Advertisement
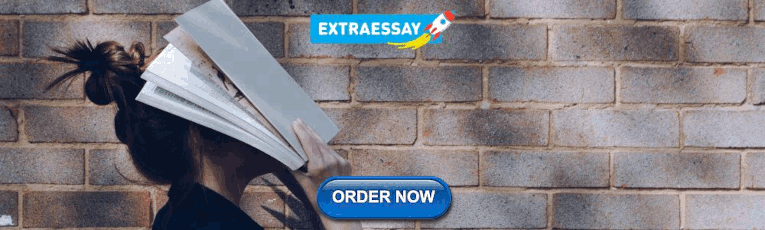
A review of waste paper recycling networks focusing on quantitative methods and sustainability
- Published: 13 October 2020
- Volume 23 , pages 55–76, ( 2021 )
Cite this article
- Cristiane Maria Defalque ORCID: orcid.org/0000-0001-6984-5405 1 , 2 ,
- Fernando Augusto Silva Marins 1 ,
- Aneirson Francisco da Silva 1 &
- Elen Yanina Aguirre Rodríguez 1
1332 Accesses
7 Citations
Explore all metrics
A discussion is currently under way in the literature on the sustainable benefits of recycling material, particularly paper, which has high global consumption and polluting capacity. Optimized planning of waste paper recycling networks stimulates sustainable processing efficiency, motivating the investigation of quantitative methods to guide decision-making. The objective of this article is to review papers that present quantitative models for planning waste paper recycling networks considering optimization of the echelons of this process, to analyze the evolution of research, find research opportunities and contribute to future research. The article presents an analysis of five categories of the selected studies: I—evolution of publications; II—echelons considered in different waste paper recycling systems; III—the sustainability pillars considered in the objectives of the formulated model; IV—formulations and techniques used; and V—uncertainty analysis. The proposal for waste paper recycling networks involves summary of the echelons considered in selected articles, to help future analysis. Research suggestions involving sustainability objectives, especially considering social issues, using different solution techniques and considering uncertainty were identified. This study, by reviewing the articles and identifying possibilities for future research, contributes to the development of research using quantitative methods for the efficient management of waste paper recycling networks or similar arrangements.
This is a preview of subscription content, log in via an institution to check access.
Access this article
Subscribe and save.
- Get 10 units per month
- Download Article/Chapter or Ebook
- 1 Unit = 1 Article or 1 Chapter
- Cancel anytime
Price includes VAT (Russian Federation)
Instant access to the full article PDF.
Rent this article via DeepDyve
Institutional subscriptions
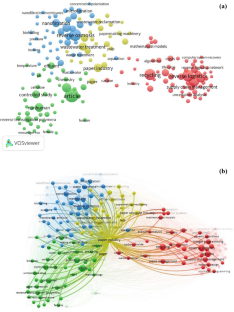
Source: prepared by the authors. The data were obtained from Scopus— www.scopus.com and Web of Science— www.webofknowledge.com . The maps were built using VOSviewer [ 63 ]
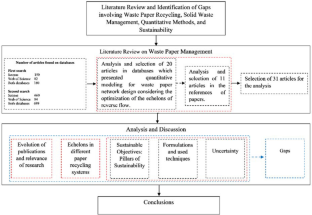
Source: prepared by the authors
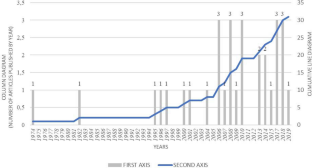
Source: prepared by the authors. Selected articles (Table 3 ) available in databases and other references described in “ Research method ”
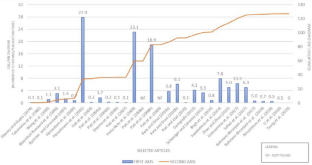
Source: prepared by the author. Selected articles (Table 3 ) available in databases and other references described in “ Research method ”. Number of citations obtained from Scopus— www.scopus.com and Web of Science— www.webofknowledge.com
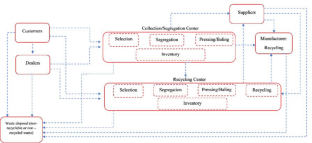
Source: prepared by the authors, based on echelons considered in the analyzed articles (Table 3 )
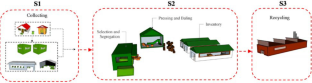
Source: prepared by the authors, based on echelons and operations verified in the analyzed articles (Table 3 )
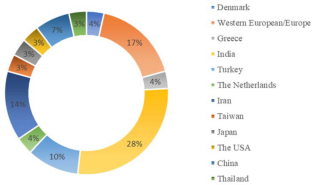
Source: prepared by the authors, based on analyses of the selected articles (Table 3 )
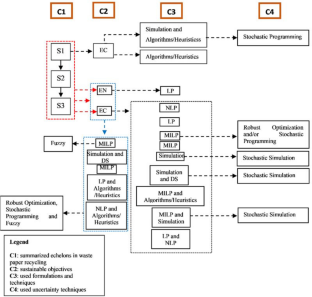
Similar content being viewed by others
Waste prevention for sustainable resource and waste management.
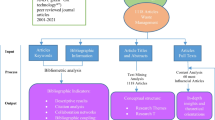
Waste management and green technology: future trends in circular economy leading towards environmental sustainability
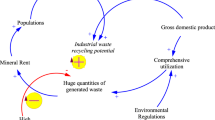
Measuring the recycling potential of industrial waste for long-term sustainability
European Parliament and Council (2008) Directive 2008/98/EC of 19 November 2008 on Waste and Repealing Certain Directives. Off J Eur Union L 312:3–30 https://eur-lex.europa.eu/legal-content/EN/TXT/PDF/?uri=CELEX:32008L0098&from=RO . Accessed 18 Dec 2019
Brasil (2010) Lei nº 12.305, de 2 de agosto de 2010. Institui a Política Nacional de Resíduos Sólidos; altera a Lei nº 9.605, de 12 de fevereiro de 1998; e dá outras providências (in Portuguese). In: Brasília, DF. Presidência da República. . https://www.planalto.gov.br/ccivil_03/_ato2007-2010/2010/lei/l12305.htm . Accessed 18 Dec 2019
Brasil (2010) Decreto nº 7.404, de 23 de dezembro de 2010. Regulamenta a Lei nº 12.305, de 2 de agosto de 2010, que institui a Política Nacional de Resíduos Sólidos, cria o Comitê Interministerial da Política Nacional de Resíduos Sólidos e o Comitê Orientador para a Implantação dos Sistemas de Logística Reversa, e dá outras providências (in Portuguese). In: Brasília, DF. Presidência da República planalto.gov.br/ccivil_03/_ato2007–2010/2010/Decreto/D7404.htm. Accessed 18 Dec 2019
Govindan K, Soleimani H, Kannan D (2015) Reverse logistics and closed-loop supply chain: A comprehensive review to explore the future. Eur J Oper Res 240:603–626. https://doi.org/10.1016/j.ejor.2014.07.012
Article MathSciNet MATH Google Scholar
Ervasti I, Miranda R, Kauranen I (2016) A global, comprehensive review of literature related to paper recycling: A pressing need for a uniform system of terms and definitions. Waste Manag 48:64–71. https://doi.org/10.1016/J.WASMAN.2015.11.020
Article Google Scholar
Kara SS, Onut S (2010) A two-stage stochastic and robust programming approach to strategic planning of a reverse supply network: The case of paper recycling. Expert Syst Appl 37:6129–6137. https://doi.org/10.1016/j.eswa.2010.02.116
Rajeev A, Pati RK, Padhi SS, Govindan K (2017) Evolution of sustainability in supply chain management: A literature review. J Clean Prod 162:299–314. https://doi.org/10.1016/j.jclepro.2017.05.026
Elkington J (1997) Cannibals with Forks: The Triple Bottom Line of 21st Century Business. Capstone, Oxford, United Kingdom
Google Scholar
IPIECA/API (2005) Oil and Gas Industry Guidance on Voluntary Sustainability Reporting. Using Environmental , Health & Safety , Social and Economic Performance Indicators. In: London, United Kingdom and Washington. DC, USA International Petroleum Industry Environmental Conservation Association and American Petroleum Institute. https://www.ingenieroambiental.com/4030/reporting_guide.pdf . Accessed 6 Oct 2017
Alumur SA, Nickel S, Saldanha-da-Gama F, Verter V (2012) Multi-period reverse logistics network design. Eur J Oper Res 220:67–78. https://doi.org/10.1016/j.ejor.2011.12.045
Melo MT, Nickel S, Saldanha-da-Gama F (2009) Facility location and supply chain management – A review. Eur J Oper Res 196:401–412. https://doi.org/10.1016/j.ejor.2008.05.007
Soleimani H, Govindan K, Saghafi H, Jafari H (2017) Fuzzy multi-objective sustainable and green closed-loop supply chain network design. Comput Ind Eng 109:191–203. https://doi.org/10.1016/j.cie.2017.04.038
Yu H, Solvang WD (2016) A general reverse logistics network design model for product reuse and recycling with environmental considerations. Int J Adv Manuf Technol 87:2693–2711. https://doi.org/10.1007/s00170-016-8612-6
Chen Y-W, Wang L-C, Wang A, Chen T-L (2017) A particle swarm approach for optimizing a multi-stage closed loop supply chain for the solar cell industry. Robot Comput Integr Manuf 43:111–123. https://doi.org/10.1016/j.rcim.2015.10.006
Hoornweg D, Bhada-Tata P (2012) What a Waste. A Global Review of Solid Waste Management.Urban Development Series Knowledge Papers; knowledge Papers No. 15.World Bank, Washington 281:44 p. https://doi.org/ https://doi.org/10.1111/febs.13058
Villanueva A, Wenzel H (2007) Paper waste – Recycling, incineration or landfilling? A review of existing life cycle assessments. Waste Manag 27:S29–S46. https://doi.org/10.1016/j.wasman.2007.02.019
Schmidt JH, Holm P, Merrild A, Christensen P (2007) Life cycle assessment of the waste hierarchy – A Danish case study on waste paper. Waste Manag 27:1519–1530. https://doi.org/10.1016/j.wasman.2006.09.004
Pati RK, Vrat P (2010) Economic paper blending optimization model with competing materials. Manag Environ Qual An Int J 21:602–617. https://doi.org/10.1108/14777831011067917
Sahamie R, Stindt D, Nuss C (2013) Transdisciplinary Research in Sustainable Operations - An Application to Closed-Loop Supply Chains. Bus Strateg Environ 22:245–268. https://doi.org/10.1002/bse.1771
Berglund C, Söderholm P, Nilsson M (2002) A note on inter-country differences in waste paper recovery and utilization. Resour Conserv Recycl 34:175–191. https://doi.org/10.1016/S0921-3449(01)00101-X
Ervasti I, Miranda R, Kauranen I (2016) Paper recycling framework, the “Wheel of Fiber”. J Environ Manage 174:35–44. https://doi.org/10.1016/j.jenvman.2016.03.004
Miranda R, Concepcion Monte M, Blanco A (2011) Impact of increased collection rates and the use of commingled collection systems on the quality of recovered paper. Part 1: Increased collection rates. Waste Manag 31:2208–2216. https://doi.org/10.1016/j.wasman.2011.06.006
Cormier D, Magnan M (1997) Investors’ assessment of implicit environmental liabilities: An empirical investigation. J Account Public Policy 16:215–241. https://doi.org/10.1016/S0278-4254(97)00002-1
ABNT (2009) NBR ISO 14040: Gestão ambiental - Avaliação do ciclo de vida - Princípios e estrutura (in Portuguese). In: Rio de Janeiro, Brazil Associação Brasileira de Normas Técnicas 1–22
Hart A, Clift R, Riddlestone S, Buntin J (2005) Use of Life Cycle Assessment to Develop Industrial Ecologies—A Case Study: Graphics Paper. Process Saf Environ Prot 83:359–363. https://doi.org/10.1205/psep.04391
Sevigné-Itoiz E, Gasol CM, Rieradevall J, Gabarrell X (2015) Methodology of supporting decision-making of waste management with material flow analysis (MFA) and consequential life cycle assessment (CLCA): case study of waste paper recycling. J Clean Prod 105:253–262. https://doi.org/10.1016/j.jclepro.2014.07.026
Berglund C, Söderholm P (2003) Complementing Empirical Evidence on Global Recycling and Trade of Waste Paper. World Dev 31:743–754. https://doi.org/10.1016/S0305-750X(03)00007-X
Van Beukering PJH, Bouman MN (2001) Empirical Evidence on Recycling and Trade of Paper and Lead in Developed and Developing Countries. World Dev 29:1717–1737. https://doi.org/10.1016/S0305-750X(01)00065-1
Nielsen L (2011) Classifications of Countries Based on Their Level of Development : How it is Done and How it Could be Done. Washington, DC, United States https://www.imf.org/external/pubs/ft/wp/2011/wp1131.pdf . Accessed 28 Dec 2019
Salema MIG, Barbosa-Povoa AP, Novais AQ (2007) An optimization model for the design of a capacitated multi-product reverse logistics network with uncertainty. Eur J Oper Res 179:1063–1077. https://doi.org/10.1016/j.ejor.2005.05.032
Article MATH Google Scholar
Roghanian E, Pazhoheshfar P (2014) An optimization model for reverse logistics network under stochastic environment by using genetic algorithm. J Manuf Syst 33:348–356. https://doi.org/10.1016/j.jmsy.2014.02.007
Sheriff KMM, Subramanian N, Rahman S, Jayaram J (2017) Integrated optimization model and methodology for plastics recycling: Indian empirical evidence. J Clean Prod 153:707–717. https://doi.org/10.1016/j.jclepro.2016.07.137
Alwaeli M (2011) An Economic Analysis of Joined Costs and Beneficial Effects of Waste Recycling. Environ Prot Eng 37:92–103. https://www.researchgate.net/publication/287455009_An_economic_analysis_of_joined_costs_and_beneficial_effects_of_waste_recycling
Feitó-Cespón M, Sarache W, Piedra-Jimenez F, Cespón-Castro R (2017) Redesign of a sustainable reverse supply chain under uncertainty: A case study. J Clean Prod 151:206–217. https://doi.org/10.1016/j.jclepro.2017.03.057
Zhalechian M, Tavakkoli-Moghaddam R, Rahimi Y (2017) A self-adaptive evolutionary algorithm for a fuzzy multi-objective hub location problem: an integration of responsiveness and social responsibility. Eng Appl Artif Intell 62:1–16. https://doi.org/10.1016/j.engappai.2017.03.006
Govindan K, Paam P, Abtahi A-R (2016) A fuzzy multi-objective optimization model for sustainable reverse logistics network design. Ecol Indic 67:753–768. https://doi.org/10.1016/j.ecolind.2016.03.017
Farrokhi-Asl H, Tavakkoli-Moghaddam R, Asgarian B, Sangari E (2017) Metaheuristics for a bi-objective location-routing-problem in waste collection management. J Ind Prod Eng 34:239–252. https://doi.org/10.1080/21681015.2016.1253619
Hahler S, Fleischmann M (2017) Strategic grading in the product acquisition process of a reverse supply chain. Prod Oper Manag 26:1498–1511. https://doi.org/10.1111/poms.12699
John ST, Sridharan R, Kumar PNR (2017) Multi-period reverse logistics network design with emission cost. Int J Logist Manag 28:127–149. https://doi.org/10.1108/IJLM-08-2015-0143
Demirel N, Özceylan E, Paksoy T, Gökçen H (2014) A genetic algorithm approach for optimising a closed-loop supply chain network with crisp and fuzzy objectives. Int J Prod Res 52:3637–3664. https://doi.org/10.1080/00207543.2013.879616
Hosseinzadeh M, Roghanian E (2012) An Optimization Model for Reverse Logistics Network under Stochastic Environment Using Genetic Algorithm. Int J Res Bus Soc Sci 3:249–264
Moghaddam KS (2015) Fuzzy multi-objective model for supplier selection and order allocation in reverse logistics systems under supply and demand uncertainty. Expert Syst Appl 42:6237–6254. https://doi.org/10.1016/j.eswa.2015.02.010
Nadizadeh A, Kafash B (2017) Fuzzy capacitated location-routing problem with simultaneous pickup and delivery demands. Transp Lett. https://doi.org/10.1080/19427867.2016.1270798
Gooran AN, Rafiei H, Rabani M (2018) Modeling risk and uncertainty in designing reverse logistics problem. Decis Sci Lett 1:13–24. https://doi.org/10.5267/j.dsl.2017.5.001
Babazadeh R, Jolai F, Razmi J (2015) Developing scenario-based robust optimisation approaches for the reverse logistics network design problem under uncertain environments. Int J Serv Oper Manag 20:418. https://doi.org/10.1504/IJSOM.2015.068526
Pishvaee MS, Rabbani M, Torabi SA (2011) A robust optimization approach to closed-loop supply chain network design under uncertainty. Appl Math Model 35:637–649. https://doi.org/10.1016/j.apm.2010.07.013
Agrawal S, Singh RK, Murtaza Q (2015) A literature review and perspectives in reverse logistics. Resour Conserv Recycl 97:76–92. https://doi.org/10.1016/j.resconrec.2015.02.009
Akçalı E, Çetinkaya S, Üster H (2009) Network design for reverse and closed-loop supply chains: An annotated bibliography of models and solution approaches. Networks 53:231–248. https://doi.org/10.1002/net.20267
Barbosa-Póvoa AP, da Silva C, Carvalho A (2018) Opportunities and challenges in sustainable supply chain: An operations research perspective. Eur J Oper Res 268:399–431. https://doi.org/10.1016/j.ejor.2017.10.036
Dekker R, Bloemhof J, Mallidis I (2012) Operations Research for green logistics - An overview of aspects, issues, contributions and challenges. Eur J Oper Res 219:671–679. https://doi.org/10.1016/j.ejor.2011.11.010
Fleischmann M, Bloemhof-Ruwaard JM, Dekker R et al (1997) Quantitative models for reverse logistics: A review. Eur J Oper Res 103:1–17. https://doi.org/10.1016/S0377-2217(97)00230-0
Govindan K, Soleimani H (2017) A review of reverse logistics and closed-loop supply chains: a Journal of Cleaner Production focus. J Clean Prod 142:371–384. https://doi.org/10.1016/j.jclepro.2016.03.126
Govindan K, Fattahi M, Keyvanshokooh E (2017) Supply chain network design under uncertainty: A comprehensive review and future research directions. Eur J Oper Res 263:108–141. https://doi.org/10.1016/j.ejor.2017.04.009
Ilgin MA, Gupta SM (2010) Environmentally conscious manufacturing and product recovery (ECMPRO): A review of the state of the art. J Environ Manage 91:563–591. https://doi.org/10.1016/j.jenvman.2009.09.037
Jaehn F, Juopperi R (2019) A Description of Supply Chain Planning Problems in the Paper Industry with Literature Review. Asia-Pacific J Oper Res. https://doi.org/10.1142/S0217595919500040
Lyeme HA, Mushi A, Nkansah-Gyekye Y (2017) Review of multi-objective optimization models for solid waste management systems with environmental considerations. J Math Comput Sci 7:150–174. https://www.researchgate.net/publication/312525358_REVIEW_OF_MULTI-OBJECTIVE_OPTIMIZATION_MODELS_FOR_SOLID_WASTE_MANAGEMENT_SYSTEMS_WITH_ENVIRONMENTAL_CONSIDERATIONS
Singh A, Trivedi A (2016) Sustainable green supply chain management: trends and current practices. Compet Rev 26:265–288. https://doi.org/10.1108/CR-05-2015-0034
Stindt D, Sahamie R (2014) Review of research on closed loop supply chain management in the process industry. Flex Serv Manuf J 26:268–293. https://doi.org/10.1007/s10696-012-9137-4
Tang CS, Zhou S (2012) Research advances in environmentally and socially sustainable operations. Eur J Oper Res 223:585–594. https://doi.org/ https://doi.org/10.1016/j.ejor.2012.07.030
Van Engeland J, Beliën J, De Boeck L, De Jaeger S (2020) Literature review: Strategic network optimization models in waste reverse supply chains. Omega 91:1–22. https://doi.org/10.1016/j.omega.2018.12.001
Rutkowski J, Rutkowski E (2017) Recycling in Brasil: Paper and Plastic Supply Chain. Resources 6:43. https://doi.org/10.3390/resources6030043
Fleischmann M, Beullens P, Bloemhof-Ruwaard JM, Van Wassenhove LN (2001) The Impact of Product Recovery on Logistics Network Design. Prod OperManag 10:156–173. https://doi.org/10.1111/j.1937-5956.2001.tb00076.x
Van Eck NJ, Waltman L (2010) Software survey: VOSviewer, a computer program for bibliometric mapping. Scientometrics 84:523–538. https://doi.org/10.1007/s11192-009-0146-3
Bloemhof-Ruwaard JM, Van Wassenhove LN, Gabel HL, Weaver PM (1996) An environmental life cycle optimization model for the European pulp and paper industry. Omega 24:615–629. https://doi.org/10.1016/S0305-0483(96)00026-6
Frota Neto JQ, Bloemhof-Ruwaard JM, Van Nunen JAEE, Van Heck E (2008) Designing and evaluating sustainable logistics networks. Int J Prod Econ 111:195–208. https://doi.org/10.1016/j.ijpe.2006.10.014
Pati RK, Vrat P, Kumar P (2006) Economic analysis of paper recycling vis-à-vis wood as raw material. Int J Prod Econ 103:489–508. https://doi.org/10.1016/j.ijpe.2005.08.006
Zhou X, Zhou Y (2015) Designing a multi-echelon reverse logistics operation and network: A case study of office paper in Beijing. Resouces Conserv Recycl 100:58–69. https://doi.org/10.1016/j.resconrec.2015.04.009
Byström S, Lönnstedt L (1997) Paper recycling: environmental and economic impact. Resour Conserv Recycl 21:109–127. https://doi.org/10.1016/S0921-3449(97)00031-1
Safaei AS, Roozbeh A, Paydar MM (2017) A robust optimization model for the design of a cardboard closed-loop supply chain. J Clean Prod 166:1154–1168. https://doi.org/10.1016/j.jclepro.2017.08.085
Glassey CR, Gupta VK (1974) A Linear Programming Analysis of Paper Recycling. Manage Sci 21:392–408. https://doi.org/10.1287/mnsc.21.4.392
Kleineidam U, Lambert AJD, Blansjaar J et al (2000) Optimising product recycling chains by control theory. Int J Prod Econ 66:185–195. https://doi.org/10.1016/S0925-5273(99)00120-6
Pati RK, Vrat P, Kumar P (2008) A MILP Model for design of paper recycling network. Int J Ecol Dev 9:69–86. https://www.researchgate.net/publication/259296422_A_MILP_Model_for_design_of_paper_recycling_network
Pati RK, Vrat P, Kumar P (2009) Decision-Making Model For Economical Wastepaper Collection. Product A Q J Natl Product Counc 49:265–271. https://www.researchgate.net/publication/259296517_Decision_Support_Model_for_Economic_Waste_Paper_Collection
Suyabatmaz AÇ, Altekin FT, Şahin G (2014) Hybrid simulation-analytical modeling approaches for the reverse logistics network design of a third-party logistics provider. Comput Ind Eng 70:74–89. https://doi.org/10.1016/j.cie.2014.01.004
Li M, Wang X, Zhang X, Li X (2018) Optimization design of multi-echelon recycling networks for third-party reverse logistics provider in the context of binary path selection. Acad J Manuf Eng 16:97–105. https://auif.utcluj.ro/images/PDF_AJME_2018_16_1/L13.pdf
Takamatsu T, Shioya S, Tsujimoto Y (1982) Optimal inter-regional distribution of waste paper and board in the waste paper recycling system. Resour Conserv 8:95–110. https://doi.org/10.1016/0166-3097(82)90035-9
Wang C-H, Even JC, Adams SK (1995) A mixed-integer linear model for optimal processing and transport of secondary materials. Resour Conserv Recycl 15:65–78. https://doi.org/10.1016/0921-3449(95)00024-D
Pati RK, Vrat P, Kumar P (2007) Three-win strategy with optimisation approach for recycled paper manufacturer. Int J Environ Waste Manag 1:269. https://doi.org/10.1504/IJEWM.2007.013636
Bogh MB, Mikkelsen H, Wohlk S (2014) Collection of recyclables from cubes - A case study. Socioecon Plann Sci 48:127–134. https://doi.org/10.1016/j.seps.2014.02.001
Entezaminia A, Heidari M, Rahmani D (2017) Robust aggregate production planning in a green supply chain under uncertainty considering reverse logistics: a case study. Int J Adv Manuf Technol 90:1507–1528. https://doi.org/10.1007/s00170-016-9459-6
Georgiadis P (2013) An integrated system dynamics model for strategic capacity planning in closed-loop recycling networks: A dynamic analysis for the paper industry. Simul Model Pract Theory 32:116–137. https://doi.org/10.1016/j.simpat.2012.11.009
Kara SS, Onut S (2010) A stochastic optimization approach for paper recycling reverse logistics network design under uncertainty. Int J Environ Sci Technol 7:717–730. https://link.springer.com/article/ https://doi.org/10.1007/BF03326181#citeas
Pati RK, Vrat P, Kumar P (2004) Cost optimisation model in recycled waste reverse logistics system. Int J Bus Perform Manag 6:245. https://doi.org/10.1504/IJBPM.2004.005631
Pati RK, Vrat P, Kumar P (2006) Integrated chain analysis of recycled vis-à-vis wood pulp paper industry: An Indian manufacturer viewpoint. Int J Value Chain Manag 1:44–63. https://doi.org/10.1504/IJVCM.2006.009023
Pati RK, Vrat P, Kumar P (2008) A goal programming model for paper recycling system. Omega 36:405–417. https://doi.org/10.1016/j.omega.2006.04.014
Rahmani-Ahranjani A, Bozorgi-Amiri A, Seifbarghy M, Najafi E (2017) Managing Environmentally Conscious in Designing Closed-loop Supply Chain for the Paper Industry. Int J Eng 30:1038–1047. https://doi.org/10.5829/ije.2017.30.07a.13
Rahmani-Ahranjani A, Seifbarghy M, Bozorgi-Amiri A, Najafi E (2018) Closed-loop supply chain network design for the paper industry: A multi-objective stochastic robust approach. Sci Iran 25:2881–2903. https://doi.org/ https://doi.org/10.24200/sci.2017.4464
Rinsatitnon N, Dijaroen W, Limpiwun T, et al (2018) Reverse logistics implementation in the construction industry: Paper waste focus. Songklanakarin J Sci Technol 40:798–805. https://doi.org/ https://doi.org/10.14456/sjst-psu.2018.113
Schweiger K, Sahamie R (2013) A hybrid Tabu Search approach for the design of a paper recycling network. Transp Res Part E Logist Transp Rev 50:98. https://doi.org/10.1016/j.tre.2012.10.006
Sharma N, Balan S, Vrat P, Kumar P (2006) Analysis of bullwhip effect in reverse supply chain. J Adv Manag Res 3:18–33. https://doi.org/10.1108/97279810680001243
Tseng S-H, Wee HM, Song PS, Jeng S (2019) Optimal green supply-chain model design considering full truckload. Kybernetes 48:2150–2174. https://doi.org/10.1108/K-07-2018-0415
Rahman MO, Hussain A, Basri H (2014) A critical review on waste paper sorting techniques. Int J Environ Sci Technol 11:551–564. https://doi.org/10.1007/s13762-013-0222-3
UNCTADStat (2018) Development status groups and composition. In: United Nations Conf. Trade Dev. - UNCTADStat. https://unctadstat.unctad.org/EN/Classifications/DimCountries_DevelopmentStatus_Hierarchy.pdf . Accessed 26 Jan 2019
World Bank (2017) Data Bank World Bank.org Population 2017. https://databank.worldbank.org/data/download/POP.pdf . Accessed 28 Dec 2019
T&A (2017) A Indústria de Papel e Celulose na Índia (in Portuguese). In: T&A Consult. https://investexportbrasil.dpr.gov.br/arquivos/PesquisasMercado/PMRIndiaSetorPapeleiro2017.pdf . Accessed 28 Dec 2019
Dijkgraaf E, Gradus RHJM (2014) The Effectiveness of Dutch Municipal Recycling Policies. Soc Sci Res Netw. https://doi.org/10.2139/ssrn.2540085
Council for the Environment Infrastructure (2013) DUTCH LOGISTICS 2040: DESIGNED TO LAST. www.rli.nl . Accessed 3 Nov 2018
Bing X, Bloemhof JM, Ramos TRP et al (2016) Research challenges in municipal solid waste logistics management. Waste Manag 48:584–592. https://doi.org/10.1016/j.wasman.2015.11.025
Sharma VK, van Beukering P, Nag B (1997) Environmental and economic policy analysis of waste paper trade and recycling in India. Resour Conserv Recycl 21:55–70. https://doi.org/10.1016/S0921-3449(97)00025-6
Alwaeli M (2015) An Overview of Municipal Solid Waste Management in Poland. The Current Situation. Problems and Challenges Environ Prot Eng 41:181–193. https://doi.org/10.5277/epe150414
Alwaeli M (2009) Editorial. Waste Manag 29:3054–3055. https://doi.org/10.1016/j.wasman.2009.09.004
Levlin J-E, Read B, Grossmann H, et al (2010) COST Action E48 – The Future of Paper Recycling in Europe: Opportunities and Limitations. The Paper Industry Technical Association (PITA), Bury, Greater Manchester, England https://www.cost.eu/publications/the-future-of-paper-recycling-in-europe-opportunities-and-limitations . Accessed 31 Jul 2020
Feng H, Tomonari S (2013) Cause analysis of low collection rate of Chinese waste paper. pp 685–695. https://www.witpress.com/elibrary/wit-transactions-on-ecology-and-the-environment/173/24503 . Accessed 31 Jul 2020
Wu X (2013) Optimization of waste paper’s enzymatic deinking processes based on neural network and particle swarm optimization. Proc - 2013 Int Conf Mechatron Sci Electr Eng Comput MEC 2013:44–47. https://doi.org/10.1109/MEC.2013.6885048
Vashisth S, Bennington CPJ, Grace JR, Kerekes RJ (2011) Column Flotation Deinking: State-of-the-art and opportunities. Resour Conserv Recycl 55:1154–1177. https://doi.org/10.1016/j.resconrec.2011.06.013
Leu HG, Lin SH (1998) Cost-benefit analysis of resource material recycling. Resour Conserv Recycl 23:183–192. https://doi.org/10.1016/S0921-3449(98)00020-2
Fleischmann M, Bloemhof-Ruwaard JM, Beullens P, Dekker R (2004) Reverse Logistics Network Design. In: Dekker R, Fleischmann M, Inderfurth K, Van Wassenhove LN (eds) Reverse Logistics: Quantitative Models for Closed-Loop Supply Chains. Springer Berlin Heidelberg, Berlin, Heidelberg, Germany, pp 83–87. https://doi.org/ https://doi.org/10.1007/978-3-540-24803-3
Cheung WM, Pachisia V (2015) Facilitating waste paper recycling and repurposing via cost modelling of machine failure, labour availability and waste quantity. Resour Conserv Recycl 101:34–41. https://doi.org/10.1016/j.resconrec.2015.05.011
Ahluwalia PK, Nema AK (2011) Capacity planning for electronic waste management facilities under uncertainty: multi-objective multi-time-step model development. Waste Manag Res 29:694–709. https://doi.org/10.1177/0734242X10382592
Van Beukering PJH, Schoon E, Mani A (1996) The Informal Sector and Waste Paper Recovery in Bombay. Amsterdam, Netherlands. https://pubs.iied.org/pdfs/8124IIED.pdf . Accessed 28 Dec 2019
Dekker R, Fleischmann M, Inderfurth K, WassenhoveVLN (2004) Quantitative Models for Reverse Logistics Decision Making. In: Dekker R, Fleischmann M, Inderfurth K, Van Wassenhove LN (eds) Reverse Logistics: Quantitative Models for Closed-Loop Supply Chains. Springer Berlin Heidelberg, Berlin, Heidelberg, Germany, p 34. https://doi.org/ https://doi.org/10.1007/978-3-540-24803-3
Xu Z, Elomri A, Pokharel S et al (2017) Global reverse supply chain design for solid waste recycling under uncertainties and carbon emission constraint. Waste Manag 64:358–370. https://doi.org/10.1016/j.wasman.2017.02.024
Fleischmann M, Krikke HR, Dekker R, Flapper SDP (2000) A characterisation of logistics networks for product recovery. Omega 28:653–666. https://doi.org/10.1016/S0305-0483(00)00022-0
Alem D (2011) Programação estocástica e otimização robusta no planejamento da produção de empresas moveleiras (in Portuguese). https://teses.usp.br/teses/disponiveis/55/55134/tde-29112011-162103/publico/alem.pdf . Accessed 27 Dec 2019
Alem D, Morabito R (2015) Planejamento da produção sob incerteza: programação estocástica versus otimização robusta (in Portuguese). Gestão & Produção 22:539–551. https://doi.org/10.1590/0104-530X1211-14
da Silva AF, Marins FAS (2014) Revisão da literatura sobre modelos de Programação por Metas determinística e sob incerteza (in Portuguese). Production 25:92–112. https://doi.org/10.1590/S0103-65132014005000003
Download references
Acknowledgements
This study was financed in part by the National Council for Scientific and Technological Development (CNPq—302730/2018; CNPq—303350/2018-0), the São Paulo State Research Foundation (FAPESP—2018/06858-0; FAPESP—2018/14433-0) and the Coordination for the Improvement of Higher Education Personnel—Brazil (CAPES)—Finance Code 001.
Author information
Authors and affiliations.
Production Department, Engineering College-São Paulo State University, Avenida Ariberto Pereira da Cunha, 333, Portal das Colinas, Guaratinguetá, SP, 12516-410, Brazil
Cristiane Maria Defalque, Fernando Augusto Silva Marins, Aneirson Francisco da Silva & Elen Yanina Aguirre Rodríguez
Agulhas Negras Military Academy (Academia Militar das Agulhas Negras-AMAN), Rodovia Presidente Dutra, km 306, s/nº, Resende, RJ, 27534-970, Brazil
Cristiane Maria Defalque
You can also search for this author in PubMed Google Scholar
Corresponding author
Correspondence to Cristiane Maria Defalque .
Ethics declarations
Conflict of interest.
The authors declare that they have no conflict of interest.
Additional information
Publisher's note.
Springer Nature remains neutral with regard to jurisdictional claims in published maps and institutional affiliations.
Rights and permissions
Reprints and permissions
About this article
Defalque, C.M., Marins, F.A.S., da Silva, A.F. et al. A review of waste paper recycling networks focusing on quantitative methods and sustainability. J Mater Cycles Waste Manag 23 , 55–76 (2021). https://doi.org/10.1007/s10163-020-01124-0
Download citation
Received : 29 April 2020
Accepted : 25 September 2020
Published : 13 October 2020
Issue Date : January 2021
DOI : https://doi.org/10.1007/s10163-020-01124-0
Share this article
Anyone you share the following link with will be able to read this content:
Sorry, a shareable link is not currently available for this article.
Provided by the Springer Nature SharedIt content-sharing initiative
- Waste paper recycling
- Network planning
- Sustainability
- Quantitative modeling
- Literature review
- Find a journal
- Publish with us
- Track your research
Accessibility Links
- Skip to content
- Skip to search IOPscience
- Skip to Journals list
- Accessibility help
- Accessibility Help
Click here to close this panel.
Purpose-led Publishing is a coalition of three not-for-profit publishers in the field of physical sciences: AIP Publishing, the American Physical Society and IOP Publishing.
Together, as publishers that will always put purpose above profit, we have defined a set of industry standards that underpin high-quality, ethical scholarly communications.
We are proudly declaring that science is our only shareholder.
Shredder machine for plastic recycling: A review paper
J H Wong 1 , M J H Gan 1,2 , B L Chua 1 , M Gakim 1 and N J Siambun 1,2
Published under licence by IOP Publishing Ltd IOP Conference Series: Materials Science and Engineering , Volume 1217 , Material and Energy Engineering for Sustainable Advancement (MEESA 2021) 29th-30th September 2021, Kota Kinabalu, Sabah, Malaysia Citation J H Wong et al 2022 IOP Conf. Ser.: Mater. Sci. Eng. 1217 012007 DOI 10.1088/1757-899X/1217/1/012007
Article metrics
8013 Total downloads
Share this article
Author e-mails.
Author affiliations
1 Mechanical Engineering, Faculty of Engineering, Universiti Malaysia Sabah, Jalan UMS, 88400 Kota Kinabalu, Sabah, Malaysia
2 Centre of Research in Energy and Advanced Materials, Faculty of Engineering Universiti Malaysia Sabah, Jalan UMS, 88400 Kota Kinabalu, Sabah, Malaysia
Buy this article in print
Plastic pollution has become a global concern due to poor plastic waste disposal management. As an alternative, independent recycling efforts are necessary. A Plastic shredder machine is a preliminary machine used to cut plastic waste into small pieces before turning it into useful products. The concept design of the shredder machine that is currently available is fairly similar. The shaft and blades are the critical components in the shredder machine that determines its performance. The geometry and orientation of the blades that were fitted into the single or double-shafts were found to directly affect the shredding performance. Therefore, this article aims to review the various geometry and orientations of the blades that give direct effect on the shredding performance, as well as identifying the research gaps related to the shredder machine for plastic waste materials
Export citation and abstract BibTeX RIS
Content from this work may be used under the terms of the Creative Commons Attribution 3.0 licence . Any further distribution of this work must maintain attribution to the author(s) and the title of the work, journal citation and DOI.
Information
- Author Services
Initiatives
You are accessing a machine-readable page. In order to be human-readable, please install an RSS reader.
All articles published by MDPI are made immediately available worldwide under an open access license. No special permission is required to reuse all or part of the article published by MDPI, including figures and tables. For articles published under an open access Creative Common CC BY license, any part of the article may be reused without permission provided that the original article is clearly cited. For more information, please refer to https://www.mdpi.com/openaccess .
Feature papers represent the most advanced research with significant potential for high impact in the field. A Feature Paper should be a substantial original Article that involves several techniques or approaches, provides an outlook for future research directions and describes possible research applications.
Feature papers are submitted upon individual invitation or recommendation by the scientific editors and must receive positive feedback from the reviewers.
Editor’s Choice articles are based on recommendations by the scientific editors of MDPI journals from around the world. Editors select a small number of articles recently published in the journal that they believe will be particularly interesting to readers, or important in the respective research area. The aim is to provide a snapshot of some of the most exciting work published in the various research areas of the journal.
Original Submission Date Received: .
- Active Journals
- Find a Journal
- Proceedings Series
- For Authors
- For Reviewers
- For Editors
- For Librarians
- For Publishers
- For Societies
- For Conference Organizers
- Open Access Policy
- Institutional Open Access Program
- Special Issues Guidelines
- Editorial Process
- Research and Publication Ethics
- Article Processing Charges
- Testimonials
- Preprints.org
- SciProfiles
- Encyclopedia
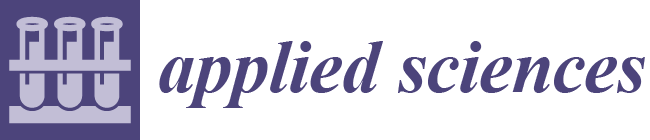
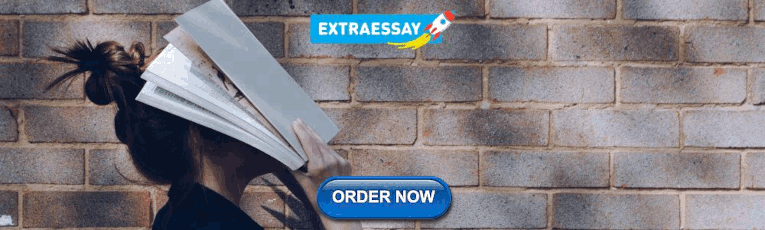
Article Menu
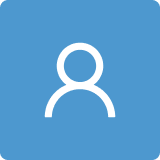
- Subscribe SciFeed
- Recommended Articles
- Google Scholar
- on Google Scholar
- Table of Contents
Find support for a specific problem in the support section of our website.
Please let us know what you think of our products and services.
Visit our dedicated information section to learn more about MDPI.
JSmol Viewer
A comprehensive review on brain–computer interface (bci)-based machine and deep learning algorithms for stroke rehabilitation.
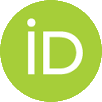
1. Introduction
2. background.
- Unlocking the potential of brain–computer interfaces (BCIs) for brain rehabilitation goes hand in hand with the remarkable power of machine learning. These intricate algorithms act as digital guides, navigating the complex landscape of brain signals to reveal the hidden pathways to recovery. By learning to recognize the unique patterns within each person’s neural code, machine learning techniques like support vector machines, deep neural networks, and random forests become adept at deciphering intentions and translating them into tangible actions. This remarkable synergy opens a world of possibilities for individualized rehabilitation, allowing us to harness the power of our own minds to regain control and rebuild skills [ 21 , 22 , 23 , 24 ].
- BCIs are able to adapt to variations in a user’s brain impulses over time because of machine learning. Increasing accuracy and resilience, adaptive models continuously modify their parameters in response to fresh data [ 20 , 25 , 26 , 27 ].
- Tailored rehabilitation interventions are made possible by machine learning. Rehabilitative techniques can be adjusted as necessary by training models to identify each user’s unique brain patterns [ 7 , 11 , 19 , 28 , 29 , 30 ].
- Instant feedback: Machine learning can process brain signals very quickly, so BCIs can react in real time. This is like having a virtual coach that gives you feedback right away, helping you learn faster [ 14 , 17 ].
- Predicting success: Machine learning can look at brain activity and predict how well someone will do in rehab. This helps doctors make better treatment plans and obtain better results [ 20 ].
- Seeing the whole picture: Machine learning can combine different types of brain data, like EEGs and fMRIs, to obtain a more complete understanding of how the brain is working during rehab [ 17 , 20 ].
3. Diverse Applications of EEG, Machine Learning, and Deep Learning in Rehabilitation
- Motor Rehabilitation: The utilization of machine learning algorithms in decoding EEG signals during motor imagery tasks opens avenues for controlling external devices, including robotic exoskeletons and prosthetics [ 13 , 19 , 31 , 33 ]. Systems providing feedback in real time not only instruct users through precise motor tasks but also contribute significantly to motor skill relearning and the promotion of neuroplasticity [ 14 , 19 , 32 , 33 ].
- Cognitive Rehabilitation: EEG signals play a pivotal role in gauging and enhancing attention stages using Neurofeedback techniques [ 8 , 11 , 13 , 34 ]. The adaptability of machine learning facilitates the tailoring of training procedures tailored to individual mental states, while the nuanced capabilities of deep learning contribute to the design of personalized memory training tasks [ 7 , 8 , 13 , 34 ].
- Neuropsychiatric Rehabilitation: EEG neurotraining emerges as a beneficial instrument for handling stress and anxiety, utilizing artificial intelligence to recognize patterns associated with stress and triggering interventions for relaxation [ 10 , 28 , 35 , 36 ]. Moreover, neurotraining based on EEG assists those with ADHD in refining concentration and controlling attention by reinforcing preferred brain activity patterns [ 13 ].
- Walking Rehabilitation: Integrating ML and EEG with systems that capture movements allows for a comprehensive analysis of gait patterns, providing real-time feedback during walking exercises and potentially revolutionizing rehabilitation approaches [ 13 , 31 , 34 , 37 ].
- Visual and Hearing Restoration: Protocols relying on EEG, in collaboration with advanced machine learning approaches, provide an intricate structure for creating customized training activities for individuals experiencing sensory perception challenges in both vision and hearing [ 32 , 38 ].
- Multimodal Rehabilitation: The integration of EEG with other cutting-edge technologies, such as virtual reality or functional near-infrared spectroscopy, paves the way for innovative multimodal rehabilitation approaches [ 19 , 20 , 33 , 39 , 40 ].
4. EEG Signal Acquisition and Motor Imagery Training
4.1. eeg-based signal acquisition, 4.2. patient training on motor imagery tasks.
- Warm-up (t = 0 s): The user focuses on a fixation point or cue to settle their mind and prepare for the upcoming task. MI Task: A visual cue, such as an arrow, instructs the user to perform a specific MI task (e.g., imagine left-hand movement).
- Data Collection (t = 3 s): During the task period, EEG sensors capture the user’s brain activity, recording the unique electrical signature of the imagined movement.
- Machine Learning (t = 3–7 s): The collected data are then analyzed by a machine learning algorithm. This algorithm identifies the key features that distinguish different MI tasks from the EEG signals.
- No Feedback: In the initial phase, no feedback is provided to the user. This allows the machine learning algorithm to focus solely on understanding the user’s individual EEG patterns.
- MI Task Repetition: Once the system has been calibrated, users repeat the MI tasks. Real-time Feedback: This time, the system provides feedback in real time. For example, a bar might grow longer or change color based on the system’s confidence in recognizing the current MI task.
- Refinement and Repetition: With each trial, the user receives feedback and can adjust their mental strategies to produce clearer EEG patterns. This iterative process strengthens the connection between the imagined movement and the corresponding EEG signature.
- Gradual Improvement: The Graz training paradigm involves multiple training sessions, spread over days or weeks. With each session, the user’s ability to generate distinct EEG patterns for different MI tasks improves, leading to more accurate recognition by the system.
- Customization: The training protocol can be adapted to individual needs and goals. The specific MI tasks, cues, and feedback types can be tailored to suit different applications, such as controlling a prosthetic limb or navigating a virtual environment.
5. EEG Signal Processing and Classification Techniques in Rehabilitation Research
- Filtering: The application of low pass, high pass, and notch filters emerges as a crucial step in refining EEG signal quality, as it effectively eliminates undesirable frequency components [ 38 , 47 ].
- Artifact Elimination: Approaches like Independent Component Analysis (ICA) and Principal Component Analysis (PCA) play a crucial role in differentiating and removing disturbances, encompassing eye blinks, muscle movements, and interference from electrocardiograms [ 17 , 18 , 48 ].
- Time Domain Features: The extraction of characteristics like average amplitude, root mean square value, and signal variance represents a nuanced approach to capturing the temporal characteristics inherent in EEG signals [ 19 , 20 , 33 ].
- Frequency Domain Features: Insights into the frequency distribution of brain activity are unveiled through the meticulous examination of power spectral density, spectral entropy, and band power ratios [ 11 , 31 , 47 ].
- Time-Frequency Features: Methods such as wavelet transformation and short-time Fourier transformation enhance complexity by unveiling the dynamic variations in EEG signal characteristics across both time and frequency domains [ 32 , 49 , 50 ].
- Functional Interconnection: Measures encompassing coherence, phase synchronization, and mutual information serve as invaluable tools in assessing the intricate functional relationships between different brain regions [ 33 , 53 , 54 ].
- Graph Theory Analysis: The innovative representation of EEG data as networks, coupled with graph theory metrics, offers a unique lens through which organizational and communication patterns within the brain can be deciphered [ 51 , 52 ].
- Pattern Recognition and Motor Imagery: A specialized focus on processing EEG signals derived from motor imagery tasks facilitates the recognition of specific patterns associated with imagined movements, thereby paving the way for tailored interventions [ 26 , 55 , 56 ].
5.1. Feature and Channel Selection
- Filter Approach: Initiating with the full set of features, filter methods meticulously identify the optimal subset through dedicated selection criteria. These criteria often revolve around key characteristics like information gain, dependency, consistency, correlation, and distance measures [ 57 ]. A significant advantage of filter methods lies in their minimal computational requirements. Additionally, the feature selection process operates independently of the chosen classifier, providing greater flexibility. Widely utilized filter methods include correlation criteria and mutual information techniques, both meticulously honing in on the most informative features within the data landscape.
- Wrapper Approach: Distinct from filter methods, wrapper approaches forge a direct partnership with the classifier to select features meticulously. They iteratively present candidate feature subsets to the classifier, diligently evaluating its performance. This feedback loop guides the selection process, prompting either acceptance of a subset based on established criteria or the proposal of new combinations for further evaluation. Algorithms within this realm encompass searching algorithms and evolutionary algorithms. The former embarks on their quest with an empty set, strategically adding or removing features until the classifier’s performance peaks. Their journey typically concludes when a designated maximum feature subset size is attained. Meanwhile, evolutionary algorithms, such as particle swarm optimization (PSO) [ 58 ], and artificial bee colony (ABC) [ 59 , 60 ], harness nature-inspired optimization techniques to uncover optimal subsets. While wrapper methods excel at identifying feature combinations that yield superior classifier performance compared to filter methods, their computational demands are considerable, rendering them less suitable for handling vast datasets.
5.2. EEG-Based Machine Learning and Deep Learning Algorithms
- Sample Size: Effective with small-to-medium-sized datasets.
- Accuracy: High accuracy in binary and multi-class classification problems as Figure 4 .
- Training Cost: Moderate, with a need for tuning hyperparameters.
- Sample Size: Suitable for small datasets.
- Accuracy: Good for pattern recognition tasks.
- Training Cost: Low, as k-NN is a lazy learner.
- Sample Size: Requires a large dataset.
- Accuracy: High for spatial feature extraction and classification.
- Training Cost: High due to the need for extensive computational resources.
- Sample Size: Requires a large dataset.
- Accuracy: High for sequential data analysis.
- Training Cost: High due to recurrent nature and complex computations.
- Accuracy: High for temporal pattern recognition.
- Training Cost: High due to complex architecture.
- Sample Size: Effective with medium to large datasets.
- Accuracy: Moderate to high, depending on the complexity of the task.
- Training Cost: Moderate.
5.3. Performance Indicators and Metrics in Evaluating the Effectiveness of Methods
- Classification Accuracy: The efficacy of EEG-based models is scrutinized through the lens of classification accuracy, providing insights into their ability to distinguish between different classes or states in activities like differentiating motor imagery or identifying cognitive states [ 11 , 13 , 14 , 19 , 31 , 38 , 38 ].
- ROC Curve and AUC: The evaluation of the balance between specificity and sensitivity in classification assignments is facilitated through the utilization of ROC curves and AUC values, adding a layer of sophistication to the assessment process [ 17 , 38 ].
- MSE or RMSE: In the realm of regression functions, the metrics of Mean Squared Error (MSE) or Root Mean Squared Error (RMSE) emerge as crucial, providing a nuanced measure of the accuracy of predictions by quantifying the difference between predicted and actual values [ 13 , 31 ].
- R-squared (R2): The extent to which the regression model fits the data is gauged through the lens of R-squared (R2), offering valuable insights into the predictive power of the model [ 49 , 50 ].
- Real-time Performance Measurements: In situations necessitating instantaneous responsiveness, parameters such as response latency, response time, and overall system latency offer a comprehensive evaluation of the system’s real-world applicability [ 26 , 55 ].
6. Present Constraints in the Ongoing Research on Rehabilitation Utilizing EEG with ML and DL Methods
- Noise and Artifacts: Concerns surrounding data quality, preprocessing methodologies, and the standardization of data gathering protocols cast a spotlight on the imperative need to address these aspects to ensure the reliability and consistency of results.
- Small Sample Sizes: The challenges when acquiring high-quality EEG data from groups of patients contribute to the prevalence of small sample sizes, potentially leading to model overfitting and hindering the generalizability of findings.
- Longitudinal EEG Datasets: The scarcity of longitudinal EEG datasets poses a significant hurdle in monitoring progress during neural rehabilitation. A dedicated focus on long-term research is indispensable for comprehensively understanding the effectiveness of diverse approaches and customizing interventions and treatments accordingly.
- Interpretability of Deep Learning: The opaque nature of deep learning models poses challenges in interpreting results, necessitating research that seamlessly combines deep learning methodologies with insights from neuroscience. This integration is crucial for gaining a deeper understanding of the fundamental neurophysiological mechanisms associated with brain rehabilitation.
- Ethical Concerns in Real-Time Applications: While offline analysis dominates several EEG-based brain rehabilitation techniques, the shift towards real-time applications introduces ethical considerations related to patient consent, data ownership, and privacy. Meticulous attention is required to ensure that these issues are addressed with the utmost care, respecting patients’ rights.
- Bridging the Gap Between Research and Clinical Implementation: Despite the strides made in research in neurological rehabilitation utilizing EEG signals, there remains a discernible discrepancy between academic research and the practical implementation of clinical solutions. Efforts to bridge this gap are essential for the seamless translation of research findings into real-world clinical practices.
7. Comparison of EEG Headsets for Rehabilitation Purposes with Various Datasets
8. most significant eeg- and motor imagery-based studies in the last 7 years, 9. conclusions, author contributions, institutional review board statement, informed consent statement, data availability statement, conflicts of interest.
- McFarland, D.J.; Wolpaw, J.R. Brain-computer interfaces for the operation of robotic and prosthetic devices. In Advances in Computers ; Elsevier: Amsterdam, The Netherlands, 2010; Volume 79, pp. 169–187. [ Google Scholar ] [ CrossRef ]
- Dobkin, B.H. Brain-computer interface technology as a tool to augment plasticity and outcomes for neurological rehabilitation. J. Physiol. 2007 , 579 , 637–642. [ Google Scholar ] [ CrossRef ]
- Dodd, K.C.; Nair, V.A.; Prabhakaran, V. Role of the contralesional vs. ipsilesional hemisphere in stroke recovery. Front. Hum. Neurosci. 2017 , 11 , 469. [ Google Scholar ] [ CrossRef ]
- Fouad, M.M.; Amin, K.M.; El-Bendary, N.; Hassanien, A.E. Brain computer interface: A review. In Brain-Computer Interfaces: Current Trends and Applications ; Springer: Berlin/Heidelberg, Germany, 2015; Volume 74, pp. 3–30. [ Google Scholar ] [ CrossRef ]
- Birbaumer, N.; Ghanayim, N.; Hinterberger, T.; Iversen, I.; Kotchoubey, B.; Kübler, A.; Perelmouter, J.; Taub, E.; Flor, H. A spelling device for the paralyzed. Nature 1999 , 398 , 297–298. [ Google Scholar ] [ CrossRef ]
- Mondal, D.; Alagirisamy, M. Brain computer interface (BCI): Mechanism and challenges–A survey. Int. J. Pharm. Res. 2020 , 12 , 2200–2208. [ Google Scholar ] [ CrossRef ]
- Wilson, J.A.; Felton, E.A.; Garell, P.C.; Schalk, G.; Williams, J.C. ECoG factors underlying multimodal control of a brain-computer interface. IEEE Trans. Neural Syst. Rehabil. Eng. 2006 , 14 , 246–250. [ Google Scholar ] [ CrossRef ]
- Rezeika, A.; Benda, M.; Stawicki, P.; Gembler, F.; Saboor, A.; Volosyak, I. Brain–computer interface spellers: A review. Brain Sci. 2018 , 8 , 57. [ Google Scholar ] [ CrossRef ]
- Hwang, H.J.; Kwon, K.; Im, C.H. Neurofeedback-based motor imagery training for brain–computer interface (BCI). J. Neurosci. Methods 2009 , 179 , 150–156. [ Google Scholar ] [ CrossRef ]
- Remsik, A.; Young, B.; Vermilyea, R.; Kiekhoefer, L.; Abrams, J.; Evander Elmore, S.; Schultz, P.; Nair, V.; Edwards, D.; Williams, J.; et al. A review of the progression and future implications of brain–computer interface therapies for restoration of distal upper extremity motor function after stroke. Expert Rev. Med. Devices 2016 , 13 , 445–454. [ Google Scholar ] [ CrossRef ]
- Ramos-Murguialday, A.; Broetz, D.; Rea, M.; Läer, L.; Yilmaz, O.; Brasil, F.L.; Liberati, G.; Curado, M.R.; Garcia-Cossio, E.; Vyziotis, A.; et al. Brain-machine interface in chronic stroke rehabilitation: A controlled study. Ann. Neurol. 2013 , 74 , 100–108. [ Google Scholar ] [ CrossRef ] [ PubMed ]
- Kitchenham, B.; Brereton, O.P.; Budgen, D.; Turner, M.; Bailey, J.; Linkman, S. Systematic literature reviews in software engineering—A systematic literature review. Inf. Softw. Technol. 2009 , 51 , 7–15. [ Google Scholar ] [ CrossRef ]
- Demers, L.; Fuhrer, M.J.; Jutai, J.; Lenker, J.; Depa, M.; De Ruyter, F. A conceptual framework of outcomes for caregivers of assistive technology users. Am. J. Phys. Med. Rehabil. 2009 , 88 , 645–655. [ Google Scholar ] [ CrossRef ] [ PubMed ]
- Jackson, A.; Zimmermann, J.B. Neural interfaces for the brain and spinal cord—Restoring motor function. Nat. Rev. Neurol. 2012 , 8 , 690–699. [ Google Scholar ] [ CrossRef ] [ PubMed ]
- López-Larraz, E.; Sarasola-Sanz, A.; Irastorza-Landa, N.; Birbaumer, N.; Ramos-Murguialday, A. Brain-machine interfaces for rehabilitation in stroke: A review. NeuroRehabilitation 2018 , 43 , 77–97. [ Google Scholar ] [ CrossRef ] [ PubMed ]
- Coscia, M.; Wessel, M.J.; Chaudary, U.; Millán, J.d.R.; Micera, S.; Guggisberg, A.; Vuadens, P.; Donoghue, J.; Birbaumer, N.; Hummel, F.C. Neurotechnology-aided interventions for upper limb motor rehabilitation in severe chronic stroke. Brain 2019 , 142 , 2182–2197. [ Google Scholar ] [ CrossRef ]
- Oguntosin, V.W.; Mori, Y.; Kim, H.; Nasuto, S.J.; Kawamura, S.; Hayashi, Y. Design and validation of exoskeleton actuated by soft modules toward neurorehabilitation—Vision-based control for precise reaching motion of upper limb. Front. Neurosci. 2017 , 11 , 352. [ Google Scholar ] [ CrossRef ]
- Cheng, N.; Phua, K.S.; Lai, H.S.; Tam, P.K.; Tang, K.Y.; Cheng, K.K.; Yeow, R.C.; Ang, K.K.; Guan, C.; Lim, J.H. Brain–computer interface-based soft robotic glove rehabilitation for stroke. IEEE Trans. Biomed. Eng. 2020 , 67 , 3339–3351. [ Google Scholar ] [ CrossRef ] [ PubMed ]
- Schwarz, A.; Höller, M.K.; Pereira, J.; Ofner, P.; Müller-Putz, G.R. Decoding hand movements from human EEG to control a robotic arm in a simulation environment. J. Neural Eng. 2020 , 17 , 036010. [ Google Scholar ] [ CrossRef ] [ PubMed ]
- Diekelmann, S.; Born, J. The memory function of sleep. Nat. Rev. Neurosci. 2010 , 11 , 114–126. [ Google Scholar ] [ CrossRef ]
- Meng, J.; Zhang, S.; Bekyo, A.; Olsoe, J.; Baxter, B.; He, B. Non-invasive electroencephalogram based control of a robotic arm for reach and grasp tasks. Sci. Rep. 2016 , 6 , 38565. [ Google Scholar ] [ CrossRef ]
- Akbari, H.; Sadiq, M.T.; Payan, M.; Esmaili, S.S.; Baghri, H.; Bagheri, H. Depression detection based on geometrical features extracted from SODP shape of EEG signals and binary PSO. Trait. Signal 2021 , 38 , 13–26. [ Google Scholar ] [ CrossRef ]
- Sadiq, M.T.; Yu, X.; Yuan, Z.; Zeming, F.; Rehman, A.U.; Ullah, I.; Li, G.; Xiao, G. Motor imagery EEG signals decoding by multivariate empirical wavelet transform-based framework for robust brain–computer interfaces. IEEE Access 2019 , 7 , 171431–171451. [ Google Scholar ] [ CrossRef ]
- Sharma, R.; Kim, M.; Gupta, A. Motor imagery classification in brain-machine interface with machine learning algorithms: Classical approach to multi-layer perceptron model. Biomed. Signal Process. Control 2022 , 71 , 103101. [ Google Scholar ] [ CrossRef ]
- Antony, J.W.; Piloto, L.; Wang, M.; Pacheco, P.; Norman, K.A.; Paller, K.A. Sleep spindle refractoriness segregates periods of memory reactivation. Current Biol. 2018 , 28 , 1736–1743. [ Google Scholar ] [ CrossRef ] [ PubMed ]
- Akbari, H.; Sadiq, M.T.; Jafari, N.; Too, J.; Mikaeilvand, N.; Cicone, A.; Serra-Capizzano, S. Recognizing seizure using Poincaré plot of EEG signals and graphical features in DWT domain. Bratisl. Med. J. 2022 , 124 , 12–24. [ Google Scholar ] [ CrossRef ]
- Sadiq, M.T.; Yu, X.; Yuan, Z.; Aziz, M.Z. Motor imagery BCI classification based on novel two-dimensional modelling in empirical wavelet transform. Electron. Lett. 2020 , 56 , 1367–1369. [ Google Scholar ] [ CrossRef ]
- LaFleur, K.; Cassady, K.; Doud, A.; Shades, K.; Rogin, E.; He, B. Quadcopter control in three-dimensional space using a noninvasive motor imagery-based brain–computer interface. J. Neural Eng. 2013 , 10 , 046003. [ Google Scholar ] [ CrossRef ] [ PubMed ]
- Wodlinger, B.; Downey, J.E.; Tyler-Kabara, E.C.; Schwartz, A.B.; Boninger, M.L.; Collinger, J.L. Ten-dimensional anthropomorphic arm control in a human brain–machine interface: Difficulties, solutions, and limitations. J. Neural Eng. 2015 , 12 , 016011. [ Google Scholar ] [ CrossRef ] [ PubMed ]
- Huang, B.; Xu, H.; Yuan, M.; Aziz, M.Z.; Yu, X. Exploiting asymmetric EEG signals with EFD in deep learning domain for robust BCI. Symmetry 2022 , 14 , 2677. [ Google Scholar ] [ CrossRef ]
- Cypher, D.; Chevrollier, N.; Montavont, N.; Golmie, N. Prevailing over wires in healthcare environments: Benefits and challenges. IEEE Commun. Mag. 2006 , 44 , 56–63. [ Google Scholar ] [ CrossRef ]
- Sarasola-Sanz, A.; López-Larraz, E.; Irastorza-Landa, N.; Klein, J.; Valencia, D.; Belloso, A.; Morin, F.O.; Spüler, M.; Birbaumer, N.; Ramos-Murguialday, A. An EEG-based brain-machine interface to control a 7-degrees of freedom exoskeleton for stroke rehabilitation. Converging Clin. Eng. Res. Neurorehabilit. II (Biosyst. Biorobot.) 2017 , 15 , 1127–1131. [ Google Scholar ] [ CrossRef ]
- Escolano, C.; Navarro-Gil, M.; Garcia-Campayo, J.; Congedo, M.; Ridder, D.D.D.; Minguez, J. A controlled study on the cognitive effect of alpha neurofeedback training in patients with major depressive disorder. Front. Behav. Neurosci. 2014 , 8 , 296. [ Google Scholar ] [ CrossRef ] [ PubMed ]
- Mortenson, W.; Demers, L.; Fuhrer, M.; Jutai, J.; Lenker, J.; DeRuyter, F. Development and preliminary evaluation of the caregiver assistive technology outcome measure. J. Rehabil. Med. 2015 , 47 , 412–418. [ Google Scholar ] [ CrossRef ] [ PubMed ]
- Chiang, H.S.; Hsiao, K.L.; Liu, L.C. EEG-based detection model for evaluating and improving learning attention. J. Med. Biol. Eng. 2018 , 38 , 847–856. [ Google Scholar ] [ CrossRef ]
- Iturrate, I.; Chavarriaga, R.; Montesano, L.; Minguez, J.; Millán, J.d.R. Teaching brain-machine interfaces as an alternative paradigm to neuroprosthetics control. Sci. Rep. 2015 , 5 , 13893. [ Google Scholar ] [ CrossRef ] [ PubMed ]
- Hamid, H.; Naseer, N.; Nazeer, H.; Khan, M.J.; Khan, R.A.; Khan, U.S. Analyzing classification performance of fNIRS-BCI for gait rehabilitation using deep neural networks. Sensors 2022 , 22 , 1932. [ Google Scholar ] [ CrossRef ] [ PubMed ]
- Buch, E.; Weber, C.; Cohen, L.G.; Braun, C.; Dimyan, M.A.; Ard, T.; Mellinger, J.; Caria, A.; Soekadar, S.; Fourkas, A.; et al. Think to move: A neuromagnetic brain–computer interface (BCI) system for chronic stroke. Stroke 2008 , 39 , 910–917. [ Google Scholar ] [ CrossRef ] [ PubMed ]
- Nakagome, S.; Luu, T.P.; He, Y.; Ravindran, A.S.; Contreras-Vidal, J.L. An empirical comparison of neural networks and machine learning algorithms for EEG gait decoding. Sci. Rep. 2020 , 10 , 4372. [ Google Scholar ] [ CrossRef ]
- Tortora, S.; Ghidoni, S.; Chisari, C.; Micera, S.; Artoni, F. Deep learning-based BCI for gait decoding from EEG with LSTM recurrent neural network. J. Neural Eng. 2020 , 17 , 046011. [ Google Scholar ] [ CrossRef ] [ PubMed ]
- Martini, M.L.; Oermann, E.K.; Opie, N.L.; Panov, F.; Oxley, T.; Yaeger, K. Sensor modalities for brain-computer interface technology: A comprehensive literature review. Neurosurgery 2020 , 86 , E108–E117. [ Google Scholar ] [ CrossRef ]
- Alotaiby, T.; El-Samie, F.E.A.; Alshebeili, S.A.; Ahmad, I. A review of channel selection algorithms for EEG signal processing. EURASIP J. Adv. Signal Process. 2015 , 2015 , 66. [ Google Scholar ] [ CrossRef ]
- Wan, X.; Zhang, K.; Ramkumar, S.; Deny, J.; Emayavaramban, G.; Ramkumar, M.S.; Hussein, A.F. A review on electroencephalogram based brain computer interface for elderly disabled. IEEE Access 2019 , 7 , 36380–36387. [ Google Scholar ] [ CrossRef ]
- Pfurtscheller, G.; Neuper, C. Motor imagery and direct brain-computer communication. Proc. IEEE 2001 , 89 , 1123–1134. [ Google Scholar ] [ CrossRef ]
- Jeunet, C.; Jahanpour, E.; Lotte, F. Why standard brain-computer interface (BCI) training protocols should be changed: An experimental study. J. Neural Eng. 2016 , 13 , 036024. [ Google Scholar ] [ CrossRef ] [ PubMed ]
- Farnsworth, B. Eeg (Electroencephalography): The Complete Pocket Guide ; IMotions, Global HQ: Copenhagen, Denmark, 2019. [ Google Scholar ]
- Shute, V.J.; Zapata-Rivera, D. Adaptive technologies. ETS Res. Rep. Ser. 2007 , 2007 , 34. [ Google Scholar ] [ CrossRef ]
- Norman, S.L.; McFarland, D.J.; Miner, A.; Cramer, S.C.; Wolbrecht, E.T.; Wolpaw, J.R.; Reinkensmeyer, D.J. Controlling pre-movement sensorimotor rhythm can improve finger extension after stroke. J. Neural Eng. 2018 , 15 , 056026. [ Google Scholar ] [ CrossRef ]
- Sadiq, M.T.; Akbari, H.; Siuly, S.; Li, Y.; Wen, P. Alcoholic EEG signals recognition based on phase space dynamic and geometrical features. Chaos Solitons Fractals 2022 , 158 , 112036. [ Google Scholar ] [ CrossRef ]
- Sadiq, M.T.; Yu, X.; Yuan, Z.; Aziz, M.Z.; Rehman, N.u.; Ding, W.; Xiao, G. Motor imagery BCI classification based on multivariate variational mode decomposition. IEEE Trans. Emerg. Top. Comput. Intell. 2022 , 6 , 1177–1189. [ Google Scholar ] [ CrossRef ]
- Yu, X.; Aziz, M.Z.; Sadiq, M.T.; Fan, Z.; Xiao, G. A new framework for automatic detection of motor and mental imagery EEG signals for robust BCI systems. IEEE Trans. Instrum. Meas. 2021 , 70 , 1–12. [ Google Scholar ] [ CrossRef ]
- Altaheri, H.; Muhammad, G.; Alsulaiman, M.; Amin, S.; Altuwaijri, G.; Abdul, W.; Bencherif, M.; Faisal, M. Deep learning techniques for classification of electroencephalogram (EEG) motor imagery (MI) signals: A review. Neural Comput. Appl. 2023 , 35 , 14681–14722. [ Google Scholar ] [ CrossRef ]
- Escolano, C.; Navarro-Gil, M.; Garcia-Campayo, J.; Congedo, M.; Minguez, J. The effects of individual upper alpha neurofeedback in ADHD: An open-label pilot study. Appl. Psychophysiol. Biofeedback 2014 , 39 , 193–202. [ Google Scholar ] [ CrossRef ]
- Ketz, N.; Jones, A.P.; Bryant, N.B.; Clark, V.P.; Pilly, P.K. Closed-loop slow-wave tACS improves sleep-dependent long-term memory generalization by modulating endogenous oscillations. J. Neurosci. 2018 , 38 , 7314–7326. [ Google Scholar ] [ CrossRef ] [ PubMed ]
- Acı, Ç.İ.; Kaya, M.; Mishchenko, Y. Distinguishing mental attention states of humans via an EEG-based passive BCI using machine learning methods. Expert Syst. Appl. 2019 , 134 , 153–166. [ Google Scholar ] [ CrossRef ]
- Kamble, A.; Ghare, P.H.; Kumar, V. Deep-learning-based BCI for automatic imagined speech recognition using SPWVD. IEEE Trans. Instrum. Meas. 2023 , 72 , 1–10. [ Google Scholar ] [ CrossRef ]
- Venkatesh, B.; Anuradha, J. A review of feature selection and its methods. Cybern. Inf. Technol. 2019 , 19 , 3–26. [ Google Scholar ] [ CrossRef ]
- Atyabi, A.; Shic, F.; Naples, A. Mixture of autoregressive modeling orders and its implication on single trial EEG classification. Expert Syst. Appl. 2016 , 65 , 164–180. [ Google Scholar ] [ CrossRef ] [ PubMed ]
- Karaboga, D. An Idea Based on Honey Bee Swarm for Numerical Optimization ; Technical Report, Technical Report-tr06; Erciyes University, Engineering Faculty, Computer Engineering Department: Kayseri, Turkey, 2005. [ Google Scholar ]
- Rakshit, P.; Bhattacharyya, S.; Konar, A.; Khasnobish, A.; Tibarewala, D.N.; Janarthanan, R. Artificial Bee Colony Based Feature Selection for Motor Imagery EEG Data. In Proceedings of the Seventh International Conference on Bio-Inspired Computing: Theories and Applications (BIC-TA 2012); Bansal, J.C., Singh, P., Deep, K., Pant, M., Nagar, A., Eds.; Springer: Gwalior, India, 2013; pp. 127–138. [ Google Scholar ]
- Proulx, C.E.; Beaulac, M.; David, M.; Deguire, C.; Haché, C.; Klug, F.; Kupnik, M.; Higgins, J.; Gagnon, D.H. Review of the effects of soft robotic gloves for activity-based rehabilitation in individuals with reduced hand function and manual dexterity following a neurological event. J. Rehabil. Assistive Technol. Eng. 2020 , 7 , 205566832091813. [ Google Scholar ] [ CrossRef ] [ PubMed ]
- Tabar, Y.R.; Halici, U. A novel deep learning approach for classification of EEG motor imagery signals. J. Neural Eng. 2016 , 14 , 016003. [ Google Scholar ] [ CrossRef ]
- Tang, Z.; Li, C.; Sun, S. Single-trial EEG classification of motor imagery using deep convolutional neural networks. Optik 2017 , 130 , 11–18. [ Google Scholar ] [ CrossRef ]
- Dose, H.; Møller, J.S.; Iversen, H.K.; Puthusserypady, S. An end-to-end deep learning approach to MI-EEG signal classification for BCIs. Expert Syst. Appl. 2018 , 114 , 532–542. [ Google Scholar ] [ CrossRef ]
- Olivas-Padilla, B.E.; Chacon-Murguia, M.I. Classification of multiple motor imagery using deep convolutional neural networks and spatial filters. Appl. Soft Comput. 2019 , 75 , 461–472. [ Google Scholar ] [ CrossRef ]
- Tayeb, Z.; Fedjaev, J.; Ghaboosi, N.; Richter, C.; Everding, L.; Qu, X.; Wu, Y.; Cheng, G.; Conradt, J. Validating Deep Neural Networks for Online Decoding of Motor Imagery Movements from EEG Signals. Sensors 2019 , 19 , 210. [ Google Scholar ] [ CrossRef ] [ PubMed ]
- Tang, X.; Zhao, J.; Fu, W.; Pan, J.; Zhou, H. A novel classification algorithm for MI-EEG based on deep learning. In Proceedings of the 2019 IEEE 8th Joint International Information Technology and Artificial Intelligence Conference (ITAIC), Chongqing, China, 24–26 May 2019; pp. 606–611. [ Google Scholar ]
- Qiao, W.; Bi, X. Deep spatial-temporal neural network for classification of EEG-based motor imagery. In Proceedings of the 2019 International Conference on Artificial Intelligence and Computer Science, Wuhan, China, 12–13 July 2019; pp. 265–272. [ Google Scholar ]
- Song, Y.; Wang, D.; Yue, K.; Zheng, N.; Shen, Z.J.M. EEG-based motor imagery classification with deep multi-task learning. In Proceedings of the 2019 International Joint Conference on Neural Networks (IJCNN), Budapest, Hungary, 14–19 July 2019; pp. 1–8. [ Google Scholar ]
- Amin, S.U.; Alsulaiman, M.; Muhammad, G.; Mekhtiche, M.A.; Shamim Hossain, M. Deep Learning for EEG motor imagery classification based on multi-layer CNNs feature fusion. Future Gener. Comput. Syst. 2019 , 101 , 542–554. [ Google Scholar ] [ CrossRef ]
- Alazrai, R.; Abuhijleh, M.; Alwanni, H.; Daoud, M.I. A Deep Learning Framework for Decoding Motor Imagery Tasks of the Same Hand Using EEG Signals. IEEE Access 2019 , 7 , 109612–109627. [ Google Scholar ] [ CrossRef ]
- Li, F.; He, F.; Wang, F.; Zhang, D.; Xia, Y.; Li, X. A Novel Simplified Convolutional Neural Network Classification Algorithm of Motor Imagery EEG Signals Based on Deep Learning. Appl. Sci. 2020 , 10 , 1605. [ Google Scholar ] [ CrossRef ]
- Miao, M.; Hu, W.; Yin, H.; Zhang, K. Spatial-Frequency Feature Learning and Classification of Motor Imagery EEG Based on Deep Convolution Neural Network. Comput. Math. Methods Med. 2020 , 2020 , 1981728. [ Google Scholar ] [ CrossRef ] [ PubMed ]
- Yang, J.; Ma, Z.; Wang, J.; Fu, Y. A Novel Deep Learning Scheme for Motor Imagery EEG Decoding Based on Spatial Representation Fusion. IEEE Access 2020 , 8 , 202100–202110. [ Google Scholar ] [ CrossRef ]
- Ieracitano, C.; Morabito, F.C.; Hussain, A.; Mammone, N. A hybrid-domain deep learning-based BCI for discriminating hand motion planning from EEG sources. Int. J. Neural Syst. 2021 , 31 , 2150038. [ Google Scholar ] [ CrossRef ]
- Zhang, C.; Kim, Y.K.; Eskandarian, A. EEG-inception: An accurate and robust end-to-end neural network for EEG-based motor imagery classification. J. Neural Eng. 2021 , 18 , 046014. [ Google Scholar ] [ CrossRef ] [ PubMed ]
- Deng, X.; Zhang, B.; Yu, N.; Liu, K.; Sun, K. Advanced TSGL-EEGNet for Motor Imagery EEG-Based Brain-Computer Interfaces. IEEE Access 2021 , 9 , 25118–25130. [ Google Scholar ] [ CrossRef ]
- Cho, J.H.; Jeong, J.H.; Lee, S.W. NeuroGrasp: Real-Time EEG Classification of High-Level Motor Imagery Tasks Using a Dual-Stage Deep Learning Framework. IEEE Trans. Cybern. 2022 , 52 , 13279–13292. [ Google Scholar ] [ CrossRef ]
- Tiwari, S.; Goel, S.; Bhardwaj, A. MIDNN-a classification approach for the EEG based motor imagery tasks using deep neural network. Appl. Intell. 2022 , 52 , 4824–4843. [ Google Scholar ] [ CrossRef ]
- Huang, E.; Zheng, X.; Fang, Y.; Zhang, Z. Classification of Motor Imagery EEG Based on Time-Domain and Frequency-Domain Dual-Stream Convolutional Neural Network. IRBM 2022 , 43 , 107–113. [ Google Scholar ] [ CrossRef ]
- Huang, W.; Chang, W.; Yan, G.; Yang, Z.; Luo, H.; Pei, H. EEG-based motor imagery classification using convolutional neural networks with local reparameterization trick. Expert Syst. Appl. 2022 , 187 , 115968. [ Google Scholar ] [ CrossRef ]
- Zhu, H.; Forenzo, D.; He, B. On the Deep Learning Models for EEG-Based Brain-Computer Interface Using Motor Imagery. IEEE Trans. Neural Syst. Rehabil. Eng. 2022 , 30 , 2283–2291. [ Google Scholar ] [ CrossRef ]
- Mattioli, F.; Porcaro, C.; Baldassarre, G. A 1D CNN for high accuracy classification and transfer learning in motor imagery EEG-based brain-computer interface. J. Neural Eng. 2022 , 18 , 066053. [ Google Scholar ] [ CrossRef ]
- Du, Y.; Liu, J. IENet: A robust convolutional neural network for EEG based brain-computer interfaces. J. Neural Eng. 2022 , 19 , 036031. [ Google Scholar ] [ CrossRef ]
- Li, Y.; Zhang, Q.; Chen, W. Adaptive CNN for EEG classification in stroke rehabilitation. J. Neurosci. Methods 2023 , 275 , 112–120. [ Google Scholar ]
- Wang, Z.; Liu, S.; Zhou, Y. Attention-based CNN for EEG motor imagery classification. IEEE Trans. Neural Syst. Rehabil. Eng. 2023 , 31 , 1365–1373. [ Google Scholar ]
- Zhang, L.; Wang, H.; Li, J. Graph-CNN for spatial feature learning in EEG classification. Med. Biol. Eng. Comput. 2023 , 61 , 451–459. [ Google Scholar ]
- Xu, Y.; Zhang, W.; Chen, S. Hybrid CNN-RNN model for EEG motor imagery classification. Front. Neurosci. 2024 , 18 , 127–135. [ Google Scholar ]
- Chen, L.; Liu, Q.; Yang, H. Transformer-based model for EEG classification in stroke rehabilitation. IEEE Trans. Biomed. Eng. 2024 , 71 , 1461–1470. [ Google Scholar ]
Click here to enlarge figure
Brand | Model | Number of Channels | Intended Use |
---|---|---|---|
Emotiv | Emotiv EPOCH | 5–14 channels | Used in research and for personal use |
Emotiv Insight | |||
BIOPAC systems | EEG100C | 16 channels | Used in sleep studies and evoked responses |
OpenBCI | OpenBCI 32-bit | 4–21 channels | Used in BCI and biosensing |
OpenBCI Cyton | |||
OpenBCI Ganglion | |||
Ultracortex BCI | |||
Narosky | Brainwave | 1 channel | Used in neurogaming and meditation |
Mindflex | |||
Mindwave |
References | Year | Dataset | DL Model | Classification Results |
---|---|---|---|---|
[ ] | 2016 | BCI competition IV dataset 2b | CNN+SAE | 72.40% |
[ ] | 2017 | Collected (109 subjects) | CNN | 86.41% |
[ ] | 2018 | Physionet EEG MI Dataset | CNN | 80.38% |
[ ] | 2019 | BCI competition IV dataset 2a | CNN | 82.09% |
[ ] | 2019 | BCI competition IV dataset 2b | CNN | 77.72% |
[ ] | 2019 | BCI competition data IV 2a | CNN+SAE | 79.90% |
[ ] | 2019 | BCI competition data IV 2a | CNN+Bi-GRU | 76.62% |
[ ] | 2019 | BCI competition data IV 2a | CNN | 73.40% |
[ ] | 2019 | BCI competition data IV 2a | CNN | 75.7% |
[ ] | 2019 | Collected (22 subjects) | CNN | 73.70% |
[ ] | 2020 | BCI Competition IV 2b | CNN | 83.20% |
[ ] | 2020 | BCI competition IVa, right index finger MI dataset | CNN | 90.00% |
[ ] | 2020 | BCI competition IV dataset 1 | CNN | 86.40% |
[ ] | 2021 | 15 subjects | CNN | 76.21% |
[ ] | 2021 | BCI Competition IV dataset 2a and 2b | CNN | 88.40% |
[ ] | 2021 | BCI Competition IV 2a, III | CNN | 85.30% |
[ ] | 2021 | Collected (12 subjects) | Bi-LSTM | 68.00% |
[ ] | 2021 | BCI competition V dataset, Emotiv dataset | CNN | 72.51% and 72% |
[ ] | 2021 | BCI competition IV dataset 2a | CNN | 90.00% |
[ ] | 2022 | PhysioNet dataset | CNN | 92.00% |
[ ] | 2022 | Med-62 | ConvNet | 72.66% |
[ ] | 2022 | EEG Motor Movement Dataset V 1.0.0 | CNN | 99.38% |
[ ] | 2022 | MRCP | CNN | 91.00% |
[ ] | 2023 | BCI competition IV dataset 2a | Adaptive CNN | 93.20% |
[ ] | 2023 | Collected (30 subjects) | Attention-based CNN | 88.75% |
[ ] | 2023 | BCI competition IV dataset 2b | Graph-CNN | 89.60% |
[ ] | 2024 | PhysioNet EEG MI Dataset | Hybrid CNN-RNN | 94.50% |
[ ] | 2024 | BCI competition IV dataset 2a | Transformer-based Model | 92.30% |
The statements, opinions and data contained in all publications are solely those of the individual author(s) and contributor(s) and not of MDPI and/or the editor(s). MDPI and/or the editor(s) disclaim responsibility for any injury to people or property resulting from any ideas, methods, instructions or products referred to in the content. |
Share and Cite
Elashmawi, W.H.; Ayman, A.; Antoun, M.; Mohamed, H.; Mohamed, S.E.; Amr, H.; Talaat, Y.; Ali, A. A Comprehensive Review on Brain–Computer Interface (BCI)-Based Machine and Deep Learning Algorithms for Stroke Rehabilitation. Appl. Sci. 2024 , 14 , 6347. https://doi.org/10.3390/app14146347
Elashmawi WH, Ayman A, Antoun M, Mohamed H, Mohamed SE, Amr H, Talaat Y, Ali A. A Comprehensive Review on Brain–Computer Interface (BCI)-Based Machine and Deep Learning Algorithms for Stroke Rehabilitation. Applied Sciences . 2024; 14(14):6347. https://doi.org/10.3390/app14146347
Elashmawi, Walaa H., Abdelrahman Ayman, Mina Antoun, Habiba Mohamed, Shehab Eldeen Mohamed, Habiba Amr, Youssef Talaat, and Ahmed Ali. 2024. "A Comprehensive Review on Brain–Computer Interface (BCI)-Based Machine and Deep Learning Algorithms for Stroke Rehabilitation" Applied Sciences 14, no. 14: 6347. https://doi.org/10.3390/app14146347
Article Metrics
Article access statistics, further information, mdpi initiatives, follow mdpi.
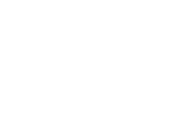
Subscribe to receive issue release notifications and newsletters from MDPI journals
Academia.edu no longer supports Internet Explorer.
To browse Academia.edu and the wider internet faster and more securely, please take a few seconds to upgrade your browser .
Enter the email address you signed up with and we'll email you a reset link.
- We're Hiring!
- Help Center
"PAPER RECYCLING MACHINE"
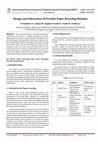
- We're Hiring!
- Help Center
- Find new research papers in:
- Health Sciences
- Earth Sciences
- Cognitive Science
- Mathematics
- Computer Science
- Academia ©2024
Grab your spot at the free arXiv Accessibility Forum
Help | Advanced Search
Computer Science > Machine Learning
Title: detecting throat cancer from speech signals using machine learning: a scoping literature review.
Abstract: Introduction: Cases of throat cancer are rising worldwide. With survival decreasing significantly at later stages, early detection is vital. Artificial intelligence (AI) and machine learning (ML) have the potential to detect throat cancer from patient speech, facilitating earlier diagnosis and reducing the burden on overstretched healthcare systems. However, no comprehensive review has explored the use of AI and ML for detecting throat cancer from speech. This review aims to fill this gap by evaluating how these technologies perform and identifying issues that need to be addressed in future research. Materials and Methods: We conducted a scoping literature review across three databases: Scopus,Web of Science, and PubMed. We included articles that classified speech using machine learning and specified the inclusion of throat cancer patients in their data. Articles were categorized based on whether they performed binary or multi-class classification. Results: We found 27 articles fitting our inclusion criteria, 12 performing binary classification, 13 performing multi-class classification, and two that do both binary and multiclass classification. The most common classification method used was neural networks, and the most frequently extracted feature was mel-spectrograms. We also documented pre-processing methods and classifier performance. We compared each article against the TRIPOD-AI checklist, which showed a significant lack of open science, with only one article sharing code and only three using open-access data. Conclusion: Open-source code is essential for external validation and further development in this field. Our review indicates that no single method or specific feature consistently outperforms others in detecting throat cancer from speech. Future research should focus on standardizing methodologies and improving the reproducibility of results.
Comments: | 15 pages, 10 figures, 5 tables |
Subjects: | Machine Learning (cs.LG); Sound (cs.SD); Audio and Speech Processing (eess.AS) |
Cite as: | [cs.LG] |
(or [cs.LG] for this version) | |
Focus to learn more arXiv-issued DOI via DataCite |
Submission history
Access paper:.
- HTML (experimental)
- Other Formats

References & Citations
- Google Scholar
- Semantic Scholar
BibTeX formatted citation

Bibliographic and Citation Tools
Code, data and media associated with this article, recommenders and search tools.
- Institution
arXivLabs: experimental projects with community collaborators
arXivLabs is a framework that allows collaborators to develop and share new arXiv features directly on our website.
Both individuals and organizations that work with arXivLabs have embraced and accepted our values of openness, community, excellence, and user data privacy. arXiv is committed to these values and only works with partners that adhere to them.
Have an idea for a project that will add value for arXiv's community? Learn more about arXivLabs .
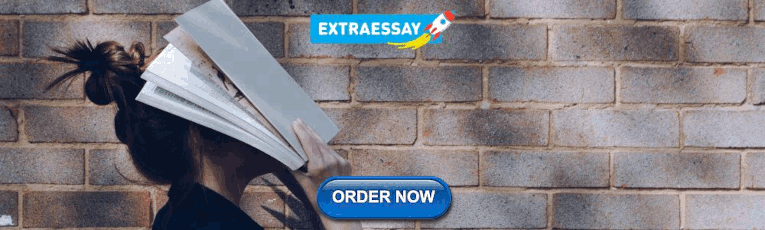
IMAGES
VIDEO
COMMENTS
M. A. Olutoye [2] developed a paper recycling system. includes main six components the hydropulper, disc. refiner, the he ad box, the driers, the rollers, and the felt. conveyor. The machine was ...
3.1.1. Analysis of systematic literature review publications. In the review paper, Ilgin and Gupta (Citation 2010) explored the area of Environmentally Conscious Manufacturing and Product Recovery. After going through more than 540 published papers they divided the entire field into four major categories namely, generation of eco-friendly ...
A discussion is currently under way in the literature on the sustainable benefits of recycling material, particularly paper, which has high global consumption and polluting capacity. Optimized planning of waste paper recycling networks stimulates sustainable processing efficiency, motivating the investigation of quantitative methods to guide decision-making. The objective of this article is to ...
Considering this, a detailed literature review was conducted on a small-scale automated paper recycling machine that is conveniently used in organizations. The recycling of paper is possible to overcome of this problem, recycling the waste paper is best way to protect the wastage of paper instead of disposing off.
The development of an automatic paper-recycling machine is much cheaper as compared to machines in recycling industries. The fabricated machine can serve dual purposes, it can be manned permanently at a stationary position or it could be shifted from one place to another as the case may be. Fig. 5: Paper Recycling Machine
Processing: Papermaking. Papermaking is the final step in the paper recycling process. The ready clean pulp can be used for the paper recycling process. The new made fiber used as alone or to need ...
Abstract—it is literature review paper on Design & Fabrication of manual operated paper recycling machine. In any big institution, especially educational institutions like ... scale manually-operated paper recycling machine has been designed which can recycle waste paper for various productive purposes. The fabricated machine can serve dual
recovered paper . and. recycling rate. For this reason, all of the terms used here have been denoted in italics. Many terms can be used to define the same product. In the literature, terms like . discarded paper, paper for recycling, paper stock, refuse paper, recovered paper, scrap paper, secondary paper, used paper . and . waste paper
To achieve this, considering the design and fabrication of automated paper recycling machine, to use in schools and colleges. In the current paperwork, a detailed review on the paper recycling machine especially used in educational institutions like schools or colleges is described.
However, there is limited literature on how to choose between monopolistic and competitive recycling modes by considering BT. This paper uses a game involving a manufacturer, a retailer, and a third-party recycler (TPR) in a closed-loop supply chain (CLSC). The retailer can recycle on itself and compete with the TPR for recycling used products.
II LITERATURE REVIEW Dibakar Bhattacharjee et al.: Automatic recycling machine will increase the turnover ratio significantly and the use of plate heater will be an advantage to heat in wet condition.[1] Kostyantyn Pivnenko et al.: In this literature we have seen that paper product
LITERATURE REVIEW. The machine consists of motor, spur gear, bearings, structural frame, cutter and shaft (single, dual etc). The machine frame is can be built using mild steel and High Carbon steel used for cutter tip preparation. cutters are mounted on shafts, which rotate parallel driven by a gear drive arrangement.
A global, comprehensive review of terms and definitions related to paper recycling was conducted in this article. Terms and definitions related to paper recycling have varied in the course of time.
Shredder machine for plastic recycling: A review paper. J H Wong 1, M J H Gan 1,2, B L Chua 1, M Gakim 1 and N J Siambun 1,2. Published under licence by IOP Publishing Ltd IOP Conference Series: Materials Science and Engineering, Volume 1217, Material and Energy Engineering for Sustainable Advancement (MEESA 2021) 29th-30th September 2021, Kota Kinabalu, Sabah, Malaysia Citation J H Wong et al ...
- This paper deals with the development of waste paper recycling machine. It encompasses the recycle and reuse of large amount of waste papers generated in institutes. ... The Effects of Paper Recycling and its Environmental Impact(SLOVAKIA). [9] Kirabira John Baptist et-al(2013),A Review on Pulp Manufacture from Non Wood Plant Materials(UK ...
This paper presents a comprehensive literature review on the evolution of data-lake technology, with a particular focus on data-lake architectures. By systematically examining the existing body of research, we identify and classify the major types of data-lake architectures that have been proposed and implemented over time. The review highlights key trends in the development of data-lake ...
The textile industry of Pakistan, a major contributor to its economy, is encountering serious sustainability challenges, akin to many developing nations. The literature is replete with assertions on the importance of sustainability; however, cross-functional research is sporadic. This paper theoretically links Green HRM (GHRM) practices, Green SCM (GSCM) practices, and Triple Bottom Line (TBL ...
LITERATURE REVIEW Reverse Vending Machines (RVMs) have gained increasing attention as innovative tools to promote recycling and sustainable waste management practices. This literature review aims to provide an overview of the existing body of research on the challenges hindering the widespread adoption of RVMs.
Plastic materials are less recycled compared to other materials consumed in large quantities, such as glass, paper, ceramics, and aluminium [15, 16].Total plastic recycling is known as a complex technique due to its multistage processing, disposal and sorting, distribution, and use [17, 18].Plastic wastes can be recycled chemically, mechanically, or thermally (Table 1).
Improving the efficiency of resource recovery by appropriate sorting and collection systems is necessary for successful plastic recycling. Therefore, this study proposed a three-step optimization process of a reverse vending machine (RVM), a small automatic recyclable waste sorter/collector system, for acquiring an optimal design and enhanced ...
Tokyo 108-0023, Japan. 2 School of Environment and Society, Tokyo Institute of Technology, 3-3-6 Shibaura, Minato-ku, Tokyo 108-0023, Japan. * Correspondence: [email protected] or tsuchimoto ...
The two terms in the calculation formula for the recycling rate, namely paper recycling and paper consumption, will be analysed in detail next. 3.1. Paper recycling. Twelve different definitions for paper recycling are listed in Table 1. These definitions were extracted from the existing literature as part of an extensive literature review that ...
This literature review explores the pivotal role of brain-computer interface (BCI) technology, coupled with electroencephalogram (EEG) technology, in advancing rehabilitation for individuals with damaged muscles and motor systems. This study provides a comprehensive overview of recent developments in BCI and motor control for rehabilitation, emphasizing the integration of user-friendly ...
A manually operated paper-recycling machine was designed and fabricated. This was done to enable waste paper conversion into useful product. The fabricated plant consists of six major component units that include the disc refiner, the hydropulper, the head box, the felt conveyor, the driers and the rollers.
Open-loop recy cling is currently the dominating form. of fabric waste recycling with a major f ocus on the construction and building sector (34%). This paper also identifies and. discusses six ...
Introduction: Cases of throat cancer are rising worldwide. With survival decreasing significantly at later stages, early detection is vital. Artificial intelligence (AI) and machine learning (ML) have the potential to detect throat cancer from patient speech, facilitating earlier diagnosis and reducing the burden on overstretched healthcare systems. However, no comprehensive review has ...
In the plastic re cycling process, the pr imary machine used is a shredde r. machine. The machine is used to cut waste plastic into tiny pieces for easy handling, easier to melt and. turn into ...
A Systematic Literature Review for the Recycling and Reuse of W asted Clothing Xiufen Xie 1 , Yan Hong 1, * , Xianyi Zeng 2 , Xiaoqun Dai 1 and Melissa Wagner 3