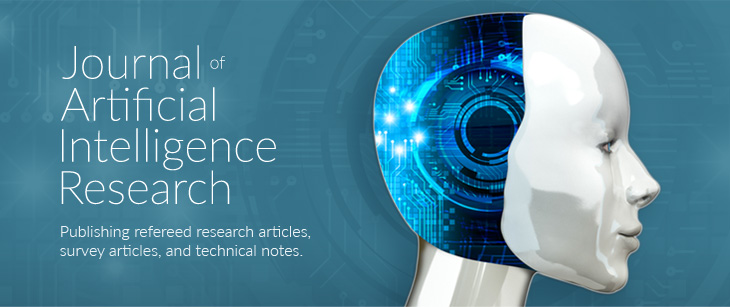
The Journal of Artificial Intelligence Research (JAIR) is dedicated to the rapid dissemination of important research results to the global artificial intelligence (AI) community. The journal’s scope encompasses all areas of AI, including agents and multi-agent systems, automated reasoning, constraint processing and search, knowledge representation, machine learning, natural language, planning and scheduling, robotics and vision, and uncertainty in AI.
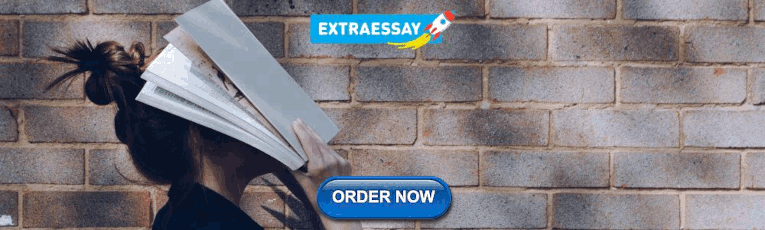
Current Issue
Vol. 79 (2024)
Published: 2024-01-10
Bt-GAN: Generating Fair Synthetic Healthdata via Bias-transforming Generative Adversarial Networks
Collision avoiding max-sum for mobile sensor teams, usn: a robust imitation learning method against diverse action noise, structure in deep reinforcement learning: a survey and open problems, a map of diverse synthetic stable matching instances, digcn: a dynamic interaction graph convolutional network based on learnable proposals for object detection, iterative train scheduling under disruption with maximum satisfiability, removing bias and incentivizing precision in peer-grading, cultural bias in explainable ai research: a systematic analysis, learning to resolve social dilemmas: a survey, a principled distributional approach to trajectory similarity measurement and its application to anomaly detection, multi-modal attentive prompt learning for few-shot emotion recognition in conversations, condense: conditional density estimation for time series anomaly detection, performative ethics from within the ivory tower: how cs practitioners uphold systems of oppression, learning logic specifications for policy guidance in pomdps: an inductive logic programming approach, multi-objective reinforcement learning based on decomposition: a taxonomy and framework, can fairness be automated guidelines and opportunities for fairness-aware automl, practical and parallelizable algorithms for non-monotone submodular maximization with size constraint, exploring the tradeoff between system profit and income equality among ride-hailing drivers, on mitigating the utility-loss in differentially private learning: a new perspective by a geometrically inspired kernel approach, an algorithm with improved complexity for pebble motion/multi-agent path finding on trees, weighted, circular and semi-algebraic proofs, reinforcement learning for generative ai: state of the art, opportunities and open research challenges, human-in-the-loop reinforcement learning: a survey and position on requirements, challenges, and opportunities, boolean observation games, detecting change intervals with isolation distributional kernel, query-driven qualitative constraint acquisition, visually grounded language learning: a review of language games, datasets, tasks, and models, right place, right time: proactive multi-robot task allocation under spatiotemporal uncertainty, principles and their computational consequences for argumentation frameworks with collective attacks, the ai race: why current neural network-based architectures are a poor basis for artificial general intelligence, undesirable biases in nlp: addressing challenges of measurement.
Thank you for visiting nature.com. You are using a browser version with limited support for CSS. To obtain the best experience, we recommend you use a more up to date browser (or turn off compatibility mode in Internet Explorer). In the meantime, to ensure continued support, we are displaying the site without styles and JavaScript.
- View all journals
- Explore content
- About the journal
- Publish with us
- Sign up for alerts
- NATURE INDEX
- 12 October 2022
Growth in AI and robotics research accelerates
It may not be unusual for burgeoning areas of science, especially those related to rapid technological changes in society, to take off quickly, but even by these standards the rise of artificial intelligence (AI) has been impressive. Together with robotics, AI is representing an increasingly significant portion of research volume at various levels, as these charts show.
Across the field
The number of AI and robotics papers published in the 82 high-quality science journals in the Nature Index (Count) has been rising year-on-year — so rapidly that it resembles an exponential growth curve. A similar increase is also happening more generally in journals and proceedings not included in the Nature Index, as is shown by data from the Dimensions database of research publications.
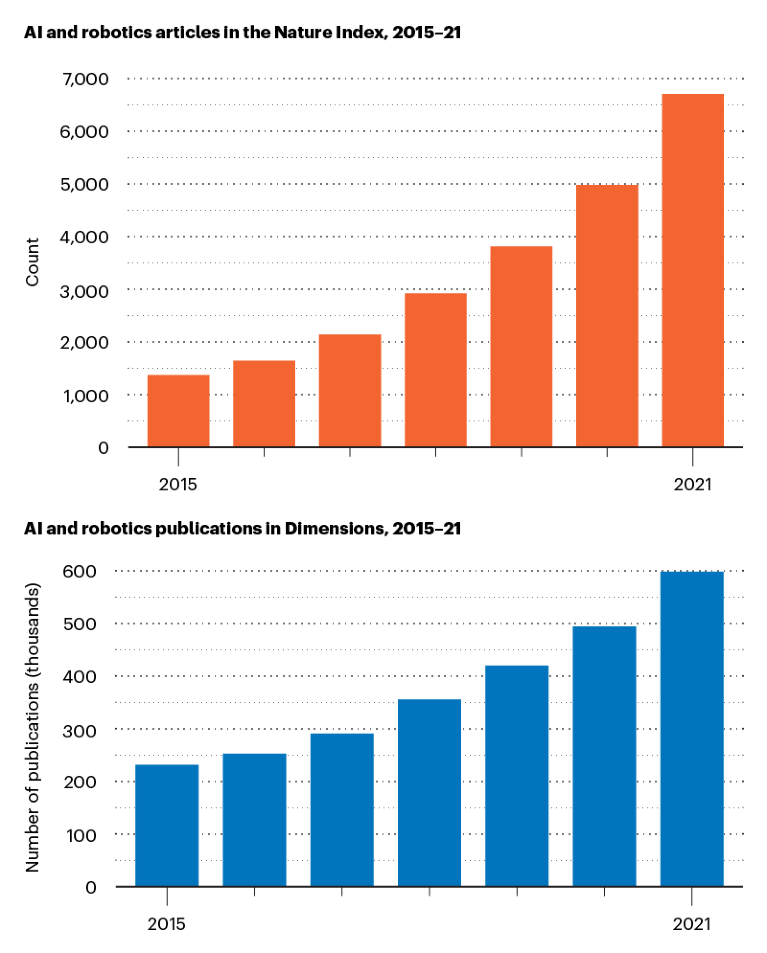
Source: Nature Index, Dimensions. Data analysis by Catherine Cheung; infographic by Simon Baker, Tanner Maxwell and Benjamin Plackett
Leading countries
Five countries — the United States, China, the United Kingdom, Germany and France — had the highest AI and robotics Share in the Nature Index from 2015 to 2021, with the United States leading the pack. China has seen the largest percentage change (1,174%) in annual Share over the period among the five nations.
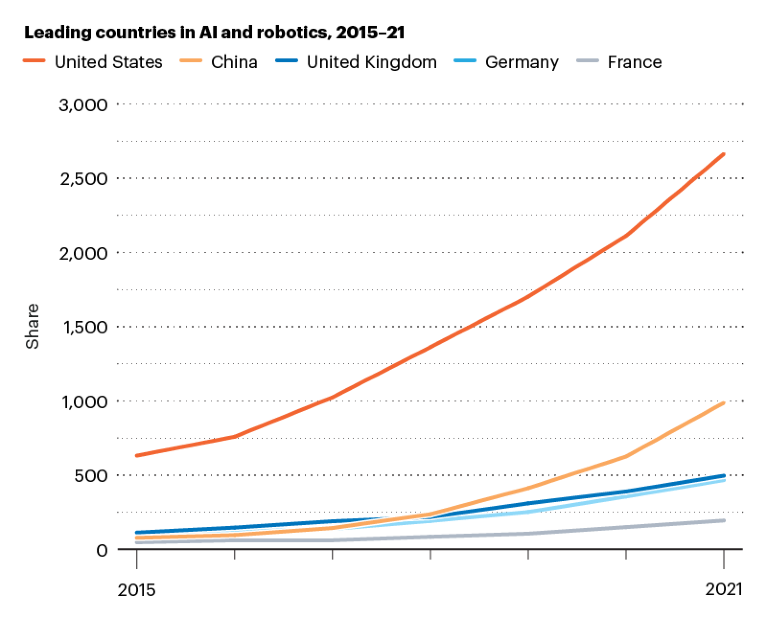
AI and robotics infiltration
As the field of AI and robotics research grows in its own right, leading institutions such as Harvard University in the United States have increased their Share in this area since 2015. But such leading institutions have also seen an expansion in the proportion of their overall index Share represented by research in AI and robotics. One possible explanation for this is that AI and robotics is expanding into other fields, creating interdisciplinary AI and robotics research.
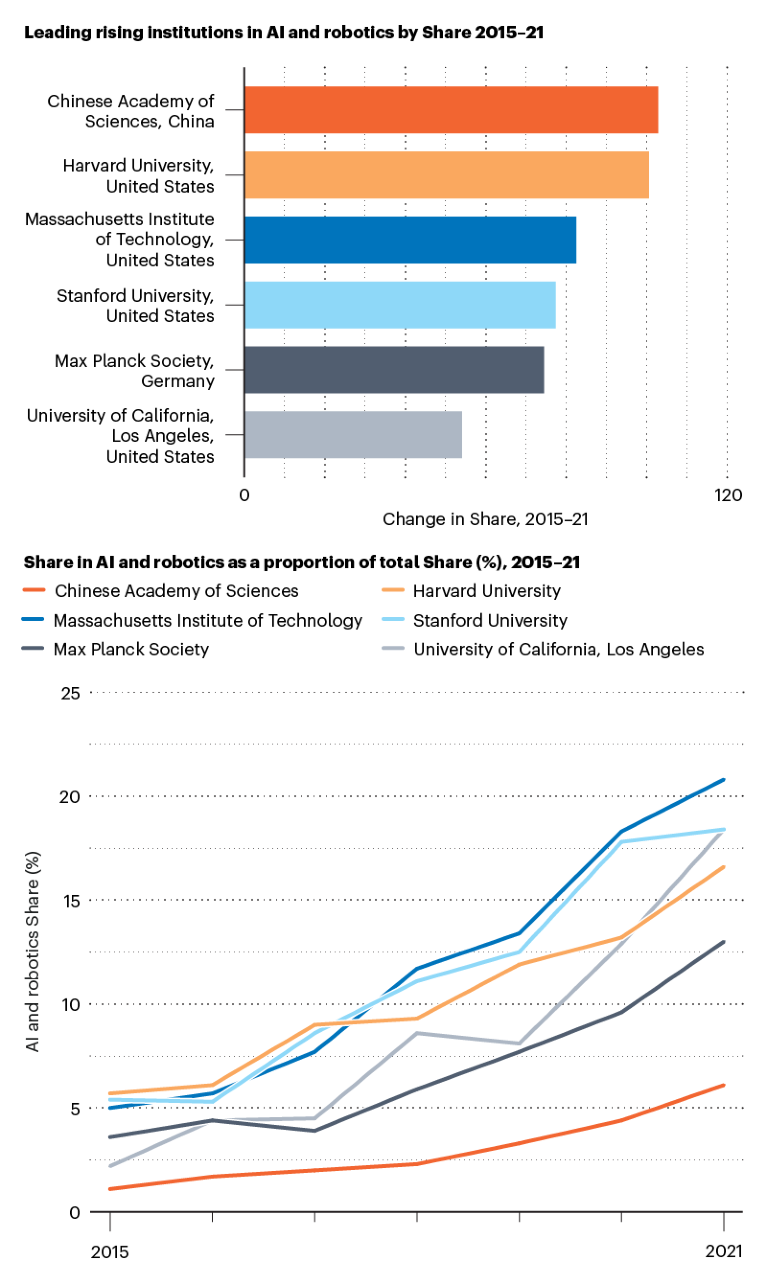
Nature 610 , S9 (2022)
doi: https://doi.org/10.1038/d41586-022-03210-9
This article is part of Nature Index 2022 AI and robotics , an editorially independent supplement. Advertisers have no influence over the content.
Related Articles
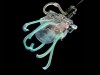
Partner content: AI helps computers to see and hear more efficiently
Partner content: Canada's welcoming artificial intelligence research ecosystem
Partner content: TINY robots inspired by insects
Partner content: Pioneering a new era of drug development
Partner content: New tool promises smarter approach to big data and AI
Partner content: Intelligent robots offer service with a smile
Partner content: Hong Kong’s next era fuelled by innovation
Partner content: Getting a grip on mass-produced artificial muscles with control engineering tools
Partner content: A blueprint for AI-powered smart speech technology
Partner content: All in the mind’s AI
Partner content: How artificial intelligence could turn thoughts into actions
Partner content: AI-powered start-up puts protein discovery on the fast track
Partner content: Intelligent tech takes on drone safety
- Computer science
- Mathematics and computing
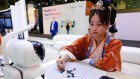
AI now beats humans at basic tasks — new benchmarks are needed, says major report
News 15 APR 24
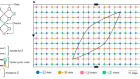
High-threshold and low-overhead fault-tolerant quantum memory
Article 27 MAR 24
Three reasons why AI doesn’t model human language
Correspondence 19 MAR 24
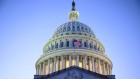
The US Congress is taking on AI —this computer scientist is helping
News Q&A 09 MAY 24
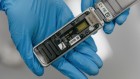
Powerful ‘nanopore’ DNA sequencing method tackles proteins too
Technology Feature 08 MAY 24
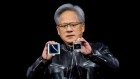
Who’s making chips for AI? Chinese manufacturers lag behind US tech giants
News 03 MAY 24
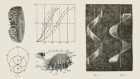
The dream of electronic newspapers becomes a reality — in 1974
News & Views 07 MAY 24
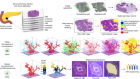
3D genomic mapping reveals multifocality of human pancreatic precancers
Article 01 MAY 24
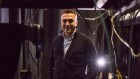
AI’s keen diagnostic eye
Outlook 18 APR 24
Staff Scientist
A Staff Scientist position is available in the laboratory of Drs. Elliot and Glassberg to study translational aspects of lung injury, repair and fibro
Maywood, Illinois
Loyola University Chicago - Department of Medicine
W3-Professorship (with tenure) in Inorganic Chemistry
The Institute of Inorganic Chemistry in the Faculty of Mathematics and Natural Sciences at the University of Bonn invites applications for a W3-Pro...
53113, Zentrum (DE)
Rheinische Friedrich-Wilhelms-Universität
Principal Investigator Positions at the Chinese Institutes for Medical Research, Beijing
Studies of mechanisms of human diseases, drug discovery, biomedical engineering, public health and relevant interdisciplinary fields.
Beijing, China
The Chinese Institutes for Medical Research (CIMR), Beijing
Research Associate - Neural Development Disorders
Houston, Texas (US)
Baylor College of Medicine (BCM)
Staff Scientist - Mitochondria and Surgery
Sign up for the Nature Briefing newsletter — what matters in science, free to your inbox daily.
Quick links
- Explore articles by subject
- Guide to authors
- Editorial policies
THE AI INDEX REPORT
Measuring trends in AI
ai iNDEX anNUAL rEPORT
Welcome to the 2024 AI Index Report
Welcome to the seventh edition of the AI Index report. The 2024 Index is our most comprehensive to date and arrives at an important moment when AI’s influence on society has never been more pronounced. This year, we have broadened our scope to more extensively cover essential trends such as technical advancements in AI, public perceptions of the technology, and the geopolitical dynamics surrounding its development. Featuring more original data than ever before, this edition introduces new estimates on AI training costs, detailed analyses of the responsible AI landscape, and an entirely new chapter dedicated to AI’s impact on science and medicine. The AI Index report tracks, collates, distills, and visualizes data related to artificial intelligence (AI). Our mission is to provide unbiased, rigorously vetted, broadly sourced data in order for policymakers, researchers, executives, journalists, and the general public to develop a more thorough and nuanced understanding of the complex field of AI.
TOP TAKEAWAYS
1. a i beats humans on some tasks, but not on all..
AI has surpassed human performance on several benchmarks, including some in image classification, visual reasoning, and English understanding. Yet it trails behind on more complex tasks like competition-level mathematics, visual commonsense reasoning and planning.
2. Industry continues to dominate frontier AI research .
In 2023, industry produced 51 notable machine learning models, while academia contributed only 15. There were also 21 notable models resulting from industry-academia collaborations in 2023, a new high.
3. Frontier models get way more expensive .
According to AI Index estimates, the training costs of state-of-the-art AI models have reached unprecedented levels. For example, OpenAI’s GPT-4 used an estimated $78 million worth of compute to train, while Google’s Gemini Ultra cost $191 million for compute.
- 4. The United States leads China, the EU, and the U.K. as the leading source of top AI models.
In 2023, 61 notable AI models originated from U.S.-based institutions, far outpacing the European Union’s 21 and China’s 15.
5. Robust and standardized evaluations for LLM responsibility are seriously lacking.
New research from the AI Index reveals a significant lack of standardization in responsible AI reporting. Leading developers, including OpenAI, Google, and Anthropic, primarily test their models against different responsible AI benchmarks. This practice complicates efforts to systematically compare the risks and limitations of top AI models.
6. Generative AI investment skyrockets.
Despite a decline in overall AI private investment last year, funding for generative AI surged, nearly octupling from 2022 to reach $25.2 billion. Major players in the generative AI space, including OpenAI, Anthropic, Hugging Face, and Inflection, reported substantial fundraising rounds.
7. The data is in: AI makes workers more productive and leads to higher quality work.
In 2023, several studies assessed AI’s impact on labor, suggesting that AI enables workers to complete tasks more quickly and to improve the quality of their output. These studies also demonstrated AI’s potential to bridge the skill gap between low- and high-skilled workers. Still other studies caution that using AI without proper oversight can lead to diminished performance.
8. Scientific progress accelerates even further, thanks to AI.
In 2022, AI began to advance scientific discovery. 2023, however, saw the launch of even more significant science-related AI applications—from AlphaDev, which makes algorithmic sorting more efficient, to GNoME, which facilitates the process of materials discovery.
9. The number of AI regulations in the United States sharply increases.
The number of AI-related regulations in the U.S. has risen significantly in the past year and over the last five years. In 2023, there were 25 AI-related regulations, up from just one in 2016. Last year alone, the total number of AI-related regulations grew by 56.3%.
10. People across the globe are more cognizant of AI’s potential impact—and more nervous.
A survey from Ipsos shows that, over the last year, the proportion of those who think AI will dramatically affect their lives in the next three to five years has increased from 60% to 66%. Moreover, 52% express nervousness toward AI products and services, marking a 13 percentage point rise from 2022. In America, Pew data suggests that 52% of Americans report feeling more concerned than excited about AI, rising from 38% in 2022.
Chapter 1: Research and Development
This chapter studies trends in AI research and development. It begins by examining trends in AI publications and patents, and then examines trends in notable AI systems and foundation models. It concludes by analyzing AI conference attendance and open-source AI software projects.
- 1. Industry continues to dominate frontier AI research.
- 2. More foundation models and more open foundation models.
- 3. Frontier models get way more expensive.
- 5. The number of AI patents skyrockets.
- 6. China dominates AI patents.
- 7. Open-source AI research explodes.
- 8. The number of AI publications continues to rise.
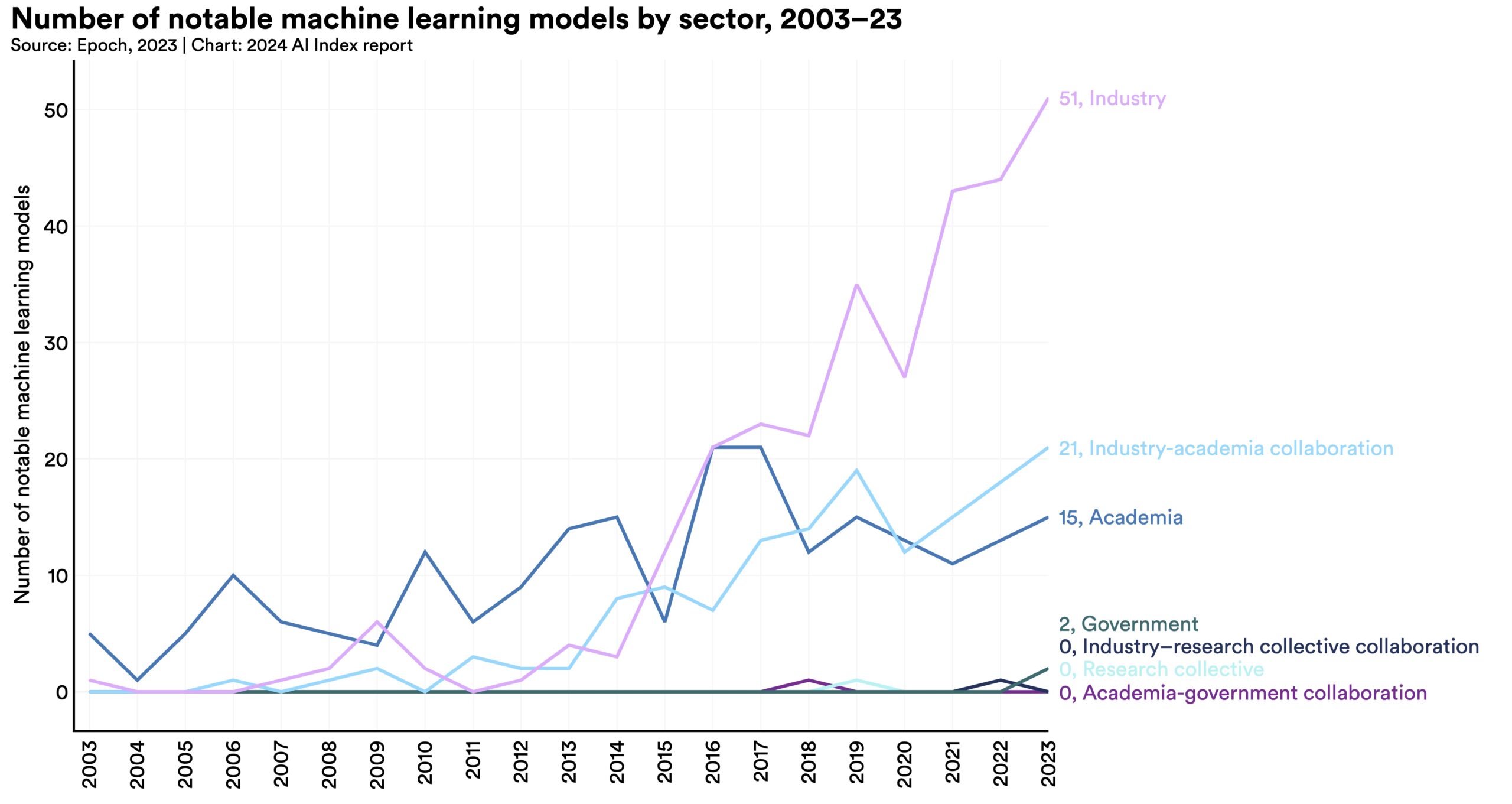
Chapter 2: Technical Performance
The technical performance section of this year’s AI Index offers a comprehensive overview of AI advancements in 2023. It starts with a high-level overview of AI technical performance, tracing its broad evolution over time. The chapter then examines the current state of a wide range of AI capabilities, including language processing, coding, computer vision (image and video analysis), reasoning, audio processing, autonomous agents, robotics, and reinforcement learning. It also shines a spotlight on notable AI research breakthroughs from the past year, exploring methods for improving LLMs through prompting, optimization, and fine-tuning, and wraps up with an exploration of AI systems’ environmental footprint.
- 1. AI beats humans on some tasks, but not on all.
- 2. Here comes multimodal AI.
- 3. Harder benchmarks emerge.
- 4. Better AI means better data which means … even better AI.
- 5. Human evaluation is in.
- 6. Thanks to LLMs, robots have become more flexible.
- 7. More technical research in agentic AI.
- 8. Closed LLMs significantly outperform open ones.
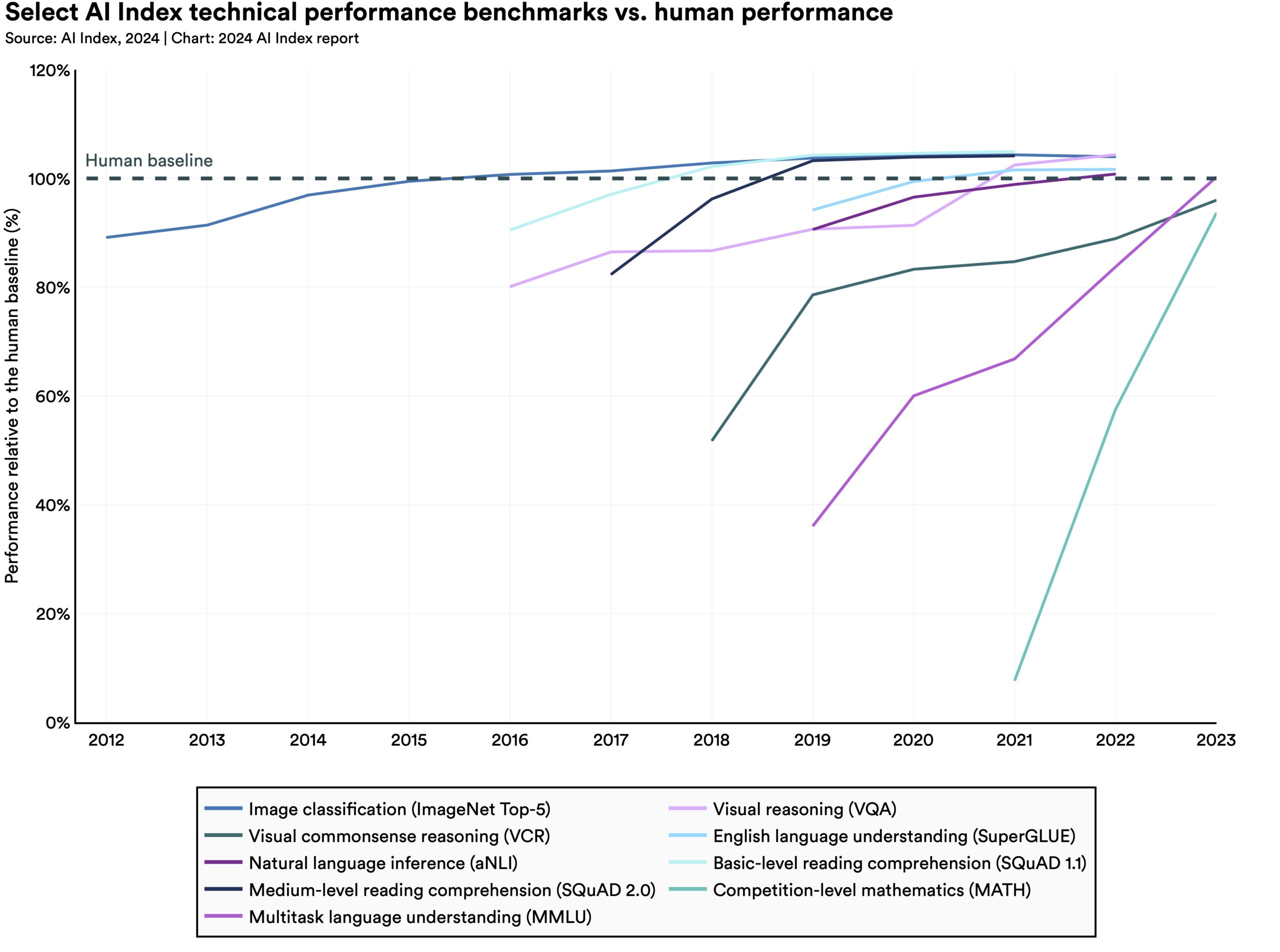
Chapter 3: Responsible AI
AI is increasingly woven into nearly every facet of our lives. This integration is occurring in sectors such as education, finance, and healthcare, where critical decisions are often based on algorithmic insights. This trend promises to bring many advantages; however, it also introduces potential risks. Consequently, in the past year, there has been a significant focus on the responsible development and deployment of AI systems. The AI community has also become more concerned with assessing the impact of AI systems and mitigating risks for those affected. This chapter explores key trends in responsible AI by examining metrics, research, and benchmarks in four key responsible AI areas: privacy and data governance, transparency and explainability, security and safety, and fairness. Given that 4 billion people are expected to vote globally in 2024, this chapter also features a special section on AI and elections and more broadly explores the potential impact of AI on political processes.
- 1. Robust and standardized evaluations for LLM responsibility are seriously lacking.
- 2. Political deepfakes are easy to generate and difficult to detect.
- 3. Researchers discover more complex vulnerabilities in LLMs.
- 4. Risks from AI are a concern for businesses across the globe.
- 5. LLMs can output copyrighted material.
- 6. AI developers score low on transparency, with consequences for research.
- 7. Extreme AI risks are difficult to analyze.
- 8. The number of AI incidents continues to rise.
- 9. ChatGPT is politically biased.
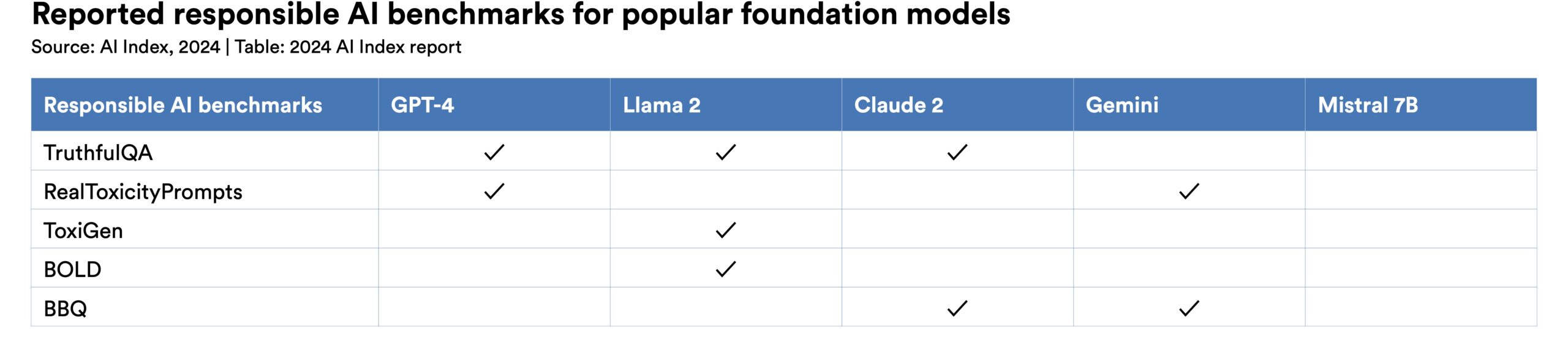
Chapter 4: Economy
The integration of AI into the economy raises many compelling questions. Some predict that AI will drive productivity improvements, but the extent of its impact remains uncertain. A major concern is the potential for massive labor displacement—to what degree will jobs be automated versus augmented by AI? Companies are already utilizing AI in various ways across industries, but some regions of the world are witnessing greater investment inflows into this transformative technology. Moreover, investor interest appears to be gravitating toward specific AI subfields like natural language processing and data management. This chapter examines AI-related economic trends using data from Lightcast, LinkedIn, Quid, McKinsey, Stack Overflow, and the International Federation of Robotics (IFR). It begins by analyzing AI-related occupations, covering labor demand, hiring trends, skill penetration, and talent availability. The chapter then explores corporate investment in AI, introducing a new section focused specifically on generative AI. It further examines corporate adoption of AI, assessing current usage and how developers adopt these technologies. Finally, it assesses AI’s current and projected economic impact and robot installations across various sectors.
- 1. Generative AI investment skyrockets.
- 2. Already a leader, the United States pulls even further ahead in AI private investment.
- 3. Fewer AI jobs, in the United States and across the globe.
- 4. AI decreases costs and increases revenues.
- 5. Total AI private investment declines again, while the number of newly funded AI companies increases.
- 6. AI organizational adoption ticks up.
- 7. China dominates industrial robotics.
- 8. Greater diversity in robot installations.
- 9. The data is in: AI makes workers more productive and leads to higher quality work.
- 10. Fortune 500 companies start talking a lot about AI, especially generative AI.
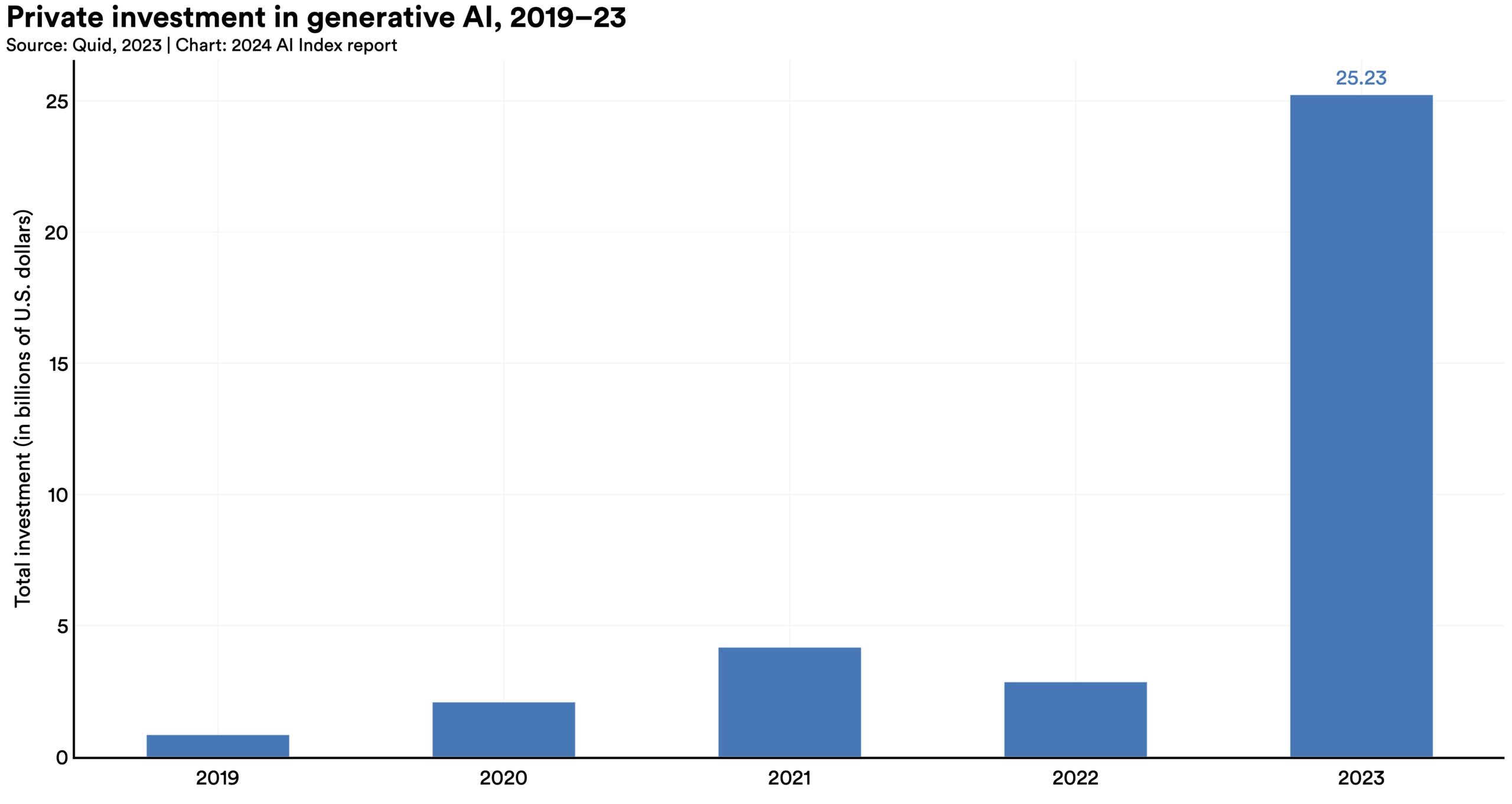
Chapter 5: Science and Medicine
This year’s AI Index introduces a new chapter on AI in science and medicine in recognition of AI’s growing role in scientific and medical discovery. It explores 2023’s standout AI-facilitated scientific achievements, including advanced weather forecasting systems like GraphCast and improved material discovery algorithms like GNoME. The chapter also examines medical AI system performance, important 2023 AI-driven medical innovations like SynthSR and ImmunoSEIRA, and trends in the approval of FDA AI-related medical devices.
- 1. Scientific progress accelerates even further, thanks to AI.
- 2. AI helps medicine take significant strides forward.
- 3. Highly knowledgeable medical AI has arrived.
- 4. The FDA approves more and more AI-related medical devices.
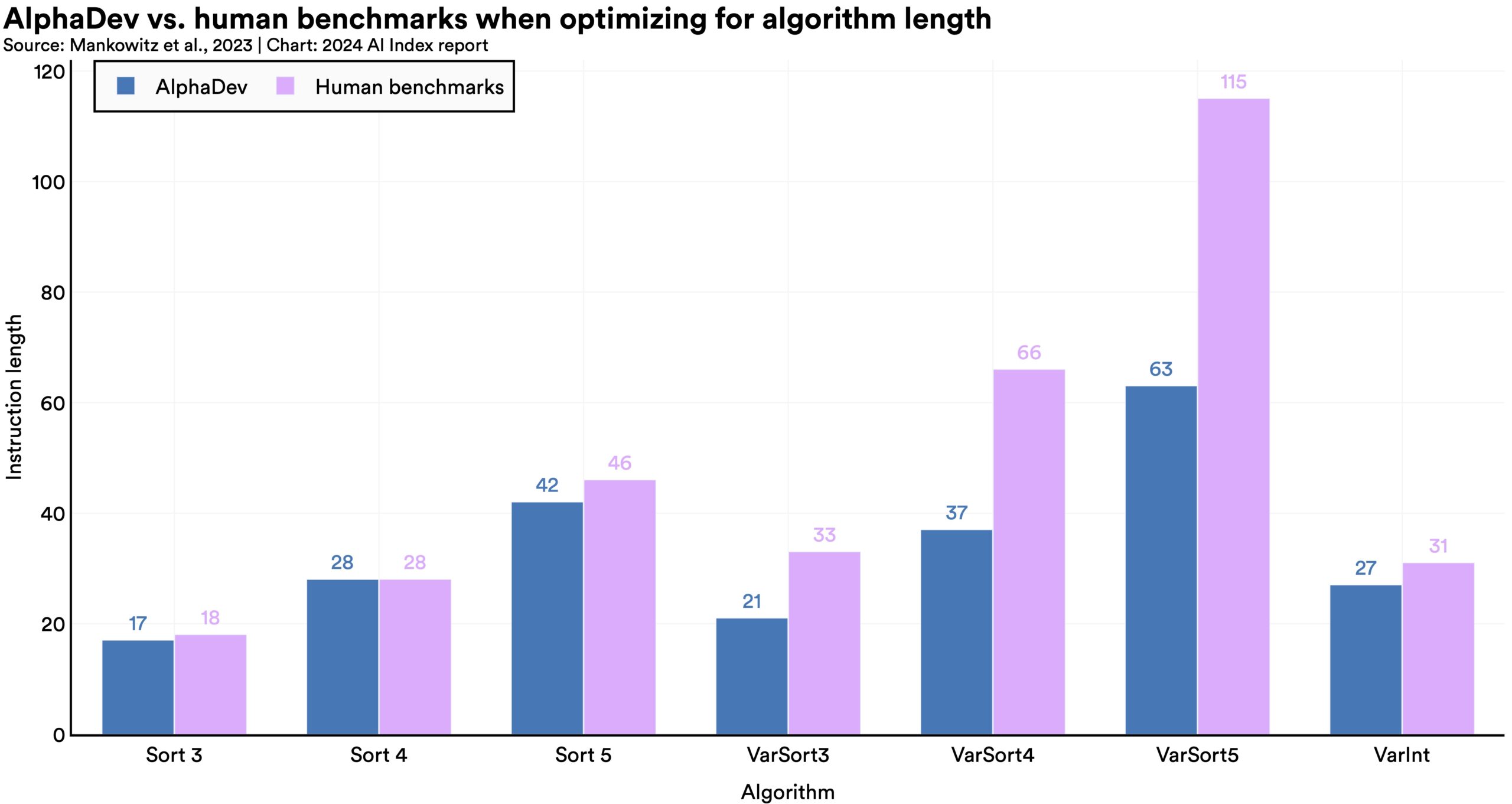
Chapter 6: Education
This chapter examines trends in AI and computer science (CS) education, focusing on who is learning, where they are learning, and how these trends have evolved over time. Amid growing concerns about AI’s impact on education, it also investigates the use of new AI tools like ChatGPT by teachers and students. The analysis begins with an overview of the state of postsecondary CS and AI education in the United States and Canada, based on the Computing Research Association’s annual Taulbee Survey. It then reviews data from Informatics Europe regarding CS education in Europe. This year introduces a new section with data from Studyportals on the global count of AI-related English-language study programs. The chapter wraps up with insights into K–12 CS education in the United States from Code.org and findings from the Walton Foundation survey on ChatGPT’s use in schools.
- 1. The number of American and Canadian CS bachelor’s graduates continues to rise, new CS master’s graduates stay relatively flat, and PhD graduates modestly grow.
- 2. The migration of AI PhDs to industry continues at an accelerating pace.
- 3. Less transition of academic talent from industry to academia.
- 4. CS education in the United States and Canada becomes less international.
- 5. More American high school students take CS courses, but access problems remain.
- 6. AI-related degree programs are on the rise internationally.
- 7. The United Kingdom and Germany lead in European informatics, CS, CE, and IT graduate production.
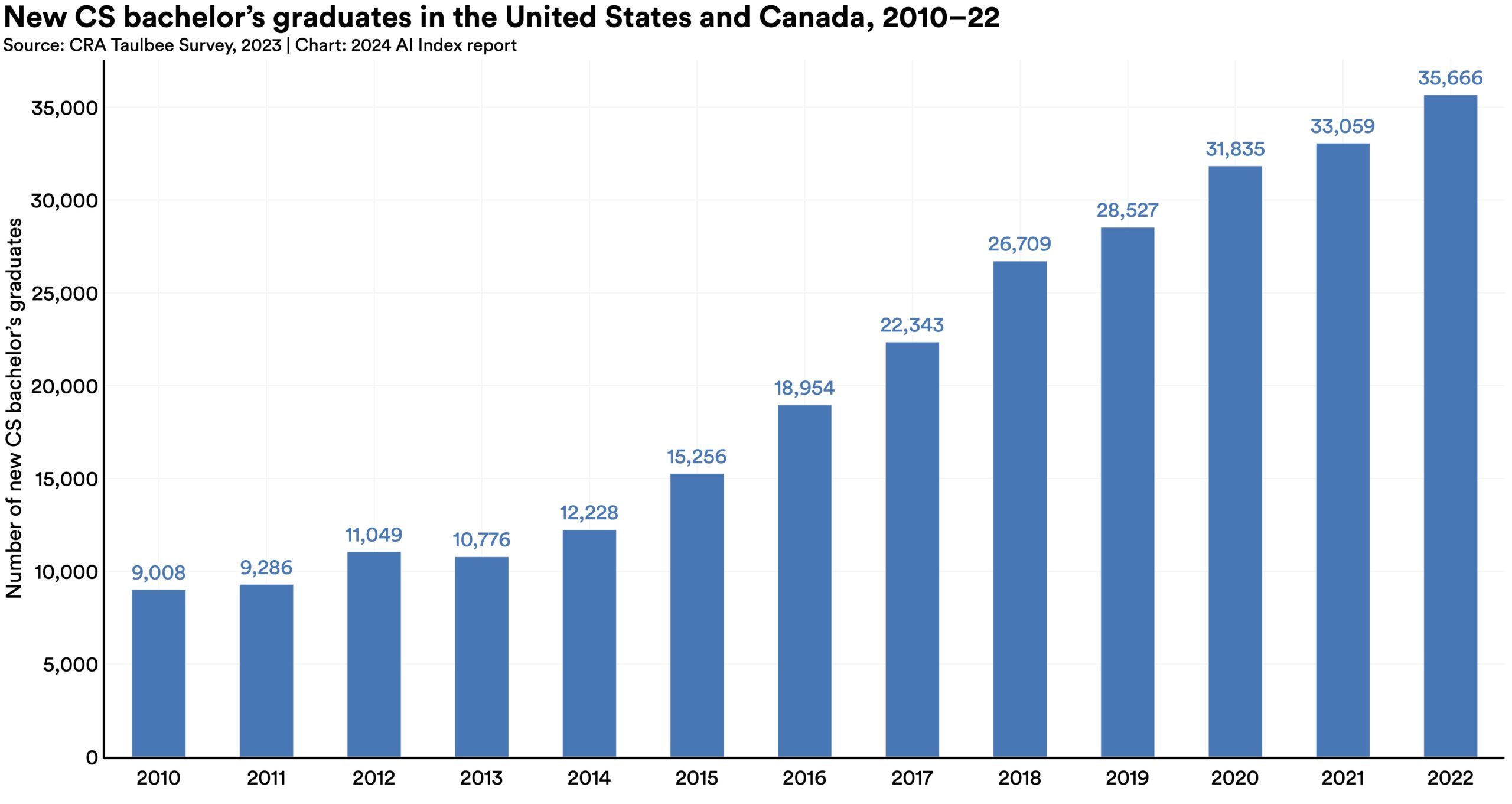
Chapter 7: Policy and Governance
AI’s increasing capabilities have captured policymakers’ attention. Over the past year, several nations and political bodies, such as the United States and the European Union, have enacted significant AI-related policies. The proliferation of these policies reflect policymakers’ growing awareness of the need to regulate AI and improve their respective countries’ ability to capitalize on its transformative potential. This chapter begins examining global AI governance starting with a timeline of significant AI policymaking events in 2023. It then analyzes global and U.S. AI legislative efforts, studies AI legislative mentions, and explores how lawmakers across the globe perceive and discuss AI. Next, the chapter profiles national AI strategies and regulatory efforts in the United States and the European Union. Finally, it concludes with a study of public investment in AI within the United States.
- 1. The number of AI regulations in the United States sharply increases.
- 2. The United States and the European Union advance landmark AI policy action.
- 3. AI captures U.S. policymaker attention.
- 4. Policymakers across the globe cannot stop talking about AI.
- 5. More regulatory agencies turn their attention toward AI.
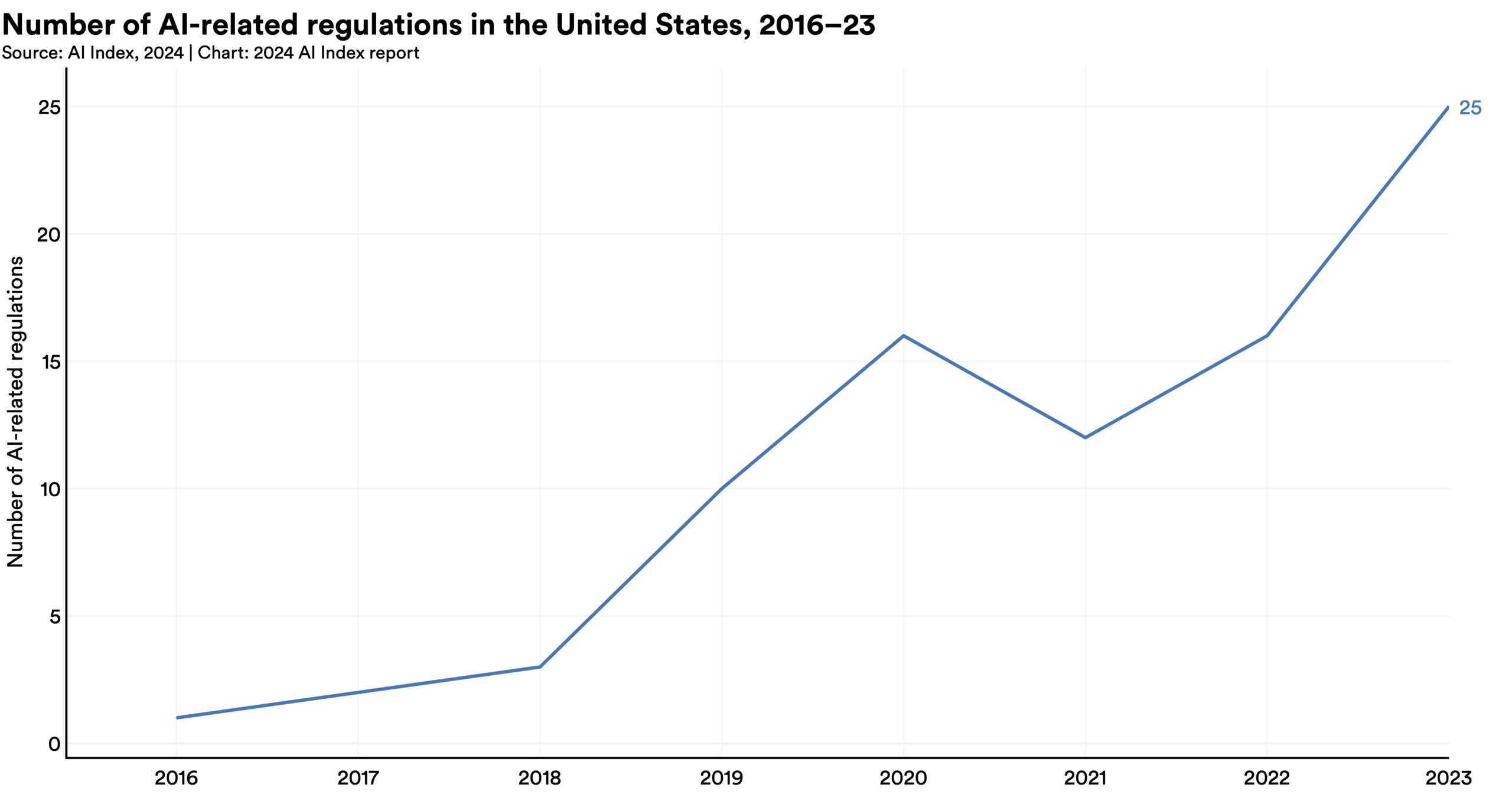
Chapter 8: Diversity
The demographics of AI developers often differ from those of users. For instance, a considerable number of prominent AI companies and the datasets utilized for model training originate from Western nations, thereby reflecting Western perspectives. The lack of diversity can perpetuate or even exacerbate societal inequalities and biases. This chapter delves into diversity trends in AI. The chapter begins by drawing on data from the Computing Research Association (CRA) to provide insights into the state of diversity in American and Canadian computer science (CS) departments. A notable addition to this year’s analysis is data sourced from Informatics Europe, which sheds light on diversity trends within European CS education. Next, the chapter examines participation rates at the Women in Machine Learning (WiML) workshop held annually at NeurIPS. Finally, the chapter analyzes data from Code.org, offering insights into the current state of diversity in secondary CS education across the United States. The AI Index is dedicated to enhancing the coverage of data shared in this chapter. Demographic data regarding AI trends, particularly in areas such as sexual orientation, remains scarce. The AI Index urges other stakeholders in the AI domain to intensify their endeavors to track diversity trends associated with AI and hopes to comprehensively cover such trends in future reports.
- 1. U.S. and Canadian bachelor’s, master’s, and PhD CS students continue to grow more ethnically diverse.
- 2. Substantial gender gaps persist in European informatics, CS, CE, and IT graduates at all educational levels.
- 3. U.S. K–12 CS education is growing more diverse, reflecting changes in both gender and ethnic representation.
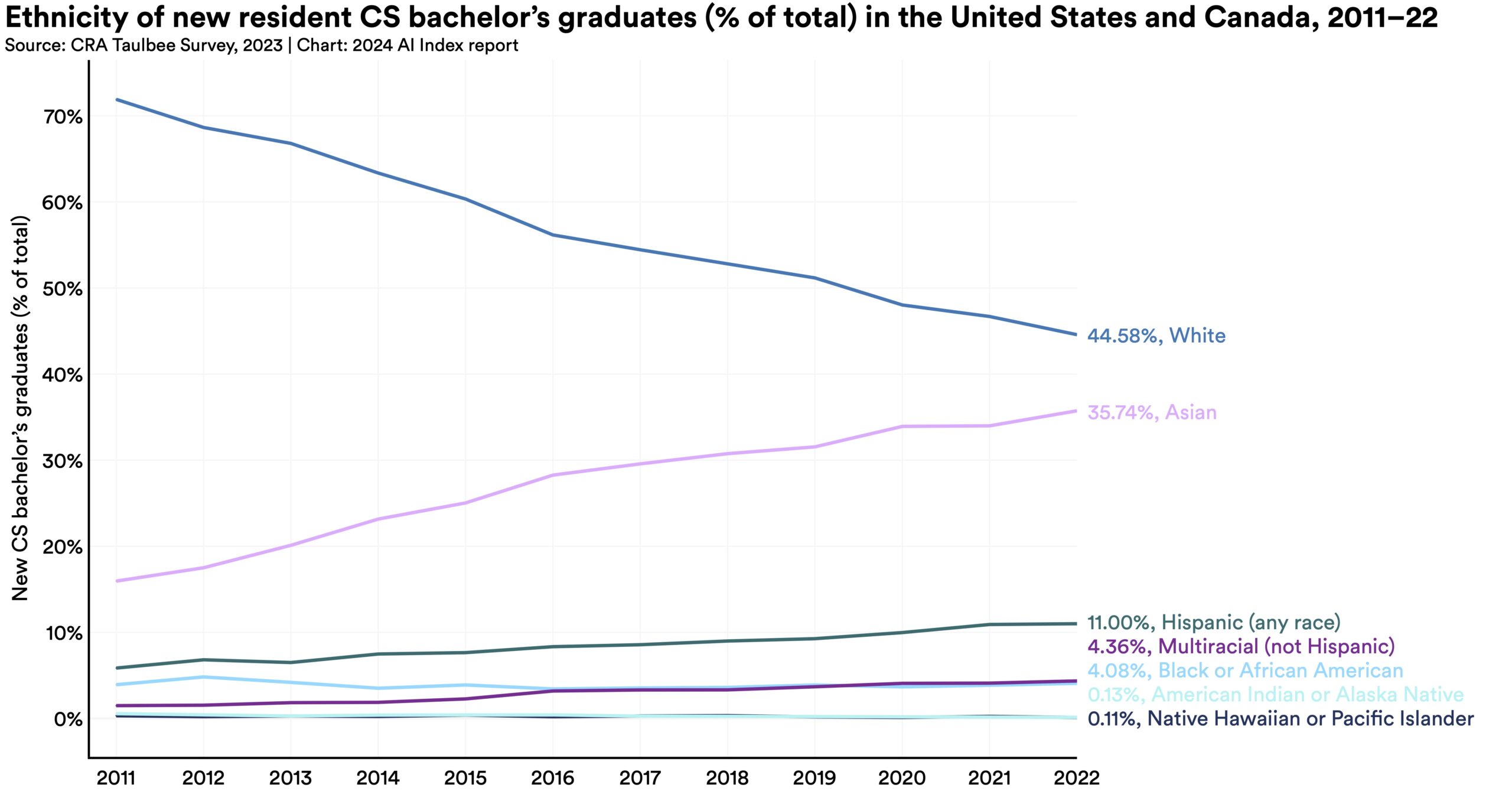
Chapter 9: Public Opinion
As AI becomes increasingly ubiquitous, it is important to understand how public perceptions regarding the technology evolve. Understanding this public opinion is vital in better anticipating AI’s societal impacts and how the integration of the technology may differ across countries and demographic groups. This chapter examines public opinion on AI through global, national, demographic, and ethnic perspectives. It draws upon several data sources: longitudinal survey data from Ipsos profiling global AI attitudes over time, survey data from the University of Toronto exploring public perception of ChatGPT, and data from Pew examining American attitudes regarding AI. The chapter concludes by analyzing mentions of significant AI models on Twitter, using data from Quid.
- 1. People across the globe are more cognizant of AI’s potential impact—and more nervous.
- 2. AI sentiment in Western nations continues to be low, but is slowly improving.
- 3. The public is pessimistic about AI’s economic impact.
- 4. Demographic differences emerge regarding AI optimism.
- 5. ChatGPT is widely known and widely used.
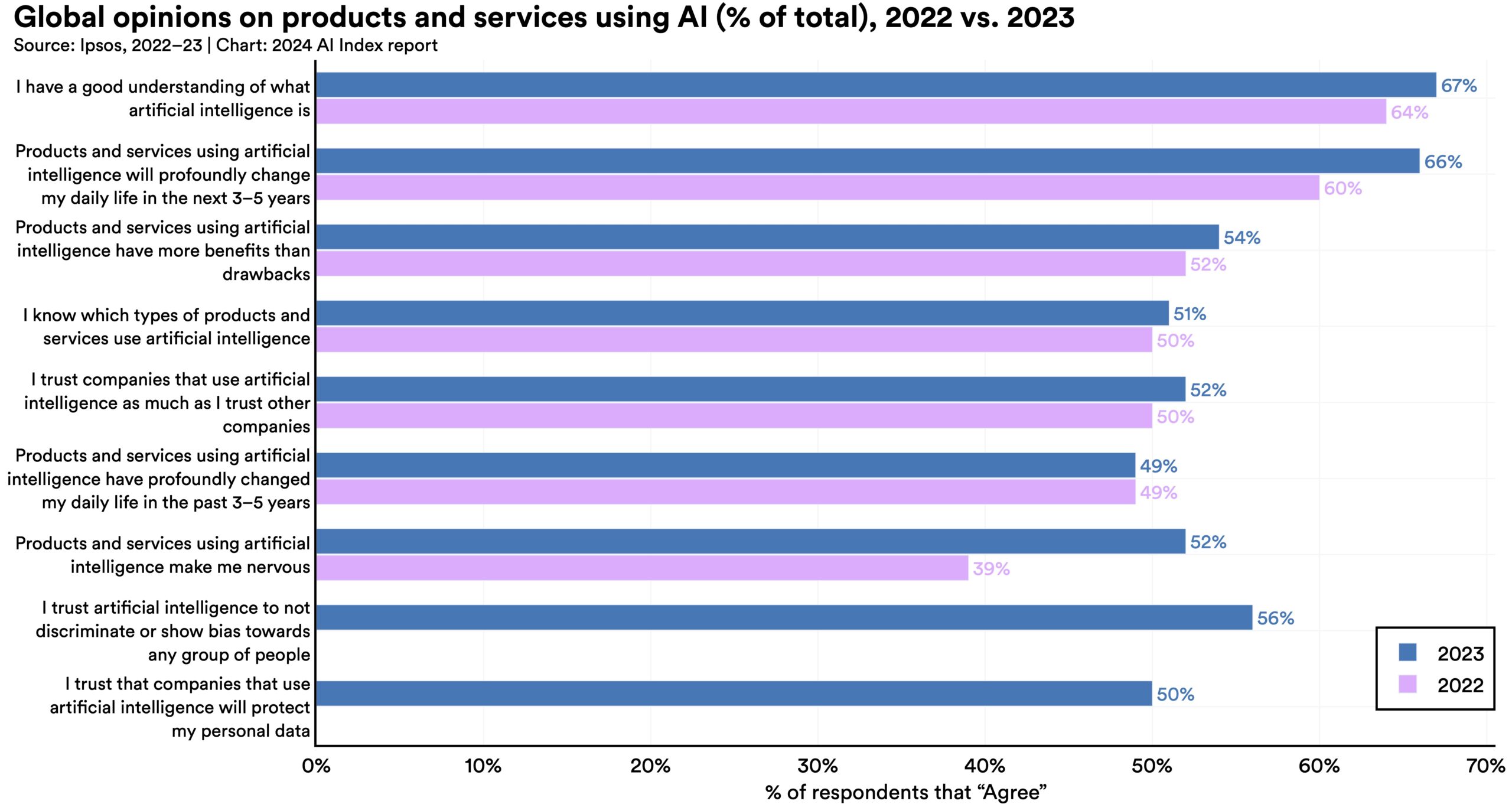
Past Reports
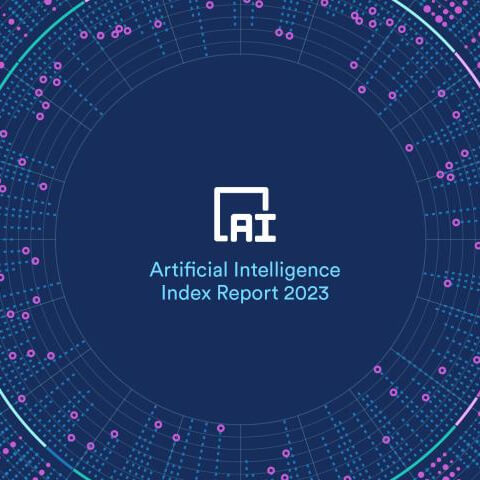
- Stanford Home
- Maps & Directions
- Search Stanford
- Emergency Info
- Terms of Use
- Non-Discrimination
- Accessibility
Artificial Intelligence in the 21st Century
Ieee account.
- Change Username/Password
- Update Address
Purchase Details
- Payment Options
- Order History
- View Purchased Documents
Profile Information
- Communications Preferences
- Profession and Education
- Technical Interests
- US & Canada: +1 800 678 4333
- Worldwide: +1 732 981 0060
- Contact & Support
- About IEEE Xplore
- Accessibility
- Terms of Use
- Nondiscrimination Policy
- Privacy & Opting Out of Cookies
A not-for-profit organization, IEEE is the world's largest technical professional organization dedicated to advancing technology for the benefit of humanity. © Copyright 2024 IEEE - All rights reserved. Use of this web site signifies your agreement to the terms and conditions.
- Search entire site
- Search for a course
- Browse study areas
Analytics and Data Science
- Data Science and Innovation
- Postgraduate Research Courses
- Business Research Programs
- Undergraduate Business Programs
- Entrepreneurship
- MBA Programs
- Postgraduate Business Programs
Communication
- Animation Production
- Business Consulting and Technology Implementation
- Digital and Social Media
- Media Arts and Production
- Media Business
- Media Practice and Industry
- Music and Sound Design
- Social and Political Sciences
- Strategic Communication
- Writing and Publishing
- Postgraduate Communication Research Degrees
Design, Architecture and Building
- Architecture
- Built Environment
- DAB Research
- Public Policy and Governance
- Secondary Education
- Education (Learning and Leadership)
- Learning Design
- Postgraduate Education Research Degrees
- Primary Education
Engineering
- Civil and Environmental
- Computer Systems and Software
- Engineering Management
- Mechanical and Mechatronic
- Systems and Operations
- Telecommunications
- Postgraduate Engineering courses
- Undergraduate Engineering courses
- Sport and Exercise
- Palliative Care
- Public Health
- Nursing (Undergraduate)
- Nursing (Postgraduate)
- Health (Postgraduate)
- Research and Honours
- Health Services Management
- Child and Family Health
- Women's and Children's Health
Health (GEM)
- Coursework Degrees
- Clinical Psychology
- Genetic Counselling
- Good Manufacturing Practice
- Physiotherapy
- Speech Pathology
- Research Degrees
Information Technology
- Business Analysis and Information Systems
- Computer Science, Data Analytics/Mining
- Games, Graphics and Multimedia
- IT Management and Leadership
- Networking and Security
- Software Development and Programming
- Systems Design and Analysis
- Web and Cloud Computing
- Postgraduate IT courses
- Postgraduate IT online courses
- Undergraduate Information Technology courses
- International Studies
- Criminology
- International Relations
- Postgraduate International Studies Research Degrees
- Sustainability and Environment
- Practical Legal Training
- Commercial and Business Law
- Juris Doctor
- Legal Studies
- Master of Laws
- Intellectual Property
- Migration Law and Practice
- Overseas Qualified Lawyers
- Postgraduate Law Programs
- Postgraduate Law Research
- Undergraduate Law Programs
- Life Sciences
- Mathematical and Physical Sciences
- Postgraduate Science Programs
- Science Research Programs
- Undergraduate Science Programs
Transdisciplinary Innovation
- Creative Intelligence and Innovation
- Diploma in Innovation
- Transdisciplinary Learning
- Postgraduate Research Degree
IJCAI 2024 Success
AAII students Zihe Liu, Wei Duan and Zhihong Deng have had papers accepted for IJCAI 2024.
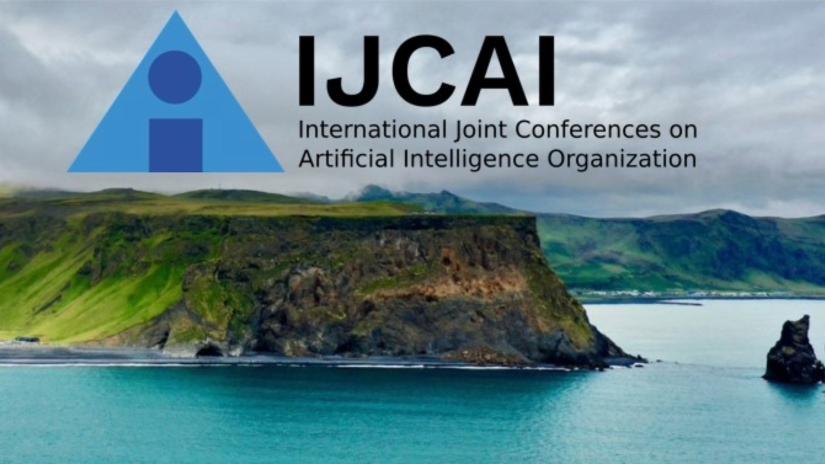
IJCAI 2024 will be held in Jeju Island, South Korea, 03 August to 09 August 2024.
IJCAI 2024: AAII Success
The International Joint Conference on Artificial Intelligence (IJCAI) is the premier international gathering of researchers in AI. The 33rd iteration of the IJCAI conference will take place in August this year in Jeju, with the following papers by AAII members accepted for presentation:
- 'A Behavior-Aware Approach for Deep Reinforcement Learning in Non-stationary Environments without Known Change Points,' Zihe Liu, Jie Lu, Guangquan Zhang & Junyu Xuan.
- ' Group-Aware Coordination Graph for Multi-Agent Reinforcement Learning ', Wei Duan, Jie Lu, & Junyu Xuan.
- ' What Hides behind Unfairness? Exploring Dynamics Fairness in Reinforcement Learning ,' Zhihong Deng, Jing Jiang, Guodong Long & Chengqi Zhang.
AAII researchers look forward to IJCAI 2024 as a chance dive deeper into their research findings and advance the next generation of AI agents that are not only intelligent, but also reliable, fair and safe.
UTS acknowledges the Gadigal people of the Eora Nation, the Boorooberongal people of the Dharug Nation, the Bidiagal people and the Gamaygal people, upon whose ancestral lands our university stands. We would also like to pay respect to the Elders both past and present, acknowledging them as the traditional custodians of knowledge for these lands.

Help | Advanced Search
Computer Science > Artificial Intelligence
Title: capabilities of gemini models in medicine.
Abstract: Excellence in a wide variety of medical applications poses considerable challenges for AI, requiring advanced reasoning, access to up-to-date medical knowledge and understanding of complex multimodal data. Gemini models, with strong general capabilities in multimodal and long-context reasoning, offer exciting possibilities in medicine. Building on these core strengths of Gemini, we introduce Med-Gemini, a family of highly capable multimodal models that are specialized in medicine with the ability to seamlessly use web search, and that can be efficiently tailored to novel modalities using custom encoders. We evaluate Med-Gemini on 14 medical benchmarks, establishing new state-of-the-art (SoTA) performance on 10 of them, and surpass the GPT-4 model family on every benchmark where a direct comparison is viable, often by a wide margin. On the popular MedQA (USMLE) benchmark, our best-performing Med-Gemini model achieves SoTA performance of 91.1% accuracy, using a novel uncertainty-guided search strategy. On 7 multimodal benchmarks including NEJM Image Challenges and MMMU (health & medicine), Med-Gemini improves over GPT-4V by an average relative margin of 44.5%. We demonstrate the effectiveness of Med-Gemini's long-context capabilities through SoTA performance on a needle-in-a-haystack retrieval task from long de-identified health records and medical video question answering, surpassing prior bespoke methods using only in-context learning. Finally, Med-Gemini's performance suggests real-world utility by surpassing human experts on tasks such as medical text summarization, alongside demonstrations of promising potential for multimodal medical dialogue, medical research and education. Taken together, our results offer compelling evidence for Med-Gemini's potential, although further rigorous evaluation will be crucial before real-world deployment in this safety-critical domain.
Submission history
Access paper:.
- HTML (experimental)
- Other Formats

References & Citations
- Google Scholar
- Semantic Scholar
BibTeX formatted citation

Bibliographic and Citation Tools
Code, data and media associated with this article, recommenders and search tools.
- Institution
arXivLabs: experimental projects with community collaborators
arXivLabs is a framework that allows collaborators to develop and share new arXiv features directly on our website.
Both individuals and organizations that work with arXivLabs have embraced and accepted our values of openness, community, excellence, and user data privacy. arXiv is committed to these values and only works with partners that adhere to them.
Have an idea for a project that will add value for arXiv's community? Learn more about arXivLabs .
This paper is in the following e-collection/theme issue:
Published on 7.5.2024 in Vol 26 (2024)
This is a member publication of National University of Singapore
Effectiveness of an Artificial Intelligence-Assisted App for Improving Eating Behaviors: Mixed Methods Evaluation
Authors of this article:

Original Paper
- Han Shi Jocelyn Chew 1 , PhD ;
- Nicholas WS Chew 2 , MBBS ;
- Shaun Seh Ern Loong 3 , MBBS ;
- Su Lin Lim 4 , PhD ;
- Wai San Wilson Tam 1 , PhD ;
- Yip Han Chin 3 , MBBS ;
- Ariana M Chao 5 , PhD ;
- Georgios K Dimitriadish 6 , MBBS, MSc ;
- Yujia Gao 7 ;
- Jimmy Bok Yan So 8 , MB ChB, FRCS, MPH ;
- Asim Shabbir 8 , MBBS, MMed, FRCS ;
- Kee Yuan Ngiam 9 , MBBS, FRCS
1 Alice Lee Centre for Nursing Studies, Yong Loo Lin School of Medicine, National University of Singapore, Singapore, Singapore
2 Department of Cardiology, National University Hospital, Singapore, Singapore
3 Yong Loo Lin School of Medicine, National University of Singapore, Singapore, Singapore
4 Department of Dietetics, National University Hospital, Singapore, Singapore
5 School of Nursing, Johns Hopkins University, Baltimore, MD, United States
6 Department of Endocrinology ASO/EASO COM, King's College Hospital NHS Foundation Trust, London, United Kingdom
7 Division of Hepatobiliary & Pancreatic Surgery, Department of Surgery, National University Hospital, Singapore, Singapore
8 Division of General Surgery (Upper Gastrointestinal Surgery), Department of Surgery, National University Hospital, Singapore, Singapore
9 Division of Thyroid & Endocrine Surgery, Department of Surgery, National University Hospital, Singapore, Singapore
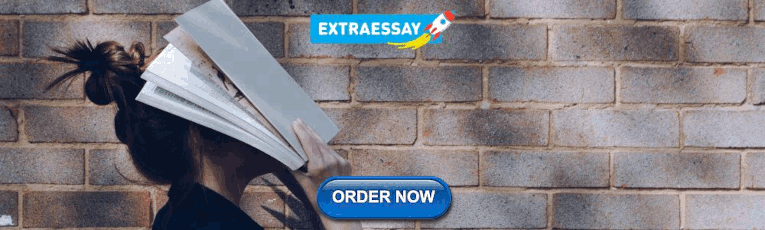
Corresponding Author:
Han Shi Jocelyn Chew, PhD
Alice Lee Centre for Nursing Studies
Yong Loo Lin School of Medicine
National University of Singapore
Level 3, Clinical Research Centre
Block MD11, 10 Medical Drive
Singapore, 117597
Phone: 65 65168687
Email: [email protected]
Background: A plethora of weight management apps are available, but many individuals, especially those living with overweight and obesity, still struggle to achieve adequate weight loss. An emerging area in weight management is the support for one’s self-regulation over momentary eating impulses.
Objective: This study aims to examine the feasibility and effectiveness of a novel artificial intelligence–assisted weight management app in improving eating behaviors in a Southeast Asian cohort.
Methods: A single-group pretest-posttest study was conducted. Participants completed the 1-week run-in period of a 12-week app-based weight management program called the Eating Trigger-Response Inhibition Program (eTRIP). This self-monitoring system was built upon 3 main components, namely, (1) chatbot-based check-ins on eating lapse triggers, (2) food-based computer vision image recognition (system built based on local food items), and (3) automated time-based nudges and meal stopwatch. At every mealtime, participants were prompted to take a picture of their food items, which were identified by a computer vision image recognition technology, thereby triggering a set of chatbot-initiated questions on eating triggers such as who the users were eating with. Paired 2-sided t tests were used to compare the differences in the psychobehavioral constructs before and after the 7-day program, including overeating habits, snacking habits, consideration of future consequences, self-regulation of eating behaviors, anxiety, depression, and physical activity. Qualitative feedback were analyzed by content analysis according to 4 steps, namely, decontextualization, recontextualization, categorization, and compilation.
Results: The mean age, self-reported BMI, and waist circumference of the participants were 31.25 (SD 9.98) years, 28.86 (SD 7.02) kg/m 2 , and 92.60 (SD 18.24) cm, respectively. There were significant improvements in all the 7 psychobehavioral constructs, except for anxiety. After adjusting for multiple comparisons, statistically significant improvements were found for overeating habits (mean –0.32, SD 1.16; P <.001), snacking habits (mean –0.22, SD 1.12; P <.002), self-regulation of eating behavior (mean 0.08, SD 0.49; P =.007), depression (mean –0.12, SD 0.74; P =.007), and physical activity (mean 1288.60, SD 3055.20 metabolic equivalent task-min/day; P <.001). Forty-one participants reported skipping at least 1 meal (ie, breakfast, lunch, or dinner), summing to 578 (67.1%) of the 862 meals skipped. Of the 230 participants, 80 (34.8%) provided textual feedback that indicated satisfactory user experience with eTRIP. Four themes emerged, namely, (1) becoming more mindful of self-monitoring, (2) personalized reminders with prompts and chatbot, (3) food logging with image recognition, and (4) engaging with a simple, easy, and appealing user interface. The attrition rate was 8.4% (21/251).
Conclusions: eTRIP is a feasible and effective weight management program to be tested in a larger population for its effectiveness and sustainability as a personalized weight management program for people with overweight and obesity.
Trial Registration: ClinicalTrials.gov NCT04833803; https://classic.clinicaltrials.gov/ct2/show/NCT04833803
Introduction
Overweight and obesity remain a public health concern that affects slightly more than half of the global adult population [ 1 ]. Across 52 Organization for Economic Co-operation and Development, Group of Twenty, and European Union 28 countries, treating conditions related to overweight and obesity costs US $425 billion per year, based on purchasing power parity. Each US dollar used to prevent obesity results in a 6-fold return in economic benefits [ 2 ]. Strategies for maintaining a healthy weight range from policy mandates on nutritional food labeling [ 3 ] to clinical treatments focused on lifestyle modifications, pharmacotherapy, and bariatric surgery [ 4 ]. However, the effectiveness of such strategies is limited by insurance coverage [ 5 ] and challenges with weight loss maintenance [ 6 - 9 ]. Some participants have been reported to regain up to 100% of their initial weight loss within 5 years [ 9 , 10 ].
With the rapid digitalization and smartphone penetration worldwide, weight loss apps have been gaining popularity, as they help overcome the temporospatial challenges of in-person weight loss programs [ 11 ]. For instance, participants enrolled in conventional weight management programs typically attend multiple face-to-face sessions at designated facilities, which could be burdensome and inconvenient as one needs to schedule appointments and travel to the facility that may be beyond one’s usual mobility pattern. Moreover, such programs are resource-intensive, requiring a multidisciplinary team of trained health care professionals (eg, physicians, dietitians, physiotherapists, nurses), infrastructure (eg, counselling room), and equipment (eg, weighing scale, stadiometer) to maintain. Well-known apps that support weight loss in the market include MyFitnessPal [ 12 ], MyPlate Calorie Tracker [ 13 ], and Fitbit [ 14 ]. In Singapore, Healthy 365 [ 15 ] is available for the public, while nBuddy [ 16 ] is used for the clinical population. These apps mostly focus on calorie tracking, health status tracking, and progress monitoring. Increasingly, apps are enhanced with features that allow intuitive synchronization of health metrics across apps to provide a more holistic progress monitoring experience. With a fee, some apps even match users to a health coach who would provide personalized weight management plans to support weight loss. However, there is a need for apps that include monitoring and support for one’s self-regulation over momentary eating impulses, which are often triggered and influenced by dietary lapse triggers such as visual food cues, eating out, negative affect, and sleep deprivation [ 17 - 20 ]. Self-regulation of eating behaviors during weight loss treatment commonly includes portion control, increasing fruit and vegetable consumption, reducing unhealthy food (sugar-sweetened beverages and high-fat food items) consumption, and reducing overall caloric consumption [ 17 ]. Therefore, we aimed to examine the feasibility and effectiveness of a novel artificial intelligence (AI)-assisted weight management app on improving eating behaviors and to explore the mechanism by which this app influences eating behaviors, as hypothesized in our earlier work [ 21 ].
Study Design
A single-group pretest-posttest study was conducted and reported according to the TREND (Transparent Reporting of Evaluations with Nonrandomized Designs) checklist ( Multimedia Appendix 1 ) [ 22 ]. Despite the limitations of the study design, it was deemed the most appropriate and feasible experimental study design for a preliminary understanding of the usability, acceptability, and effectiveness of the app [ 23 ].
Participant Recruitment
Participants older than 21 years with BMI ≥23 kg/m 2 and not undergoing a commercial weight loss program were recruited from January 2022 to October 2022 through social media platforms and physical recruitment at a local tertiary hospital’s specialist weight management clinic in Singapore. Using G*Power (version 3.1.9.7) [ 24 ], to detect a small effect size of 0.2 at .05 significance level and 80% power while accounting for an attrition rate of 20%, 248 participants are required. To be conservative, 250 participants were recruited.
Intervention
Immediately after completing the pretest questionnaire, participants were onboarded to the Eating Trigger-Response Inhibition Program (eTRIP) app by a trained research assistant to complete the 1-week run-in of the program. During the onboarding, participants were invited to enter their anthropometric details, desired weight loss goals, and motivation. They were also encouraged to personalize certain app functions such as the timing of the check-in prompts and preferred name for interaction with a chatbot. At every mealtime (at least 3 times a day), the participants were prompted to take a picture of their food, which was immediately recognized by a food-based computer vision image recognition technology, which then triggered a set of chatbot-initiated questions on eating triggers (eg, how they are feeling). These questions were developed based on our past work on eating behaviors [ 25 - 30 ]. Participants were able to view their image-based food log and eating habits on a dashboard, reflect upon their eating habits throughout the day, and set their goals and action plans for the next day. On the 8th day, all participants’ user accounts were locked, and they were unable to make any changes but were able to still view their check-in logs. Participants could also provide feedback on the app by filling out the comments section in one of the app pages. Participants were reimbursed SGD 25 (SGD 1=US $0.74) for completing this program.
The eTRIP app was developed as a 12-week AI-assisted, app-based, self-regulation program targeted at improving weight loss through healthy eating. eTRIP was developed largely based on a modified temporal self-regulation theory [ 31 , 32 ], behavioral change taxonomy [ 33 ], and our previous work on healthy eating and weight loss [ 27 - 29 , 34 , 35 ]. This includes studies on people with overweight and obesity in the areas of personal motivators, self-regulation facilitators, and barriers [ 27 ]; the potential of AI, apps, and chatbots in improving weight loss [ 6 , 25 , 29 ]; perceptions and needs of AI to increase its adoption in weight management [ 26 ]; and the essential elements of a weight loss app [ 28 ]. The development of eTRIP was split into 2 phases: (1) development of an AI-assisted self-monitoring system and (2) development of an AI-assisted behavioral nudging system. In this paper, we report the feasibility and effectiveness of an AI-assisted self-monitoring system after a 1-week run-in. The self-monitoring system is built upon 3 main components, namely, (1) chatbot-based check-ins on eating lapse triggers, (2) food-based computer vision image recognition (system built based on local food items), and (3) automated time-based nudges and meal stopwatch.
All participants completed the same self-report questionnaire before and after the 1-week run-in of the app, which reflected their sociodemographic profile, BMI, waist circumference, intention to improve eating behaviors, habits of overeating (Self-Report Habit Index) [ 36 ], habits of snacking [ 36 ], consideration of future consequences (Consideration of Future Consequences Scale-6 items) [ 37 ], self-regulation of eating behavior (Self-Regulation of Eating Behavior Questionnaire) [ 38 ], physical activity (International Physical Activity Questionnaire-Short Form) [ 39 ], anxiety symptoms (Generalized Anxiety Disorder-2 items) [ 40 ], and depressive symptoms (Patient Health Questionnaire-2 items) [ 41 ]. Details are reported in Multimedia Appendix 2 . The primary outcomes were overeating habits, snacking habits, immediate thinking, self-regulation of eating habits, depression, anxiety, and physical activity. The secondary outcomes were their subscale scores.
Data Analysis
SPSS statistical software (version 27; IBM Corp) [ 42 ] was used for the analyses. The baseline characteristics of the participants were presented in mean (SD) and frequency (%). Paired 2-sided t tests were used to compare the differences in the psychobehavioral constructs before and after the 7-day program, including overeating habits, snacking habits, consideration of future consequences, self-regulation of eating behaviors, anxiety, depression, and physical activity. To account for the increased risk of a type 1 error due to multiple comparisons [ 43 ], the Bonferroni-corrected significant level was set to P ≤.007. Qualitative feedback were analyzed using content analysis according to 4 steps, namely, decontextualization, recontextualization, categorization, and compilation [ 44 ]. Feedback was first consolidated verbatim and read iteratively by 2 coders (Nagadarshini Nicole Rajasegaran and HSJC). The verbatim feedback was then analyzed independently by 2 reviewers into meaning units. Meaning units were then reconstituted, categorized, and reported as themes and subthemes.
Ethics Approval
This single-group pretest-posttest study was approved by the National Healthcare Group Domain Specific Review Board (ref 2020/01439), registered with the ClinicalTrials.gov (ref NCT04833803) on April 6, 2021.
Baseline Characteristics of the Participants
A total of 251 participants were enrolled in this study (Chew HSJ, unpublished data, 2023); 20 (7.9%) participants dropped out of the 1-week program due to the inability to perform check-ins every day. Among those who completed the program (n=231), 1 participant was removed from the analyses due to ineligibility. The mean age, self-reported BMI, and waist circumference of the participants was 31.25 (SD 9.98) years, 28.86 (SD 7.02) kg/m 2 , and 92.6 (SD 18.24) cm, respectively ( Table 1 ). Approximately 47.8% (111/230) of the participants were males, indicating a good mix of participants from both sexes, and most of the participants were single (169/230, 73.6%), Chinese (181/230, 78.7%), and had a university education (148/230, 64.1%).
a SGD 1=US $0.74.
Mean Baseline Scores on Each Outcome Variable
The mean baseline scores on each outcome variable of the participants who completed and who dropped out from the 1-week program were calculated ( Table 2 ). As the dropout rate was only 8.4% (20/251), statistical comparisons between those who dropped out and those who completed the program was not necessary.
a SRHI: Self-Report Habit Index.
b CFCS-6: Consideration of Future Consequences Scale-6 items.
c SREBQ: Self-Regulation of Eating Behavior Questionnaire.
d IPAQ-SF: International Physical Activity Questionnaire-Short Form.
e MET: metabolic equivalent task.
Pretest and Posttest Mean Differences
There were significant improvements in all the 7 psychobehavioral constructs, except for anxiety. After adjusting for multiple comparisons, there were only statistically significant improvements in the overeating habit, snacking habit, self-regulation of eating behavior, depression, and physical activity ( Table 3 ). Forty-one participants reported skipping at least 1 meal (ie, breakfast, lunch, or dinner), summing to a total of 578 (67.1%) of the 862 meals skipped.
b Significant at P <.007.
c CFCS-6: Consideration of Future Consequences Scale-6 items.
d Consideration of Future Consequences Scale-6 immediate subscale.
e Consideration of Future Consequences Scale-6 future subscale.
f SREBQ: Self-Regulation of Eating Behavior Questionnaire.
g IPAQ-SF: International Physical Activity Questionnaire-Short Form.
User Engagement
Among those who completed the program, 97% (46,867/48,316) chatbot-based questions were completed. As participants were given the option to add additional check-ins for snacks, the percentage of completed check-ins could not be accurately computed.
Qualitative Feedback
Of the 230 participants, 80 (34.8%) provided textual feedback that indicated satisfactory experience with eTRIP. Four themes emerged, namely, (1) becoming more mindful of self-monitoring, (2) personalized reminders with prompts and chatbot, (3) food logging with image recognition, and (4) engaging with a simple, easy, and appealing user interface.
Becoming More Mindful of Self-Monitoring
By checking in with the app for every meal, the participants mentioned being more aware of their unhealthy eating habits and more mindful of their next meal. One participant said, “It (eTRIP) incentivizes me to stick to my diet plan because I am reminded of my diet plan daily. Ticking the box that indicates ‘I did not meet my diet plan’ made me guilty and it motivates me to opt for healthier food choice the next time round” (Female, Chinese, 22 years old). Another participant said, “I really liked the eTRIP app! Has a lot of potential for further expansion and use by more people. I like how it sends prompts during selected times of the day to be careful of what we see on social media. The rating of our mood before meals also helps me know how mood can affect my eating patterns. Lastly, the stopwatch function is great because it reminds me to eat more mindfully” (Male, Chinese, 27 years old).
Personalized Reminders With Prompts and Chatbot
Some participants mentioned the appreciation for reminders to check in with themselves in terms of the triggers of overeating. One participant said, “I like that there’s a reminder to check in for every meal and users get to decide what time the app should prompt!” (Female, Indian, 27 years old). Some also suggested to develop the prompting system to prompt based on the user’s previous check-in timings to optimize the prediction of mealtimes and prompt the check-in sessions intuitively. One participant suggested, “I think what would make this better is if you could aggregate the time the meals are entered from the past few days and estimate the time the user will normally eat and auto-adjust the timing…” (Female, Chinese, 23 years old). Others suggested to include reminders of how to make their meal options healthier, “Might be good to have reminders that reminds us to eat healthy with some tips on how to choose food” (Male, Chinese, 25 years old).
Food Logging With Image Recognition
Many participants highlighted their appreciation for the image recognition-based food logging, as it was accurate and convenient for food logging. One participant said, “It is very accurate in determining the food I’ve eaten just from the picture, and this saved me a lot of time from typing out the food I’ve eaten” (Male, Chinese, 21 years old).
Engaging With a Simple, Easy, and Appealing User Interface
All the participants who commented on the user experience expressed being impressed with the user interface and structure. One participant said, “the flow was smooth, quite clear. Graphics were cute. Very easy to input my info (information) especially from the homepage, I like how there’s the ability to skip a meal” (Female, Malay, 25 years old). Another participant said, “The app is very smart, … yes it’s very easy to fill and I loss (lost) like 0.5kg?” (Female, Chinese, 25 years old).
Participants’ Suggestions
In terms of the areas for improvement, the participants preferred to have (1) more options and rating scales for each domain of eating trigger instead of typing out in the “others” field (although there was a stored text for repeated entries); (2) summary of the instances where one was able to achieve the goal of the day, which the user sets daily for the next day (based on a user preset list of goals); (3) examples of standard portions and frequency of meals; and (4) feedback on how to improve upon the unhealthy meals logged.
Real-time interventions that can effectively address eating lapse triggers and improve eating behavior self-regulation, lapse events, weight loss, and weight maintenance remain unclear [ 45 ]. OnTrack, a just-in-time adaptive intervention that has been tested, is a smartphone app that uses machine learning to predict dietary lapses based on the repeated assessments of lapse triggers (ecological momentary assessment). OnTrack is used in conjunction with existing weight loss apps such as WeightWatchers app and provides personalized recommendations to prevent dietary lapses. The compliance rate for completing the lapse trigger survey in OnTrack was 62.9% over 3 months, and the studied sample was mostly females who were Whites [ 46 ]. Evidence has shown that factors influencing obesity and overweight are population-specific, influenced by socioeconomic, cultural, and genetic factors among others [ 45 , 47 ]. Singapore is a multiethnic society with a unique food culture influenced by various racial beliefs and traditions [ 48 ]. The differences in geographical, social, environmental, and genetic characteristics could define a different set of triggers and response to such weight loss apps.
Principal Findings
In this paper, we report the effectiveness of a weeklong AI-assisted weight loss app for improving overeating habits, snacking habits, immediate thinking, self-regulation of eating habits, depression, and physical activity. Interestingly, there were no significant improvements in the anxiety symptoms before and after using eTRIP, potentially due to the already low level of anxiety in those who completed the program (ie, ceiling effect). We also report corresponding qualitative user feedback on the experience with using eTRIP, where the users appreciated the app for enabling them to become more mindful of self-monitoring; personalized reminders with prompts and chatbot; food logging with image recognition; and engaging with a simple, easy, and appealing user interface. The significant improvements observed among the participants in this study reveal the potential of this app to influence weight loss in the context of a Southeast Asian cohort with overweight and obesity. The qualitative feedback also informs future app development to enhance user engagement and reduce dropout rates.
Eating habits contribute to overweight and obesity [ 49 , 50 ]. Encouraged by an obesogenic environment, overeating is commonly triggered by situational factors such as food novelty or variety, social company (eg, eating with certain people), affect emotional states (which trigger emotional eating), and distractions (eg, concurrent tasks) [ 30 , 50 - 53 ]. Other studies have suggested that people at risk for obesity exhibit hyperresponsivity in the neural reward system to calorie-dense foods, which is associated with increased food consumption [ 54 ]. Alongside users’ feedback that the app made them more mindful of their eating patterns, the significant improvement in overeating and snacking habits could have been due to an increased awareness of one’ maladaptive eating habits and subsequently, the motivation to change. This coincides with a review that reported the effectiveness of mindful eating interventions on reducing food consumption in people with overweight and obesity [ 55 ]. Our qualitative findings showed that by self-monitoring one’s eating behavior through chatbot-initiated check-ins, one could enhance mindful eating and reduce overeating without the need for undergoing mindful eating training. This could eventually lead to a reduction in total food consumption and weight loss. However, more quantitative evidence is needed to support this point.
It is noteworthy that some participants reported skipping meals as planned, which might have led to reduced energy consumption. However, this has to be examined further, as studies have shown that the calories avoided during a skipped meal may be compensated by an increase in snacking or overeating during mealtimes [ 56 ]. One additional element that can be explored in future studies is the effectiveness of promoting healthy snacking, which includes snacking on foods rich in proteins, fruits, vegetables, and whole grains, as opposed to nutrient-poor and energy-dense foods [ 57 , 58 ]. These healthier alternatives have been found not only to be associated with earlier satiety but also to be more nutritious, with their contents being more consistent with the established dietary recommendations and guidelines [ 59 , 60 ]. This strategy can be explored in conjunction with the current approach to decrease participants’ overall snacking habits.
The improvement in the self-regulation of eating habits could be attributed to several factors, including the app content focused on reminding participants of their weight loss goals and to adopt healthier eating habits of less snacking and overeating during mealtimes. In particular, in commonly stigmatized populations like those with overweight and obesity, personalization of interventions enhances one’s feeling of being taken care of, nurtured, and respected, providing them with a sense of confidence [ 61 ]. This may have improved individuals’ willingness to engage with the eTRIP content, knowing that they would be well-respected and seen as individuals through personalized chatbot conversations and reminders [ 62 ]. Other studies have shown that personalized eHealth interventions are more effective than conventional programs in enhancing weight loss maintenance, BMI, waist circumference, and various other metabolic indicators [ 63 ].
In conjunction with improvements in eating habits, participants also engaged in greater levels of physical activity by the end of this study. Increased health consciousness and self-education about the impacts and types of physical activity are factors that may explain the observed increased level of physical activity among the participants [ 64 ]. Various studies have found that a combination of diet and exercise is superior to diet-only interventions in inducing weight loss [ 65 ]. The level of physical activity is also an important factor for improving long-term weight loss [ 66 - 68 ]. Moderate amounts of physical activity were observed to prevent weight regain after weight loss [ 65 , 68 , 69 ]. In addition, the American College of Sports Medicine recommends 200-300 minutes of moderate physical activity a week to prevent similar weight regain [ 70 ]. In our study, low and moderate levels of exercise were seen to increase significantly among the participants. Although the sustainability of the increase in the exercise levels is still unknown, the preliminary data are encouraging to show the potential of the app in impacting physical activity. The amounts of high levels of exercise were, however, not impacted significantly. Additional interventions, including the provision of educational materials about the benefits of and types of exercise, along with personalized reminders for exercise can potentially further increase the success of the app in increasing moderate and high levels of exercise among its participants [ 71 , 72 ].
In addition to improvements in the eating habits and levels of physical activity, there were changes in the psychological factors among the participants. The mean depressive symptoms were significantly decreased at the end of the weeklong program. Various studies have shown that healthy living characterized by various factors such as healthy eating and sufficient levels of physical activity have the potential to positively impact psychological factors such as mood and emotions [ 73 ]. Healthy eating with adherence to dietary recommendations has been found to reduce the levels of inflammation, increase the levels of various micronutrients such as vitamins, and regulate the levels of simple sugars, all of which are protective against mental illnesses, especially depression [ 73 - 76 ]. Studies have also shown that the use of chatbots in the app may decrease depressive symptoms among some participants. Chatbots provide individuals the ability to provide self-care in an environment that is neither costly nor stigmatizing [ 77 ]. This may enable participants to be more open with their emotions, as well as to have an outlet to gain relief through their interaction with the chatbot as a proxy of human interaction [ 77 ]. Studies have shown that improvements in psychological factors such as depressive symptoms have been positively associated with weight loss and maintenance, further increasing the effectiveness of weight loss efforts among participants with overweight and obesity [ 45 ].
Strengths and Limitations
This study was the first to characterize the effectiveness of an AI-assisted weight loss app in the context of a Southeast Asian cohort. One strength of this study was the demographics of the participants, which was generally representative of the Singaporean population in terms of sex and ethnicity. The consideration of population-specific determinants of obesity and overweight during the design of the app would have also increased its applicability in this population [ 78 ], having considered the various nuances and practical needs of its target demographic. For example, the food image recognition system, which was built based on local food items, reduced the amount of time and effort required for the logging of food, improved the usability of the intervention, and enhanced user experience. The success of this app thus provides evidence that the consideration of population-specific underpinnings and practical requirements were essential toward the successful design and implementation of a weight loss intervention [ 79 ].
Although the app presents significant potential in this weeklong trial, this study is limited due to its short time frame. This presents with difficulties in understanding the midterm to long-term impacts of using the app. However, it is reassuring that despite the limited time frame of this study, the various behavioral and psychological indicators were observed to be significantly improved. Through the feedback gathered from the participants, the app may be improved in specific aspects, including (1) refining choices available for the various survey fields such as the provision of a drop-down menu for the selection of weight loss goals; (2) providing additional feedback and weekly summaries to the participants for knowledge of their progress in various aspects; and (3) providing educational materials to provide participants with the means to improve, especially for what to do after eating lapses and suggestions for healthy snacking. Another limitation was the lack of feedback quotes from older individuals as opposed to those from younger individuals. This could be due to various reasons, of which decreased media literacy among older individuals might present additional obstacles for the provision of feedback [ 80 ]. Lastly, we did not collect information on the participants’ medical and pharmacological history, where certain diseases and drugs are known to influence weight gain through various metabolic and neural pathways. Weight and waist circumference were also self-reported, and thus, data from these measures should be interpreted cautiously.
This study was the first to characterize the effectiveness of an AI-assisted weight loss app in the context of a Southeast Asian cohort. The positive findings of this study show the feasibility of implementing this app and the large potential it has in impacting weight loss efforts, especially among individuals with overweight and obesity. Efforts should be made to lengthen and upscale this program for a greater understanding of the midterm to long-term effects of this app.
Conflicts of Interest
AMC has served on advisory boards to Eli Lilly and Boehringer Ingelheim and received grant support, on behalf of the University of Pennsylvania, from Eli Lilly and WW (Weight Watchers). No other authors declare conflicts of interest.
TREND (Transparent Reporting of Evaluations with Nonrandomized Designs) checklist.
Details on outcome measures.
- Abdelaal M, le Roux CW, Docherty NG. Morbidity and mortality associated with obesity. Ann Transl Med. Apr 2017;5(7):161. [ FREE Full text ] [ CrossRef ] [ Medline ]
- OECD. The heavy burden of obesity: the economics of prevention. OECD Health Policy Studies. Oct 10, 2019.:1-100. [ FREE Full text ] [ CrossRef ]
- Hawkes C, Smith TG, Jewell J, Wardle J, Hammond RA, Friel S, et al. Smart food policies for obesity prevention. The Lancet. Jun 2015;385(9985):2410-2421. [ CrossRef ]
- Johns DJ, Hartmann-Boyce J, Jebb SA, Aveyard P, Behavioural Weight Management Review Group. Diet or exercise interventions vs combined behavioral weight management programs: a systematic review and meta-analysis of direct comparisons. J Acad Nutr Diet. Oct 2014;114(10):1557-1568. [ FREE Full text ] [ CrossRef ] [ Medline ]
- Clarke B, Kwon J, Swinburn B, Sacks G. Understanding the dynamics of obesity prevention policy decision-making using a systems perspective: A case study of Healthy Together Victoria. PLoS One. 2021;16(1):e0245535. [ FREE Full text ] [ CrossRef ] [ Medline ]
- Chew HSJ, Koh WL, Ng JSHY, Tan KK. Sustainability of weight loss through smartphone apps: systematic review and meta-analysis on anthropometric, metabolic, and dietary outcomes. J Med Internet Res. Sep 21, 2022;24(9):e40141. [ FREE Full text ] [ CrossRef ] [ Medline ]
- Booth HP, Prevost TA, Wright AJ, Gulliford MC. Effectiveness of behavioural weight loss interventions delivered in a primary care setting: a systematic review and meta-analysis. Fam Pract. Dec 2014;31(6):643-653. [ FREE Full text ] [ CrossRef ] [ Medline ]
- LeBlanc ES, Patnode CD, Webber EM, Redmond N, Rushkin M, O'Connor EA. Behavioral and pharmacotherapy weight loss interventions to prevent obesity-related morbidity and mortality in adults: updated evidence report and systematic review for the US Preventive Services Task Force. JAMA. Sep 18, 2018;320(11):1172-1191. [ CrossRef ] [ Medline ]
- MacLean PS, Wing RR, Davidson T, Epstein L, Goodpaster B, Hall KD, et al. NIH working group report: Innovative research to improve maintenance of weight loss. Obesity (Silver Spring). Jan 2015;23(1):7-15. [ FREE Full text ] [ CrossRef ] [ Medline ]
- Daley A, Jolly K, Madigan C, et al. A brief behavioural intervention to promote regular self-weighing to prevent weight regain after weight loss: a RCT. Public Health Research. 2019.:7. [ CrossRef ] [ Medline ]
- Hadžiabdić MO, Mucalo I, Hrabač P, Matić T, Rahelić D, Božikov V. Factors predictive of drop-out and weight loss success in weight management of obese patients. J Hum Nutr Diet. Feb 2015;28 Suppl 2:24-32. [ CrossRef ] [ Medline ]
- Evans D. MyFitnessPal. Br J Sports Med. Jan 27, 2016;51(14):1101-1102. [ CrossRef ]
- Garcia R. Utilization, Integration & Evaluation of LIVESTRONG's MyPlate Telehealth Technology. URL: https://www.researchgate.net/publication/273635099_Utilization_Integration_Evaluation_of_LIVESTRONG's_MyPlate_Telehealth_Technology [accessed 2023-04-01]
- Hartman SJ, Nelson SH, Weiner LS. Patterns of Fitbit use and activity levels throughout a physical activity intervention: exploratory analysis from a randomized controlled trial. JMIR Mhealth Uhealth. Feb 05, 2018;6(2):e29. [ FREE Full text ] [ CrossRef ] [ Medline ]
- Yao J, Tan CS, Chen C, Tan J, Lim N, Müller-Riemenschneider F. Bright spots, physical activity investments that work: National Steps Challenge, Singapore: a nationwide mHealth physical activity programme. Br J Sports Med. Sep 2020;54(17):1047-1048. [ CrossRef ] [ Medline ]
- Lim SL, Johal J, Ong KW, Han CY, Chan YH, Lee YM, et al. Lifestyle intervention enabled by mobile technology on weight loss in patients with nonalcoholic fatty liver disease: randomized controlled trial. JMIR Mhealth Uhealth. Apr 13, 2020;8(4):e14802. [ FREE Full text ] [ CrossRef ] [ Medline ]
- Birkett D. Disinhibition. In: The Psychiatry of Stroke. London, UK. Routledge; 2012;150-161.
- Carels RA, Hoffman J, Collins A, Raber AC, Cacciapaglia H, O'Brien WH. Ecological momentary assessment of temptation and lapse in dieting. Eat Behav. 2001;2(4):307-321. [ CrossRef ] [ Medline ]
- Kwasnicka D, Dombrowski SU, White M, Sniehotta FF. N-of-1 study of weight loss maintenance assessing predictors of physical activity, adherence to weight loss plan and weight change. Psychol Health. Jun 2017;32(6):686-708. [ FREE Full text ] [ CrossRef ] [ Medline ]
- McKee HC, Ntoumanis N, Taylor IM. An ecological momentary assessment of lapse occurrences in dieters. Ann Behav Med. Dec 2014;48(3):300-310. [ CrossRef ] [ Medline ]
- Chew HSJ, Loong SSE, Lim SL, Tam WSW, Chew NWS, Chin YH, et al. Socio-demographic, behavioral and psychological factors associated with high BMI among adults in a Southeast Asian multi-ethnic society: a structural equation model. Nutrients. Apr 10, 2023;15(8):1826. [ FREE Full text ] [ CrossRef ] [ Medline ]
- Des Jarlais DC, Lyles C, Crepaz N, TREND Group. Improving the reporting quality of nonrandomized evaluations of behavioral and public health interventions: the TREND statement. Am J Public Health. Mar 2004;94(3):361-366. [ CrossRef ] [ Medline ]
- Knapp T. Why is the one-group pretest-posttest design still used? Clin Nurs Res. Oct 2016;25(5):467-472. [ CrossRef ] [ Medline ]
- Faul F, Erdfelder E, Lang AG, Buchner A. G*Power 3: a flexible statistical power analysis program for the social, behavioral, and biomedical sciences. Behav Res Methods. May 2007;39(2):175-191. [ CrossRef ] [ Medline ]
- Chew HSJ. The use of artificial intelligence-based conversational agents (chatbots) for weight loss: scoping review and practical recommendations. JMIR Med Inform. Apr 13, 2022;10(4):e32578. [ FREE Full text ] [ CrossRef ] [ Medline ]
- Chew HSJ, Achananuparp P. Perceptions and needs of artificial intelligence in health care to increase adoption: scoping review. J Med Internet Res. Jan 14, 2022;24(1):e32939. [ FREE Full text ] [ CrossRef ] [ Medline ]
- Chew HSJ, Gao Y, Shabbir A, Lim SL, Geetha K, Kim G, et al. Personal motivation, self-regulation barriers and strategies for weight loss in people with overweight and obesity: a thematic framework analysis. Public Health Nutr. Feb 22, 2022;25(9):2426-2435. [ CrossRef ]
- Chew H, Lim S, Kim G, Kayambu G, So BYJ, Shabbir A, et al. Essential elements of weight loss apps for a multi-ethnic population with high BMI: a qualitative study with practical recommendations. Transl Behav Med. Apr 03, 2023;13(3):140-148. [ CrossRef ] [ Medline ]
- Chew HSJ, Ang WHD, Lau Y. The potential of artificial intelligence in enhancing adult weight loss: a scoping review. Public Health Nutr. Feb 17, 2021;24(8):1993-2020. [ CrossRef ]
- Chew HSJ, Lau ST, Lau Y. Weight-loss interventions for improving emotional eating among adults with high body mass index: A systematic review with meta-analysis and meta-regression. Eur Eat Disord Rev. Jul 2022;30(4):304-327. [ FREE Full text ] [ CrossRef ] [ Medline ]
- Chew HSJ, Sim KLD, Choi KC, Chair SY. Effectiveness of a nurse-led temporal self-regulation theory-based program on heart failure self-care: A randomized controlled trial. Int J Nurs Stud. Mar 2021;115:103872. [ CrossRef ] [ Medline ]
- Hall PA, Fong GT. Temporal self-regulation theory: A model for individual health behavior. Health Psychology Review. Mar 2007;1(1):6-52. [ CrossRef ]
- Abraham C, Michie S. A taxonomy of behavior change techniques used in interventions. Health Psychol. May 2008;27(3):379-387. [ CrossRef ] [ Medline ]
- Chew HSJ, Li J, Chng S. Improving adult eating behaviours by manipulating time perspective: a systematic review and meta-analysis. Psychol Health. Jan 24, 2023.:1-17. [ CrossRef ] [ Medline ]
- Chew HSJ, Rajasegaran NN, Chng S. Effectiveness of interactive technology-assisted interventions on promoting healthy food choices: a scoping review and meta-analysis. Br J Nutr. Jan 25, 2023;130(7):1250-1259. [ CrossRef ]
- Verplanken B, Orbell S. Reflections on past behavior: a self‐report index of habit strength. J Applied Social Pyschol. Jul 31, 2006;33(6):1313-1330. [ CrossRef ]
- Chng S, Chew HSJ, Joireman J. When time is of the essence: Development and validation of brief consideration of future (and immediate) consequences scales. Personality and Individual Differences. Feb 2022;186:111362. [ CrossRef ]
- Kliemann N, Beeken RJ, Wardle J, Johnson F. Development and validation of the Self-Regulation of Eating Behaviour Questionnaire for adults. Int J Behav Nutr Phys Act. Aug 02, 2016;13:87. [ FREE Full text ] [ CrossRef ] [ Medline ]
- Craig CL, Marshall AL, Sjöström M, Bauman AE, et al. International Physical Activity Questionnaire: 12-country reliability and validity. Medicine & Science in Sports & Exercise. 2003;35(8):1381-1395. [ CrossRef ]
- Kroenke K, Spitzer RL, Williams JB, Monahan PO, Löwe B. Anxiety disorders in primary care: prevalence, impairment, comorbidity, and detection. Ann Intern Med. Mar 06, 2007;146(5):317-325. [ CrossRef ] [ Medline ]
- Kroenke K, Spitzer RL, Williams JBW. The Patient Health Questionnaire-2: validity of a two-item depression screener. Med Care. Nov 2003;41(11):1284-1292. [ CrossRef ] [ Medline ]
- IBM SPSS statistics for Windows. IBM Corp. May 21, 2021. URL: https://www.ibm.com/products/spss-statistics [accessed 2024-03-28]
- VanderWeele T, Mathur M. Some desirable properties of the Bonferroni correction: is the Bonferroni correction really so bad? Am J Epidemiol. Mar 01, 2019;188(3):617-618. [ FREE Full text ] [ CrossRef ] [ Medline ]
- Bengtsson M. How to plan and perform a qualitative study using content analysis. NursingPlus Open. 2016;2:8-14. [ CrossRef ]
- Varkevisser RDM, van Stralen MM, Kroeze W, Ket JCF, Steenhuis IHM. Determinants of weight loss maintenance: a systematic review. Obes Rev. Feb 2019;20(2):171-211. [ FREE Full text ] [ CrossRef ] [ Medline ]
- Forman EM, Goldstein SP, Crochiere RJ, Butryn ML, Juarascio AS, Zhang F, et al. Randomized controlled trial of OnTrack, a just-in-time adaptive intervention designed to enhance weight loss. Transl Behav Med. Nov 25, 2019;9(6):989-1001. [ CrossRef ] [ Medline ]
- Qasim A, Turcotte M, de Souza RJ, Samaan MC, Champredon D, Dushoff J, et al. On the origin of obesity: identifying the biological, environmental and cultural drivers of genetic risk among human populations. Obes Rev. Feb 2018;19(2):121-149. [ CrossRef ] [ Medline ]
- Executive summary on National Population Health Survey 2016/17. Singapore MoH. URL: https://www.moh.gov.sg/docs/librariesprovider5/resources-statistics/reports/executive-summary-nphs-2016_17.pdf [accessed 2023-04-01]
- McCrory MA, Suen VM, Roberts SB. Biobehavioral influences on energy intake and adult weight gain. The Journal of Nutrition. Dec 2002;132(12):3830S-3834S. [ CrossRef ]
- Davis C, Levitan RD, Muglia P, Bewell C, Kennedy JL. Decision-making deficits and overeating: a risk model for obesity. Obes Res. Jun 2004;12(6):929-935. [ FREE Full text ] [ CrossRef ] [ Medline ]
- Hetherington MM. Cues to overeat: psychological factors influencing overconsumption. Proc. Nutr. Soc. Feb 28, 2007;66(1):113-123. [ CrossRef ]
- Borer K. Understanding human physiological limitations and societal pressures in favor of overeating helps to avoid obesity. Nutrients. Jan 22, 2019;11(2):227. [ CrossRef ]
- Borer KT. Why we eat too much, have an easier time gaining than losing weight, and expend too little energy: suggestions for counteracting or mitigating these problems. Nutrients. Oct 26, 2021;13(11):3812. [ CrossRef ]
- Stice E, Burger K. Neural vulnerability factors for obesity. Clin Psychol Rev. Mar 2019;68:38-53. [ FREE Full text ] [ CrossRef ] [ Medline ]
- Warren JM, Smith N, Ashwell M. A structured literature review on the role of mindfulness, mindful eating and intuitive eating in changing eating behaviours: effectiveness and associated potential mechanisms. Nutr. Res. Rev. Jul 18, 2017;30(2):272-283. [ CrossRef ]
- Savige G, Macfarlane A, Ball K, Worsley A, Crawford D. Snacking behaviours of adolescents and their association with skipping meals. Int J Behav Nutr Phys Act. Sep 17, 2007;4:36. [ FREE Full text ] [ CrossRef ] [ Medline ]
- Njike VY, Smith TM, Shuval O, Shuval K, Edshteyn I, Kalantari V, et al. Snack food, satiety, and weight. Adv Nutr. Sep 2016;7(5):866-878. [ FREE Full text ] [ CrossRef ] [ Medline ]
- Larson N, Story M. A review of snacking patterns among children and adolescents: what are the implications of snacking for weight status? Child Obes. Apr 2013;9(2):104-115. [ CrossRef ] [ Medline ]
- Lucan SC, Karpyn A, Sherman S. Storing empty calories and chronic disease risk: snack-food products, nutritive content, and manufacturers in Philadelphia corner stores. J Urban Health. May 2010;87(3):394-409. [ FREE Full text ] [ CrossRef ] [ Medline ]
- Hess J, Jonnalagadda S, Slavin J. What is a snack, why do we snack, and how can we choose better snacks? The definitions of snacking, motivations to snack, contributions to dietary intake, and recommendations for improvement. The FASEB Journal. Apr 2016;30(S1):466-475. [ CrossRef ]
- Coulter A, Entwistle V, Eccles A, Ryan S, Shepperd S, Perera R. Personalised care planning for adults with chronic or long-term health conditions. Cochrane Database Syst Rev. 2015;2015(3):1-117. [ CrossRef ]
- Elwyn G, Frosch D, Thomson R, Joseph-Williams N, Lloyd A, Kinnersley P, et al. Shared decision making: a model for clinical practice. J Gen Intern Med. Oct 2012;27(10):1361-1367. [ FREE Full text ] [ CrossRef ] [ Medline ]
- Lau Y, Chee DGH, Chow XP, Cheng LJ, Wong SN. Personalised eHealth interventions in adults with overweight and obesity: A systematic review and meta-analysis of randomised controlled trials. Prev Med. Mar 2020;132:106001. [ CrossRef ] [ Medline ]
- Wang Q, Egelandsdal B, Amdam GV, Almli VL, Oostindjer M. Diet and physical activity apps: perceived effectiveness by app users. JMIR Mhealth Uhealth. Apr 07, 2016;4(2):e33. [ FREE Full text ] [ CrossRef ] [ Medline ]
- Kim B, Choi D, Jung C, Kang S, Mok J, Kim C. Obesity and physical activity. J Obes Metab Syndr. Mar 2017;26(1):15-22. [ FREE Full text ] [ CrossRef ] [ Medline ]
- Anderson JW, Konz EC, Frederich RC, Wood CL. Long-term weight-loss maintenance: a meta-analysis of US studies. Am J Clin Nutr. Nov 2001;74(5):579-584. [ CrossRef ] [ Medline ]
- Fogelholm M, Kukkonen-Harjula K. Does physical activity prevent weight gain--a systematic review. Obes Rev. Oct 2000;1(2):95-111. [ CrossRef ] [ Medline ]
- Thomas DM, Bouchard C, Church T, Slentz C, Kraus WE, Redman LM, et al. Why do individuals not lose more weight from an exercise intervention at a defined dose? An energy balance analysis. Obesity Reviews. Jun 11, 2012;13(10):835-847. [ CrossRef ]
- Saris WHM, Blair SN, van Baak MA, Eaton SB, Davies PSW, Di Pietro L, et al. How much physical activity is enough to prevent unhealthy weight gain? Outcome of the IASO 1st Stock Conference and consensus statement. Obes Rev. May 2003;4(2):101-114. [ CrossRef ] [ Medline ]
- Haskell W, Lee I, Pate R, Powell K, Blair S, Franklin B. Physical activity and public health: updated recommendation for adults from the American College of Sports Medicine and the American Heart Association. Med Sci Sports Exerc. 2007;39(8):1423-1434. [ CrossRef ]
- Fredriksson SV, Alley SJ, Rebar AL, Hayman M, Vandelanotte C, Schoeppe S. How are different levels of knowledge about physical activity associated with physical activity behaviour in Australian adults? PLoS One. 2018;13(11):e0207003. [ FREE Full text ] [ CrossRef ] [ Medline ]
- Muntaner-Mas A, Sanchez-Azanza VA, Ortega FB, Vidal-Conti J, Borràs PA, Cantallops J, et al. The effects of a physical activity intervention based on a fatness and fitness smartphone app for University students. Health Informatics J. 2021;27(1):1460458220987275. [ FREE Full text ] [ CrossRef ] [ Medline ]
- Hautekiet P, Saenen ND, Martens DS, Debay M, Van der Heyden J, Nawrot TS, et al. A healthy lifestyle is positively associated with mental health and well-being and core markers in ageing. BMC Med. Sep 29, 2022;20(1):328. [ FREE Full text ] [ CrossRef ] [ Medline ]
- Lassale C, Batty GD, Baghdadli A, Jacka F, Sánchez-Villegas A, Kivimäki M, et al. Healthy dietary indices and risk of depressive outcomes: a systematic review and meta-analysis of observational studies. Mol Psychiatry. Jul 2019;24(7):965-986. [ FREE Full text ] [ CrossRef ] [ Medline ]
- Petridou ET, Kousoulis AA, Michelakos T, Papathoma P, Dessypris N, Papadopoulos FC, et al. Folate and B12 serum levels in association with depression in the aged: a systematic review and meta-analysis. Aging Ment Health. Sep 2016;20(9):965-973. [ CrossRef ] [ Medline ]
- Wang C, Yang T, Wang G, Zhao Y, Yang L, Bi B. Association between dietary patterns and depressive symptoms among middle-aged adults in China in 2016-2017. Psychiatry Res. Feb 2018;260:123-129. [ CrossRef ] [ Medline ]
- Vaidyam AN, Wisniewski H, Halamka JD, Kashavan MS, Torous JB. Chatbots and conversational agents in mental health: a review of the psychiatric landscape. Can J Psychiatry. Jul 2019;64(7):456-464. [ FREE Full text ] [ CrossRef ] [ Medline ]
- Population in brief. National Population and Talent Division SG. 2022. URL: https://www.strategygroup.gov.sg/files/media-centre/publications/population-in-brief-2022.pdf [accessed 2023-04-01]
- Hebden L, Cook A, van der Ploeg HP, King L, Bauman A, Allman-Farinelli M. A mobile health intervention for weight management among young adults: a pilot randomised controlled trial. J Hum Nutr Diet. Aug 2014;27(4):322-332. [ CrossRef ] [ Medline ]
- Rasi P, Vuojärvi H, Rivinen S. Promoting media literacy among older people: a systematic review. Adult Education Quarterly. May 25, 2020;71(1):37-54. [ CrossRef ]
Abbreviations
Edited by A Mavragani; submitted 26.01.23; peer-reviewed by YC Liu, V Jennings; comments to author 07.12.23; revised version received 12.12.23; accepted 12.03.24; published 07.05.24.
©Han Shi Jocelyn Chew, Nicholas WS Chew, Shaun Seh Ern Loong, Su Lin Lim, Wai San Wilson Tam, Yip Han Chin, Ariana M Chao, Georgios K Dimitriadish, Yujia Gao, Jimmy Bok Yan So, Asim Shabbir, Kee Yuan Ngiam. Originally published in the Journal of Medical Internet Research (https://www.jmir.org), 07.05.2024.
This is an open-access article distributed under the terms of the Creative Commons Attribution License (https://creativecommons.org/licenses/by/4.0/), which permits unrestricted use, distribution, and reproduction in any medium, provided the original work, first published in the Journal of Medical Internet Research, is properly cited. The complete bibliographic information, a link to the original publication on https://www.jmir.org/, as well as this copyright and license information must be included.
- Bihar Board
SRM University
Tn sslc result 2024.
- TN Board Result 2024
- GSEB Board Result 2024
- Karnataka Board Result 2024
- CG Board Result 2024
- Kerala Board Result 2024
- Shiv Khera Special
- Education News
- Web Stories
- Current Affairs
- नए भारत का नया उत्तर प्रदेश
- School & Boards
- College Admission
- Govt Jobs Alert & Prep
- GK & Aptitude
- State Boards
CBSE Class 10 Artificial Intelligence Latest Syllabus Free PDF Download
Cbse class 10 artificial intelligence latest syllabus free pdf download: the central board of secondary education has made available the latest syllabus for artificial intelligence. you can download the pdf for free. .
.jpeg)
OBJECTIVES OF THE COURSE:
- Helping learners understand the world of Artificial Intelligence and its applications through games, activities and multi-sensorial learning to become AI-ready.
- Introducing the learners to three domains of AI in an age-appropriate manner.
- Allowing the learners to construct the meaning of AI through interactive participation and engaging hands-on activities.
- Introducing the learners to the AI Project Cycle.
- Introducing the learners to programming skills - Basic Python coding language.
Total Units To Be Covered
Detailed curriculum/topics for class x.
Note: The detailed curriculum/ topics to be covered under Part A: Employability Skills can be downloaded from the CBSE website
- Unit 1: Introduction to Artificial Intelligence (AI)
- Unit 2: AI Project Cycle
- Unit 3: Advance Python
- Unit 4: Data Science
- Unit 5: Computer Vision
- Unit 6: Natural Language Processing
- Unit 7: Evaluation
UNIT 1: INTRODUCTION TO ARTIFICIAL INTELLIGENCE
Unit 2: ai project cycle, unit 3: advance python (to be assessed through practicals), unit 4: data sciences (to be assessed through theory), unit 4: data sciences (to be assessed through practicals), unit 5: computer vision (to be assessed through theory), unit 5: computer vision (to be assessed through practicals), unit 6: natural language processing, unit 7: evaluation, part-c: practical work.
PART-D: Project Work / Field Visit / Student Portfolio
* relate it to Sustainable Development Goals
CBSE Class 10 Artificial Intelligence Syllabus 2024-25 Free PDF Download
Also, check
HBSE Class 12th Business Studies Syllabus 2024-25: Download PDF for Board Examination
Get here latest School , CBSE and Govt Jobs notification in English and Hindi for Sarkari Naukari and Sarkari Result . Download the Jagran Josh Sarkari Naukri App . Check Board Result 2024 for Class 10 and Class 12 like CBSE Board Result , UP Board Result , Bihar Board Result , MP Board Result , Rajasthan Board Result and Other States Boards.
- SSC GD Result 2024
- RBSE 10th, 12th Result 2024
- SSLC Result 2024 Tamil Nadu
- tnresults.nic.in 10th Result 2024
- dge.tn.gov.in Result 2024
- 10th Public Exam Result 2024 Tamil Nadu
- DHSE Kerala Plus Two Result 2024
- CGBSE 10th Result 2024
- CGBSE 12th Result 2024
- NDA Result 2024
Latest Education News
SSLC Result 2024 TN (முடிவு) LIVE: 91.55% Overall Passed, Check TNDGE Tamil Nadu 10th Results Website Link at tnresults.nic.in, dge.tn.gov.in
GSEB Gujarat 10th Result 2024 LIVE: ગુજરાત બોર્ડ Class 10th Results Link Online at gseb.org, How to Check via WhatsApp with Seat Number Here
GSEB Result 2024: Check Gujarat Board 10th, 12th Result Online at gseb.org
GSEB SSC Result 2024: Gujarat Board 10th Result Date And Time at gseb.org
[Declared] tnresults.nic.in 10th Result 2024: Check Official LINKS to Check TNDGE TN SSLC Class Xth Std Results Online
[LINK ACTIVE] Kerala Plus Two Result 2024 School-Wise, Link Declared: Check DHSE Results, Marks with School Code at keralaresults.nic.in
Std 10 Result 2024 Link: Gujarat Board SSC Results Tomorrow at gseb.org, Check Latest News and Updates
SSC GD Result 2024 Live: Constable Results Direct Link on ssc.gov.in; Check Expected Cut Off, Merit List Date
SSC GD Constable Result 2024 Live Update: जल्द जारी होने वाला है एसएससी कांस्टेबल रिजल्ट ssc.gov.in पर, यहाँ देखें एक्सपेक्टेड कटऑफ मार्क्स
Kerala SSLC Result 2024 [ഫലം] Out LIVE: Check KBPE Class 10 Results Link at Official Website: results.kite.kerala.gov.in by Roll Number, School-wise
SSLC Result 2024 Karnataka LIVE (ಫಲಿತಾಂಶ) OUT: KSEEB 10th Results Online at kseab.karnataka.gov.in, karresults.nic.in by Login and Registration Number
UGC NET Previous Year Question Paper PDF for Paper 1 & 2, Download Subject-wise PYQs
WBPSC Clerkship Salary 2024: Check In-Hand Pay, Structure, Perks and Allowances
BSEB STET Exam Date 2024: इसी माह हो सकती है बिहार एसटीईटी की परीक्षा, जल्द घोषित होगी तारीखें
[यहां देखें] Purple Cap in IPL 2024: किसके नाम सबसे ज्यादा विकेट, कौन निकलेगा सबसे आगे?
Microsoft Gears Up for Mobile Domination: Xbox Game Store Arrives on iOS and Android
Lok Sabha Elections 2024: प्रधानमंत्री पद पर रहते हुए लोकसभा चुनाव में किस नेता को मिली है हार? पढ़ें
GT IPL 2024 Match Schedule: गुजरात टाइटंस के सभी मैचों का वेन्यू, डेट और टाइम यहां देखें
IPL 2024 CSK Players: चेन्नई सुपर किंग्स के खिलाड़ियों की पूरी लिस्ट यहां देखें
ICC T20 World Cup 2024: T20 वर्ल्ड कप का शेड्यूल जारी, कब और किससे है भारत का मैच देखें यहां
- Work & Careers
- Life & Arts
Become an FT subscriber
Try unlimited access Only $1 for 4 weeks
Then $75 per month. Complete digital access to quality FT journalism on any device. Cancel anytime during your trial.
- Global news & analysis
- Expert opinion
- Special features
- FirstFT newsletter
- Videos & Podcasts
- Android & iOS app
- FT Edit app
- 10 gift articles per month
Explore more offers.
Standard digital.
- FT Digital Edition
Premium Digital
Print + premium digital, weekend print + standard digital, weekend print + premium digital.
Essential digital access to quality FT journalism on any device. Pay a year upfront and save 20%.
- Global news & analysis
- Exclusive FT analysis
- FT App on Android & iOS
- FirstFT: the day's biggest stories
- 20+ curated newsletters
- Follow topics & set alerts with myFT
- FT Videos & Podcasts
- 20 monthly gift articles to share
- Lex: FT's flagship investment column
- 15+ Premium newsletters by leading experts
- FT Digital Edition: our digitised print edition
- Weekday Print Edition
- Videos & Podcasts
- Premium newsletters
- 10 additional gift articles per month
- FT Weekend Print delivery
- Everything in Standard Digital
- Everything in Premium Digital
Complete digital access to quality FT journalism with expert analysis from industry leaders. Pay a year upfront and save 20%.
- 10 monthly gift articles to share
- Everything in Print
Terms & Conditions apply
Explore our full range of subscriptions.
Why the ft.
See why over a million readers pay to read the Financial Times.
International Edition
A review of deep learning methods for digitisation of complex documents and engineering diagrams
- Open access
- Published: 09 May 2024
- Volume 57 , article number 136 , ( 2024 )
Cite this article
You have full access to this open access article
- Laura Jamieson 1 ,
- Carlos Francisco Moreno-García 1 na1 &
- Eyad Elyan 1 na1
This paper presents a review of deep learning on engineering drawings and diagrams. These are typically complex diagrams, that contain a large number of different shapes, such as text annotations, symbols, and connectivity information (largely lines). Digitising these diagrams essentially means the automatic recognition of all these shapes. Initial digitisation methods were based on traditional approaches, which proved to be challenging as these methods rely heavily on hand-crafted features and heuristics. In the past five years, however, there has been a significant increase in the number of deep learning-based methods proposed for engineering diagram digitalisation. We present a comprehensive and critical evaluation of existing literature that has used deep learning-based methods to automatically process and analyse engineering drawings. Key aspects of the digitisation process such as symbol recognition, text extraction, and connectivity information detection, are presented and thoroughly discussed. The review is presented in the context of a wide range of applications across different industry sectors, such as Oil and Gas, Architectural, Mechanical sectors, amongst others. The paper also outlines several key challenges, namely the lack of datasets, data annotation, evaluation and class imbalance. Finally, the latest development in digitalising engineering drawings are summarised, conclusions are drawn, and future interesting research directions to accelerate research and development in this area are outlined.
Avoid common mistakes on your manuscript.
1 Introduction
Engineering diagrams are considered one of the most complex to digitise. This is due to multiple reasons such as the combination of vast variety of symbols and text, dense representation of equipment and non standard formatting. Furthermore, there can be scientific annotations and the drawings can be edited over time to contain annotations from multiple disciplines. These diagrams are prevalent across multiple industries, including electrical (De et al. 2011 ), oil and gas (Elyan et al. 2020a ), and architecture (Kim et al. 2021a ). Manual analysis of these diagrams is time-consuming, prone to human error (Paliwal et al. 2021a , b ) and requires subject matter experts (Paliwal et al. 2021a ). There has recently been an increasing demand to digitise these diagrams for use in processes including asset performance management (Mani et al. 2020 ), safety studies (Gao et al. 2020 ), and data analytics (Moreno-García et al. 2018 ). Due to its importance, the problem of complex diagram digitisation is receiving interest from academia and industry (Moreno-Garcia and Elyan 2019 ; Hantach et al. 2021 ). For instance, engineering was the field with the most recent digitalisation-related publications in the Scopus database (Espina-Romero and Guerrero-Alcedo 2022 ). Engineering diagrams are complex and used for different purposes, as seen in Fig. 1 . Fig. 1 a represents part of a Piping and Instrumentation Diagram (P&ID). These are commonly used in offshore oil and gas installations, while Fig. 1 b presents part of a HVAC diagram, commonly utilised in construction projects.
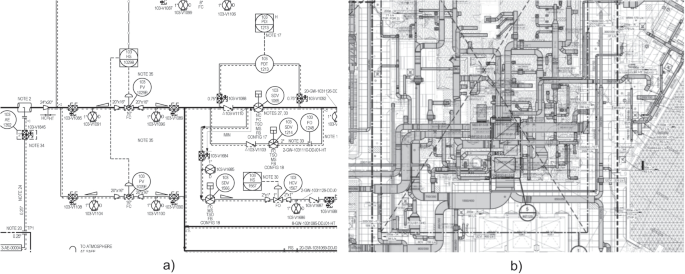
a Small section of a P&ID. b Small section of a HVAC diagram
Various methods have been developed over the past four decades to automate the processing, analysing and interpretation of these diagrams (Kang et al. 2019 ; Groen et al. 1985 ; Okazaki et al. 1988 ; Nurminen et al. 2020 ; Ablameyko and Uchida 2007 ). A relatively recent review by Moreno-García et al. ( 2018 ) showed that most relevant literature followed a traditional machine learning approach to automate these drawings. Traditional approaches are based on hand-crafting a set of features which are then input to a specific supervised machine learning algorithm (LeCun et al. 1998 ). Extensive feature engineering and expert knowledge were often required to design suitable feature extractors (LeCun et al. 1998 ). Image features were typically based on colour, edge and texture. Examples of commonly used image features include Histogram of Oriented Gradient (HOG) (Dalal and Triggs 2005 ), Scale Invariant Feature Transform (SIFT) (Lowe 2004 ), Speeded Up Robust Features (SURF) (Bay et al. 2006 ) and Local Binary Pattern (LBP) (Ojala et al. 2002 ). The feature vectors were classified using algorithms, such as a Support Vector Machine (SVM). Whilst traditional methods were shown to work well in specific use cases, they were not suited to the extensive range of characteristics present in engineering diagrams (Moreno-García et al. 2019 ). For example, traditional symbol classification methods may be limited by variations in symbol appearance, including rotation, translation and degradation (Moreno-García et al. 2019 ). Morphological changes and noise also compromised traditional methods’ accuracy (Yu et al. 2019 ). The reliance of traditional methods on pre-established rules resulted in weak generalisation ability across variations (Zhao et al. 2020 ).
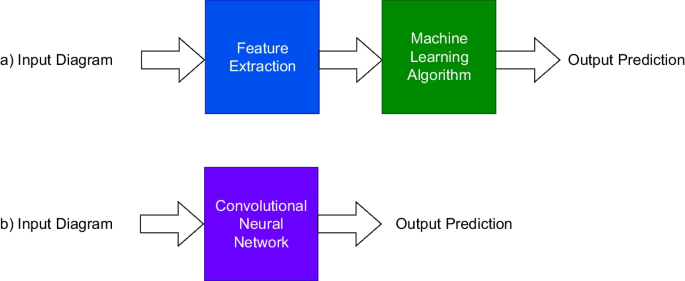
Comparison of traditional and deep learning approaches for engineering diagram digitisation. a Traditional Approach and b deep learning approach
In recent years, deep learning has significantly advanced the domain of computer vision (LeCun et al. 2015 ). Deep learning is a subfield of machine learning, which is itself a subfield of artificial intelligence. Figure 2 illustrates the key differences between traditional and deep learning methods. In contrast to traditional machine learning-based methods, deep learning-based methods learn features automatically. Deep learning models contain multiple computation layers which can be trained to extract relevant features from data. Convolutional Neural Networks (CNN) have improved computer vision methods, including image classification, segmentation and object detection (LeCun et al. 2015 ). In 1998, LeCun et al. ( 1998 ) introduced the influential LeNet model. The authors presented a CNN-based method for handwritten character recognition. They showed that a CNN could automatically learn features from pixel data and outperform traditional approaches. However, a significant improvement in methods was seen mainly since 2012 when Krizhevsky et al. ( 2012 ) presented the AlexNet model. AlexNet was used to classify images in the 2012 ImageNet Large Scale Visual Recognition Challenge (ILSVRC) (Russakovsky et al. 2015 ). The authors obtained the winning score by a large margin. The top 5 error rate was 15.3%, compared to 26.2% for the second-place method. Since then, there has been a considerable rise in deep learning. This was facilitated by algorithm developments, improvements in computing hardware, and a significant increase in available data.
Despite the recent and unprecedented progress, digitising engineering drawings continues to be a challenging problem (Moreno-García et al. 2018 ). First of all, these diagrams are very complex, containing a large number of similar (Paliwal et al. 2021a ; Rahul et al. 2019 ) and overlapping (Rahul et al. 2019 ) shapes. For example, Elyan et al. ( 2020a ) reported on average 180 symbols of different types in a real-world P&ID dataset. The presence of text is another challenging problem. There is no consistent pattern for engineering equipment layout, meaning the text can be present anywhere in the diagram. It is also commonly present in multiple fonts (Rahul et al. 2019 ), scales and orientations (Gao et al. 2020 ). Contextualisation of the extracted data is a further challenge. This involves determining the relationships between extracted data, for example, associating a tag with the relevant symbol. Moreno-Garcia and Elyan ( 2019 ) identified three additional challenges as document quality, imbalanced data and topology. Although a large proportion of the related literature analysed high-quality drawings, in practice, the drawings can be low-quality (Moreno-Garcia and Elyan 2019 ). Another factor restricting the development of deep learning models in this area is the lack of publicly available datasets (Hantach et al. 2021 ; Moreno-García et al. 2019 ). Furthermore, annotation of these datasets is required for use with supervised learning algorithms, which is typically a time-consuming and often impractical manual process.
In this paper, we present a comprehensive critical investigation of existing literature that utilises state-of-the-art deep learning methods for digitising complex engineering drawings. In a related area, Pizarro et al. ( 2022 ) provided a review on the automatic analysis and recognition of floor plans. They focussed on both rule-based and learning-based approaches. However, there is a gap in the literature, as there is no published review which covers the surge in the deep learning research in engineering diagram digitisation published in the last five years.
The reviewed literature was selected according to several criteria. First, the paper should present a deep learning method for the digitisation of engineering drawings. This covers a wide variety of drawing types, such as P&IDs and architectural diagrams. This review also covers the literature that focussed on the digitisation of specific elements, such as presenting a detection method for symbols, aswell as that which presented multiple methods to digitise more than one diagram component. Papers which presented a mixture of deep learning and traditional methods were included. Second, we reviewed peer-reviewed articles from academic databases including IEEE Xplore, ACM Digital Library and Science Direct. Third, we focus on the recent literature that was published in the last five years. This shows there is an urgent need for more accurate and stable methods to handle such complex documents and engineering diagrams. Furthermore, from analysing these papers, remaining challenges were elicited, which were datasets, data annotation, evaluation and class imbalance.
The main contributions of this paper are outlined as follows:
A critical and comprehensive investigation of deep learning-based methods for digitising engineering diagrams.
A thorough discussion of the open research challenges associated with deep learning solutions for complex diagrams.
Recommendations for future research directions are provided to overcome the remaining challenges and improve the field of complex engineering diagram digitisation.
The rest of this paper is structured as follows:
Section 2 presents the reviewed literature in terms of application domains across various sectors. It also covers a thorough critical investigation of deep learning-based methods for digitising engineering drawings. This includes an in-depth technical discussion of state-of-the-art methods for handling symbols, text, and connectivity information in these diagrams. In Sect. 3 , the challenges associated with deep learning methods for complex diagram digitisation are discussed. Finally, Sect. 4 provides the conclusion and suggestions for future work.
2 Related work
Deep learning has been used for diagram digitisation across various domains. The diagrams are composed of three elements. These are symbols, text and connectors. Connectors link symbols together and represent various line types, including continuous or dashed lines. Specialised computer vision methods are required to digitise each element type. This section introduces and discusses the application domains, together with the state-of-the-art deep learning methods used in the recent and relevant literature on complex engineering diagram digitisation.
2.1 Application domains
The reviewed literature is listed by application and extracted data type in Table 1 . Amongst these applications, there has been a considerable research focus on P&IDs (Rahul et al. 2019 ; Sinha et al. 2019 ; Yu et al. 2019 ; Mani et al. 2020 ; Gao et al. 2020 ; Elyan et al. 2020a ; Moreno-García et al. 2020 ; Jamieson et al. 2020 ; Nurminen et al. 2020 ; Paliwal et al. 2021a ; Moon et al. 2021 ; Kim et al. 2021b ; Stinner et al. 2021 ; Paliwal et al. 2021b ; Toral et al. 2021 ; Bhanbhro et al. 2022 ; Hantach et al. 2021 ). Another research area is architecture diagram digitisation (Ziran and Marinai 2018 ; Zhao et al. 2020 ; Rezvanifar et al. 2020 ; Kim et al. 2021a ; Renton et al. 2021 ; Jakubik et al. 2022 ). Deep learning methods were also applied to technical drawings (Nguyen et al. 2021 ), construction drawings (Faltin et al. 2022 ) engineering documents (Francois et al. 2022 ) and engineering drawings (Sarkar et al. 2022 ; Scheibel et al. 2021 ; Haar et al. 2023 ).
Most of the P&ID digitisation literature focussed on the extraction of specific data types (Sinha et al. 2019 ; Gao et al. 2020 ; Elyan et al. 2020a ; Jamieson et al. 2020 ; Nurminen et al. 2020 ; Moon et al. 2021 ; Kim et al. 2021b ; Stinner et al. 2021 ; Paliwal et al. 2021b ; Toral et al. 2021 ). There is a particular focus on P&ID symbols (Elyan et al. 2020a ; Nurminen et al. 2020 ; Paliwal et al. 2021b ). For example, Elyan et al. ( 2020a ) presented a You Only Look Once (YOLO) v3 (Redmon and Farhadi 2018 ) based detection method for symbols in real-world P&IDs. A Generative Adversarial Network (GAN) based (Ali-Gombe and Elyan 2019 ) approach was used to synthesise more data to improve classification. Meanwhile, Paliwal et al. ( 2021b ) used a graph-based approach for symbol recognition. Other studies focussed on the text (Jamieson et al. 2020 ; Francois et al. 2022 ) or connectors (Moon et al. 2021 ). Studies that presented methods for multiple element types were also seen (Gao et al. 2020 ; Stinner et al. 2021 ). For instance, Gao et al. ( 2020 ) created a Region-based Fully Convolutional Network (R-FCN) (Dai et al. 2016 ) component detection method and a SegLink (Shi et al. 2017a ) based text detection method. Meanwhile, Stinner et al. ( 2021 ) presented work on extracting symbols, lines and line crossings, however they did not consider the text.
There are only a few recent P&ID digitisation studies that presented methods for symbols, text and connectors (Paliwal et al. 2021a ; Rahul et al. 2019 ; Yu et al. 2019 ; Mani et al. 2020 ; Hantach et al. 2021 ). These were often focused on specific elements of interest. For example, Mani et al. ( 2020 ) created symbols, text and connection detection methods. They considered two symbol classes and recognised the text associated with these symbols. Hantach et al. ( 2021 ) also proposed symbol, text and lines methods. The authors only had access to a limited dataset of eight P&IDs and considered one symbol class. Meanwhile, Yu et al. ( 2019 ) created methods for tables aswell as symbols, lines and text. Deep learning was used for symbols and text, while the lines and table detection methods were based on traditional image processing.
Extracted elements have been associated to each other using distance-based or graph-based methods (Mani et al. 2020 ; Paliwal et al. 2021a ; Rahul et al. 2019 ; Bickel et al. 2023 ; Theisen et al. 2023 ). For instance, Mani et al. ( 2020 ) determined symbol-to-symbol connections by representing the P&ID in graph format and implementing a depth-first search. Paliwal et al. ( 2021a ) used a graph-based method to associate lines with relevant symbols and text. Meanwhile, Rahul et al. ( 2019 ) used the euclidean distance to associate detected symbols, tags and pipeline codes with the closest pipeline. Theisen et al. ( 2023 ) presented methods for the digitisation of process flow diagrams. They used a Faster Regions with CNN features (Faster R-CNN) (Girshick et al. 2014 ) model to detect the unit operations, and a pixel search based algorithm to detect the connections between them. Then, the data was converted to a graph.
Deep learning has also been recently applied for the digitisation of architecture diagrams (Ziran and Marinai 2018 ; Zhao et al. 2020 ; Rezvanifar et al. 2020 ; Kim et al. 2021a ; Renton et al. 2021 ; Jakubik et al. 2022 ). These present similar challenges to engineering diagrams, such as various semantically equivalent symbol representations (Rezvanifar et al. 2020 ), relatively small objects (Kim et al. 2021a ) and the presence of occlusion and clutter (Rezvanifar et al. 2020 ). One example is the work by Zhao et al. ( 2020 ), which proposed a YOLO (Redmon et al. 2016 ) based method to detect components in scanned structural diagrams. The authors suggested the method as a basis for reconstructing a Building Information Model (BIM). Various approaches have been presented for symbol detection in floor plans, including YOLO (Rezvanifar et al. 2020 ), Faster R-CNN (Jakubik et al. 2022 ; Ziran and Marinai 2018 ) and graph-based (Renton et al. 2021 ) methods.
There are a wide variety of uses of the digitised diagram data. This includes similarity search (Bickel et al. 2023 ), diagram comparison (Daele et al. 2021 ) and classification (Xie et al. 2022 ). For instance, Daele et al. ( 2021 ) used deep learning to create a technical diagram similarity search tool (Daele et al. 2021 ). They used 5000 technical diagrams. A traditional method based on Density-Based Spatial Clustering of Applications with Noise (DBSCAN) (Ester et al. 1996 ) was used to partition the diagram. A CNN containing three convolutional layers classified drawing segments as ‘table’, ‘two-dimensional CAD drawing’ or ‘irrelevant’. A siamese neural network classified a pair of CAD images as either ‘same’ or ‘different’ based on cosine similarity. An accuracy of 96.9% was reported.
Xie et al. ( 2022 ) used deep learning to classify engineering diagrams according to the manufacturing method. A dataset of 1692 industry diagrams of engineering equipment was used. First, the diagrams were pre-processed by removing tables and dimension lines. Information tables were identified using CascadeTabNet (Prasad et al. 2020 ). The model contained two neural networks. The first, HRNet, was used for feature extraction and the second, Cascade R-CNN, for bounding box proposal. Reported precision was 97%. In comparison, the precision of a heuristic method based on watershed segmentation was lower at 78%. Dimension lines were detected using a Graph Neural Network (GNN), which outperformed a heuristic method. However, the authors reported that the network predictions allowed higher fault tolerance. The pre-processed diagram was then converted to graph format. Each node was embedded with line start and end positions. A GNN was used to predict the appropriate manufacturing method. This was shown to outperform various CNN and graph-based approaches. Overall accuracy of 90.8% was reported.
Digitised data from engineering diagrams can be used towards creating a digital twin (Vilgertshofer et al. 2019 ), (Mafipour et al. 2023 ). For instance, Vilgertshofer et al. ( 2019 ) created a CNN-based symbol detection method to check for discrepancies between archived railway technical drawings and built infrastructure. They noted that the method provided significant support towards creating a digital twin of railway infrastructure.
Dzhusupova et al. ( 2022 ) proposed a YOLOv4 (Bochkovskiy et al. 2020 ) based model to detect specific combinations of shapes in P&IDs that represented engineering errors. Domain experts manually labelled 2253 industry P&IDs with eight classes of equipment combinations. A balanced dataset was obtained by creating new examples of rare symbol instances manually. The authors reported around 70% correct recognition, however the results per class were not presented.
The literature shows that deep learning has been employed for various digitisation applications. Amongst the different types of complex engineering diagrams and documents used, there was considerable research attention on P&IDs. Diagrams were sourced from a range of industries such as nuclear (Gao et al. 2020 ), construction (Zhao et al. 2020 ), and oil and gas (Elyan et al. 2020a ). In addition to digitising diagram elements, existing literature showed that deep learning was also used for related diagram analysis purposes. These include creating a diagram search tool (Daele et al. 2021 ), determining the appropriate manufacturing method (Xie et al. 2022 ) and detecting engineering errors (Dzhusupova et al. 2022 ). Data contained within engineering diagrams is of critical importance, and there is potential for deep learning to be used for additional digitisation applications.
2.2 Metrics
Evaluation metrics are calculated using model predictions and the ground truth. The precision, recall and F1 score are calculated using True Positives, False Positives and False Negative detections. Precision is the ratio of True Positives to the number of predicted positives, refer to Eq. 1 . Recall is the ratio of True Positives to the number of actual positives, refer to Eq. 2 . The F1 score combines the previous two metrics and is defined as the harmonic mean of precision and recall, as shown in Eq. 3 .
A True Positive detection is defined using object class and location. Firstly, the predicted symbol class must match that of the ground truth. Secondly, the Intersection Over Union (IOU) (Eq. 4 ) is considered.
Symbol detection methods were also commonly evaluated using the mean Average Precision (mAP). This is defined as the mean of the Average Precision (AP) across all classes, as shown in Eq. 5 . Here \(AP_{\textit{i}}\) is the AP of the i -th class and C is the total number of classes.
The AP for each class is defined as the Area Under the Curve (AUC) of the precision-recall curve. This metric is commonly specified at an IOU threshold of 0.5. Note that other IOU thresholds may be specified, for example the COCO dataset (Lin et al. 2014 ) uses AP @[.5 : .05 : .95], which calculates the average AP at ten different IOU thresholds.
2.3 Symbols
Symbols are considered one of the main drawing elements in engineering diagrams. Examples of symbols are shown in Fig. 3 . Symbol recognition can be a complex task for multiple reasons. Each diagram typically contains numerous symbol instances, for example, one study reported on average 180 symbols per P&ID (Elyan et al. 2020a ). Symbols represent a wide range of equipment types, and consequently, they vary in size and shape. Additionally, there is often a low amount of interclass variation (Paliwal et al. 2021a ; Rahul et al. 2019 ) which can result in difficulty distinguishing between symbol classes, refer to Fig. 4 . Moreover, symbols may be overlapped by other drawing elements (Nurminen et al. 2020 ), shown in varying orientations (Nurminen et al. 2020 ), represented by simple shapes (Ziran and Marinai 2018 ) or even by only a few lines (Rezvanifar et al. 2020 ).

Examples of engineering symbols as shown in the diagram legend
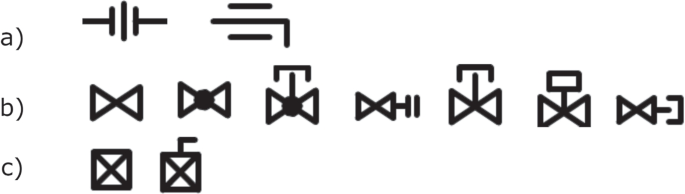
Visually similar symbols from mechanical engineering diagrams: a Union and Butterfly Valve, b gate valve, globe valve, lockable flow control valve, hose-end drain valve, lockshield valve, automatic control valve, valve and capped provision, c flow switch and balancing valve (plug)
Recent literature shows an increasing number of deep learning-based methods for recognising symbols in engineering diagrams, as shown in Table 2 . The most commonly used methods were object detection models. These models predict the location, defined by a bounding box, and the class of objects within an image.
Faster R-CNN (Ren et al. 2015 ) based methods were popular for engineering symbol detection (Ziran and Marinai 2018 ; Nguyen et al. 2021 ; Gao et al. 2020 ; Stinner et al. 2021 ; Hu et al. 2021 ; Joy and Mounsef 2021 ; Sarkar et al. 2022 ; Jakubik et al. 2022 ; Zheng et al. 2022 ). Faster R-CNN is a two-stage object detector presented in 2015. Two related models were published earlier (Girshick et al. 2014 ; Girshick 2015 ). R-CNN (Girshick et al. 2014 ) was created in 2014. The selective search algorithm (Uijlings et al. 2013 ) was used to generate around 2000 region proposals from the input image. CNN features were extracted from each region. These features were then input into class-specific linear SVMs for classification purposes. On the prominent PASCAL Visual Object Classes (VOC) (Everingham et al. 2010 ) dataset, 30% relative improvement was reported over traditional methods based on features such as HOG (Dalal and Triggs 2005 ). However, the method was computationally slow. Separate CNN computation was required for each region proposal. Fast Region-based CNN (Fast R-CNN) (Girshick 2015 ) was presented the following year. The model was designed to speed up computation compared to R-CNN. One convolutional feature map was produced for the whole input image. Then, a feature vector was extracted for each region using a Region of Interest (RoI) pooling layer. Class probabilities and bounding box positions were predicted for each region. Later that same year, Faster R-CNN (Ren et al. 2015 ) was proposed. A Region Proposal Network (RPN) was introduced to speed up the costly region proposal. Convolutional features were shared between the RPN and the downstream CNN.
The feature extraction network used in Faster R-CNN was changed in several studies (Gao et al. 2020 ; Dai et al. 2016 ; Hu et al. 2021 ). For example, Gao et al. ( 2020 ) developed a Faster R-CNN component detection method. A dataset of 68 nuclear power plant diagrams was used. Components were split into three groups based on aspect ratio and scaling factor. These groups were small symbols, steam generator symbols and pipes. A separate model was trained for each group. ResNet-50 (He et al. 2016 ) was used as the feature extractor. ResNet-50 is a type of residual network with 50 layers. The mAP was 96.6%, 98% and 92% for each group. Two other models were evaluated for the detection of the small symbols. The first was Faster R-CNN with Inception (Szegedy et al. 2015 ) network. Although 100% AP was still obtained for certain classes, lower performance was observed overall. A R-FCN model (Dai et al. 2016 ) with ResNet-50 was also evaluated. Dai et al. ( 2016 ) introduced R-FCN in 2016. All trainable layers in R-FCN are convolutional. Faster inference time was reported compared to Faster R-CNN (Dai et al. 2016 ). Although the authors of (Dai et al. 2016 ) reported comparative performance to Faster R-CNN on the PASCAL VOC dataset (Everingham et al. 2007 ), this was not the case on the nuclear power plant diagrams. The reported AP was significantly lower at 16.24%. The authors used publicly available diagrams, which may be simplified compared to those in a real-world scenario.
Hu et al. ( 2021 ) presented an approach to detect the surface roughness symbol from mechanical drawings. A dataset of 3612 mechanical drawings was used. The approach involved symbol detection and text detection. Various object detection models were evaluated. The highest recall and F1 score were reported with Faster R-CNN using ResNet-101 (He et al. 2016 ) in surface roughness detection. The authors used Single Shot Detector (SSD) (Liu et al. 2015 ) with ResNet-50 for localising text and LeNet (Cun et al. 1990 ) for character recognition. An F1 score of 96% was reported. The approach was designed specifically for the surface roughness symbol and may be limited in applicability to a wider range of symbols.
Several engineering diagram studies required the use of a diagram legend (Joy and Mounsef 2021 ; Sarkar et al. 2022 ). For example, Joy and Mounsef ( 2021 ) used a Faster R-CNN method with ResNet-50 for symbol detection in electrical engineering diagrams. First, symbol shapes were obtained using morphological operations to identify symbol grid cells in the legend table. Next, data augmentation was used to increase the available training data. Detection and recognition rates of 83% and above were reported on a small test set of five diagrams. Increasing the training data diversity may help to improve the results. Sarkar et al. ( 2022 ) also used a Faster R-CNN model for symbol detection in engineering drawings. All symbols were treated as belonging to one class. Detected symbols were then assigned a class based on similarity with the symbols in the diagram legend. Two similarity measures were evaluated. The first was based on traditional SIFT (Lowe 2004 ) features. The second employed a CNN as a feature extractor. Better performance was reported using the SIFT-based approach. These studies relied on the use of a diagram legend, however, this may not be available in practice. Moreover, symbols can be present in the diagrams that do not appear in the legend (Sarkar et al. 2022 ).
Yun et al. ( 2020 ) also created an R-CNN-based method for symbol recognition from P&IDs. Ten industry P&IDs were used. Region proposals were generated using image processing methods customised for each symbol type. Positive and negative regions were obtained. The negative regions were divided into classes using negative class decomposition through unsupervised learning models, namely k-means and Deep Adaptive image Clustering (DAC) (Chang et al. 2017 ). Positive regions were assigned classes manually. Results showed that the incorporation of the negative classes reduced false positives. A slight improvement was reported using DAC compared to k-means. This method is rule-based and requires manual adjustment for a different use case.
Faster R-CNN based symbol detection methods were also used on floor plan images (Ziran and Marinai 2018 ; Jakubik et al. 2022 ). For instance, Ziran and Marinai ( 2018 ) presented a Faster R-CNN method for object detection in floor plan images. Two datasets were used. The first contained 135 diverse floor plans obtained from internet search queries. The second consisted of 160 industry floor plans sourced from an architectural firm. Although detailed results of the preliminary experiments were unavailable, improved performance using Faster R-CNN compared to SSD was reported. The initial performance on the first dataset was comparatively low, at 0.26 mAP. Data augmentation and anchor specification increased the mAP to 0.31. For the second, more standardised dataset, the mAP was higher at 0.86. Additionally, the authors used transfer learning to improve performance on the more diverse dataset. The model was pre-trained on the second dataset and then fine-tuned on the first dataset. Performance improved by 0.08 mAP.
Jakubik et al. ( 2022 ) presented a human-in-the-loop system for object detection and classification in floor plans. The symbol detection method was based on Faster R-CNN. A training dataset of 20, 000 synthetic images was created using legend symbols and data augmentation. The test set of 44 industry floor plans was manually annotated with 5907 symbols from 39 classes. An uncertainty score was calculated for each detected and then classified symbol. Symbols were then labelled by a human expert in order of decreasing uncertainty. A range of uncertainty measures was evaluated. Increased accuracy was reported compared to random selection at 50% of the labelling budget, using all but one uncertainty measure.
One-stage object detection models have also been used for engineering symbol detection (Zhao et al. 2020 ; Rezvanifar et al. 2020 ; Elyan et al. 2020a ; Toral et al. 2021 ; Zheng et al. 2022 ). These models are faster than two-stage models. One of the most well-known one-stage object detection models is YOLO (Redmon et al. 2016 ), which was created in 2016. A real-time inference speed of 45 fps was reported. In contrast, the authors of Faster R-CNN (Ren et al. 2015 ) reported a lower processing speed of 5 fps. YOLO is comparatively faster as a single neural network was used to predict bounding boxes and class probabilities. The network had 24 convolutional layers followed by 2 fully connected layers. The input image is divided into a S x S grid. Objects are assigned to the grid cell that contains the object centre. Each grid cell predicts B bounding boxes. The centre of the bounding box is defined relative to the grid cell, whereas the width and height are predicted relative to the whole image. Class-specific confidence scores for each box are also predicted. Several extensions to the initial YOLO version (Redmon et al. 2016 ) were proposed. YOLOv2 (Redmon and Farhadi 2017 ) contained several modifications, including multi-scale training and anchor boxes. The base network, Darknet-19, had 19 convolutional layers. In YOLOv3 (Redmon and Farhadi 2018 ), the bounding boxes were predicted at three different scales. A feature extractor with 53 convolutional layers was used. Newer versions, YOLOv4 (Bochkovskiy et al. 2020 ), YOLOv5 (Jocher et al. 2020 ), YOLOv6 (Li et al. 2022 ) and YOLOv7 (Wang et al. 2022 ) were also proposed. Another one-stage object detection model is SSD (Liu et al. 2015 ). The single network employs multi-scale feature maps for predictions. RetinaNet (Lin et al. 2017 ) is also a one-stage detector. The model was introduced in 2017 and employs the novel focal loss function.
YOLO-based methods have been used for symbol detection in several different diagram types, including structural diagrams (Zhao et al. 2020 ), floor plans (Rezvanifar et al. 2020 ), and P&IDs (Elyan et al. 2020a ). For example, Zhao et al. ( 2020 ) presented a YOLO-based method to detect components in scanned structural diagrams. Five symbol classes were considered. Related semantic information, such as the symbol tag, was included in the symbol bounding box. Data augmentation increased the dataset size from 500 to 1500 images. F1 score of 86.7% and above was reported.
Focusing on architectural floor plans, Rezvanifar et al. ( 2020 ) proposed a YOLOv2 symbol detection method. A private dataset of 115 diagrams was used. Various backbone networks were evaluated. Higher mAP was reported using ResNet-50 compared to Darknet-19 and Xception (Chollet 2017 ). However, detection performance varied widely across the 12 classes considered. For example, the accuracy for the window symbol was 76% compared to 100% for the shower symbol. This may be due to the window symbol’s varying aspect ratio and visual similarity compared to other image components. Additionally, 70 floor plans from the public Systems Evaluation SYnthetic Documents (SESYD) dataset were used. Results improved compared to traditional symbol spotting methods. However, the authors observed that the SESYD diagrams were simpler than typical real-world floor plans. Moreover, there were no intra-class symbol variations. Although YOLOv3 performance was not evaluated, its multi-scale prediction may improve the performance on the relatively small symbols (Redmon and Farhadi 2018 ).
In another study, Elyan et al. ( 2020a ) created methods for symbol detection and classification in P&IDs. A dataset of 172 industry P&IDs from an oil and gas company was used. The symbol detection method was based on YOLOv3. Accuracy was 95% across 25 symbol classes. The authors observed lower class accuracy for the least represented classes. Additionally, a Deep Generative Adversarial Neural Network was presented to handle class imbalance for symbol classification. GAN (Goodfellow et al. 2014 ) are deep learning models designed to generate data. GANs contain two models. These are a generator and a discriminator. A generative model is trained to produce fake data which is indistinguishable from real data by the discriminator. The authors used a Multiple Fake Class GAN (MFC-GAN) (Ali-Gombe and Elyan 2019 ) to generate synthetic instances of the minority class. Experiments showed that realistic synthetic samples were generated. The synthetic instances improved CNN classification. Note that these results were based on using only a few training samples per class. For instance, the Angle Choke Valve class was represented by only two instances in the initial dataset.
A number of researchers used a CNN classifier with a sliding window approach to detect symbols in engineering diagrams (Mani et al. 2020 ; Yu et al. 2019 ). Classifiers predict an object class for a given image. For instance, Mani et al. ( 2020 ) created a classification-based method for extracting two symbol classes from P&IDs. A dataset of 29 P&IDs was used. The sliding window method extracted fixed-size image patches from the diagram. The CNN had three convolutional layers and two fully connected layers. Patches were classified as ‘tag’, ‘Locally Mounted Instrument’ (LMI) or ‘no symbol’. On 11 test diagrams, tags were classified with a precision of 100% and recall of 98%. LMIs were classified with a precision of 85% and recall of 95%. According to the authors, results were poorer for LMIs due to visually similar components.
Yu et al. ( 2019 ) used a similar approach to detect symbols in P&IDs. A dataset of 70 industry P&IDs was used. First, image processing techniques were employed for diagram realignment and to remove the outer border. An AlexNet (Krizhevsky et al. 2012 ) classifier was then used with a sliding window approach. Candidate symbol regions were identified by means of morphological close and open operations. The window size was customised for each symbol class. The symbol recognition accuracy was 91.6%. This method was tested on a limited test set of only two P&IDs. Moreover, the test diagrams contained a simple equipment layout with little interference between components. Whilst promising results were reported in these studies, this method would likely become computationally expensive for a more extensive use case. Although the sliding window approach was frequently used with traditional methods, including Haar cascades (Viola and Jones 2001 ) and Deformable Part Models (Felzenszwalb et al. 2008 ), there is a prohibitive computational cost of classifying each window using a CNN. Moreover, small stride and multi-scale windows are typically required to obtain high localisation accuracy.
Segmentation-based methods have also been used to digitise symbols from engineering diagrams (Paliwal et al. 2021a ; Rahul et al. 2019 ). Rather than predicting a symbol bounding box, segmentation methods generate pixel-level predictions. For instance, Rahul et al. ( 2019 ) created a Fully Convolutional Network (FCN) (Long et al. 2015 ) method to segment 10 symbol classes from P&IDs. The authors used four real-world P&IDs from an oil company. F1 scores of 0.87 and above were recorded. However, the authors reported that their methods’ performance dropped in the presence of visually similar symbols. This was observed in a dataset of P&IDs with a relatively blank background.
Paliwal et al. ( 2021a ) used a combination of methods to recognise symbols in P&IDs. Basic shape symbols were detected using traditional methods, such as Hough transform for circle detection. Complex symbols were localised using an FCN (Long et al. 2015 ) segmentation model and classified using Three-branch and Multi-scale learning Network (TBMSL-Net) (Zhang et al. 2020 ). The methods were evaluated on 100 synthetic P&IDs and a smaller private dataset of 12 real-world P&IDs. An F1 score of 0.820 and above across 32 symbol classes was reported on the synthetic test set. Improved performance compared to Rahul et al. ( 2019 ) was observed on the real-world P&IDs. The use of the Hough transform for basic shapes is unlikely to generalise well across different symbol sizes and appearance variations.
Graph-based methods have been used to recognise symbols in engineering diagrams (Paliwal et al. 2021b ; Renton et al. 2019 , 2021 ). A graph in this context is comprised of nodes connected by edges. For example, Paliwal et al. ( 2021b ) created a Dynamic Graph Convolutional Neural Network (DGCNN) (Wang et al. 2018 ) to recognise symbols in P&IDs. The symbols were represented in graph form and then classified using the DGCNN. Classification accuracy of 86% was recorded on 100 synthetic P&IDs. Symbol misclassifications were observed due to noise and clutter. The method was compared to the FCN based-method presented by Rahul et al. ( 2019 ) on 12 real-world P&IDs, and improved F1 scores were reported for 3 out of 11 classes. Only one instance per class was used to train the DGCNN. To increase the model’s robustness, it was augmented with embeddings from a ResNet-34 network pre-trained on symbols.
Renton et al. ( 2019 ) introduced a GNN method for symbol detection and classification in floor plans. A dataset of 200 floor plans was used. First, the floor plans were converted into Region Adjacency Graphs (RAGs). The nodes represented parts of images, and the edges represented relationships between these parts. Using a GNN, nodes were classified as one of 17 symbol types. This work was developed further in Renton et al. ( 2021 ), when the authors clustered the nodes into subgraphs corresponding to symbols. Here a symbol detection accuracy of 86% was reported.
Mizanur Rahman et al. ( 2021 ) employed a combination of graph-based methods and Faster R-CNN for symbol detection in circuit diagrams. A dataset of 218 diagrams was used. The symbol detection method was based on Faster R-CNN with ResNet-50. Graph methods were then used to refine the model. Detected symbols were graph nodes. Symbol-to-symbol connectors, identified through image processing-based blob detection, were graph edges. Graph Convolutional Networks (GCN) and node degree comparison were used to identify graph anomalies, which were potentially false negative predictions from Faster R-CNN. The Faster R-CNN model was then fine-tuned using the anomaly regions. An improvement in recall between 2 and 4% was reported, although the overall F1 score decreased by up to 3%. Additionally, graph refinement techniques were used to identify incorrectly labelled nodes. However, the recall was reduced by up to 3% compared to Faster R-CNN alone. One drawback of the symbol-to-symbol connection method was that it missed complex connections which looped around a symbol.
Studies on engineering symbols classification are also available in the published literature (Elyan et al. 2020b , 2018 ). For example, Elyan et al. ( 2018 ) presented work on engineering symbols classification. Symbols were classified using Random Forest (RF), Support Vector Machine (SVM) and CNN. Comparable results with all three methods were reported. The authors also applied a clustering-based approach to find within-class similarities. This benefitted RF and SVM performance. However, there was a slight decrease in CNN performance, potentially due to the limited dataset size.
In summary, it can be said that despite the use of state-of-the-art deep learning methods, detecting and recognising symbols in complex documents and engineering drawings continues to be an inherently challenging problem. Many factors contribute to the challenge including symbol characteristics such as a lack of features (Ziran and Marinai 2018 ; Rezvanifar et al. 2020 ), high intra-class variation (Rezvanifar et al. 2020 ) and low inter-class variation (Paliwal et al. 2021a ; Rahul et al. 2019 ). Moreover, the lack of publicly available annotated datasets (Moreno-García et al. 2019 ) increases the difficulty of the task. Consequently, further research is required to improve methods for symbol digitisation from complex diagrams.
Text is another major component that exists in almost all types of engineering diagrams. Text digitisation here involves two stages, first, the detection of the text and second, the recognition of the text. This is illustrated in Fig. 5 . Both the detection and recognition steps are considered challenging for multiple reasons. Each diagram typically contains numerous text strings. For example, Jamieson et al. ( 2020 ) used 172 P&IDs and reported on average 415 text instances per diagram, whilst Francois et al. ( 2022 ) used 330 engineering documents and reported on average 440 text boxes. Unlike text in documents with a specific format, text in complex diagrams can be present anywhere in the drawing (Francois et al. 2022 ), including within symbols (Mani et al. 2020 ). Additionally, these text strings are often shown in various fonts (Rahul et al. 2019 ), printed in multiple orientations (Jamieson et al. 2020 ; Gao et al. 2020 ; Toral et al. 2021 ) and vary widely in length (Francois et al. 2022 ). Moreover, this text is often present in a cluttered environment and can overlap other diagram elements (Kang et al. 2019 ), as is shown in Fig. 6 .
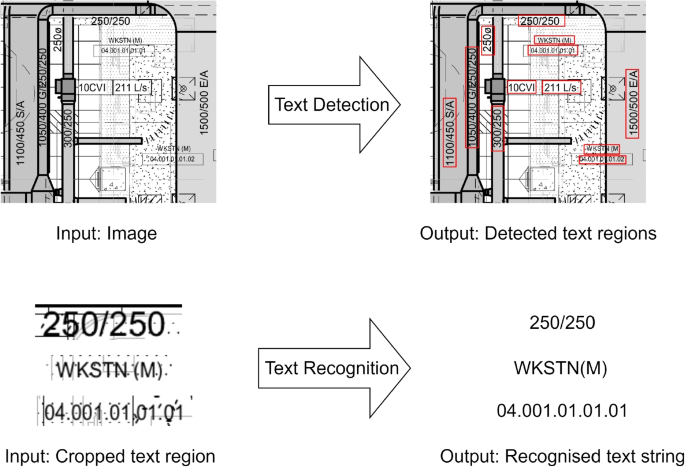
Text digitisation is most commonly approached in recent engineering diagram literature in two steps. Firstly, a text detection model predicts text regions within an image. Secondly, a text recognition model predicts a text string from a cropped text instance
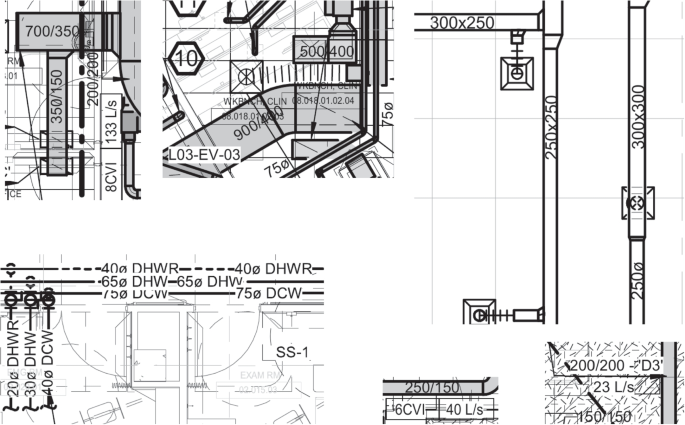
The text within engineering diagrams is commonly shown in multiple orientations, a cluttered environment and overlapped by separate text strings or other shapes
Whilst there has been a considerable amount of research on text digitisation, most of it was focused on scene text (Ye and Doermann 2015 ). Scene text is defined as text that appears in natural environments (Long et al. 2018 ; Liu et al. 2020 ). However, text in undigitised complex documents presents unique challenges that are generally not observed for text in natural scenes. These specific challenges include image degradation (Moreno-García et al. 2018 ) and the presence of multiple visually similar drawing elements. Complex documents often lack colour features that can be used to distinguish text from the background. Moreover, the task is more complicated than digitising text from standard format documents, where text is typically presented in straight lines and composed of known words.
There is a clear shift toward using deep learning-based methods in text digitisation, as shown in a relatively recent extensive review paper (Long et al. 2018 ). Deep learning models automatically extract image features, whereas traditional text methods rely heavily on manually extracted features. For instance, text detection methods commonly used image features based on colour, edge, stroke and texture (Ye and Doermann 2015 ). Specific features used included HOG, Stroke Width Transform, and Maximally Stable Extremal Regions. Two popular traditional text detection methods were based on Connected Components Analysis (CCA) and sliding window classification (Ye and Doermann 2015 ; Long et al. 2018 ). CCA methods extract candidate text components and then filter out non-text regions using heuristic or feature-based methods (Long et al. 2018 ).
Various deep learning models were used to detect text in complex diagrams, as shown in Table 3 . The majority of studies used models designed for text detection, including Character Region Awareness for Text Detection (CRAFT) (Baek et al. 2019 ), Efficient and Accurate Scene Text Detector (EAST) (Zhou et al. 2017 ), Connectionist Text Proposal Network (CTPN) (Tian et al. 2016 ) and SegLink (Shi et al. 2017a ). CRAFT (Baek et al. 2019 ) was designed to localise individual characters, whereas EAST (Zhou et al. 2017 ) uses a FCN to predict word or text line instances from full images. Meanwhile, CTPN (Tian et al. 2016 ) localises text lines, while SegLink (Shi et al. 2017a ) decomposes text into oriented boxes (segments) connected by links.
Object detection models have also been used to detect text in engineering diagrams (Nguyen et al. 2021 ; Hu et al. 2021 ; Toral et al. 2021 ). For example, Nguyen et al. ( 2021 ) created a Faster R-CNN method to detect symbols and text in scanned technical diagrams. A large dataset of 4630 technical diagrams was used. Five classes were considered. Individual characters were recognised from the text regions using a CNN separation line classifier and a CNN character classifier. The average F1 score was 89%, although performance varied across object classes. The lowest F1 score, 78%, was reported for the least represented class. Text recognition exact match accuracy was 68.5%. Toral et al. ( 2021 ) also used an object detection model for text detection. They created a YOLOv5 method to detect pipe specifications and connection points. Pipe specifications are text strings with a specific format, whereas the connection point symbol contains a short text string. A heuristic method was applied to the detected object regions to obtain text regions. The text was recognised using Tesseract. Detection and recognition accuracy of 93% and 94% was reported. Rumalshan et al. ( 2023 ) presented methods for component detection in railway technical maps. The components were a combination of text codes and simple shapes. Their Faster-RCNN method outperformed YOLOv3 and SSD methods. Seeded region growing (Adams and Bischof 1994 ) was used to preprocess the detected regions prior to OCR. White pixels at the edge of the regions were the seeds.
Whilst there is a range of deep learning models designed for text recognition, a popular choice was to use Tesseract software (Smith 2007 ), as shown in Table 3 . The latest versions of this employ deep learning. Deep learning text recognition models can be considered segmentation-based or segmentation-free methods (Chen et al. 2021 ). Segmentation methods generally contain preprocessing, character segmentation and character recognition steps. In contrast, segmentation-free approaches predict a text string from the entire text instance. For example, these methods may comprise image preprocessing, feature extraction, sequence modelling, and prediction steps (Chen et al. 2021 ). Sequence modelling considers contextual information within a character sequence. A type of Recurrent Neural Network (RNN) known as a Bi-directional Long-Short Term Memory (LSTM) Network is often used. The two main prediction methods are attention based (Bahdanau et al. 2015 ) and Connectionist Temporal Classification (CTC) (Graves et al. 2006 ). One example of a deep learning text recognition method is the Convolutional Recurrent Neural Network (CRNN) (Shi et al. 2017b ). It combines a CNN, an RNN and a transcription layer.
Engineering diagrams may contain symbols and shapes that are visually similar to text. This was reported in a study by Jamieson et al. ( 2020 ). Here, the authors built a framework to digitise engineering drawings. They used EAST (Zhou et al. 2017 ) to localise text and LSTM-based Tesseract (Smith 2007 ) for text recognition. Good performance was achieved overall with 90% of text instances detected. However, false positives were predicted for shapes visually similar to text, including dashed lines and symbol sections. Yu et al. ( 2019 ) also reported a similar challenge. They used a CTPN (Tian et al. 2016 ) based method to detect text in P&IDs. Character recognition accuracy was 83.1%. Although the two test diagrams used had a simple equipment layout, part of a symbol was recognised as a character.
Another challenging problem with text digitisation is the orientation of the text. This was reported in several studies (Kim et al. 2021b ; Gao et al. 2020 ; Paliwal et al. 2021a ), and various methods were proposed to handle it. For example, Kim et al. ( 2021b ) created methods to recognise symbols and text in P&IDs. The text was detected using the easyOCR Footnote 1 framework and recognised using Tesseract (Smith 2007 ). EasyOCR is based on CRAFT (Baek et al. 2019 ) and CRNN methods. Text rotation was estimated based on aspect ratio and text recognition score. Text detection and recognition combined precision and recall were 0.94 and 0.92, respectively. The authors used P&IDs that contained no noise or transformations, however this is not necessarily the case in practice (Moreno-Garcia and Elyan 2019 ). Text digitisation methods were also applied on rotated diagrams (Gao et al. 2020 ; Paliwal et al. 2021a ). For instance, Paliwal et al. ( 2021a ) proposed methods to digitise P&IDs. First, the text was detected using CRAFT and recognised using Tesseract. Then, the diagram was rotated and the process was repeated to capture missing vertical text strings. Text detection and recognition accuracy of 87.18% and 79.21% was reported.
Another key challenge is that text in engineering diagrams is often composed of codes rather than known words. This differs from the text in other document types, which typically belongs to a specific lexicon. Rahul et al. ( 2019 ) used prior knowledge of the text structure when they digitised pipeline codes from P&IDs. The method was based on a CTPN model (Tian et al. 2016 ) and Tesseract. Text detection accuracy was 90%. The pipeline codes had a fixed structure, which was used to filter out false positive text strings. However, complex diagrams contain text for numerous reasons, and details of the various structures are not always available.
Francois et al. ( 2022 ) proposed a correction method for recognised text. The dataset comprised 330 industry engineering documents, including P&IDs and isometrics. Their text method was based on the EAST model (Zhou et al. 2017 ) and Tesseract. A post-OCR correction step involved text clustering using affinity propagation. The Levenshtein distance was used as the similarity measure. Clusters were defined to maximise the similarity score between data points. The post-OCR correction improved tag recognition from 75 to 82%. However, the application of this method to other scenarios relies on the text character structure being known in advance.
Text digitisation from complex engineering diagrams remains challenging. Although text detection and recognition has received large research interest (Long et al. 2018 ; Ye and Doermann 2015 ; Chen et al. 2021 ), the majority was focussed on scene text (Ye and Doermann 2015 ). The literature shows that text within engineering diagrams presents different challenges. In engineering diagrams, the text can be present anywhere in the image (Francois et al. 2022 ), of multiple orientations (Jamieson et al. 2020 ), and is frequently overlapped by other shapes. One particular challenge for deep learning models is distinguishing text from other similar shapes in the diagram (Jamieson et al. 2020 ; Yu et al. 2019 ). Moreover, compared to other domains, there is a lack of publicly available annotated text datasets. Further research is necessary to enable accurate text detection and recognition from complex engineering diagrams.
2.5 Connectors
Connectors in engineering diagrams represent the relationship between symbols. The simplest representation of a connector is a solid line, which typically represents a pipeline. More complex line types such as dotted lines and dashed lines are also used, which represent specialised connectors such as electrical signal or air lines. Examples of different connectors can be seen in Fig. 7 . Although connector extraction may seem a simple task, it can be difficult for computer vision methods to distinguish between connectors and other shapes in the diagram. This problem occurs as all diagram elements are essentially composed of lines. For instance, the character ‘l’ may also be considered a short line. Methods to overcome this challenge and accurately digitise connectors are required, as their information is vital for understanding the flow through a system.
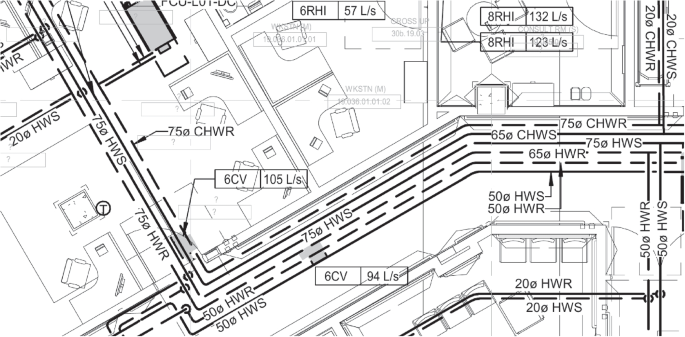
Section of engineering diagram showing different line representations
Despite the recent advances in deep learning, methods employed for line detection are still primarily based on traditional approaches (Rahul et al. 2019 ; Stinner et al. 2021 ; Yu et al. 2019 ; Kang et al. 2019 ). For instance, Yu et al. ( 2019 ) introduced methods for line recognition in P&IDs. First, image processing techniques were employed for diagram realignment and to remove the outer border. A series of image processing methods was used for line recognition. This involved determining the most common line thickness. Reported accuracy was 90.6%. The authors reported that symbol sections were recognised as lines. Difficulty in recognising dotted and diagonal lines was also reported in this study. This was observed even in a very limited test set of only two P&IDs which contained a simple equipment layout with little interference between components. Kang et al. ( 2019 ) also used a traditional method for line extraction from P&IDs. Lines were extracted based on the symbol connection point and sliding window method. Particular difficulties recognising diagonal and separated lines were reported.
Other traditional line extraction methods include those based on the Hough transform or kernels. In a study by Stinner et al. ( 2021 ), lines were detected using binarisation and Hough transform. Line crossings were detected using a line intersection algorithm. Meanwhile, Rahul et al. ( 2019 ) used the more efficient Probabilistic Hough Transform (PHT) (Kiryati et al. 1991 ) to detect pipelines in P&IDs. Although the P&IDs appear to have a relatively blank background, the pipeline detection accuracy, 65%, was still effected by noise and overlapping drawing elements. In the kernel-based method, a small filter is passed over the diagram and a convolution operation is applied. Paliwal et al. ( 2021a ) used a kernel-based method to detect lines in P&IDs. A higher detection accuracy for complete lines (99%) than for dashed lines (83%) was reported. The authors considered the line width and image spatial resolution when designing the structuring element matrix. It should be noted, however, that kernel-based methods are very sensitive to noise and the thickness of lines.
Although not commonly seen in the literature, line detection may be considered as an object detection problem. This approach was employed by Moon et al. ( 2021 ) in their study on line detection in P&IDs. A dataset of 82 remodelled industry P&IDs was used. First, the P&ID border was removed using binarisation, pixel processing and morphological operations. A RetinaNet (Lin et al. 2017 ) object detection model was used to detect flow arrows and specialised line types, such as electrical signal lines. These lines were composed of either a line with a shape overlaid, or a series of dashes. In the latter case, each dash was treated as an object. A post-processing step was needed to merge the detected line sections. Continuous lines were detected using traditional image processing methods, including line thinning and Hough transform. Symbol and text regions detected using the method created by Kim et al. ( 2021b ) were removed to discard false-positive lines. A precision of 96.1% and recall of 89.6% was reported. The dataset was imbalanced, although the results showed that highest performance was not always obtained for the most represented class.
Connector detection is also considered a challenging problem. Despite the recent popularity of deep learning digitisation methods for symbols and text, this is not the case for connector digitisation methods. Methods used for this task are still primarily based on traditional approaches (Rahul et al. 2019 ; Kang et al. 2019 ; Stinner et al. 2021 ). Such approaches include the Hough transform, Probabilistic Hough Transform (Kiryati et al. 1991 ) and kernel-based methods. Furthermore, the scale of the problem is increased as multiple line types can be present in one diagram (Moon et al. 2021 ; Rahul et al. 2019 ; Kang et al. 2019 ). Distinguishing connectors from other shapes in the diagram can be difficult for computer vision methods. Moreover, there is a lack of connector-labelled datasets for use with deep learning models. Therefore, accurate connector detection from complex engineering diagrams remains difficult, and improved methods are required.
3 Challenges
Although there are numerous benefits of using deep learning methods for diagram digitisation, such as their generalisability to the variations seen in the drawings and automatic feature extraction, the existing literature also suggests various challenges. These are a lack of public datasets, data annotation, evaluation, class imbalance and contextualisation. Compared to traditional methods, deep learning methods typically require large quantities of training data. Due to proprietary and confidentiality reasons, diagram datasets are generally not available in the public domain. Furthermore, when datasets can be obtained, they typically need to be labelled for use with supervised deep learning models. The lack of annotated datasets increases the difficulty of evaluating digitisation methods. The fourth challenge arises from the fact that while deep learning models are typically designed for balanced datasets, engineering diagram datasets are inherently imbalanced. A detailed discussion of these challenges is presented in this section.
3.1 Datasets
The lack of publicly available engineering diagram datasets makes it difficult to compare and benchmark various methods. As can be seen in Table 4 , most methods are evaluated using proprietary datasets. It should also be pointed out that there is a vast variety of formats for these drawings. Specific organisations or even specific projects may adopt their own drawing formats, which would not be captured in publicly available datasets. This means that retraining models to suit specific engineering drawing datasets is an important and necessary factor to consider. One example of a public dataset used in the digitisation literature is the Systems Evaluation SYnthetic Documents (SESYD) floor plan dataset (Rezvanifar et al. 2020 ). However, this dataset is synthetic, contained no intra-class symbol variations and was considered simpler than typical real-world floor plans (Rezvanifar et al. 2020 ). Moreover, researchers working on floor plan digitisation still report a lack of available training data (Ziran and Marinai 2018 ).
Synthetic diagrams have been utilised in the absence of sufficient real-world data (Paliwal et al. 2021a ; Sierla et al. 2021 ; Nurminen et al. 2020 ; Haar et al. 2023 ; Bickel et al. 2021 ). For instance, Paliwal et al. ( 2021a ) generated a dataset comprising 500 annotated synthetic P&IDs. Image noise was added. The dataset contained 32 equally represented symbol classes. However, class imbalance is inherent in real-world P&IDs and can cause models to be biased towards overrepresented classes. Sierla et al. ( 2021 ) included data extraction from scanned P&IDs as a step in their methodology for the semi-automatic generation of digital twins. YOLO was used for symbol detection. The authors generated artificial images by placing symbols from process simulation software on a white background. However, these images were relatively simple and did not present the challenges associated with scanned P&IDs. Similarly, Nurminen et al. ( 2020 ) created artificial images using process simulation software. They created a YOLOv3-based model for symbol detection in P&IDs. The method was evaluated on artificial images and scanned industrial P&IDs. Meanwhile, Bickel et al. ( 2021 , 2023 ) generated synthetic training data for symbol detection in principle sketches. They used a fixed set of rules to generate symbols, which was practical in this case owing to the defined representation limits of the drawings used.
Stinner et al. ( 2021 ) used images from symbol standards and internet search images to increase the training dataset size. They presented work on extracting symbols, lines and line crossings from P&IDs. The authors used five industry P&IDs. They used a Faster R-CNN-based method to detect four symbol types. The authors reported 93% AP over all symbol classes. However, performance was lower for certain object classes compared to others.
Haar et al. ( 2023 ) presented symbol and text detection methods for engineering and manufacturing drawings. A dataset of 15 real drawings and 1000 synthetic images was used. Synthetic data was generated by cropping symbols from the real drawings and randomly placing them on the basic drawings with varying orientations and sizes. YOLOv5 was used to detect symbols. EasyOCR was used for the text. The model utilised VGG and ResNet for feature extraction, LSTM and CTC. The YOLOv5 model performance on the real diagrams (36.4 mAP) was lower than on the synthetic dataset (87.6 mAP). The text method was evaluated on five diagrams and correctly recognised 68% of text characters. Mathematical special characters and rotated texts were highlighted as a challenge.
Although there is a lack of text datasets for engineering diagrams, many text datasets exist in other domains. In 2015, commonly used text datasets were discussed in a review (Ye and Doermann 2015 ). The largest dataset mentioned was IIIT5K Word (Mishra et al. 2012 ), which contains 5, 000 cropped images. Since then, demand for significantly bigger datasets to train deep learning models has increased. Today, the largest text datasets contain millions of synthetic text instances (Chen et al. 2021 ). For example, Synth90K (Jaderberg et al. 2014 ) contains 9 million synthetic annotated text instances. The Unreal text dataset (Long and Yao 2020 ) comprises 12 million cropped text instances. In contrast, realistic text datasets are smaller, containing thousands of data samples (Chen et al. 2021 ). Veit et al. ( 2016 ) introduced the COCO-Text dataset in 2016. The dataset contained over 173k annotated instances of text in natural images, making it the largest dataset of its type at the time. The International Conference for Document Analysis and Recognition (ICDAR) also introduced text datasets (Karatzas et al. 2013 , 2015 ).
The literature shows an urgent need to have more engineering diagram datasets available in the public domain. Most of the proposed digitisation methods were evaluated on proprietary datasets, which may contain a limited number of diagrams (Hantach et al. 2021 ; Yu et al. 2019 ). Although synthetic datasets were also used, these diagrams were typically simple in appearance and not as complex as those in the real-world (Rezvanifar et al. 2020 ; Sierla et al. 2021 ). Public access to diagram datasets would also allow for improved comparison between proposed methods. Therefore, the release of public datasets is crucial to accelerate research and development in the area of engineering diagram digitisation.
3.2 Data annotation
Obtaining sufficient annotated data is also regarded as a challenge. When datasets are available, they must be annotated for use with supervised deep learning models. Typically, a large annotated dataset is required for training purposes (Jakubik et al. 2022 ). Acquiring such data is usually carried out manually. Various software can be used to facilitate this, such as Sloth, Footnote 2 LabelImg Footnote 3 and LabelMe (Russell et al. 2008 .). For example, to obtain a symbol dataset, the user needs to draw a bounding box around the symbol and then label it with the relevant class. These steps are required for every symbol of interest in the diagram. Given the high number of symbols per diagram, the process is very time-consuming, costly and prone to human error. Furthermore, given the technical nature of these drawings, a subject matter expert is normally required to complete this task.
One method to reduce the required labelling effort is to create synthetic training data (Gao et al. 2020 ; Bin et al. 2022 ; Gupta et al. 2022 ). The simplest approach is to use traditional image processing algorithms. For instance, Gao et al. ( 2020 ) presented a method for component detection in nuclear power plant diagrams. They manually annotated symbols and then used traditional data augmentation techniques, such as image resizing, to increase the training symbol instances (Gao et al. 2020 ). The AP increased from 40 to 82% when the training dataset increased from 100 to 1000 images. Gupta et al. ( 2022 ) created a YOLOv2 method for valve detection in P&IDs. A dataset of three P&IDs was used. Synthetic training data was generated by cropping a symbol and randomly placing it on the background. Experiments showed that model performance improved when the amount of background and similar symbols in the training data was increased. However, evaluation of more than one symbol type and one test diagram is required to determine if the method can be applied to other scenarios.
Synthetic training data was also created using generative deep learning models (Bin et al. 2022 ; Khallouli et al. 2022 ). For example, Bin et al. ( 2022 ) used a method based on CycleGAN (Zhu et al. 2017 ) and CNN for P&ID symbol recognition. A dataset of seven P&ID sheets was used. CycleGAN (Zhu et al. 2017 ) uses unpaired images. The accuracy improved from 90.75 to 92.85% when equal representations of synthetic to authentic samples were used for training. However, the authors reported that the performance gain decreased with a 2:1 ratio of synthetic to authentic samples, as an accuracy of 91.88% was reported. Khallouli et al. ( 2022 ) presented work on OCR from industrial engineering documents. Nine drawings of ships were used. They used a method based on ScrabbleGAN (Fogel et al. 2020 ) to generate synthetic word images. The model contains a generator, discriminator and text recogniser. When the synthetic data was added to manually labelled training data, the character recognition accuracy increased from 96.83 to 97.45% and the word recognition accuracy increased from 88.79 to 92.1%.
Most of the relevant literature used supervised deep learning, which learns from labelled training data. An alternative approach is semi-supervised learning, which uses both labelled and unlabelled data (Van Engelen and Hoos 2020 ). In contrast, weakly supervised methods use partially labelled data. For example, weakly supervised object detection methods mostly use image-level labels (Zhang et al. 2022 ). In the area of scene text detection, Liu et al. ( 2020 ) presented a semi-supervised method named Semi-Text. ICDAR 2013 (Karatzas et al. 2013 ), ICDAR 2015 (Karatzas et al. 2015 ) and Total-Text (Ch’ng and Chan 2017 ) datasets were used. A Mask R-CNN based model was pre-trained on the SynthText dataset (Gupta et al. 2016 ). Then, positive samples were obtained by applying the model to unannotated images. The model was then retrained using a dataset of positive samples and SynthText data. The performance improved compared to the baseline model.
Data annotation continues to be largely carried out manually, which proved to be extremely time-consuming and costly. Furthermore, as the diagrams are highly technical, identifying the different symbol classes within a diagram typically requires a domain expert. Therefore, improved methods to speed up the data annotation process, or reduce the need for annotated data, are required.
3.3 Evaluation
Evaluating deep learning methods for complex document digitisation is considered a complex task. Methods used for symbols, text and connectors must all be evaluated separately. Moreover, multiple different metrics are used for the same task. For instance, symbol digitisation methods are evaluated with various metrics including precision, recall, F1 score and mAP. The lack of standard evaluation protocol, along with the use of disparate datasets, increases the difficulty of thoroughly comparing proposed methods.
Symbol detection methods define a True Positive at a specific IOU threshold. The PASCAL (Everingham et al. 2010 ) evaluation metric was often used in the related work (Jakubik et al. 2022 ). This defines a correct detection if the IOU is over a threshold of 0.5. More stringent criteria to define a correct detection were also seen. For instance, Rezvanifar et al. ( 2020 ) defined a correct detection if the IOU was over 0.75. Meanwhile, Paliwal et al. ( 2021a ) defined a correct symbol detection based on an IOU greater than 0.75 and a correct associated text label. Different symbol evaluation metrics may be used in the case of graph-based methods. For example, Renton et al. ( 2021 ) used a GNN for symbol detection and classification. They defined a correct detection if all the symbol nodes representing a symbol were found without any extra node.
Evaluation of diagram digitisation methods is further complicated as the ground truth information is often unavailable. This is a particular issue for the evaluation of text and connector digitisation methods. Manually labelling these components would require substantially more effort than symbol annotation. Therefore, the current evaluation of text and connector digitisation methods is generally subjective (Mani et al. 2020 ). For instance, Mani et al. ( 2020 ) used EAST (Zhou et al. 2017 ) and Tesseract to digitise text in a set of industry P&IDs. They presented sample output detection and recognition results, however evaluation metrics were not used. Objective evaluation methods were used for text and connector digitisation in a limited number of cases. This occurred when ground truth data was available owing to the use of digital (Francois et al. 2022 ) or synthetic diagrams (Paliwal et al. 2021a ). For example, Paliwal et al. ( 2021a ) created a synthetic dataset of 500 P&IDs. The ground truth data of horizontal and vertical line locations, text locations and text strings were available. Their digitisation methods were evaluated on 100 synthetic P&IDs and a smaller private dataset of 12 real-world P&IDs. However, the text and lines methods were objectively evaluated on the synthetic dataset only. The text was considered correct if the string exactly matched the ground truth. Francois et al. ( 2022 ) used text locations extracted from PDF engineering documents as the ground truth. A detection was considered correct if the predicted area corresponded to the ground truth area within an acceptable margin of 10 pixels.
The performance of text recognition methods can be objectively measured by comparing the predicted string to the ground truth. This was seen in cases where digital or synthetic diagrams were used, or for a subset of the text. For instance, Nguyen et al. ( 2021 ) extracted two specific text strings from technical diagrams. They applied the Exact Match accuracy for text recognition. The text was considered to be correct if it exactly matched the ground truth. In another study, Kim et al. ( 2021b ) used digital P&IDs for which the text ground truth metadata was available. In addition to text detection precision and recall, Kim et al. ( 2021b ) also evaluated the combined text detection and recognition performance. More specifically, they used the Character Level Evaluation (CLEval) (Baek et al. 2020 ) metric to obtain precision and recall scores that combined text detection and recognition. CLEval (Baek et al. 2020 ) employs both instance matching and character scoring. Meanwhile, Khallouli et al. ( 2022 ) evaluated their text recognition method using three metrics. These were character recognition rate, word recognition rate and average Levenshtein distance. The latter metric is the number of character edits (such as substitution, insertion or deletion) required to alter the predicted text to the ground truth text.
3.4 Class imbalance
Class imbalance occurs when one or more classes are over-represented in a dataset. It is inherent in engineering diagrams as equipment types are represented with varying frequencies. The problem of class imbalance is known to occur in both deep learning and traditional machine learning (Buda et al. 2018 ). Learning algorithms trained on imbalanced data are typically biased towards the majority class, which causes minority class instances to be classified as majority classes (Johnson and Khoshgoftaar 2019 ).
Class imbalance was shown to occur in both engineering symbols classification and detection (Elyan et al. 2020b , a ; Kim et al. 2021b ; Ziran and Marinai 2018 ). An example is the work presented by Elyan et al. ( 2020b ), which showed that class imbalance effected the CNN classification performance of a P&ID symbols dataset. Lower performance on underrepresented classes compared to overrepresented classes was reported. In work on object detection, Elyan et al. ( 2020a ) created a YOLOv3 (Redmon and Farhadi 2018 ) based method for symbol detection of an imbalanced dataset. Overall accuracy was high at \(95\%\) , although it varied across classes. A class accuracy of 98% for the majority class with 2810 instances was reported, whereas the accuracy for the minority classes with only 11 instances was 0%.
Similarly, Kim et al. ( 2021b ) reported comparable results in their study on P&ID symbol detection. In particular, a lack of data for large symbols was reported. Lower class-accuracies were observed for underrepresented instances. Ziran and Marinai ( 2018 ) also recorded imbalanced symbol distribution in two floor plan datasets. Interestingly, class representation was not strictly correlated with the performance of the Faster R-CNN based model. The highest precision and recall values were not all for the most represented classes. This may be due to the high within-class diversity in the majority classes.
3.5 Contextualisation
In a previous review (Moreno-García et al. 2019 ), authors defined contextualisation as the process of converting the digitised information (i.e. the shapes detected by the computer vision algorithms) into structured information, which can be used to better explore, manipulate or redraw the diagrams in more interactive and representative ways. In this subsection, we discuss the most common solutions in literature that have been presented for this purpose. We have split the contextualisation challenge into three sub-challenges: (1) the storing challenge , where systems have to be devised in order to save the structural representation in an easy to read/access manner, (2) the connectivity challenge , which refers to how the digitised objects are arranged in from their spatial representation in a way that users are able to know how symbols are connected and (3) the matching challenge , in which we address the issue of how to use these structural representations for real-life purposes, such as finding certain sections within a larger drawing, localising which portions of the drawing have relation to a 3D representation (i.e. the real facility or a digital twin), and ensuring consistency of the structural representation by inspecting it in semi-automated ways.
Since the earliest stages of P&ID digitisation, researchers have realised the need to convert the digitised information into some sort of structural graph representation to address the storing challenge. In the 90s, Howie et al. ( 1998 ) proposed a symbolic model output with each of the shapes (symbols and pipes) as a node, and edges connecting them. This means that, despite pipes being connectors within the drawing, these should be represented as another node, as pipes themselves have their own attributes. A toy example is presented in Fig. 8 .

Left: A snippet of a P&ID with two shapes connected by a pipe. Right: The structural graph representation as proposed in Howie et al. ( 1998 )
To address both the connectivity and storing challenges simultaneously, other authors have used the notions of graphs to find the connectivity between the symbols, bypassing the line detection. For instance, Mani et al. ( 2020 ) used graph search to discover symbol to symbol connections in a P&ID. Each pixel was represented as a node, and links between neighbouring pixels were represented as graph edges. Then, symbol to symbol connections were determined using a depth-first search starting a symbol node. This approach results interesting when drawings have a high quality and the algorithm can traverse from one symbol to another with relative ease. This system relies on connectors not overlapping with each other (since the graph search algorithm could be confused by the direction to take) and thus, have limited applicability when the drawing is complex and presents an entangled connector structure.
There are a handful of applications found in literature to address the matching challenge. For instance, Wen et al. ( 2017b , 2017a ) presented a system to measure 2D–3D process plant model similarities based on their topological distribution, establishing a relation between a 2D engineering drawing and a 3D hydrocarbon plant model. To do this, each model was extracted as a graph, and then the feature similarity is calculated to measure a degree of matching between the two models using a geometric deformation invariant algorithm. Contrary to most of the literature reviewed in this study, authors used a type of CAD drawing called ISO drawing, which is relatively easier to digitise compared to classical engineering drawings mentioned before (e.g. P&IDs) since it is more standardised and contains far more measurements and indicators. Still ISO drawings require vast knowledge and field experience to be correctly digitised and, therefore, the extraction of the attributed graph is done in a semi-automated way. Regarding the 3D plant, extracting the attributed graph is easier since the 3D model is still contained in a CAD file which retains all the meta-data needed for this reconstruction.
Rantala et al. ( 2019 ) also applied graph matching techniques to better use plant design information from older designs. Authors performed a review of graph matching techniques and evaluated six algorithms using an illustrative dataset built for purpose. In their evaluation, authors concluded that an algorithm based on simulated annealing with a certain combination of parameters was the best option for this task, as it was capable to detect spurious and inexact correlations. Later on, Sierla et al. ( 2020 , 2021 ) presented related work on automatic generation of graphs from P&IDs. In this study the input was a P&ID represented in XML format, which was able to be converted into an attributed graph. To this end, authors used a recursive algorithm which also relies in pictures taken from the actual facilities, but that reconstruct the graph with an increased accuracy.
In more recent work presented by Rica et al. ( 2020 , 2021 ), authors propose graph embeddings which are used to train NNs on how to distinguish local substructures which may be incorrect, this reducing the human effort on performing manual validation of the digitised information. To this end, authors first construct the graphs based on proximity information provided by the digitisation module, and then learn the most common substructures that can be found in the particular drawing set. For instance, a drawing may depict three valves connected in a loop, but no more than that. Afterwards, a GNN is trained to retain this information and validate the drawings. As in most graph-based problems, the complexity of this review increases with the size of the graph; therefore, authors tested this method in a smaller dataset.
4 Conclusion and future directions
Significant progress has taken place in the area of processing and analysing engineering diagrams and complex documents. This includes aspects such as symbol detection, text recognition, and contextualisation. A wide variety of deep learning models were used, for instance the literature shows that symbol digitisation methods are not only based on object detectors but also segmentation, classification and graph approaches. Meanwhile text digitisation methods were based on both specialised text methods and object detectors. Methods for connector detection have received comparatively less attention than symbol and text methods. Only 21% of the reviewed papers presented a method for connector detection. Overall, deep learning methods used for digitisation have proved to be beneficial compared to traditional methods and result in improved performance.
However, further research is still required to solve the timely and challenging problem of complex engineering diagram digitisation. Improved methods are still needed for all diagram components, namely symbols, text and connectors. Newly developed deep learning models such as transformers (Dosovitskiy et al. 2020 ) maybe of benefit to engineering drawing digitisation, such as in recent related work on CAD drawings (Fan et al. 2022 ).
The literature shows that engineering diagram digitisation is still regarded as challenging. This can be attributed to several factors including diagram complexity, visually similar drawing components (Kim et al. 2021a ; Mani et al. 2020 ), large intra-class variance (Rezvanifar et al. 2020 ) and low inter-class variance (Paliwal et al. 2021a ; Rahul et al. 2019 ), amongst others. The remaining key challenges for engineering diagram digitisation were identified as dataset acquisition, data annotation, imbalanced class distribution, evaluation methods and contextualisation. Although methods such as synthetic data generation and data augmentation exist, the literature suggests that further work is needed to address the specific challenges of engineering drawing digitisation.
Therefore, the first and most important need in this area is to develop and release datasets to the public domain to accelerate research and development. Real-world datasets are typically confidential however, datasets released publicly should ideally be of similar complexity and contain properties such as noise, overlapping elements and a wide range of symbols. Furthermore, allowing researchers to use standard datasets would facilitate benchmarking of proposed methods.
Another area that requires improvement is the data annotation process, which is typically time-consuming and consequently costly. One potential research direction that aims to reduce the amount of required labelled data is active learning. These algorithms aim to choose the most informative samples from the unlabelled data (Ren et al. 2021 ). Labelling only the most informative samples could reduce the amount of data required to train the learning algorithm, reducing the effort required compared to random labelling.
An additional suggestion to reduce the annotation requirement is to include synthetic images in the training data. This was seen in the literature through various methods, including specialist engineering visualisation software (Kim et al. 2021b ) and image processing data augmentation techniques (Gao et al. 2020 ; Joy and Mounsef 2021 ; Ziran and Marinai 2018 ; Jakubik et al. 2022 ). Another method that has been explored is the use of deep learning generative models such as GAN-based approaches (Bin et al. 2022 ; Elyan et al. 2020a ; Khallouli et al. 2022 ). For the synthetic images to be of the most benefit, they should closely represent the real-world data.
An alternative approach that could reduce the reliance on labelled data is to use methods other than supervised learning. One possible solution is the use of semi-supervised methods. These methods are designed to learn from both labelled and unlabelled data (Van Engelen and Hoos 2020 ). Another potential future research direction is the use of deep learning methods that learn from a few instances. This could be of particular use given the frequent presence of underrepresented and rare symbols within engineering diagrams. State-of-the-art methods such as few-shot learning are suggested. Unlike supervised learning models, which typically require vast amounts of labelled training data, few-shot methods aim to learn from only a few samples (Antonelli et al. 2022 ).
https://github.com/JaidedAI/EasyOCR/ .
https://sloth.readthedocs.io/en/latest/ .
https://github.com/tzutalin/labelImg .
Ablameyko S, Uchida S (2007) Recognition of engineering drawing entities: review of approaches. Int J Image Graph 7:709–733. https://doi.org/10.1142/S0219467807002878
Article Google Scholar
Adams R, Bischof L (1994) Seeded region growing. IEEE Trans Pattern Anal Mach Intell 16(6):641–647. https://doi.org/10.1109/34.295913
Ali-Gombe A, Elyan E (2019) MFC-GAN: class-imbalanced dataset classification using multiple fake class generative adversarial network. Neurocomputing 361:212–221. https://doi.org/10.1016/j.neucom.2019.06.043
Antonelli S, Avola D, Cinque L et al (2022) Few-shot object detection: a survey. ACM Comput Surv. https://doi.org/10.1145/3519022
Baek Y, Lee B, Han D et al (2019) Character region awareness for text detection. In: 2019 IEEE/CVF conference on computer vision and pattern recognition (CVPR), pp 9357–9366. https://doi.org/10.1109/CVPR.2019.00959
Baek Y, Nam D, Park S et al (2020) Cleval: Character-level evaluation for text detection and recognition tasks. In: 2020 IEEE/CVF conference on computer vision and pattern recognition workshops (CVPRW), pp 2404–2412. https://doi.org/10.1109/CVPRW50498.2020.00290
Bahdanau D, Cho K, Bengio Y (2015) Neural machine translation by jointly learning to align and translate. In: Bengio Y, LeCun Y (eds) 3rd International conference on learning representations, ICLR 2015, San Diego, CA, USA, 7–9 May 2015, conference track proceedings. arXiv:1409.0473
Bay H, Tuytelaars T, Van Gool L (2006) SURF: speeded up robust features. In: European conference on computer vision. Springer, Berlin, pp 404–417
Bhanbhro H, Hooi YK, Hassan Z et al (2022) Modern deep learning approaches for symbol detection in complex engineering drawings. In: 2022 International conference on digital transformation and intelligence (ICDI), pp 121–126. https://doi.org/10.1109/ICDI57181.2022.10007281
Bickel S, Schleich B, Wartzack S (2021) Detection and classification of symbols in principle sketches using deep learning. Proc Des Soc 1:1183–1192. https://doi.org/10.1017/pds.2021.118
Bickel S, Goetz S, Wartzack S (2023) From sketches to graphs: a deep learning based method for detection and contextualisation of principle sketches in the early phase of product development. Proc Des Soc 3:1975–1984
Bin OK, Hooi YK, Kadir SJA et al (2022) Enhanced symbol recognition based on advanced data augmentation for engineering diagrams. Int J Adv Comput Sci Appl. https://doi.org/10.14569/IJACSA.2022.0130563
Bochkovskiy A, Wang CY, Liao HYM (2020) Yolov4: optimal speed and accuracy of object detection. arXiv preprint. arXiv:2004.10934
Buda M, Maki A, Mazurowski MA (2018) A systematic study of the class imbalance problem in convolutional neural networks. Neural Netw 106:249–259. https://doi.org/10.1016/j.neunet.2018.07.011
Chang J, Wang L, Meng G et al (2017) Deep adaptive image clustering. In: 2017 IEEE International conference on computer vision (ICCV), pp 5880–5888. https://doi.org/10.1109/ICCV.2017.626
Chen X, Jin L, Zhu Y et al (2021) Text recognition in the wild: a survey. ACM Comput Surv. https://doi.org/10.1145/3440756
Ch’ng CK, Chan CS (2017) Total-text: a comprehensive dataset for scene text detection and recognition. In: 2017 14th IAPR international conference on document analysis and recognition (ICDAR). IEEE, pp 935–942
Chollet F (2017) Xception: deep learning with depthwise separable convolutions. In: 2017 IEEE conference on computer vision and pattern recognition (CVPR), pp 1800–1807. https://doi.org/10.1109/CVPR.2017.195
Cun YL, Boser B, Denker JS et al (1990) handwritten digit recognition with a back-propagation network. Morgan Kaufmann, San Francisco, pp 396–404
Google Scholar
Daele DV, Decleyre N, Dubois H et al (2021) An automated engineering assistant: Learning parsers for technical drawings. In: AAAI
Dai J, Li Y, He K et al (2016) R-FCN: object detection via region-based fully convolutional networks. CoRR. arXiv:1605.06409
Dalal N, Triggs B (2005) Histograms of oriented gradients for human detection. In: 2005 IEEE computer society conference on computer vision and pattern recognition (CVPR’05), vol 1, pp 886–893. https://doi.org/10.1109/CVPR.2005.177
De P, Mandal S, Bhowmick P (2011) Recognition of electrical symbols in document images using morphology and geometric analysis. In: 2011 International conference on image information processing, pp 1–6. https://doi.org/10.1109/ICIIP.2011.6108910
Dosovitskiy A, Beyer L, Kolesnikov A et al (2020) An image is worth 16 × 16 words: transformers for image recognition at scale. CoRR. arXiv:2010.11929
Dzhusupova R, Banotra R, Bosch J et al (2022) Pattern recognition method for detecting engineering errors on technical drawings. In: 2022 IEEE World AI IoT congress (AIIoT), pp 642–648. https://doi.org/10.1109/AIIoT54504.2022.9817294
Elyan E, Garcia CM, Jayne C (2018) Symbols classification in engineering drawings. In: 2018 International joint conference on neural networks (IJCNN), pp 1–8
Elyan E, Jamieson L, Ali-Gombe A (2020a) Deep learning for symbols detection and classification in engineering drawings. Neural Netw 129:91–102. https://doi.org/10.1016/j.neunet.2020.05.025
Elyan E, Moreno-García CF, Johnston P (2020b) Symbols in engineering drawings (SIED): an imbalanced dataset benchmarked by convolutional neural networks. In: Iliadis L, Angelov PP, Jayne C et al (eds) Proceedings of the 21st EANN (Engineering Applications of Neural Networks) 2020 conference. Springer, Cham, pp 215–224
Espina-Romero L, Guerrero-Alcedo J (2022) Fields touched by digitalization: analysis of scientific activity in Scopus. Sustainability. https://doi.org/10.3390/su142114425
Ester M, Kriegel HP, Sander J et al (1996) A density-based algorithm for discovering clusters in large spatial databases with noise. In: KDD, pp 226–231
Everingham M, Van Gool L, Williams CKI et al (2007) The PASCAL visual object classes challenge 2007 (VOC2007) results. http://www.pascal-network.org/challenges/VOC/voc2007/workshop/index.html
Everingham M, Van Gool L, Williams CK et al (2010) The pascal visual object classes (VOC) challenge. Int J Comput Vis 88(2):303–338
Faltin B, Schönfelder P, König M (2022) Inferring interconnections of construction drawings for bridges using deep learning-based methods. In: ECPPM 2022—eWork and eBusiness in architecture, engineering and construction 2022, pp 343–350. CRC Press, Boca Raton. https://doi.org/10.1201/9781003354222-44
Fan Z, Chen T, Wang P et al (2022) Cadtransformer: Panoptic symbol spotting transformer for cad drawings. In: 2022 IEEE/CVF conference on computer vision and pattern recognition (CVPR), pp 10976–10986. https://doi.org/10.1109/CVPR52688.2022.01071
Felzenszwalb P, McAllester D, Ramanan D (2008) A discriminatively trained, multiscale, deformable part model. In: 2008 IEEE conference on computer vision and pattern recognition, pp 1–8. https://doi.org/10.1109/CVPR.2008.4587597
Fogel S, Averbuch-Elor H, Cohen S et al (2020) Scrabblegan: semi-supervised varying length handwritten text generation. In: 2020 IEEE/CVF conference on computer vision and pattern recognition (CVPR), pp 4323–4332. https://doi.org/10.1109/CVPR42600.2020.00438
Francois M, Eglin V, Biou M (2022) Text detection and post-ocr correction in engineering documents. In: Uchida S, Barney E, Eglin V (eds) Document analysis systems. Springer, Cham, pp 726–740
Chapter Google Scholar
Gao W, Zhao Y, Smidts C (2020) Component detection in piping and instrumentation diagrams of nuclear power plants based on neural networks. Prog Nucl Energy 128:103491. https://doi.org/10.1016/j.pnucene.2020.103491
Girshick R (2015) Fast R-CNN. In: 2015 IEEE International conference on computer vision (ICCV), pp 1440–1448. https://doi.org/10.1109/ICCV.2015.169
Girshick R, Donahue J, Darrell T et al (2014) Rich feature hierarchies for accurate object detection and semantic segmentation. In: 2014 IEEE conference on computer vision and pattern recognition, pp 580–587. https://doi.org/10.1109/CVPR.2014.81
Goodfellow I, Pouget-Abadie J, Mirza M et al (2014) Generative adversarial nets. In: Ghahramani Z, Welling M, Cortes C et al (eds) Advances in neural information processing systems, vol 27. Curran Associates, San Francisco, pp 2672–2680. http://papers.nips.cc/paper/5423-generative-adversarial-nets.pdf
Graves A, Fernández S, Gomez F et al (2006) Connectionist temporal classification: labelling unsegmented sequence data with recurrent neural networks. In: Proceedings of the 23rd International conference on machine learning (ICML ’06). ACM, New York, pp 369–376. https://doi.org/10.1145/1143844.1143891 ,
Groen FC, Sanderson AC, Schlag JF (1985) Symbol recognition in electrical diagrams using probabilistic graph matching. Pattern Recogn Lett 3(5):343–350
Gupta A, Vedaldi A, Zisserman A (2016) Synthetic data for text localisation in natural images. In: IEEE conference on computer vision and pattern recognition
Gupta M, Wei C, Czerniawski T (2022) Automated valve detection in piping and instrumentation (P&ID) diagrams. In: Proceedings of the 39th international symposium on automation and robotics in construction, ISARC 2022. International Association for Automation and Robotics in Construction (IAARC), pp 630–637
Haar C, Kim H, Koberg L (2023) AI-based engineering and production drawing information extraction. In: International conference on flexible automation and intelligent manufacturing, Springer, Berlin, pp 374–382
Hantach R, Lechuga G, Calvez P (2021) Key information recognition from piping and instrumentation diagrams: where we are? In: Barney Smith EH, Pal U (eds) Document analysis and recognition—ICDAR 2021 workshops. Springer, Cham, pp 504–508
He K, Zhang X, Ren S et al (2016) Deep residual learning for image recognition. In: 2016 IEEE conference on computer vision and pattern recognition (CVPR), pp 770–778. https://doi.org/10.1109/CVPR.2016.90
He K, Gkioxari G, Dollár P et al (2017) Mask R-CNN. In: 2017 IEEE international conference on computer vision (ICCV), pp 2980–2988
Howie C, Kunz J, Binford T et al (1998) Computer interpretation of process and instrumentation drawings. Adv Eng Softw 29(7):563–570. https://doi.org/10.1016/S0965-9978(98)00022-2
Hu H, Zhang C, Liang Y (2021) Detection of surface roughness of mechanical drawings with deep learning. J Mech Sci Technol 35(12):5541–5549
Jaderberg M, Simonyan K, Vedaldi A et al (2014) Synthetic data and artificial neural networks for natural scene text recognition. In: Workshop on deep learning, NIPS
Jakubik J, Hemmer P, Vossing M et al (2022) Designing a human-in-the-loop system for object detection in floor plans. Karlsruhe Institute of Technology, Karlsruhe
Jamieson L, Moreno-Garcia CF, Elyan E (2020) Deep learning for text detection and recognition in complex engineering diagrams. In: 2020 International joint conference on neural networks (IJCNN), pp 1–7. https://doi.org/10.1109/IJCNN48605.2020.9207127
Jocher G, Nishimura K, Mineeva T et al (2020) YOLOv5. Code repository. http://github.com/ultralytics/yolov5
Johnson JM, Khoshgoftaar TM (2019) Survey on deep learning with class imbalance. J Big Data 6(1):27
Joy J, Mounsef J (2021) Automation of material takeoff using computer vision. In: 2021 IEEE international conference on industry 4.0, artificial intelligence, and communications technology (IAICT), pp 196–200. https://doi.org/10.1109/IAICT52856.2021.9532514
Kang SO, Lee EB, Baek HK (2019) A digitization and conversion tool for imaged drawings to intelligent piping and instrumentation diagrams P&ID. Energies. https://doi.org/10.3390/en12132593 ,
Karatzas D, Shafait F, Uchida S et al (2013) ICDAR 2013 robust reading competition. In: 2013 12th International conference on document analysis and recognition, pp 1484–1493
Karatzas D, Gomez-Bigorda L, Nicolaou A et al (2015) Icdar 2015 competition on robust reading. In: 2015 13th International conference on document analysis and recognition (ICDAR), pp 1156–1160. https://doi.org/10.1109/ICDAR.2015.7333942
Khallouli W, Pamie-George R, Kovacic S et al (2022) Leveraging transfer learning and gan models for OCR from engineering documents. In: 2022 IEEE World AI IoT Congress (AIIoT), pp 015–021. https://doi.org/10.1109/AIIoT54504.2022.9817319
Kim H, Kim S, Yu K (2021a) Automatic extraction of indoor spatial information from floor plan image: a patch-based deep learning methodology application on large-scale complex buildings. ISPRS Int J Geo-Inf. https://doi.org/10.3390/ijgi10120828
Kim H, Lee W, Kim M et al (2021b) Deep-learning-based recognition of symbols and texts at an industrially applicable level from images of high-density piping and instrumentation diagrams. Expert Syst Appl 183:115337. https://doi.org/10.1016/j.eswa.2021.115337
Kiryati N, Eldar Y, Bruckstein AM (1991) A probabilistic hough transform. Pattern Recogn 24(4):303–316
Article MathSciNet Google Scholar
Krizhevsky A, Sutskever I, Hinton GE (2012) Imagenet classification with deep convolutional neural networks. In: Pereira F, Burges CJC, Bottou L et al (eds) Advances in neural information processing systems 25. Curran Associates, San Francisco, pp 1097–1105. http://papers.nips.cc/paper/4824-imagenet-classification-with-deep-convolutional-neural-networks.pdf
LeCun Y, Bottou L, Bengio Y et al (1998) Gradient-based learning applied to document recognition. Proc IEEE 86(11):2278–2324
LeCun Y, Bengio Y, Hinton G (2015) Deep learning. Nature 521(7553):436
Li C, Li L, Jiang H et al (2022) Yolov6: a single-stage object detection framework for industrial applications. Comput Vis Pattern Recog. arXiv:2209.02976
Lin TY, Maire M, Belongie S et al (2014) Microsoft coco: common objects in context. In: Fleet D, Pajdla T, Schiele B et al (eds) Computer vision—ECCV 2014. Springer, Cham, pp 740–755
Lin T, Goyal P, Girshick R et al (2017) Focal loss for dense object detection. In: 2017 IEEE international conference on computer vision (ICCV), pp 2999–3007. https://doi.org/10.1109/ICCV.2017.324
Liu W, Anguelov D, Erhan D et al (2015) SSD: single shot multibox detector. CoRR. arXiv:1512.02325
Liu J, Zhong Q, Yuan Y et al (2020) Semitext: scene text detection with semi-supervised learning. Neurocomputing 407:343–353. https://doi.org/10.1016/j.neucom.2020.05.059
Long S, Yao C (2020) Unrealtext: Synthesizing realistic scene text images from the unreal world. CoRR. arXiv:2003.10608
Long J, Shelhamer E, Darrell T (2015) Fully convolutional networks for semantic segmentation. In: 2015 IEEE conference on computer vision and pattern recognition (CVPR), pp 3431–3440. https://doi.org/10.1109/CVPR.2015.7298965
Long S, He X, Yao C (2018) Scene text detection and recognition: the deep learning era. CoRR. arXiv:1811.04256
Lowe DG (2004) Distinctive image features from scale-invariant keypoints. Int J Comput Vis 60(2):91–110
Mafipour MS, Ahmed D, Vilgertshofer S et al (2023) Digitalization of 2D bridge drawings using deep learning models. In: Proceedings of the 30th international conference on intelligent computing in engineering (EG-ICE)
Mani S, Haddad MA, Constantini D et al (2020) Automatic digitization of engineering diagrams using deep learning and graph search. In: 2020 IEEE/CVF conference on computer vision and pattern recognition workshops (CVPRW), pp 673–679
Mishra A, Alahari K, Jawahar C (2012) Scene text recognition using higher order language priors. In: Proceedings of the British machine vision conference. BMVA Press, Guildford, pp 127.1–127.11 https://doi.org/10.5244/C.26.127
Mizanur Rahman S, Bayer J, Dengel A (2021) Graph-based object detection enhancement for symbolic engineering drawings. In: Document analysis and recognition—ICDAR 2021 workshops: Lausanne, Switzerland, 5–10 Sept 2021, proceedings, Part I. Springer, Berlin. pp 74–90. https://doi.org/10.1007/978-3-030-86198-8_6
Moon Y, Lee J, Mun D et al (2021) Deep learning-based method to recognize line objects and flow arrows from image-format piping and instrumentation diagrams for digitization. Appl Sci 11(21):10054
Moreno-Garcia CF, Elyan E (2019) Digitisation of assets from the oil and gas industry: challenges and opportunities. In: 2019 International conference on document analysis and recognition workshops (ICDARW), pp 2–5. https://doi.org/10.1109/ICDARW.2019.60122
Moreno-García CF, Elyan E, Jayne C (2018) New trends on digitisation of complex engineering drawings. Neural Comput Appl. https://doi.org/10.1007/s00521-018-3583-1
Moreno-García CF, Elyan E, Jayne C (2019) New trends on digitisation of complex engineering drawings. Neural Comput Appl 31(6):1695–1712. https://doi.org/10.1007/s00521-018-3583-1
Moreno-García CF, Johnston P, Garkuwa B (2020) Pixel-based layer segmentation of complex engineering drawings using convolutional neural networks. In: 2020 International joint conference on neural networks (IJCNN), pp 1–7. https://doi.org/10.1109/IJCNN48605.2020.9207479
Nguyen T, Pham LV, Nguyen C et al (2021) Object detection and text recognition in large-scale technical drawings. In: Proceedings of the 10th international conference on pattern recognition applications and methods, vol 1: ICPRAM, INSTICC. SciTePress, Setúbal, pp 612–619. https://doi.org/10.5220/0010314406120619
Nurminen JK, Rainio K, Numminen JP et al (2020) Object detection in design diagrams with machine learning. In: Burduk R, Kurzynski M, Wozniak M (eds) Progress in computer recognition systems. Springer, Cham, pp 27–36
Ojala T, Pietikainen M, Maenpaa T (2002) Multiresolution gray-scale and rotation invariant texture classification with local binary patterns. IEEE Trans Pattern Anal Mach Intell 24(7):971–987. https://doi.org/10.1109/TPAMI.2002.1017623
Okazaki A, Kondo T, Mori K et al (1988) An automatic circuit diagram reader with loop-structure-based symbol recognition. IEEE Trans Pattern Anal Mach Intell 10(3):331–341. https://doi.org/10.1109/34.3898
Paliwal S, Jain A, Sharma M et al (2021a) Digitize-PID: automatic digitization of piping and instrumentation diagrams. In: Gupta M, Ramakrishnan G (eds) Trends and applications in knowledge discovery and data mining—PAKDD 2021 Workshops, WSPA, MLMEIN, SDPRA, DARAI, and AI4EPT, Delhi, India, 11 May 2021, proceedings. Lecture notes in computer science, vol 12705. Springer, Berlin, pp 168–180. https://doi.org/10.1007/978-3-030-75015-2_17 ,
Paliwal S, Sharma M, Vig L (2021b) OSSR-PID: one-shot symbol recognition in P&ID sheets using path sampling and GCN. In: 2021 International joint conference on neural networks (IJCNN), pp 1–8. https://doi.org/10.1109/IJCNN52387.2021.9534122
Pizarro PN, Hitschfeld N, Sipiran I et al (2022) Automatic floor plan analysis and recognition. Autom Constr 140:104348. https://doi.org/10.1016/j.autcon.2022.104348
Prasad D, Gadpal A, Kapadni K et al (2020) Cascadetabnet: An approach for end to end table detection and structure recognition from image-based documents. In: 2020 IEEE/CVF Conference on Computer Vision and Pattern Recognition Workshops (CVPRW), pp 2439–2447, https://doi.org/10.1109/CVPRW50498.2020.00294
Rahul R, Paliwal S, Sharma M et al (2019) Automatic information extraction from piping and instrumentation diagrams. In: Marsico MD, di Baja GS, Fred ALN (eds) Proceedings of the 8th international conference on pattern recognition applications and methods, ICPRAM 2019, Prague, Czech Republic, 19–21 Feb 2019. SciTePress, Setúbal, pp 163–172. https://doi.org/10.5220/0007376401630172
Rantala M, Niemistö H, Karhela T et al (2019) Applying graph matching techniques to enhance reuse of plant design information. Comput Ind 107:81–98
Redmon J, Farhadi A (2017) Yolo9000: better, faster, stronger. In: 2017 IEEE conference on computer vision and pattern recognition (CVPR), pp 6517–6525. https://doi.org/10.1109/CVPR.2017.690
Redmon J, Farhadi A (2018) Yolov3: an incremental improvement. CoRR. arXiv:1804.02767
Redmon J, Divvala S, Girshick R et al (2016) You only look once: unified, real-time object detection. In: Proceedings of the IEEE conference on computer vision and pattern recognition, pp 779–788
Ren S, He K, Girshick R et al (2015) Faster R-CNN: Towards real-time object detection with region proposal networks. In: Proceedings of the 28th international conference on neural information processing systems, NIPS’15, vol 1. MIT, Cambridge, pp 91–99. http://dl.acm.org/citation.cfm?id=2969239.2969250
Ren P, Xiao Y, Chang X et al (2021) A survey of deep active learning. ACM Comput Surv. https://doi.org/10.1145/3472291
Renton G, Héroux P, Gaüzère B et al (2019) Graph neural network for symbol detection on document images. In: 2019 International conference on document analysis and recognition workshops (ICDARW), pp 62–67. https://doi.org/10.1109/ICDARW.2019.00016
Renton G, Balcilar M, Héroux P et al (2021) Symbols detection and classification using graph neural networks. Pattern Recogn Lett 152:391–397. https://doi.org/10.1016/j.patrec.2021.09.020
Rezvanifar A, Cote M, Albu AB (2020) Symbol spotting on digital architectural floor plans using a deep learning-based framework. In: 2020 IEEE/CVF conference on computer vision and pattern recognition workshops (CVPRW), pp 2419–2428. https://doi.org/10.1109/CVPRW50498.2020.00292
Rica E, Moreno-García CF, Álvarez S et al (2020) Reducing human effort in engineering drawing validation. Comput Ind 117:103198. https://doi.org/10.1016/j.compind.2020.103198
Rica E, Álvarez S, Serratosa F (2021) Group of components detection in engineering drawings based on graph matching. Eng Appl Artif Intell 104:104404. https://doi.org/10.1016/j.engappai.2021.104404
Rumalshan OR, Weerasinghe P, Shaheer M et al (2023) Transfer learning approach for railway technical map (RTM) component identification. In: Proceedings of 7th international congress on information and communication technology, Springer, pp 479–488
Russakovsky O, Deng J, Su H et al (2015) Imagenet large scale visual recognition challenge. Int J Comput Vis (IJCV) 115(3):211–252. https://doi.org/10.1007/s11263-015-0816-y
Russell BC, Torralba A, Murphy KP et al (2008) Labelme: a database and web-based tool for image annotation. Int J Comput Vis 77(1):157–173
Sarkar S, Pandey P, Kar S (2022) Automatic detection and classification of symbols in engineering drawings. Comput Vis Pattern Recogn. https://doi.org/10.48550/arxiv.2204.13277 ,
Scheibel B, Mangler J, Rinderle-Ma S (2021) Extraction of dimension requirements from engineering drawings for supporting quality control in production processes. Comput Ind 129:103442. https://doi.org/10.1016/j.compind.2021.103442
Shi B, Bai X, Belongie S (2017a) Detecting oriented text in natural images by linking segments. In: 2017 IEEE conference on computer vision and pattern recognition (CVPR), pp 3482–3490. https://doi.org/10.1109/CVPR.2017.371
Shi B, Bai X, Yao C (2017) An end-to-end trainable neural network for image-based sequence recognition and its application to scene text recognition. IEEE Trans Pattern Anal Mach Intell 39(11):2298–2304. https://doi.org/10.1109/TPAMI.2016.2646371
Sierla S, Azangoo M, Fay A et al (2020) Integrating 2D and 3D digital plant information towards automatic generation of digital twins. In: 2020 IEEE 29th international symposium on industrial electronics (ISIE), pp 460–467. https://doi.org/10.1109/ISIE45063.2020.9152371
Sierla S, Azangoo M, Rainio K et al (2021) Roadmap to semi-automatic generation of digital twins for brownfield process plants. J Ind Inf Integr. https://doi.org/10.1016/j.jii.2021.100282
Sinha A, Bayer J, Bukhari SS (2019) Table localization and field value extraction in piping and instrumentation diagram images. In: 2019 International Conference on Document Analysis and Recognition Workshops (ICDARW), pp 26–31, https://doi.org/10.1109/ICDARW.2019.00010
Smith R (2007) An overview of the tesseract OCR engine. In: 9th International conference on document analysis and recognition (ICDAR 2007). IEEE, pp 629–633
Stinner F, Wiecek M, Baranski M et al (2021) Automatic digital twin data model generation of building energy systems from piping and instrumentation diagrams. Comput Vis Pattern Recogn. arXiv:2108.13912
Szegedy C, Vanhoucke V, Ioffe S et al (2015) Rethinking the inception architecture for computer vision. CoRR. arXiv:1512.00567
Theisen MF, Flores KN, Schulze Balhorn L et al (2023) Digitization of chemical process flow diagrams using deep convolutional neural networks. Digit Chem Eng 6:100072. https://doi.org/10.1016/j.dche.2022.100072
Tian Z, Huang W, He T et al (2016) Detecting text in natural image with connectionist text proposal network. CoRR. arXiv:1609.03605
Toral L, Moreno-García CF, Elyan E et al (2021) A deep learning digitisation framework to mark up corrosion circuits in piping and instrumentation diagrams. In: Barney Smith EH, Pal U (eds) Document analysis and recognition—ICDAR 2021 workshops. Springer, Cham, pp 268–276
Uijlings JR, Van De Sande KE, Gevers T et al (2013) Selective search for object recognition. Int J Comput Vis 104(2):154–171
Van Engelen JE, Hoos HH (2020) A survey on semi-supervised learning. Mach Learn 109(2):373–440
Veit A, Matera T, Neumann L et al (2016) Coco-text: Dataset and benchmark for text detection and recognition in natural images. arXiv:1601.07140
Vilgertshofer S, Stoitchkov D, Borrmann A et al (2019) Recognising railway infrastructure elements in videos and drawings using neural networks. Proc Inst Civ Eng Smart Infrastruct Constr 172(1):19–33. https://doi.org/10.1680/jsmic.19.00017
Viola P, Jones M (2001) Rapid object detection using a boosted cascade of simple features. In: Proceedings of the 2001 IEEE computer society conference on computer vision and pattern recognition. CVPR 2001, pp I–I. https://doi.org/10.1109/CVPR.2001.990517
Wang Y, Sun Y, Liu Z et al (2018) Dynamic graph CNN for learning on point clouds. CoRR. arXiv:1801.07829
Wang CY, Bochkovskiy A, Liao HYM (2022) Yolov7: trainable bag-of-freebies sets new state-of-the-art for real-time object detectors. arXiv:2207.02696
Wen R, Tang W, Su Z (2017a) Measuring 3D process plant model similarity based on topological relationship distribution. Computer Aid Des Appl 14(4):422–435
Wen R, Tang W, Su Z (2017b) Topology based 2D engineering drawing and 3d model matching for process plant. Graph Models 92:1–15. https://doi.org/10.1016/j.gmod.2017.06.001
Xie L, Lu Y, Furuhata T et al (2022) Graph neural network-enabled manufacturing method classification from engineering drawings. Comput Ind 142(103):697. https://doi.org/10.1016/j.compind.2022.103697
Ye Q, Doermann D (2015) Text detection and recognition in imagery: a survey. IEEE Trans Pattern Anal Mach Intell 37(7):1480–1500. https://doi.org/10.1109/TPAMI.2014.2366765
Yu ES, Cha JM, Lee T et al (2019) Features recognition from piping and instrumentation diagrams in image format using a deep learning network. Energies. https://doi.org/10.3390/en12234425
Yun DY, Seo SK, Zahid U et al (2020) Deep neural network for automatic image recognition of engineering diagrams. Appl Sci. https://doi.org/10.3390/app10114005
Zhang F, Zhai G, Li M et al (2020) Three-branch and mutil-scale learning for fine-grained image recognition (TBMSL-NET). CoRR. arXiv:2003.09150
Zhang D, Han J, Cheng G et al (2022) Weakly supervised object localization and detection: a survey. IEEE Trans Pattern Anal Mach Intell 44(9):5866–5885. https://doi.org/10.1109/TPAMI.2021.3074313
Zhao Y, Deng X, Lai H (2020) A deep learning-based method to detect components from scanned structural drawings for reconstructing 3D models. Appl Sci. https://doi.org/10.3390/app10062066
Zheng Z, Li J, Zhu L et al (2022) GAT-CADNet: graph attention network for panoptic symbol spotting in CAD drawings. In: Proceedings of the IEEE/CVF conference on computer vision and pattern recognition, pp 11747–11756
Zhou X, Yao C, Wen H et al (2017) EAST: an efficient and accurate scene text detector. CoRR. arXiv:1704.03155
Zhu JY, Park T, Isola P et al (2017) Unpaired image-to-image translation using cycle-consistent adversarial networks. In: Proceedings of the IEEE international conference on computer vision, pp 2223–2232
Ziran Z, Marinai S (2018) Object detection in floor plan images. In: Pancioni L, Schwenker F, Trentin E (eds) Artificial neural networks in pattern recognition. Springer, Cham, pp 383–394
Download references
Acknowledgements
We would like to thank TaksoAI for providing the engineering diagrams, through a related project.
Author information
Carlos Francisco Moreno-García and Eyad Elyan have contributed equally to this work.
Authors and Affiliations
School of Computing, Robert Gordon University, Garthdee Road, Aberdeen, AB10 7QB, Scotland, UK
Laura Jamieson, Carlos Francisco Moreno-García & Eyad Elyan
You can also search for this author in PubMed Google Scholar
Contributions
L.J., C.F.M.G. and E.E. all contributed to this paper.
Corresponding author
Correspondence to Laura Jamieson .
Ethics declarations
Competing interests.
The authors have no competing interests to declare that are relevant to the content of this article.
Additional information
Publisher's note.
Springer Nature remains neutral with regard to jurisdictional claims in published maps and institutional affiliations.
Rights and permissions
Open Access This article is licensed under a Creative Commons Attribution 4.0 International License, which permits use, sharing, adaptation, distribution and reproduction in any medium or format, as long as you give appropriate credit to the original author(s) and the source, provide a link to the Creative Commons licence, and indicate if changes were made. The images or other third party material in this article are included in the article's Creative Commons licence, unless indicated otherwise in a credit line to the material. If material is not included in the article's Creative Commons licence and your intended use is not permitted by statutory regulation or exceeds the permitted use, you will need to obtain permission directly from the copyright holder. To view a copy of this licence, visit http://creativecommons.org/licenses/by/4.0/ .
Reprints and permissions
About this article
Jamieson, L., Francisco Moreno-García, C. & Elyan, E. A review of deep learning methods for digitisation of complex documents and engineering diagrams. Artif Intell Rev 57 , 136 (2024). https://doi.org/10.1007/s10462-024-10779-2
Download citation
Accepted : 24 April 2024
Published : 09 May 2024
DOI : https://doi.org/10.1007/s10462-024-10779-2
Share this article
Anyone you share the following link with will be able to read this content:
Sorry, a shareable link is not currently available for this article.
Provided by the Springer Nature SharedIt content-sharing initiative
- Deep learning
- Object detection
- Engineering diagram
- Piping and Instrumentation Diagram
- Convolutional neural networks
- Find a journal
- Publish with us
- Track your research
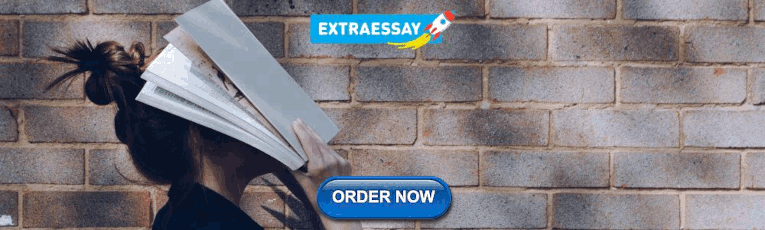
COMMENTS
ABSTRACT. Artificial intelligence may greatly increase the efficiency of the existing economy. But it may have an even larger impact by serving as a new general-purpose "method of invention" that can reshape the nature of the innovation process and the organization of R&D.
The paper focuses specifically on the incorporation of artificial intelligence (AI), which includes a wide range of technologies and methods, such as machine learning, adaptive learning, natural ...
Artificial Intelligence | Explore the latest full-text research PDFs, articles, conference papers, preprints and more on ARTIFICIAL INTELLIGENCE. Find methods information, sources, references or ...
The Journal of Artificial Intelligence Research (www.jair.org) covers all areas of artificial intelligence, publishing refereed research articles, survey articles, and technical notes. JAIR was established in 1993 as one of the very first open access scientific journals on the Web. Since it began publication in 1993, JAIR has had a major impact on the field, and has been continuously ranked as ...
The journal of Artificial Intelligence (AIJ) welcomes papers on broad aspects of AI that constitute advances in the overall field including, but not limited to, cognition and AI, automated reasoning and inference, case-based reasoning, commonsense reasoning, computer vision, constraint processing, ethical AI, heuristic search, human interfaces, intelligent robotics, knowledge representation ...
The Journal of Artificial Intelligence Research (JAIR) is dedicated to the rapid dissemination of important research results to the global artificial intelligence (AI) community. The journal's scope encompasses all areas of AI, including agents and multi-agent systems, automated reasoning, constraint processing and search, knowledge ...
Artificial intelligence and machine learning research: towards digital transformation at a global scale ... A variety of innovative topics are included in the agenda of the published papers in this special issue including topics such as: ... such as Artificial Intelligence technologies, are seen today as promising instruments that could pave ...
Artificial intelligence (AI) is a rapidly evolving field that has transformed various domains of scientific research. This article provides an overview of the history, applications, challenges, and opportunities of AI in science. It also discusses how AI can enhance scientific creativity, collaboration, and communication. Learn more about the potential and impact of AI in science by reading ...
The number of AI and robotics papers published in the 82 high-quality science journals in the Nature Index (Count) has been rising year-on-year — so rapidly that it resembles an exponential ...
Journal of Artificial Intelligence Research (311) Evolutionary Computation (278) Computational ... By clicking download,a status dialog will open to start the export ... Prior work has shown that training static word embeddings can expose such biases. In this short paper, we apply both a conventional Word2Vec ... 0; Metrics. Total Citations 0.
Artificial Intelligence. The AI industry could top $1 trillion in 2018 and almost $4 trillion by 2022. As well as being a feat of engineering and computing there are a significant amount of social and moral implications that need to be considered. This microsite brings together cutting edge research across many of these disciplines to help ...
1. Industry continues to dominate frontier AI research. 2. More foundation models and more open foundation models. 3. Frontier models get way more expensive. 4. The United States leads China, the EU, and the U.K. as the leading source of top AI models. 5. The number of AI patents skyrockets. 6. China dominates AI patents. 7. Open-source AI ...
at our disposal, AI is going to add a new level of ef ficiency and. sophistication to future technologies. One of the primary goals of AI field is to produce fully au-. tonomous intelligent ...
The field of artificial intelligence (AI) has shown an upward trend of growth in the 21st century (from 2000 to 2015). The evolution in AI has advanced the development of human society in our own time, with dramatic revolutions shaped by both theories and techniques. However, the multidisciplinary and fast-growing features make AI a field in which it is difficult to be well understood. In this ...
Explainable Artificial Intelligence (XAI) is an emerging area of research in the field of Artificial Intelligence (AI). XAI can explain how AI obtained a particular solution (e.g., classification or object detection) and can also answer other "wh" questions. This explainability is not possible in traditional AI. Explainability is essential for critical applications, such as defense, health ...
In the current age of the Fourth Industrial Revolution (4IR or Industry 4.0), the digital world has a wealth of data, such as Internet of Things (IoT) data, cybersecurity data, mobile data, business data, social media data, health data, etc. To intelligently analyze these data and develop the corresponding smart and automated applications, the knowledge of artificial intelligence (AI ...
Artificial Intelligence is gradually changing the landscape of software engineering in general [5] and software testing in particular [6] both in research and industry as well. In the last two decades, AI has been found to have made a considerable impact on the way we are approach-ing software testing.
Best Paper of the International Conference on Integrated Emerging Methods of Artificial Intelligence & Cloud Computing (IEMAICLOUD 2021) "False Alarm Detection in Wind Turbine Management by K-Nearest Neighbors Models", and one of the most downloaded in first 12 months of publications in Progress in Photovoltaic: Research and Applications ...
Artificial Intelligence. Gheorghe Tecuci. Learning Agents Center and Computer Science Department. George Mason University, Fairfax, VA 22030. Abstract. Artificial Intelligence is the Science and ...
The 10 groundbreaking AI research papers for 2024 collectively symbolize the frontiers of innovation in artificial intelligence. These papers represent the culmination of diverse advancements, from ethical frameworks to quantum computing, and healthcare applications to climate modeling.
Artificial intelligence forms the basis for all computer learning and is the future of all complex decision making. This paper examines features of artificial Intelligence, introduction, definitions of AI, history, applications, growth and achievements. KEYWORDS-machine learning,deep learning,neural networks,Natural Language Processing and ...
Download PDF. You have full access to this open access article. Artificial Intelligence Review Aims and scope Submit manuscript ... Drawing upon the existing research findings, this paper identifies and proposes six novel research challenges and directions within the realm of imbalanced learning. These challenges and directions hold significant ...
The impact of artificial intelligence on output and inflation I Aldasoro BIS S Doerr BIS & CEPR L Gambacorta BIS & CEPR D Rees BIS April 11, 2024 Abstract This paper studies the effects of artificial intelligence (AI) on sectoral and aggregate employment, output and inflation in both the short and long run. We construct an
IJCAI 2024: AAII Success. The International Joint Conference on Artificial Intelligence (IJCAI) is the premier international gathering of researchers in AI. The 33rd iteration of the IJCAI conference will take place in August this year in Jeju, with the following papers by AAII members accepted for presentation:
Excellence in a wide variety of medical applications poses considerable challenges for AI, requiring advanced reasoning, access to up-to-date medical knowledge and understanding of complex multimodal data. Gemini models, with strong general capabilities in multimodal and long-context reasoning, offer exciting possibilities in medicine. Building on these core strengths of Gemini, we introduce ...
Background: A plethora of weight management apps are available, but many individuals, especially those living with overweight and obesity, still struggle to achieve adequate weight loss. An emerging area in weight management is the support for one's self-regulation over momentary eating impulses. Objective: This study aims to examine the feasibility and effectiveness of a novel artificial ...
Abstract and Figures. This paper focus on the History of A.I. and how it begun as an idea and, the definition of artificial intelligence and gives a detailed description of Artificial Intelligence ...
Class 10 Artificial Intelligence Syllabus 2024-2025: Get here detailed CBSE Class 10 Artificial Intelligence Syllabus chapter-wise, marking scheme, weightage, paper pattern and Download PDF.
According to a Financial Times analysis of hundreds of LinkedIn profiles as well as public job postings and research papers, the $2.7tn company has undertaken a hiring spree over recent years to ...
This paper presents a review of deep learning on engineering drawings and diagrams. These are typically complex diagrams, that contain a large number of different shapes, such as text annotations, symbols, and connectivity information (largely lines). Digitising these diagrams essentially means the automatic recognition of all these shapes. Initial digitisation methods were based on ...