Advertisement
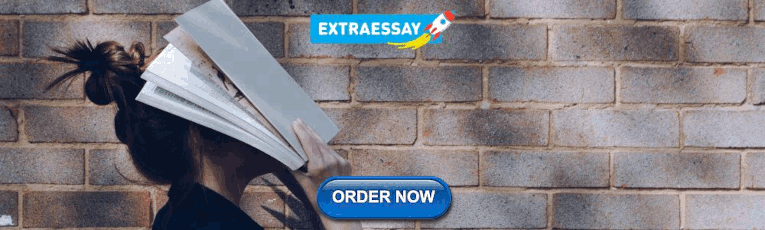
Automatic Speech Emotion Recognition: a Systematic Literature Review
- Published: 07 April 2024
- Volume 27 , pages 267–285, ( 2024 )
Cite this article
- Haidy H. Mustafa ORCID: orcid.org/0009-0006-3490-8596 2 ,
- Nagy R. Darwish 2 &
- Hesham A. Hefny 1
454 Accesses
Explore all metrics
Automatic Speech Emotion Recognition (ASER) has recently garnered attention across various fields including artificial intelligence, pattern recognition, and human–computer interaction. However, ASER encounters numerous challenges such as a shortage of diverse datasets, appropriate feature selection, and suitable intelligent recognition techniques. To address these challenges, a systematic literature review (SLR) was conducted following established guidelines. A total of 60 primary research papers spanning from 2011 to 2023 were reviewed to investigate, interpret, and analyze the related literature by addressing five key research questions. Despite being an emerging area with applications in real-life scenarios, ASER still grapples with limitations in existing techniques. This SLR provides a comprehensive overview of existing techniques, datasets, and feature extraction tools in the ASER domain, shedding light on the weaknesses of current research studies. Additionally, it outlines a list of limitations for consideration in future work.
This is a preview of subscription content, log in via an institution to check access.
Access this article
Subscribe and save.
- Get 10 units per month
- Download Article/Chapter or eBook
- 1 Unit = 1 Article or 1 Chapter
- Cancel anytime
Price includes VAT (Russian Federation)
Instant access to the full article PDF.
Rent this article via DeepDyve
Institutional subscriptions
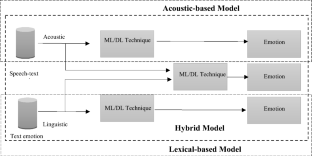
Similar content being viewed by others
A Survey of Human Emotion Recognition Using Speech Signals: Current Trends and Future Perspectives
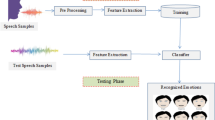
Speech Emotion Recognition Systems: A Comprehensive Review on Different Methodologies
Speech emotion recognition research: an analysis of research focus, explore related subjects.
- Artificial Intelligence
Data availability
Most of the datasets and tools presented in this study are available on the internet.
“audeering,” audEERING ® (2023). Retrieved May 23, 2023, from https://www.audeering.com/research/opensmile/
Abdel-Hamid, L. (2020). Egyptian Arabic speech emotion recognition using prosodic, spectral and wavelet features. Speech Communication, 122 , 19–30.
Article Google Scholar
Aldeneh, Z., & Provost, E. M. (2017). Using regional saliency for speech emotion recognition. In 2017 IEEE international conference on acoustics, speech and signal processing (ICASSP) , New Orleans, LA, USA.
Al-Faham, A., & Ghneim, N. (2016). Towards enhanced Arabic speech emotion recognition: Comparison between three methodologies. Asian Journal of Science and Technology, 7 (3), 2665–2669.
Google Scholar
AL-Sarayreh, S., Mohamed, A., & Shaalan, K. (2023). Challenges and solutions for Arabic natural language processing in social media. In Hassanien, A.E., Zheng, D., Zhao, Z., & Fan, Z. (Eds) Business intelligence and information technology. 2022 . Smart innovation, systems and technologies 358. Springer. https://doi.org/10.1007/978-981-99-3416-4_24
An, X. D., & Ruan, Z. (2021). Speech emotion recognition algorithm based on deep learning algorithm fusion of temporal and spatial features. Journal of Physics: Conference Series, 1861 (1), 012064.
Anusha, R., Subhashini, P., Jyothi, D., Harshitha, P., Sushma, J., & Mukesh, N. (2021). Speech emotion recognition using machine learning. In 2021 5th international conference on trends in electronics and informatics (ICOEI) , Tirunelveli, India.
Aouani, H., & Ayed, Y. B. (2020). Speech emotion recognition using deep learning. In 24th international conference on knowledge-based and intelligent information & engineering , Verona, Italy.
Atmaja, B. T., & Sasou, A. (2022a). Evaluating self-supervised speech representations for speech emotion recognition. IEEE Access, 10 , 124396–124407.
Atmaja, B. T., & Sasou, A. (2022b). Effects of data augmentations on speech emotion recognition. Sensors (Basel), 22 (16), 5941.
Atmaja, B. T., & Sasou, A. (2022c). Sentiment analysis and emotion recognition from speech using universal speech representations. Sensors, 22 (17), 6369.
Atmaja, B. T., Shirai, K., & Akagi, M. (2019). Speech emotion recognition using speech feature and word embedding. In Pacific signal and information processing association annual summit and conference (APSIPA ASC) , Lanzhou, China.
Badshah, A. M., Ahmad, J., Rahim, N., & Baik, S. W. (2017). Speech emotion recognition from spectrograms with deep convolutional neural network. In 2017 international conference on platform technology and service (PlatCon) , Busan, Korea (South).
Bertero, D., & Fung, P. (2017). A first look into a convolutional neural network for speech emotion detection. In IEEE international conference on acoustics, speech and signal processing (ICASSP) , New Orleans, LA, USA.
Bojanić, M., Delić, V., & Karpov, A. (2020). Call redistribution for a call center based on speech emotion recognition. Applied Sciences, 10 (13), 4653.
Cho, J., & Kato, S. (2011). Detecting emotion from voice using selective Bayesian pairwise classifiers. In 2011 IEEE symposium on computers & informatics , Kuala Lumpur, Malaysia.
Dangol, R., Alsadoon, A., Prasad, P. W. C., Seher, I., & Alsadoon, O. H. (2020). Speech emotion recognition usingconvolutional neural network and long-short term memory. Multimedia Tools and Applications, 79 , 32917–32934.
Dasgupta, P. B. (2017). Detection and analysis of human emotions through voice and speech pattern processing. International Journal of Computer Trends and Technology (IJCTT), 52 (1), 1–3.
Deng, J., Xu, X., Zhang, Z., Frühholz, S., & Schuller, B. (2017). Semisupervised autoencoders for speech emotion recognition. IEEE/ACM Transactions on Audio, Speech, and Language Processing, 26 (1), 31–43.
Dennis, J., Dat, T. H., & Li, H. (2011). Spectrogram image feature for sound event classification in mismatched conditions. Signal Processing Letters, 18 (2), 130–133.
Dissanayake, V., Zhang, H., Billinghurst, M., & Nanayakkara, S. (2020). Speech emotion recognition ‘in the wild’ using an Autoencoder. In INTERSPEECH 2020 , Shanghai, China.
Er, M. B. (2020). A novel approach for classification of speech emotions based on deep and acoustic features. IEEE Access, 8 , 221640–221653.
Eskimez, S. E., Imade, K., Yang, N., Sturge-Apple, M., Duan, Z., & Heinzelman, W. (2016). Emotion classification: How does an automated system compare to Naive human coders? In IEEE international conference on acoustics, speech and signal processing (ICASSP) , Shanghai, China.
Etienne, C., Fidanza, G., Petrovskii, A., Devillers, L., & Schmauch, B. (2018). CNN+LSTM architecture for speech emotion recognition with data augmentation. In Workshop on speech, music and mind (SMM 2018) , Hyderabad, India.
Evgeniou, T. P. M. (2001). Machine learning and its applications. In Support vector machines: Theory and applications (ACAI 1999) . Lecture notes in computer science, (vol. 2049). Springer.
Feugère, L., Doval, B., & Mifune, M.-F. (2015). Using pitch features for the characterization of intermediate vocal productions. In 5th international workshop on folk music analysis (FMA) , Paris.
Flower, T. M. L., & Jaya, T. (2022). Speech emotion recognition using Ramanujan Fourier transform. Applied Acoustics, 201 , 109133.
Gamage, K. W., Sethu, V., & Ambikairajah, E. (2017). Salience based lexical features for emotion recognition. In 2017 IEEE international conference on acoustics, speech and signal processing (ICASSP 2017) , New Orleans, LA, USA,
Ghosh, A., Sufian, A., Sultana, F., Chakrabarti, A., & De, D. (2020). Fundamental concepts of convolutional neural network. In Recent trends and advances in artificial intelligence and Internet of Things. Intelligent systems reference . Springer.
“Google Cloud”. Retrieved May 23, 2023, from https://cloud.google.com/speech-to-text/?utm_source=google&utm_medium=cpc&utm_campaign=emea-eg-all-en-dr-bkws-all-all-trial-e-gcp-1011340&utm_content=text-ad-none-any-DEV_c-CRE_495056377393-ADGP_Hybrid%20%7C%20BKWS%20-%20EXA%20%7C%20Txt%20~%20AI%20%26%20M
Gupta, P., & Rajput, N. (2007). Two-stream emotion recognition for call center monitoring. In INTERSPEECH , Antwerp, Belgium.
Hadjadji, I., Falek, L., Demri, L., & Teffahi, H. (2019). Emotion recognition in Arabic speech. In International conference on advanced electrical engineering (ICAEE) , Algiers, Algeria.
Jithendran, A., Pranav Karthik, P., Santhosh, S., & Naren, J. (2020). Emotion recognition on e-learning community to improve the learning outcomes using machine learning concepts: A pilot study . Springer.
Book Google Scholar
Kacur, J., Puterka, B., Pavlovicova, J., & Oravec, M. (2021). On the speech properties and feature extraction methods in speech emotion recognition. Sensors, 21 (5), 1888.
Kannan, V., & Rajamohan, H. R. (2019). Emotion recognition from speech, vol. 10458. arXiV:abs/1912.
Kanwal, S., Asghar, S., & Ali, H. (2022). Feature selection enhancement and feature space visualization for speech-based emotion recognition. PeerJ Computer Science, 8 , e1091.
Khanna, P., & Sasikumar, M. (2011). Recognizing emotions from human speech. In S. J. Pise (Ed.), Thinkquest . Springer.
Kim, E., & Shin, J. W. (2019). DNN-based emotion recognition based on bottleneck acoustic features and lexical features. In 2019 IEEE international conference on acoustics, speech and signal processing (ICASSP) , Brighton, UK.
Kim, E., Song, H., & Shin, J. W. (2020a). Affective latent representation of acoustic and lexical features for emotion recognition. Sensors (Basel), 20 (9), 2614.
Kim, E., Song, H., & Shin, J. W. (2020b). Affective latent representation of acoustic and lexical features for emotion recognition. Sensors, 20 (9), 2614.
Kitchenham, B., & Charters, S. (2007). Guidelines for performing systematic literature reviews in software engineering version 2.3. Engineering, 45 (4), 1051.
Klaylat, S., Osman, Z., Hamandi, L., & Zantout, R. (2018). Emotion recognition in Arabic speech. Analog Integrated Circuits and Signal Processing, 96 , 337–351.
Le, Q. V. (2015). Autoencoders, convolutional neural networks and recurrent neural networks . Google Inc.
Lee, C. M., & Narayanan, S. S. (2005). Toward detecting emotions in spoken dialogs. IEEE Transactions on Speech and Audio Processing, 13 (2), 293–303.
Lee, Y., Yoon, S., & Jung, K. (2018). Multimodal speech emotion recognition using audio and text. In 2018 IEEE spoken language technology workshop (SLT), Athens, Greece.
Li, B., Dimitriadis, D., & Stolcke, A. (2019). Acoustic and lexical sentiment analysis for customer service calls. In IEEE international conference on acoustics, speech and signal processing (ICASSP) , Brighton, UK.
Li, G. M., Liu, N., & Zhang, J.-A. (2022). Speech emotion recognition based on modified relief. Sensors (Basel), 22 (21), 8152.
Li, Y., Zhang, Y.-T., Ng, G. W., Leau, Y.-B., & Yan, H. (2023). A deep learning method using gender-specific features for emotion recognition: A deep learning method using gender-specific features for emotion recognition. Sensors, 23 (3), 1355–1356.
“librosa”. Retrieved May 23, 2023, from https://librosa.org/doc/latest/index.html
Lieskovska, E., Jakubec, M., & Jarina, R. (2022). RNN with improved temporal modeling for speech emotion recognition. In 2022 32nd international conference radioelektronika (RADIOELEKTRONIKA) , Kosice, Slovakia.
Liu, M. (2022). English speech emotion recognition method based on speech recognition. International Journal of Speech Technology, 25 , 391–398.
Liu, N., Zhang, B., Liu, B., Shi, J., Yang, L., Li, Z., & Zhu, J. (2021). Transfer subspace learning for unsupervised cross-corpus speech emotion recognition. IEEE Access, 9 , 95925–95937.
Lun, X., Wang, F., & Yu, Z. (2021). Human speech emotion recognition via feature selection and analyzing. Journal of Physics Conference Series, 1748 (4), 042008.
Maghilnan, S., & Kumar, M. R. (2017). Sentiment analysis on speaker specific speech data. In 2017 international conference on intelligent computing and control (I2C2) , Coimbatore, India.
Majeed, S. A., Husain, H., Samad, S. A., & Idbeaa, T. F. (2015). Mel frequency cepstral coefficients (MFCC) feature extraction enhancement in theapplication of speech recognition: A comparison study. Journal of Theoretical and Applied Information Technology, 79 (1), 38.
“MathWorks”. Retrieved May 23, 2023, from https://www.mathworks.com/products/matlab.html
Meddeb, M., Karray, H., & Alimi, A. M. (2016). Automated extraction of features from arabic emotional speech corpus. International Journal of Computer Information Systems and Industrial Management Applications, 8 , 184–194.
Mefiah, A., Alotaibi, Y. A., & Selouani, S.-A. (2015). Arabic speaker emotion classification using rhythm metrics and neural networks. In 2015 23rd European signal processing conference (EUSIPCO) , Nice, France.
Meftah, A., Selouani, S.-A., & Alotaibi, Y. A. (2015). Preliminary Arabic speech emotion classification. In 2014 IEEE international symposium on signal processing and information technology (ISSPIT) , Noida, India.
Meftah, A., Qamhan, M., Alotaibi, Y. A., & Zakariah, M. (2020). Arabic speech emotion recognition using KNN and KSU emotions corpus. International Journal of Simulation -- Systems, Science & Technology, 21 (2), 1–5.
Mehmet, B., & Kaya, O. (2020). Speech emotion recognition: Emotional models, databases, features, preprocessing methods, supporting modalities, and classifiers. Speech Communication, 116 , 56–76.
Murugan, H. (2020). Speech emotion recognition using CNN. International Journal of Psychosocial Rehabilitation . https://doi.org/10.37200/IJPR/V24I8/PR280260
Naziya, S., & Ratnadeep, R. D. (2016). Speech recognition system—a review. IOSR Journal of Computer Engineering, 18 (4), 1–9.
Pengfei, X., Houpan, Z., & Weidong, Z. (2020). PAD 3-D speech emotion recognition based on feature fusion. Journal of Physics Conference Series 1616 , 012106.
Płaza, M., Trusz, S., Kęczkowska, J., Boksa, E., Sadowski, S., & Koruba, Z. (2022). Machine learning algorithms for detection and classifications of emotions in contact center applications. Sensors, 22 , 5311.
“python”. Retrieved May 23, 2023, from https://www.python.org/
Rawat, A., & Mishra, P. K. (2015). Emotion recognition through speech using neural network. International Journal of Advanced Research in Computer Science and Software Engineering, 5 (5), 422–428.
Sahu, S., Mitra, V., Seneviratne, S., & Espy-Wilson, C. (2019). Multi-modal learning for speech emotion recognition: An analysis and comparison of ASR outputs with ground truth transcription. In Proceedings of Interspeech (pp. 3302–3306).
Sato, S., Kimura, T., Horiuchi, Y., & Nishida, M. (2008). A method for automatically estimating F0 model parameters and a speech re-synthesis tool using F0 model and STRAIGHT. In INTERSPEECH 2008, 9th annual conference of the international speech communication association , Brisbane, Australia.
Schuller, B., Rigoll, G., &. Manfred, L. (2004). Speech emotion recognition combining acoustic features and linguistic information in a hybrid support vector machine-belief network architecture. In IEEE international conference on acoustics, speech, and signal processing (ICASSP) , Montreal, QC, Canada.
Seknedy, M. E., & Fawzi, S. (2021). Speech emotion recognition system for human interaction applications. In 10th international conference on intelligent computing and information systems (ICICIS) , Cairo, Egypt.
Selvara, M., Bhuvana, R., & Padmaja, S. (2016). Human speech emotion recognition. International Journal of Engineering and Technology (IJET), 8 (1), 311–323.
Shixin, P., Kai, C., Tian, T., & Jingying, C. (2022). An autoencoder-based feature level fusion for speech emotion recognition. Digital Communications and Networks . https://doi.org/10.1016/j.dcan.2022.10.018
Singh, Y. B., & Goel, S. (2022). A systematic literature review of speech emotion recognition approaches. Neurocomputing, 492 , 245–263.
Srivastava, B. M. L., Kajarekar, S., & Murthy, H. A. (2019). Challenges in automatic transcription of real-world phone calls. In Proceedings of Interspeech , Graz, Austria.
Sun, C., Li, H., & Ma, L. (2023). Speech emotion recognition based on improved masking EMD and convolutional recurrent neural network. Frontiers in Psychology, 13 , 1075624.
Sun, L., Fu, S., & Wang, F. (2019). Decision tree SVM model with Fisher feature selection for speech emotion recognition. EURASIP Journal on Audio, Speech, and Music Processing, 2 , 1–14.
Tacconi, D., Mayora, O., Lukowicz, P., Arnrich, B., Setz, C., Troster, G., & Haring, C. (2008). Activity and emotion recognition to support early diagnosis of psychiatric diseases. In International conference on pervasive computing technologies for healthcare .
“The University of Waikato”. Retrieved May 23, 2023, from https://www.cs.waikato.ac.nz/ml/weka/
Trigeorgis, G., Ringeval, F., Brueckner, R., Marchi, E., Nicolaou, M. A., Schuller, B., & Zafeiriou, S. (2016). Adieu features? End-to-end speech emotion recognition using a deep convolutional recurrent network. In IEEE international conference on acoustics, speech and signal processing (ICASSP) , Shanghai, China.
Wani, T. M., Gunawan, T. S., Qadri, S. A. A., Mansor, H., Kartiwi, M., & Ismail, N. (2020). Speech emotion recognition using convolution neural networks and deep stride convolutional neural networks. In 6th international conference on wireless and telematics (ICWT) , Yogyakarta, Indonesia.
“WavePad Audio Editing Software”. Retrieved May 23, 2023, from https://www.nch.com.au/wavepad/index.html
Yang, N., Yuan, J., Zhou, Y., Demirkol, I., Duan, Z., Heinzelman, W., & Sturge-Apple, M. (2017). Enhanced multiclass SVM with thresholding fusion for speech-based emotion classification. International Journal of Speech Technology, 20 , 27–41.
Yazdani, A., Simchi, H., & Shekofteh, Y. (2021). Emotion recognition in persian speech using deep neural networks. In 11th international conference on computer engineering and knowledge (ICCKE) , Mashhad, Iran.
Yu, Y., & Kim, Y.-J. (2020). Attention-LSTM-attention model for speech emotion recognition and analysis of IEMOCAP database. Electronics, 9 (5), 713.
Zhang, Y., & Srivastava, G. (2022). Speech emotion recognition method in educational scene based on machine learning. EAI Endorsed Transactions on Scalable Information Systems, 9 (5), e9.
Zhao, J., Mao, X., & Chen, L. (2018). Speech emotion recognition using deep 1D & 2D CNN LSTM networks. Biomedical Signal Processing and Control, 47 , 312–323.
Zheng, C., Wang, C., & Jia, N. (2020). An ensemble model for multi-level speech emotion recognition. Applied Sciences, 10 (1), 205–224.
ZiaUddin, M., & Nilsson, E. G. (2020). Emotion recognition using speech and neural structured learning to facilitate edge intelligence. Engineering Applications of Artificial Intelligence, 94 , 103775.
Zvarevashe, K., & Olugbara, O. O. (2020). Recognition of speech emotion using custom 2D-convolution neural network deep learning algorithm. Intelligent Data Analysis, 24 (5), 1065–1086.
Download references
Acknowledgements
The authors thank the Associate Editor and the reviewers for their insightful remarks, which greatly improved the paper's clarity.
Not applicable.
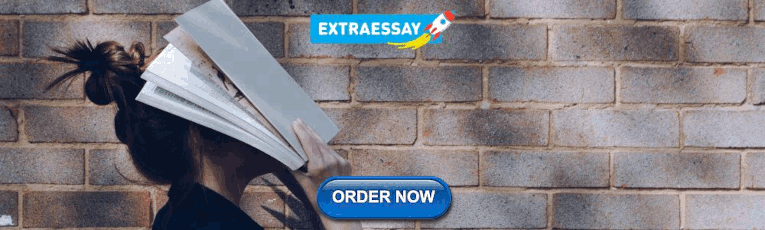
Author information
Authors and affiliations.
Computer Science Department, Faculty of Graduate Studies for Statistical Research, Cairo University, Giza, 12613, Egypt
Hesham A. Hefny
Information Systems and Technology Department, Faculty of Graduate Studies for Statistical Research, Cairo University, Giza, 12613, Egypt
Haidy H. Mustafa & Nagy R. Darwish
You can also search for this author in PubMed Google Scholar
Contributions
Haidy was the main contributor in preparing and writing the research. Nagy and Hesham revised the research. Three authors read and revised the research paper.
Corresponding author
Correspondence to Haidy H. Mustafa .
Ethics declarations
Competing interests.
None of the authors have any competing interests.
Additional information
Publisher's note.
Springer Nature remains neutral with regard to jurisdictional claims in published maps and institutional affiliations.
Rights and permissions
Springer Nature or its licensor (e.g. a society or other partner) holds exclusive rights to this article under a publishing agreement with the author(s) or other rightsholder(s); author self-archiving of the accepted manuscript version of this article is solely governed by the terms of such publishing agreement and applicable law.
Reprints and permissions
About this article
Mustafa, H.H., Darwish, N.R. & Hefny, H.A. Automatic Speech Emotion Recognition: a Systematic Literature Review. Int J Speech Technol 27 , 267–285 (2024). https://doi.org/10.1007/s10772-024-10096-7
Download citation
Received : 19 December 2023
Accepted : 18 February 2024
Published : 07 April 2024
Issue Date : March 2024
DOI : https://doi.org/10.1007/s10772-024-10096-7
Share this article
Anyone you share the following link with will be able to read this content:
Sorry, a shareable link is not currently available for this article.
Provided by the Springer Nature SharedIt content-sharing initiative
- Speech recognition
- Speech emotion recognition
- Automatic speech recognition
- Emotional speech
- Find a journal
- Publish with us
- Track your research
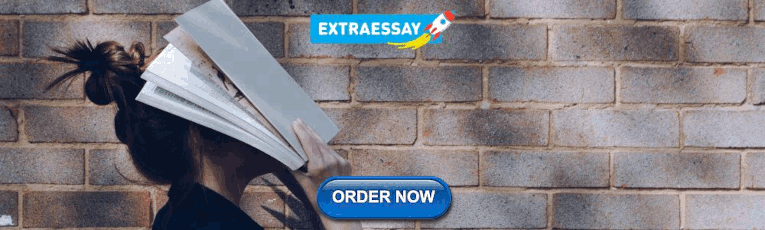
IMAGES
VIDEO