- Entertainment
- Environment
- Information Science and Technology
- Social Issues
Home Essay Samples Education Online vs. Traditional Classes
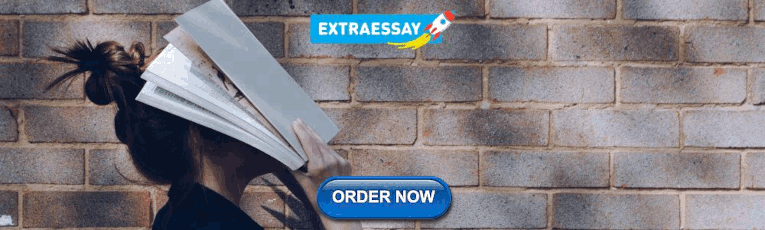
The Value of Face-to-Face Classes: An Argumentative Exploration of In-Person Learning
Table of contents, effective communication and engagement, social interaction and collaboration, personalized learning and motivation, holistic development, 1. real-time interaction, 2. non-verbal communication, 1. peer learning, 2. networking opportunities, 1. tailored instruction, 2. motivation and accountability, 1. soft skills enhancement, 2. emotional connection.
- Bain, K. (2004). What the best college teachers do. Harvard University Press.
- Chickering, A. W., & Gamson, Z. F. (1987). Seven principles for good practice in undergraduate education. AAHE Bulletin, 3, 7.
- Friedman, T. L. (2013). Revolution hits the universities. The New York Times, A25.
- Hattie, J. A. C., & Donoghue, G. M. (2016). Learning strategies: A synthesis and conceptual model. npj Science of Learning, 1(1), 16013.
- Pascarella, E. T., & Terenzini, P. T. (2005). How college affects students: A third decade of research (Vol. 2). Jossey-Bass.
*minimum deadline
Cite this Essay
To export a reference to this article please select a referencing style below
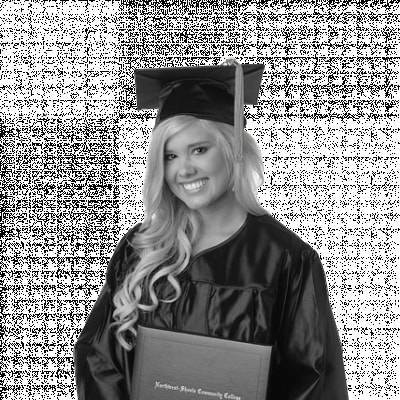
- Importance of Conceptual Frameworks
- American Education System
- Education Goals
- Studying Abroad
Related Essays
Need writing help?
You can always rely on us no matter what type of paper you need
*No hidden charges
100% Unique Essays
Absolutely Confidential
Money Back Guarantee
By clicking “Send Essay”, you agree to our Terms of service and Privacy statement. We will occasionally send you account related emails
You can also get a UNIQUE essay on this or any other topic
Thank you! We’ll contact you as soon as possible.
- Research article
- Open access
- Published: 18 August 2023
Exploring student perceptions and use of face-to-face classes, technology-enhanced active learning, and online resources
- Joanne M. Lewohl ORCID: orcid.org/0000-0002-7577-0734 1
International Journal of Educational Technology in Higher Education volume 20 , Article number: 48 ( 2023 ) Cite this article
17k Accesses
1 Citations
3 Altmetric
Metrics details
The current cohort of undergraduate students is often said to value technology and is assumed to prefer immersive, interactive, and personalized learning experiences. In contrast, many educators recognise the value of face-to-face classes and believe that attending class positively impacts student performance. A novel teaching strategy, including traditional lectures and interactive workshops using an educational technology platform were implemented in an undergraduate neurobiology course. Attendance in class and use of lecture capture recording were associated with improved student performance. Further, student attitudes toward the teaching strategy were evaluated via a survey. The survey respondents included those that regularly attended class and those that did not. Overall, irrespective of attendance, students thought that face-to-face classes were beneficial to their learning and the use of active learning activities helped them to understand the course content. The most common reasons for non-attendance in class were attributed to factors such as the class schedule, work and family commitments and were not related to the availability of class recordings and other online resources. In contrast, the most common reasons for attendance in class included the perceived benefit, the standard of teaching and the level of interest in the course. The novel teaching strategy had a positive impact on student learning, and can be used for in-person, online and asynchronous learning, providing a mechanism for educators to cater for students who wish to attend in-person classes as well as providing options for flexible delivery.
Graphical Abstract
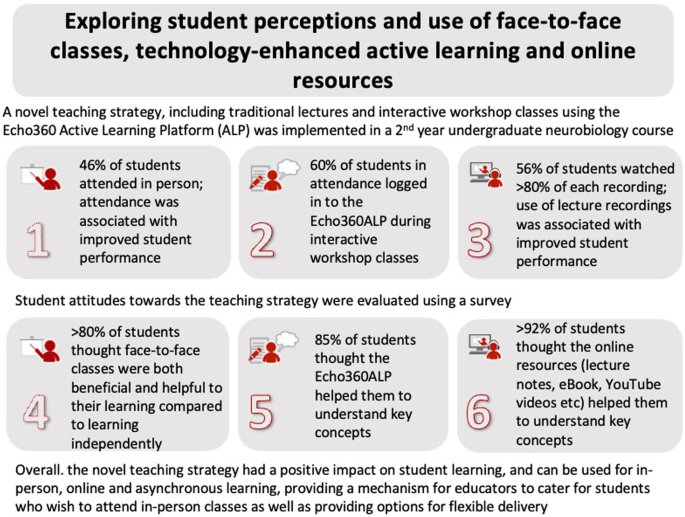
Introduction
Each new generation of students has characteristics, interests and learning preferences that set them apart from the previous generation, and understanding these differences is necessary for educators to create learning environments that are engaging, inspiring and productive (Poláková & Klímová, 2019 ). The current cohort of undergraduate students are often described as individuals who have grown up with technology as an integral part of their daily lives (Seemiller & Grace, 2016 ). They are thought to be highly adaptable to new technology and expect their learning experiences to be immersive, interactive, and personalized (Reviewed in (Shorey et al., 2021 )). This cohort of students are also considered to be more independent learners, often relying on online resources to support their education, with a preference for and the ability to learn at their own pace (Chicca & Shellenbarger, 2018 ; Seemiller & Grace, 2016 ).
In 2020, the global coronavirus pandemic necessitated a rapid pivot to online and blended learning at universities in Australia and around the world, accelerating the trends that were already in process (Watermeyer et al., 2021 ). As a result, there has been a rapid expansion into the online learning space and an increasing reliance on the use of educational technology and virtual learning environments to deliver content and to facilitate online learning (Reviewed in (Arday, 2022 )). As educators, we are entering an unprecedented era, one in which we are tasked with providing high quality instruction to engage students in their own learning despite the potential for ongoing educational disruption. There are many challenges in this changing landscape including how to cater to students who want the flexibility of studying online or asynchronously with those that want to return to face-to-face delivery.
Prior to the pandemic, a common mode of instruction at university was the traditional didactic lecture, although technology-enhanced active learning, problem-based learning and flipped classroom strategies have also become popular (Kirkwood & Price, 2014 ). Educators often placed value on in-class attendance which was viewed as an important indicator of student success (Crede et al., 2010 ; Guleker & Keci, 2014 ). Indeed, a systematic review of the relationship between lecture attendance and academic achievement revealed that 75% of studies showed a significant positive association between class attendance and academic performance for undergraduate students in the biosciences (Doggrell, 2020b ). However, there is an increasing trend at our Institution and others to provide lecture capture recordings and to develop online digital resources to facilitate student learning. The provision of these resources offers increased flexibility for students to engage with the course content, but a common concern is that this may negatively affect attendance and may not improve student outcomes (Gosper et al., 2010 ; Kinash et al., 2015 ; Preston et al., 2010 ). Specifically, the availability of captured lectures has been postulated to reduce student interaction in face-to-face classes (Mark et al., 2010 ). Attendance rates for students vary widely and the reasons for absenteeism often include student perception of the value of traditional lectures as well as the availability of class recordings and other online resources (Reviewed in (James & Seary, 2019 )). There is also the potential for traditional modes of delivery to be at odds with the learning preferences of the current generation of students (Shorey et al., 2021 ).
Technology-enhanced learning is a broad term that can be used to describe any form of e-learning. Accordingly, technology-enhanced learning strategies can refer the use of technology to improve learning in face-to-face classes, the creation and use of digital resources for asynchronous learning or using social media (and other platforms) to encourage collaborative learning (Ansari & Khan, 2020 ; Voorn & Kommers, 2013 ). The impact of these strategies on student learning is reliant on the student’s engagement with and usage of the specific technological platform that is implemented (Dunn & Kennedy, 2019 ). While the impact of in-class attendance on academic achievement has been extensively studied (Crede et al., 2010 ; Guleker & Keci, 2014 ), when technology-enhanced learning strategies are implemented, the relationship between student attendance and academic performance is more difficult to ascertain. Some studies have shown no correlation between class attendance and performance in courses where lectures are recorded and class materials are available online (Doggrell, 2020a ; Kauffman et al., 2018 ). Other studies have shown that students who study independently, using online resources, can have similar academic outcomes and may even outperform those who attend class (Eisen et al., 2015 ; Lukkarinen et al., 2016 ).
Active learning is a key component to undergraduate science, technology, engineering, and mathematics (STEM) education (Freeman et al., 2014 ) however, lectures at higher degree institutions are often held in learning spaces that are not conducive to in-class participation (Büchele, 2021 ; Fadelelmoula, 2018 ; O'Keeffe et al., 2017 ). To overcome this challenge, educators often use technology to enhance the learning experiences for students (Wood et al., 2018 ). Echo360 is a platform that is commonly used for the automatic recording of classes. The newest iteration of this product, the Echo360 Active Learning Platform (Echo360ALP), is a technology-enhanced learning platform designed to facilitate active learning, promoting student engagement and participation (Shaw et al., 2015 ). The Echo360ALP has been available at Griffith University from 2018. Its functionality includes the ability for educators to embed polling questions at strategic points in their presentations and students can log in and answer these questions in real time. This active learning platform also includes the ability to directly embed multimedia into in-class presentations which is likely to appeal to learners who prefer to seek information through visual learning (Seemiller & Grace, 2016 ). Using a technology-enhanced active learning platform as a tool, it is possible to create novel and innovative learning experiences which may encourage students to attend class and engage with class material.
The present study
The learning preferences of the current cohort of students for immersive, interactive, and flexible learning experiences are at odds with the traditional didactic delivery of lectures at university. To address this issue, a novel teaching strategy was implemented in a second-year undergraduate neurobiology course incorporating a unique blend of traditional lectures, active and interactive learning strategies, and online learning resources. Specifically, face-to-face classes included traditional didactic lectures which were used to deliver course content, and workshop classes that used an active learning platform to facilitate student interaction and engagement during class (Freeman et al., 2014 ; Shaw et al., 2015 ). In addition, all classes, were recorded and made available to students asynchronously. The teaching strategy was designed to meet the diverse needs of students and was aimed at fostering student engagement and motivation to attend class and engage with the course materials (Dunn & Kennedy, 2019 ). Thus, a key objective of the current study was to investigate student attendance in face-to-face classes, their use of class recordings, and the impact of these on student performance in the course. Students were also surveyed to establish their views on the teaching strategy including the use of an active learning platform in the classroom, their use of the available resources as well as factors that influenced their decision to attend, or not attend classes in person.
Cohort characteristics
The study participants were second-year undergraduate neurobiology students who completed the course as part of their program of study at Griffith University. Ethical Clearance for this project was obtained from the Griffith University Human Ethics Committee (GU Ref No: 2018/651). The course is offered in one 12-week trimester each year with two distinct cohorts analysed in this study (2018 and 2019). The course is a requirement for students in the Bachelor of Biomedical Science program and an elective for students in other health programs. Many of the students in these programs have career trajectories that include medicine, medical research, or allied health professions. In 2018 the cohort consisted of 115 students; 85 (74%) were from the Biomedical Science program, and 21 (18.3%) were from the Health Science program. The remaining nine students were from other health-related programs. In 2019 the cohort consisted of 93 students; 63 (72%) were from the Biomedical Science program, 25 (26.9%) were from the Health Science program, and the remaining student was from another health-related program. In the 2018 cohort there were 67 female students (58.3%) and 48 male students (41.7%). In the 2019 cohort there were 54 female students (58.1%) and 39 male students (41.9%). Data from both cohorts were combined in the analysis.
Educational context and course structure
The course was designed using a constructivist approach (Biggs, 2014 ) and consists of a series of scaffolded weekly topics starting with fundamental topics (e.g. neuroanatomy) and progressing to more complex integrated topics (e.g. dementia). The main objective of the course is to teach students about the function of the brain and specifically how damage to discrete areas of the brain results in the symptoms associated with various neurological and neuropathological conditions.
Each topic was designed and structured using Bloom’s Taxonomy (Krathwohl, 2002 ). The theoretical content was taught in face-to-face lectures which were automatically recorded using the Echo360 lecture capture system and made available within a few hours of the scheduled class. Additional digital resources including detailed learning objectives, presentation slides, and review questions were available for each topic. Students also had access to an online interactive textbook hosted by a third-party vendor that was authored by the course instructor, free to access under specific conditions and directly aligned to the course outcomes. The online textbook included formative assessments in the form of quiz questions as well as embedded multimedia usually in the form of YouTube videos that were vetted for appropriateness and accuracy of content.
To encourage student engagement and facilitate deep learning, each topic also included clinical case studies to provide a real-world context for students (Meil, 2007 ; Mickley & Hoyt, 2010 ). Students were expected to engage with and acquire knowledge about each topic from one of the available resources (in-person lecture, recorded lecture, or interactive textbook), and then apply that knowledge to analyse case studies in the workshop classes. For some topics, the theory and applied components were combined in a single class. The workshops (14 in total) were designed to be interactive and used an active learning platform. Each workshop included at least one case study and included polling questions which the students could answer in real-time as well as multimedia (video) which was used to showcase patient symptoms. The workshop classes were also automatically recorded using the lecture capture system. Students had access to the recording itself as well as the presentation files which included the embedded polling questions.
The timetable, timing and form of assessment, venue and teaching staff were consistent for the course offering in both 2018 and 2019. All lectures and interactive workshop classes were delivered by a single instructor in both 2018 and 2019.
Student attainment measures
The final exam was worth 50% of the overall grade and was held during the exam period at the end of the trimester. This item of assessment was conducted in-person, under exam conditions and included case study questions like those presented in interactive workshop classes. The dependent measures used as measures of student performance were the final exam percentage ( Final Exam ) and final overall percentage ( Overall Percentage ). Student grades for two pre-requisite anatomy and physiology courses were available for most students (83.5% of students in 2018 and 92.5% in 2019). The average pre-requisite grade was determined for each student individually and was used as a variable in the bivariate Pearson’s correlation analysis. Students without grades for the first-year courses were external transfers who received credit for the course.
Class attendance & in-class participation
Attendance was recorded in 14 classes during the trimester. A list of student names was circulated during the class. Students could sign in on entry to the class or mark their name off as the clipboard circulated through the room. The sign-in sheets were also available during the 10-min break in the middle of the two-hour class and at the end of class for any student who had not marked off their name. Attendance, as expressed as a percentage of enrolled students, was determined for each individual class ( Class Attendance ). Student Attendance was calculated as the total number of classes attended by each individual student (0–14). In-class participation was defined as the number of students who logged in to the active learning platform during class expressed as a percentage of the number of students who attended in-person.
Lecture capture analytics
Lecture capture data was downloaded once for each cohort on the day of the final exam and therefore reflects the number of views during the trimester and in the review period on the lead-up to the final exam. For each individual student, the viewing data was extracted for each class recording and included the view duration, capture duration and percentage of video viewed. If a student accessed and watched more than 10% of a recording it was counted as a “ View ”. If a student accessed and watched more than 80% of the recording it was counted as a “ Complete View ”. If a student accessed and watched between 10 and 80% of the recording it was counted as a “ Partial View ”.
Data analysis
The data was analysed using IBM SPSS Statistics 28 software (SPSS Inc. Chicago, IL, USA). The relationship between Student Attendance (total number of classes attended; 0 – 14), Complete Views (number of class recordings where > 80% of the recording was watched by the student; 0 – 14) and Partial Views (number of class recordings where 10 – 80% of the recording was watched by the student; 0–14) and performance in both the final exam and the course overall was investigated using bivariate Pearson’s correlation analysis. To determine if performance in the pre-requisite courses influenced the relationship between these variables, a partial correlation analysis was performed. Analysis of Variance (ANOVA) and post hoc testing (Tukey HSD) was used to determine if differences in student performance measures reached statistical significance (using an α of 0.05).
Student attitudinal survey and data analysis
Data was collected by means of an attitudinal survey. The survey was adapted from previous studies assessing student perspectives to lecture attendance in undergraduate engineering (Fitzpatrick et al., 2011 ) and neuroscience courses (O'Keeffe et al., 2017 ). In 2018, the survey was administered in person in the final class of the year. In 2019, the survey was administered online. The survey included questions regarding demographic information, questions about their attendance in each type of class, their opinions about face-to-face classes and lecture capture as well as questions about the resources provided in the learning environment (Table 1 ). Students were also asked to indicate their reasons for attendance or non-attendance in face-to-face classes by completing a matrix of possible predetermined options. Students who identified as “ non-attenders ” were given a choice of 17 options and asked to indicate whether it was “never a reason”, “sometimes a reason” or “definitely a reason” for their non-attendance (Table 2 ). Students who identified as “ attenders ” were asked to respond to 14 options with the same three possible responses (Table 3 ). The survey also included three open questions designed to solicit opinions about attending class and active learning strategies.
The data from each survey was exported to Excel and responses to each question were counted to determine the percentage of students with each response. For questions regarding student opinion of lectures and workshops, data was collected using a 5-point Likert scale. The 5-point Likert scale consisted of the following options: “strongly disagree”, “disagree”, “undecided”, “agree” and “strongly agree”. The responses were converted to ordinal data ranging from 1 to 5 with 1 = “strongly disagree” to 5 = “strongly agree”. A positive response was indicated by selection of either the “strongly agree” or “agree” option, a negative response was indicated by selection of either the “strongly disagree” or disagree” option. The final option was “undecided” indicating no clear agreement or disagreement with the statement.
The data was analysed using IBM SPSS Statistics 25 software (SPSS Inc. Chicago, IL, USA). For each statement, descriptive statistics including the mean score were calculated. Further, Pearson’s Chi-squared tests were performed to determine whether the student’s choice significantly deviated from chance where the expected outcome was defined as equal numbers of students selecting each option. Students were also asked to indicate their reasons for attendance or non-attendance in face-to-face classes by completing a matrix of possible (predetermined) options. However, students were able to contribute additional responses and reasons via open ended questions.
What resources did the students use?
Attendance in lectures and workshops was not mandatory and students were able to choose whether to attend class in person, use the class recordings as a substitute or a combination of both according to their own preferences. The class materials, including the class recordings and online interactive textbook, were available to all students enrolled in the course and the variety and comprehensiveness of the resources allowed students the flexibility to study independently if they chose.
Workshop attendance varied from 25.8 to 73.1% throughout the trimester for an average of 46%. Individual student attendance ranged from 0 to 100%. While students were encouraged to bring a laptop or other mobile device for in-class polling activities using the active learning platform it was not mandatory. In both cohorts there was a mixture of students who logged in and those who did not. The average percentage of students who logged in to the active learning platform during class was 59.6% (range: 17.8–86.1%).
Lecture capture usage varied across classes, with the average number of views per recording ranging from 99 to 263 (average: 152 views/class). The percentage of students viewing the recorded lectures ranged from 38 to 74% (average: 53.5%). Further, the percentage of students watching more than 80% of the recording ranged from 22 to 60% (average: 56%). Of the 205 analyzed students, 14 attended class in-person but did not watch the recordings (“ Attenders ”), 26 watched more than 80% of each class recording but did not attend in-person (“ Viewers ”), 29 attended class and watched more than 80% of each recording (“ High Engagers ”) and 15 neither attended class in person nor watched the recordings (“ Low Engagers ”).
The online textbook, hosted by a third-party vendor, was accessible at no cost to students under specific circumstances. Approximately 45% of students in the cohort signed up to access the online textbook but since it was hosted externally, precise tracking data was not available.
How did the students perform in the course?
In terms of in-person attendance, a weak but significant positive relationship was found between Student Attendance and performance on the final exam (R 205 = 0.284, P < 0.001) and in the overall course percentage (R 205 = 0.268, P < 0.001). These relationships remained significant even after controlling for average pre-requisite grade (Final Exam Percentage: R 185 = 0.258, P < 0.001; Final Overall Percentage: R 205 = 0.235, P = 0.001).
Regarding the impact of watching class recordings, a weak but significant positive relationship was found between watching more than 80% of each recording ( Complete Views ) and performance on both the final exam ( R 203 = 0.29, P < 0.001) and in the overall course percentage ( R 203 = 0.307, P < 0.001). These relationships remained significant even when controlling for average pre-requisite grade (Final Exam: R 182 = 0.279, P < 0.001; Final Overall Percentage: R 183 = 0.316, P = 0.001). However, no significant relationship was found between partial lecture capture views ( Partial Views ) and performance on the final exam ( R 203 = 0.02, P = 0.774) or in the overall course percentage ( R 205 = 0.004, P = 0.955).
To determine if watching more than 80% of each class recordings is equivalent to attending class in person, the performance of “ Attenders ” was compared with that of “ Viewers ”. These two groups of students performed similarly in both the final exam (Tukey HSD; P = 0.965) and overall course percentage ( P = 0.975) suggesting that watching the class recordings can serve as an adequate substitute for attending in person. Further, both “ Attenders ” and “ Viewers ” outperformed “ Low Engagers ” on the final exam (“ Attenders ” vs “ Low Engagers ”, P = 0.004; “ Viewers ” vs “ Low Engagers ”, P = 0.032) and in the course overall (“ Attenders ” vs “ Low Engagers ”, P = 0.001; “ Viewers ” vs “ Low Engagers ”, P = 0.009). “ High Engagers ” performed at a similar level to “ Attenders ” (Final Exam, P = 0.899; Overall Percentage, P = 0.975) and “ Viewers ” (Final Exam, P = 1.00; Overall Percentage, P = 1.00) on both the final exam and in the course overall.
Student perspectives on the relevance of face-to-face classes
In total, 105 students completed surveys: 68 students in 2018 (59.1%) and 37 students in 2019 (40.2%). Overall, 78.1% of the students were 15–20 years of age and a further 20% of students were 21–30 years of age. There was one student who as 31–40 years of age and one who was in the 41–50-year age bracket. There were more females (66.67%) than males (33.33%). The majority (86.67%) of students used English as their first language and 93.33% of the cohort were domestic students. Most of the respondents were students in the Bachelor of Biomedical Science program (76.2%) and a further 17.14% were in the Bachelor of Health Science program. The remaining students were enrolled in a variety of other programs in the Faculty of Health. Of the students who completed the survey, 62.9% attended more than 50% of lectures, 17.1% attended less than 50% of lectures and 20% did not attend any lectures. Of the students who completed the survey, 72.4% attended more than 50% of workshops, 19% attended less than 50% of workshops and 8.6% did not attend any workshops.
Students were asked to indicate their level of agreement with five statements related to their experience of the course (see Table 1 for details). Out of 105 surveyed students, the majority found lectures (82%) and workshops (93%) beneficial to their learning (mean Likert score, 4.18 and 4.64 respectively). Chi-squared analysis showed a significant deviation in student preference from chance for both statements (Lectures: χ 2 = 81.73, df = 4, P < 0.001; Workshops: χ 2 = 134.01, df = 4, P < 0.001). The majority of students (76.7%; mean Likert score, 4.15) agreed that “ Attending lectures and workshop classes helped me to understand the course material much better than just reading through or watching the supplied resources ”. Chi-squared analysis showed a significant deviation in student preference from chance for this statement ( χ 2 = 75.30, df = 4, P < 0.001). The majority of students (85%; mean Likert score, 4.15) agreed that “ The use of in-class interactive tools helped me to understand key course concepts ”. Chi-squared analysis showed a significant deviation in student preference from chance for this statement ( χ 2 = 89.37, df = 4, P < 0.001). Most students (62%; mean Likert score 2.59) responded negatively to the statement “ Since the lecture and workshop classes were recorded there was no real reason to attend class ”. Chi-squared analysis showed a significant deviation in student preference from chance for this statement ( χ 2 = 48.31, df = 4, P < 0.001).
To understand how the students felt about the online resources that were provided by the instructor, students were asked to respond to two statements. The majority of students (92.4%; mean Likert score, 4.5), agreed that “ The Instructor provided lecture notes, ebooks, YouTube videos and other resources which helped me to understand key concepts in neurobiology ”. Further, 47.5% of students responded positively to the statement “ I accessed and used the online interactive textbook (Neurobiology: A Case Study Approach) which helped me to understand key concepts in neurobiology ” (mean Likert score, 3.27). Chi-squared analysis showed a significant deviation in student preference from chance for both statements (Resources: χ 2 = 140.86, df = 4, P < 0.001; Online textbook: χ 2 = 10.14, df = 4, P < 0.038).
The final statement was designed to assess the student’s overall opinion about face-to-face classes. The majority of students (76%) responded negatively to the statement “ I think face-to-face lectures and workshop classes are an out-dated mode of education in the modern world of information technology, distance learning and self-directed learning” (mean Likert score 1.96). Chi-squared analysis showed a significant deviation in student preference from chance for this statement ( χ 2 = 60.52, df = 4, P < 0.001).
Factors affecting attendance in lectures and workshops
To determine which factors affected the decision not to attend lectures, students were given a choice of 17 possible options and asked to indicate whether it was “never a reason”, “sometimes a reason” or “definitely a reason” (Table 2 ). In a similar fashion, students were asked about the factors which affected their decision to attend lectures and workshops. For this question they were asked to respond to 14 options with the same three possible responses (Table 3 ).
Various factors influenced student attendance in class. The lecture schedule and the availability of class recordings were reported as the primary reasons for non-attendance. Interestingly, the schedule of the workshop classes was of less concern to students. Of note, work and family commitments were also given as reasons for non-attendance with some students choosing to use the scheduled time for self-directed study instead. Also, of note is that students’ reasons for non-attendance were not related to the standard of teaching in the course, the perceived benefit of attending class, the student’s interest in the content covered in the course, or the availability of online resources. The complete list of options and the distribution of responses can be found in Table 2 .
The perceived benefit gained by attending class, the quality of teaching and the level of interest in the course content played significant roles in determining student attendance. Of note, students who attended class responded positively to the three options related to the active learning activities and participation in class. The complete list of options and the distribution of responses can be found in Table 3 .
Most undergraduate students currently studying at university use technology as an integral part of their daily lives (Seemiller & Grace, 2016 ). These students have a preference for and the ability to use online resources to learn independently and at their own pace (Chicca & Shellenbarger, 2018 ; Seemiller & Grace, 2016 ), are predominantly visual and kinaesthetic learners and tend to embrace gamified, active and interactive learning experiences (Roberts, 2015 ) (Shorey et al., 2021 ). Creating engaging learning experiences is dependent on understanding the needs, interests, and learning preferences of the students we teach.
The global coronavirus pandemic necessitated a rapid pivot to online and blended learning strategies to minimize disruption to student education (Arday, 2022 ). The experience of students during that time is likely to be highly variable and dependent on the individual skill and experience of the instructors in their courses as well as availability of educational technology and virtual learning environments (Koh & Daniel, 2022 ; Sum & Oancea, 2022 ). For some courses and institutions lectures may have been delivered live but online, for others the classes may have been delivered asynchronously with pre-recorded lectures available for students to view in their own time. Thus, student attitudes toward and preferences for online versus face-to-face classes will likely be influenced by this recent experience. However, reflecting on and critically evaluating the factors that motivated students to attend classes before the pandemic can provide valuable insight to inform our decisions as educators whether to continue teaching in the online space or return to the classroom.
Are face-to-face classes an outdated mode of education?
Even before the pandemic, the decline in attendance in face-to-face lectures was well documented with many educators questioning the value of this mode of teaching (Golding, 2011 ; O'Keeffe et al., 2017 ). Many studies attributed the decline in lecture attendance to the increasing availability of digital recordings and other online resources (Edwards & Clinton, 2019 ; Johnston et al., 2013 ). While the provision of these resources offers increased flexibility for students, a common concern has been the potential negative impact this may have on attendance and ultimately student performance (Gosper et al., 2010 ; Kinash et al., 2015 ; Preston et al., 2010 ). A similar trend was observed in the undergraduate neurobiology course analyzed in this study following the university mandated digital recording of lecture and workshop classes from 2013 onwards. However, despite reduced attendance, one of the recurring themes in student feedback was a desire for more discussion and interactivity during class.
With a view to improving the student experience and to encourage students to attend class, an active learning platform was used to augment neurobiology workshop classes to include videos and in-class polling. Overall, student attendance fluctuated during the trimester for an average of 46% which is similar to or greater than other courses in the biosciences (Doggrell, 2019 , 2020a ). While it is not possible to correlate in-class attendance to the use of the active learning platform directly, the survey responses indicated that this mode of teaching was popular among the students. Similar to other studies, attending class was weakly associated with better performance (Doggrell, 2019 ). More importantly, students who chose to attend class did so because of the high standard of teaching, their interest in the course material and thought the classes were beneficial to their learning. There is also a perception among the surveyed students that they will miss important information if they miss class, despite the availability of other resources including class recordings. In contrast, reasons for non-attendance were not related to the quality of teaching, interest in the course content or the perceived benefit of attending class. The main reason for non-attendance were factors outside of the control of the course instructor and included the time of the scheduled lectures (5 – 7 pm on a Monday evening), as well as part-time work and family commitments. An important finding of this study is that the availability of digital recordings and other online resources allowed students with external commitments and time constraints to continue their studies and perform as well as their peers.
Students who did not regularly attend class stated that the availability of digital recordings influenced their decision not to attend. However, a high proportion of students who attended class accessed and used the class recordings, with most indicating that the availability of these resources was not a factor in their decision to attend class. Interestingly, watching the digital recordings was associated with better performance in the course but only if more than 80% of the recording was viewed. Further, students who exclusively used the digital recordings to access the course content had similar academic outcomes to those who came to class. Studies investigating the correlation between lecture attendance and academic performance when lecture capture was available have reported mixed results. A systematic review published in 2020 showed that in the biosciences, 69% of studies show a weak but positive association between lecture attendance and academic performance when lecture capture was available (Doggrell, 2020b ). However, whether students had access to digital recordings was only indicated in 11 of the 29 studies analysed, and no data on how the students used the recordings was presented.
It should be noted that the students in the course take three other courses, some of which have mandatory laboratory classes as well as assessments at varying times during the trimester. However, unlike previous studies, only 9% of students indicated that assessments and demands for other courses was a reason for their non-attendance. In prior studies using a similar survey, 47% of neuroscience students (O'Keeffe et al., 2017 ) and 38% of engineering students (Fitzpatrick et al., 2011 ) indicated that this was a reason for their non-attendance. Further, approximately 30% of students expressed that their decision to attend class was sometimes influenced by assessments in other courses. Throughout the trimester, class attendance fluctuated, and the classes with the lowest student turnout coincided with mid-trimester assessments in other courses.
Since attendance in class was not mandatory and students had access to a variety of digital resources in addition to the class recordings, they had the flexibility to study independently if they chose. However, very few students (~ 11%) stated that the availability of the digital resources was a reason for their non-attendance and only 16% of students stated that they used the time for self-directed study. A notable distinction between students who did not attend class and those that did was their perception of the sufficiency of the digital resources. Only a small percentage of Non-attenders stated that they accessed the YouTube videos (less than 3%) or the interactive textbook (less than 7%) and therefore did not need to attend class. In contrast, approximately 45% of Attenders accessed the YouTube video links, and around 32% of Attenders used the interactive textbook. Interestingly, Attenders viewed these resources as valuable supplements to their learning but still attended class to enhance their overall understanding of the course material.
Do active learning strategies improve the face-to-face learning experience?
One of the strategies that appealed to students the most was the use of an active learning platform during class. The platform was used to facilitate active learning, and to promote student engagement and participation (Shaw et al., 2015 ). Presentation files for each interactive class were uploaded to the platform directly, and multimedia and polling questions were embedded. At appropriate times during the class, the students were polled and given a few minutes to contribute their answers. All answers were anonymous, and students could change their answer if they chose. Multiple choice, short answer and click-on-target style questions were deliberately chosen to clarify key points and to prompt discussion. After a few minutes, the instructor switched to the “live” view of the responses and discussed the correct answers and reasoning with the class. Students could ask questions or seek further clarification and the polling questions commonly prompted detailed discussion of key concepts. While all students were encouraged to log in to the active learning platform during class, it was not compulsory to do so. The classes were automatically recorded and these recording captured the class in its entirety including the in-class polling, answers and resulting discussion. Overall, the interactive workshop classes were very popular with students as the system allowed them to actively participate in class, even though the classes were held in a lecture theatre that was not conducive to active learning (Büchele, 2021 ; Fadelelmoula, 2018 ). Students who attended class indicated that the active learning activities complemented the course resources, helped them to gauge their understanding of key course concepts and factored into their decision to attend class in person. Despite the availability of class recordings, students found greater benefit in attending class than working through the class materials by themselves. Students who did not attend class did not directly experience the benefits of the active learning strategy. Moreover, since their non-attendance was primarily due to external commitments, it is unlikely that the utilization of the platform, or any other teaching strategy, would encourage in-class attendance.
It is interesting to note that the impact of the active learning strategy was not limited to those students who logged in to the platform during class. Students who attended class but did not log in as well as those students who used the class recordings as a substitute for in-person attendance, performed well in the course. One explanation is that the students are learning vicariously by observing their peers’ responses to questions and were thus able to gauge their understanding of key concepts without contributing answers themselves (Mayes, 2015 ; Roberts, 2015 ).
One of the potential limitations of using an active learning platform during class is that encouraging the use of laptops and other devices may be distracting to not just the students using the device but also their peers (Aagaard, 2015 ; Dontre, 2021 ; Fried, 2008 ; Sana et al., 2013 ; Wood et al., 2018 ). A proportion of students (~ 14%) attended class in person and watched more than 80% of each recording. The academic achievement of these students was comparable to those students who either attended class or watched the complete recording. One possible explanation is that despite coming to class in-person, these students were either distracted during class or otherwise disengaged and felt the need to make up the class by watching the recording. However, informal feedback from students suggested that students who came to class used the recordings for review purposes.
In this paper we have examined the impact of a novel teaching strategy designed to improve student engagement in a second-year neurobiology course. This strategy was developed with the preferences of students in mind and included a combination of lectures, technology-enhanced interactive workshops, and online learning resources. Historically, educators have placed emphasis on the value of in-class attendance viewing it as an important indicator for student success (Crede et al., 2010 ; Guleker & Keci, 2014 ). In this study, in line with this belief, students who attended class found the experience to be beneficial to their learning. These students performed well overall, and better than those who did not attend class. However, one of the key outcomes is that students who had to depend on class recordings due to scheduling conflicts or other issues, achieved comparable results to their peers who attended class in person. Consequently, the availability of class recordings and other digital resources enhances flexibility without detrimentally affecting student performance. Students could choose how to access the course content based on their own personal preferences and circumstances and this likely lead to increased engagement and satisfaction with the learning experience. Although the study was limited to a single course, the outcome may be broadly applicable across other disciplines.
Lectures at tertiary institutions are often held in learning spaces that are not conducive to in-class participation (Büchele, 2021 ; Fadelelmoula, 2018 ; O'Keeffe et al., 2017 ). However, leveraging technology to enhance the in-class experience of students has been shown to improve student learning (Wood et al., 2018 ) but the impact is dependent on the students’ engagement with and usage of the specific platform that is implemented (Dunn & Kennedy, 2019 ). In this study, an active learning platform was used to embed in-class polling questions and multimedia at strategic points during workshop classes. The questions and videos were chosen to showcase specific learning outcomes and provide opportunities for students to gauge their understanding of key concepts. Overall, student perception of the interactive workshops was positive, with most students stating that the classes were beneficial to their learning experience. Further, students felt that the in-class experience was enhanced by using the active learning platform and that this mode of teaching helped them to understand and apply the course concepts. The benefits of using this teaching approach is that it can be adapted for use in any discipline that involves both the acquisition and application of knowledge, it is readily scalable to accommodate large classes and can be used for both online and hybrid learning environments. This strategy can also be implemented using various platforms since there are several different in-class polling tools available.
The current generation of students, known for their adaptability to technology and inclination toward independent learning, highly value and often use digital resources (Chicca & Shellenbarger, 2018 ; Seemiller & Grace, 2016 ). Nevertheless, this study reveals that attending face-to-face classes still holds significant value, as students reported greater benefits from in-person interactions compared to relying on independent study. In summary, this research underscores the efficacy of a student-centred teaching approach, leveraging technology and providing flexible access to course materials. By recognizing the evolving preferences and learning styles of students, educators can optimize engagement and learning outcomes in a variety of educational settings.
Availability of data and materials
Ethical clearance for the project was granted by the Griffith University Human Ethics committee and as per human-subject research approval, the student attainment and usage data as well as the raw data from the attitudinal survey is only available upon revision of the protocol.
Abbreviations
Science, technology, engineering, and mathematics
Echo360 Active Learning Platform
Griffith University
IBM SPSS Statistics 28 software Incorporated
Analysis of Variance
Tukey Highest Significant Difference
Aagaard, J. (2015). Drawn to distraction: A qualitative study of off-task use of educational technology. Computers & Education, 87 , 90–97.
Article Google Scholar
Ansari, J. A. N., & Khan, N. A. (2020). Exploring the role of social media in collaborative learning the new domain of learning. Smart Learning Environments, 7 (1), 1–16.
Arday, J. (2022). COVID-19 and higher education: The times they are a’changin (Vol. 74, pp. 365–377). Taylor & Francis.
Google Scholar
Biggs, J. (2014). Constructive alignment in university teaching. HERDSA Review of Higher Education, 1 , 25.
Büchele, S. (2021). Evaluating the link between attendance and performance in higher education: The role of classroom engagement dimensions. Assessment & Evaluation in Higher Education, 46 (1), 132–150.
Chicca, J., & Shellenbarger, T. (2018). Generation Z: Approaches and teaching–learning practices for nursing professional development practitioners. Journal for Nurses in Professional Development, 34 (5), 250–256.
Crede, M., Roch, S. G., & Kieszczynka, U. M. (2010). Class attendance in college: A meta-analytic review of the relationship of class attendance with grades and student characteristics. Review of Educational Research., 80 (2), 272–295. https://doi.org/10.3102/0034654310362998
Doggrell, S. A. (2019). The relationships between lecture attendance or accessing lecture recordings and academic outcomes: Results from a pharmacology course in a biomedical science degree. International Journal of Innovation in Science and Mathematics Education, 27 (5), 1–12.
Doggrell, S. A. (2020a). No apparent association between lecture attendance or accessing lecture recordings and academic outcomes in a medical laboratory science course. BMC Medical Education, 20 , 1–12.
Doggrell, S. A. (2020b). A systematic review of the relationship between lecture attendance and academic outcomes for students studying the human biosciences. International Journal of Innovation in Science and Mathematics Education., 28 , 1.
Dontre, A. J. (2021). The influence of technology on academic distraction: A review. Human Behavior and Emerging Technologies, 3 (3), 379–390.
Dunn, T. J., & Kennedy, M. (2019). Technology Enhanced Learning in higher education; motivations, engagement and academic achievement. Computers & Education, 137 , 104–113. https://doi.org/10.1016/j.compedu.2019.04.004
Edwards, M., & Clinton, M. (2019). A study exploring the impact of lecture capture availability and lecture capture usage on student attendance and attainment. Higher Education, 77 (3), 403–442.
Eisen, D. B., Schupp, C. W., Isseroff, R. R., Ibrahimi, O. A., Ledo, L., & Armstrong, A. W. (2015). Does class attendance matter? Results from a second-year medical school dermatology cohort study. International Journal of Dermatology, 54 (7), 807–816. https://doi.org/10.1111/ijd.12816
Fadelelmoula, T. (2018). The impact of class attendance on student performance. International Research Journal of Medicine and Medical Sciences, 6 (2), 47–49.
Fitzpatrick, J., Cronin, K., & Byrne, E. (2011). Is attending lectures still relevant in engineering education? European Journal of Engineering Education, 36 (3), 301–312. https://doi.org/10.1080/03043797.2011.585226
Freeman, S., Eddy, S., McDonough, M., Smith, M., Okoroafor, N., Jordt, H., & Wenderoth, M. (2014). Active learning increases student performance in science, engineering, and mathematics. Proceedings of the National Academy of Sciences, 111 (23), 8410–8415.
Fried, C. B. (2008). In-class laptop use and its effects on student learning. Computers & Education, 50 (3), 906–914.
Golding, J. M. (2011). The role of attendance in lecture classes: You can lead a horse to water. Teaching of Psychology, 38 , 40. https://doi.org/10.1177/0098628310390915
Gosper, M., McNeill, M., Phillips, R., Preston, G., Woo, K., & Green, D. (2010). Web-based lecture technologies and learning and teaching: A study of change in four Australian universities. ALT-J . https://doi.org/10.1080/09687769.2010.529111
Guleker, R., & Keci, J. (2014). The effect of attendance on academic performance. Mediterranean Journal of Social Sciences, 5 (23), 961.
James, D. T., & Seary, K. (2019). Why aren’t they attending class like they are supposed to? A review into students’ perception of the value of class attendance. Student Success, 10 (1), 115–129.
Johnston, A. N. B., Massa, H., & Burne, T. H. J. (2013). Digital lecture recording: A cautionary tale. Nurse Education in Practice . https://doi.org/10.1016/j.nepr.2012.07.004
Kauffman, C. A., Derazin, M., Asmar, A., & Kibble, J. D. (2018). Relationship between classroom attendance and examination performance in a second-year medical pathophysiology class. Advances in Physiology Education, 42 (4), 593–598. https://doi.org/10.1152/advan.00123.2018
Kinash, S., Knight, D., & McLean, M. (2015). Does digital scholarship through online lectures affect student learning? Journal of Educational Technology & Society., 18 (2), 129–139.
Kirkwood, A., & Price, L. (2014). Technology-enhanced learning and teaching in higher education: What is ‘enhanced’ and how do we know? A critical literature review. Learning, Media and Technology, 39 (1), 6–36. https://doi.org/10.1080/17439884.2013.770404
Koh, J. H. L., & Daniel, B. K. (2022). Shifting online during COVID-19: A systematic review of teaching and learning strategies and their outcomes. International Journal of Educational Technology in Higher Education, 19 (1), 56.
Krathwohl, D. (2002). A revision of Bloom’s taxonomy: An overview. Theory into Practice, 41 (4), 212–218.
Lukkarinen, A., Koivukangas, P., & Seppälä, T. (2016). Relationship between class attendance and student performance. Procedia - Social and Behavioral Sciences., 228 , 341–347. https://doi.org/10.1016/j.sbspro.2016.07.051
Mark, K., Vogel, D., & Wong, E. (2010). Developing learning system continuance with teachers and students: Case study of the Echo360 lecture capturing system . PACIS.
Mayes, J. T. (2015). Still to learn from vicarious learning. E-Learning and Digital Media, 12 (3–4), 361–371.
Meil, W. (2007). The use of case studies in teaching undergraduate neuroscience. Journal of Undergraduate Neuroscience Education., 5 (2), A53.
Mickley, G., & Hoyt, D. (2010). Narratives and neurons stories of damaged brains. JUNE., 8 (2), 91.
O’Keeffe, G., Sullivan, A., & McCarthy, M. (2017). An attitudinal survey of undergraduate neuroscience students regarding their views on the relevance of lectures to their education. June, 16 (1), 28–33.
Poláková, P., & Klímová, B. (2019). Mobile technology and generation Z in the english language classroom—A preliminary study. Education Sciences, 9 (3), 203.
Preston, G., Phillips, R., Gosper, M., McNeill, M., Woo, K., & Green, D. (2010). Web-based lecture technologies Highlighting the changing nature of teaching and learning. Australasian Journal of Educational Technology . https://doi.org/10.14742/ajet.1038
Roberts, J. C. (2015). Evaluating the effectiveness of lecture capture: lessons learned from an undergraduate political research class. Journal of Political Science Education . https://doi.org/10.1080/15512169.2014.985104
Sana, F., Weston, T., & Cepeda, N. J. (2013). Laptop multitasking hinders classroom learning for both users and nearby peers. Computers & Education, 62 , 24–31.
Seemiller, C., & Grace, M. (2016). Generation Z goes to college . Wiley.
Shaw, J., Kominko, S., & Terrion, J. (2015). Using LectureTools to enhance student–instructor relations and student engagement in the large class. Research in Learning Technology, 23 , 27197.
Shorey, S., Chan, V., Rajendran, P., & Ang, E. (2021). Learning styles, preferences and needs of generation Z healthcare students: Scoping review. Nurse Education in Practice., 57 , 103247. https://doi.org/10.1016/j.nepr.2021.103247
Sum, M., & Oancea, A. (2022). The use of technology in higher education teaching by academics during the COVID-19 emergency remote teaching period: A systematic review. International Journal of Educational Technology in Higher Education, 19 (1), 59.
Voorn, R. J. J., & Kommers, P. A. M. (2013). Social media and higher education: Introversion and collaborative learning from the student’s perspective. International Journal of Social Media and Interactive Learning Environments, 1 (1), 59–73.
Watermeyer, R., Crick, T., Knight, C., & Goodall, J. (2021). COVID-19 and digital disruption in UK universities: Afflictions and affordances of emergency online migration. Higher Education, 81 (3), 623–641. https://doi.org/10.1007/s10734-020-00561-y
Wood, E., Mirza, A., & Shaw, L. (2018). Using technology to promote classroom instruction: Assessing incidences of on-task and off-task multitasking and learning. Journal of Computing in Higher Education, 30 , 553–571.
Download references
Acknowledgements
The author would like to thank Mrs S. Poulsen, Project Leader, Echo360ALP Project, Griffith University Learning Futures for technical assistance, execution, and feedback on the integration of the active learning platform in the classroom as well as the students in 2020MSC Neurobiology for their participation, patience, and feedback.
Not applicable.
Author information
Authors and affiliations.
School of Pharmacy & Medical Sciences, Griffith University, Gold Coast Campus, Southport, 4222, Australia
Joanne M. Lewohl
You can also search for this author in PubMed Google Scholar
Contributions
Corresponding author.
Correspondence to Joanne M. Lewohl .
Ethics declarations
Competing interests.
The author declares no competing interests.
Additional information
Publisher's note.
Springer Nature remains neutral with regard to jurisdictional claims in published maps and institutional affiliations.
Rights and permissions
Open Access This article is licensed under a Creative Commons Attribution 4.0 International License, which permits use, sharing, adaptation, distribution and reproduction in any medium or format, as long as you give appropriate credit to the original author(s) and the source, provide a link to the Creative Commons licence, and indicate if changes were made. The images or other third party material in this article are included in the article's Creative Commons licence, unless indicated otherwise in a credit line to the material. If material is not included in the article's Creative Commons licence and your intended use is not permitted by statutory regulation or exceeds the permitted use, you will need to obtain permission directly from the copyright holder. To view a copy of this licence, visit http://creativecommons.org/licenses/by/4.0/ .
Reprints and permissions
About this article
Cite this article.
Lewohl, J.M. Exploring student perceptions and use of face-to-face classes, technology-enhanced active learning, and online resources. Int J Educ Technol High Educ 20 , 48 (2023). https://doi.org/10.1186/s41239-023-00416-3
Download citation
Received : 04 April 2023
Accepted : 25 July 2023
Published : 18 August 2023
DOI : https://doi.org/10.1186/s41239-023-00416-3
Share this article
Anyone you share the following link with will be able to read this content:
Sorry, a shareable link is not currently available for this article.
Provided by the Springer Nature SharedIt content-sharing initiative
- Asynchronous learning
- Synchronous learning
- Attitudinal survey
- Lecture capture
Home — Essay Samples — Education — Online Vs. Traditional Classes — Online Learning vs Face-to-Face

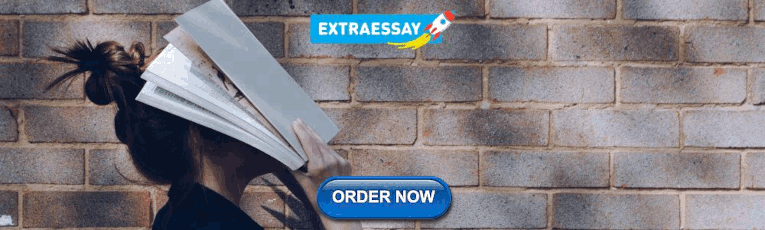
Online Learning Vs Face-to-face
- Categories: Online Vs. Traditional Classes Technology in Education
About this sample

Words: 768 |
Published: Aug 24, 2023
Words: 768 | Pages: 2 | 4 min read
Table of contents
Advantages of online learning, disadvantages of online learning, advantages of face-to-face education, disadvantages of face-to-face education.
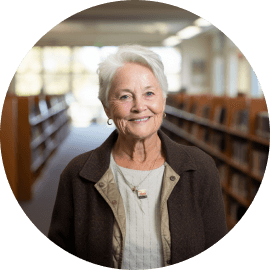
Cite this Essay
Let us write you an essay from scratch
- 450+ experts on 30 subjects ready to help
- Custom essay delivered in as few as 3 hours
Get high-quality help

Dr. Karlyna PhD
Verified writer
- Expert in: Education Information Science and Technology
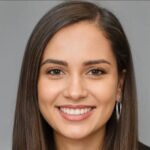
+ 120 experts online
By clicking “Check Writers’ Offers”, you agree to our terms of service and privacy policy . We’ll occasionally send you promo and account related email
No need to pay just yet!
Related Essays
3 pages / 1435 words
1 pages / 580 words
5 pages / 2159 words
1 pages / 462 words
Remember! This is just a sample.
You can get your custom paper by one of our expert writers.
121 writers online
Still can’t find what you need?
Browse our vast selection of original essay samples, each expertly formatted and styled
Related Essays on Online Vs. Traditional Classes
Students may have the opportunities to attend an online school and they can make a choice between online schools and traditional schools. The debate of "online school vs traditional school" is prevalent, as both have their [...]
Technology is rapidly taking over today’s society and how we accomplish things. We are very lucky to be living in a society that is centered upon multiple types of technology and advancements. There are major benefits to [...]
The debate between online education and in-class education has been ongoing for several years, with proponents and detractors arguing the benefits and drawbacks of each mode of learning. The rise of online education has brought [...]
Online education has become an increasingly popular option for students seeking to further their education and obtain a degree. With the advancement of technology, the accessibility and convenience of online education have made [...]
Whether or not to take an online class is something almost every college student has to decide at some point in their college career. Taking an online class has both advantages and disadvantages, and students differ on their [...]
Education on top priority Today, education stands on top of the priority list. Anywhere you go or anything you want to achieve in your life, you require education. The opportunities to get educated and earn degrees are [...]
Related Topics
By clicking “Send”, you agree to our Terms of service and Privacy statement . We will occasionally send you account related emails.
Where do you want us to send this sample?
By clicking “Continue”, you agree to our terms of service and privacy policy.
Be careful. This essay is not unique
This essay was donated by a student and is likely to have been used and submitted before
Download this Sample
Free samples may contain mistakes and not unique parts
Sorry, we could not paraphrase this essay. Our professional writers can rewrite it and get you a unique paper.
Please check your inbox.
We can write you a custom essay that will follow your exact instructions and meet the deadlines. Let's fix your grades together!
Get Your Personalized Essay in 3 Hours or Less!
We use cookies to personalyze your web-site experience. By continuing we’ll assume you board with our cookie policy .
- Instructions Followed To The Letter
- Deadlines Met At Every Stage
- Unique And Plagiarism Free
- Search Menu
- Advance articles
- Editor's Choice
- Supplements
- Author Guidelines
- Submission Site
- Open Access
- About Journal of Public Health
- About the Faculty of Public Health of the Royal Colleges of Physicians of the United Kingdom
- Editorial Board
- Self-Archiving Policy
- Dispatch Dates
- Advertising and Corporate Services
- Journals Career Network
- Journals on Oxford Academic
- Books on Oxford Academic

Article Contents
Acknowledgment, conflict of interest, authors’ contribution.
- < Previous
Face-to-face classes during COVID-19: a call for deliberate and well-planned school health protocols in the Philippine context
- Article contents
- Figures & tables
- Supplementary Data
Philip Joseph D Sarmiento, Cora Lyn T Sarmiento, Rina Lyn B Tolentino, Face-to-face classes during COVID-19: a call for deliberate and well-planned school health protocols in the Philippine context, Journal of Public Health , Volume 43, Issue 2, June 2021, Pages e305–e306, https://doi.org/10.1093/pubmed/fdab006
- Permissions Icon Permissions
Schooling is one of the most affected aspects of human life due to coronavirus disease-2019 (COVID-19) pandemic. In a recent correspondence published, the authors reminded every country of their responsibility to come up with strategies to reopen schools safely. This paper reiterates the adherence of school health protocols as significant in the delivery of face-to-face classes following national and international guidelines in mitigating the effects of COVID-19 pandemic as a public health crisis.
Schooling is one of the most affected aspects of human life due to coronavirus disease-2019 (COVID-19) pandemic. Since the rise and threat of the pandemic, many countries around the world have decided to temporarily close schools that have affected millions of students. 1 Consequently, students who are mostly children have been facing a learning crisis due to the pandemic. 2 In a recent correspondence published in this journal, the authors cited that every country has the responsibility to come up with strategies to reopen schools in a safe manner. 3
In the Philippines, the government’s Department of Education has come up with guidelines to implement online and modular distance learning delivery of instruction. 4 This is to safeguard students from being infected by the disease. However, plans to conduct the pilot implementation of limited face-to-face delivery in low-risk areas of COVID-19 transmission for January 2021 have been approved by the president 5 but later recalled 6 due to the threat of the new strain of COVID-19. Predicaments are raised whether the country is ready to open its schools for students to go for face-to-face learning despite having been one of the longest and strictest lockdowns in the world.
School reopening for face-to-face interactions must be carefully planned to ensure the safety of students as well as teachers and school staff in a staged fashion especially in following physical distancing. 7 , 8 Planning and execution of school health protocols during this pandemic must be supported by the truthful data 9 being given by various institutions. Last 11 December 2020, the World Health Organization (WHO) has published a checklist to support school reopening and the preparation for the possible resurgence of COVID-19. 10 WHO cited that ‘The checklist is aligned with, and builds upon, existing COVID-19-related WHO guidelines and is structured around protective measures related to: 1) hand hygiene and respiratory etiquette; 2) physical distancing; 3) use of masks in schools; 4) environmental cleaning and ventilation; and 5) respecting procedures for isolation of all people with symptoms.’ 10 The checklist helps policymakers and school officials to enhance compliance and adherence to public health protocols in the time of the pandemic. 10
In conclusion, school health protocols in conducting face-to-face classes must be planned carefully following national and international guidelines to ensure that students will be safe or at least mitigate the effects of COVID-19. After all, students’ lives matter as education does to them. That is the responsibility of every government to ensure its fulfillment.
No funding was received from this paper.
The authors declare no conflict of interest in this paper.
All authors contributed to all aspects of the manuscript.
UNESCO . UNESCO’s support: Educational response to COVID-19 . 2020 January 1, 2021]; Available from: https://en.unesco.org/covid19/educationresponse/support .
UN . Policy Brief:The Impact of COVID-19 on children , 2020 , 1 – 17 . https://unsdg.un.org/sites/default/files/2020-04/160420_Covid_Children_Policy_Brief.pdf .
Fernandez-Guzman D , Sangster-Carrasco L , Pinedo-Soria A . COVID-19 in Latin America and the Caribbean: what is known about the status of school reopening? J Pub Health 2020 . https://doi.org/10.1093/pubmed/fdaa205 .
Google Scholar
DEPED . DepEd prepares Self-Learning Modules for education’s new normal . 2020 July 11, 2020 January 1, 2021 ]; Available from: https://www.deped.gov.ph/2020/07/02/deped-prepares-self-learning-modules-for-educations-new-normal/ .
DEPED . Official Statement on the Pilot Implementation of Limited Face-to-Face Classes , 2020 . https://www.deped.gov.ph/2020/12/15/official-statement-on-the-pilot-implementation-of-limited-face-to-face-classes/ .
DEPED . On the recall of the President’s approval of the pilot run of face-to-face classes , 2020 . https://www.deped.gov.ph/2020/12/26/on-the-recall-of-the-presidents-approval-of-the-pilot-run-of-face-to-face-classes/#:∼:text=December%2026%2C%202020%20%E2%80%93%20Secretary%20Leonor,originally%20scheduled%20in%20January%202021 .
Viner RM , Bonell C , Drake L et al. Reopening schools during the COVID-19 pandemic: governments must balance the uncertainty and risks of reopening schools against the clear harms associated with prolonged closure . Arch Dis Child Manila, Philippines: Department of Education, 2020 . https://doi.org/10.1136/archdischild-2020-319963 .
Sheikh A , Sheikh A , Sheikh Z , Dhami S . Reopening schools after the COVID-19 lockdown . J Glob Health 2020 ; 10 ( 1 ): 010376 .
Sarmiento PJD , Yap JFC , Espinosa KAG et al. The truth must prevail: citizens’ rights to know the truth during the era of COVID-19 . J Pub Health 2020 ;fdaa240. https://doi.org/10.1093/pubmed/fdaa240 .
WHO . Checklist to support schools re-opening and preparation for COVID-19 resurgences or similar public health crises . 2020 December 11, 2020 January 1, 2021]; Available from : https://www.who.int/publications/i/item/9789240017467 .
Email alerts
Citing articles via.
- Recommend to your Library
Affiliations
- Online ISSN 1741-3850
- Print ISSN 1741-3842
- Copyright © 2024 Faculty of Public Health
- About Oxford Academic
- Publish journals with us
- University press partners
- What we publish
- New features
- Open access
- Institutional account management
- Rights and permissions
- Get help with access
- Accessibility
- Advertising
- Media enquiries
- Oxford University Press
- Oxford Languages
- University of Oxford
Oxford University Press is a department of the University of Oxford. It furthers the University's objective of excellence in research, scholarship, and education by publishing worldwide
- Copyright © 2024 Oxford University Press
- Cookie settings
- Cookie policy
- Privacy policy
- Legal notice
This Feature Is Available To Subscribers Only
Sign In or Create an Account
This PDF is available to Subscribers Only
For full access to this pdf, sign in to an existing account, or purchase an annual subscription.
By providing an email address. I agree to the Terms of Use and acknowledge that I have read the Privacy Policy .
Face-to-face classes
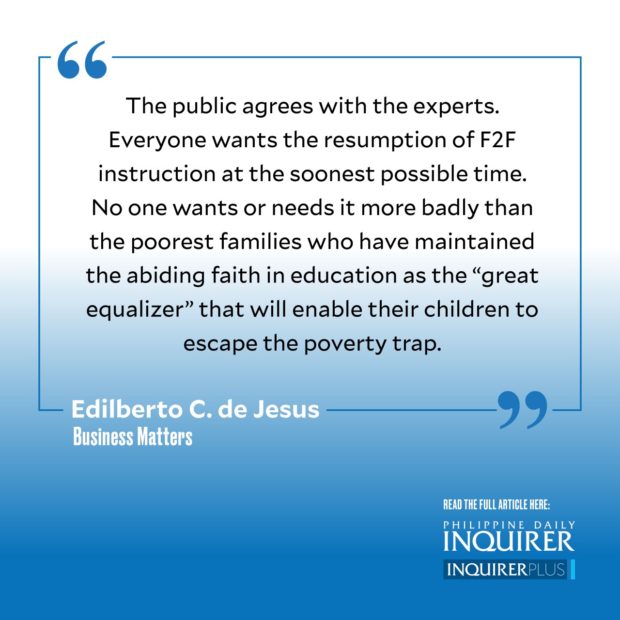
A rare consensus among experts from different disciplines point to the protracted suspension of classes, followed by the restrictions on face-to-face (F2F) instruction, as the biggest blow inflicted by the pandemic on education and society. The public agrees with the experts. Everyone wants the resumption of F2F instruction at the soonest possible time. No one wants or needs it more badly than the poorest families who have maintained the abiding faith in education as the “great equalizer” that will enable their children to escape the poverty trap.
Children have better learning prospects when parents are involved in their education. But the poor must look to the government to educate their children. If they are not literate themselves, they cannot help their children learn to read, much less to cope with the different, progressively more difficult subjects in the curriculum. They also tend to have more children requiring attention. Even if they had the ability and will, their available time must go to the more urgent task of providing shelter and food for the family. Whatever its limitations, Department of Education (DepEd) schools at least assume responsibility for the children for a portion of the day and permit their parents to earn a living.
Since no one disputes the importance of resuming regular F2F instruction, why make an issue of a mandatory requirement for all schools to reopen for in-person classes by a certain fixed date? Especially when the government is also relaxing precautionary measures against the pandemic, such as the waiver of the requirement for the vaccination of schoolchildren and the dispensation of the rule on social distancing in the classroom.
Regardless of their concerns about education, parents will still give priority to life over learning. If they fear that school attendance will mean severe risks of COVID infection, they will keep their children at home. Parents also have to worry about dealing with the potential costs of medical treatment for their children and themselves, which most of them are unprepared to bear. This is also the concern of teachers, most of whom prefer F2F education. Should parents make the understandable decision to keep their children at home because of health concerns, what would DepEd do? Punish the parents? Or the children?
From statements by DepEd and other government officials, whatever date the agency prescribes for F2F classes cannot be cast in concrete. The date must still be subject to confirmation by health authorities that the pandemic is under control. Since this assessment will likely be made on an area-by-area basis, we can also expect the local government authorities, presumably with more grounded information on health conditions, as well as the concerns of their parents and teachers, to have a better basis for deciding on the issue.
The pandemic has proven the futility of one-size-fits-all policy prescriptions. People respond differently to the virus; different areas have better protection or more resources to deal with the infection. Not all schools require the same level of precautionary measures. Having declared its resolve to restore “normal class conditions,” DepEd could have indicated a date during which it expects most schools to reopen, while empowering local authorities to determine the extent to which it will implement F2F instruction.
Why the mandatory requirement for in-person classes should apply to all private schools, which account for less than 15 percent of elementary education enrollment, also needs explanation. Children in preschool and up to Grade 3 doubtless need mainly in-person classes. Private schools that have responded best to the pandemic challenge have discovered that a blended system may work better for older students—even apart from the savings in time and money for those who have to struggle with inadequate public transportation facilities to get to school.
Some schools have invested in the acquisition of online learning resources, the development of curricula and pedagogy, and teacher training for blended education. Why should they be prohibited from building on their investments to deliver a better system to their students? DepEd must establish some checks on the academic performance of hybrid systems. But education’s “new normal” apparently need not require universal, daily campus attendance at all levels for F2F instruction.
——————
Edilberto C. de Jesus is professor emeritus at the Asian Institute of Management.
Business Matters is a project of the Makati Business Club ( [email protected] ).
Subscribe to our daily newsletter
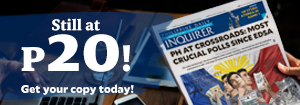
Fearless views on the news
Disclaimer: Comments do not represent the views of INQUIRER.net. We reserve the right to exclude comments which are inconsistent with our editorial standards. FULL DISCLAIMER
© copyright 1997-2024 inquirer.net | all rights reserved.
We use cookies to ensure you get the best experience on our website. By continuing, you are agreeing to our use of cookies. To find out more, please click this link.
Home / Essay Samples / Education / Online Classes / Comparing the Contrast: Online Classes VS Face-to Face Classes
Comparing the Contrast: Online Classes VS Face-to Face Classes
- Category: Education
- Topic: Online Classes
Pages: 2 (786 words)
Views: 1486
- Downloads: -->
Benefits of Online Classes
- Flexibility: One of the most significant benefits of online classes is flexibility. Students can access course materials and complete assignments at their own pace and time, making it easier to balance academic pursuits with other commitments such as work or family.
- Access to Digital Resources: Online classes offer access to a wealth of digital resources, including e-books, online libraries, and video tutorials. This allows students to access information and materials beyond what may be available in a traditional classroom setting.
- Comfortable Learning Environment: Online classes allow students to learn from the comfort of their own homes or any other place with internet access. This can create a more relaxed and comfortable learning environment for students, leading to improved focus and retention.
- Lower Cost: Online classes are generally more affordable than traditional face-to-face classes as they do not require physical facilities or transportation costs. This can make higher education more accessible to individuals who may not have the financial means to attend traditional classes.
Disadvantages of Online Classes
- Limited Personal Interaction: Online classes lack the personal interaction that face-to-face classes provide, making it difficult for students to develop strong relationships with instructors and peers. This can limit opportunities for discussion, collaboration, and feedback.
- Lack of Structure: Online classes require self-discipline and motivation to stay on track, as students are responsible for managing their time and completing assignments without direct supervision. This lack of structure can be challenging for some students and may lead to procrastination or lack of engagement.
- Technical Issues: Online classes rely heavily on technology, such as high-speed internet and specific software. Technical issues can arise that can disrupt learning, and students may need to be proficient with technology to succeed.
- Cheating: Online classes can make it easier for students to cheat on assignments or exams, as there is less direct supervision and oversight.
Benefits of Face-to-Face Classes
- Personal Interaction: One of the most significant benefits of face-to-face classes is the personal interaction that takes place between students, instructors, and peers. This creates opportunities for discussion, collaboration, and feedback that are not possible in an online environment.
- Structured Learning Environment: Face-to-face classes provide a structured learning environment that can be beneficial for some students. This can help students stay on track, manage their time effectively, and stay engaged with the material.
- Active Learning: Face-to-face classes often incorporate active learning strategies such as group work, role-playing, and hands-on activities. This can enhance the learning experience and help students develop practical skills that may be difficult to learn in an online environment.
- Networking Opportunities: Face-to-face classes provide networking opportunities that can be beneficial for students' future careers. This can include building relationships with instructors and peers, attending events, and joining student organizations.
Disadvantages of Face-to-Face Classes
- Limited Flexibility: Face-to-face classes have set schedules and attendance requirements, which can be challenging for students who have other commitments such as work or family.
- Cost: Face-to-face classes can be more expensive than online classes as they require physical facilities, transportation costs, and materials such as textbooks.
- Commuting: Face-to-face classes require commuting to a physical location, which can be time-consuming and costly.
- Limited Access to Digital Resources: Face-to-face classes may have limited access to digital resources, such as e-books and online libraries, which are readily available in an online environment.
--> ⚠️ Remember: This essay was written and uploaded by an--> click here.
Found a great essay sample but want a unique one?
are ready to help you with your essay
You won’t be charged yet!
Reading Essays
Homework Essays
Importance of Education Essays
Indian Education Essays
Critical Thinking Essays
Related Essays
We are glad that you like it, but you cannot copy from our website. Just insert your email and this sample will be sent to you.
By clicking “Send”, you agree to our Terms of service and Privacy statement . We will occasionally send you account related emails.
Your essay sample has been sent.
In fact, there is a way to get an original essay! Turn to our writers and order a plagiarism-free paper.
samplius.com uses cookies to offer you the best service possible.By continuing we’ll assume you board with our cookie policy .--> -->