
- Walden University
- Faculty Portal
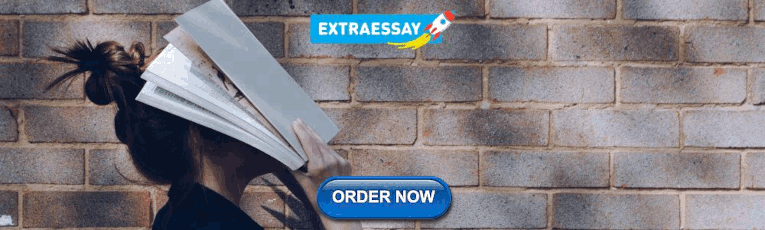
Writing a Paper: Comparing & Contrasting
A compare and contrast paper discusses the similarities and differences between two or more topics. The paper should contain an introduction with a thesis statement, a body where the comparisons and contrasts are discussed, and a conclusion.
Address Both Similarities and Differences
Because this is a compare and contrast paper, both the similarities and differences should be discussed. This will require analysis on your part, as some topics will appear to be quite similar, and you will have to work to find the differing elements.
Make Sure You Have a Clear Thesis Statement
Just like any other essay, a compare and contrast essay needs a thesis statement. The thesis statement should not only tell your reader what you will do, but it should also address the purpose and importance of comparing and contrasting the material.
Use Clear Transitions
Transitions are important in compare and contrast essays, where you will be moving frequently between different topics or perspectives.
- Examples of transitions and phrases for comparisons: as well, similar to, consistent with, likewise, too
- Examples of transitions and phrases for contrasts: on the other hand, however, although, differs, conversely, rather than.
For more information, check out our transitions page.
Structure Your Paper
Consider how you will present the information. You could present all of the similarities first and then present all of the differences. Or you could go point by point and show the similarity and difference of one point, then the similarity and difference for another point, and so on.
Include Analysis
It is tempting to just provide summary for this type of paper, but analysis will show the importance of the comparisons and contrasts. For instance, if you are comparing two articles on the topic of the nursing shortage, help us understand what this will achieve. Did you find consensus between the articles that will support a certain action step for people in the field? Did you find discrepancies between the two that point to the need for further investigation?
Make Analogous Comparisons
When drawing comparisons or making contrasts, be sure you are dealing with similar aspects of each item. To use an old cliché, are you comparing apples to apples?
- Example of poor comparisons: Kubista studied the effects of a later start time on high school students, but Cook used a mixed methods approach. (This example does not compare similar items. It is not a clear contrast because the sentence does not discuss the same element of the articles. It is like comparing apples to oranges.)
- Example of analogous comparisons: Cook used a mixed methods approach, whereas Kubista used only quantitative methods. (Here, methods are clearly being compared, allowing the reader to understand the distinction.
Related Webinar

Didn't find what you need? Email us at [email protected] .
- Previous Page: Developing Arguments
- Next Page: Avoiding Logical Fallacies
- Office of Student Disability Services
Walden Resources
Departments.
- Academic Residencies
- Academic Skills
- Career Planning and Development
- Customer Care Team
- Field Experience
- Military Services
- Student Success Advising
- Writing Skills
Centers and Offices
- Center for Social Change
- Office of Academic Support and Instructional Services
- Office of Degree Acceleration
- Office of Research and Doctoral Services
- Office of Student Affairs
Student Resources
- Doctoral Writing Assessment
- Form & Style Review
- Quick Answers
- ScholarWorks
- SKIL Courses and Workshops
- Walden Bookstore
- Walden Catalog & Student Handbook
- Student Safety/Title IX
- Legal & Consumer Information
- Website Terms and Conditions
- Cookie Policy
- Accessibility
- Accreditation
- State Authorization
- Net Price Calculator
- Contact Walden
Walden University is a member of Adtalem Global Education, Inc. www.adtalem.com Walden University is certified to operate by SCHEV © 2024 Walden University LLC. All rights reserved.

Want to create or adapt books like this? Learn more about how Pressbooks supports open publishing practices.
10.7 Comparison and Contrast
Learning objectives.
- Determine the purpose and structure of comparison and contrast in writing.
- Explain organizational methods used when comparing and contrasting.
- Understand how to write a compare-and-contrast essay.
The Purpose of Comparison and Contrast in Writing
Comparison in writing discusses elements that are similar, while contrast in writing discusses elements that are different. A compare-and-contrast essay , then, analyzes two subjects by comparing them, contrasting them, or both.
The key to a good compare-and-contrast essay is to choose two or more subjects that connect in a meaningful way. The purpose of conducting the comparison or contrast is not to state the obvious but rather to illuminate subtle differences or unexpected similarities. For example, if you wanted to focus on contrasting two subjects you would not pick apples and oranges; rather, you might choose to compare and contrast two types of oranges or two types of apples to highlight subtle differences. For example, Red Delicious apples are sweet, while Granny Smiths are tart and acidic. Drawing distinctions between elements in a similar category will increase the audience’s understanding of that category, which is the purpose of the compare-and-contrast essay.
Similarly, to focus on comparison, choose two subjects that seem at first to be unrelated. For a comparison essay, you likely would not choose two apples or two oranges because they share so many of the same properties already. Rather, you might try to compare how apples and oranges are quite similar. The more divergent the two subjects initially seem, the more interesting a comparison essay will be.
Writing at Work
Comparing and contrasting is also an evaluative tool. In order to make accurate evaluations about a given topic, you must first know the critical points of similarity and difference. Comparing and contrasting is a primary tool for many workplace assessments. You have likely compared and contrasted yourself to other colleagues. Employee advancements, pay raises, hiring, and firing are typically conducted using comparison and contrast. Comparison and contrast could be used to evaluate companies, departments, or individuals.
Brainstorm an essay that leans toward contrast. Choose one of the following three categories. Pick two examples from each. Then come up with one similarity and three differences between the examples.
- Romantic comedies
- Internet search engines
- Cell phones
Brainstorm an essay that leans toward comparison. Choose one of the following three items. Then come up with one difference and three similarities.
- Department stores and discount retail stores
- Fast food chains and fine dining restaurants
- Dogs and cats
The Structure of a Comparison and Contrast Essay
The compare-and-contrast essay starts with a thesis that clearly states the two subjects that are to be compared, contrasted, or both and the reason for doing so. The thesis could lean more toward comparing, contrasting, or both. Remember, the point of comparing and contrasting is to provide useful knowledge to the reader. Take the following thesis as an example that leans more toward contrasting.
Thesis statement: Organic vegetables may cost more than those that are conventionally grown, but when put to the test, they are definitely worth every extra penny.
Here the thesis sets up the two subjects to be compared and contrasted (organic versus conventional vegetables), and it makes a claim about the results that might prove useful to the reader.
You may organize compare-and-contrast essays in one of the following two ways:
- According to the subjects themselves, discussing one then the other
- According to individual points, discussing each subject in relation to each point
See Figure 10.1 “Comparison and Contrast Diagram” , which diagrams the ways to organize our organic versus conventional vegetables thesis.
Figure 10.1 Comparison and Contrast Diagram
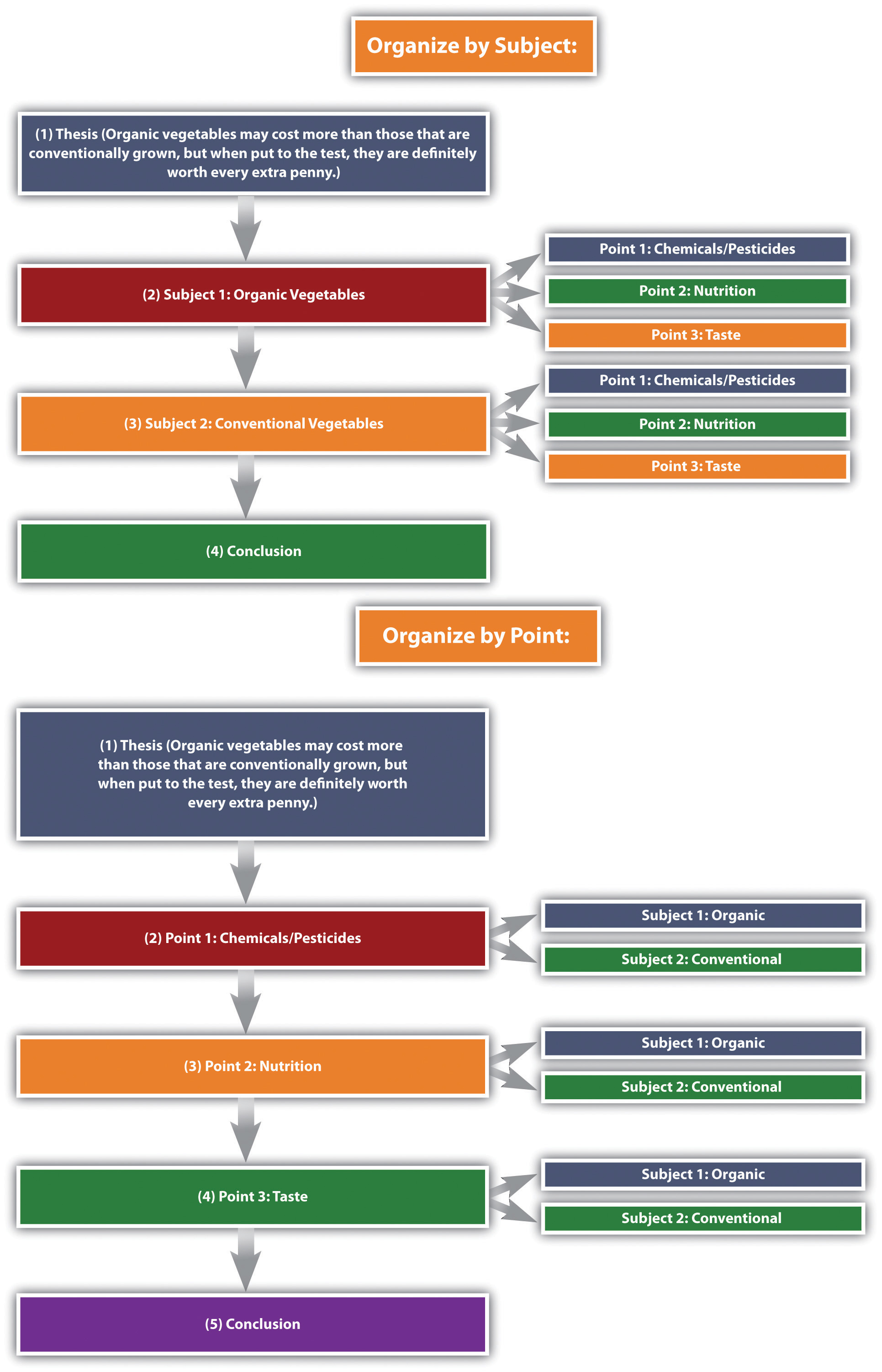
The organizational structure you choose depends on the nature of the topic, your purpose, and your audience.
Given that compare-and-contrast essays analyze the relationship between two subjects, it is helpful to have some phrases on hand that will cue the reader to such analysis. See Table 10.3 “Phrases of Comparison and Contrast” for examples.
Table 10.3 Phrases of Comparison and Contrast
Create an outline for each of the items you chose in Note 10.72 “Exercise 1” and Note 10.73 “Exercise 2” . Use the point-by-point organizing strategy for one of them, and use the subject organizing strategy for the other.
Writing a Comparison and Contrast Essay
First choose whether you want to compare seemingly disparate subjects, contrast seemingly similar subjects, or compare and contrast subjects. Once you have decided on a topic, introduce it with an engaging opening paragraph. Your thesis should come at the end of the introduction, and it should establish the subjects you will compare, contrast, or both as well as state what can be learned from doing so.
The body of the essay can be organized in one of two ways: by subject or by individual points. The organizing strategy that you choose will depend on, as always, your audience and your purpose. You may also consider your particular approach to the subjects as well as the nature of the subjects themselves; some subjects might better lend themselves to one structure or the other. Make sure to use comparison and contrast phrases to cue the reader to the ways in which you are analyzing the relationship between the subjects.
After you finish analyzing the subjects, write a conclusion that summarizes the main points of the essay and reinforces your thesis. See Chapter 15 “Readings: Examples of Essays” to read a sample compare-and-contrast essay.
Many business presentations are conducted using comparison and contrast. The organizing strategies—by subject or individual points—could also be used for organizing a presentation. Keep this in mind as a way of organizing your content the next time you or a colleague have to present something at work.
Choose one of the outlines you created in Note 10.75 “Exercise 3” , and write a full compare-and-contrast essay. Be sure to include an engaging introduction, a clear thesis, well-defined and detailed paragraphs, and a fitting conclusion that ties everything together.
Key Takeaways
- A compare-and-contrast essay analyzes two subjects by either comparing them, contrasting them, or both.
- The purpose of writing a comparison or contrast essay is not to state the obvious but rather to illuminate subtle differences or unexpected similarities between two subjects.
- The thesis should clearly state the subjects that are to be compared, contrasted, or both, and it should state what is to be learned from doing so.
There are two main organizing strategies for compare-and-contrast essays.
- Organize by the subjects themselves, one then the other.
- Organize by individual points, in which you discuss each subject in relation to each point.
- Use phrases of comparison or phrases of contrast to signal to readers how exactly the two subjects are being analyzed.
Writing for Success Copyright © 2015 by University of Minnesota is licensed under a Creative Commons Attribution-NonCommercial-ShareAlike 4.0 International License , except where otherwise noted.
- Writing Worksheets and Other Writing Resources
- Thesis, Analysis, & Structure
Comparing and Contrasting
About the slc.
- Our Mission and Core Values

The professor says to compare and contrast A and B ...
Determining the Structure of your Essay:
Determining the structure of your essay is the most important step towards conducting and presenting to the reader a well-developed comparison. Students are often asked to compare things in twos. For example, compare these two articles, or two characters in a novel, or a film and a novel or an article and a poem... The possibilities are endless.
When you are faced with the task of having to compare and contrast, it can be overwhelming. You're thinking about two pieces of writing that you know are different, and perhaps there are some similarities, too, but how can you suddenly start talking about them both? Which one should I talk about first? Which one should I talk about last?
Sometimes, comparisons are done in the following manner:
You pick one article to describe: Article A. Then you talk about Article B. Perhaps at the end, you talk about the similarities in both articles.
This format will consist of three main parts: A, B, and, finally, their similarities.
Although this format is an acceptable way of making comparisons, and it is sometimes used to present well-developed "compare and contrast" essays, the format has its weaknesses that can jeopardize an effective comparison.
What could happen when you use this format and you completely isolate Article A from Article B is that you make it more difficult to compare. Your final essay might end up divided in two parts: half of the paper talks about only Article A and the second half talks about only Article B . You do not want to split your essay into a description of Article A and a description of Article B because then it will be harder to compare them since you invested most of your energy into describing them and not comparing them.
How to avoid the "Split Essay": A Second Option for Comparison
The best way to avoid the Split Essay is to unify both split ends. Do not discuss Article B at the end. Talk about both A and B from the beginning. The question now is:
What do I do to eliminate the Split?
Break it down:
You do not get rid of the gap between the two halves of the essay that are split. You simply break it down . This is done by finding common themes, or points of comparison in Article A and Article B. Once you find those points of comparison, you can discuss each individual theme and how each shows up in Article A and B . Consider the following questions:
- What major themes are discussed in each of the essays?
- What doe the writer of Article A say about the first theme, and how is this similar to or different than what the writer of Article B says about the same topic?
- What conclusions can you make about these differences or similarities?
After developing a thorough explanation of the first theme, you can mow move on to discuss the second theme that appears in both essays and write about it. Ideally, each theme will be discussed thoroughly in its own paragraph, explaining how each is similar or different in Article A and Article B
During the seventies, Gabriel Garcia Marquez wrote his most famous novel, One Hundred Years of Solitude , in which he discussed themes regarding the solitude of Latin America.
In 1982, Marquez received the Nobel Prize in Literature for his novel and wrote a speech for this occasion. In his speech, he called attention to Latin American economic struggles and their historical context.
In 1990, Enrique Krauze, a Mexican economist, published an article in which he discussed the same topic: problems in Latin American economics.
The prompt says:
Compare and contrast Enrique Krauze's essay to the speech written by Marquez.
Possible approaches:
Option #1: Text by text comparison
First paragraph:
A: An explanation of Marquez's entire speech
Second paragraph:
B: An explanation of Krauze's entire essay
Third paragraph:
Similarities or differences
(this might lead to the "Split Essay" comparison)
Option #2: Point by point comparison
The breakdown: Finding common themes or points of comparison:
• Neoliberalism (free trade)
• US involvement
• Proposed solutions to the problems (macro or micro economy?)
A: Krauze's opinion on neoliberalism
B: Marquez's opinion on neoliberalism
A: US involvement good or bad? According to Marquez
B: US involvement good or bad? According to Krauze
Whatever other theme that stands out as significant for explaining the differences of opinions.
Sample paragraph:
Enrique Krauze and Gabriel Garcia Marquez take different positions in regards to the implementation of more neoliberalist policies in Latin American countries. While Krauze argues the need to expand open trade in Latin America to improve its economy, Marquez opposes this idea and argues that an open trade economy would only aid foreign investors in further exploiting the natural resources in Latin America. Krauze's support of neoliberalism is based on the idea that through a macro economy, the "undeveloped" countries will soon see the light at the end of the tunnel. On the other hand, Marquez rebuts this argument, claiming that the World Bank and the International Monetary Fund, which forced neoliberalist policies onto Latin American countries, only served to increase their foreign debt.
Notice how the beginning of this paragraph discusses only one theme: neoliberalism. Also notice how the writer was able to incorporate both articles and not just one. Pay attention, too, to the use of words and phrases that juxtapose or suggest comparison. These words establish links between A and B .
Handout created by Rubén Garibaldo, Student Learning Center, University of California, Berkeley
©2006 UC Regents
Handout revised by Carolyn Swalina, Student Learning Center, University of California, Berkeley
©2011 UC Regents
This work is licensed under a Creative Commons Attribution-NonCommercial-NoDerivs 3.0 Unported License.
- Features for Creative Writers
- Features for Work
- Features for Higher Education
- Features for Teachers
- Features for Non-Native Speakers
- Learn Blog Grammar Guide Community Events FAQ
- Grammar Guide
Comparing and Contrasting: A Guide to Improve Your Essays
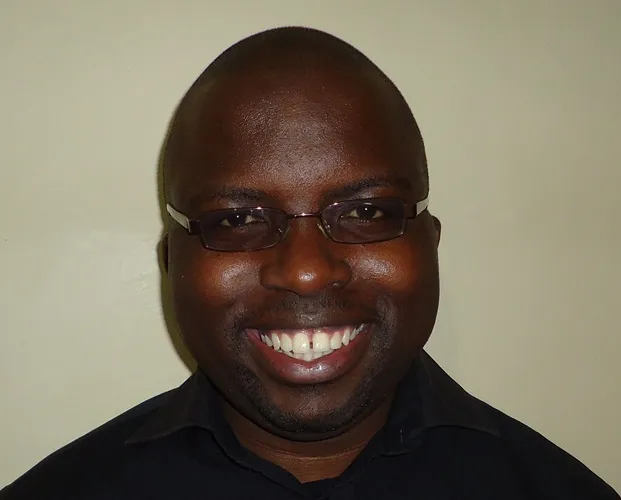
Walter Akolo
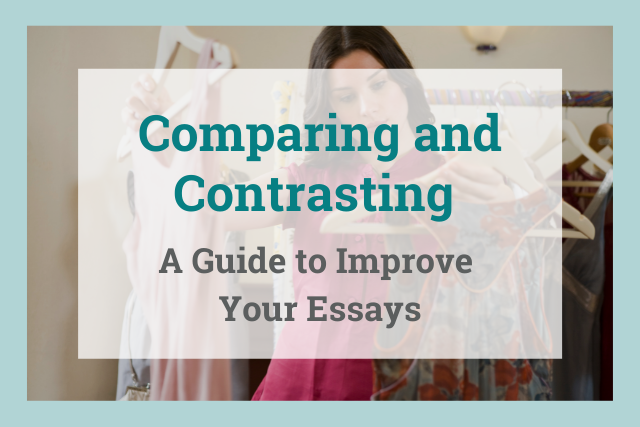
Essays that require you to compare and contrast two or more subjects, ideas, places, or items are common.
They call for you to highlight the key similarities (compare) and differences (contrast) between them.
This guide contains all the information you need to become better at writing comparing and contrasting essays.
This includes: how to structure your essay, how to decide on the content, and some examples of essay questions.
Let’s dive in.
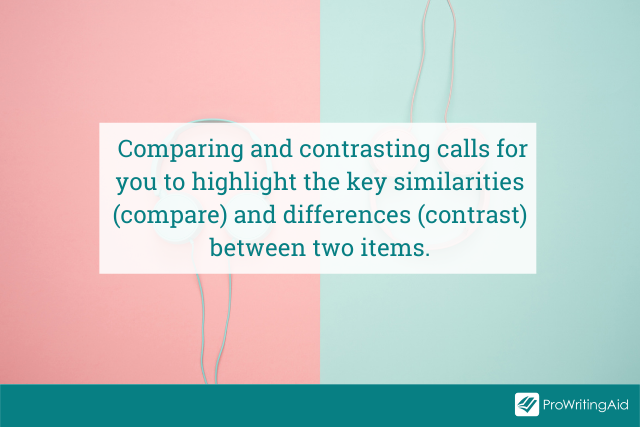
What Is Comparing and Contrasting?
Is compare and contrast the same as similarities and differences, what is the purpose of comparing and contrasting, can you compare and contrast any two items, how do you compare and contrast in writing, what are some comparing and contrasting techniques, how do you compare and contrast in college level writing, the four essentials of compare and contrast essays, what can you learn from a compare and contrast essay.
At their most basic, both comparing and contrasting base their evaluation on two or more subjects that share a connection.
The subjects could have similar characteristics, features, or foundations.
But while a comparison discusses the similarities of the two subjects, e.g. a banana and a watermelon are both fruit, contrasting highlights how the subjects or items differ from each other, e.g. a watermelon is around 10 times larger than a banana.
Any question that you are asked in education will have a variety of interesting comparisons and deductions that you can make.
Compare is the same as similarities.
Contrast is the same as differences.
This is because comparing identifies the likeness between two subjects, items, or categories, while contrasting recognizes disparities between them.
When you compare things, you represent them regarding their similarity, but when you contrast things, you define them in reference to their differences.
As a result, if you are asked to discuss the similarities and differences between two subjects, you can take an identical approach to if you are writing a compare and contrast essay.
In writing, the purpose of comparing and contrasting is to highlight subtle but important differences or similarities that might not be immediately obvious.
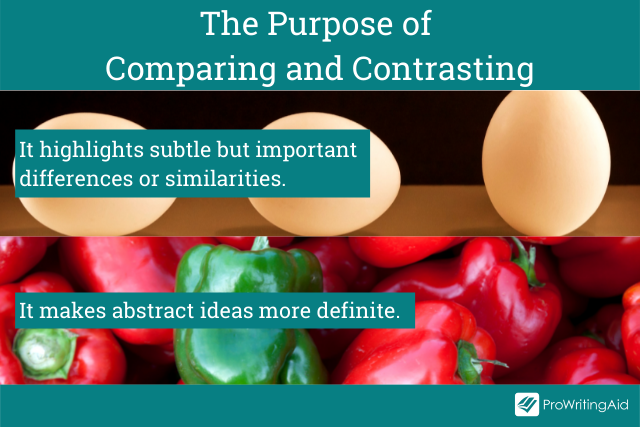
By illustrating the differences between elements in a similar category, you help heighten readers’ understanding of the subject or topic of discussion.
For instance, you might choose to compare and contrast red wine and white wine by pointing out the subtle differences. One of these differences is that red wine is best served at room temperature while white is best served chilled.
Also, comparing and contrasting helps to make abstract ideas more definite and minimizes the confusion that might exist between two related concepts.
Can Comparing and Contrasting Be Useful Outside of Academia?
Comparing enables you to see the pros and cons, allowing you to have a better understanding of the things under discussion. In an essay, this helps you demonstrate that you understand the nuances of your topic enough to draw meaningful conclusions from them.
Let's use a real-word example to see the benefits. Imagine you're contrasting two dresses you could buy. You might think:
- Dress A is purple, my favorite color, but it has a difficult zip and is practically impossible to match a jacket to.
- Dress B is more expensive but I already have a suitable pair of shoes and jacket and it is easier to move in.
You're linking the qualities of each dress to the context of the decision you're making. This is the same for your essay. Your comparison and contrast points will be in relation to the question you need to answer.
Comparing and contrasting is only a useful technique when applied to two related concepts.
To effectively compare two or more things, they must feature characteristics similar enough to warrant comparison.
In addition to this they must also feature a similarity that generates an interesting discussion. But what do I mean by “interesting” here?
Let’s look at two concepts, the Magna Carta and my third grade poetry competition entry.
They are both text, written on paper by a person so they fulfil the first requirement, they have a similarity. But this comparison clearly would not fulfil the second requirement, you would not be able to draw any interesting conclusions.
However, if we compare the Magna Carta to the Bill of Rights, you would be able to come to some very interesting conclusions concerning the history of world politics.
To write a good compare and contrast essay, it’s best to pick two or more topics that share a meaningful connection .
The aim of the essay would be to show the subtle differences or unforeseen similarities.
By highlighting the distinctions between elements in a similar category you can increase your readers’ understanding.
Alternatively, you could choose to focus on a comparison between two subjects that initially appear unrelated.
The more dissimilar they seem, the more interesting the comparison essay will turn out.
For instance, you could compare and contrast professional rugby players with marathon runners.
Can You Compare and Contrast in an Essay That Does Not Specifically Require It?
As a writer, you can employ comparing and contrasting techniques in your writing, particularly when looking for ideas you can later apply in your argument.
You can do this even when the comparison or contrast is not a requirement for the topic or argument you are presenting. Doing so could enable you to build your evaluation and develop a stronger argument.
Note that the similarities and differences you come up with might not even show up in the final draft.
While the use of compare and contrast can be neutral, you can also use it to highlight one option under discussion. When used this way, you can influence the perceived advantages of your preferred option.
As a writing style, comparing and contrasting can encompass an entire essay. However, it could also appear in some select paragraphs within the essay, where making some comparisons serves to better illustrate a point.
What Should You Do First?
Before you compare two things, always start by deciding on the reason for your comparison, then outline the criteria you will use to compare them.
Words and phrases commonly used for comparison include:
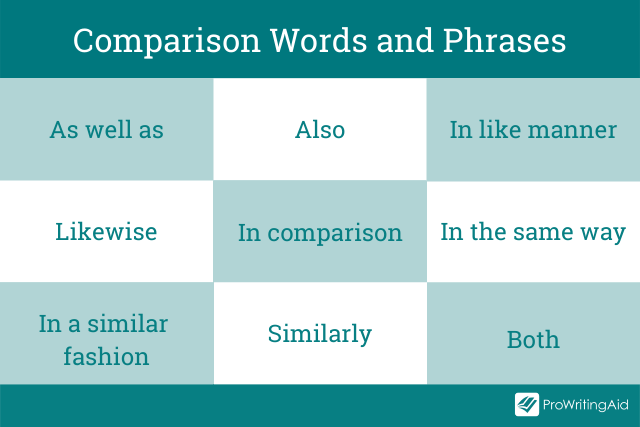
In writing, these words and phrases are called transitions . They help readers to understand or make the connection between sentences, paragraphs, and ideas.
Without transition words writing can feel clumsy and disjointed making it difficult to read. ProWritingAid’s transition report highlights all of a documents transitions and suggests that 25% of any sentences in a piece include a transition.
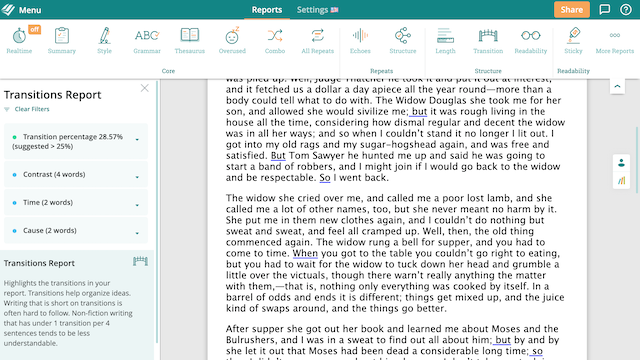
Sign up for a free ProWritingAid account to use the Transitions Report.
So, how do you form all of this into a coherent essay? It's a good idea to plan first, then decide what your paragraph layout will look like.
Venn diagrams are useful tool to start generating ideas. The, for your essay, you need to choose between going idea by idea and going point by point.
Using a Venn Diagram
A Venn diagram helps you to clearly see the similarities and differences between multiple objects, things, or subjects.
The writing tool comprises two, or more, simple, overlapping circles in which you list down the things that are alike (within the overlapping area) and those that differ (outside the overlapping area).
It’s great for brainstorming ideas and for creating your essay’s outline. You could even use it in an exam setting because it is quick and simple.
Going Subject by Subject
Going subject by subject is a structural choice for your essay.
Start by saying all you have to say on the first subject, then proceed to do the same about the second subject.
Depending on the length of your essay, you can fit the points about each subject into one paragraph or have several sections per each subject, ending with a conclusion.
This method is best for short essays on simple topics. Most university-level essays will go point by point instead.
Going Point by Point
Going point by point, or alternating, is the opposite essay structure from going subject by subject. This is ideal when you want to do more direct comparing and contrasting. It entails discussing one comparison point at a time. It allows you to use a paragraph to talk about how a certain comparing/contrasting point relates to the subjects or items you are discussing.
Alternatively, if you have lots of details about the subject, you might decide to use a paragraph for each point.
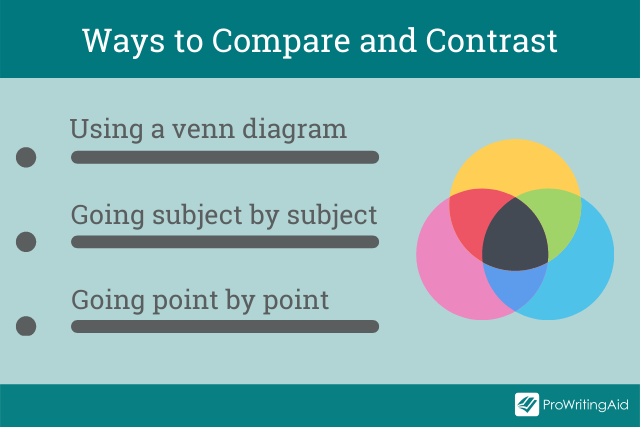
An academic compare and contrast essay looks at two or more subjects, ideas, people, or objects, compares their likeness, and contrasts their differences.
It’s an informative essay that provides insights on what is similar and different between the two items.
Depending on the essay’s instructions, you can focus solely on comparing or contrasting, or a combination of the two.
Examples of College Level Compare and Contrast Essay Questions
Here are eleven examples of compare and contrast essay questions that you might encounter at university:
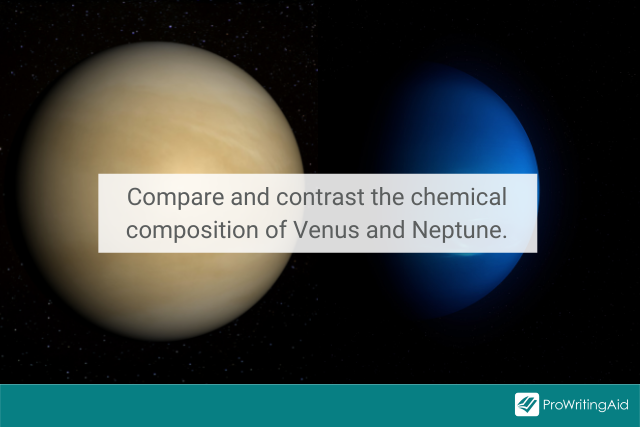
- Archaeology: Compare and contrast the skulls of homo habilis, homo erectus, and homo sapiens.
- Art: Compare and contrast the working styles of any two Neoclassic artists.
- Astrophysics: Compare and contrast the chemical composition of Venus and Neptune.
- Biology: Compare and contrast the theories of Lamarck and Darwin.
- Business: Compare and contrast 2 or more business models within the agricultural industry.
- Creative writing: Compare and contrast free indirect discourse with epistolary styles.
- English Literature: Compare and contrast William Wordsworth with Robert Browning.
- Geography: Compare and contrast the benefit of solar panels with the benefit of wind turbines.
- History: Compare and contrast WWI to WWII with specific reference to the causes and outcomes.
- Medicine: Compare and contrast England’s health service with America’s health service.
- Psychology: Compare and contrast the behaviorist theory with the psychodynamic theory.
So, the key takeaways to keep in mind are:
Have a basis for comparison. The two things need to have enough in common to justify a discussion about their similarities and disparities.
Don’t go back and forth when using the block method. The best way to write your essay is to begin with a paragraph discussing all the facets of the first topic. Then, move on to another paragraph and talk through all the aspects of the second subject.
You can use both alternating and blocking techniques. Combining the two approaches is also an option. You can apply the alternating method in some paragraphs, then switch and use the block method. This method will help you offer a much deeper analysis of the subjects.
Have a reason for comparing the two things. Only select the points of comparison that resonate with your purpose.
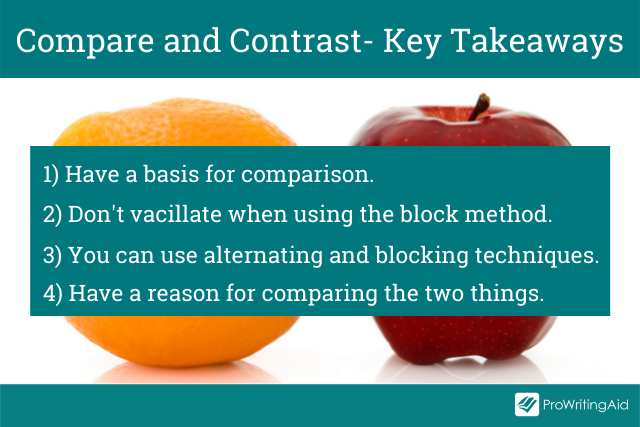
Comparing and contrasting are essential analytical skills in academic writing. When your professor issues you with such an essay, their primary goal is to teach you how to:
- Engage in critical thinking
- See and make connections between words or ideas
- Move beyond mere descriptions or summaries to developing interesting analysis
- Get a deeper understanding of the subjects or items under comparison, their key features, and their interrelationships with each other.
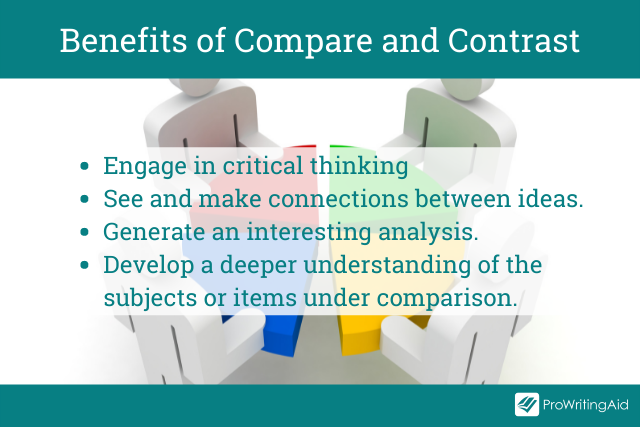
Ultimately, your essay should enlighten readers by providing useful information.
Want to use ProWritingAid with your classroom? Download this free book now:
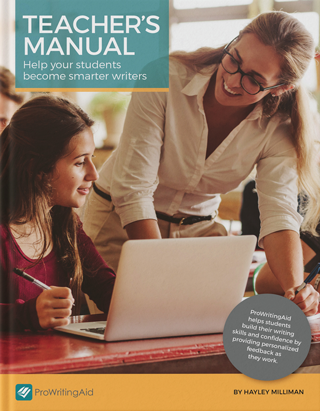
ProWritingAid Teacher’s Manual
Editing technology like prowritingaid provides immediate, personalized feedback that will help students to better understand grammar and writing techniques., in this guide , we walk you through exactly how to use prowritingaid in your classroom and give you tools and templates for creating a rigorous, effective independent writing practice with your students..
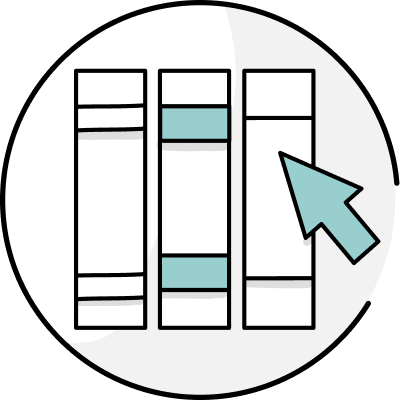
Be confident about grammar
Check every email, essay, or story for grammar mistakes. Fix them before you press send.
Walter Akolo is a freelance writer, internet marketer, trainer, and blogger for hire. He loves helping businesses increase their reach and conversion through excellent and engaging content. He has gotten millions of pageviews on his blog, FreelancerKenya, where he mentors writers. Check out his website walterakolo.com.
Get started with ProWritingAid
Drop us a line or let's stay in touch via :

Sophomore Research: Compare & Contrast
- Researching
- Finding Sources
- Compare & Contrast
- Cause & Effect
- Video Resources
Comparative Analysis
A comparative paper asks that you compare at least two (possibly more) items. These items will differ depending on the assignment. You might be asked to compare:
- positions on an issue (e.g., responses to midwifery in Canada and the United States)
- theories (e.g., capitalism and communism)
- figures (e.g., GDP in the United States and Britain)
- texts (e.g., Shakespeare’s Hamlet and Macbeth)
- events (e.g., the Great Depression and the global financial crisis of 2008–9)
Although the assignment may say “compare,” the assumption is that you will consider both the similarities and differences; in other words, you will compare and contrast .
University of Toronto
Comparing and Contrasting - UNC Writing Center
Comparing and Contrasting Handout - UNC-CH Writing Center
Finding Similarities and Differences
Write down as many ideas as you can about your two topics.
Use a Venn diagram or a Chart when writing down similarities and differences.
Venn Diagrams can be key for a visualization of comparing two or more items. To make a Venn diagram, simply draw a circle for each item you are comparing/contrasting and overlap them. In the central area, list the traits that all items have in common.
Charts are more useful when you have specific criteria in mind. To make a chart, list the names of items across the top of each column then list the criteria in each row. You will then have a box to describe each of the criteria for each item.
Pick out the three best ideas to show the three main ways the two items are similar or different for the focus of your paper.
Questions to Ask
Some Questions to consider when looking for similarities or differences between your two topics.
- Who had something to do with your topic?
- What happened to your topic?
- Where did your topic take place?
- When did your topic happen?
- Why did your topic happen?
- How did your topic happen?
Signal Transitions for Compare/Contrast

- on the contrary
- on the other hand
These expressions signal comparison :
- in common with
Signal words such as these help the reader understand the relationships between your sentences, paragraphs, and ideas. In particular, if you are both comparing and contrasting, signal words help sort out what's what. (Butte College)
Transitions Handout - UNC-CH Writing Center
Point by Point Comparison Outline Format
The most popular form of compare/contrast papers, Point by Point Outlines focus on the topics of similarities or differences and then view each item in comparison. This method can support any number of items to compare as it will be the topics that form the paragraphs.
Point by Point Outline Format
1. Introduction a. Introduce Items (works/objects) b. Thesis Statement i. Topic 1 ii. Topic 2 iii. Topic 3 iv. Etc. 2. Topic 1 a. Summary of Topic i. Relation to Item 1 ii. Relation to Item 2 iii. Etc. 3. Topic 2 a. Summary of Topic i. Relation to Item 1 ii. Relation to Item 2 iii. Etc. 4. Topic 3...4...5...etc. a. Summary of Topic i. Relation to Item 1 ii. Relation to Item 2 iii. Etc. 5. Conclusion a. Restate Thesis b. Summarize how you proved your argument
Example: Point by Point Outline
1. Introduction a. Introduce Items i. First Work – The Lord of the Rings Trilogy had ample preparation time, budget, and a generous release schedule but used practical effects to attain greater realism and focused more on story telling rather than “filmable moments” that added little to the plot. ii. Second Work – The Hobbit Trilogy did not have the preparation time, budget, or generous release schedule in order to be successful films. b. Thesis Statement i. When comparing the Lord of the Rings Trilogy and the Hobbit Trilogy, it is clear that preparation time, budget, special effects, and a well-spaced release schedule can have a tremendous effect on film quality. 2. Preparation Time a. Hobbit Trilogy Examples b. Lord of the Rings Examples 3. Budget a. Hobbit Trilogy Examples b. Lord of the Rings Examples 4. Release Schedule a. Hobbit Trilogy Examples b. Lord of the Rings Examples 5. Practical Effects/CGI a. Hobbit Trilogy Examples b. Lord of the Rings Examples 6. Conclusion a. Restate Thesis b. Summarize how you proved your argument
Texas State Technical College
Subject Comparison Outline Format
This style of paper focuses more on the items themselves rather than focusing on the points. This can lead to a weaker argument as to why one item is superior to another or be nothing more than a list of points with no connection between the two items. Not recommended for comparing/contrasting more than two items.
Subject Outline
1. Introduction a. Introduce Items (works/objects) b. Thesis Statement i. Supporting Point 1 ii. Supporting Point 2 iii. Etc. 2. Explanation of First Item (work/object) a. Information on Supporting Point 1 b. Information on Supporting Point 2 c. Etc. 3. Explanation of Second Item (work/object) a. Information on Supporting Point 1 b. Information on Supporting Point 2 c. Etc. 4. Conclusion a. Restate Thesis b. Summarize how you proved your argument
Example: Subject Outline
1. Introduction a. Introduce Items i. First Work – The Lord of the Rings Trilogy had ample preparation time, budget, and a generous release schedule but used practical effects to attain greater realism and focused more on story telling rather than “filmable moments” that added little to the plot. ii. Second Work – The Hobbit Trilogy did not have the preparation time, budget, or generous release schedule in order to be successful films. b. Thesis Statement i. When comparing the Lord of the Rings Trilogy and the Hobbit Trilogy, it is clear that preparation time, budget, special effects, and a well-spaced release schedule can have a tremendous effect on film quality. 2. Lord of the Rings Trilogy a. Preparation Time b. Budget c. Release Schedule d. Practical Effects/CGI 3. Hobbit Trilogy a. Preparation Time b. Budget c. Release Schedule d. Practical Effects/CGI 4. Conclusion a. Restate Thesis b. Summarize how you proved your argument
Compare Then Contrast Outline Format
Compare Then Contrast : Useful for when your assignment asks you to both compare AND contrast items. Each paragraph immediately focuses on discussions of similarities and weaknesses. While this method works best for short answer or essays, it is possible to take each similarity/difference and turn it into its own paragraph.
Compare Then Contrast Outline
1. Introduction a. Introduce Items (works/objects) b. Thesis Statement i.Topic 1 ii. Topic 2 iii. Topic 3 iv. Etc. 2. Similarities between Works/Objects a. Similarity 1 b. Similarity 2 c. Etc. 3. Differences between Works/Objects a. Difference 1 b. Difference 2 c. Etc. 4. Summary as to why one work/object is better than the other a. Supporting Point 1 b. Supporting Point 2 c. Etc. 5. Conclusion a. Restate Thesis b. Summarize how you proved your argument
Example: Compare Then Contrast Outline
1. Introduction a. Introduce Items i. First Work – The Lord of the Rings Trilogy had ample preparation time, budget, and a generous release schedule but used practical effects to attain greater realism and focused more on story telling rather than “filmable moments” that added little to the plot. ii. Second Work – The Hobbit Trilogy did not have the preparation time, budget, or generous release schedule in order to be successful films. b. Thesis Statement i. When comparing the Lord of the Rings Trilogy and the Hobbit Trilogy, it is clear that preparation time, budget, special effects, and a well-spaced release schedule can have a tremendous effect on film quality. 2. Similarities a. Release Schedule b. Budget 3. Differences a. Preparation Time b. Practical Effects/CGI 4. Conclusion a. Restate Thesis b. Summarize how you proved your argument
- << Previous: Finding Sources
- Next: Cause & Effect >>
- Last Updated: Nov 15, 2022 3:18 PM
- URL: https://ashley.nhcs.libguides.com/sophomoreresearch
Compare/Contrast Essay
Writing for success: compare/contrast, learning objectives.
This section will help you determine the purpose and structure of comparison/contrast in writing.
The Purpose of Compare/Contrast in Writing
Comparison in writing discusses elements that are similar, while contrast in writing discusses elements that are different. A compare-and-contrast essay, then, analyzes two subjects by comparing them, contrasting them, or both.
The key to a good compare-and-contrast essay is to choose two or more subjects that connect in a meaningful way. The purpose of conducting the comparison or contrast is not to state the obvious but rather to illuminate subtle differences or unexpected similarities. For example, if you wanted to focus on contrasting two subjects you would not pick apples and oranges; rather, you might choose to compare and contrast two types of oranges or two types of apples to highlight subtle differences. For example, Red Delicious apples are sweet, while Granny Smiths are tart and acidic. Drawing distinctions between elements in a similar category will increase the audience’s understanding of that category, which is the purpose of the compare-and-contrast essay.
Similarly, to focus on comparison, choose two subjects that seem at first to be unrelated. For a comparison essay, you likely would not choose two apples or two oranges because they share so many of the same properties already. Rather, you might try to compare how apples and oranges are quite similar. The more divergent the two subjects initially seem, the more interesting a comparison essay will be.
The Structure of a Compare/Contrast Essay
The compare-and-contrast essay starts with a thesis that clearly states the two subjects that are to be compared, contrasted, or both and the reason for doing so. The thesis could lean more toward comparing, contrasting, or both. Remember, the point of comparing and contrasting is to provide useful knowledge to the reader. Take the following thesis as an example that leans more toward contrasting:
Thesis Statement: Organic vegetables may cost more than those that are conventionally grown, but when put to the test, they are definitely worth every extra penny.
Here the thesis sets up the two subjects to be compared and contrasted (organic versus conventional vegetables), and it makes a claim about the results that might prove useful to the reader.
You may organize compare-and-contrast essays in one of the following two ways:
- According to the subjects themselves, discussing one then the other
- According to individual points, discussing each subject in relation to each point
The organizational structure you choose depends on the nature of the topic, your purpose, and your audience.
Given that compare-and-contrast essays analyze the relationship between two subjects, it is helpful to have some phrases on hand that will cue the reader to such analysis.
Phrases of Comparison and Contrast
Writing an Compare/Contrast Essay
First choose whether you want to compare seemingly disparate subjects, contrast seemingly similar subjects, or compare and contrast subjects. Once you have decided on a topic, introduce it with an engaging opening paragraph. Your thesis should come at the end of the introduction, and it should establish the subjects you will compare, contrast, or both as well as state what can be learned from doing so.
The body of the essay can be organized in one of two ways: by subject or by individual points. The organizing strategy that you choose will depend on, as always, your audience and your purpose. You may also consider your particular approach to the subjects as well as the nature of the subjects themselves; some subjects might better lend themselves to one structure or the other. Make sure to use comparison and contrast phrases to cue the reader to the ways in which you are analyzing the relationship between the subjects.
After you finish analyzing the subjects, write a conclusion that summarizes the main points of the essay and reinforces your thesis.
Compare/Contrast Essay Example
Comparing and Contrasting London and Washington, DC
By Scott McLean in Writing for Success
Both Washington, DC, and London are capital cities of English-speaking countries, and yet they offer vastly different experiences to their residents and visitors. Comparing and contrasting the two cities based on their history, their culture, and their residents show how different and similar the two are.
Both cities are rich in world and national history, though they developed on very different time lines. London, for example, has a history that dates back over two thousand years. It was part of the Roman Empire and known by the similar name, Londinium. It was not only one of the northernmost points of the Roman Empire but also the epicenter of the British Empire where it held significant global influence from the early sixteenth century on through the early twentieth century. Washington, DC, on the other hand, has only formally existed since the late eighteenth century. Though Native Americans inhabited the land several thousand years earlier, and settlers inhabited the land as early as the sixteenth century, the city did not become the capital of the United States until the 1790s. From that point onward to today, however, Washington, DC, has increasingly maintained significant global influence. Even though both cities have different histories, they have both held, and continue to hold, significant social influence in the economic and cultural global spheres.
Both Washington, DC, and London offer a wide array of museums that harbor many of the world’s most prized treasures. While Washington, DC, has the National Gallery of Art and several other Smithsonian galleries, London’s art scene and galleries have a definite edge in this category. From the Tate Modern to the British National Gallery, London’s art ranks among the world’s best. This difference and advantage has much to do with London and Britain’s historical depth compared to that of the United States. London has a much richer past than Washington, DC, and consequently has a lot more material to pull from when arranging its collections. Both cities have thriving theater districts, but again, London wins this comparison, too, both in quantity and quality of theater choices. With regard to other cultural places like restaurants, pubs, and bars, both cities are very comparable. Both have a wide selection of expensive, elegant restaurants as well as a similar amount of global and national chains. While London may be better known for its pubs and taste in beer, DC offers a different bar-going experience. With clubs and pubs that tend to stay open later than their British counterparts, the DC night life tend to be less reserved overall.
Both cities also share and differ in cultural diversity and cost of living. Both cities share a very expensive cost of living—both in terms of housing and shopping. A downtown one-bedroom apartment in DC can easily cost $1,800 per month, and a similar “flat” in London may double that amount. These high costs create socioeconomic disparity among the residents. Although both cities’ residents are predominantly wealthy, both have a significantly large population of poor and homeless. Perhaps the most significant difference between the resident demographics is the racial makeup. Washington, DC, is a “minority majority” city, which means the majority of its citizens are races other than white. In 2009, according to the US Census, 55 percent of DC residents were classified as “Black or African American” and 35 percent of its residents were classified as “white.” London, by contrast, has very few minorities—in 2006, 70 percent of its population was “white,” while only 10 percent was “black.” The racial demographic differences between the cities is drastic.
Even though Washington, DC, and London are major capital cities of English-speaking countries in the Western world, they have many differences along with their similarities. They have vastly different histories, art cultures, and racial demographics, but they remain similar in their cost of living and socioeconomic disparity.
KEY TAKEAWAYS
- A compare-and-contrast essay analyzes two subjects by either comparing them, contrasting them, or both.
- The purpose of writing a comparison or contrast essay is not to state the obvious but rather to illuminate subtle differences or unexpected similarities between two subjects.
- The thesis should clearly state the subjects that are to be compared, contrasted, or both, and it should state what is to be learned from doing so.
- There are two main organizing strategies for compare-and-contrast essays.
- Organize by the subjects themselves, one then the other.
- Organize by individual points, in which you discuss each subject in relation to each point.
- Use phrases of comparison or phrases of contrast to signal to readers how exactly the two subjects are being analyzed.
- Provided by : Lumen Learning. Located at : http://lumenlearning.com/ . License : CC BY-NC-SA: Attribution-NonCommercial-ShareAlike
- Successful Writing. Provided by : Anonymous. Located at : http://2012books.lardbucket.org/books/successful-writing/s14-07-comparison-and-contrast.html . License : CC BY-NC-SA: Attribution-NonCommercial-ShareAlike
- Comparing and Contrasting London and Washington, DC. Authored by : Scott McLean. Located at : http://2012books.lardbucket.org/books/successful-writing/s14-07-comparison-and-contrast.html . License : CC BY-NC-SA: Attribution-NonCommercial-ShareAlike
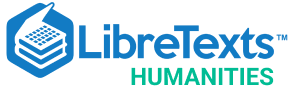
- school Campus Bookshelves
- menu_book Bookshelves
- perm_media Learning Objects
- login Login
- how_to_reg Request Instructor Account
- hub Instructor Commons
- Download Page (PDF)
- Download Full Book (PDF)
- Periodic Table
- Physics Constants
- Scientific Calculator
- Reference & Cite
- Tools expand_more
- Readability
selected template will load here
This action is not available.
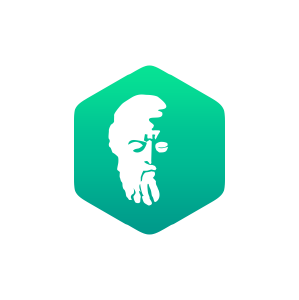
3.9: Comparing and Contrasting Arguments
- Last updated
- Save as PDF
- Page ID 81417
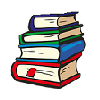
- Saramanda Swigart
- City College of San Francisco
\( \newcommand{\vecs}[1]{\overset { \scriptstyle \rightharpoonup} {\mathbf{#1}} } \)
\( \newcommand{\vecd}[1]{\overset{-\!-\!\rightharpoonup}{\vphantom{a}\smash {#1}}} \)
\( \newcommand{\id}{\mathrm{id}}\) \( \newcommand{\Span}{\mathrm{span}}\)
( \newcommand{\kernel}{\mathrm{null}\,}\) \( \newcommand{\range}{\mathrm{range}\,}\)
\( \newcommand{\RealPart}{\mathrm{Re}}\) \( \newcommand{\ImaginaryPart}{\mathrm{Im}}\)
\( \newcommand{\Argument}{\mathrm{Arg}}\) \( \newcommand{\norm}[1]{\| #1 \|}\)
\( \newcommand{\inner}[2]{\langle #1, #2 \rangle}\)
\( \newcommand{\Span}{\mathrm{span}}\)
\( \newcommand{\id}{\mathrm{id}}\)
\( \newcommand{\kernel}{\mathrm{null}\,}\)
\( \newcommand{\range}{\mathrm{range}\,}\)
\( \newcommand{\RealPart}{\mathrm{Re}}\)
\( \newcommand{\ImaginaryPart}{\mathrm{Im}}\)
\( \newcommand{\Argument}{\mathrm{Arg}}\)
\( \newcommand{\norm}[1]{\| #1 \|}\)
\( \newcommand{\Span}{\mathrm{span}}\) \( \newcommand{\AA}{\unicode[.8,0]{x212B}}\)
\( \newcommand{\vectorA}[1]{\vec{#1}} % arrow\)
\( \newcommand{\vectorAt}[1]{\vec{\text{#1}}} % arrow\)
\( \newcommand{\vectorB}[1]{\overset { \scriptstyle \rightharpoonup} {\mathbf{#1}} } \)
\( \newcommand{\vectorC}[1]{\textbf{#1}} \)
\( \newcommand{\vectorD}[1]{\overrightarrow{#1}} \)
\( \newcommand{\vectorDt}[1]{\overrightarrow{\text{#1}}} \)
\( \newcommand{\vectE}[1]{\overset{-\!-\!\rightharpoonup}{\vphantom{a}\smash{\mathbf {#1}}}} \)
Media Alternative
Listen to an audio version of this page (9 min, 35 sec):
So far we’ve learned how to summarize a single argument, but there are, of course, many arguments on any given topic, and in college and beyond we are often asked to compare and contrast more than one source. In this case we need to provide summaries of two (or more) related but distinct arguments; let’s call them A and B here. We might find common ground between two unlike authors, tease out the subtle differences between two seemingly similar authors, or point out the opposing assumptions underlying competing claims. Ultimately, we'll be asked to go beyond summarizing the two to explore the implications of their similarity and/or difference. What can the comparison teach us? What insight do we gain by juxtaposing A and B?
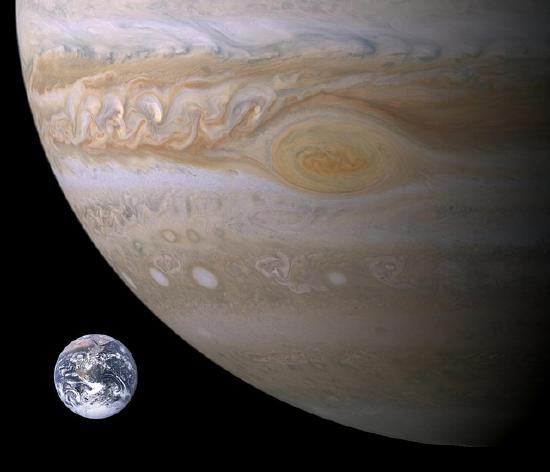
Establishing a topic in common
To frame the compare-and-contrast essay, it helps to describe a common context, something happening in the world, that both texts respond to. What unites these arguments: A theme, a current or historical event, a theoretical lens? Let's say we want to compare and contrast the essay we have already discussed in Chapters 2 and 3, Anna Mills’ “Wouldn’t We All Cross the Border?” with a new argument about borders, “The Weight of the World” by Saramanda Swigart:
"The Weight of the World" by Saramanda Swigart
While illegal immigrants crossing the border to the United States may come from desperate circumstances, it is unjust, impractical, and unrealistic for one nation to solve the problems of so many non-citizens.
Illegal immigration challenges the rule of law. If laws can be broken simply because lawbreakers had good intentions, this suggests that obeying the law is merely optional—that the law is something to be obeyed only when it is convenient to do so. It is understandable that plenty of people who break the law do so with good intentions, but enforcement of the law cannot be reduced to investigations of intentions—it must ultimately spring from concrete actions.
The truth is that illegal immigration presents a security risk. Because illegal immigrants are not tracked by any immigration agency and thus remain largely anonymous, it is impossible to verify which immigrants come in search of a new life and plan to abide by the laws of their host country and which do not. A porous border may allow for waves of well-meaning immigrants and their families to seek new lives in a new country, but no country should be blamed for wanting to secure its borders or its territory.
An influx of immigration also strains a nation's resources. Understandably, in many cases, immigrants seeking shelter in the United States have left desperate circumstances and arrive seeking support. In a perfect world, this would not be a problem; however, because a nation's resources are finite, this means that the financial and material burden of taking care of incoming immigrants falls on their host county. In small, manageable numbers this isn’t a problem (this is what legal immigration is for) but one can see how a nation tasked with taking care of immigrants from around the world would be burdened beyond its resources if it must solve the whole world's humanitarian problems.
Ultimately, we shouldn’t increase our tolerance of illegal border crossings. In order to address the plight of immigrants, maintain national security, and manage internal resources, all policy changes should involve balancing the needs of non-citizens with the needs of citizens before carefully and thoughtfully expanding legal immigration.
In a paper comparing Mills’ and Swigart’s theses, we need to frame the problem central to both arguments; the implications of illegal immigrants crossing the U.S.-Mexico border, a controversial issue of some urgency today. Consider the following sentences, which place both articles in the cultural context within which they are written:
“In recent years illegal immigration into the United States at the U.S.-Mexico border has become a divisive political topic, resulting in a widening partisan divide as to whose priorities we should privilege: the immigrants’ or the nation’s. Are we global citizens or American citizens first?”
Identifying areas of agreement and disagreement
Now, what points do the two articles make? Are there any overlapping claims? Are these two authors in complete disagreement or do you see areas in which they share values and/or concede points to one another? We can start by brainstorming the ways A is similar to B and the ways they differ. As you’ll recall, Mills’ essay appeals to empathy, suggesting that we would become border-crossers ourselves in the right circumstances. She argues for a reevaluation of immigration policies and practices with an increased emphasis on compassion for immigrant families. Swigart’s essay, on the other hand, asks us to make pragmatic assessments about national security and resource allocation, placing national interests before concern for immigrants’ wellbeing. Swigart emphasizes the necessity for a nation to secure its borders and enforce its laws.
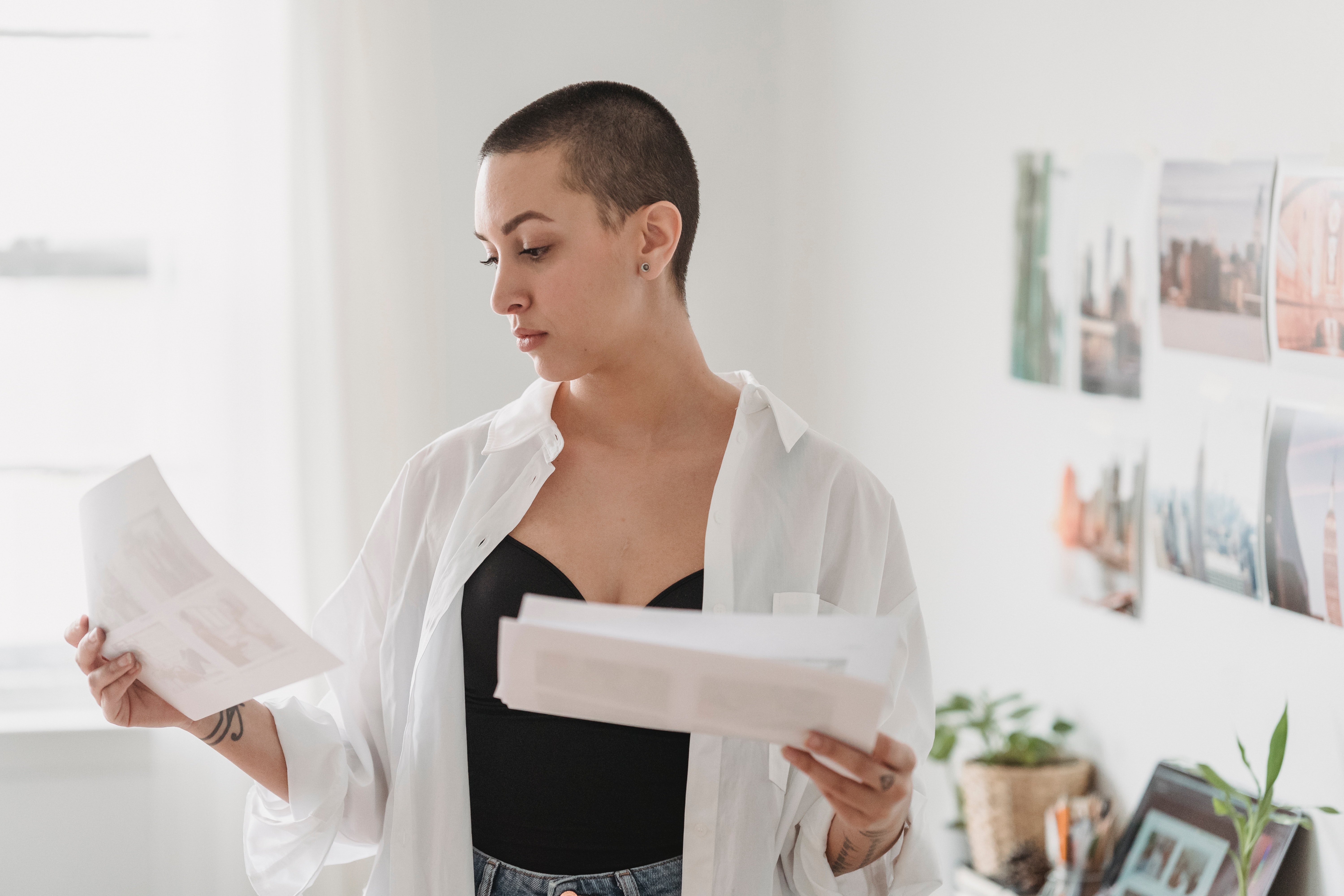
How to organize a compare-and-contrast essay
In the introduction, we will want to identify what topic the two arguments have in common and offer a thesis statement that explains the relationship between A and B. The strategies below may help. In the next section, we will look at a complete sample essay that compares Mills’ and Swigart’s arguments.
Forming the thesis
In the case of compare-and-contrast essays, the thesis can summarize the essential differences or surprising similarities between the texts.
Example \(\PageIndex{1}\)
Thesis : Though Mills and Swigart agree on the urgency as well as the root causes of our border crisis, they disagree on whether the solution should prioritize American citizens' or refugees' needs.
Text-by-text organization
Then we’ll need to select a way to organize the compare and contrast essay. Here are two basic ways to organize the body of a compare and contrast essay: text by text and point by point. If we think that B extends A, or if A is a lens through which to see B, we might decide to use a text-by-text scheme. That means we'll summarize the claims, reasons, and warrants of A followed by the claims, reasons, warrants of B. For instance, if Mills’ essay were outlining the need for immigration reform, and essay B were outlining policy to create such immigration reform, we could quickly summarize Mills’ ideas in a body paragraph before moving on to B’s proposals in their own body paragraph.
Point-by-point organization
If A and B approach a series of similar issues from different standpoints, a point-by-point scheme can highlight their different approaches. That means we’ll break the argument into the different topics that both essays address. In the immigration example, we might include a paragraph about the two “sides” of the debate; a paragraph devoted to whether it is ethical to break the law in desperate circumstances; a paragraph devoted to issues of national security; and a paragraph that compares the proposed solutions.
Topic sentences
In all essays, each new point needs to refer back to some part of the thesis. Each topic sentence should refer to one of the points of comparison that was already mentioned in the thesis. The sample phrases below may be useful as we emphasize particular similarities or differences.
Phrases for compare and contrast essays
Common phrases that indicate similarity and difference can help to clarify how each point about A relates to another point about B. See Section 12.3: Showing How a New Idea Fits in for more on this.
Phrases that highlight a similarity
Just as A does, B believes that______________.
Both A and B see ______________ as an important issue.
We have seen how A maintains that ______________. Similarly, B ______________.
A argues that______________. Likewise, B ______________.
A and B agree on the idea that ______________.
Phrases that highlight a difference
A focuses on______________; however B is more interested in______________.
A’s claim is that______________. Conversely, B maintains that ______________.
Whereas A argues that______________, B______________.
While A emphasizes______________, B______________.
Unlike A, B believes that______________.
Rather than ______________ like A, B______________,
Whereas A argues that ______________, B maintains ______________.
Juxtaposing a similarity with a difference
We can also describe a similarity and a difference in close proximity. Here are some sample sentences that do that:
Both A and B assert that ______________, but they differ in their approach to ______________.
While A condemns the weaknesses of ______________, B praises its strengths.
A outlines the problem of ______________ in the abstract while B proposes solutions to the problem.
Though A and B agree on the root cause of ______________, they differ on its solution.
- PRO Courses Guides New Tech Help Pro Expert Videos About wikiHow Pro Upgrade Sign In
- EDIT Edit this Article
- EXPLORE Tech Help Pro About Us Random Article Quizzes Request a New Article Community Dashboard This Or That Game Popular Categories Arts and Entertainment Artwork Books Movies Computers and Electronics Computers Phone Skills Technology Hacks Health Men's Health Mental Health Women's Health Relationships Dating Love Relationship Issues Hobbies and Crafts Crafts Drawing Games Education & Communication Communication Skills Personal Development Studying Personal Care and Style Fashion Hair Care Personal Hygiene Youth Personal Care School Stuff Dating All Categories Arts and Entertainment Finance and Business Home and Garden Relationship Quizzes Cars & Other Vehicles Food and Entertaining Personal Care and Style Sports and Fitness Computers and Electronics Health Pets and Animals Travel Education & Communication Hobbies and Crafts Philosophy and Religion Work World Family Life Holidays and Traditions Relationships Youth
- Browse Articles
- Learn Something New
- Quizzes Hot
- This Or That Game
- Train Your Brain
- Explore More
- Support wikiHow
- About wikiHow
- Log in / Sign up
- Education and Communications
- College University and Postgraduate
- Academic Writing
How to Write a Compare and Contrast Essay
Last Updated: May 12, 2023 Approved
This article was co-authored by Megan Morgan, PhD . Megan Morgan is a Graduate Program Academic Advisor in the School of Public & International Affairs at the University of Georgia. She earned her PhD in English from the University of Georgia in 2015. wikiHow marks an article as reader-approved once it receives enough positive feedback. This article has 29 testimonials from our readers, earning it our reader-approved status. This article has been viewed 3,102,325 times.
The purpose of a compare and contrast essay is to analyze the differences and/or the similarities of two distinct subjects. A good compare/contrast essay doesn’t only point out how the subjects are similar or different (or even both!). It uses those points to make a meaningful argument about the subjects. While it can be a little intimidating to approach this type of essay at first, with a little work and practice, you can write a great compare-and-contrast essay!
Formulating Your Argument

- You could pick two subjects that are in the same “category” but have differences that are significant in some way. For example, you could choose “homemade pizza vs. frozen grocery store pizza.”
- You could pick two subjects that don’t appear to have anything in common but that have a surprising similarity. For example, you could choose to compare bats and whales. (One is tiny and flies, and the other is huge and swims, but they both use sonar to hunt.)
- You could pick two subjects that might appear to be the same but are actually different. For example, you could choose "The Hunger Games movie vs. the book."

- For example, ask yourself: What can we learn by thinking about “The Hunger Games” and “Battle Royale” together that we would miss out on if we thought about them separately?
- It can be helpful to consider the “So what?” question when deciding whether your subjects have meaningful comparisons and contrasts to be made. If you say “The Hunger Games and Battle Royale are both similar and different,” and your friend asked you “So what?” what would your answer be? In other words, why bother putting these two things together?

- A “Venn diagram” can often be helpful when brainstorming. This set of overlapping circles can help you visualize where your subjects are similar and where they differ. In the outer edges of the circle, you write what is different; in the overlapping middle area, you write what’s similar. [2] X Trustworthy Source University of North Carolina Writing Center UNC's on-campus and online instructional service that provides assistance to students, faculty, and others during the writing process Go to source
- You can also just draw out a list of all of the qualities or characteristics of each subject. Once you’ve done that, start looking through the list for traits that both subjects share. Major points of difference are also good to note.

- For example, if you are comparing and contrasting cats and dogs, you might notice that both are common household pets, fairly easy to adopt, and don’t usually have many special care needs. These are points of comparison (ways they are similar).
- You might also note that cats are usually more independent than dogs, that dogs may not provoke allergies as much as cats do, and that cats don’t get as big as many dogs do. These are points of contrast (ways they are different).
- These points of contrast can often be good places to start thinking about your thesis, or argument. Do these differences make one animal a superior type of pet? Or a better pet choice for a specific living situation (e.g., an apartment, a farm, etc.)?

- Show readers why one subject is more desirable than the other. Example: "Cats are better pets than dogs because they require less maintenance, are more independent, and are more adaptable."
- Help readers make a meaningful comparison between two subjects. Example: "New York City and San Francisco are both great cities for young professionals, but they differ in terms of their job opportunities, social environment, and living conditions."
- Show readers how two subjects are similar and different. Example: "While both The Catcher in the Rye and To Kill a Mockingbird explore the themes of loss of innocence and the deep bond between siblings, To Kill a Mockingbird is more concerned with racism while The Catcher in the Rye focuses on the prejudices of class."
- In middle school and high school, the standard format for essays is often the “5-paragraph form,” with an introduction, 3 body paragraphs, and a conclusion. If your teacher recommends this form, go for it. However, you should be aware that especially in college, teachers and professors tend to want students to break out of this limited mode. Don’t get so locked into having “three main points” that you forget to fully explore your topic.
Organizing Your Essay

- Subject by subject. This organization deals with all of the points about Topic A, then all of the points of Topic B. For example, you could discuss all your points about frozen pizza (in as many paragraphs as necessary), then all your points about homemade pizza. The strength of this form is that you don’t jump back and forth as much between topics, which can help your essay read more smoothly. It can also be helpful if you are using one subject as a “lens” through which to examine the other. The major disadvantage is that the comparisons and contrasts don’t really become evident until much further into the essay, and it can end up reading like a list of “points” rather than a cohesive essay. [4] X Trustworthy Source University of North Carolina Writing Center UNC's on-campus and online instructional service that provides assistance to students, faculty, and others during the writing process Go to source
- Point by point. This type of organization switches back and forth between points. For example, you could first discuss the prices of frozen pizza vs. homemade pizza, then the quality of ingredients, then the convenience factor. The advantage of this form is that it’s very clear what you’re comparing and contrasting. The disadvantage is that you do switch back and forth between topics, so you need to make sure that you use transitions and signposts to lead your reader through your argument.
- Compare then contrast. This organization presents all the comparisons first, then all the contrasts. It’s a pretty common way of organizing an essay, and it can be helpful if you really want to emphasize how your subjects are different. Putting the contrasts last places the emphasis on them. However, it can be more difficult for your readers to immediately see why these two subjects are being contrasted if all the similarities are first.

- Introduction. This paragraph comes first and presents the basic information about the subjects to be compared and contrasted. It should present your thesis and the direction of your essay (i.e., what you will discuss and why your readers should care).
- Body Paragraphs. These are the meat of your essay, where you provide the details and evidence that support your claims. Each different section or body paragraph should tackle a different division of proof. It should provide and analyze evidence in order to connect those proofs to your thesis and support your thesis. Many middle-school and high-school essays may only require three body paragraphs, but use as many as is necessary to fully convey your argument.
- Acknowledgement of Competitive Arguments/Concession. This paragraph acknowledges that other counter-arguments exist, but discusses how those arguments are flawed or do not apply.
- Conclusion. This paragraph summarizes the evidence presented. It will restate the thesis, but usually in a way that offers more information or sophistication than the introduction could. Remember: your audience now has all the information you gave them about why your argument is solid. They don’t need you to just reword your original thesis. Take it to the next level!

- Introduction: state your intent to discuss the differences between camping in the woods or on the beach.
- Body Paragraph 1 (Woods): Climate/Weather
- Body Paragraph 2 (Woods): Types of Activities and Facilities
- Body Paragraph 3 (Beach): Climate/Weather
- Body Paragraph 4 (Beach): Types of Activities and Facilities

- Introduction

- Body Paragraph 1: Similarity between woods and beaches (both are places with a wide variety of things to do)
- Body Paragraph 2: First difference between woods and beaches (they have different climates)
- Body Paragraph 3: Second difference between woods and beaches (there are more easily accessible woods than beaches in most parts of the country)
- Body Paragraph 4: Emphasis on the superiority of the woods to the beach

- Topic sentence: This sentence introduces the main idea and subject of the paragraph. It can also provide a transition from the ideas in the previous paragraph.
- Body: These sentences provide concrete evidence that support the topic sentence and main idea.
- Conclusion: this sentence wraps up the ideas in the paragraph. It may also provide a link to the next paragraph’s ideas.
Putting It All Together

- If you are having trouble finding evidence to support your argument, go back to your original texts and try the brainstorming process again. It could be that your argument is evolving past where it started, which is good! You just need to go back and look for further evidence.

- For example, in a body paragraph about the quality of ingredients in frozen vs. homemade pizza, you could close with an assertion like this: “Because you actively control the quality of the ingredients in pizza you make at home, it can be healthier for you than frozen pizza. It can also let you express your imagination. Pineapple and peanut butter pizza? Go for it! Pickles and parmesan? Do it! Using your own ingredients lets you have fun with your food.” This type of comment helps your reader understand why the ability to choose your own ingredients makes homemade pizza better.

- Reading your essay aloud can also help you find problem spots. Often, when you’re writing you get so used to what you meant to say that you don’t read what you actually said.

- Avoid bias. Don't use overly negative or defamatory language to show why a subject is unfavorable; use solid evidence to prove your points instead.
- Avoid first-person pronouns unless told otherwise. In some cases, your teacher may encourage you to use “I” and “you” in your essay. However, if the assignment or your teacher doesn’t mention it, stick with third-person instead, like “one may see” or “people may enjoy.” This is common practice for formal academic essays.
- Proofread! Spelling and punctuation errors happen to everyone, but not catching them can make you seem lazy. Go over your essay carefully, and ask a friend to help if you’re not confident in your own proofreading skills.
Sample Body Paragraphs

- "When one is deciding whether to go to the beach or the woods, the type of activities that each location offers are an important point to consider. At the beach, one can enjoy the water by swimming, surfing, or even building a sandcastle with a moat that will fill with water. When one is in the woods, one may be able to go fishing or swimming in a nearby lake, or one may not be near water at all. At the beach, one can keep one's kids entertained by burying them in sand or kicking around a soccer ball; if one is in the woods, one can entertain one's kids by showing them different plans or animals. Both the beach and the woods offer a variety of activities for adults and kids alike."

- "The beach has a wonderful climate, many activities, and great facilities for any visitor's everyday use. If a person goes to the beach during the right day or time of year, he or she can enjoy warm, yet refreshing water, a cool breeze, and a relatively hot climate. At the beach, one can go swimming, sunbathe, or build sandcastles. There are also great facilities at the beach, such as a changing room, umbrellas, and conveniently-located restaurants and changing facilities. The climate, activities, and facilities are important points to consider when deciding between the beach and the woods."
Sample Essay Outline

Community Q&A

- Collect your sources. Mark page numbers in books, authors, titles, dates, or other applicable information. This will help you cite your sources later on in the writing process. Thanks Helpful 0 Not Helpful 2
- Don't rush through your writing. If you have a deadline, start early. If you rush, the writing won't not be as good as it could be. Thanks Helpful 0 Not Helpful 0
- Use reputable sources. While Wikipedia may be an easy way to start off, try to go to more specific websites afterwards. Many schools refuse to accept Wikipedia as a valid source of information, and prefer sources with more expertise and credibility. Thanks Helpful 0 Not Helpful 0

- If you have external sources, make sure you always cite them. Otherwise, you may be guilty of plagiarism. Thanks Helpful 3 Not Helpful 0
You Might Also Like

- ↑ http://writingcenter.unc.edu/handouts/comparing-and-contrasting/
- ↑ http://www.readwritethink.org/files/resources/interactives/compcontrast/
About This Article

To write a compare and contrast essay, try organizing your essay so you're comparing and contrasting one aspect of your subjects in each paragraph. Or, if you don't want to jump back and forth between subjects, structure your essay so the first half is about one subject and the second half is about the other. You could also write your essay so the first few paragraphs introduce all of the comparisons and the last few paragraphs introduce all of the contrasts, which can help emphasize your subjects' differences and similarities. To learn how to choose subjects to compare and come up with a thesis statement, keep reading! Did this summary help you? Yes No
- Send fan mail to authors
Reader Success Stories

Huma Bukhari
Feb 16, 2019
Did this article help you?

Alain Vilfort
Mar 2, 2017

Aida Mirzaie
Aug 19, 2018

Michaela Mislerov
Apr 2, 2017

Subhashini Gunasekaran
Jul 31, 2016

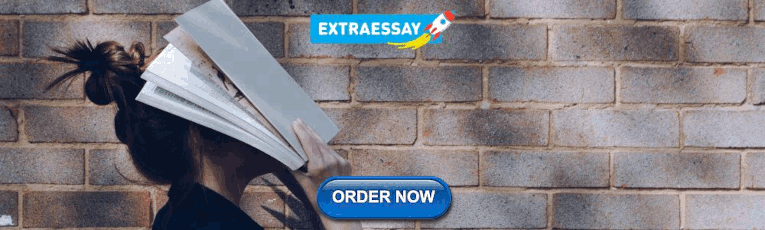
Featured Articles

Trending Articles

Watch Articles

- Terms of Use
- Privacy Policy
- Do Not Sell or Share My Info
- Not Selling Info
Get all the best how-tos!
Sign up for wikiHow's weekly email newsletter
info This is a space for the teal alert bar.
notifications This is a space for the yellow alert bar.

- National University
Ask Us! National University Library
- NCU Office of the Registrar
- NU Library (formerly NCU)
- 2 Academic Success Center
- 44 Business Research
- 1 Database Help
- 39 Database Problems
- 64 Database Questions
- 7 Database Subscriptions
- 5 Dissertation Center Documents
- 67 Dissertation Research
- 13 Ebook Central Questions
- 30 Education Research
- 10 Evaluating Information
- 24 Interlibrary Loan
- 14 Legal Research
- 28 Library Access
- 1 Library Guides
- 2 Library Workshops
- 82 Locate items in the Library
- 42 Locate Items Outside the Library
- 1 Login Help
- 22 Marriage & Family Therapy Research
- 1 Off-Site Access
- 22 OpenAthens
- 16 Organizing Research and Citations
- 1 Psychology and Counseling Research
- 23 Psychology Research
- 1 Publications
- 44 Reference Management
- 33 RefWorks
- 2 Research Consultations
- 126 Research Techniques
- 7 Service Desk
- 3 Technical Assistance
- 19 Technical Issues
- 10 Tests & Measurements
- 10 Textbooks
NU LibChat Widget
Can't find what you need? Submit a ticket through email, and we'll get back to you as soon as possible.
How do I locate articles that compare and contrast a particular topic?
Typically, scholarly articles do not compare and contrast two particular theories, positions, or topics. Rather, they will cover one particular study or perspective in depth. It will be up to you, as a student, to synthesize the information you have learned from your research and draw your own comparisons and conclusions. For additional information, please see our Synthesis FAQ . For assistance on how to synthesize in a paper, visit the Academic Success Center's (ASC) Synthesis and Analysis guide or attend a synthesis and analysis group session .
The following resources also provide additional assistance with writing a comparative analysis:
Comparing and Contrasting (UNC Chapel Hill)
How to Write a Comparative Analysis (Harvard University)
Writing in Literature: Writing the Prompt Paper (Purdue University)
Compare and Contrast Two Competing Psychological Theories
Evaluating and Comparing Two Theories of Cognitive Development
Please note that this information only serves as guidance. Use the Academic Success Center website to learn about coaching and access writing , statistics , editing , and APA Style resources.
For questions about academic coaching services, please contact the Academic Success Center via their Ask a Coach page. On this page, you can search their FAQs, ask a question, or chat live with a coach via ASC Chat.
Still need help?
Didn't get the answer you needed? Contact a librarian through email, phone, SMS, or chat for personalized assistance!

© Copyright 2024 National University. All Rights Reserved.
Privacy Policy | Consumer Information
Thank you for visiting nature.com. You are using a browser version with limited support for CSS. To obtain the best experience, we recommend you use a more up to date browser (or turn off compatibility mode in Internet Explorer). In the meantime, to ensure continued support, we are displaying the site without styles and JavaScript.
- View all journals
- My Account Login
- Explore content
- About the journal
- Publish with us
- Sign up for alerts
- Open access
- Published: 05 April 2023
Systematic comparison of tools used for m 6 A mapping from nanopore direct RNA sequencing
- Zhen-Dong Zhong 1 na1 ,
- Ying-Yuan Xie 1 na1 ,
- Hong-Xuan Chen 1 ,
- Ye-Lin Lan 1 ,
- Xue-Hong Liu 1 ,
- Jing-Yun Ji 1 ,
- Lingmei Jin ORCID: orcid.org/0000-0003-2909-2730 2 ,
- Jiekai Chen ORCID: orcid.org/0000-0001-5168-7074 2 ,
- Daniel W. Mak 3 ,
- Zhang Zhang 1 &
- Guan-Zheng Luo ORCID: orcid.org/0000-0002-6797-9319 1
Nature Communications volume 14 , Article number: 1906 ( 2023 ) Cite this article
10k Accesses
14 Citations
21 Altmetric
Metrics details
- Data processing
- RNA sequencing
N6-methyladenosine (m6A) has been increasingly recognized as a new and important regulator of gene expression. To date, transcriptome-wide m6A detection primarily relies on well-established methods using next-generation sequencing (NGS) platform. However, direct RNA sequencing (DRS) using the Oxford Nanopore Technologies (ONT) platform has recently emerged as a promising alternative method to study m6A. While multiple computational tools are being developed to facilitate the direct detection of nucleotide modifications, little is known about the capabilities and limitations of these tools. Here, we systematically compare ten tools used for mapping m6A from ONT DRS data. We find that most tools present a trade-off between precision and recall, and integrating results from multiple tools greatly improve performance. Using a negative control could improve precision by subtracting certain intrinsic bias. We also observed variation in detection capabilities and quantitative information among motifs, and identified sequencing depth and m6A stoichiometry as potential factors affecting performance. Our study provides insight into the computational tools currently used for mapping m6A based on ONT DRS data and highlights the potential for further improving these tools, which may serve as the basis for future research.
Similar content being viewed by others
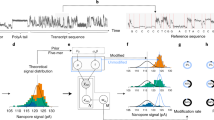
Identification of differential RNA modifications from nanopore direct RNA sequencing with xPore
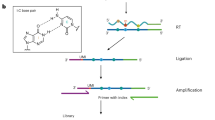
GLORI for absolute quantification of transcriptome-wide m6A at single-base resolution
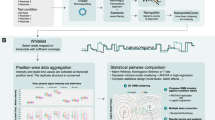
RNA modifications detection by comparative Nanopore direct RNA sequencing
Introduction.
Over 150 types of RNA modifications have been discovered across all domains of life 1 . The most abundant and best-characterized type of modification found in the eukaryotic mRNA is N6-methyladenosine (m6A) 2 , 3 . The discovery of the m6A demethylase fat mass- and obesity-associated protein (FTO) highlights the reversible and dynamic nature of this RNA modification, which may serve as a novel regulator in gene expression 4 . Subsequent studies have revealed that m6A is modulated by a complex network composed of “writer”, “eraser” and “reader” proteins 3 , through which it can co-transcriptionally and post-transcriptionally influence essential RNA metabolic processes, including pre-mRNA splicing and polyadenylation as well as mRNA export, decay and translation 5 . Recent functional studies have demonstrated the essential role of m6A in physiological and pathological processes in various organisms, further advancing our knowledge and understanding of RNA biology 6 , 7 .
Owing to the rapid development of m6A detection methods that have been developed on the next-generation sequencing (NGS) platform, the m6A landscape has since been documented in a number of different organisms 8 . Currently, there are two main NGS-based strategies that are employed in transcriptome-wide m6A mapping: (i) antibody-dependent methods, such as MeRIP-seq and miCLIP 9 , 10 , and (ii) antibody-independent methods, such as m6A-REF-seq/MAZTER-seq 11 , 12 and DART-seq 13 . The most widely used MeRIP-seq protocol employs antibodies to enrich RNA fragments containing m6A; however, this approach suffers from the drawback of high false-positive rates as a result of non-specific antibody binding 14 , 15 , 16 and hence, inaccurate stoichiometric estimates. Meanwhile, m6A-REF-seq/MAZTER-seq utilizes an endonuclease that explicitly cleaves unmethylated ACA motifs but leaves the (m6A)CA intact. This approach precisely identifies m6A sites with single-base resolution and accurately quantifies methylation rates, yet it is limited to a specific sequence context. For example, while the endonuclease MazF specifically recognizes ACA motifs, it only covers approximately 16-25% of m6A sites of the whole transcriptome 11 . In DART-seq, the efficiency and specificity of exogenously expressed APOBEC1-YTH fusion proteins, which are known to induce C-to-U deamination at sites adjacent to m6A methylated sites, have not yet been thoroughly characterized. Most importantly, all of these NGS-based methods are limited to the detection of aggregate RNA molecule information and are incapable in accurately quantifying the stoichiometry at the molecular level. Accordingly, a novel m6A detection method with both high resolution and quantification capability is greatly needed to overcome these limitations.
In the last decade, third-generation sequencing (TGS) technologies represented by two platforms, namely Pacific Biosciences (PacBio) and Oxford Nanopore Technologies (ONT), have emerged as promising alternatives for mapping nucleotide modifications 17 . PacBio sequencing identifies DNA modifications using the interpulse duration (IPD), an approach requiring high sequencing coverage but suffers from low discriminatory power 18 . On the other hand, the ONT sequencing platform directly sequences DNA or RNA based on the principle of monitoring shifts in electric current caused by the traversing of a single molecule across a nanopore embedded within a synthetic polymer membrane. The effect on the flow of the current through the pore by modified bases may be distinct from their unmodified counterpart, thereby allowing for the detection of nucleotide modifications based on the identified electric current differentials 19 , 20 .
A pioneering study published in 2018 reported for the first time the direct sequencing of native RNA molecules using the ONT platform 20 . Since then, at least ten computational tools have been developed to map the location of m6A methylation and to determine its stoichiometry from direct RNA sequencing (DRS) (Supplementary Fig. 1a and Supplementary Table 1 ). These tools can generally be divided into two classes based on the strategies employed to identify modified nucleotides (Fig. 1a ). The first class relies on the detection of electric current differentials. Briefly, a continuous current is subdivided into “events” and then assigned to each nucleotide in a reference sequence using one of the two widely-used algorithms, namely Nanopolish-eventalign 19 or Tombo-resquiggle 21 . For each nucleotide, current features such as the median, mean, standard deviation and dwell-time (i.e., the time a 5-mer sequence resided in the nanopore) are extracted and used as inputs for one of three different classification methods: statistical testing (e.g., used in Tombo 21 ), machine learning (e.g., used in MINES 22 , Nanom6A 23 and m6Anet 24 ) or clustering (e.g., used in Nanocompore 25 and xPore 26 ) (Fig. 1a ). The second class of tools utilizes base-calling “errors” resulting from the change in current caused by the presence of RNA modifications. Base-calling “errors” may represent mismatches, insertions, deletions or variability of base quality scores. These types of information are collected and classified using alignment results. To identify modified bases among the “errors”, Epinano 27 uses a pre-trained machine learning model, while other tools (e.g., DiffErr 28 , DRUMMER 29 and ELIGOS 30 ) perform statistical testing to compare “errors” with an internal model or control sample. Given the high false-positive rate in defining “errors”, a control sample with no (or low levels of) RNA modification is usually necessary.
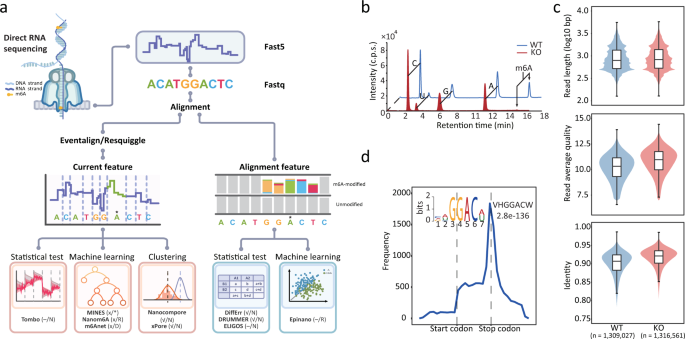
a Pipeline and strategies employed by various m6A mapping tools. Information on the requirement for a negative control, minimum coverage and motif specificity for each tool is shown in brackets. “√” and “x” indicate the requirement for a negative control, while “-” indicates that a negative control is optional. “N” indicates a lack of motif limitations, while “D” and “R” indicate DRACH and RRACH, respectively. Note that MINES detects m6A in AGACT and GGACH motifs. b m6A detection and quantification by LC-MS/MS using WT and Mettl3 KO samples. c Violin plots of ONT data features for WT and KO samples, the upper and lower limits represent the 75th and 25th percentiles, respectively, while the center line represents the median; upper and lower whiskers indicate ±1.5× the interquartile range (IQR). The number of reads is indicated in parentheses. d Metagene distribution plot with motif preferences of m6A peaks identified from the MeRIP-seq data.
As ONT DRS sequencing captures both isoform and nucleotide modification information, it has been successfully applied in dissecting the role of m6A in shaping transcriptome complexity, such as through alternative splicing 29 , 31 , alternative polyadenylation 23 , circular RNA biogenesis 32 and other transcriptional events 33 . Despite a number of highly sophisticated state-of-the-art computational tools that have been developed to detect and quantify m6A, a thorough evaluation and comparison of these tools is currently lacking. To address this gap, we compared ten computational tools used for m6A mapping from ONT DRS data and quantitatively evaluated their performance based on two continuous evaluation metrics (Receiver Operating Characteristic (ROC) and Precision Recall (PR) curves), followed by the identification of m6A under a cutoff that is associated with the best F1 score. We found that most tools presented a trade-off between precision and recall, and their performance largely improved with the integration of the results from multiple tools. We also assessed the intrinsic preference for m6A-irrelevant sites and demonstrated that introduction of a m6A-free negative control improved the performances of most tools. In addition, we found that the detection capability varied among motifs, as current differentials were less easily detected in certain sequence contexts. For the quantitative tools, a wide discrepancy was observed among their stoichiometric estimates, albeit with acceptable results in some of the motifs. We showed that this comprehensive comparison between the multiple tools serves as a guide for best practices in m6A mapping and quantification, as well as conducting of further analysis on the ONT DRS platform.
Datasets used for ONT tool comparisons
In order to compare the computational tools currently available for m6A detection (Fig. 1a , Supplementary Fig. 1a , and Supplementary Table 1 ), we conducted ONT DRS for mRNA from wild-type (WT) mouse embryonic stem cells (mESCs) and corresponding m6A-deficient samples with the major m6A methyltransferase Mettl3 knockout (KO). The mRNA derived from Mettl3 KO samples were confirmed to be virtually absent of m6A by liquid chromatography-mass spectrometry (LC-MS/MS) (Fig. 1b ). We obtained 1.31 and 1.32 million reads for the WT and KO samples, respectively, with similar median read length of 1 kb (Fig. 1c ). The average read quality scores were greater than 10 and the median identities of aligned reads were 90.7% and 92.1% for WT and KO samples, respectively (Fig. 1c ). The average quality and alignment identity of reads from WT samples were slightly lower than that from KO samples, suggesting that the presence of m6A may cause electric current variability, and thus lead to more base-calling “errors” 27 (Fig. 1c ). We also calculated the coverage of aligned reads to annotated transcripts and found that a large proportion of reads were almost full-length in both samples (Supplementary Fig. 1b ). Overall, the quality of the DRS data was sufficient for m6A detection.
To obtain validation datasets, we performed the well-characterized MeRIP-seq assay on WT and Mettl3 KO samples. Peaks identified in WT samples were distributed around stop codons with a consensus sequence RRACH (R = A/G, H = A/T/C), while peaks from KO samples were randomly distributed (Supplementary Fig. 1c ). Given the absence of m6A methylation in the KO samples, these m6A-irrelevant peaks were mainly due to the non-specific antibody binding. After excluding the m6A-irrelevant peaks, a total of 18,069 high-confidence m6A peaks were identified (Fig. 1d ). The ONT DRS platform detected 903,637 and 895,031 RRACH motifs in WT and KO samples, respectively, and more than 40% (370,000) were supported by at least five reads (Supplementary Fig. 1d ). The m6A sites identified in the same cell line by three independent NGS-based methods, i.e., m6A-REF-seq 12 , miCLIP 34 and miCLIP2 35 , were also included as validation sets. To further validate our results, two published DRS datasets derived from WT and Mettl3 KO mESCs samples were included as replicates 30 (Supplementary Table 2 ). In addition, we also included published DRS datasets from WT Arabidopsis (Col-0) and mutants defective of VIRILIZER ( vir-1 ) as validation in distant species 28 (Supplementary Table 2 ).
A comparison between the performance of ten computational tools
In order to assess the aforementioned ten tools’ capability to identify m6A sites, we evaluated their performance based on m6A sites identified using MeRIP-seq, miCLIP and miCLIP2 assays as ground truth (see Methods). In considering the limitations of each NGS-based method, we took the m6A sites identified by each NGS-based method or their intersection and union as validation sets separately. Since some tools are limited to RRACH motifs, we only retained the sites found in this specific sequence context (Supplementary Table 3 ). We then applied each computational tool to predict m6A and ranked the sites according to the confidence scores reported by each tool. As some tools require (DiffErr, DRUMMER, xPore, Nanocompore) or support (ELIGOS_diff, Epinano_delta, Tombo_com) to use negative control sample, while others (ELIGOS, Epinano, Tombo, m6Anet, MINES and Nanom6A) do not, we therefore evaluated their performances in two separate modes which are hereinafter referred to as the compare-mode and single-mode.
We first quantified their performance using two continuous evaluation metrics, the area under the ROC curve (ROC AUC) and PR curve (PR AUC). Among the tools using single-mode, m6Anet outperformed others by achieving a ROC AUC of 0.68-0.94 and PR AUC of 0.20-0.52, followed by ELIGOS, Epinano and Nanom6A, while Tombo achieved the lowest ROC AUC and PR AUC (Fig. 2a, b and Supplementary Fig. 2a ). As MINES trained its models on 12 RRACH motifs but filtered the output m6A sites except that located on four sequences (GGACT, GGACA, GGACC, AGACT), we modified its code to output all sites. Even though MINES achieved the second lowest ROC AUC, the PR AUC was relatively high (Fig. 2a, b and Supplementary Fig. 2a ). Notably, all tools performed much better on four motifs (GGACT, GGACA, GGACC, AGACT), though their ranking remained unchanged (Supplementary Fig. 3 ). In the compare-mode, xPore achieved the best ROC AUC of 0.75-0.91 and PR AUC of 0.27-0.67, which were slightly higher than those of Epinano_delta. Three error-based tools, ELIGOS_diff, DRUMMER and DiffErr, achieved almost the same performance, which may be due to the similar principle they follow (Fig. 2c, d and Supplementary Fig. 2b ). When using different validation sets, the ranking of each tool remained consistent, indicating that the relative performance of the tools is not affected by the choice of validation set (Fig. 2a–d and Supplementary Fig. 2a, b ). In addition, overall ranking was not changed though the ROC and PR AUC of all tools improved by taking the maximum of the predicted probability within each peak from MeRIP-seq results (see Methods) (Supplementary Fig. 2c ).
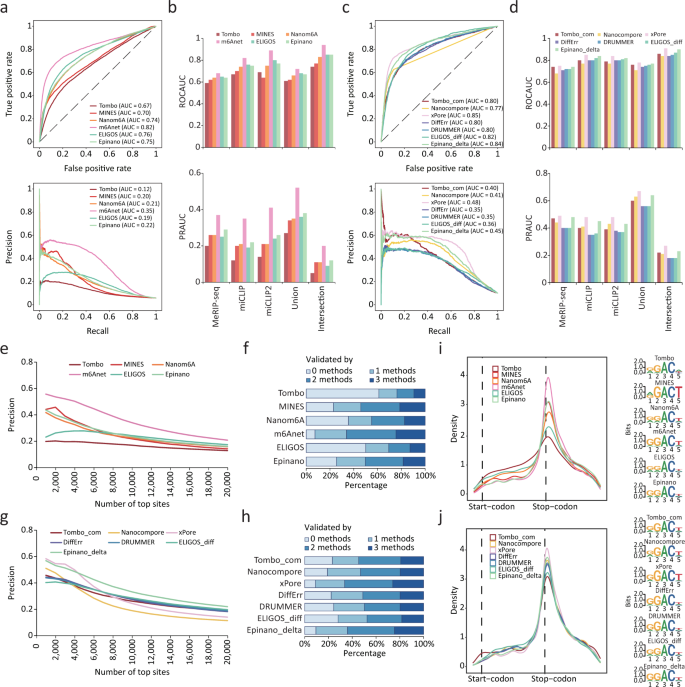
a Receiver operating characteristic (ROC) curve (top) and Precision Recall (PR) curve (bottom) for candidate sites detected by single-mode tools using the miCLIP data as ground truth. Area under the curve (AUC) is indicated in parentheses. b ROC AUC (top) and PR AUC (bottom) for candidate sites detected by single-mode tools using different NGS-based datasets as ground truth. c ROC curve (top) and PR curve (bottom) for candidate sites detected by compare-mode tools using the miCLIP data as ground truth. AUC is indicated in parentheses. d ROC AUC (top) and PR AUC (bottom) for candidate sites detected by compare-mode tools using different NGS-based datasets as ground truth. e Precision curve for top 20,000 m6A sites detected by single-mode tools using the miCLIP data as ground truth. f Percentage for m6A sites supported by various number of NGS-based methods. Top 2000 m6A sites detected by single-mode tools are counted. g Precision curve for top 20,000 m6A sites detected by compare-mode tools using the miCLIP data as ground truth. h Percentage for m6A sites supported by various number of NGS-based methods. Top 2000 m6A sites detected by compare-mode tools are counted. i Metagene plots (left) of the transcriptome-wide m6A distribution for top 2000 m6A sites detected by single-mode tools. The sequence motifs of these sites plotted by Seqlogo are shown on the right. j Metagene plots (left) of the transcriptome-wide m6A distribution for top 2000 m6A sites detected by compare-mode tools. The sequence motifs of these sites plotted by Seqlogo are shown on the right.
We next assessed the precision of the top 20,000 m6A sites predicted by each tool. Among the tools in single-mode, m6Anet constantly showed the highest precision, with 90% of the top 2000 sites or 80% of the top 5000 sites being supported by at least one NGS-based method (Fig. 2e, f and Supplementary Fig. 2d, e ). In the compare-mode, xPore achieved the best precision for top 4000 m6A sites, but its performance decreased sharply with the inclusion of additional sites. In contrast, Epinano_delta constantly showed the best precision for top 4000 to 20,000 m6A sites (Fig. 2g, h and Supplementary Fig. 2f, g ). When analyzing the relative location of top m6A sites, we found that those with higher precision showed greater enrichment around stop codons, a characteristic distribution of m6A (Fig. 2i, j ). This was consistent with previous findings in mammalian species 9 , 10 . The presence of the GGACU consensus sequence was more pronounced in m6A sites identified by tools with higher precision, also supporting previous observations about the distribution of m6A (Fig. 2i, j ).
To further confirm the evaluation of each tool, we repeated the aforementioned process on a published ONT DRS dataset that was derived from WT and Mettl3 KO mESCs samples 30 . We found that all single-mode tools achieved nearly identical performance to their performance using our data; tools in the compare-mode, on the other hand, generally performed worse, though their ranking remained unchanged (Supplementary Fig. 4a–d ). Notably, the published mESCs was found to be an incomplete Mettl3 KO cell line with an estimated ~40% of m6A still present 30 , 36 . This implies that a perfect negative control absent of any m6A would be crucial for these compare-mode tools. Likewise, these tools showed superior m6A detection capability in the aforementioned motifs (GGACT, GGACA, GGACC, AGACT) (Supplementary Fig. 4c, d ).
As some tools were constructed and tested on training data from mammalian species, they may have different performance in non-mammalian species in which the sequence preference and stoichiometry of m6A may vary. To further compare these tools in non-mammalian species, we evaluated their performance using published DRS data from the Arabidopsis 28 . As expected, the performance of machine-learning based tools was compromised when applied to the Arabidopsis dataset, particularly m6Anet and MINES which were trained on DRS data from human samples using miCLIP as the ground truth (Supplementary Fig. 5 ). We reasoned that these two tools are not trained extensively on AAACH motifs, which are the predominantly occurring m6A context in Arabidopsis rather than AGACT and GGACH motifs in human or mouse (Supplementary Table 4 ). On the other hand, most error-based tools performed better in Arabidopsis (Supplementary Fig. 5 ), likely benefiting from a higher coverage (Supplementary Table 3 ). Accordingly, given that the size of Arabidopsis genome is only 1/20 compared to that of the mouse genome, a higher coverage was achieved with similar sequencing reads (Supplementary Table 3 ), leading to more base-calling “errors” occurred in the presence of m6A and increasing the statistical power of error-based tools. However, as more than 10% of m6A were retained in vir-1 samples 28 , the performance of the compare-mode did not significantly improve (Supplementary Fig. 5 ). Similarly, the performance of the tools improved in four motifs (GGACT, GGACA, GGACC, AGACT), particularly for m6Anet and MINES (Supplementary Fig. 6 ). We observed exactly the same results from other replicate dataset from Arabidopsis (Supplementary Fig. 7 ).
In summary, we found that the performance of computational tools for detecting m6A sites varied significantly. Among those that only used a wild-type (WT) sample, m6Anet performed the best. In contrast, tools that used both WT and m6A-deficient (KO) samples exhibited a comparable performance, with the exception of xPore and Nanocompore, which omitted a number of m6A sites to be tested but had higher precision within the top sites. Tombo, ELIGOS and Epinano all had improved performance when using their compare-mode with a nearly m6A-free sample as negative control. In addition, machine-learning based tools may be affected by species-specific differences in m6A distribution and stoichiometry.
Intrinsic bias of ONT tools in m6A detection
To determine the optimal cut-off value for each tool, we calculated the F1 scores under continuous cut-offs and selected the value that corresponded to the summed maximum F1 score when applied to the validation sets from miCLIP and miCLIP2 (Supplementary Fig. 8 and Supplementary Table 5 ). We detected 6410 to 35,475 m6A sites from WT samples and compared them to the validation sets from miCLIP and miCLIP2 (Fig. 3a, b and Supplementary Fig. 9a, b ). Among the single-mode tools, Tombo, Nanom6A, ELIGOS and Epinano detected more m6A sites with higher recall rates, but at the cost of lower precision (Fig. 3a ). While m6Anet and MINES omitted many of the sites to be tested and lost recall rates due to the high coverage requirement or motifs limitation, they achieved the highest F1 score thanks to their high precision (Fig. 3a ). Among the tools in the compare-mode, two tools based on clustering principle achieved the highest precision; however, it was challenging to balance precision and recall rate for other tools, likely due to the failure of clustering (see Methods; Fig. 3b ). DiffErr, DRUMMER and ELIGOS_diff achieved almost the same performances (Fig. 3b ). Similar results were found when using the validation set from miCLIP2 (Supplementary Fig. 9a, b ). We also calculated precision, recall and F1 score with higher coverage requirement. Increasing the coverage from 5 to 20 resulted in a better F1 score for all tools, with most of them achieving a better precision and some achieving a better recall. However, increasing the coverage from 20 to 50 did not result in better performance for most tools (and even slightly worse for some). Notably, the ranking of tools was nearly the same regardless of different coverage requirements (Supplementary Fig. 9 ). The performance of Tombo, ELIGOS and Epinano was better in compare-mode than in single-mode, particularly in terms of precision (Fig. 3a, b ). This suggests that there might be an intrinsic preference for certain sites that is unrelated to m6A and can be avoided when using the compare-mode.
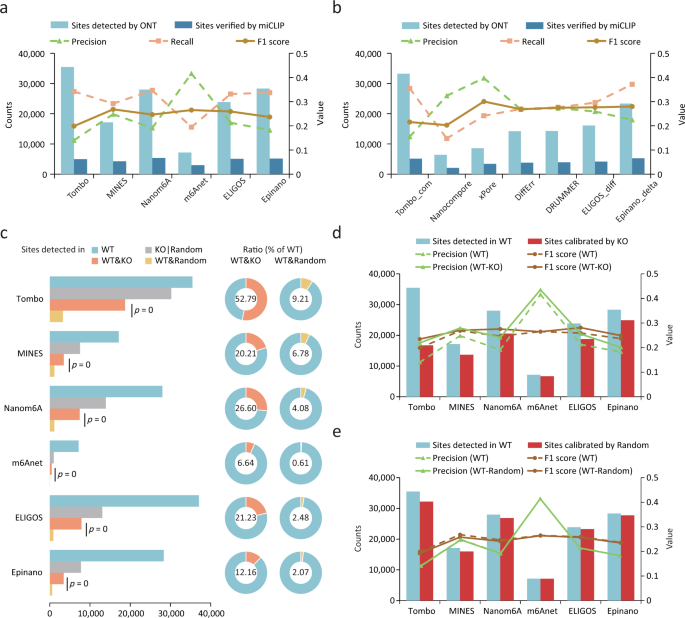
a , b Precision, recall and F1 scores for single-mode tools ( a ) and compare-mode tools ( b ) using miCLIP results as validation set. c Counts and percentages of recurrent m6A sites identified amongst samples. One-sided Hypergeometric tests were applied to calculate significance. WT&KO, both in WT and KO; WT&Random, both in WT and Random; KO|Random, the total number in KO or Random. d , e The m6A site counts and precision and F1 scores before and after calibration with the results from KO ( d ) or random ( e ) samples. miCLIP results were used as a validation set. WT-KO, precision and F1 score for sites calibrated using KO results. WT-random, precision and F1 score for sites calibrated with a randomly selected dataset.
As the Mettl3 KO cell we used were verified to be free of m6A (Fig. 1b ), we expected no (or few) m6A sites would be detected using the single-mode tools. Strikingly, a substantial number of m6A sites (from 983 to 30,207) were identified in the KO sample by most tools, with Tombo even detecting a similar number of m6A sites in KO and WT samples (Fig. 3c ). This suggests a systematic overestimation of m6A abundance by the tools. Sites detected in the KO sample did not show clear enrichment around the stop codons when compared to the background (Supplementary Fig. 9c ), thus implying a prevalence of false positives in the results. We found that the recurrence of m6A sites in WT samples was significantly higher in KO samples than in random datasets (see Methods; Fig. 3c ). For instance, 52.79% and 28.68% of the m6A sites detected in the WT samples were also detected in the KO samples by Tombo and Nanom6A, respectively, while only 9.21% and 4.52% of sites could be found in the random datasets (Fig. 3c ), thus suggesting a potential bias towards certain m6A-irrelevant sites. We inspected these sites that were detected in both WT and KO samples and found that single-nucleotide polymorphisms (SNPs) and insertions/deletions (indels) near RRACH motifs could contribute to this bias, particularly for ELIGOS, which detects m6A by comparing “errors” to the background model and treat SNPs/indels as “errors” during m6A detection (Supplementary Fig. 9d, e ). In addition, homopolymer near the RRACH motif was also one of the causes of the bias (Supplementary Fig. 9d, e ), as they may lead to deletion “errors” signal and inaccurate events assignment. Despite these observations, a proportion of sites detected in both WT and KO samples could not be explained.
We next employed the KO samples as a negative control to test whether it could help to identify false positives and thereby refine the list of reported m6A sites. After subtracting the sites detected in the negative control, we found that the precision of most tools in the single-mode were significantly improved, especially for Tombo and Nanom6A (Fig. 3d and Supplementary Fig. 9h ). Despite decreased recall rates, the F1 scores of these tools were improved (Fig. 3d and Supplementary Fig. 9f, h ). In contrast, calibrating m6A sites using sites in random datasets mentioned above did not improve the precision, but decreased the recall rates (Fig. 3e and Supplementary Fig. 9g, i ). ELIGOS and Epinano performed better when using the compare-mode than directly subtracting the sites in negative control sample (Supplementary Fig. 9j, k ). Overall, the intrinsic bias of each tool may lead to pervasive false positives and using a negative control can improve their performance.
Detection capabilities varied among motifs
Most tools train separate machine learning models or build unique reference models to detect m6A sites with different sequence contexts (or motifs). Notably, m6A sites in AGACT/GGACH motifs tend to have better evaluation metrics (Supplementary Fig. 3 ). We hypothesized that detection capabilities may vary among motifs. We found that the recall rates for certain motifs, such as GGACT, GGACA, GGACC and AGACT, were significantly higher than that of other motifs. On the other hand, some motifs such as AAACC, AAACA and GAACA showed extremely low recall rates (Fig. 4a ). In addition to the highest recall rates observed in the four motifs (GGACT, GGACA, GGACC and AGACT), they also consistently showed the highest precision (Fig. 4b ), suggesting much better detection capabilities of most tools for these motifs. This finding was consistent for both current-based and error-based tools (Supplementary Table 6 ), implying a difficulty in differentiating or detecting m6A sites within some 5-mer motifs. Similar results were observed when using the miCLIP2 result as a validation set (Supplementary Fig. 10a, b and Supplementary Table 6 ).
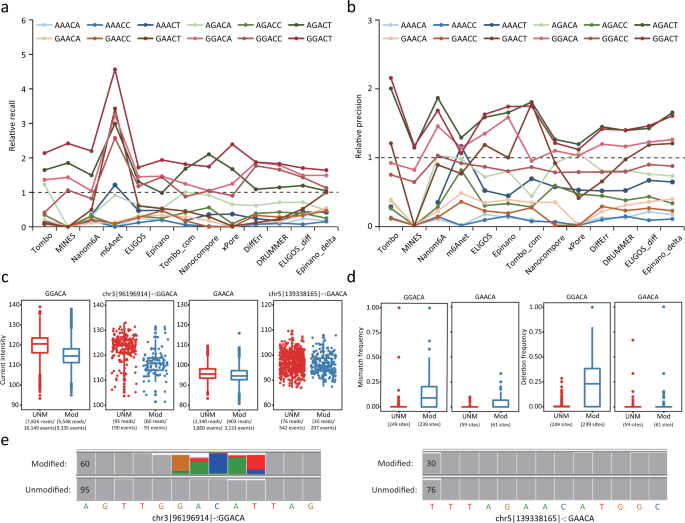
a , b Relative recall rates ( a ) and precision ( b ) for 12 motifs bearing the consensus sequence RRACH. Relative recall rates/precision were calculated as the ratio of the recall/precision for the sites in a specific motif to the overall mean (across all sites). A m6A profile created from miCLIP results was used for validation. c Current intensity of modified and unmodified sites in GGACA or GAACA motifs. The m6A candidate sites (methylation rate of WT–KO > 0.8) were identified using m6A-REF-seq and marked as “Mod” (modified, sites in WT sample) or “UNM” (unmodified, sites in KO sample). This dataset was also used in d , e . For boxplots in c , d , the upper and lower limits represent the 75th and 25th percentiles, respectively, while the center line represents the median; upper and lower whiskers indicate ±1.5× the interquartile range (IQR). The number of reads and events aligned to the sites are indicated in parentheses. d A comparison of mismatch and deletion frequencies for modified and unmodified sites in GGACA and GAACA motifs. The number of sites is indicated in parentheses. e IGV snapshots of two specific sites in different motifs (GGACA and GAACA). Modification status (modified or unmodified) was determined by m6A-REF-seq. Sites with allele frequency ≥0.1 are indicated with corresponding colors. Coverage for each site is indicated on the left of each short sequence.
Given these findings, we suspected that electric current differentials between the modified and unmodified bases may be modest for some motifs, resulting in difficulties of distinguishing m6A from the Adenosine base (A). To test this hypothesis, we inspected 239 GGACA and 59 GAACA sites identified by m6A-REF-seq that were almost fully methylated in WT samples but non-methylated in KO samples (methylation rate of WT - KO > 0.8). Next, we analyzed ONT reads covering these m6A sites (for both WT and KO samples) to examine discrepancies in currents and base-calling “errors” profiles. Notably, current intensity differed greatly between modified and unmodified GGACA motifs but not GAACA motifs (Fig. 4c ). In addition, modified reads with GGACA motif resulted in more base-calling “errors” compared to unmodified reads, especially for deletions and mismatches; in contrast, modified reads with GAACA motif showed little effect on the frequency of base-calling “errors” (Fig. 4d, e and Supplementary Fig. 10c ). Nevertheless, the qualities of modified reads with either GGACA or GAACA motif were significantly lower than that of unmodified reads (Supplementary Fig. 10c ). We concluded that current differentials between m6A and A were relatively small at some sequence contexts, leading to poor performance of most tools encountering such motifs.
Performance of m6A quantification
Among the ten computational tools evaluated, three of the current-based tools (Tombo/Tombo_com, Nanom6A and xPore) are able to quantify methylation rates. Tombo/Tombo_com and Nanom6A detect m6A signal of single reads and thus allow for quantification of m6A at each genomic location covered by multiple reads, while xPore estimates the methylation rate by modeling a mixture of two Gaussian distributions corresponding to unmodified and modified reads. To evaluate the ability of each tool to quantify m6A sites, we compared their results to those obtained from NGS-based methods. We first calculated the enrichment score of each site using MeRIP-seq data, then divided the sites into seven categories according to their enrichment scores. When comparing the methylation rates for sites belonging to different categories, we found that sites with high enrichment scores also showed higher methylation rates (Fig. 5a ). For all three tools, methylation rates were positively correlated with the enrichment score, and xPore showed the highest correlation (Fig. 5a ). To further assess their performance quantitatively, we utilized the validation set from m6A-REF-seq results containing quantitative information for individual m6A sites in four motifs. These three tools showed largely different correlations with the validation set, among which Tombo and xPore achieved the lowest and the highest correlations, respectively (Pearson’ r = 0.21 for Tombo and Pearson’ r = 0.57 for xPore) (Fig. 5b ). Correspondingly, the results of different tools were poorly correlated, with the lowest correlation between Tombo and Nanom6A (Pearson’ r = 0.27), and the highest correlation between Nanom6A and xPore (Pearson’ r = 0.65) (Fig. 5b ). In addition, Tombo_com always showed a higher correlation with other methods than Tombo.
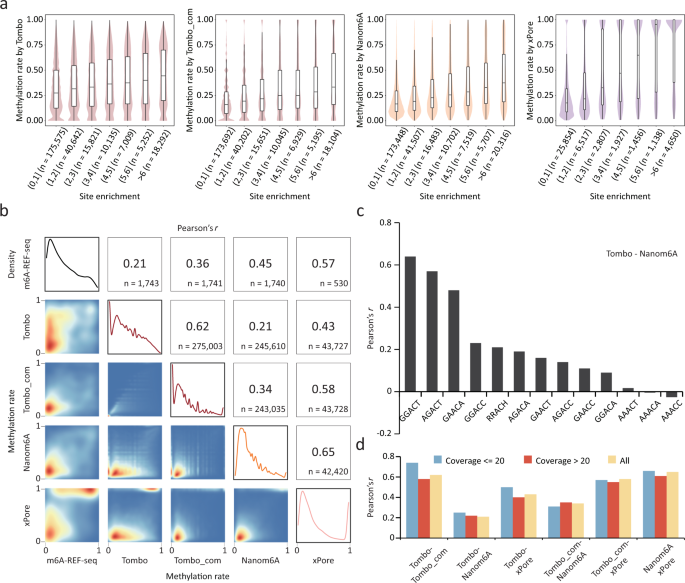
a The distribution of methylation rates determined by each of the three tools. m6A sites are classified into categories according to enrichment scores derived from MeRIP-seq. Violin plots show the distribution of detected methylation rates. In the boxplots, the lower and upper limits represent the 25th and 75th percentiles, respectively; the center line represents the median; and upper and lower whiskers indicate ±1.5×IQR. The number of sites occurring in each peak category are indicated in parentheses. b Correlations of methylation rates derived from m6A-REF-seq and those from each of the three tools. Pearson correlation coefficients and the number of sites for each paired comparison are indicated at the top-right, with 2D-density plots of the methylation rates at the lower-left. The four density plots on the diagonal show the distribution of methylation rates in the m6A-REF-seq results and those of each tool. c Correlation coefficients of methylation rates derived from Tombo and that from Nanom6A. m6A sites in different motifs are separately calculated. d Correlation coefficients of methylation rates derived from paired tools. m6A sites with different sequencing coverage are separately calculated.
Given that previous reports on variable detection capabilities among different motifs, we investigated whether the performance of m6A quantification also varied among different sequence contexts. Despite the low overall consistency among the three tested tools, we found that they consistently showed higher correlations in specific motifs (Fig. 5c and Supplementary Fig. 11a–f ). For instance, when comparing Tombo and Nanom6A, Pearson correlation coefficients for m6A sites within the GGACT and AGACT motifs increased to 0.7, a value significantly higher than that for all sites (0.27) (Fig. 5c ). Similar results were observed for the other five pairs of comparisons (Supplementary Fig. 11a–e ). Furthermore, increasing the coverage did not improve the quantification performance (Fig. 5d and Supplementary Fig. 11g ).
Detection capability is influenced by sequence coverage and m6A stoichiometry
In order to test whether the detection capability is affected by sequencing coverage, we compared the recall and precision across different coverage intervals for each tool. Three error-based tools (ELIGOS/ELIGOS_diff, DRUMMER and DiffErr) and Nanocompore were susceptible to low coverage, with their recall rates increasing dramatically as coverage increased (Fig. 6a ), which explains the superior performance of these tools in Arabidopsis with high sequencing depth (Supplementary Fig. 5 ). xPore and Tombo_com showed slightly lower recall rates at higher coverage interval, while other tools remained stable (Fig. 6a ). In terms of precision, Tombo_com and Epinano_delta benefited most from the high coverage as did the performance of Epinano, Nanom6A and MINES, whereas other tools were less affected by the coverage (Fig. 6b ). These results were consistent when using data from miCLIP2 as a positive control (Supplementary Fig. 12 and Supplementary Table 7 ). In general, the detection capabilities of different tools varied in their response to coverage (Fig. 6c and Supplementary Table 7 ).
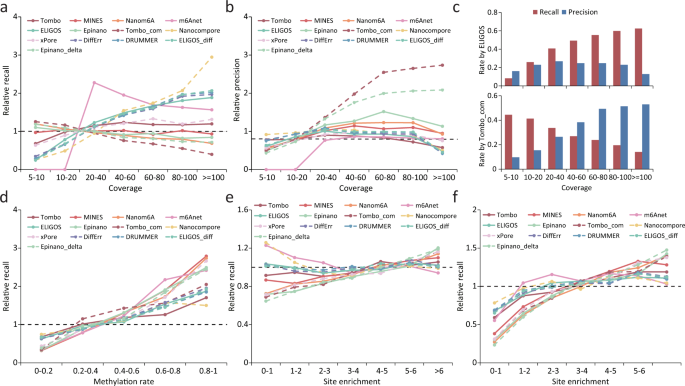
a , b Relative recall rates ( a ) and precision ( b ) for m6A sites under different coverage. Relative recall rates/precision were calculated as the ratio of the recall rate/precision for m6A sites with a given coverage to the overall mean (across all sites). The validation set was derived from the miCLIP results. The same validation set is also used in c . c Recall rates and precision of ELIGOS and Tombo_com for m6A detection under different sequencing coverage. d – f Relative recall rates for m6A sites with different methylation rate/site enrichment. Relative recall rates were calculated as the ratio of the recall rate for m6A sites with a given methylation rate/site enrichment to the overall mean (across all sites). The validation sets were derived from the m6A-REF-seq ( d ), miCLIP ( e ) and miCLIP2 ( f ) results.
As most m6A sites were partially methylated, we wondered whether the algorithms employed by different tools were also effective in detecting m6A sites with low methylation level. To test this, we divided m6A sites that were detected by m6A-REF-seq into five categories according to the methylation rate and compared the recall rate between different categories for each tool. As expected, it is difficult for all the tools to detect sites with low m6A levels (0-0.2); in contrast, the recall rate is largely improved for the sites with high m6A levels (0.8–1) (Fig. 6d and Supplementary Table 8 ). To further investigate all RRACH motifs, we divided the m6A sites detected by miCLIP/miCLIP2 into six categories according to the site enrichment score quantified by MeRIP-seq. Similar results were observed for most tools, with some exception when using data from the miCLIP as positive control (Fig. 6e, f and Supplementary Table 8 ). In conclusion, m6A sites with higher methylation rates were easier to detect than those with lower methylation rates.
Integrated analyses of multiple tools
As presented in the above, it can be difficult to balance precision and recall rates for most individual tools, even under their optimal cut-off settings. For example, Epinano_delta achieved the highest recall rate (0.37) but with a very low precision (0.22), whereas m6Anet achieved the best precision (0.42) at the cost of a low recall rate (0.19) (Fig. 3a, b ). To test whether a combination of multiple tools can improve performance, we intersected the top 5000 m6A sites reported by the different tools. Though the results of ELIGIS_diff, DRUMMER and DiffErr overlapped well with each other (0.6–0.8), wherein the overlap ratio was less than 0.5 for most pairs of other tools (Fig. 7a ). A low overlap ratio may indicate many false-positives as reported by most tools; alternatively, different tools may capture different sets of authentic m6A sites and the integration of these results could improve the prediction performance. After merging the results reported by ten tools, we identified a total of 112,885 m6A sites. However, 54.98% (62,060) of the m6A sites were supported by only one tool, and only 6.66% (7523) of m6A sites were supported by more than half of the tools (Fig. 7b ). Strikingly, only 251 (0.22%) of the m6A sites were identified by all of the tools (Fig. 7b ). We then compared the precision, recall and F1 scores of the integrated predictions to that of the same number of top m6A sites from each individual tool and found that the integrated predictions achieved a better performance in terms of precision, recall rate and F1 score (Fig. 7c ). Moreover, using m6A sites supported by more than 4 or 5 tools achieved the highest F1 score (Fig. 7c ), thus highlighting the effectiveness of integrating the predictions from multiple tools.
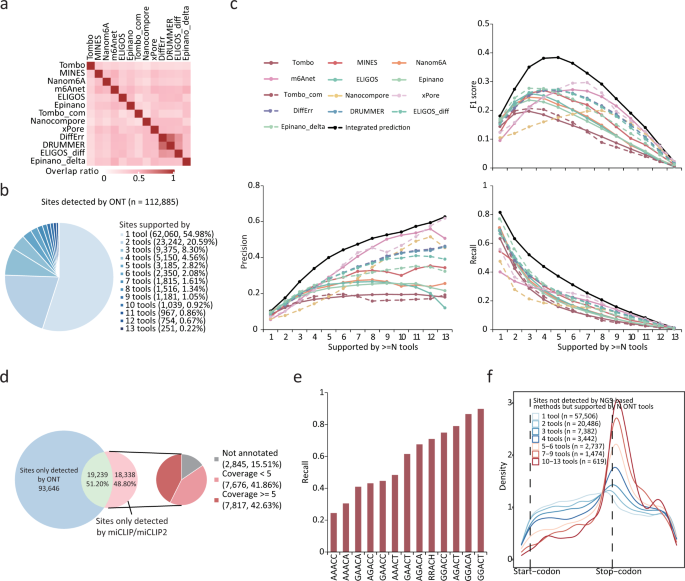
a Recurrent sites ratio among top 5000 m6A sites detected by ONT tools. Heatmap shows the percentage of sites simultaneously detected by each pair of tools. b Percentage of sites identified by multiple tools. c Precision, recall rate and F1 scores of sites supported by more than N tools versus identical number of top sites from individual tool. The validation set was derived from the miCLIP results. d Merged sites detected across all tools and percentage of these sites found in the miCLIP/miCLIP2 results (left). Non-recalled sites were classified according to coverage (right). e Recall rates for m6A sites located in different motifs, using the merged sites detected across all tools after removing intergenic and low-coverage m6A sites. f Metagene plots of the transcriptome-wide distribution of m6A sites that could not be detected by NGS-based methods but supported by one or more ONT tools.
Considering that TGS-based methods or NGS-based methods for m6A profiling may have their own superiorities and limitations, we compared the m6A sites detected by TGS (obtained by merging the m6A sites from ten ONT tools) to those detected by NGS (obtained by merging the m6A sites from miCLIP and miCLIP2). Even though the number of m6A sites from all ONT tools totaled 112,885, only 51.20% (19,239/37,577) of these sites that were detected by the NGS methods could be recalled (Fig. 7d ). We further investigated the m6A sites detected by the NGS methods but were not recalled by any of the ONT tools and found that 15.51% were located in intergenic regions and 40.51% were covered by less than five ONT reads (Fig. 7d ). After removing the intergenic and low-coverage sites, we re-analyzed the non-recall rates for sites with various sequence motifs, and found that m6A sites within the GGACT motifs exhibited a recall rate of more than 0.9 while AAACC and AAACA motifs exhibited recall rates of 0.3 (Fig. 7e ). Since only a small proportion (19,239/112,885) of merged sites were supported by NGS methods, we investigated the characteristics of the remaining sites. These sites showed a clearer enrichment around the stop codons when supported by more ONT tools (Fig. 7f ), while the overlap ratio of the m6A sites that were detected in mESCs KO samples decreased (Supplementary Fig. 13f ). This implies that the NGS method failed to detect some of authentic m6A sites that were captured by the ONT tools.
With the development of NGS-based m6A detection methods, our understanding of the biological role of m6A has advanced considerably. However, such methods have inherent limitations. Recently, ONT DRS has emerged as a promising alternative method of detecting RNA modifications. To date, approximately ten computational tools have been developed. With the goal of comprehensively evaluating the performance of these tools, we conducted a comparative analysis of each tool’s detection capabilities, thereby providing a guide for future studies using ONT DRS. Our analysis revealed a variable performance when evaluated using the ROC and PR curves as continuous metrics, with which m6Anet and Epinano_delta performed the best in the single-mode tools and compare-mode tools, respectively. Moreover, a similar ranking was observed when using different validation datasets or the union and intersection sets. We then applied these tools to the detected m6A sites under a cut-off which correlated to the highest F1 score and found that it was still challenging to balance precision and recall rates for some tools. For example, m6Anet, xPore and Nanocompore achieved the highest precision at the cost of lowest recall, while Tombo and Tombo_com achieved the opposite. Nevertheless, integrated predictions from all tools achieved better performance than individual tools, especially when using m6A sites which were supported by multiple tools (>4). As for quantitation, methylation rates reported by Tombo/Tombo_com, Nanom6A and xPore showed positive correlation to the enrichment score determined by MeRIP-seq, but were poorly agreed across ONT tools or with the methylation rates quantified by m6A-REF-seq. Taken together, there is still room for improvement in the algorithms for the identification and quantification of RNA modifications from ONT DRS datasets.
There are two main challenges that need to be addressed. The first challenge relates to the variance in electric current intensity observed during repeated sequencing of the same 5-mer motif, which is determined by the capability of ONT platform. Given that the presence of m6A corresponds to small differences in current intensity, large amounts of background variation could render A and m6A indistinguishable 37 . The second challenge relates to the data used to train machine learning models 38 . One common method to generate positive samples involves in vitro transcription with dm6ATP instead of dATP; however, the distribution of m6A on this artificial RNA may differ greatly from that found on a natural RNA. In addition, models trained on the DRS data containing organism-specific site information would have an inherent sequence bias. We believe synthetic positive samples with m6A sites located in predetermined locations with various motifs would be more appropriate 39 .
We observed varying degrees of intrinsic bias towards some specific sites in various tools. Benefited from the use of nearly m6A-free negative control, compared-mode tools generally performed better than single-mode tools. Analogously, introducing a negative control (mESC Mettl3 KO samples) as an extra calibrator effectively improved the precision of single-mode tools, although negative control sample with residual m6A confounded the analysis. However, it is relatively complicated to knockout Mettl3 in most cell types 36 , 40 , 41 with some m6A sites having been shown to be Mettl3 -independent 42 , thereby making it virtually impossible to generate an ideal negative control from a natural sample. Recently, we used a transcriptome-wide modification-free RNA library synthesized through in vitro transcription (IVT) to systematically calibrate epitranscriptomic maps profiled by NGS based methods 16 . In the detection of RNA modifications via ONT DRS, this IVT RNA library holds great promise to serve as a gold standard negative control in the future.
Unexpectedly, all tools displayed very divergent recall rates and precision in different motifs. After excluding poorly sequenced reads and intronic or intergenic m6A sites, approximately 70% of the m6A sites detected by miCLIP/miCLIP2 were recalled from the merged m6A sites. High recall rates of up to 90% were seen in GGACT motifs, whereas lower rates of 20% were observed in AAACC motifs. Minor current differentials between modified and unmodified A’s in some motifs may induce false-negative results. To overcome this bottleneck, chemical decorations would assist in making m6A more distinguishable from unmodified A when traversing the nanopore. Similar methods have been developed to detect inosine using the nanopore sequencing 43 .
Here we represent the first comprehensive comparison of computational tools focused on detecting m6A in the ONT DRS dataset. Rational oligo models and well-established BS-seq data have already been applied to the benchmarking of 5mC detection by nanopore sequencing 44 , 45 . However, there is no gold-standard method for m6A detection with both single-base resolution and stoichiometric information on the NGS platform. Therefore, we conducted MeRIP-seq on the same samples used in the ONT sequencing and employed published m6A sets as identified by miCLIP, miCLIP2 and m6A-REF-seq. Though the value of evaluation metrics (ROC AUC, PR AUC, precision, recall, F1 score) given in this study may not reflect the performance of these tools completely and may be underestimated due to the low sequencing depth of DRS data, the comparison between tools would be informative. Moreover, most of our findings still hold when using different validation sets. In addition, we further observed that a proportion of m6A sites which were consistently captured by ONT tools were likely to be authentic, but were not detected by previous NGS-based methods. In conclusion, we provide a comprehensive comparison of computational tools commonly used in m6A mapping depending on the ONT DRS platform. Comparative information on the detection capability, intrinsic bias and motif preferences between the different tools provide valuable insights for the development of new detection strategies and algorithms in future.
Sample collection
The WT and Mettl3 KO mESCs were validated in a previous study 46 . Briefly, exons 5–7 of Mettl3 were replaced with puromycin and hygromycin resistance genes using CRISPR–Cas9 system. After selecting with 1 μg/ml puromycin (Gibco, A1113802) and 200 μg/ml hygromycin B (Sigma, V900372) for one week, diallelic KO colonies were cultured and verified by western blotting (see results in Liu et al. 46 ). After extracting total RNA from the samples using TRIzol (Thermo Fisher Scientific, 15596026), mRNA was isolated via a Dynabeads mRNA Purification Kit (Thermo Fisher Scientific, 61006) with genomic DNA removal using TuRBO DNase kit (Thermo Fisher, AM2238).
Nanopore direct RNA sequencing and data preprocessing
Nanopore direct RNA sequencing was conducted following instructions provided by Oxford Nanopore Technologies (Oxford, UK) using DRS kits (SQK-RNA002) and MinION flowcells (FLO-MIN106D, R9.4.1 pore). After live basecalling using Guppy (v4.0.11) in MinKNOW, reads that passed the quality threshold (7) were then subjected to post-run basecalling with the latest version of Guppy (v5.0.7) under default parameters. A reference transcriptome file was generated from the mouse genome reference and corresponding annotation (GENCODE.VM18) using convert2bed (v2.4.38) and bedtools (v2.26.0). The resulting FASTQ files were aligned to the mouse reference transcriptome using Minimap2 (v2.17) with parameter settings “-ax map-ont --MD”. Samtools (v1.6) was used to filter secondary and supplementary alignments and convert aligned reads to the bam format. Public datasets were preprocessed in the same way except that Arabidopsis samples were aligned to the reference transcriptome generated from TAIR10 reference genome with Araport11 reference annotation.
NGS-based validation sets
The raw MeRIP-seq data was downloaded from Zhang et al. 16 (GSE151028). After quality control, clean reads were aligned to the mouse genome reference file (GRCm38/mm10) with HISAT2 (v2.1.0) for peak calling using MACS2 (v2.1.2) (callpeak --keep-dup all -g mm --nomodel --extsize 200 -q 0.0001 --fe-cut-off 2). High confidence peaks were obtained by first merging peaks from two WT replicates and then subtracting peaks identified in both KO replicates. Only peaks containing RRACH motif were retained since most ONT tools were limited to this motif. Sites detected by ONT tools would be considered as true positives when they were found to be located in the peaks from MeRIP-seq. When using public miCLIP 34 , miCLIP2 35 and m6A-REF-seq 12 datasets, m6A sites only in the RRACH motifs were kept. m6A profiles derived from miCLIP and miCLIP2 results were two main validation sets for all analysis. MeRIP-seq results were also used to compare tools with continuous evaluation metrics. When using MeRIP-seq data as evaluation set, we roughly considered all sites falling in the peaks as true positives. m6A-REF-seq results provided reference modification rate in the quantification part. As for the validation sets for Arabidopsis , we also filtered sites not containing RRACH motifs from the public MeRIP-seq 47 and miCLIP 28 datasets.
m6A detection with multiple tools
We ran the tools with parameter settings described as follows, and the detailed command and code are available at https://github.com/zhongzhd/ont_m6a_detection 48 .
Tombo (v1.5.1) needs to re-squiggle reads to assign raw current signals to each base before further processing can be carried out. Tombo now provides two methods for m6A detection: (1) a de novo non-canonical base method with the command “detect_modifications de_novo” (represented by Tombo); and (2) a canonical sample comparison method with the command “detect_modifications model_sample_compare” (represented by Tombo_com). The first approach was used for routine analysis of single samples, while the second approach was used to combine analyses for negative control samples. Several statistics were obtained from Tombo, including the coverage and estimated fraction-modified values, and the latter were treated as scores for ranking.
MINES uses coverage and fraction-modified values calculated by Tombo as input to its random forest models (only for AGACT and GGACH motifs) and only reports significant sites. Therefore, we modified the code to output the modification probability of all sites in all RRACH motifs. The modification probabilities of sites were treated as scores for ranking. The modified version is available at https://github.com/zhongzhd/ont_m6a_detection 48 .
Nanom6A (v2.0) extracts the median, mean, standard deviation and dwell time from normalized raw signals for each read after re-squiggling with Tombo. The above features are then used as input for its XGBoost model which assigns a modification probability for each read. Reads with a modification probability >0.5 were considered modified, and the modified fraction of sites were treated as scores for ranking.
m6Anet (v1.0) requires the results generated from running the eventalign module in Nanopolish (0.13.2), which assigns raw current signal to each base (like Tombo). The results are used as input to the m6Anet’s neural-network based Multiple Instance Learning model which produces probability-modified values per site. The probability-modified values corresponding to each site were treated as scores for ranking.
Nanocompore (v1.0.0) collapses the results from the Nanopolish eventalign module to generate a file containing the median intensity and dwell time; it also makes pairwise comparisons to the collapsed results from a control sample. The Gaussian mixture model (GMM) logit p values from statistical test results of sites were treated as scores for ranking. Note that this tool outputs many sites with “nan” value due to failure in clustering, so sites with the “nan” values were assigned a p value of 1.
xPore (v2.0) also uses the results from the Nanopolish eventalign module for all samples to make a configuration file, and applies the multi-sample two-Gaussian mixture model to obtain the estimated modification rate for each sample, a test statistic ( z score) and p value on differential modification rates for each pairwise condition. The z scores from statistical test results were treated as scores for ranking (as recommended by the authors). Similar to Nanocompore, this tool omitted many sites in its result due to failure in clustering, so we assigned a z score of 0 to these sites. Sites with modified distributions of current assigned in the opposite direction, were assigned a z score of 0 according to xPore’s methods. In addition, sites with higher methylation rate in KO than WT were also assigned a z score of 0.
DiffErr (v0.2) uses alignment files with a control as input. The −log10 p values from statistical test results of sites were treated as scores for ranking, and sites filtered internally or with odds ratio (log) ≤0 were assigned a −log10 p value of 0.
DRUMMER also uses alignment files for the sample and a control as input. The p values (odds ratio) from statistical test results of sites were treated as scores for ranking, and sites filtered internally or with odds ratio ≤1 were assigned a p value of 1.
ELIGOS (v2.0.1) offers two methods for the detection of modified nucleotides: (1) the identification of RNA modifications compared to a rBEM5 + 2 model using “rna_mod” (represented by ELIGOS); and (2) the identification of RNA modifications compared to control conditions using “pair_diff_mod” (represented by ELIGOS_diff). The first method was used for routine analyses of single samples, while the second was implemented for combined analyses including negative control samples. The p values (odds ratio) from statistical test results of sites were treated as scores for ranking, and sites filtered internally or with odds ratio ≤1 were assigned a p value of 1.
Epinano (v1.2.0) offers two kinds of Support Vector Machine models for m6A detection. These models have different features: (1) the mean quality, and mismatch, insertion and deletion frequency of each base (represented by Epinano); and (2) the difference in mean quality, and in the mismatch, insertion and deletion frequency between the sample and a control for each base (represented by Epinano_delta). The first method was used by default and the second when comparing to a negative control. The probability-modified from predicted results were treated as scores for ranking.
Tools comparison using ROC and PR curves
Sites in RRACH motif with more than 5 reads (except for m6Anet that required at least 20 reads) were tested by each tool and ranked according to the significant score as described above. We then converted the transcriptome coordinates to the genomic coordinates to match with the validation sets. Sites derived from multiple transcriptomic coordinates but aligned to the same genomic coordinate were merged as follows: for Tombo/Tombo_com, MINES, Nanom6A, m6Anet and Epinano/Epinano_delta, the scores (fraction-modified/probability-modified) were weighted averaging according coverage; for Nanocompore, xPore, DiffErr, DRUMMER and ELIGOS, the best scores ( p value/ z score) were selected as representative. To objectively compare the tools, we only kept the sites that were covered by all methods and with a coverage ≥20 in the final dataset for evaluation using the ROC and PR curve. MeRIP-seq, miCLIP and miCLIP2 results mentioned above were applied as ground truth, and we also used the validation sets in the form of union and intersection of all the NGS-based methods. As for Arabidopsis , MeRIPseq and miCLIP results and their union and intersection were used as the validation sets.
m6A determination with optimal cut-off
To determine the optimal cut-off for m6A detection, we varied the cut-offs and calculated F1 scores of sites in the RRACH motif covered by at least 5 reads using the MeRIP-seq, miCLIP and miCLIP2 results as validation sets separately (note that m6Anet requires at least 20 reads and MINES only output results in 4 motifs).
As the distribution of F1 scores were inconsistent between the validation set from MeRIP-seq and miCLIP/miCLIP2, we selected the cut-off corresponded to the maximum F1 score when adding up that of miCLIP and miCLIP2 for following m6A detection process. Validation sets of miCLIP and miCLIP2 were used for the following precision and recall calculation. Note that in evaluating the performance of these m6A detection tools, we only considered sites detected by NGS methods (MeRIP-seq, miCLIP and miCLIP2) that were covered by minimal DRS reads (5, 20, 50 DRS reads requirement). When setting the requirement at 5 DRS reads, we also took into consideration sites omitted by m6Anet (<20 reads) and MINES (not in four specific motif) and treated them with the lowest score for recall calculation. m6A sites detected under 5 DRS reads requirement were used for further analysis.
Intrinsic bias assessment
Sites in the RRACH motif with minimum 5 reads (the pool) in Mettl3 KO samples were tested by each tool and determined as m6A using the optimal cut-off described above. We then randomly sampled an identical number of sites (== the number of m6A sites detected in KO samples) from the pool as the random dataset for each tool. To test whether these tools prefer some irrelevant m6A sites (intrinsic bias), we compared two intersection “WT&KO” (m6A sites detected in WT and KO samples) and “WT&Random” (m6A sites detected in WT samples and existed in random dataset). If there were certain intrinsic bias, the WT&KO intersection should be greater than WT&Random intersection; we applied one-side Hypergeometric tests to calculate the significance. To determine for the cause of the intrinsic bias, the input data of MeRIP-seq were used to call SNPs/indels compared to mm10 reference using bcftools with the key parameter “QUAL > 100”, and SNPs/indels were kept when supported by more than 2 of 4 samples (WT and KO with replicates). In addition, the flanking sequence (upstream and downstream 10 bp) of all candidate sites were extracted and inspected for homopolymer. We then compared the ratio of sites near SNPs/indels (within 5 bp) or homopolymer between the “WT&KO” intersection and sites in the RRACH motif with more than 5 reads (background) and applied one-sided Binomial tests to calculate significance.
Comparisons of current intensities and base-calling “errors” between modified and unmodified 5-mer
Nanopolish eventalign was used to assign raw current to each base in the reference genome. The results of sam2tsv pileup (v0558422) were used to calculate the frequency of mismatch, deletion and insertion and base quality. Highly-methylated m6A sites in GGACA and GAACA motifs with a methylation rate differences >0.8 between WT and KO samples as determined by m6A-REF-seq, were selected. The current intensities and base-calling “errors” of these sites extracted from WT samples were treated as current intensities and base-calling “errors” of modified 5-mer, while these extracted from KO samples were treated as current intensities and base-calling “errors” of unmodified 5-mer. We compared the current intensities and base-calling “errors” between modified and unmodified 5-mer for both GGACA and GAACA motifs.
m6A quantification
Tombo/Tombo_com and Nanom6A detect m6A at read level and support methylation rates calculation. Sites in the RRACH motif with more than 5 reads were calculated. xPore outputs methylation rates directly in its result and tests the difference of methylation rates between WT and KO samples, so we used the methylation rates of WT samples for all sites in the RRACH motif with more than 5 reads except for sites which failed to cluster. Methylation rates from the m6A-REF-seq results were used for comparison. We also calculated the enrichment score for these sites using the MeRIP-seq data (IP FPKM/Input FPKM) with featureCounts (v2.0.1) for comparison.
Reporting summary
Further information on research design is available in the Nature Portfolio Reporting Summary linked to this article.
Data availability
All data generated for this paper have been deposited in the NCBI Gene Expression Omnibus (GEO) database under accession number GSE195618 . Published DRS datasets derived from WT and Mettl3 KO mESC samples are obtained from the work of Jenjaroenpun et al. 30 and are available through NCBI Sequence Read Archive (SRA) database under accession number SRP166020 . Published Arabidopsis DRS datasets along with their KO variants are form Parker et al. 28 and are available through European Nucleotide Archive (ENA) database under accession number PRJEB32782 .
Code availability
The analysis pipeline and all custom scripts used for this paper are available at https://github.com/zhongzhd/ont_m6a_detection 48 .
Boccaletto, P. et al. MODOMICS: a database of RNA modification pathways. 2021 update. Nucleic Acids Res. 50 , D231–D235 (2022).
Article CAS PubMed Google Scholar
Roundtree, I. A., Evans, M. E., Pan, T. & He, C. Dynamic RNA modifications in gene expression regulation. Cell 169 , 1187–1200 (2017).
Article CAS PubMed PubMed Central Google Scholar
Zaccara, S., Ries, R. J. & Jaffrey, S. R. Reading, writing and erasing mRNA methylation. Nat Rev Mol Cell Biol. 20 , 608–624 (2019).
Jia, G. et al. N6-methyladenosine in nuclear RNA is a major substrate of the obesity-associated FTO. Nat. Chem. Biol. 7 , 885–887 (2011).
Yang, Y., Hsu, P. J., Chen, Y. S. & Yang, Y. G. Dynamic transcriptomic m(6)A decoration: writers, erasers, readers and functions in RNA metabolism. Cell Res. 28 , 616–624 (2018).
Yu, Q. et al. RNA demethylation increases the yield and biomass of rice and potato plants in field trials. Nat. Biotechnol. 39 , 1581–1588 (2021).
Jiang, X. et al. The role of m6A modification in the biological functions and diseases. Signal Transduct. Target. Ther. 6 , 74 (2021).
Tang, Y. et al. m6A-Atlas: a comprehensive knowledgebase for unraveling the N6-methyladenosine (m6A) epitranscriptome. Nucleic Acids Res. 49 , D134–D143 (2021).
Meyer, K. D. et al. Comprehensive analysis of mRNA methylation reveals enrichment in 3′ UTRs and near stop codons. Cell 149 , 1635–1646 (2012).
Dominissini, D. et al. Topology of the human and mouse m6A RNA methylomes revealed by m6A-seq. Nature 485 , 201–206 (2012).
Article ADS CAS PubMed Google Scholar
Garcia-Campos, M. A. et al. Deciphering the “m(6)A Code” via antibody-independent quantitative profiling. Cell. 178 , 731–747 (2019).
Zhang, Z. et al. Single-base mapping of m(6)A by an antibody-independent method. Sci. Adv. 5 , x250 (2019).
Article ADS Google Scholar
Meyer, K. D. DART-seq: an antibody-free method for global m(6)A detection. Nat. Methods 16 , 1275–1280 (2019).
Helm, M., Lyko, F. & Motorin, Y. Limited antibody specificity compromises epitranscriptomic analyses. Nat. Commun. 10 , 5669 (2019).
Article ADS PubMed PubMed Central Google Scholar
McIntyre, A. et al. Limits in the detection of m(6)A changes using MeRIP/m(6)A-seq. Sci. Rep. 10 , 6590 (2020).
Article ADS CAS PubMed PubMed Central Google Scholar
Zhang, Z. et al. Systematic calibration of epitranscriptomic maps using a synthetic modification-free RNA library. Nat. Methods 18 , 1213–1222 (2021).
Amarasinghe, S. L. et al. Opportunities and challenges in long-read sequencing data analysis. Genome Biol. 21 , 30 (2020).
Article PubMed PubMed Central Google Scholar
Flusberg, B. A. et al. Direct detection of DNA methylation during single-molecule, real-time sequencing. Nat. Methods 7 , 461–465 (2010).
Simpson, J. T. et al. Detecting DNA cytosine methylation using nanopore sequencing. Nat. Methods 14 , 407–410 (2017).
Garalde, D. R. et al. Highly parallel direct RNA sequencing on an array of nanopores. Nat. Methods 15 , 201–206 (2018).
Stoiber, M. et al. De novo identification of DNA modifications enabled by genome-guided nanopore signal processing. Preprint at bioRxiv https://doi.org/10.1101/094672, (2017).
Lorenz, D. A., Sathe, S., Einstein, J. M. & Yeo, G. W. Direct RNA sequencing enables m(6)A detection in endogenous transcript isoforms at base-specific resolution. RNA 26 , 19–28 (2020).
Gao, Y. et al. Quantitative profiling of N(6)-methyladenosine at single-base resolution in stem-differentiating xylem of Populus trichocarpa using Nanopore direct RNA sequencing. Genome Biol. 22 , 22 (2021).
Hendra, C. et al. Detection of m6A from direct RNA sequencing using a multiple instance learning framework. Nat. Methods 19 , 1590–1598 (2022).
Leger, A. et al. RNA modifications detection by comparative Nanopore direct RNA sequencing. Nat. Commun. 12 , 7198 (2021).
Pratanwanich, P. N. et al. Identification of differential RNA modifications from nanopore direct RNA sequencing with xPore. Nat. Biotechnol. 39 , 1394–1402 (2021).
Liu, H. et al. Accurate detection of m(6)A RNA modifications in native RNA sequences. Nat. Commun. 10 , 4079 (2019).
Parker, M. T. et al. Nanopore direct RNA sequencing maps the complexity of Arabidopsis mRNA processing and m(6)A modification. ELife 9 , e49658 (2020).
Price, A. M. et al. Direct RNA sequencing reveals m(6)A modifications on adenovirus RNA are necessary for efficient splicing. Nat. Commun. 11 , 6016 (2020).
Jenjaroenpun, P. et al. Decoding the epitranscriptional landscape from native RNA sequences. Nucleic Acids Res. 49 , e7 (2021).
Watabe, E. et al. m(6) A-mediated alternative splicing coupled with nonsense-mediated mRNA decay regulates SAM synthetase homeostasis. EMBO J. 40 , e106434 (2021).
Wang, Y. et al. Profiling of circular RNA N6‐methyladenosine in moso bamboo (Phyllostachys edulis) using nanopore‐based direct RNA sequencing. J. Integr. Plant Biol. 62 , 1823–1838 (2020).
Wang, Y., Zhao, Y., Bollas, A., Wang, Y. & Au, K. F. Nanopore sequencing technology, bioinformatics and applications. Nat. Biotechnol. 39 , 1348–1365 (2021).
Ke, S. et al. m(6)A mRNA modifications are deposited in nascent pre-mRNA and are not required for splicing but do specify cytoplasmic turnover. Genes Dev. 31 , 990–1006 (2017).
Kortel, N. et al. Deep and accurate detection of m6A RNA modifications using miCLIP2 and m6Aboost machine learning. Nucleic Acids Res. 49 , e92 (2021).
Batista, P. J. et al. m(6)A RNA modification controls cell fate transition in mammalian embryonic stem cells. Cell Stem Cell. 15 , 707–719 (2014).
Begik, O. et al. Quantitative profiling of pseudouridylation dynamics in native RNAs with nanopore sequencing. Nat. Biotechnol. 39 , 1278–1291 (2021).
Alfonzo, J. D. et al. A call for direct sequencing of full-length RNAs to identify all modifications. Nat. Genet. 53 , 1113–1116 (2021).
Workman, R. E. et al. Nanopore native RNA sequencing of a human poly(A) transcriptome. Nat. Methods 16 , 1297–1305 (2019).
Poh, H. X., Mirza, A. H., Pickering, B. F. & Jaffrey, S. R. Alternative splicing of METTL3 explains apparently METTL3-independent m6A modifications in mRNA. PLoS Biol. 20 , e3001683 (2022).
Lin, Z. et al. Mettl3-/Mettl14-mediated mRNA N(6)-methyladenosine modulates murine spermatogenesis. Cell Res. 27 , 1216–1230 (2017).
Satterwhite, E. R. & Mansfield, K. D. RNA methyltransferase METTL16: targets and function. Wiley Interdiscip. Rev. RNA 13 , e1681 (2021).
PubMed PubMed Central Google Scholar
Ramasamy, S. et al. Chemical probe-based nanopore sequencing to selectively assess the RNA modifications. ACS Chem. Biol. 17 , 2704–2709 (2022).
Liu, Y. et al. DNA methylation-calling tools for Oxford Nanopore sequencing: a survey and human epigenome-wide evaluation. Genome Biol. 22 , 295 (2021).
Yuen, Z. W. et al. Systematic benchmarking of tools for CpG methylation detection from nanopore sequencing. Nat. Commun. 12 , 3438 (2021).
Liu, J. et al. The RNA m(6)A reader YTHDC1 silences retrotransposons and guards ES cell identity. Nature 591 , 322–326 (2021).
Shen, L. et al. N(6)-methyladenosine RNA modification regulates shoot stem cell fate in Arabidopsis. Dev. Cell 38 , 186–200 (2016).
Zhong, Z. et al. Systematic comparison of tools used for m6A mapping from nanopore direct RNA sequencing. https://github.com/zhongzhd/ont_m6a_detection , https://doi.org/10.5281/zenodo.7651738 (2023).
Download references
Acknowledgements
This work was supported by the Ministry of Science and Technology of China (National Science and Technology Major Project, 2018YFA0109100, 2019YFA0802203, 2022YFA0912900, and 2022YFC3400400), National Natural Science Foundation of China (92253202, 32271499, 32270644, and 32100461), and Shenzhen Bay Scholars Program.
Author information
These authors contributed equally: Zhen-Dong Zhong, Ying-Yuan Xie.
Authors and Affiliations
MOE Key Laboratory of Gene Function and Regulation, Guangdong Province Key Laboratory of Pharmaceutical Functional Genes, State Key Laboratory of Biocontrol, School of Life Sciences, Sun Yat-sen University, 510275, Guangzhou, China
Zhen-Dong Zhong, Ying-Yuan Xie, Hong-Xuan Chen, Ye-Lin Lan, Xue-Hong Liu, Jing-Yun Ji, Fu Wu, Zhang Zhang & Guan-Zheng Luo
CAS Key Laboratory of Regenerative Biology, Guangzhou Institutes of Biomedicine and Health, Chinese Academy of Sciences, Guangzhou, China
Lingmei Jin & Jiekai Chen
School of Biomedical Sciences, LKS Faculty of Medicine, The University of Hong Kong, Hong Kong, China
Daniel W. Mak
You can also search for this author in PubMed Google Scholar
Contributions
G.-Z.L. conceived the project; Z.-D.Z. and Y.-Y.X. analyzed the data and wrote the manuscript; H.-X.C. conducted the experiments with the assistance from X.-H.L., J.-Y.J., Y.-L.L., F.W., L.J., and J.C.; Z.Z., D.W.M. and G.-Z.L. revised the manuscript. All authors reviewed the results and approved the final version of the manuscript.
Corresponding authors
Correspondence to Zhang Zhang or Guan-Zheng Luo .
Ethics declarations
Competing interests.
The authors declare no competing interests.
Peer review
Peer review information.
Nature Communications thanks the anonymous reviewers for their contribution to the peer review of this work. Peer reviewer reports are available.
Additional information
Publisher’s note Springer Nature remains neutral with regard to jurisdictional claims in published maps and institutional affiliations.
Supplementary information
Peer review file, reporting summary, rights and permissions.
Open Access This article is licensed under a Creative Commons Attribution 4.0 International License, which permits use, sharing, adaptation, distribution and reproduction in any medium or format, as long as you give appropriate credit to the original author(s) and the source, provide a link to the Creative Commons license, and indicate if changes were made. The images or other third party material in this article are included in the article’s Creative Commons license, unless indicated otherwise in a credit line to the material. If material is not included in the article’s Creative Commons license and your intended use is not permitted by statutory regulation or exceeds the permitted use, you will need to obtain permission directly from the copyright holder. To view a copy of this license, visit http://creativecommons.org/licenses/by/4.0/ .
Reprints and permissions
About this article
Cite this article.
Zhong, ZD., Xie, YY., Chen, HX. et al. Systematic comparison of tools used for m 6 A mapping from nanopore direct RNA sequencing. Nat Commun 14 , 1906 (2023). https://doi.org/10.1038/s41467-023-37596-5
Download citation
Received : 23 March 2022
Accepted : 21 March 2023
Published : 05 April 2023
DOI : https://doi.org/10.1038/s41467-023-37596-5
Share this article
Anyone you share the following link with will be able to read this content:
Sorry, a shareable link is not currently available for this article.
Provided by the Springer Nature SharedIt content-sharing initiative
By submitting a comment you agree to abide by our Terms and Community Guidelines . If you find something abusive or that does not comply with our terms or guidelines please flag it as inappropriate.
Quick links
- Explore articles by subject
- Guide to authors
- Editorial policies
Sign up for the Nature Briefing newsletter — what matters in science, free to your inbox daily.

How to evaluate perfusion imaging in post-treatment glioma: a comparison of three different analysis methods
- Diagnostic Neuroradiology
- Open access
- Published: 08 May 2024
Cite this article
You have full access to this open access article
- Siem D. A. Herings ORCID: orcid.org/0000-0003-4791-5681 1 , 2 ,
- Rik van den Elshout 1 , 2 ,
- Rebecca de Wit 1 , 2 ,
- Manoj Mannil 3 ,
- Cécile Ravesloot 1 , 2 ,
- Tom W. J. Scheenen 1 , 2 ,
- Anne Arens 1 , 2 ,
- Anja van der Kolk 1 ,
- Frederick J. A. Meijer 1 , 2 &
- Dylan J. H. A. Henssen 1 , 2
99 Accesses
Explore all metrics
Introduction
Dynamic susceptibility contrast (DSC) perfusion weighted (PW)-MRI can aid in differentiating treatment related abnormalities (TRA) from tumor progression (TP) in post-treatment glioma patients. Common methods, like the ‘hot spot’, or visual approach suffer from oversimplification and subjectivity. Using perfusion of the complete lesion potentially offers an objective and accurate alternative. This study aims to compare the diagnostic value and assess the subjectivity of these techniques.
50 Glioma patients with enhancing lesions post-surgery and chemo-radiotherapy were retrospectively included. Outcome was determined by clinical/radiological follow-up or biopsy. Imaging analysis used the ‘hot spot’, volume of interest (VOI) and visual approach. Diagnostic accuracy was compared using receiving operator characteristics (ROC) curves for the VOI and ‘hot spot’ approach, visual assessment was analysed with contingency tables. Inter-operator agreement was determined with Cohens kappa and intra-class coefficient (ICC).
29 Patients suffered from TP, 21 had TRA. The visual assessment showed poor to substantial inter-operator agreement (κ = -0.72 – 0.68). Reliability of the ‘hot spot’ placement was excellent (ICC = 0.89), while reference placement was variable (ICC = 0.54). The area under the ROC (AUROC) of the mean- and maximum relative cerebral blood volume (rCBV) (VOI-analysis) were 0.82 and 0.72, while the rCBV-ratio (‘hot spot’ analysis) was 0.69. The VOI-analysis had a more balanced sensitivity and specificity compared to visual assessment.
Conclusions
VOI analysis of DSC PW-MRI data holds greater diagnostic accuracy in single-moment differentiation of TP and TRA than ‘hot spot’ or visual analysis. This study underlines the subjectivity of visual placement and assessment.
Similar content being viewed by others
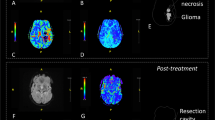
Reliability of dynamic susceptibility contrast perfusion metrics in pre- and post-treatment glioma
Discrimination between glioma grades ii and iii in suspected low-grade gliomas using dynamic contrast-enhanced and dynamic susceptibility contrast perfusion mr imaging: a histogram analysis approach, posttreatment dsc-mri is predictive of early treatment failure in children with supratentorial high-grade glioma treated with erlotinib.
Avoid common mistakes on your manuscript.
In the post-therapeutic setting of gliomas, it has been recommended to perform MRI within two days after surgery to assess the extent of resection, the presence of residual tumor tissue and the detection of complications [ 1 ]. However, an increasingly important part of neuroimaging concerns the visualisation of biological changes and the (early) prediction of response during or after treatment. In the follow-up imaging of gliomas treatment decisions are based on the observed effects, or lack of effect of the treatment. The Response Assessment in Neuro-Oncology (RANO) guidelines state that the response to therapy can be assessed by studying the extent of the T2/FLAIR signal and the size of the post-contrast enhancing region on the T 1 -weighted MRI over time [ 2 ]. However, early detection of tumor progression (TP) in post-treatment imaging of glioma is complicated due to the occurrence of treatment-related abnormalities (TRA; e.g., pseudoprogression and radiation necrosis). TRA and TP have similar imaging features on conventional MRI sequences, which results in poor differentiation between these two entities [ 3 ]. Therefore, more advanced MRI techniques (e.g., diffusion weighted and perfusion weighted MRI) play an important role in this setting.
Perfusion weighted imaging (PWI) is sensitive to changes in hemodynamics by differences in (micro-)vascularization such as local (neo)vascularization; it is therefore widely implemented in glioma imaging [ 4 ]. Three main PWI techniques are known: dynamic susceptibility contrast (DSC) imaging, dynamic contrast enhancing (DCE) imaging and arterial spin labelling (ASL). In ASL, labelling of bloodflow is used as endogenous contrast to measure perfusion, whereas the other two techniques use exogenous, gadolinium-based contrast agents which are intravenously injected [ 5 ]. DSC imaging is most commonly used in glioma imaging[ 4 ], as it is the most accurate and sensitive of the mentioned perfusion techniques [ 6 ], while also having a short acquisition time [ 7 ]. DSC perfusion imaging is based on a T 2 *-weighted pulse sequence in which the susceptibility induced signal loss resulting from the passage of a bolus of contrast agent is used for perfusion analysis. Various meta-analyses underline the diagnostic capacity of DSC perfusion-weighted imaging in the post-treatment setting to distinguish TRA from TP [ 8 , 9 , 10 ]. Despite its promise, perfusion–derived biomarkers have yet to be widely adopted. This discrepancy in potential and actual usage can be attributed to a shortfall of clinical technical skill, time constraints [ 11 ], inter-operator subjectivity in producing relative cerebral blood volume (rCBV) values, large variation in reported reliability of sequence processing and, disputed diagnostic criteria [ 6 ].
The inter-operator subjectivity can be explained by the often used ‘hot spot’ region of interest analysis, a lack of clinically validated cut-off values to differentiate TP from TRA, and a lack of standardization of imaging-acquisition techniques and postprocessing software [ 4 , 10 , 12 , 13 , 14 , 15 ]. More specifically, the ‘hot spot’ region of interest methodology is based on selecting and evaluating a region within the tumor with the highest perceived rCBV on a single image.
The’hot spot’ method, however, grossly underrepresents the complexity and heterogeneity of the region of interest (ROI) and substantially underestimates the volume of interest (VOI) as it often only partially covers the lesion in the most hyperintense region as seen on perfusion sequences. A more complete representation of the actual perfusion of the lesion can be acquired by segmenting its entire volume and then taking the mean rCBV of the entire contrast enhancing lesion. By selecting the entire volume, T1-weighted contrast-enhancing areas with low perfusion within the lesion are also taken into account and the heterogeneity in perfusion is thus accounted for in the mean rCBV value. The selection of the complete volume of the lesion allows for a mean rCBV value for the entire lesion and simultaneously allows for automatic detection of the actual highest rCBV value within a lesion (rCBVmax). Both of these metrics theoretically provide a more accurate representation of the perfusion of the lesion as a whole, compared to eyeballing the area of highest perfusion, which can be difficult in a lesion with multiple ‘hot spots’ or with mixed perfusion in the ROI. A more qualitative way of assessing perfusion data is a exclusively visual approach, in which the radiologist scores a tumor based on the visual perfusion without the use of rCBV values. Which of the mentioned techniques provides the best clinical differentiation between TP and TRA is not clear.
Therefore, the current study compared the diagnostic value of three approaches used to analyse DSC PW-MRI in distinguishing TRA from TP in post-treatment glioma patients. The compared approaches were I) a VOI based approach, II) a ‘hot spot’ based approach and III) a visual assessment of the same single-moment DSC PW-MRI data. Furthermore, the subjectivity of the ‘hot spot’ and visual assessment was studied. All analyses were carried out by independent experienced neuro-radiologists who were blinded to the outcome.
Materials and methods
Ethical approval, in- and exclusion criteria.
Ethical approval was waived by our institution’s ethical review board due to the retrospective nature of this study. We evaluated patients with suspected tumor recurrence between January 2021 and May 2022. Inclusion criteria comprised: I) 18 years of age or older with histopathologically proven glioma, II) treated with surgical resection, chemotherapy and radiation therapy, III) enlarging or new contrast-enhancing mass on follow-up MRI, IV) available pre-contrast T 1 -weighted images, post-contrast T 1 -weighted images and DSC PW-MRI perfusion weighted images and V) histopathological examination after lesion biopsy or surgical resection or clinical and radiological follow-up to determine the ground truth of the new enhancing lesion (TRA vs. TP). Exclusion criteria included: I) non-contrast enhancing tumor on MRI, II) distortion of MR signal due to blood products or other artefacts, III) histopathological entities other than glioma and IV) patients that opted-out of participating to research. As the inclusion criteria require a new-enhancing mass to be visible on follow-up MRI the duration until the follow-up scan used in the analyses can differ.
Defining the final outcome of the lesions
To determine if the final state of a lesion was TRA or TP, two methods were used in this study. If available, the gold-standard of histopathological confirmation was chosen as the primary means of determining the state of a lesion. If pathological material was unavailable, the often-used silver standard confirmation was used, consisting of clinical and radiological follow-up over a period of at least 3 months. The approach of clinical and radiological evaluation of the lesions over time complied with the RANO criteria [ 2 ].
MRI acquisition protocol
All MR imaging was performed on a 1.5 Tesla scanner (Magnetom Avanto Fit, Siemens Healthineers, Erlangen). The protocol included a 3D T 1 -weighted MPRAGE sequence (repetition time (TR) 2100 ms; echo time (TE) 2.42 ms; Inversion time (Ti) 900 ms; isotropic voxel size 1 mm; acquisition time 5 min) before intravenous injection of gadoteric acid (Dotarem, Guerbet, Villepinte) as contrast agent. Then, a preload bolus of gadoteric acid contrast agent was administered by continuous flow of contrast (Dotarem 0.1 ml/kg bodyweight at a flow rate of 5 ml/sec). After this an axial multi-slice 2D T 2 -weighted sequence (TR 5310 ms; TE 85 ms; resolution 1 × 1x5 mm; acquisition time 2:30 min) and a 2D Fluid-Attenuated Inversion Recovery (FLAIR) sequence (2D, TR 9000 ms; TE 87 ms; inversion time 2500 ms; resolution 1 × 1x5 mm; acquisition time 3:30 min) was acquired. Then a bolus of contrast (20 ml Dotarem at 5 ml/sec) was injected, followed by a T 2 *-weighted sequence (TR 1350 ms; TE 43 ms; voxel size 1.8 × 1.8x5 mm;acquisition time 2 min) and a T 1 -weighted 3D fast turbo spin echo sequence (TR 600 ms; TE 7.1 ms; isotropic voxel size 1 mm; acquisition time 3:26 min) The susceptibility induced signal loss on T 2 *-weighted sequences, resulting from the passage of the contrast agent, was used to acquire the DSC PW-MRI data.
Semi-quantitative ‘hot spot’ analysis
Three radiologists or radiology residents (between 5 and 23 years of experience in neuroimaging) were tasked with placing a 70 mm 2 circular ROI on the perceived ‘hot spot’, as seen in Fig. 1 . The readers were also asked to place a reference ROI of the same size in the contralateral centrum semiovale [ 16 ]. This reference placement has been shown to result in the most robust results when analysing perfusion metrics between different observers [ 17 ]. The mean rCBV value retrieved from the ROI placed on the ‘hot spot’ of the lesions was recorded as rCBV hotspot , while the reference was rCBV ref . The rCBV ratio was then calculated by dividing the rCBV hotspot by the rCBV ref . This was done to take the variation in base hemodynamics between patients into account, making the produced rCBV ratio a more standardized measure of perfusion.
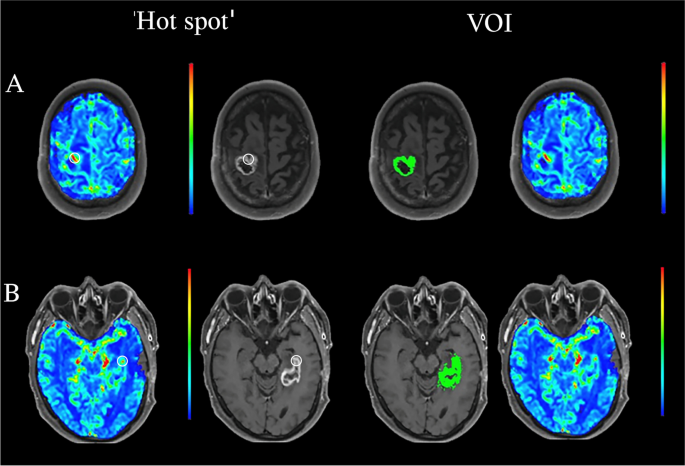
‘Hot spot’ and VOI processing of dynamic susceptibility contrast (DSC) images of two glioblastoma lesions. The white circular region of interest (ROI) markers seen in the DSC-PW (left) and post-contrast T1-weighted (right) sequences in the ‘hot spot’ column serve as an example of ROI placement using the ‘hot spot’ methodology commonly used in clinical practice. The area of slightly higher CBV measured in the perceived ‘hot spot’ correlates to a part of the enhancing region. In the VOI column the automatically selected lesion volume of interest in the same two patients can be seen in the post-contrast T1-weighted sequence (left), the respective lesion perfusion can be seen in the DSC-PW sequence (right). The contrast-enhancing region illustrated in A turned out to be tumor progression, while the lesions in B involuted over time and was therefore considered a treatment related abnormality
Semi-automatic VOI analysis
A workstation equipped with OsiriX MD (Version 12.0; http://www.osirix-viewer.com ) and a commercially available plug-in (IB Neuro; Imaging Biometrics, Elm Grove, Wisconsin), which uses a leakage-correction algorithm to process perfusion data and calculate perfusion maps [ 15 , 18 , 19 ] was used for this analysis. For semiautomated image analysis, we used IB Rad Tech (Imaging Biometrics, Elm Grove, Wisconsin), which is a workflow engine that plots rCBV maps in semi-automatically defined volumes of interest [ 18 , 20 , 21 ]. A standardisation of the cerebral blood volume map was automatically performed by IB Rad Tech using a standardisation protocol developed by Bedekar et al. [ 22 ]. To visualise the T1-enhancing lesion more clearly IB Rad Tech first subtracted the intensities of the standardized pre-contrast T1 from the standardized post-contrast T1, creating a ΔT1-weighted sequence. This was followed by the semi-automatic selection of defined volumes of interest, which concerns the manual drawing of an ROI around the ΔT1-weighted area of interest, after which the protocol automatically selected the voxels that showed contrast enhancement in the drawn ROI, thus creating a complete selection of the volume of interest as seen in Fig. 1 . Mean and maximum rCBV values and volumetric parameters of the contrast enhancing VOIs were automatically generated by the software package for each lesion. This semi-automatic analysis was done by a single radiology resident (D.H.) with profound experience using the software. This approach was chosen because the standardization protocol of the software package is known to produce highly reproducible rCBV values as it does not rely on manual reference placement and compensates for variability in acquisition differences [ 22 , 23 , 24 ]. Secondly, the semi-automatic VOI selection based on contrast-enhancing pixels in the ΔT1-weighted sequence left little room for inter-operator variability, as it is only susceptible to other contrast enhancing artifacts such as blood vessels, which trained radiologists should be able to identify.
Visual assessment of DSC PW-MRI data
Three radiologists or radiology residents with between 5 and 23 years of experience in (experimental) neuroimaging assessed the DSC PW-MRI data visually or by drawing an ROI and recorded their suspected outcome of the lesion, being either TP or TRA. These predictions were mainly based on the perfusion of the lesion, as it is known that features observed on conventional imaging do not contribute to accurate differentiation [ 3 ]. This analysis was based on a single imaging session, so there was no follow-up or predating imaging available during the analysis. The analysis was performed on a workstation equipped with Syngo.via VB60 (Siemens Healthineers, Erlangen, Germany). Next to the DSC PW-MRI sequences, the readers were provided with a T 1 -weighted sequence pre- and post-contrast and a T 2 -FLAIR sequence to aid with anatomical orientation and lesion identification. The colour scale used to analyse the PW-MRI data was spectrum 10-step, a calibrated colour scale provided in the Syngo.via program, meaning that the colours visible were chosen to fit on a set scale, meaning that the impact of the chosen colours on decision making is minimized. The predicted outcome was then compared to the definitive outcome. The definitive outcome was determined based on available clinical and radiological follow-up and/or histopathological data. The definitive outcomes were then dichotomized by one of the authors, who was still blinded to the definite outcome at this stage of the research process.
Statistical analyses
IBM SPSS Statistics for Windows (version 28.01.1, Armonk, USA) was used for most of the statical analyses of this study. To assess the variability between the different readers of the ‘hot spot’ DSC PW-MRI data the intraclass correlation coefficient (ICC) was determined for the measured rCBV ratio, rCBV hotspot and rCBV ref . After this, the ICC was used to assess the inter-observer variability of the rCBV ratio measured in the ‘hot spot’ analysis.
Normality of the complete VOI segmentation voxel-wise rCBV data was assessed by use of the Kolmogorov–Smirnov test. When the data was normally distributed, mean, and standard deviation values were compared between groups using the independent Student’s t-test. If the data showed a non-normal distribution, the Mann–Whitney U-test was used to compare the median and range data between patients with TRA and TP. To evaluate whether the volume of the lesion had any correlation with the rCBV values of the lesions, a Pearson Correlation analysis was carried out.
The visual assessment data was analysed using contingency tables, resulting in the sensitivity and specificity achieved per reader. Cohen’s kappa was then used as a measure for the interobserver agreement.
A Receiver Operating Characteristic (ROC) curve was created to determine a VOI-based rCBV cut-off value with a balanced sensitivity–specificity ratio, ultimately able to classify TRA and TP. The same analysis was done to determine the semi-quantitative ‘hot spot’ based rCBV ratio cut-off value, able to classify TRA and TP. In both cases the most balanced sensitivity–specificity ratio was determined by selecting the point on the ROC-curve most closely located to the top-left corner of the unit square. The corresponding rCBV cut-off value of this sensitivity–specificity ratio was then retrieved from SPSS, together with an AUROC value indicating the diagnostic accuracy. Then Delong’s test as available in the pROC package for R [ 25 ] was used to compare the AUC’s of the created ROC curves, for this analysis R was used (version 4.3.1, R Foundation for Statistical Computing, Vienna, Austria.).
Demographics of the included patients
In total, 50 glioma patients were included in this study. Based on the WHO classification of gliomas [ 26 ], thirty-eight patients had a medical history of glioblastoma (76%), nine patients were treated for an IDH-mutant astrocytoma grade 2–4 (16%) and three patients were previously diagnosed with an oligodendroglioma (8%). An overview of the included patients can be found in Table 1 .
Three patients underwent stereotactic biopsy; in those cases, histopathological confirmation of the tumor outcome was used. In all other included patients, the final outcome was defined by use of clinical and radiological follow-up over a follow-up period of at least three months. In twenty-nine cases, the new contrast enhancing lesion was found to reflect TP (58%). In the other twenty-one cases the enhancing region turned out to involute over time and was interpreted as TRA. The mean ages in the TP and TRA groups differed significantly (t(48) = 2.09, p = 0.04).
Statistical analysis of the semi-quantitative ‘hot spot’ data
The ICC of the measured rCBV ratio was 0.83 (95%-CI: 0.73–0.90). While the ICC of the measured rCBV hotspot was 0.89 (95%-CI: 0.82–0.93) and the ICC of the rCBV ref was 0.54 (95%-CI: 0.26–0.72). The ICC of a comparison between the ‘hot spot’ rCBV ratio and the rCBV mean from the VOI assessment, resulted in an ICC of 0.72 (95%-CI: 0.56–0.83).
Statistical analysis of the VOI PWI data
The Kolmogorov–Smirnov test showed that the VOI rCBV mean data was normally distributed (D(50) = 0.09, p = 0.20), whereas the rCBV max and volumetric data showed a non-normal, right-skewed distribution (D(50) = 0.13, p = 0.03 and D(50) = 0.28, p < 0.001 respectively). The retrieved rCBV values and volumes per group can be seen in Table 2 .
Significant differences were observed between the measured rCBV mean and rCBV max values in TP and TRA lesions (t(48) = 4.3, p < 0.001 and U = 168, p = 0.007 respectively). The median volumes in the TP and TRA lesions also showed significant differences between the groups (U = 175, p = 0.011). Pearson correlation analysis showed that rCBV max value was significantly correlated with volume of the lesions ( p < 0.001; r = 0.64). The rCBV mean value, on the other hand, was not significantly correlated with volume of the lesions ( p = 0.24; r = 0.17).
Statistical analysis of the visual assessment data
The radiologists or radiology residents were anonymized as reader X, Y and Z, their results can be seen in Table 3 . The inter-observer agreement between reader X and Y was κ = 0.65, between reader X and Z it was κ = -0.72 and between reader Y and Z it was κ = -0.98.
Diagnostic accuracy of different post-processing methods of PWI data
The Receiver Operating Characteristic (ROC) curves can be found in Fig. 2 ; in both curves TP was seen as the positive state. The VOI-based analysis showed an AUROC of 0.72 (95%-CI: 0.58–0.86) and 0.82 (95%-CI: 0.70–0.94) for rCBV max values and rCBV mean values, respectively. Regarding rCBV max , a cut-off value of 5.1 mL/100 g resulted in a sensitivity of 69% and specificity of 67%. A cut-off value of the rCBV mean of 1.11 mL/100 g resulted in a balanced sensitivity and specificity value of 72% and 76%, respectively, to distinguish TP from TRA.
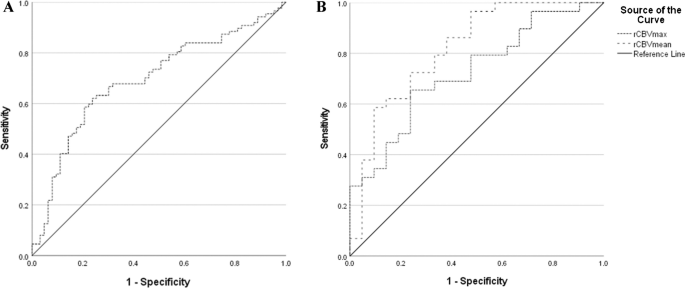
Receiver operator characteristics (ROC) curves based on the ‘hot spot’ ( A ) and semi-automatic volume of interest ( B ) analysis of relative cerebral blood volume (rCBV) data to distinguish tumor progression from treatment related abnormalities. Area under the ROC curve (AUROC) for the rCBV ratio of the ‘hot spot’ analysis was 0.69 (95%-CI: 0.61–0.78). The AUROC of rCBV max and rCBV mean are 0.72 (95%-CI: 0.58–0.86) and 0.82 (95%-CI: 0.70–0.94) respectively
For the semi-quantitative ‘hot spot’ analysis an ROC curve based on measured rCBV ratio was made based on the combined analysis of the three readers. This resulted in an AUROC of 0.69 (95%-CI: 0.61–0.78). The achieved sensitivity and specificity of this analysis is 67% and 70%, based on a balanced rCBV cut-off of 1.63 mL/100 g. DeLong’s test used on the semi-automatic VOI analysis ROC curve indicated a significant difference between the AUROC of the rCBV mean and rCBV ratio from the ‘hot spot’ analysis (Z = -2.26, p = 0.023). It also showed a significant difference between the AUROC of rCBV mean and rCBV max (Z = -2.655, p = 0.0079) and a non-significant difference between the AUROC of the rCBV max and the rCBV ratio from the ‘hot spot’ analysis (Z = -0.50, p = 0.61).
This study illustrates that at its current state the usage of VOI based DSC PW-MRI analysis compared to a ‘hot spot’ or purely visual approach results in a slightly higher diagnostic accuracy. It also elucidates the subjectivity of the ‘hot spot’ and visual approach, which is mostly circumvented by the usage of VOI based analyses.
When used to distinguish TP from TRA in this study, a VOI cut-off value of rCBV mean of 1.11 mL/100 g resulted in a sensitivity and specificity value of 72% and 76%, respectively (AUROC = 0.82). In comparison, the highest scoring observer using the visual assessment methodology, had a sensitivity and specificity of 41% and 91% respectively. The sensitivity and specificity achieved with ‘hot spot’ assessment of DSC perfusion data was 67% and 70% respectively (AUROC = 0.69). While the achieved sensitivity and specificity in this study are mediocre, it should be noted that in clinical reality a treatment decision would be made after a follow-up period and with availability of predating scans, in that setting a higher sensitivity and specificity is to be expected. This study is meant to analyse the difference in predictive accuracy of these different techniques based on a single moment analysis.
On a group level, the rCBV max of a lesion showed a moderate correlation with the volume of the lesion ( p < 0.001; r = 0.64), while the mean rCBV value does not seem to be influenced by the volume ( p = 0.24; r = 0.17). The nature of this correlation on an individual level has not been tested in this study, so the assumption that a large lesion will have a higher rCBV max and is thus more likely to be TP should not be made without consulting other means of differentiating TP and TRA. The differences found in rCBV max, rCBV mean and median volumes between the TP and TRA group in this study match the hypothesis that perfusion is different between the two mentioned states. However, as the ages between the groups differ significantly (t(48) = 2.09, p = 0.04), with TRA having a higher mean age, this comparison should be used with caution as the age difference could also potentially explain the found differences.
DSC PW-MRI in the radiological follow-up of post-treatment glioma lesions is widely used to distinguish TRA from TP. Three recent reviews on this topic provided a sensitivity ranging from 83–93% and a specificity ranging from 75–88%, indicating good diagnostic accuracy with regard to distinguishing TRA from TP [ 8 , 9 , 10 ]. However, the included studies in these three meta-analyses mainly used the ‘hot spot’ technique. Next to that these studies differed from ours, as some of the studies they base their results on differentiated between pseudoprogression and radiation necrosis, while we used TRA as a term to cover both. Included studies also often used predating or follow up scans in their analysis and not single moment data. In this study the single-moment ‘hot spot’ methodology was performed by three experienced neuro-radiologists and resulted in an AUROC (0.69) which was significantly lower than the AUROC of the ROC graph based on the rCBV mean from the VOI study (0.82), as tested with the DeLong’s test (Z = -2.26, p = 0.023). In the ‘hot spot’ assessment, the ICC showed poor reliability in the placement of references (ICC = 0.54), despite using a clearly communicated reference placement in the contralateral centrum semiovale that should have had the least inter-observer variability [ 17 ]. This might be explained by variation in the chosen slice in which the readers chose to place their reference, however this level of subjectivity with an agreed placement of reference underlines the weakness of manual placement. The placement of the ‘hot spot’ itself resulted in an excellent reliability (ICC = 0.89) between the observers, indicating that the manually placed ‘hot spot’ does provide similar values between researchers. Besides that, the ‘hot spot’ placement also showed moderate reliability when compared to a complete VOI analysis (ICC = 0.72), indicating that if done correctly the ‘hot spot’ placement does match the rCBV found in a complete VOI analysis.
Additionally, a single moment visual assessment of the same DSC perfusion data was also included in this study, showing large differences between the achieved sensitivity and specificity per neuro-radiologist, ranging from 41–86% and 33–91% respectively. The found agreement between the neuro-radiologists or residents differed from poor (κ = -0.98, κ = -0.72) to substantial (κ = 0.65), indicating that there was a high degree of subjectivity. While this assessment deviated from clinical practice due to a lack of available follow-up or predating images, the found sensitivity–specificity ratios found per radiologist indicate that in order to get a high sensitivity or specificity they greatly sacrifice the other. In this diagnostic dilemma a high specificity is preferred, as you want to correctly diagnose TP to allow for earlier treatment, but the sacrificed sensitivity means that TP is often missed if specificity is prioritized.
A comparison between the inter-operator agreement of the ‘hot spot’ analysis and the agreement between the readers in the visual assessment illustrates that the visual approach is most susceptible to subjectivity. In the visual assessment the differences between readers were very large, while in the ‘hot spot’ approach the placement of the ROI was reliable between all readers. Only the reference placement showed variability. We feel the disagreement in the visual assessment reflects the clinical tendencies of radiologists as the expert readers were not instructed to act more defensively or otherwise. In this case a defensive choice would be to opt for the ‘worst-case’ scenario, being TP. Reader Z chose to utilise a more defensive approach than the others, resulting in more frequent TP diagnoses (predictions reader Z: 39 TP, reader X: 14 TP and reader Y: 18 TP), thus causing the found disagreement. This disagreement underlines the subjectivity of visual assessment even between trained radiologists.
All in all, this study confirms that a single-moment exclusively visual assessment of post-operative glioma DSC PW-MRI data is vulnerable to inter-observer variability. This finding and the low ICC found in the reference placement of the ‘hot spot’ analysis matches the high inter-observer variability found in Kouwenberg et al. [ 27 ] and Smits et al. [ 16 ]. Both studies describe that the placement of ‘hot spots’ and references in post-treatment glioma DSC PW-MRI data shows low reliability and reproducibility. It is therefore recommended that if rCBV is visually measured in post-operative glioma patients it should be carried out by two readers and with precaution.
The use of semi-automatic complete VOI DSC has been attributed good discriminative power to distinguish TP from TRA. For example, in a recent study rCBV values of a complete VOI obtained from DSC PW-MRI yielded an AUROC of 0.81 to distinguish TRA from TP [ 21 ]. Similar results were obtained in the rCBV values of a complete VOI analysis of DSC PW-MRI data in the setting of metastatic neuro-oncological disease. In the study of Kuo et al., thirty subjects with 37 lesions were investigated (20 TRA; 17 TP). When using rCBV values of a VOI obtained from DSC PW-MRI data, an AUROC of 0.79 was yielded to discriminate TRA from TP [ 20 ]. The outcome of these papers is corroborated by the current results, as we found an AUROC of 0.82 (95%-CI: 0.70–0.94) for the mean rCBV in the semi-automatic VOI analysis.
It has been reported that implementation of DSC PW-MRI in routine follow-up MRI of glioma can aid the early diagnosis of TP [ 28 ]. In this study, a standardised perfusion acquisition protocol and standardised methodology to process data with well-validated criteria was used. This has been recommended by others for research on post-treatment radiological evaluation of glioma patients [ 13 ]. However, harmonization of the imaging protocol and the post-processing work-flow remains lacking, probably explaining the wide range of cut-off values that have been reported in literature [ 6 ]. Also, these differences hinder the sharing and pooling of imaging data. Nevertheless, important steps with regard to the standardisation of DSC acquisition parameters have been taken during the last years [ 12 , 13 , 14 ].
Strengths and limitations
In the VOI analysis, the entire T1-weighted contrast enhancing lesion was included, which limits the inter- and intra-observer variability when compared to manual placement of regions of interest. The used IB-Rad Tech software and it’s semi-automatic processing of DSC-PWI images has been described to further reduce user-related variability [ 22 ], as its automated standardisation circumvents the manual placement of references which was shown to be especially vulnerable to subjectivity. Another strength of the current study concerns the fact that for the first time, single-moment rCBV values of VOIs were directly compared with rCBV values of ‘hot spots’ and a purely visual assessment, which are commonly used in standard clinical reading of DSC PW-MRI data in the post-treatment evaluation of gliomas to distinguish TP from TRA.
An important limitation of the current study comprised its retrospective nature and the lack of an external validation cohort in which the observed threshold values can be tested. It must be emphasized that the differences between the rCBV values of the VOIs were valid on a group level; the usefulness of this technique in individual patients needs further investigation.
The single-moment analysis of the PWI data can also be seen as a limitation, as a three to six-month period of follow-up imaging and pre-operative scans are usually utilised to differentiate between TP and TRA. However, readers were informed with regard to the fact that the MR data they read were the first imaging study on which a new or growing contrast-enhancing lesion appeared. Therefore, it was not expected that this single-moment analysis impacted the outcome of the reader study.
The heterogeneity of the studied population can be seen as a limitation as they possess inherent differences in malignancy grade and therefore in behaviour and treatment options. However, all included subtypes can progress on follow-up imaging and can show new enhancing lesions in the post-treatment setting. As this study investigated the diagnostic accuracy of three approaches to analyse DSC PW-MRI data when new contrast-enhancing lesions occur, the impact of the rather heterogenous population is considered minimal.
The small number of patients per subgroup (when stratified by for example tumor type or treatment schedule) prevented a statistically sound analysis of the used subgroups and identification of potential differences between the used pathologies. As the scope of this study was aimed at elucidating differences between the used methodologies, this was deemed acceptable by the authors, however a future study with larger subgroups per pathology and treatment used should aid in the identification of potential differences.
A final limitation is the usage of a 1,5 T MRI system instead of a 3 T system. A 3 T scanner would allow for an increased signal-to-noise (SNR) ratio, increased temporal- and spatial resolution. However, DSC PW-MRI is often not limited by SNR, and the usage of 1,5 T in glioma imaging shows an almost perfect correlation with 3 T in MR modalities such as rCBV and identified lesion volume [ 29 ] so using a 1,5 T system should be sufficient in answering our study objectives.
This paper shows that a semi-automatic processing of the complete lesion achieves a greater diagnostic accuracy in the post-treatment radiological follow-up of glioma patients on a group level compared to a ‘hot spot’ or visual approach in a single moment analysis of DSC PWI data. The visual and ‘hot spot’ based approaches are also more subject to inter-observer variability, especially in the placement of references.
Data availability
The datasets generated during and/or analyzed during the current study are not available to ensure complete patient anonymity.
Abbreviations
Arterial Spin Labelling
Area Under the Receiver Operating Characteristics
Dynamic Contrast Enhancement
Dynamic Susceptibility imaging
Fluid Attenuated Inversion Recovery
Intraclass correlation coefficient
Isocitrate Dehydrogenases
Methylguanine Methyltransferase
Perfusion Weighted Imaging
Response Assessment in Neuro-Oncology
Relative Cerebral Blood Volume
Receiver Operating Characteristic
Region Of Interest
Inversion time
Tumor Progression
Repetition Time
Tumor Related Abnormalities
Signal-to-noise ratio
Volume Of Interest
World Health Organization
Zikou A, Sioka C, Alexiou GA, Fotopoulos A, Voulgaris S, Argyropoulou MI (2018) Radiation necrosis, pseudoprogression, pseudoresponse, and tumor recurrence: imaging challenges for the evaluation of treated gliomas. Contrast Media Mol Imaging 2018:6828396. https://doi.org/10.1155/2018/6828396
Article CAS PubMed PubMed Central Google Scholar
Wen PY, Macdonald DR, Reardon DA, Cloughesy TF, Sorensen AG, Galanis E, Degroot J, Wick W, Gilbert MR, Lassman AB, Tsien C, Mikkelsen T, Wong ET, Chamberlain MC, Stupp R, Lamborn KR, Vogelbaum MA, van den Bent MJ, Chang SM (2010) Updated response assessment criteria for high-grade gliomas: response assessment in neuro-oncology working group. J Clin Oncol 28(11):1963–1972. https://doi.org/10.1200/JCO.2009.26.3541
Article PubMed Google Scholar
Young RJ, Gupta A, Shah AD, Graber JJ, Zhang Z, Shi W, Holodny AI, Omuro AM (2011) Potential utility of conventional MRI signs in diagnosing pseudoprogression in glioblastoma. Neurology 76(22):1918–1924. https://doi.org/10.1212/WNL.0b013e31821d74e7
Thust SC, Heiland S, Falini A, Jager HR, Waldman AD, Sundgren PC, Godi C, Katsaros VK, Ramos A, Bargallo N, Vernooij MW, Yousry T, Bendszus M, Smits M (2018) Glioma imaging in Europe: a survey of 220 centres and recommendations for best clinical practice. Eur Radiol 28(8):3306–3317. https://doi.org/10.1007/s00330-018-5314-5
Jahng GH, Li KL, Ostergaard L, Calamante F (2014) Perfusion magnetic resonance imaging: a comprehensive update on principles and techniques. Korean J Radiol 15(5):554–577. https://doi.org/10.3348/kjr.2014.15.5.554
Article PubMed PubMed Central Google Scholar
Smits M (2021) MRI biomarkers in neuro-oncology. Nat Rev Neurol 17(8):486–500. https://doi.org/10.1038/s41582-021-00510-y
Wintermark M, Sesay M, Barbier E, Borbely K, Dillon WP, Eastwood JD, Glenn TC, Grandin CB, Pedraza S, Soustiel JF, Nariai T, Zaharchuk G, Caille JM, Dousset V, Yonas H (2005) Comparative overview of brain perfusion imaging techniques. J Neuroradiol 32(5):294–314. https://doi.org/10.1016/s0150-9861(05)83159-1
Article CAS PubMed Google Scholar
Wang L, Wei L, Wang J, Li N, Gao Y, Ma H, Qu X, Zhang M (2020) Evaluation of perfusion MRI value for tumor progression assessment after glioma radiotherapy: a systematic review and meta-analysis. Medicine (Baltimore) 99(52):e23766. https://doi.org/10.1097/MD.0000000000023766
van Dijken BRJ, van Laar PJ, Holtman GA, van der Hoorn A (2017) Diagnostic accuracy of magnetic resonance imaging techniques for treatment response evaluation in patients with high-grade glioma, a systematic review and meta-analysis. Eur Radiol 27(10):4129–4144. https://doi.org/10.1007/s00330-017-4789-9
Patel P, Baradaran H, Delgado D, Askin G, Christos P, John Tsiouris A, Gupta A (2017) MR perfusion-weighted imaging in the evaluation of high-grade gliomas after treatment: a systematic review and meta-analysis. Neuro Oncol 19(1):118–127. https://doi.org/10.1093/neuonc/now148
Manfrini E, Smits M, Thust S, Geiger S, Bendella Z, Petr J, Solymosi L, Keil VC (2021) From research to clinical practice: a European neuroradiological survey on quantitative advanced MRI implementation. Eur Radiol 31(8):6334–6341. https://doi.org/10.1007/s00330-020-07582-2
Welker K, Boxerman J, Kalnin A, Kaufmann T, Shiroishi M, Wintermark M, American Society of Functional Neuroradiology MRPS, Practice Subcommittee of the ACPC (2015) ASFNR recommendations for clinical performance of MR dynamic susceptibility contrast perfusion imaging of the brain. AJNR Am J Neuroradiol 36(6):E41-51. https://doi.org/10.3174/ajnr.A4341
Boxerman JL, Quarles CC, Hu LS, Erickson BJ, Gerstner ER, Smits M, Kaufmann TJ, Barboriak DP, Huang RH, Wick W, Weller M, Galanis E, Kalpathy-Cramer J, Shankar L, Jacobs P, Chung C, van den Bent MJ, Chang S, Al Yung WK, Cloughesy TF, Wen PY, Gilbert MR, Rosen BR, Ellingson BM, Schmainda KM, Jumpstarting Brain Tumor Drug Development Coalition Imaging Standardization Steering C (2020) Consensus recommendations for a dynamic susceptibility contrast MRI protocol for use in high-grade gliomas. Neuro Oncol 22(9):1262–1275. https://doi.org/10.1093/neuonc/noaa141
Alsop DC, Detre JA, Golay X, Gunther M, Hendrikse J, Hernandez-Garcia L, Lu H, MacIntosh BJ, Parkes LM, Smits M, van Osch MJ, Wang DJ, Wong EC, Zaharchuk G (2015) Recommended implementation of arterial spin-labeled perfusion MRI for clinical applications: a consensus of the ISMRM perfusion study group and the European consortium for ASL in dementia. Magn Reson Med 73(1):102–116. https://doi.org/10.1002/mrm.25197
Schmainda KM, Prah MA, Hu LS, Quarles CC, Semmineh N, Rand SD, Connelly JM, Anderies B, Zhou Y, Liu Y, Logan B, Stokes A, Baird G, Boxerman JL (2019) Moving toward a consensus DSC-MRI protocol: validation of a low-flip angle single-dose option as a reference standard for brain tumors. AJNR Am J Neuroradiol 40(4):626–633. https://doi.org/10.3174/ajnr.A6015
Smits M, Bendszus M, Collette S, Postma LA, Dhermain F, Hagenbeek RE, Clement PM, Liu Y, Wick W, van den Bent MJ, Heiland S (2019) Repeatability and reproducibility of relative cerebral blood volume measurement of recurrent glioma in a multicentre trial setting. Eur J Cancer 114:89–96. https://doi.org/10.1016/j.ejca.2019.03.007
Oei MTH, Meijer FJA, Mordang JJ, Smit EJ, Idema AJS, Goraj BM, Laue HOA, Prokop M, Manniesing R (2018) Observer variability of reference tissue selection for relativecerebral blood volume measurements in glioma patients. Eur Radiol 28(9):3902–3911. https://doi.org/10.1007/s00330-018-5353-y
Hu LS, Eschbacher JM, Heiserman JE, Dueck AC, Shapiro WR, Liu S, Karis JP, Smith KA, Coons SW, Nakaji P, Spetzler RF, Feuerstein BG, Debbins J, Baxter LC (2012) Reevaluating the imaging definition of tumor progression: perfusion MRI quantifies recurrent glioblastoma tumor fraction, pseudoprogression, and radiation necrosis to predict survival. Neuro Oncol 14(7):919–930. https://doi.org/10.1093/neuonc/nos112
Prah MA, Al-Gizawiy MM, Mueller WM, Cochran EJ, Hoffmann RG, Connelly JM, Schmainda KM (2018) Spatial discrimination of glioblastoma and treatment effect with histologically-validated perfusion and diffusion magnetic resonance imaging metrics. J Neurooncol 136(1):13–21. https://doi.org/10.1007/s11060-017-2617-3
Kuo F, Ng NN, Nagpal S, Pollom EL, Soltys S, Hayden-Gephart M, Li G, Born DE, Iv M (2022) DSC perfusion mri-derived fractional tumor burden and relative CBV differentiate tumor progression and radiation necrosis in brain metastases treated with stereotactic radiosurgery. AJNR Am J Neuroradiol 43(5):689–695. https://doi.org/10.3174/ajnr.A7501
Iv M, Liu X, Lavezo J, Gentles AJ, Ghanem R, Lummus S, Born DE, Soltys SG, Nagpal S, Thomas R, Recht L, Fischbein N (2019) Perfusion MRI-based fractional tumor burden differentiates between tumor and treatment effect in recurrent glioblastomas and informs clinical decision-making. AJNR Am J Neuroradiol 40(10):1649–1657. https://doi.org/10.3174/ajnr.A6211
Bedekar D, Jensen T, Schmainda KM (2010) Standardization of relative cerebral blood volume (rCBV) image maps for ease of both inter- and intrapatient comparisons. Magn Reson Med 64(3):907–913. https://doi.org/10.1002/mrm.22445
Prah MA, Stufflebeam SM, Paulson ES, Kalpathy-Cramer J, Gerstner ER, Batchelor TT, Barboriak DP, Rosen BR, Schmainda KM (2015) Repeatability of standardized and normalized relative cbv in patients with newly diagnosed glioblastoma. AJNR Am J Neuroradiol 36(9):1654–1661. https://doi.org/10.3174/ajnr.A4374
Hu LS, Kelm Z, Korfiatis P, Dueck AC, Elrod C, Ellingson BM, Kaufmann TJ, Eschbacher JM, Karis JP, Smith K, Nakaji P, Brinkman D, Pafundi D, Baxter LC, Erickson BJ (2015) Impact of software modeling on the accuracy of perfusion MRI in glioma. AJNR Am J Neuroradiol 36(12):2242–2249. https://doi.org/10.3174/ajnr.A4451
Robin X, Turck N, Hainard A, Tiberti N, Lisacek F, Sanchez JC, Muller M (2011) pROC: an open-source package for R and S+ to analyze and compare ROC curves. BMC Bioinformatics 12:77. https://doi.org/10.1186/1471-2105-12-77
Louis DN, Perry A, Wesseling P, Brat DJ, Cree IA, Figarella-Branger D, Hawkins C, Ng HK, Pfister SM, Reifenberger G, Soffietti R, von Deimling A, Ellison DW (2021) The 2021 WHO classification of tumors of the central nervous system: a summary. Neuro Oncol 23(8):1231–1251. https://doi.org/10.1093/neuonc/noab106
Kouwenberg V, van Santwijk L, Meijer FJA, Henssen D (2022) Reliability of dynamic susceptibility contrast perfusion metrics in pre- and post-treatment glioma. Cancer Imaging 22(1):28. https://doi.org/10.1186/s40644-022-00466-2
Kim HS, Goh MJ, Kim N, Choi CG, Kim SJ, Kim JH (2014) Which combination of MR imaging modalities is best for predicting recurrent glioblastoma? Study of diagnostic accuracy and reproducibility. Radiology 273(3):831–843. https://doi.org/10.1148/radiol.14132868
Tselikas L, Souillard-Scemama R, Naggara O, Mellerio C, Varlet P, Dezamis E, Domont J, Dhermain F, Devaux B, Chretien F, Meder JF, Pallud J, Oppenheim C (2015) Imaging of gliomas at 1.5 and 3 Tesla - A comparative study. Neuro Oncol 17(6):895–900. https://doi.org/10.1093/neuonc/nou332
Download references
Acknowledgements
No acknowledgements
The authors declare that no funds, grants, or other support were received during the preparation of this manuscript.
Author information
Authors and affiliations.
Department of Medical Imaging, Radboud University Medical Center, Nijmegen, The Netherlands
Siem D. A. Herings, Rik van den Elshout, Rebecca de Wit, Cécile Ravesloot, Tom W. J. Scheenen, Anne Arens, Anja van der Kolk, Frederick J. A. Meijer & Dylan J. H. A. Henssen
Radboudumc Center of Expertise Neuro-Oncology, Nijmegen, The Netherlands
Siem D. A. Herings, Rik van den Elshout, Rebecca de Wit, Cécile Ravesloot, Tom W. J. Scheenen, Anne Arens, Frederick J. A. Meijer & Dylan J. H. A. Henssen
University Clinic for Radiology, Westfälische Wilhelms-University Muenster and University Hospital Muenster, Albert-Schweitzer-Campus 1, E48149, Muenster, Germany
Manoj Mannil
You can also search for this author in PubMed Google Scholar
Contributions
All authors contributed to the study conception and design. Material preparation, data collection and analysis were performed by Rebecca de Wit, Siem Herings and Dylan Henssen. The first draft of the manuscript was written by Rik van den Elshout and Dylan Henssen. The final manuscript was written by Siem Herings. All authors commented on different versions of the manuscript. All authors read and approved the final manuscript.
Corresponding author
Correspondence to Siem D. A. Herings .
Ethics declarations
Ethical approval & consent to participate.
After reviewing the research protocol, ethical approval was waived due to the retrospective nature of this study. Patients who did not provide consent to have their imaging data used in scientific research were not included in this study.
Consent for publication
All authors read and approved the final manuscript and gave consent for publication.
Competing interests
The authors have no relevant financial or non-financial interests to disclose.
FM: Speaker bureau Canon Medical Systems (unrelated).
Additional information
Publisher's note.
Springer Nature remains neutral with regard to jurisdictional claims in published maps and institutional affiliations.
Rights and permissions
Open Access This article is licensed under a Creative Commons Attribution 4.0 International License, which permits use, sharing, adaptation, distribution and reproduction in any medium or format, as long as you give appropriate credit to the original author(s) and the source, provide a link to the Creative Commons licence, and indicate if changes were made. The images or other third party material in this article are included in the article's Creative Commons licence, unless indicated otherwise in a credit line to the material. If material is not included in the article's Creative Commons licence and your intended use is not permitted by statutory regulation or exceeds the permitted use, you will need to obtain permission directly from the copyright holder. To view a copy of this licence, visit http://creativecommons.org/licenses/by/4.0/ .
Reprints and permissions
About this article
Herings, S.D.A., van den Elshout, R., de Wit, R. et al. How to evaluate perfusion imaging in post-treatment glioma: a comparison of three different analysis methods. Neuroradiology (2024). https://doi.org/10.1007/s00234-024-03374-3
Download citation
Received : 16 November 2023
Accepted : 01 May 2024
Published : 08 May 2024
DOI : https://doi.org/10.1007/s00234-024-03374-3
Share this article
Anyone you share the following link with will be able to read this content:
Sorry, a shareable link is not currently available for this article.
Provided by the Springer Nature SharedIt content-sharing initiative
- Volume of interest
- Dynamic susceptibility contrast
- Perfusion weighted magnetic resonance imaging
- Tumor progression
- Treatment-related abnormality
- Find a journal
- Publish with us
- Track your research
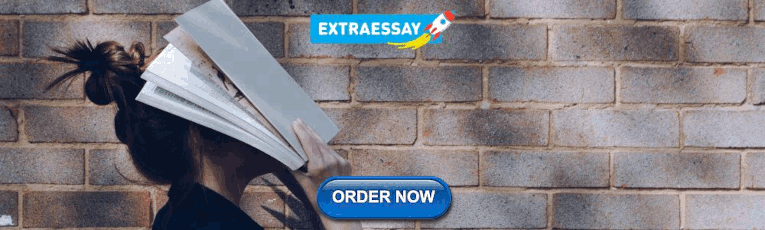
VIDEO
COMMENTS
Use Clear Transitions. Transitions are important in compare and contrast essays, where you will be moving frequently between different topics or perspectives. Examples of transitions and phrases for comparisons: as well, similar to, consistent with, likewise, too. Examples of transitions and phrases for contrasts: on the other hand, however ...
Making effective comparisons. As the name suggests, comparing and contrasting is about identifying both similarities and differences. You might focus on contrasting quite different subjects or comparing subjects with a lot in common—but there must be some grounds for comparison in the first place. For example, you might contrast French ...
Making a Venn diagram or a chart can help you quickly and efficiently compare and contrast two or more things or ideas. To make a Venn diagram, simply draw some overlapping circles, one circle for each item you're considering. In the central area where they overlap, list the traits the two items have in common.
The compare-and-contrast essay starts with a thesis that clearly states the two subjects that are to be compared, contrasted, or both and the reason for doing so. The thesis could lean more toward comparing, contrasting, or both. Remember, the point of comparing and contrasting is to provide useful knowledge to the reader.
Determining the structure of your essay is the most important step towards conducting and presenting to the reader a well-developed comparison. Students are often asked to compare things in twos. For example, compare these two articles, or two characters in a novel, or a film and a novel or an article and a poem... The possibilities are endless.
Step One: Identify the key concepts of the two things to be contrasted/compared. You must have a clear understanding of each text or concept before you try to compare them to each other. Once you get the question, go back and look over your lecture notes. Then, if you have time, go back and re-read (or at least skim) the texts you will be using ...
An academic compare and contrast essay looks at two or more subjects, ideas, people, or objects, compares their likeness, and contrasts their differences. It's an informative essay that provides insights on what is similar and different between the two items. Depending on the essay's instructions, you can focus solely on comparing or ...
4.1: Introduction to Comparison and Contrast Essay. The key to a good compare-and-contrast essay is to choose two or more subjects that connect in a meaningful way. Comparison and contrast is simply telling how two things are alike or different. The compare-and-contrast essay starts with a thesis that clearly states the two subjects that are to ...
A comparative paper asks that you compare at least two (possibly more) items. These items will differ depending on the assignment. You might be asked to compare: positions on an issue (e.g., responses to midwifery in Canada and the United States) theories (e.g., capitalism and communism) figures (e.g., GDP in the United States and Britain)
The Structure of a Compare/Contrast Essay. The compare-and-contrast essay starts with a thesis that clearly states the two subjects that are to be compared, contrasted, or both and the reason for doing so. The thesis could lean more toward comparing, contrasting, or both. Remember, the point of comparing and contrasting is to provide useful ...
This page titled 3.9: Comparing and Contrasting Arguments is shared under a CC BY-NC 4.0 license and was authored, remixed, and/or curated by Saramanda Swigart. To compare two different arguments, we can start by summarizing each and then use common phrases to highlight key similarities and differences.
How do I compare and contrast theories and ideas in my literature review or research paper? Last Updated: Mar 29, 2022 Views: 28223. ... See the links below to access the tutorial and to get additional explanation of how to compare and contrast ideas, topics, and resources. Links & Files. Writing Help Guide - The Literature Review;
Compare and Contrast Papers. The compare and contrast writing prompt requires you to compare two things that are similar yet different. By the end of the paper, you must reach a conclusion that explains the connections or differences you see between the two subjects. If you write about the Civil War, your thesis might read, "While the North and ...
1. Begin by Brainstorming With a Venn Diagram. The best compare and contrast essays demonstrate a high level of analysis. This means you will need to brainstorm before you begin writing. A Venn diagram is a great visual tool for brainstorming compare and contrast essay topics.
4. Outline your body paragraphs based on point-by-point comparison. This is the more common method used in the comparison and contrast essay. [6] You can write a paragraph about each characteristic of both locations, comparing the locations in the same paragraph.
Laboratory experiments have higher internal validity but lower external validity. Fixed design vs flexible design. In a fixed research design the subjects, timescale and location are set before data collection begins, while in a flexible design these aspects may develop through the data collection process.
All five approaches have in common the general process of research that begins with . a research problem and proceeds to the questions, the data, the data analysis and interpretations, and the research report. Qualitative researchers have found it helpful to see at this point an overall sketch for each of the five approaches. From these sketches
Writing in the compare-contrast text structure presents unique problems for students (Spivey, 1990, 1991), and students' ability to write compare-contrast compositions seems to be resistant to change (Englert & Thomas, 1987). Students ' difficulties in writing compare-contrast may be related to: (a) ability (Hiebert et al.,
This article reports on a corpus-based study that examined the comparison-and-contrast markers in research articles of applied linguistics. The main argument is that comparison-and-contrast in academic writing functions as a rhetorical act that realises text organisation or dialogic contraction and as a cognitive act that fulfils representational changes and knowledge creation.
Answer: The Discussion section provides an explanation and interpretation of the results or findings of your study by comparing them with the results of previous studies. The Discussion section should relate back to the introduction in two ways: 1. You should explain what light your results shed to the questions you posed in the introduction. 2.
Typically, scholarly articles do not compare and contrast two particular theories, positions, or topics. Rather, they will cover one particular study or perspective in depth. It will be up to you, as a student, to synthesize the information you have learned from your research and draw your own comparisons and conclusions.
In contrast, research shows that textbook conversations differ considerably from authentic conversations (Le Foll, 2021). Although some textbook conversations can be linguistically close to actual conversations, many include a disproportionate number of linguistic features associated with the literate end of Biber's dimension 1 (such as nouns ...
Compare-and-contrast essays can take many forms, and the subject matter can range from books to people to historical events or even abstract concepts and theories. Regardless of the topic, the key to any successful compare-and-contrast essay lies in your ability to articulate your thesis and highlight the parallels between the two subjects ...
Abstract. Attentional control over sensory processing has been linked to neural alpha oscillations and related inhibition of cerebral cortex. Despite the wide consensus on the functional relevance of alpha oscillations for attention, precise neural mechanisms of how alpha oscillations shape perception and how this top-down modulation is implemented in cortical networks remain unclear.
Here, we systematically compare ten tools used for mapping m6A from ONT DRS data. We find that most tools present a trade-off between precision and recall, and integrating results from multiple ...
Purpose: Affibodies are a class of versatile affinity proteins with a wide variety of therapeutic applications, ranging from guided contrast agents for imaging to cell-targeting therapeutics. We have identified several affibodies specific to bone morphogenetic protein-2 (BMP-2) with a range of binding affinities and demonstrated the ability to tune release rate of BMP-2 from affibody ...
Many or all of the products here are from our partners that compensate us. It's how we make money. But our editorial integrity ensures our experts' opinions aren't influenced by compensation ...
Recognizing comparison/contrast in assignments Some assignments use words—like compare, contrast, similarities, and differences—that make it easy for you to see that they are asking you to compare and/or contrast. Here are a few hypothetical examples: Compare and contrast Frye's and Bartky's accounts of oppression.
Introduction Dynamic susceptibility contrast (DSC) perfusion weighted (PW)-MRI can aid in differentiating treatment related abnormalities (TRA) from tumor progression (TP) in post-treatment glioma patients. Common methods, like the 'hot spot', or visual approach suffer from oversimplification and subjectivity. Using perfusion of the complete lesion potentially offers an objective and ...
1. Decide how much you want to invest. Some CDs have no minimum balance requirements at all. You can invest with whatever amount of money you have available.