Help | Advanced Search
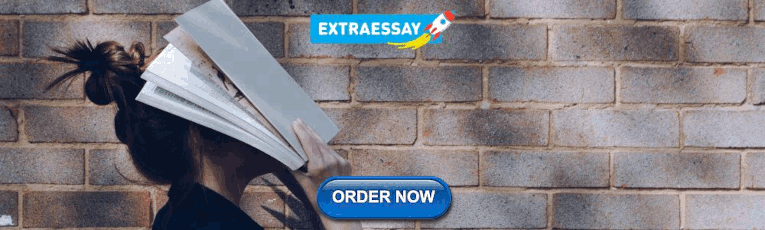
Electrical Engineering and Systems Science > Signal Processing
Title: a review of driver drowsiness detection systems: techniques, advantages and limitations.
Abstract: Driver Drowsiness is one of the most factors of road accidents, leading to severe injuries and deaths every year. Drowsiness means difficulty staying awake, which can lead to falling asleep. This paper introduces a literature review of driver drowsiness detection systems based on an analysis of physiological signals, facial features, and driving patterns. The paper also presents and details the recently proposed techniques for each class. We have also provided a comparative study of recently published works regarding the accuracy, reliability, hardware requirement, and intrusiveness. We have summarized and discussed the advantages and limitations of each class. As a result, each class of techniques has advantages and limitations. A hybrid system that combines two and more techniques will be efficient, robust, accurate, and used in real-time to take advantage of each technique.
Submission history
Access paper:.
- Other Formats

References & Citations
- Google Scholar
- Semantic Scholar
BibTeX formatted citation

Bibliographic and Citation Tools
Code, data and media associated with this article, recommenders and search tools.
- Institution
arXivLabs: experimental projects with community collaborators
arXivLabs is a framework that allows collaborators to develop and share new arXiv features directly on our website.
Both individuals and organizations that work with arXivLabs have embraced and accepted our values of openness, community, excellence, and user data privacy. arXiv is committed to these values and only works with partners that adhere to them.
Have an idea for a project that will add value for arXiv's community? Learn more about arXivLabs .
Driver drowsiness detection using behavioral measures and machine learning techniques: A review of state-of-art techniques
Ieee account.
- Change Username/Password
- Update Address
Purchase Details
- Payment Options
- Order History
- View Purchased Documents
Profile Information
- Communications Preferences
- Profession and Education
- Technical Interests
- US & Canada: +1 800 678 4333
- Worldwide: +1 732 981 0060
- Contact & Support
- About IEEE Xplore
- Accessibility
- Terms of Use
- Nondiscrimination Policy
- Privacy & Opting Out of Cookies
A not-for-profit organization, IEEE is the world's largest technical professional organization dedicated to advancing technology for the benefit of humanity. © Copyright 2024 IEEE - All rights reserved. Use of this web site signifies your agreement to the terms and conditions.
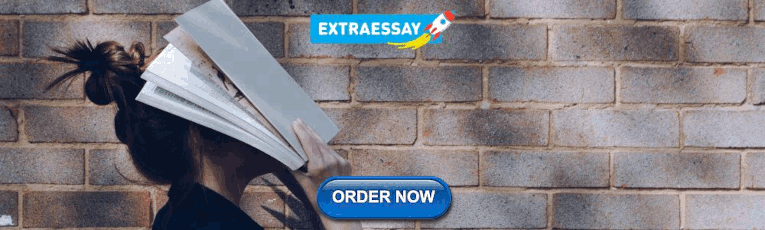
Driver Drowsiness Detection for Road Safety Using Deep Learning
- Conference paper
- First Online: 26 May 2023
- Cite this conference paper
- Parul Saini 40 ,
- Krishan Kumar 40 ,
- Shamal Kashid 40 ,
- Alok Negi 40 &
- Ashray Saini 40
Part of the book series: Lecture Notes in Electrical Engineering ((LNEE,volume 1009))
243 Accesses
1 Citations
Detecting sleepiness in drivers while driving is essential in order to prevent accidents and reduce the number of mortality caused by drivers sleeping behind the wheels. With the rapid growth of the population, the number of accidents on the roads has increased dramatically, and many people have died as a result of those accidents. It’s a serious issue that needs to be addressed in order to reduce accidents. According to research conducted by the National Sleep Foundation, roughly 20% of drivers experience drowsiness while driving. These figures paint a terrible picture. This paper discusses a method for reducing accidents due to driver drowsiness. The VGG-16 architecture is used for detecting driver drowsiness and achieved 96.79% accuracy with 0.08 loss.
This is a preview of subscription content, log in via an institution to check access.
Access this chapter
- Available as PDF
- Read on any device
- Instant download
- Own it forever
- Available as EPUB and PDF
- Durable hardcover edition
- Dispatched in 3 to 5 business days
- Free shipping worldwide - see info
Tax calculation will be finalised at checkout
Purchases are for personal use only
Institutional subscriptions
Balam VP, Sameer VU, Chinara S (2021) Automated classification system for drowsiness detection using convolutional neu ral network and electroencephalogram. IET Intell Transp Syst 15(4):514–524
Google Scholar
Dua M, Singla R, Raj S, Jangra A (2021) Deep CNN models-based ensemble approach to driver drowsiness detection. Neural Comput Appl 33(8):3155–3168
Article Google Scholar
Dang K, Sharma S (2017) Review and comparison of face detection algorithms. In: 2017 7th international conference on cloud computing, data scienceand engineering confluence. IEEE, pp 629–633
VenkataRamiReddy C, Kishore KK, Bhattacharyya D, Kim TH (2014) Multi-feature fusion based facial expression classification using DLBPand DCT. Int J Softw Eng Its Appl 8(9):55–68
Ramireddy CV, Kishore KK (2013)Facial expression classification using Kernel based PCA with fused DCT and GWT features. In: 2013 IEEE international conference on computational intelligence and computing research. IEEE, pp 1–6
Chirra VR, Reddy SR, Kolli VKK (2019) Deep CNN: a machine learning approach for driver drowsiness detection based on eye state. Rev d’Intelligence Artif 33(6):461–466
Altameem A, Kumar A, Poonia RC, Kumar S, Saudagar AKJ (2021) Early identification and detection of driver drowsiness by hybrid machine learning. IEEE Access 9:162805–162819
Esteves T, Pinto JR, Ferreira PM, Costa PA, Rodrigues LA, Antunes I, ... Rebelo A (2021) AUTOMOTIVE: a case study on AUTOmatic multiMOdal drowsiness detecTIon for smart VEhicles. IEEE Access 9:153678–153700
Negi A, Kumar K, Chauhan P, Rajput RS (2021) Deep neu ral architecture for face mask detection on simulated masked face dataset against COVID-19 pandemic. In: 2021 international conference on computing, communication, and intelligent systems (ICCCIS). IEEE, pp 595–600
Babaeian M, Mozumdar M (2019)Driver drowsiness detection algorithms using electrocardiogram data analysis. In: 2019 IEEE 9th annual computing and communication workshop and conference (CCWC). IEEE, pp 0001–0006
Jabbar R, Shinoy M, Kharbeche M, Al-Khalifa K, Krichen M, Barkaoui K (2020) Driver drowsiness detection model using convolutional neural networks techniques for android application. In: 2020 IEEE international conference on informatics, IoT, and enabling technologies (ICIoT). IEEE, pp237–242
Saifuddin AFM, Mahayuddin ZR (2020) Robust drowsiness detection for vehicle driver using deep convolutional neural network. Int J Adv Comput Sci Appl 11(10)
McDonald AD, Lee JD, Schwarz C, Brown TL (2018) A contextual and temporal algorithm for driver drowsiness detection. Accid Anal Prev 113:25–37
Zhao L, Wang Z, Wang X, Liu Q (2018) Driver drowsiness detection using facial dynamic fusion information and a DBN. IET Intel Transp Syst 12(2):127–133
Reddy B, Kim YH, Yun S, Seo C, Jang J (2017)Real-time driver drowsiness detection for embedded system using model compression of deep neural networks. In: Proceedings of the IEEE conference on computer vision and pattern recognition workshops, pp 121–128
Jabbar R, Al-Khalifa K, Kharbeche M, Alhajyaseen W, Jafari M, Jiang S (2018) Real-time driver drowsiness detection for android application using deep neural networks techniques. Procedia Comput Sci 130:400–407
Deng W, Ruoxue W (2019) Real-time driver-drowsiness detection system using facial features. IEEE Access 7:118727–118738
Download references
Author information
Authors and affiliations.
Computer Science and Engineering, National Institute of Technology, Srinagar, Uttarakhand, India
Parul Saini, Krishan Kumar, Shamal Kashid, Alok Negi & Ashray Saini
You can also search for this author in PubMed Google Scholar
Corresponding author
Correspondence to Parul Saini .
Editor information
Editors and affiliations.
National Institute of Technology Uttarakhand, Srinagar, India
Hariharan Muthusamy
Eötvös Loránd University, Budapest, Hungary
János Botzheim
Queensland University of Technology, Brisbane, QLD, Australia
Richi Nayak
Rights and permissions
Reprints and permissions
Copyright information
© 2023 The Author(s), under exclusive license to Springer Nature Singapore Pte Ltd.
About this paper
Cite this paper.
Saini, P., Kumar, K., Kashid, S., Negi, A., Saini, A. (2023). Driver Drowsiness Detection for Road Safety Using Deep Learning. In: Muthusamy, H., Botzheim, J., Nayak, R. (eds) Robotics, Control and Computer Vision. Lecture Notes in Electrical Engineering, vol 1009. Springer, Singapore. https://doi.org/10.1007/978-981-99-0236-1_15
Download citation
DOI : https://doi.org/10.1007/978-981-99-0236-1_15
Published : 26 May 2023
Publisher Name : Springer, Singapore
Print ISBN : 978-981-99-0235-4
Online ISBN : 978-981-99-0236-1
eBook Packages : Intelligent Technologies and Robotics Intelligent Technologies and Robotics (R0)
Share this paper
Anyone you share the following link with will be able to read this content:
Sorry, a shareable link is not currently available for this article.
Provided by the Springer Nature SharedIt content-sharing initiative
- Publish with us
Policies and ethics
- Find a journal
- Track your research
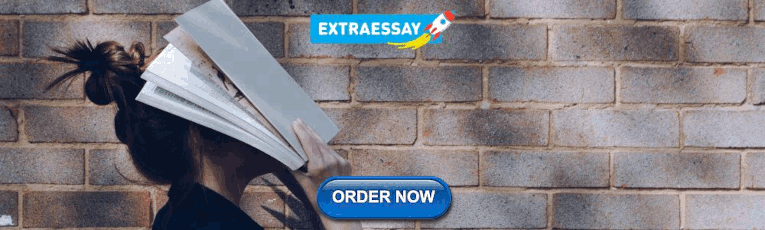
IMAGES
VIDEO
COMMENTS
In order to detect the different stages of drowsiness, researchers have studied driver responses and vehicle driving patterns. In this section, we provide a review of the four widely used measures for DDD. The diagram in Figure 1 illustrates all the currently used measures for classifying driver drowsiness levels.
Abstract. Driver drowsiness has emerged as one of the key factors in recent times' traffic accidents, which can result in fatalities, serious physical losses, large monetary losses, and significant property damage. Drowsiness in a driver can be brought on by long hours behind the wheel, drowsiness, fatigue, medicine, difficulty sleeping, and ...
Traffic accidents always cause great material and human losses. One of the most important causes of these accidents is the human factor, which is usually caused by fatigue or drowsiness. To address this problem, several approaches were proposed to predict the driver state. Some solutions are based on the measurement of the driver behavior such as: the head movement, the duration of the blink ...
Drowsiness among drivers is a significant contributing factor to traffic injuries, leading to a yearly toll of severe injuries and fatalities. This study reviews the literature in-depth on driving force sleepiness detection systems, considering physiological signals, facial expressions, and riding habits. It not only describes the current approaches for each class but also offers a comparative ...
Caryn FH, Rahadianti L (2021) Driver drowsiness detection based on drivers' physical behaviours: a systematic literature review. Comput Eng Appl J 10:161-175. Google Scholar Chang T-C, Wu M-H, Kim P-Z, Yu M-H (2021) Smart driver drowsiness detection model based on analytic hierarchy process. Sens Mater 33:485-497
study determined that 4.8% of trips involved a driver in KSS levels 6-9, for which drowsy driving is present (See Figure 1). Most drowsy drivers report only low levels of drowsiness (Some signs of drowsiness = 3.3%). A driver makes only 0.5% of trips with "Sleepy, some effort to keep alert" and 0.1% with "Extremely Sleepy, fighting sleep".
This paper introduces a literature review of driver drowsiness detection systems based on an analysis of physiological signals, facial features, and driving patterns.
The results showed that the RF classifier gave the best. results, with 82.4% accuracy of alert vs slightly drowsy case. In contrast, majority voting. performed the best for alert vs moderately ...
Literature Review on Driver's Drowsiness and Fatigue Detection. June 2020. DOI: 10.1109/ISCV49265.2020.9204306. Conference: 4th International Conference on Intelligent Systems and Computer ...
Section 2 presents the literature review on drowsiness detection with deep learning networks. ... In order to overcome this issue, we construct a driver drowsiness detection system using deep learning combined with IoT to be able to detect, alert and potentially save a person's life. For our system, a surveillance camera is used to capture ...
Driver Drowsiness is one of the most factors of road accidents, leading to severe injuries and deaths every year. Drowsiness means difficulty staying awake, which can lead to falling asleep. This paper introduces a literature review of driver drowsiness detection systems based on an analysis of physiological signals, facial features, and driving patterns. The paper also presents and details ...
This paper presents a literature review of driver drowsiness detection based on behavioral measures using machine learning techniques. Faces contain information that can be used to interpret levels of drowsiness. There are many facial features that can be extracted from the face to infer the level of drowsiness. These include eye blinks, head movements and yawning. However, the development of ...
Detecting drowsiness among drivers is critical for ensuring road safety and preventing accidents caused by drowsy or fatigued driving. Research on yawn detection among drivers has great significance in improving traffic safety. Although various studies have taken place where deep learning-based approaches are being proposed, there is still room for improvement to develop better and more ...
Abstract. This work presented analysis and implementation of driver drowsiness, distraction, and detection systems using image processing techniques. The literature review based on drowsiness, distraction, and detection have been taken with their parameters in tabulation form. Flow charts of software and hardware have been presented for the ...
This section describes Drowsiness Detection models [7, 8] and their limitations, along with some deep learning [] processes that can automatically be fea tured directly from the raw data.Babaeian et al. [] introduced a unique technique for measuring driver drowsiness that uses biomedical signal analysis based on machine learning and is applied to heart rate variation (HRV), which is measured ...
In recent years, driver drowsiness has been one of the major causes of road accidents and can lead to severe physical injuries, deaths and significant economic losses. Statistics indicate the need of a reliable driver drowsiness detection system which could alert the driver before a mishap happens. Researchers have attempted to determine driver drowsiness using the following measures: (1 ...
DRIVER DROWSINESS DETECTION SYSTEM. School of Computer Engineering, KIIT, BBSR [4] ABSTRACT. This document is a review report on the research conducted and the project made. in the field of ...
A literature review on the recent related works in this field of measurement approach based on the measurements of the physiological signals to get information about the internal state of the driver's body is presented. Traffic accidents always cause great material and human losses. One of the most important causes of these accidents is the human factor, which is usually caused by fatigue or ...
The percentage of eyelid closure over the pupil over time (PERCLOS) is one of the major methods for the detection of the driver's drowsiness. Physiological measurements like electroencephalogram (EEG), electrocardiogram (ECG) [4], capturing eye closure, facial features [5] [6], or driving performance (such as steering characteristics, lane ...
1. This paper relates the street mishaps that happen because of driver's drowsiness. Recent studies stat e that more disasters. are caused due to doziness. Drivers can feel dr. owsiness due to sle ...