 879–903. doi: 10.1037/0021-9010.88.5.879
Preacher, K. J. (2015). Advances in mediation analysis: a survey and synthesis of new developments. Annu. Rev. Psychol. 66, 825–852.
Prezza, M., Trombaccia, F. R., and Armento, L. (1997). La scala dell’autostima di Rosenberg: Traduzione e validazione Italiana. Florence: Giunti Organizzazioni Speciali.
Pyszczynski, T., Solomon, S., Greenberg, J., Arndt, J., and Schimel, J. (2004). Why do people need self-esteem? A theoretical and empirical review. Psychol. Bull. 130, 435–468. doi: 10.1037/0033-2909.130.3.435
R Core Team (2014). The R Project for Statistical Computing. Vienna: R Foundation for Statistical Computing.
R Core Team (2017). R: A Language and Environment for Statistical Computing. Vienna: R Foundation for Statistical Computing.
Ratti, M. M., Rossi, A., Delli Zotti, G. B., Sarno, L., and Spotti, D. (2017). Social support, psychological distress and depression in hemodialysis patients. Psicol. Della Salute 1, 112–122. doi: 10.3280/PDS2017-001006
Revelle, W. (2018). psych: Procedures for Personality and Psychological Research. Evanston, IL: Northwestern University.
Rico-Uribe, L. A., Caballero, F. F., Martín-María, N., Cabello, M., Ayuso-Mateos, J. L., and Miret, M. (2018). Association of loneliness with all-cause mortality: a meta-analysis. PLoS One 13:e0190033. doi: 10.1371/journal.pone.0190033
Rosenberg, M. (1965). Society and the Adolescent Self-Image. Princeton, NJ: Princeton University Press.
Rosseel, Y. (2012). lavaan: an R package for structural equation modeling. J. Stat. Softw. 48, 1–36. doi: 10.18637/jss.v048.i02
Rosseel, Y., Oberski, D., Byrnes, J., Vanbrabant, L., Savalei, V., Merkle, E., et al. (2015). Package ‘lavaan’.
Rossi, A., and Mannarini, S. (2019). The Italian version of the attitudes toward seeking professional psychological help scale – short form: the first contribution to measurement invariance. TPM Test. Psychometr. Methodol. Appl. Psychol. 26, 93–100. doi: 10.4473/tpm26.1.5
Rossi Ferrario, S., and Panzeri, A. (2020). Exploring illness denial of LVAD patients in cardiac rehabilitation and their caregivers: a preliminary study. Artif. Organs 44, 655–660. doi: 10.1111/aor.13630
Rossi Ferrario, S., Panzeri, A., Anselmi, P., and Vidotto, G. (2019). Development and psychometric properties of a short form of the illness denial questionnaire. Psychol. Res. Behav. Manag. 12, 1–13.
Rucker, D. D., Preacher, K. J., Tormala, Z. L., and Petty, R. E. (2011). Mediation analysis in social psychology: current practices and new recommendations. Soc. Pers. Psychol. Compass 5, 359–371. doi: 10.1111/j.1751-9004.2011.00355.x
Russell, D. W. (1996). UCLA loneliness scale (Version 3): reliability, validity, and factor structure. J. Pers. Assess. 66, 20–40. doi: 10.1207/s15327752jpa6601_2
Santini, Z. I., Nielsen, L., Hinrichsen, C., Meilstrup, C., Madsen, K. R., Koushede, V., et al. (2020). Social disconnectedness, perceived isolation, and symptoms of depression and anxiety among older Americans (NSHAP): a longitudinal mediation analysis. Articles Lancet Public Health 5, E62–E70. doi: 10.1016/S2468-2667(19)30230-0
Sareen, J., Jagdeo, A., Cox, B. J., Clara, I., ten Have, M., Belik, S.-L., et al. (2007). Perceived barriers to mental health service utilization in the United States, Ontario, and the Netherlands. Psychiatr. Serv. 58, 357–364. doi: 10.1176/ps.2007.58.3.357
Schaller, M., Murray, D. R., and Bangerter, A. (2015). Implications of the behavioral immune system for social behavior and human health in the modern world. Philos. Trans. R. Soc. Lond. B Biol. Sci. 370:20140105.
Soraci, P., Ferrari, A., Abbiati, F. A., Del Fante, E., De Pace, R., Urso, A., et al. (2020). Validation and psychometric evaluation of the Italian version of the fear of COVID-19 scale. Int. J. Ment. Health Addict. [Epub ahead of print]. doi: 10.1007/s11469-020-00277-1
Sowislo, J. F., and Orth, U. (2013). Does low self-esteem predict depression and anxiety? A meta-analysis of longitudinal studies. Psychol. Bull. 139, 213–240.
Starr, L. R., and Davila, J. (2012). Responding to anxiety with rumination and hopelessness: mechanism of anxiety-depression symptom co-occurrence? Cogn. Ther. Res. 36, 321–337. doi: 10.1007/s10608-011-9363-1
Steiger, J. H. (1990). Structural model evaluation and modification: an interval estimation approach. Multivariate Behav. Res. 25, 173–180. doi: 10.1207/s15327906mbr2502_4
Steiger, J. H., and Lind, J. C. (1980). “Statistically-based test for the number of common factors,” in Proceedings of the Annual Meeting of the Psychometric Society , Iowa City, IA.
Tabachnick, B. G., and Fidell, L. S. (2014). Using Multivariate Statistics. Harlow: Pearson.
Thakur, V., and Jain, A. (2020). COVID 2019-suicides: a global psychological pandemic. Brain Behav. Immun. 88, 952–953. doi: 10.1016/j.bbi.2020.04.062
Thompson, E. A., Mazza, J. J., Herting, J. R., Randell, B. P., and Eggert, L. L. (2005). The mediating roles of anxiety, depression, and hopelessness on adolescent suicidal behaviors. Suicide Life Threat. Behav. 35, 14–34. doi: 10.1521/suli.35.1.14.59266
Tomarken, A. J., and Waller, N. G. (2005). Structural equation modeling: strengths, limitations, and misconceptions. Annu. Rev. Clin. Psychol. 1, 31–65. doi: 10.1146/annurev.clinpsy.1.102803.144239
Torales, J., O’Higgins, M., Castaldelli-Maia, J. M., and Ventriglio, A. (2020). The outbreak of COVID-19 coronavirus and its impact on global mental health. Int. J. Soc. Psychiatry 66, 317–320. doi: 10.1177/0020764020915212
Usher, K., Bhullar, N., and Jackson, D. (2020). Life in the pandemic: social isolation and mental health. J. Clin. Nurs. 29, 2756–2757. doi: 10.1111/jocn.15290
van de Schoot, R., Lugtig, P., and Hox, J. (2012). A checklist for testing measurement invariance. Eur. J. Dev. Psychol. 9, 486–492. doi: 10.1080/17405629.2012.686740
Vandenberg, R. J., and Lance, C. E. (2000). A review and synthesis of the measurement invariance literature: suggestions, practices, and recommendations for organizational research. Organ. Res. Methods 3, 4–70. doi: 10.1177/109442810031002
VanderWeele, T., and Vansteelandt, S. (2014). Mediation analysis with multiple mediators. Epidemiol. Methods 2:95. doi: 10.1515/em-2012-0010
Vidotto, G., Anselmi, P., Filipponi, L., Tommasi, M., and Saggino, A. (2018). Using overt and covert items in self-report personality tests: susceptibility to faking and identifiability of possible fakers. Front. Psychol. 9:1100. doi: 10.3389/fpsyg.2018.01100
Wang, C., Pan, R., Wan, X., Tan, Y., Xu, L., Ho, C. S., et al. (2020). Immediate psychological responses and associated factors during the initial stage of the 2019 coronavirus disease (COVID-19) epidemic among the general population in China. Int. J. Environ. Res. Public Health 17:1729. doi: 10.3390/ijerph17051729
Weston, R., and Gore, P. A. (2006). A brief guide to structural equation modeling. Counsel. Psychol. 34, 719–751. doi: 10.1177/0011000006286345
Wiedermann, W., and von Eye, A. (2015). Direction of effects in mediation analysis. Psychol. Methods 20, 221–244. doi: 10.1037/met0000027
Wilson, C. J., and Deane, F. P. (2012). Brief report: need for autonomy 554 and other perceived barriers relating to adolescents’ intentions to seek professional mental health care. J. Adolesc. 35, 233–237. doi: 10.1016/j.adolescence.2010.06.011
Wind, T. R., Rijkeboer, M., Andersson, G., and Riper, H. (2020). The COVID-19 pandemic: the ‘black swan’ for mental health care and a turning point for e-health. Internet Intervent. 20:100317. doi: 10.1016/j.invent.2020.100317
World Health Organization [WHO] (2020). WHO Director-General’s opening remarks at the media briefing on COVID-19 - 11 March 2020. Geneva: WHO.
Yang, C., Nay, S., and Hoyle, R. H. (2010). Three approaches to using lengthy ordinal scales in structural equation models:parceling, latent scoring, and shortening scales. Appl. Psychol. Meas. 34, 122–142. doi: 10.1177/0146621609338592
Yao, H., Chen, J.-H., and Xu, Y.-F. (2020). Patients with mental health disorders in the COVID-19 epidemic. Lancet Psychiatry 7:e21. doi: 10.1016/S2215-0366(20)30090-0
Yu, C. Y. (2002). Evaluating Cutoff Criteria of Model Fit Indices for Latent Variable Models with Binary and Continuous Outcomes. Ph.D. thesis, University of California, California.
Zheng, Y. Y., Ma, Y. T., Zhang, J. Y., and Xie, X. (2020). COVID-19 and the cardiovascular system. Nat. Rev. Cardiol. Nat. Res. 17, 259–260. doi: 10.1038/s41569-020-0360-5
Zhou, X., Snoswell, C. L., Harding, L. E., Bambling, M., Edirippulige, S., Bai, X., et al. (2020). The role of telehealth in reducing the mental health burden from COVID-19. Telemed. E Health 26, 377–379. doi: 10.1089/tmj.2020.0068
Keywords : COVID-19, anxiety buffer hypothesis, terror management theory, anxiety, depression, self-esteem, fear, loneliness
Citation: Rossi A, Panzeri A, Pietrabissa G, Manzoni GM, Castelnuovo G and Mannarini S (2020) The Anxiety-Buffer Hypothesis in the Time of COVID-19: When Self-Esteem Protects From the Impact of Loneliness and Fear on Anxiety and Depression. Front. Psychol. 11:2177. doi: 10.3389/fpsyg.2020.02177
Received: 01 June 2020; Accepted: 03 August 2020; Published: 10 November 2020.
Reviewed by:
Copyright © 2020 Rossi, Panzeri, Pietrabissa, Manzoni, Castelnuovo and Mannarini. This is an open-access article distributed under the terms of the Creative Commons Attribution License (CC BY) . The use, distribution or reproduction in other forums is permitted, provided the original author(s) and the copyright owner(s) are credited and that the original publication in this journal is cited, in accordance with accepted academic practice. No use, distribution or reproduction is permitted which does not comply with these terms.
*Correspondence: Alessandro Rossi, [email protected] ; [email protected]
† ORCID: Alessandro Rossi, orcid.org/0000-0001-7000-5999 ; Anna Panzeri, orcid.org/0000-0001-5999-858X ; Giada Pietrabissa, orcid.org/0000-0002-5911-5748 ; Gian Mauro Manzoni, orcid.org/0000-0003-3384-0359 ; Gianluca Castelnuovo, orcid.org/0000-0003-2633-9822 ; Stefania Mannarini, orcid.org/0000-0002-8446-785X
Disclaimer: All claims expressed in this article are solely those of the authors and do not necessarily represent those of their affiliated organizations, or those of the publisher, the editors and the reviewers. Any product that may be evaluated in this article or claim that may be made by its manufacturer is not guaranteed or endorsed by the publisher.
Log in using your username and password
- Search More Search for this keyword Advanced search
- Latest content
- Supplements
- BMJ Journals More You are viewing from: Google Indexer
You are here
- Volume 5, Issue 6
- Hypothesis to explain the severe form of COVID-19 in Northern Italy
- Article Text
- Article info
- Citation Tools
- Rapid Responses
- Article metrics

- http://orcid.org/0000-0002-6285-7355 Luca Cegolon 1 , 2 ,
- Jennifer Pichierri 3 ,
- Giuseppe Mastrangelo 4 ,
- Sandro Cinquetti 1 ,
- Giovanni Sotgiu 5 ,
- Saverio Bellizzi 6 ,
- Giuseppe Pichierri 7
- 1 Public Health Department , Local Health Unit N. 2 "Marca Trevigiana" , Treviso , Veneto Region , Italy
- 2 IRCCS Materno Infantile Burlo Garofolo , Trieste , Friuli-Venezia Giulia Region , Italy
- 3 Children and Young People’s Diabetes , University College London Hospitals NHS Foundation Trust , London , UK
- 4 Cardiac, Thoracic and Vascular Sciences , Padua University Hospital , Padua , Veneto Region , Italy
- 5 Department of Medical, Surgical and Experimental Sciences , University of Sassari , Sassari , Sardinia Region , Italy
- 6 Partnership for Maternal, Newborn and Child Health , World Health Organization , Geneve , Switzerland
- 7 Microbiology Department , Kingston Hospital NHS Foundation Trust , Kingston upon Thames , London , UK
- Correspondence to Dr Luca Cegolon; l.cegolon{at}gmail.com
https://doi.org/10.1136/bmjgh-2020-002564
Statistics from Altmetric.com
Request permissions.
If you wish to reuse any or all of this article please use the link below which will take you to the Copyright Clearance Center’s RightsLink service. You will be able to get a quick price and instant permission to reuse the content in many different ways.
- public health
- prevention strategies
- epidemiology
Summary box
What is already known about this subject.
Human coronaviruses are known to cause respiratory re-infections, regardless of pre-existing humoural immunity.
There is evidence suggesting that severe acute respiratory syndrome coronavirus type 2 (SARS-CoV-2) had been circulating in Italy before the first COVID-19 case was detected in the country.
What are the new findings?
Prior infections with SARS-CoV-2 (or other viruses/coronaviruses) may arguably predispose to more severe forms of the disease following re-infection with SARS-CoV-2, with an immunological mechanism known as Antibody-Dependent-Enhancement, already observed with infections sustained by other coronaviruses (MERS-CoV and SARS-CoV) or other viruses such as the West Nile Virus and Dengue.
What are the recommendations for policy and practice?
If confirmed by in vivo studies, this hypothesis may have relevant implications for the treatment of severe forms of COVID-19, yet the possibility to produce an effective vaccine against SARS-CoV-2 might be hampered.
The ongoing COVID-19 pandemic, caused by the novel severe acute respiratory syndrome coronavirus type 2 (SARS-CoV-2), has affected 212 countries worldwide at various degrees as of 8 May 2020. 1
In this paper we discuss a hypothesis that prior viral infections—either by SARS-CoV-2 or different strains of coronaviruses, or potentially even other respiratory viruses—may predispose to more severe forms of COVID-19, following a secondary infection with SARS-CoV-2.
Most COVID-19 infections are asymptomatic or manifest with mild to moderate respiratory symptoms (fever, cough, sore throat, myalgia, fatigue and even non-severe pneumonia). Of patients with COVID-19, 14%–15% develop severe pneumonia and 5%–6% a critical condition requiring admission to intensive care unit (ICU). 2–4 Death may eventually occur after an average of 17.8 days since the onset of symptoms. 5
Among all countries, Italy (which was the first European COVID-19 cluster) presents a critical disease pattern as of 8 May 2020, having the third highest number of COVID-19 cases in the world after the USA and Spain, the fourth highest prevalence of the disease after Spain, Belgium and the USA, the third highest total number of deaths attributed to COVID-19 after the USA and the UK, and the third highest prevalence of COVID-19 mortality after Belgium and Spain, despite a current 1% rate of severe/critical disease among active cases, which has been progressively decreasing over time. 1
The cross-country discrepancies in the burden of COVID-19 observed so far across the globe cannot be explained only by differences in population age structures. 6–8 In fact, Japan has a population double that of Italy, with the proportion of subjects older than 65 being 28.8% in Japan vs 21.7% in Italy. 9 10 Nonetheless, as of 8 May 2020, the difference in COVID-19 prevalence between Japan (122 per million) and Italy (3570 per million) is massive. 1 Likewise, in Germany the percentage of individuals >65 is reportedly 22.1% (hence slightly higher than Italy), but the prevalence of COVID-19 is currently 2022 per million. 1 11 In Iran the proportion of people >65 is 5.5% (hence much younger than the Italian, German and Japanese populations), but the prevalence of COVID-19 is 1246 per million, as of 8 May 2020. 1 12
The mortality rate for COVID-19 is reportedly enhanced by 5.6%–10.5% in the presence of any comorbidities (hypertension, diabetes, cardiovascular diseases, cancer and/or chronic respiratory conditions) and becomes significantly and progressively higher after 50 years of age, 4 6 although the severe form of the disease increases linearly at any age stage. 5 Cold dry weather is a recognised risk factor for respiratory infections, rendering viruses as influenza more stable and individuals more susceptible. 13 14 This applies also to SARS-CoV-2, the viability and transmissibility of which reportedly reduce with hot and humid weather conditions. 14 Moreover, unfavourable disease progression and clinical outcomes of COVID-19 were found to be associated with cigarette smoking in a systematic review. 15
A number of factors may have contributed to enhancing the risk of infection with SARS-CoV-2 in Northern Italy. The age by which half of all young people leave parental home is higher in Italy than other European Union countries, 16 and such multigenerational cohabitation probably contributed to increase COVID-19 contagion among elderly individuals. The universal use of face masks was initially discouraged in Italy in order to preserve the limited supplies of personal protective equipment for professional use in healthcare settings; another argument initially was that face masks are ineffective in protecting against coronavirus infections. 17 Further major findings of the relevant literature have been summarised in figure 1 . An extraordinary elevated incidence of COVID-19 could have been the result of a perfect storm triggered by multiple interplaying factors. The affected areas in Northern Italy (regions of Lombardy, Emilia-Romagna, Piedmont and Veneto) are characterised by high population density 18 and recognised air pollution, 19 20 especially from fine particulate matter (PM2.5), which was found to increase the risk of death from COVID-19 in the USA. 21 Northern Italy includes several cities which, similarly to Philadelphia (USA) during the Spanish flu pandemic in 1918, 22 are historically important and densely populated, where social gatherings as well as business activities are certainly fundamental—the latter being vital to the economy of the entire country. These cultural and social dynamics might have influenced the initial resistance and reluctance of the general population to comply with the social restrictions progressively enforced by the Italian government (until full lockdown was established on 21 March). Moreover, the intense case finding in Italy was preceded by an initial overall underestimation of the COVID-19 threat by the Italian government and subsequently by the general population, who perceived the disease as just some sort of influenza, despite worrying news from the first affected country (China). 23 Thereafter SARS-CoV-2 was also going to spread to other European countries, which have also now been heavily affected by the disease. 1
- Download figure
- Open in new tab
- Download powerpoint
Conceptual framework explaining the relationships between various factors and incidence and severe/critical form of COVID-19. Established items: orange boxes; hypothetical items: blue boxes. ADE, antibody-dependent enhancement; ARDS, acute respiratory distress syndrome; COVID-19, coronavirus infectious disease 2019; IPC, infection prevention and control; SARS-CoV-2, severe acute respiratory syndrome coronavirus type.
The epidemiological investigations conducted by the Italian National Institute of Health ( Istituto Superiore di Sanità ) suggest that the vast majority of cases but the first three acquired the infection in Italy. It can therefore be reasonably argued that SARS-CoV-2 had been circulating in the country—especially in Lombardy, Emilia-Romagna, Piedmont and Veneto regions—for weeks before the first patient was found. 24 Human coronaviruses are known to cause respiratory reinfections regardless of pre-existing humoural immunity, both at the individual and community level. 25 At the same time, presumed hospital-associated transmission of SARS-CoV-2 has been reported since the initial stages of the COVID-19 outbreak in Wuhan (China) in 41% of the total number of patients, 70% of whom were healthcare staff. 26 This could have also occurred in Italy, where healthcare workers reportedly make up 9% out of all COVID-19 cases. 27 We therefore hypothesise (see blue boxes in figure 1 ) that repeated cycles of infection within a community (especially in older adults)—or even more worryingly in a healthcare setting—could have the potential to cause more severe forms of COVID-19, with acute respiratory distress syndrome (ARDS), the fundamental pathophysiology of severe viral pneumonia due to COVID-19, requiring admission to ICU. 28 ARDS associated with COVID-19 shares clinical features with the critical form of the 2003 severe acute respiratory syndrome coronavirus (SARS-CoV) epidemic, in particular lymphopaenia, massive infiltration of phagocytes and inflammation sustained by cytokines. 4 8 29
A plausible mechanistic hypothesis could be the antibody-dependent enhancement (ADE), sustained by prior exposure to SARS-CoV-2 or other viruses/coronaviruses. 8 Previous circulation and exposure to other coronaviruses similar to SARS-CoV-2 causing mild/asymptomatic cold-like symptoms shall in fact not be ruled out. 8 Binding and neutralising antibody response against other types of human coronaviruses was recently reported to increase with age in adult patients, 25 and this may explain the increased linear risk of severe COVID-19 with age, with death being significantly higher in patients older than 50. 5
Non-neutralising, subneutralising or even fully neutralising antibodies may play a key role in ADE. 30 Wan et al 30 have recently described a molecular mechanism for ADE involving the Middle East respiratory syndrome coronavirus (MERS-CoV), similar to what is already known for SARS-CoV and flaviviruses as Dengue and the West Nile virus. 31–35 While the entry of SARS-CoV into the phagocytes occurred principally through the human ACE2 receptor, the ADE mechanism was shown to be enhanced by antibodies specific for the spike (S) envelope glycoprotein binding with the macrophage receptor and subsequent enhancement of target cell infections. 33–35 Likewise, the antibody/opsonised SARS-CoV-2 particles may bind avidly with the IgFc receptors of target cells, increasing the virus yield as well as the production of cytokines. This may also explain the higher risk of thromboembolism reportedly associated with severe/critical COVID-19. 4 36 37
Anecdotal clinical reports of ‘biphasic infection’ and ‘cytokine storm’ seem to possibly point towards this direction, biphasic infection simply being the immunological result of a secondary infection by other coronaviruses or a reinfection due to SARS-CoV-2. 38–41 An early elevation of serum proinflammatory cytokines, suggesting a pathological mechanism mediated by cytokine storm, has been observed with both severe forms of SARS-CoV and MERS-CoV infections. The latter two viruses share a genomic similarity of about 79% and 50% with SARS-CoV-2, respectively. 41 42 Several Rhinolophus bats-related coronavirus strains have been found to share even higher sequence homology with SARS-CoV-2. 33 An abnormal humoural response due to ADE, in the early stages of a secondary infection by SARS-CoV-2, may delay the innate antiviral immune response relying on the production of type 1 interferon (IFN-1). This would compromise the initial antiviral response of the host, with subsequent elevated influx of proinflammatory cytokines, hyperinflammatory neutrophils and monocytes-macrophages and hypercoagulable state accountable for ARDS and typical pneumonia observed in patients affected by severe/critical COVID-19 ( figure 1 ). 4 41 43 44
If confirmed, this hypothesis would have relevant implications for the treatment of COVID-19 and the development of an effective vaccine. The licensing of a vaccine against human coronaviruses has failed thus far, partly because immunised individuals could potentially be at higher risk of ADE sustained by facilitated uptake of viral antigen-antibody complexes by target cells. 4 31 33 44 The approval of a vaccine against SARS-CoV-2 may encounter similar obstacles. Likewise, herd immunity would not be a possibility with COVID-19. WHO recommends passive immunotherapy when vaccine and antivirals are not available for emerging infections. 45 In a preliminary uncontrolled case study on five critically ill patients with COVID-19 who developed ARDS, the administration of convalescent plasma—drawn from five patients who recovered from COVID-19 and containing SARS-CoV-2-specific neutralising antibodies (IgG)—between 10 and 22 days since admission significantly improved the clinical status of all, resolving ARDS in four of them within 12 days since transfusion. 46 On the other hand, the treatment of severe COVID-19 may also benefit from monoclonal antibodies targeting proinflammatory cytokines 4 as well as supplements of IFN-1 in combination with other antiviral drugs. 47–50
Whether secondary infections from other coronaviruses or repeated community reinfections of SARS-CoV-2 may account for more severe presentations of COVID-19 observed in some countries compared with others, 8 and whether it is only a matter of time for the virus to circulate and infect a significant proportion of the population before causing reinfections and therefore more severe clinical features, are something which will require more indepth epidemiological and immunological/serological investigations. A better understanding of any underlying immunological mechanism or any additional risk factor which could explain cross-country differences in the rates of severe disease and mortality attributable to COVID-19 will help to guide international public health responses during this ongoing pandemic. It will be important to clarify if the ARDS mechanism responsible for the severe respiratory infection could potentially be attributable to ADE.
Two different in vivo strategies could be employed to endorse this hypothesis.
First (observational design), all healthcare workers and blood donors should undergo serum test for COVID-19. Individuals presenting SARS-CoV-2 IgG antibodies should be included in a local/regional/national ad-hoc register and monitored over time for the possible development of severe disease sustained by ADE, which would need to be confirmed by blood count, dosage of IFN and proinflammatory cytokines, in addition to chest CT scan. The risk of developing severe COVID-19 should be estimated and stratified by relevant risk factors, including baseline serum level of SARS-CoV-2-specific IgG antibodies, age, sex, potential occupational exposure, medical history (particularly previous infections and vaccination status), any comorbidities, area of residence and health status of household members, among others.
Second (experimental laboratory design), animal models (hamsters, rodents, palm civets, monkeys, ferrets) 33 51 52 could be infected by SARS-CoV-2 (or other viruses/coronaviruses) and subsequently re-exposed to SARS-CoV-2 to verify the possibility of onset of ARDS sustained by ADE.
- Worldometers
- World Health Organization
- Chan JF-W ,
- Kok K-H , et al
- Dorigatti I , et al
- Index Mundi
- Feng K , et al
- Vardavas CI ,
- Iacovou M ,
- Xia N , et al
- Office for National Statistics (ISTAT)
- Ghermandi G ,
- Harrison RM
- Liang S , et al
- Nethery RC ,
- Sabath BM , et al
- Hannigan T ,
- Steele C , et al
- Start Magazine
- National Institute of Health (ISS)
- Donovan MM ,
- Hu C , et al
- International Council of Nurses
- Xu J , et al
- Cheung CY ,
- Ng IHY , et al
- Sun S , et al
- Gollins SW ,
- Porterfield JS
- Urbina AN ,
- Chang MR , et al
- Yang Z-yong ,
- Werner HC ,
- Kong W-pui , et al
- Tseng S-P ,
- Yen C-H , et al
- Tirado SMC ,
- Zhao L , et al
- Thachil J ,
- Iba T , et al
- McAuley DF ,
- Brown M , et al
- Mahallawi WH ,
- Khabour OF ,
- Zhang Q , et al
- Wu AKL , et al
- Prompetchara E ,
- Li J , et al
- Pedersen SF ,
- Corapi WV ,
- Ngichabe CK , et al
- Zhao F , et al
- Sallard E ,
- Lescure F-X ,
- Yazdanpanah Y , et al
- Chen Q , et al
- Loutfy MR ,
- Siminovitch KA , et al
- Omrani AS ,
- Baig K , et al
- Cheung CY , et al
- Roberts A , et al
Handling editor Seye Abimbola
Contributors LC, JP, SB, GP and GM equally contributed to conceiving the idea and drafting the manuscript. SC and GS contributed to drafting the manuscript.
Funding The authors have not declared a specific grant for this research from any funding agency in the public, commercial or not-for-profit sectors.
Competing interests None declared.
Patient consent for publication Not required.
Provenance and peer review Not commissioned; externally peer reviewed.
Data availability statement There are no data in this work.
Read the full text or download the PDF:
Masks Strongly Recommended but Not Required in Maryland, Starting Immediately
Due to the downward trend in respiratory viruses in Maryland, masking is no longer required but remains strongly recommended in Johns Hopkins Medicine clinical locations in Maryland. Read more .
- Vaccines
- Masking Guidelines
- Visitor Guidelines
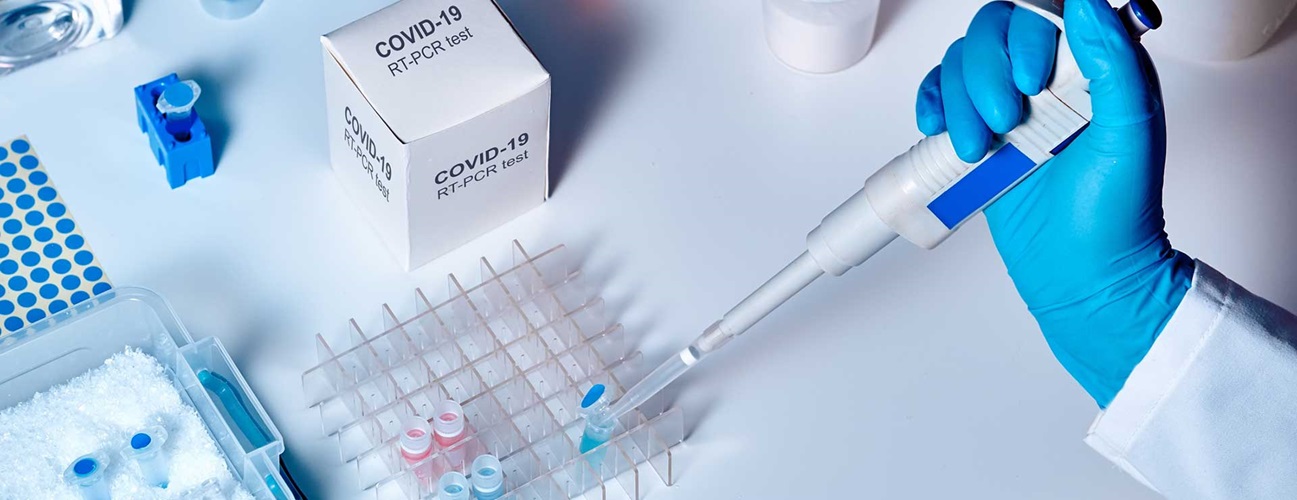
COVID Test FAQs
Reviewed By:

Lisa Maragakis, M.D., M.P.H.
Testing is an important tool in helping to limit the spread of COVID-19. Lisa Maragakis, M.D., M.P.H. , senior director of infection prevention, answers common questions about testing.
What are the types of coronavirus tests?
There are two basic types of tests for COVID-19.
Viral test: This test can tell you if you are currently infected with the coronavirus. A swab is placed in the nose or mouth to gather samples. There are two types of viral tests: laboratory tests (sometimes called polymerase chain reaction [PCR] tests), and rapid tests that detect portions of the virus called antigens.
Laboratory coronavirus tests are given by health care professionals, and results may take a day or more to receive. Since the samples are analyzed in a laboratory, they are very accurate and are often recommended for people with COVID-19 symptoms. Laboratory tests sometimes require an additional test to confirm results.
Due to the rapid spread of COVID-19 in the community, Johns Hopkins Medicine is only offering COVID-19 testing under certain conditions. Review our COVID-19 testing information to learn more.
Rapid antigen tests may be performed in pharmacies or health care facilities, or as self-tests at home. Rapid tests provide results in about 15 minutes. These tests are recommended if you do not have symptoms or if you are taking the test as a risk-prevention measure, such as before going on a work trip or seeing a friend, and you want to be sure you don’t have the coronavirus that causes COVID-19.
Antibody test: This is a blood test that can show if you were previously exposed to or infected with the virus that causes COVID-19, and if your body has created antibodies in an attempt to defend itself. It takes at least 12 days after exposure for your body to make enough antibodies to show up on a test. Antibody tests are not recommended to determine if you currently have COVID-19.
This test helps scientists gather data about how the immune system fights off COVID-19 in recovered patients. A positive antibody test means the person has some level of immune protection either from prior infection or from vaccination, but it is not a guarantee of protection from getting re-infected with the virus. Antibody levels decrease over time, and it is not clear how long antibody protection lasts.
Should I get a coronavirus test?
The answer varies based on many factors including your symptoms, exposure history and underlying risk factors for severe disease.
A rapid test is useful as a preventive measure if you are traveling or if you do not have symptoms but think you may have been exposed to the coronavirus that causes COVID-19. Other important prevention tools are: getting fully vaccinated and receiving a booster when eligible, wearing a mask, maintaining physical distance and frequently washing your hands.
A viral test is important when a person has COVID-19 symptoms, particularly if they are moderate to severe. A viral test may also be helpful for a person with mild symptoms. People with any symptoms should contact their doctor and review Centers for Disease Control and Prevention (CDC) guidelines for isolation and quarantine .
Where can I get a coronavirus test?
Demand for tests is currently high, so you may need to check several resources. Testing sites may include your doctor’s office, a local urgent care facility or a testing location in your area. Maryland.gov provides a list of test locations . You may also be able to get self-tests at selected pharmacies or health care centers.
Due to the high demand, Johns Hopkins Medicine only offers COVID-19 testing under certain conditions. Review our COVID-19 testing information to learn more. We urge people not to go to emergency rooms for routine COVID testing, so that Emergency Department staff are available to prioritize care for patients with urgent or emerging medical needs.
How long do COVID test results take?
Laboratory analysis of viral tests (sometimes called PCR tests) can take a day or more. Rapid test results are available in about 15 minutes. Antibody test results may take a day or longer to obtain.
If I’ve been tested once, do I need to get tested again?
A negative COVID-19 test result reflects only one point in time, so it may be necessary to test more than once if you develop symptoms or were potentially exposed to the virus. If you are tested during the earliest stages of infection, before the virus is detectable, you might be infected despite having a negative test result. And after receiving a negative test result, it is possible to catch the coronavirus later and become infected.
If you are exposed to someone with COVID-19, you should quarantine, watch for symptoms and consider getting tested on day four or five following the exposure. Please review CDC guidelines for isolation and quarantine .
If you have symptoms, let your health care provider know if they persist or worsen. You might need to be tested again.
Coronavirus (COVID-19)
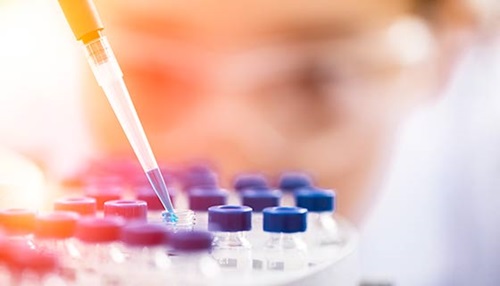
What you need to know from Johns Hopkins Medicine.
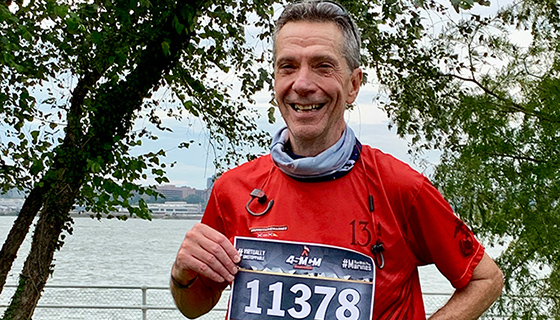
Trauma Team Puts an Athlete Back in the Saddle
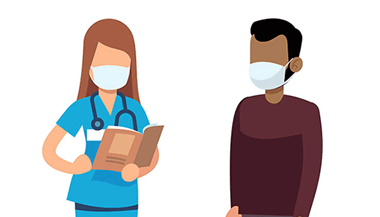
Patient Safety Infographic
Coronavirus: Younger Adults Are at Risk, Too
Related Topics
Former NIH Director admits lab-leak theory of COVID origin is not conspiracy theory
T he leading public health official during the early stages of the COVID-19 pandemic admitted that the science is not settled on the origins of the coronavirus, according to transcripts from closed-door testimony released by House Republicans on Thursday.
The released transcripts reveal that former National Institutes of Health Director Francis Collins does not believe that the idea that the virus originated in a lab is a conspiracy theory, despite publicly denouncing the theory in 2020 and 2021.
Collins, who was the longest-serving NIH director, in office from 2009 to December 2021, was called before the Select Subcommittee on the Coronavirus Pandemic to provide transcribed interview testimony in January 2024 to explain his role in the scientific inquiry into the origins of the COVID-19 pandemic. Republicans have accused him and other public health officials of chilling debate.
Public health officials at the NIH during the initial stages of the pandemic in 2020 and 2021 largely downplayed the theory that the virus may have originated from an incident at the Wuhan Institute of Virology in China where scientists were conducting coronavirus research with U.S. taxpayer funding through a subgrant administered by the research firm EcoHealth Alliance .
Republicans on the Subcommittee directly asked Collins in the interview this year whether the coronavirus virus could have resulted from “a laboratory or research-related accident, a researcher doing something in the lab, getting infected with the virus, and then sparking the pandemic.”
When asked whether or not this scenario was a conspiracy theory, Collins responded “not at this point.”
Collins also responded in the affirmative when asked by Republicans if “the origin of COVID-19 still unsettled science.”
Regardless of whether the virus originated in the Wuhan lab or in a live-animal wet market, Collins told the Subcommittee, it is in the Chinese government’s best interest “for this to be unresolved.”
“If it was a lab leak, they're responsible,” said Collins. “If it was a natural origin in a wet market that was selling wild animals that they were not supposed to be doing, they're responsible. So they love it that this hasn't gotten resolved.”
Other public health officials at NIH have also walked back early denouncement of the lab-leak theory of the origin of the coronavirus.
The Select Subcommittee held a hearing on Thursday with testimony from Lawrence Tabak , who served as acting director of NIH following Collins’s resignation, who agreed that the lab-leak theory of origin is not necessarily a conspiracy theory.
When asked by Rep. Nicole Malliotakis (R-NY) whether or not he believed the lab leak hypothesis was a conspiracy theory, Tabak replied that it is “just an alternate theory that needs to be considered.”
CLICK HERE TO READ MORE FROM THE WASHINGTON EXAMINER
“While NIH is not an investigative agency, we do support scientific research into the origins of SARS-CoV-2 and will continue to make this a priority,” said Tabak in his opening testimony. “We are open to all possibilities and will follow where the science leads us.”
Tabak told the Subcommittee that the NIH still maintains that the virus likely originated from a natural zoonotic spillover from the wet market in Wuhan because the virus is too genetically removed from the known samples of bat coronaviruses tested at the WIV under the NIH grant administered by EcoHealth Alliance.

An official website of the United States government
The .gov means it’s official. Federal government websites often end in .gov or .mil. Before sharing sensitive information, make sure you’re on a federal government site.
The site is secure. The https:// ensures that you are connecting to the official website and that any information you provide is encrypted and transmitted securely.
- Publications
- Account settings
Preview improvements coming to the PMC website in October 2024. Learn More or Try it out now .
- Advanced Search
- Journal List
- BMJ - PMC COVID-19 Collection

Side effects of COVID-19 vaccines: a systematic review and meta-analysis protocol of randomised trials
Kleyton santos medeiros.
1 Health Sciences Postgraduate Program, Federal University of Rio Grande do Norte, Natal, Rio Grande do Norte, Brazil
2 Instituto de Ensino, Pesquisa e Inovação, Liga Contra o Câncer, Natal, Rio Grande do Norte, Brazil
Ana Paula Ferreira Costa
Ayane cristine alves sarmento, cijara leonice freitas, ana katherine gonçalves.
3 Department of Obstetrics and Gynecology, Universidade Federal do Rio Grande do Norte, Natal, Brazil
Associated Data
Introduction.
SARS-CoV-2 is responsible for a large number of global COVID-19 cases. Strategies such as social isolation, personal hygiene and frequent hand washing have been implemented; however, a protective vaccine is required to achieve sufficient herd immunity to SARS-CoV-2 infection to ultimately control the COVID-19 pandemic. To meet the urgent need for a vaccine, a reduction in the development schedule has been proposed from 10–15 years to 1–2 years. For this reason, this systematic review and meta-analysis protocol aims to compare the side effects, safety and toxicity of COVID-19 vaccines available globally, including their combinations.
Methods and analysis
We will select randomised controlled trial-type studies that evaluate the side effects of the COVID-19 vaccine. PubMed, Web of Science, Embase, CINAHL, PsycINFO, LILACS, SCOPUS, ClinicalTrials.gov, International Clinical Trials Registry Platform (ICTRP), medRxiv.org, biorxiv.org, preprints.org and the Cochrane Library will be searched for eligible studies until December 2021. Three reviewers will independently screen and select studies, assess methodological quality and extract data. A meta-analysis will be performed, if possible, and the Grading of Recommendations, Assessment, Development and Evaluations summary of findings will be presented.
Ethics and dissemination
This study will review published data, and thus it is unnecessary to obtain ethical approval. The findings of this systematic review will be published in a peer-reviewed journal.
PROSPERO registration number
CRD42021231101.
Strengths and limitations of this study
- Four authors (KSM, APFC, ACAS, CLF) will select the articles independently using titles and abstracts.
- To the best of our knowledge, there are no existing reviews regarding the side effects of COVID-19 vaccines.
- The DerSimonian and Laird method may underestimate the true between-study variance, potentially producing overly narrow CIs for the mean effect. This fact is a limitation, so the collection of studies will be done with care and the assumptions of the analytical methods will be assessed.
SARS-CoV-2 is responsible for a large number of global COVID-19 cases. It is a highly transmissible virus among humans that has become a significant public health issue. 1 Symptoms include fever, dry cough, fatigue, shortness of breath, chills, muscle pain, headache, gastric disorders and weight loss, often leading to death. 2
Strategies such as social isolation, personal hygiene and frequent hand washing have been implemented; however, a protective vaccine is required to achieve sufficient herd immunity to SARS-CoV-2 infection to ultimately control the COVID-19 pandemic. 3 To meet the urgent need for a vaccine, a reduction in the development schedule has been proposed from 10–15 years to 1–2 years. 4
SARS-CoV-2 is an RNA virus with a high mutation rate, and that on the envelope surface has three important structural proteins that can be identified: spike protein (S), envelope protein (E) and membrane protein (M). Most innovative vaccines have focused their efforts on inducing an immune response against the S protein. Attenuated virus vaccines are based on weakened microorganisms, effective in stimulating the immune system. The inactivated ones (dead microorganisms) are more stable than the attenuated ones, but they have a short duration of immunological memory that requires the association of adjuvants. mRNA vaccines are stable—and can be easily produced in large quantities. Vaccines against COVID-19 differ in composition and mechanism of action, which may be relevant for their safety and efficacy, being essential for the success and eradication of this infection. 5 6 The viral vector (mRNA) vaccine encodes full-length S protein ectodomains of SARS-CoV-2, which contains both T and B cell epitopes that can induce cellular and humoral immune responses against viral infection. 7
Assessing the safety, efficacy and side effects of the vaccine is urgently needed, and has been heavily scrutinised by the leading medical agencies around the world, like the Centers for Disease Control and Prevention and the Food and Drug Administration. Developing any vaccine needs to ensure that safety risks are identified and quantified against potential benefits. Among the potential risks raised in the context of COVID-19, vaccine development is the security and effectiveness of immune responses elicited by a vaccine. Here, this systematic review protocol aims to assess the side effects, safety and toxicity of vaccines against COVID-19.
This systematic review and meta-analysis protocol aims to compare the side effects, safety and toxicity of COVID-19 vaccines available globally, including their combination.
Review question
What are the rates of adverse reactions (local and systemic) to COVID-19 vaccines?
The meta-analysis protocol follows the Preferred Reporting Items for Systematic Review and Meta-Analysis Protocols guidelines. 8 9 This protocol is registered with the International Prospective Register of Systematic Reviews (PROSPERO).
Eligibility criteria
The inclusion criteria involved: (1) randomised controlled trial (RCT)-type studies that evaluated the side effects of the COVID-19 vaccine; (2) experiments involving human beings; (3) studies evaluating the safety, immunogenicity and efficacy parameters of the vaccines; (4) studies that presented similar vaccination protocols; (5) studies published since January 2020 until December 2021; and (6) studies published in any language.
The exclusion criteria were as follows: (1) observational studies, and (2) case reports, meeting abstracts, review papers and commentaries.
Patients, intervention, comparison, outcome strategy and types of studies
- Patients: healthy adults aged 18 years or older who were HIV negative and previously SARS-CoV-2 infection free.
- Intervention: COVID-19 vaccine or a combination of vaccines against COVID-19.
- Comparator/control: placebo.
- Outcome: safety, tolerability and immunogenicity of the COVID-19 vaccine or the combination of vaccines against COVID-19.
- Types of studies: RCTs.
Information sources
The following databases will be searched: Medline / PubMed, Web of Science, Embase, CINAHL, PsycINFO, Latin American and Caribbean Health Sciences Literature (LILACS), SCOPUS, ClinicalTrials.gov, International Clinical Trials Registry Platform (ICTRP), medRxiv.org, biorxiv.org, preprints.org and Cochrane Central Controlled Trials Registry. Furthermore, eligible studies may also be selected from the reference lists of retrieved articles.
Patient and public involvement
The individual patient data will not be presented. A literature search will be carried out from defined databases. No patient will be involved in the study planning and application process during neither the analysis nor the dissemination of results.
Search strategy
Our keyword search will be based on Medical Subject Headings according to the following combination: (COVID-19 OR SARS-CoV-2 OR 2019-nCoV OR coronavirus) AND (vaccines OR vaccination OR COVID-19 vaccine OR SARS-CoV-2 vaccine OR BNT162 vaccine OR mRNA-1273 vaccine OR COVID-19 aAPC vaccine OR INO-4800 vaccine OR LV-SMENP-DC COVID-19 vaccine OR Ad5-nCoV vaccine OR ChAdOx1 COVID-19 vaccine OR MNA SARS-CoV-2 S1 subunit vaccines OR PittCoVacc OR Inactivated novel coronavirus 2019-CoV vaccine Vero cells OR Inactivated Vaccines OR SARS-CoV-2 inactivated vaccines OR Viral Vaccines OR Gam-COVID-Vac vaccine OR Ad26.COV2.S vaccine OR EpiVacCorona vaccine) AND (Toxicity OR Vaccine Immunogenicity OR side effects OR adverse events) AND (randomized controlled trial OR double blind method OR clinical trial) ( table 1 ). A list of vaccines available at WHO was also used.
Medline search strategy
Study records
Four researchers (KSM, APFC, ACAS, CLF) performed the selection of the studies of interest. Titles and abstracts will be read independently, and duplicate studies will be excluded. The same authors analysed the selected texts to assess the compliance with the inclusion criteria. A fifth reviewer, AKG, solves the discrepancies. The flow chart of this study is shown in figure 1 .

Flow diagram of the search for eligible studies on the side effects, safety and toxicity of the COVID-19 vaccine. CENTRAL, Cochrane Central Register of Controlled Trials.
Data collection process and management
A standardised data extraction form was developed and tested. Data from each included study will be extracted independently by two reviewers (ACAS and APFC), and any subsequent discrepancies will be resolved through discussion with a third reviewer (AKG). The data extracted will include information on authors, the year of publication, study location, type of study, main objectives, population, type of vaccine, follow-up of participants, rates of systemic events, gastrointestinal symptoms, injection site-related adverse effects and serious vaccine-related adverse events ( table 2 ). Furthermore, participant characteristics (eg, mean age, gender) and results for immunogenicity will be collected.
Adverse events of COVID-19 vaccines
The study authors will be contacted in case of missing data and/or to resolve any uncertainties. In addition, any additional information will be recorded. All data entries will be checked twice. If we find a set of articles with similar characteristics based on the information in the data extraction table, we will perform a meta-analysis using a random-effects model. If there are data that are not clear in some articles, the corresponding author will be contacted for possible clarification.
Risk of bias in individual studies
Three authors (KSM, ACAS, APFC) will independently assess the risk of bias in the eligible studies using the Cochrane risk-of-bias tool. 10 The Risk of Bias 2 tool 11 will be used to assess the risk of bias. Bias is assessed as a judgement (high, low or unclear) for individual elements from five domains (selection, performance, attrition, reporting and others).
Data will be entered into the Review Manager software (RevMan V.5.2.3). This software allows the user to enter protocols; complete reviews; include text, characteristics of the studies, comparison tables and study data; and perform meta-analyses. For dichotomous outcomes, we extracted or calculated the OR and 95% CI for each study. In case of heterogeneity (I 2 ≥50%), the random-effects model will be used to combine the studies to calculate the OR and 95% CI using the DerSimonian-Laird algorithm 12 .
Data synthesis and analysis
To grade the strength of evidence from the included data, we will use the Grading of Recommendations, Assessment, Development and Evaluation 13 approach. The summary of the assessment will be incorporated into broader measurements to ensure the judgement of the risk of bias, consistency, directness and precision. The quality of the evidence will be assessed based on the risk of bias, indirectness, inconsistency, imprecision and publication bias.
The COVID-19 pandemic represents one of the most significant global public health crises of this generation. Lockdown, quarantine, contact tracing and case isolation are suggested as effective interventions to control the epidemic; however, they may present different results in different contexts because of the specific features of the COVID-19. The lack of implementation of continued interventions or effective treatments further contributes to discovering and using effective and safe vaccines. 14 15
For all these reasons, scientists worldwide entered a race to find a vaccine candidate useful in fighting the new coronavirus pandemic. Nevertheless, it is essential to note that a vaccine’s production is not easy and quick. Before being released to the population, a vaccine must go through three phases of clinical trials that prove its safety and effectiveness. More volunteers are recruited at each stage, and the researchers analyse the test results to ensure that a vaccine can be licensed. 16–18
One hundred and seventy-three vaccines were in preclinical development and 64 in clinical trials until 20 January 2021. On 31 December 2020, the WHO listed the mRNA vaccine against COVID-19 for emergency use, making this Pfizer/BioNTech immuniser the first to receive WHO emergency validation from the beginning outbreak. Already, in January 2021, emergency approval was granted to nine vaccines by regulatory authorities in different parts of the world. 14 19
With the starting vaccination, several studies were carried out to ascertain the safety of these vaccines, since they were produced in record time. 20–22 Currently, one systematic review about the thematic showed that of 11 published clinical trials of COVID-19 vaccines included in the study, adverse reactions reported were considered mild to moderate with few severe reactions which were unrelated to the test vaccine. Common adverse events were pain at the site of injection, fever, myalgia, fatigue and headache. Serious adverse events (SAE) were reported in four trials: COVID-19 Vaccine AstraZeneca (AZD1222)—168 SAEs with only three related to the vaccine; Ad26.COV2.S—four with none related to the testing vaccine; five with Comirnaty (BNT162b1) vaccine and one with Covaxin (BBV152) vaccine. 19
One limitation about the COVID-19 vaccine safety tested until now is that clinical trials of the safety and effectiveness have had low inclusion of vulnerable groups, for example, older persons, the first population to receive the whole vaccine. That’s why pharmacovigilance postmarketing is necessary to surveillance of new drugs, as a critical aspect of evaluating medicine safety and effectiveness, particularly in risk groups.
Other prevention approaches are likely to emerge in the coming months, including antiviral agents, drugs may be to decrease disease progression, monoclonal antibodies, hyperimmune globulin and convalescent titre. If proven effective, these approaches could be used in high-risk individuals, including healthcare workers, other essential workers and older adults. 23–26 It is essential to maintain protective measures such as washing hands frequently with soap and water or gel alcohol and covering the mouth with a forearm when coughing or sneezing.
For all the reasons mentioned above, this review is necessary and essential. The latter is a well-defined protocol registered with PROSPERO, well planned to include the largest possible number of vaccines, a significant number of vaccinated patients, thus providing safe and reliable results regarding the use of vaccines.
Supplementary Material
Contributors: KSM, ACAS and APFC contributed to the design of this review. KSM and ACAS drafted the protocol manuscript. APFC and AKG revised the manuscript. KSM, AKG and APFC developed the search strategies. KSM, CLF and ACAS implemented the search strategies. KSM, CLF, ACAS and APFC tracked the potential studies, extracted the data and assessed the quality. In case of disagreement between the data extractors, AKG advised on the methodology and worked as a referee. KSM completed the data synthesis. All authors approved the final version for publication.
Funding: The authors have not declared a specific grant for this research from any funding agency in the public, commercial or not-for-profit sectors.
Competing interests: None declared.
Patient and public involvement: Patients and/or the public were not involved in the design, or conduct, or reporting, or dissemination plans of this research.
Provenance and peer review: Not commissioned; externally peer reviewed.
Ethics statements
Patient consent for publication.
Not applicable.
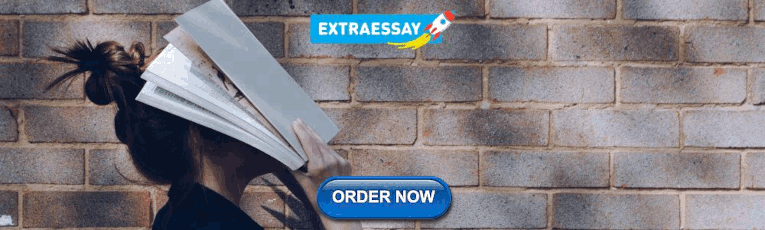
IMAGES
VIDEO
COMMENTS
In simplified terms, the GWAS can be viewed as hypothesis-generating and the CGAS as hypothesis-testing approaches ... COVID-19 Host Genetics Initiative (2020) The COVID-19 host genetics initiative a global initiative to elucidate the role of host genetic factors in susceptibility and severity of the SARS-CoV-2 virus pandemic.
In late November 2020, there was a flurry of media coverage of two companies' claims of 95% eficacy rates of newly developed COVID-19 vaccines, but information about the confidence interval was not reported. This paper presents a way of teaching the concept of hypothesis testing and the construction of confidence intervals using numbers ...
The enormous U.S. toll of Covid-19 is attributable in part to the lack of an effective testing regimen. ... The more we delay prioritizing and creating the infrastructure needed for hypothesis ...
The COVID lab-leak hypothesis: what scientists do and don't know ... COVID-19 could have come from a lab in a few ways. Researchers might have collected SARS-CoV-2 from an animal and maintained ...
Population-scale testing is an essential component of responses to the COVID-19 pandemic and is likely to become increasingly important in public health. Here, Mercer and Salit describe the roles ...
COVID-19: impact on Public Health and hypothesis-driven investigations on genetic susceptibility and severity Immunogenetics. 2022 Aug;74(4):381-407. doi: 10.1007/s00251-022-01261-w. ... COVID-19 is a new complex multisystem disease caused by the novel coronavirus SARS-CoV-2. In slightly over 2 years, it infected nearly 500 million and killed 6 ...
Table of contents. Step 1: State your null and alternate hypothesis. Step 2: Collect data. Step 3: Perform a statistical test. Step 4: Decide whether to reject or fail to reject your null hypothesis. Step 5: Present your findings. Other interesting articles. Frequently asked questions about hypothesis testing.
Hypothesis Testing Sustained Declines in COVID-19 Intensity. By Scott Ganz. AEI Economic Policy Working Paper Series. June 17, 2020. By Scott Ganz. Editor's note: This paper has been updated ...
The origins of SARS-CoV-2, the virus that causes COVID-19 and has infected more than 171 million people, killing close to 3.7 million worldwide as of June 4, remain unclear. Many scientists ...
In late November 2020, there was a flurry of media coverage of two companies' claims of 95% efficacy rates of newly developed COVID-19 vaccines, but information about the confidence interval was not reported. This paper presents a way of teaching the concept of hypothesis testing and the construction of confidence intervals using numbers announced by the drug makers Pfizer and Moderna ...
8 min read. ·. Nov 21, 2020. 6. Hypothesis Testing is commonly used when trials for vaccine testing are performed. With all the recent vaccine news for Covid-19, I wanted to shed light on the general hypothesis testing process for vaccines. In this article, I will go over the following topics: · Why is Hypothesis Testing Even Needed for ...
In this blog, I'll explain both techniques using recent covid-19 related use-cases and will attempt to drive home the similarities and differences between the two theories. ... Hypothesis Testing.
Considering this background, the present study aimed at testing the anxiety-buffer hypothesis during the COVID-19 pandemic. More in detail, self-esteem should buffer the relationships from both a fear of COVID-19 and dispositional loneliness to anxiety symptoms - that in turn lead to depressive symptoms.
Figure.2 A walkthrough using the hypothesis testing (and p-value) to test the null hypothesis that there is no difference in the time of recovery between drug A and placebo. After the test, we reject the null hypothesis. Example 1. Mask wearing and the risk of non-contact transmission in a hamster model for COVID19.
7) Patients will become consumers. The Covid-19 pandemic literally impacts each and every one of us. The boundary between "healthy" and "sick" becomes blurred. People who have otherwise ...
I first learned about hypothesis testing in the first year of my Bachelor's in Statistics. Ever since I've always felt that I was missing something about it.. What particularly bothered me was the presence of elements that seemed quite arbitrary, like those "magic numbers" such as 80% Power or 97.5% Confidence.. So I recently tried to make a deep dive into the topic and, at some point ...
For example, When testing the new vaccine on a random sample of 2500 previously uninfected people, its found that these people are 70 % less likely to contract to COVID-19 compared to those who ...
The major finding was that even when a COVID-19 vaccine always produces sterilizing immunity against every SARS-CoV-2 variant, its ability to control the epidemic can be compromised by multiple common conditions. ... This hypothesis is not supported by the data on US hospital admissions. In the 20 weeks from 14 May 2022 through 24 September ...
The ongoing COVID-19 pandemic, caused by the novel severe acute respiratory syndrome coronavirus type 2 (SARS-CoV-2), has affected 212 countries worldwide at various degrees as of 8 May 2020.1 In this paper we discuss a hypothesis that prior viral infections—either by SARS-CoV-2 or different strains of coronaviruses, or potentially even other respiratory viruses—may predispose to more ...
There are two basic types of tests for COVID-19. Viral test: This test can tell you if you are currently infected with the coronavirus. A swab is placed in the nose or mouth to gather samples. There are two types of viral tests: laboratory tests (sometimes called polymerase chain reaction [PCR] tests), and rapid tests that detect portions of ...
One of the entry mechanisms of the SARS-CoV-2 coronavirus into host cells involves endosomal acidification. It has been proposed that under acidic conditions the Fusion Peptide Proximal Region (FPPR) of the SARS-CoV-2 spike glycoprotein acts as a pH-dependent switch, modulating immune response accessibility by influencing the positioning of the Receptor Binding Domain (RBD). This would provide ...
Collins also responded in the affirmative when asked by Republicans if "the origin of COVID-19 still unsettled science." ... whether or not he believed the lab leak hypothesis was a conspiracy ...
Introduction. SARS-CoV-2 is responsible for a large number of global COVID-19 cases. It is a highly transmissible virus among humans that has become a significant public health issue.1 Symptoms include fever, dry cough, fatigue, shortness of breath, chills, muscle pain, headache, gastric disorders and weight loss, often leading to death.2 Strategies such as social isolation, personal hygiene ...