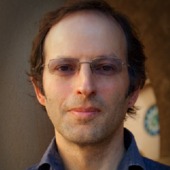
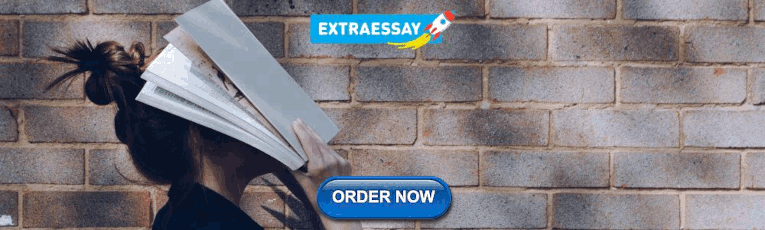
Technophrenia
On the interface between technology, people and society
How technology is changing language and the way we think about the world
Director of UWA Centre for Software Practice, The University of Western Australia
University of Western Australia provides funding as a founding partner of The Conversation AU.
View all partners
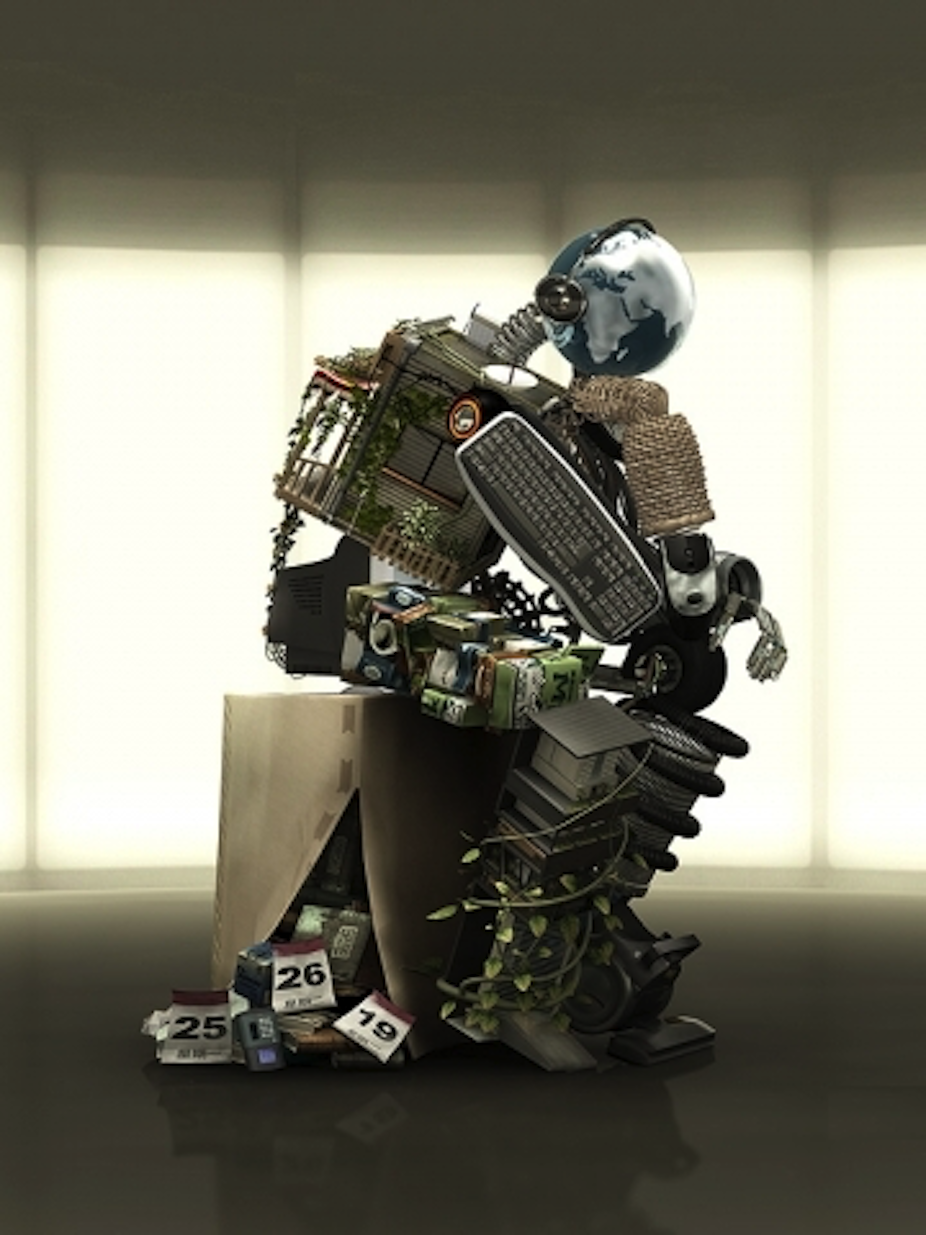
We are getting used to the idea of rapidly developing technologies changing what we can do and how we do things. What most people haven’t considered is how technologies affect our language and how these changes are affecting the way we speak and even the way we think.
One of the key ways we see this is when the name of a company becomes a way of doing something involving any product that is similar. Classic examples are “to hoover” which came from the early dominance of vaccuum cleaners from the Hoover Company .
Googling becomes a verb
More significantly is the use of the term “to google” which first came to prominence in 2002 when the American Dialect Society declared it word of the year. Later, in 2009, they declared “google” to be the the word of the decade.
Google had become a generic word meaning “to search the Internet” with any search engine, not just Google itself. But googling has become much more than just the mere act of typing words into a text box and clicking a button. We now understand the subtext when someone declares “I have googled you” or even that they have googled themselves. The idea that this act can now exert a powerful effect on the opinion we form of others has even resulted in laws formulated by the European Union giving individuals rights over search engine companies to have information about them removed in order to be “forgotten”.
Google has now become our collective global memory and googling is the process by which we access those memories. This, in turn, is simply a process that we have always engaged with called “ transactive memory ” in which we turn to people around us, usually people we know, to help us recall facts and memories. The invention of the Gutenberg press allowed us to outsource people to books. The difference now though is that Google is now always with us, has a vast database of information that it is getting increasingly better at letting you access, with the vaguest of questions. This in turn has had a dramatic effect on what we are able to achieve, not only as individuals, but as a society.
The act of computer programming for example has become much easier through the ability to learn new computer languages and solve problems by “googling” the answers. It could be argued that the boom in mobile phone apps would not have been possible without Ggoogle providing a mechanism to access the “transactive memories” of the thousands of knowledgeable programmers with the answers to any developer’s questions.
Interestingly, it has been [Google] themselves who have resisted , even at times through legal threats, the spread of “google” being used beyond its reference to the company. This is because if it does enter the language as a common term, Google could lose the protection of the name as a trademark. If Google becomes a common term, to mean any generic search, it could become a “generic trademark” like Cellophane, Aspirin, Escalator and others.
Industries become uberized
In a different example of a verb that has come from a proper noun but may have just as significant an impact on our social lives, we have “to uberize”. This comes from the company Uber whose business approach has disrupted an industry by using mobile apps backed with data analytics to provide cheaper taxi services to consumers. The concept of “uberization” has taken the general meaning of disrupting any industry through the use of technology to circumvent unnecessary bureaucracy and legislation. What is interesting about the use of the term uberization is that again, the subtext is not just about the actual process of transforming an industry into something more efficient or productive. Saying that an industry needs to be “uberized” is as much a commentary about its unwillingness to change, modernise and really meet consumers’ needs. This context is being built up with every new development in the ongoing battles and controversies that Uber is facing as it pushes through its disruption of the taxi industry.
Uber’s less successful contribution to our language has been the concept of “ surge pricing ”. The concept embodies basic economic principles to ensure that there are taxis willing to pick up consumers at the busiest times. It turns out that this is too hard for most consumers (and reporters) to understand and they have interpreted it simply as unfair price gouging.
As a new term, it is an interesting example however of how a term that was supposed to have a specific meaning has been turned into something completely different through popular usage.
How much has changed
There are many conversations that we could have today that would mean little to someone from 2005. Even though the definition of specific words could be given, it would need the entire context of how they have developed through the interplay of technology, individuals and society to have any real meaning. This is not the first time this has happened in history but certainly the increase in the pace of change has resulted in our language changing equally rapidly, and with it, our thoughts.
On a final historical note, you can wonder what George Bernard Shaw would have understood by the following statements? “My mother was hacked last night.” “What a great meal - I’ll upload it!” “If anyone’s out there, can you inbox me?’ "How many steps did you get today?” “Will you torrent me the next series?” “I’ve given up on windows.”

Research Fellow in Tropical Climate Variability

Director, Defence and Security

Opportunities with the new CIEHF

School of Social Sciences – Public Policy and International Relations opportunities

Deputy Editor - Technology
Home — Essay Samples — Science — Language — The Power of Language: How Words Shape Our World

The Power of Language: How Words Shape Our World
- Categories: Language
About this sample

Words: 795 |
Published: Sep 7, 2023
Words: 795 | Pages: 2 | 4 min read
Table of contents
The essence of language and communication, the complex relationship between language and power, using language to promote transparency, accountability, and equity.
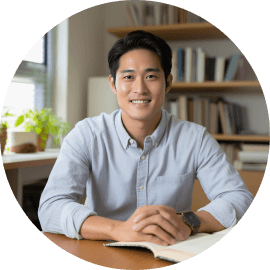
Cite this Essay
Let us write you an essay from scratch
- 450+ experts on 30 subjects ready to help
- Custom essay delivered in as few as 3 hours
Get high-quality help
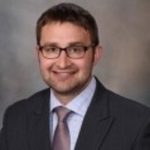
Dr. Heisenberg
Verified writer
- Expert in: Science
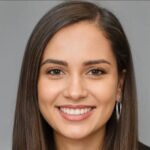
+ 120 experts online
By clicking “Check Writers’ Offers”, you agree to our terms of service and privacy policy . We’ll occasionally send you promo and account related email
No need to pay just yet!
Related Essays
2 pages / 713 words
1 pages / 589 words
2 pages / 799 words
5 pages / 2289 words
Remember! This is just a sample.
You can get your custom paper by one of our expert writers.
121 writers online
Still can’t find what you need?
Browse our vast selection of original essay samples, each expertly formatted and styled
Related Essays on Language
Language plays a crucial role in shaping one's identity, serving as a powerful tool for communication, self-expression, and cultural belonging. From the words we choose to the accents we carry, language reflects our unique [...]
Roald Dahl is a renowned British author and his short story, "The Landlady," is a masterpiece of suspense and mystery. One of the distinguishing features of this story is Dahl's use of diction. Diction plays an essential role in [...]
My journey in the field of Speech-Language Pathology has been one filled with passion, determination, and a drive to make a difference in the lives of individuals with communication disorders. Throughout my academic career, I [...]
Native language is often overlooked as something that people use instinctively, but it actually plays a crucial role in shaping one's identity (Norton, 2019). The connection between language and identity is not only direct [...]
George Orwell’s 1984 portrays a dystopian society whose values and freedoms have been marred through the manipulation of language and thus thought processes. Language has become a tool of mind control for the oppressive [...]
In today's visually saturated world, it is often said that a picture is worth a thousand words. However, in his article "Words Triumph Over Images," published in The New York Times in 2011, Errol Morris argues the opposite: that [...]
Related Topics
By clicking “Send”, you agree to our Terms of service and Privacy statement . We will occasionally send you account related emails.
Where do you want us to send this sample?
By clicking “Continue”, you agree to our terms of service and privacy policy.
Be careful. This essay is not unique
This essay was donated by a student and is likely to have been used and submitted before
Download this Sample
Free samples may contain mistakes and not unique parts
Sorry, we could not paraphrase this essay. Our professional writers can rewrite it and get you a unique paper.
Please check your inbox.
We can write you a custom essay that will follow your exact instructions and meet the deadlines. Let's fix your grades together!
Get Your Personalized Essay in 3 Hours or Less!
We use cookies to personalyze your web-site experience. By continuing we’ll assume you board with our cookie policy .
- Instructions Followed To The Letter
- Deadlines Met At Every Stage
- Unique And Plagiarism Free
Standardization of language Expository Essay
Necessity is the mother of all invention, this is a common phrase and it applies to language. The need to communicate led to the development of languages. With time, these languages have developed to suit the requirements and preferences of the people. These changes are responsible for the various languages and accent that are available worldwide.
Change in language is therefore an inevitable and a natural process (Crystal). Although change in language is unavoidable, people always want to stick to their known ways and methods of speech. The issue of what is the correct language remains a big issue.
If there is a perfect language, to what standards should it be. People have expectation on their language and they make a judgment. This essay therefore begins by looking at standardization followed by language expectations and finally the judgment on language use.
Standardization of language is the process through which a language has been codified, this process also include grammar and spelling development. It is also through standardization that writing of a language in an official format becomes possible. Standardization is of great benefits to a language.
Through standardization learning and teaching of a language is facilitated. The benefits that results from standardization of a language are numerous but the main issue that follows standardization is who is responsible for standardization of a language. The next issue is of course the need for standardization and if there are some persons responsible for standardization.
In the past, language standardization process has been hijacked from the hands of linguistics. In fact, the process has been utilized as a way of creating identity for a state or a group of people. Institutions of learning have also been linked with language standardization process.
Politics have also played a big role in standardization of language with the bottom line being the creation of dominance. Different standards in language have also been responsible in the creation of social classes that are present in today’s world. Language has been viewed as a cultural capital and that it create the differences between social classes, the educated and the uneducated (Finegant PBS. Org ).
Deresiewicz emphasized on the role language standards play in society classification by stating that “mastery of standard English language was the ticket of cultural admission” (New York Times). This shows that although standardization of language is important for development of the language and people’s culture the process has often been misused.
In order for the full benefits of standardization to be realized then it is important that standardization of a language be done for promoting the language.
As stated, the natural process of language change still applies in standardization of a language and therefore the people should be the main stakeholders in the standardization process. The process should also be a natural process, which implies that the process should not be influenced externally.
Expectation of a language to people goes beyond a tool or means of communication. People therefore have varied expectations for their language. With language, people expect to share their personality and to express their message beyond their words. As claimed by (Baron PBS. Org ) people expect their “language to express their solidarity or group identity.”
For this reason, people expect language to create a distinction between genders, educated and uneducated. Expectation on language variation across gender is of great interest compared to the other expectations.
The main reason for this interest follows from the fact that there is a variation in language across gender even if other factors that cause language variation among people are constant. In the English language, for example “men are said to swear a lot, to be more coarse and casual.
Studies claim that American women know more color terms and men know tool names; that women use more qualifiers and diminutives” (Dennis Baron PBS. Org ).
Language change and use is subject to people’s judgment, people therefore judge a language as a bad and good language. How people use and judge a language determines its effectiveness and development. This in turn determines the survival and continued use of a particular language. As noted by (Nunberg PBS. Org ) “the English language will survive its current critics complain.”
This shows that even though the English language is under harsh judgment it has a well-established structures that will ensure its survival. Survival of a language should be therefore the key factor that should govern its standardization, expectation and judgment.
The use of any particular language reflects the changes that have taken place in the language. Language change is an inevitable natural process and people have not readily accepted these changes. Language standardization is essential in development of the language but the process has been applied for other varied reason.
People expect their language to serve a role that is beyond communication. After all is said and done language remains a subject of judgment from the people , where the judgment should be aimed at development of the language.
Works Cited
Baron, Dennis. Language & society Do you speak American, PBS. Org , n.d. Web.
Crystal, David. The Prescriptive Tradition . n.d. Web.
Deresiewicz, William. You Talkin’ to Me? New York times . 2005. Print.
Finegant, Edward. What is ‘Correct’ Language? Do You Speak American, PBS. Org , n.d. Web.
Nunberg, Geoffrey. The Decline of Grammar Do You Speak American, PBS. Org , n.d. Web.
- Chicago (A-D)
- Chicago (N-B)
IvyPanda. (2023, October 31). Standardization of language. https://ivypanda.com/essays/standardization-of-language/
"Standardization of language." IvyPanda , 31 Oct. 2023, ivypanda.com/essays/standardization-of-language/.
IvyPanda . (2023) 'Standardization of language'. 31 October.
IvyPanda . 2023. "Standardization of language." October 31, 2023. https://ivypanda.com/essays/standardization-of-language/.
1. IvyPanda . "Standardization of language." October 31, 2023. https://ivypanda.com/essays/standardization-of-language/.
Bibliography
IvyPanda . "Standardization of language." October 31, 2023. https://ivypanda.com/essays/standardization-of-language/.
- Standardization of English-Arabic Audiovisual Translation Practices
- Language Planning
- The Standardization vs. Adaptation Debate
- Andrew Jacobs’ Article “Shanghai Is Trying to Untangle the Mangled English of Chinglish”
- The Global Standardization Strategy
- The International Organization for Standardization
- International Standardization Organization
- Coca-Cola Company's Global Standardization Strategy
- Japanese Advertisement and Its Standardization for the US
- Marketing Standardization and Local Adaptation in the Hospitality Sector
- Language Exploration: Spanish and English
- English as a Global Language
- The Argument on the Specific Roles of Language, Knowledge, and Ideology
- Theories of the Language Evolution
- Globalization of the English Language: One of the Most Widely Used Languages in the World

An official website of the United States government
The .gov means it’s official. Federal government websites often end in .gov or .mil. Before sharing sensitive information, make sure you’re on a federal government site.
The site is secure. The https:// ensures that you are connecting to the official website and that any information you provide is encrypted and transmitted securely.
- Publications
- Account settings
Preview improvements coming to the PMC website in October 2024. Learn More or Try it out now .
- Advanced Search
- Journal List

How individuals change language
Richard a. blythe.
1 SUPA, School of Physics and Astronomy, University of Edinburgh, Edinburgh, United Kingdom
William Croft
2 Department of Linguistics, University of New Mexico, Albuquerque, New Mexico, United States of America
Associated Data
Relevant data and methods are within the paper and its Supporting information files. All codes and the raw data they work with are publicly available at https://git.ecdf.ed.ac.uk/rblythe3/gram-cycles which is a non-commercial git repository hosted by the University of Edinburgh.
Languages emerge and change over time at the population level though interactions between individual speakers. It is, however, hard to directly observe how a single speaker’s linguistic innovation precipitates a population-wide change in the language, and many theoretical proposals exist. We introduce a very general mathematical model that encompasses a wide variety of individual-level linguistic behaviours and provides statistical predictions for the population-level changes that result from them. This model allows us to compare the likelihood of empirically-attested changes in definite and indefinite articles in multiple languages under different assumptions on the way in which individuals learn and use language. We find that accounts of language change that appeal primarily to errors in childhood language acquisition are very weakly supported by the historical data, whereas those that allow speakers to change incrementally across the lifespan are more plausible, particularly when combined with social network effects.
Introduction
Human language is a multiscale phenomenon. A language is shared by a large population, that is, the speech community: it is a set of linguistic conventions, characteristic of the population as a whole. Yet language originates in individuals. Individuals in a population use language to achieve specific communicative goals, and through repeated interactions there emerge the linguistic conventions of the speech community. These conventions also change over time, and as speech communities split, the linguistic conventions of the speech communities diverge, leading to variation across languages.
How does the behaviour of individual speakers lead to change in linguistic conventions and ultimately the emergence of linguistic diversity? It transpires that this is one of the most debated questions in the study of language change for at least a century [ 1 ]. A widely-held view is that the locus of language change is in child language acquisition, in particular the process of inferring a grammar that is consistent with the sentences that have been heard [ 2 – 5 ]. Where these sentences do not fully specify a grammar, a child can infer a different grammar from its parents. If enough children infer a different grammar, then the language changes as the generations succeed each other. Variations on this basic idea exist, for example, where a child may have multiple grammars representing old and new linguistic variants, with the relative weighting of the two grammars shifting across generations [ 4 ]. A competing account is the usage-based theory [ 6 – 9 ], where linguistic innovation occurs at any point in a speaker’s lifespan, and speakers vary the frequencies that they use different structures incrementally across the lifespan [ 10 – 13 ].
One reason that this question has not been resolved during the century-long debate is that direct evidence of the origin of a change that develops into a new linguistic convention is generally lacking. Research in child language acquisition has demonstrated that children are very good at acquiring and conforming to the conventions of the speech community. In fact, the primary research question in child language acquisition is how children are so successful in mastering not only general rules of language but also the many exceptions and irregularities in adult language conventions [ 14 ]. Child-based approaches argue that children find the patterns rapidly on the basis of specific innate language structures, while usage-based approaches argue that child language acquisition is incremental and general patterns are expanded gradually [ 15 ]. The fate of any innovations that are produced in the acquisition phase tends not to be investigated in this line of research. Meanwhile, sociolinguistic research on variation and change begins with a situation in which the novel variant has already been produced, and in fact the novel variant is already changing in frequency on the way to becoming a new linguistic convention. It is virtually impossible to capture the innovation as it happens; linguists are always analysing situations in which the new variant is already present.
Hence linguists have tended to rely on indirect evidence that would shed light on the role of the individual in language change. For example, it has been observed that the sound changes that are produced by children—innovations, or “errors” from the perspective of adult grammar—are not the same as the sound changes that have been documented in language history [ 16 – 21 ]. However, the innovative variation produced spontaneously by adults in both sound and grammar is of the same type that has been documented in language history [ 22 , 23 ]. These observations support the usage-based theory over the child-based theory. Also, while children are extremely good at acquiring the linguistic conventions of adults, by late adolescence they develop into the leaders propagating a novel variant through the speech community, which suggests that language change does not originate in childhood [ 10 , 13 , 24 , 25 ].
Here we take a novel approach to addressing the question of the locus of language change in the individual: we quantify and compare the plausibility of different theories of individual behaviour in producing population-level language changes and the resultant worldwide diversity of language traits. We achieve this by introducing a mathematical model that allows us to test a variety of hypotheses about how individuals ultimately bring about language change at the population level. The model is applied to diachronic and crosslinguistic data of one common type of language change, the grammatical evolution of definite and indefinite articles, such as English the and a respectively. The evolution of articles can be analysed as a cycle of states in which a language without an article may develop an article which may then disappear, allowing a simple unidirectional model of innovation and propagation of a change in a finite set of states. We draw on data of attested changes in definite and indefinite articles for 52 languages, and on the cross-linguistic distribution of article states (620 languages for definite articles, 534 languages for indefinite articles; see below for further details).
Our model allows us to access a very wide range of different individual-level processes of language learning and use which appear in different combinations, whilst remaining amenable to mathematical analysis with methods from population genetics [ 26 ]. Specifically, we can estimate the likelihood of our set of empirical language changes at the population scale, given a certain set of assumptions on the behaviour at the individual level. This then means we can determine the regions within this model space that have the strongest empirical support. As we will show below, we find that explanations of language change that appeal exclusively to childhood language learning receive considerably less support than those that allow incremental change across the lifespan. Our analysis further suggests that the complex structure of social networks—in which the degree of influence that different speakers may have over others is highly variable—may play an important role in the diffusion of linguistic innovations.
Data and methods
In this section we first set out empirical properties of changes in articles that guide us towards a statistical model of language change over historical time at the population scale. The basic picture, illustrated in Fig 1a , is one in which the population is initially at some stage of the cycle, for example, the situation where there is no definite article (stage 0). As a consequence of individual speaker innovations, an article is occasionally introduced into the population by recruiting a pre-existing word for the article function. This is indicated by diamonds in the figure. In later stages, different linguistic processes lead to a divergence in form, reduction of that form to an affix and the loss of the form. Eventually, one of the innovations propagates so that its frequency , defined as the proportion of relevant contexts in which the innovation is used, rises to 100%. Once this occurs, the next stage of the cycle has been reached and the process begins afresh. Following [ 26 ], we refer to this population-scale model as an origin-fixation model : the introduction of an innovation that successfully propagates (denoted by a circle in the figure) is referred to as origination , and the point at which it reaches a frequency of 100% is called fixation .

(a) Origin-fixation model at the population scale, showing a transition between two stages of a grammaticalisation cycle (set out in Table 1 ). Innovations are repeatedly introduced to the population; most fail (diamonds), but some successfully originate a change that propagates and goes to fixation (circles). The fixation time T F is a random variable (see text). (b) Underlying individual-based (Wright-Fisher) model. Individuals are characterised by the frequency with which they use the innovation (orange portion of pie charts). In the case shown, individuals update their innovation frequencies by retaining a fraction 1 − ϵ their existing value, and acquiring the remaining fraction ϵ through exposure to one other member of the speech community. In the figure, ϵ = 1 2 for illustrative purposes. The two levels of description are connected by averaging over the individual speaker-level innovation frequencies in the Wright-Fisher model to obtain the population-level frequency plotted for the origin-fixation model.
This population-scale process is the product of interactions between individual speakers in the population, that is, acquisition or use, or a combination of the two. These interactions are illustrated schematically in Fig 1b and will be discussed in detail in the second part of this section. The individual-based model is very similar to the Wright-Fisher model in population genetics (see e.g. [ 27 ]), and we refer to it as such. In this model, each speaker is characterised by the frequency with which they use an innovation in the relevant linguistic context. The Wright-Fisher and origin-fixation models are connected by averaging over the individual frequencies to obtain the corresponding frequency at the population level. This then provides a quantitative model for language change over historical timescales that is grounded in individual speaker interactions.
Language change at the population level
Empirical properties.
We draw on two sources of data to characterise language change at the population level: (i) a survey of documented instances of historical language change (detailed in S1 Appendix ); and (ii) the typological distribution of the current stage in the cycle across the world’s languages (as recorded in the World Atlas of Language Structures, WALS [ 28 ]). As stated in the Introduction, we focus on definite and indefinite articles for this analysis. There are a number of reasons for this. First, the evolution of articles predominantly follows a single cycle of grammaticalisation. Definite articles are predominantly derived from demonstratives such as that [ 29 ], and indefinite articles are predominantly derived from the numeral one [ 30 ]. Both articles proceed to being affixed and then disappear. Second, articles are unstable: several find articles to rank among the least stable of a large set of features [ 31 – 33 ]. This means that our historical survey includes many documented instances of multiple stages in the article grammaticalisation cycle, which in turn leads to a more sensitive likelihood-based analysis than is possible when changes are rare. Finally, this instability implies that the current distribution of stages in the cycle across languages is likely to be close to the stationary distribution, which simplifies the analysis. Although articles are at one end of the stability spectrum, we expect that similar results to those reported below would be found for more stable features: we return to this point in the Discussion.
We divide the stages of the cycle following the classification of WALS Features 37A and 38A [ 28 ]: (0) no explicit article; (1) use of that and one for definite and indefinite article meaning respectively; (2) use of a distinct word usually derived from that or one for the article; and (3) use of an affix. WALS provides the current crosslinguistic distribution of these four stages for definite and indefinite articles (see Table 1 ). One can also look at the joint distribution of the two features to establish whether they are correlated. A χ 2 test on the contingency table indicates that the features are unlikely to be independent ( p < 10 −6 ; although the conditions for the validity of the χ 2 test do not strictly apply, this level of significance was confirmed by a Monte Carlo sampling procedure).
The number of languages in each state is taken from [ 28 ].
We collected data on the documented history of articles in 52 languages from multiple sources (see S1 Appendix ), and divided their history into the same four stages. Importantly, at any given point in time, one of these conventions typically dominates; over time the dominant convention changes to the next in the sequence 0–3 above, before returning to stage 0 via loss of the article. In our analysis of the 52 languages, we find only a single instance of a stage of the cycle that was skipped. For each article and language, we can estimate the rate of change as m + 1 t , where m is the number of changes observed and t is the observation period. (Technically, this is the mean of the posterior distribution over rates when the prior is uniform and the changes assumed to occur as a Poisson process.) We plot the distribution of these rates for each article in Fig 2 . This shows that the median rate of change is roughly once every 1000 years and that the distribution is somewhat skewed towards slower rates of change. Our survey further suggests that the time taken for a change to propagate is somewhat shorter than this, perhaps of the order of 100 years. We further find that, for any given language, the number of changes in one article is not independent of the other ( χ 2 test p = 0.00058; Monte Carlo estimate p = 0.0026). In the following we present results for the two articles separately, as combining probabilities from the two analyses is not justified when measurements are correlated.

The vertical dotted line indicates the median of the distribution.
Origin-fixation model
We use the historical properties of article grammaticalisation cycles, set out above, to flesh out our statistical model of the process at the population scale. Recall from Fig 1a the picture of an initial state in which all speakers are at a given stage of the cycle (say, stage 0), and as speakers interact, instances of the next stage are repeatedly introduced. In a child-based model [ 2 , 4 , 5 ], the next convention is introduced by children in the acquisition process. In the usage-based model, by contrast, the next convention is introduced in language use by speakers of any age [ 7 , 9 , 22 ].
Under whatever mechanism one has in mind, only some of the individual innovations are replicated sufficiently often that they become used by the entire population, reaching the frequency of 100% that defines the state of fixation and therewith the onset of the next stage of the cycle [ 22 , 23 , 34 ].
We assume that the rate at which speakers introduce a specific innovation (e.g., introducing a particular form for an article) in individual instances of acquisition or use is constant over time, as is the probability that this innovation then propagates and reaches fixation. This means that at any given stage in the cycle, origination events occur at a constant rate. In mathematical terms, origination is a Poisson process with rate ω i when the population is in stage i of the cycle (and so the innovations correspond to stage i + 1).
Specifically, we take ω i = ω ¯ 4 f i , where f i is the fraction of languages currently at stage i in the cycle ( Table 1 ). This choice ensures, for any value of the parameter ω ¯ , that the stationary distribution of the origin-fixation is one in which the probability of being at stage i of the cycle is f i , and consequently matches the WALS distribution (although our conclusions do not depend on this being the case). By including the factor 4 (i.e., the number of stages in the cycle) ω ¯ can be interpreted as a mean origination rate obtained by averaging over one complete cycle. In general we will treat this rate as a free parameter (see Results , below).
Once the originating innovation has entered the population, it takes a time T F , called the fixation time to become adopted as the convention by all speakers in the population. In origin-fixation models applied to the invasion of mutant genes in a biological population [ 26 , 35 ], the origination process is generally much slower than the fixation process, and T F is typically set to zero. This is not appropriate in the application to language change: the historical survey above suggests that T F is only one order of magnitude smaller than the time between the origination of a change. Moreover, T F is unlikely to be exactly the same for each change, due to the unpredictability of human interactions and individual speech acts.
We account for this unpredictability by drawing each fixation time T F from a probability distribution. The fixation time distribution can be calculated for certain individual-based models, such as the Wright-Fisher model set out below [ 27 , 36 ]. However, the mathematical form is too complicated to be of practical use, so we approximate it by the simpler Gamma distribution. This distribution is a natural choice for a quantity that is required to be positive (like a fixation time), and whose mean and variance can be controlled independently. In fact, we will arrive at the population-scale model by setting these two quantities equal to those that derive from an underlying individual-based model. Fig 3 shows the Gamma-distribution approximation to the fixation time distribution obtained numerically for the Wright-Fisher model with and without a selection bias. Although the Gamma distribution does not fit perfectly, it captures the location and width of the peak well, and is preferable to simply assuming that T F is zero.

In (a) the Wright-Fisher model has N = 100 individuals and no selection. In (b) N = 150 and s = 0.01.
We now provide a formal mathematical definition of the origin-fixation model that is equivalent to the verbal description above. Starting from stage i of the cycle, a time T O , i at which a change to the next stage in the cycle is originated is drawn from the exponential distribution
as is appropriate for a Poisson process. Then, the time T F from origination to fixation is drawn from the Gamma distribution
At this point, stage i + 1 is entered, and origination of a change to stage i + 2 can begin (by sampling a Poisson process and Gamma-distributed fixation time, as above).
The crucial point is that once these distributions are specified, one can compute the likelihood of the observed changes in our historical survey for any desired combination of parameters ω i , T F ¯ and σ F 2 . Specifically, we ask for the probability that a language in stage i at the beginning of the observation period reaches stage j by the end of that period. The set of periods, changes, and procedure for calculating the likelihood are detailed in S1 Appendix . In the likelihood calculation, each language is treated as independent of the others: we do however consider a mother and its daughters after a split as separate languages, so that changes in the mother language are not included multiple times in the sample. It is important to note that the origin-fixation parameters are not arbitrary, but depend on the underlying behaviour of individuals. A specific choice of individual-based model will lead to specific values of the parameters ω i , T F ¯ and σ F 2 , as we establish below.
Language change at the individual level
Wright-fisher model.
We now set out a model of language behaviour at the individual level which allows us to determine parameter values for the origin-fixation model in regimes of interest. We start with the fact that all theories of language learning and use involve the linguistic behaviour of one individual in the population being adopted (in some way) by another. Looking backwards in time, one can construct a ‘genealogy’ that shows who acquired linguistic behaviour from whom, analogously to the inheritance of genetic material under biological reproduction. It is well understood in population genetics that many superficially different individual-based models of inheritance generate a common distribution of genealogies [ 37 ]. Therefore, one obtains a generic and robust description of an evolutionary process by selecting a specific individual-based model that is adapted to the context at hand. Here we construct a model of the Wright-Fisher type [ 27 ] that allows us to manipulate key properties of the individual speaker, such as how often they can change their behaviour (though learning or use, as appropriate), whether biases towards or against the innovation are operating, and which other members of the speech community they interact with.
The basic structure of this model is shown in Fig 1b . Each circle in the figure represents an individual’s linguistic behaviour at a given point in time. Each individual uses the existing convention (stage 0 in the figure) some fraction of the time, and the incoming innovation (stage 1) the remaining fraction of the time. As in the origin-fixation model, we assume that at most two linguistic variants are widely used at any given time. A variable x n specifies the relative frequency (in the range 0 to 1 inclusive) that speaker n uses the innovation. For example, the left-most speaker in the figure is using the innovation in around x 1 = 1 3 of the relevant contexts at time t . In this work, we take x n to be an average over occurrences of a particular form of the article in a general Noun Phrase construction that expresses (in)definiteness of the referent of the Noun Phrase. The forms are: no article; article identical to a source form (demonstrative for definite article, the numeral ‘one’ for indefinite article); article distinct from source form; and article attached to noun. Although this general construction may be made up of more specific subtypes of Noun Phrase constructions, there is reason to believe that a regular trajectory of change emerges from the aggregation of occurrences over subtypes [ 38 ].
In the traditional Wright-Fisher model, x n takes only the extremal values 0 or 1. In a linguistic context, this corresponds to classic child-based models [ 2 , 3 , 5 ] in which a speaker’s grammar is specified in terms of binary parameters. Other models allow for intermediate values of x n : these include variational learning [ 4 ] and usage-based [ 15 ] models.
The innovation frequencies x n are updated at a rate R for each of the N speakers in the population. We define the update rule in a way that includes the child- and usage-based models as special cases. What these have in common is that, in an interaction, each individual is exposed to the behaviour of one other speaker in the population. Each then replaces a fraction ϵ of their stored linguistic experience with a record of the variant that was perceived in this interaction. That is, x n ′ = ( 1 − ϵ ) x n + ϵ τ , where x n ′ is the updated innovation frequency, and τ = 1 if the innovation was perceived in the interaction, and τ = 0 otherwise. Fig 1b illustrates this update for the case ϵ = 1 2 .
The child-based model is obtained when ϵ = 1. The update then corresponds to a child being exposed to the behaviour of a parent, applying some learning rule to determine if the grammar of the language corresponds to the convention or the innovation, and setting x = 0 or 1 accordingly. Importantly, the learning rule can allow the child to infer a grammar that is different from that of the parent: cue-based learning [ 39 ] is one mechanism that allows for this. A general model for such mechanisms can be obtained by introducing a probability η i that, given a behaviour that is consistent with the parent holding grammar i in the cycle, the child nevertheless adopts grammar i + 1 (for example, because the sentences produced by the parent are more consistent with the next stage of the grammaticalisation cycle). In the child-based model, the appropriate choice for the update rate R would be once per generation. Under these conditions, the timescale of the cultural evolutionary process of language change is necessarily tied to that of biological evolution (although the two processes differ in other respects, for example, the number and identity of parents).
By contrast, the usage-based model allows for the cultural evolutionary dynamics to proceed more quickly than their biological counterparts, as individuals interact many times in the course of a generation. However, the impact of each interaction is likely to be smaller, implying that the parameter ϵ that quantifies this impact should be small. Fig 1b illustrates the case of ϵ = 1 2 , in which after the update (time t + Δ t ), half of the usage frequency derives from their behaviour before the interaction (light shading in the figure), and the other half (dark shading) corresponds to whether a conventional or innovative utterance was perceived in an interaction with the speaker shown by the connecting line. As in the child-based model, there is a small probability η i that a conventional behaviour is perceived as an innovation. This can represent a variety of processes that might apply in single instances of use, e.g., auditory and articulatory constraints [ 40 , 41 ] or cognitive biases [ 41 – 43 ], along with indeterminacy in inferring a phonological form [ 22 , 34 ] or meaning [ 23 , 44 ], that may favour one construction over another (see e.g. [ 7 ] for an extended discussion of innovation in language change).
To complete the description of the Wright-Fisher model, we need to specify how the interlocutor —the speaker who provides the linguistic data to the learner (or listener)—is chosen. There are two components to this: (i) a social network structure; and (ii) a possible biasing of interlocutors based on their linguistic behaviour. We describe these in turn.
The social network is set up so that speaker i has z i immediate neighbours, with z i drawn from a degree distribution p z . Thus different individuals can have different numbers of neighbours. In the absence of the bias, each neighbour is chosen as an interlocutor with equal probability in an interaction. A generic model for social networks is the power-law degree distribution p z ∝ z −(1 + ν ) in which the exponent ν controls the heterogeneity of the network. Values of ν > 2 are regarded as homogeneous, in the sense that innovations spread in the population in the same way as on a network in which all speakers have the same number of neighbours (even though there is variation). When ν < 2, the networks become increasingly heterogeneous as ν is decreased: these feature a small number of highly-connected individuals and a large number of relatively isolated individuals. Evolutionary dynamics tend to run faster on heterogeneous networks [ 45 – 47 ], and there is some evidence that human social networks are heterogeneous (1.1 < ν < 1.3, [ 48 – 50 ]). Fig 4 illustrates the distinction between homogeneous and heterogeneous random networks.

The case ν > 2 (left) corresponds to a homogeneous network in which individuals all have a similar number of neighbours. The case ν < 2 (right) is heterogeneous: the central individuals are well-connected whilst the peripheral individuals are not.
The interlocutor bias is implemented by choosing a neighbour m with a probability proportional to 1 + sx m instead of uniformly. The selection strength s serves to favour (if s > 0) or disfavour (if s < 0) the innovation, which may originate in one of a number of processes. For example, in the variational learning framework [ 4 ], there is a systematic bias towards a grammar that parses a larger number of sentences. In a sociolinguistic setting, association between a linguistic variant and a socially prestigious group may lead to a bias towards (or against) that variant [ 10 , 51 ]. The case s = 0 describes a neutral model for language change, which has been discussed in the context of new-dialect formation [ 52 , 53 ].
We emphasise that a large number of models for language learning and use that have been discussed in the literature fall into the Wright-Fisher class, even though they may differ in detail and may not be presented as such. A non-exhaustive list includes those that appeal to cue-based learning [ 39 ], Bayesian learning from one or more teachers [ 54 – 56 ], variational learning [ 4 ] and usage-based models [ 57 ]. Moreover, the Wright-Fisher model has been used as a phenomenological model for changes in word frequencies [ 58 – 60 ].
We conclude this section with a formal mathematical specification of the Wright-Fisher model. The distribution P ( x , t ) of the innovation frequency, x , at the population level, at a time t after it is originated, is generally well-described by the forward Kolmogorov equation
in which a dot and prime denote derivatives with respect to t and x , respectively [ 27 , 61 ]. The parameters T M , s and N e correspond to a memory lifetime, an innovation bias and an effective population size, respectively. We emphasise that this equation applies between successive origination events, and describes the process by which the innovation propagates (rises to x = 1) or fails (falls to x = 0). Therefore the origination rate does not appear in this equation. However, it does enter into a correction factor, set out in S1 Appendix , that accounts for the possibility that a second origination occurs before either of these endpoints is reached.
The main difference between models within the Wright-Fisher class is how T M , s and N e relate to the parameters that apply to a specific model. In the present case, which has the set of parameters specified in Table 2 , we have T M = 1/( Rϵ ), s is as specified above and N e = N ( z ¯ 2 / z 2 ¯ ) / ϵ in which z is the number of neighbours a speaker has on the social network, and the overline denotes an average over speakers [ 45 – 47 ].
The parameters in the Origin-Fixation model that characterise the dynamics at the population scale can all be expressed in terms of those relating to the behaviour of individuals (see Data and Methods ).
In S1 Appendix we demonstrate that Eq 3 applies more generally than to the specific agent-based model set out here, and furthermore that the quantities T M , s and N e have a similar interpretation. This is achieved by considering a model that has many additional features—for example, ongoing birth and death of speakers, changes in social network structure and variation in interaction rates between speakers and over time—and showing that the changes in the innovation frequency x over short time intervals are the same as those described by Eq 3 . Therefore the results we present below do not rely on this model being an accurate representation of language learning and use.
Connection to origin-fixation model
We connect the individual to the population scale by determining how the parameters in the origin-fixation model (also specified in Table 2 ) relate to those in the Wright-Fisher model. The origination rates ω i are given by the formula ω i = NRη i Q ( ϵ / N ), where N is the number of speakers in the speech community, η i is the individual innovation rate per interaction, R is the interaction rate and Q ( x 0 ) is the probability that an innovation goes to fixation starting from some frequency x 0 . In the Wright-Fisher model, this initial frequency is x 0 = ϵ / N , because exactly one speaker uses the innovation with probability ϵ . We then have
This result is obtained by solving the backward equation that corresponds to Eq 3 (see [ 27 , 36 ] and S1 Appendix ). We see that the effective population size, N e (which depends on the actual population size N , the update fraction ϵ and the social network structure) plays an important part in determining the probability that an innovation propagates. It also determines how quickly an innovation may reach fixation. Numerical methods, described in S1 Appendix with the code available at [ 62 ], are used to determine exactly how the mean and the variance in the fixation time, T F ¯ and σ F 2 , in the origin-fixation model depend on the Wright-Fisher model parameters. Here we note that the characteristic timescale is of order T M N e when the bias s is small, and of order T M ln( N e ) when it is large, which turns out to have important consequences for the plausibility of the historical data for specific models of language learning and use in our analysis below.
In summary, then, our basic approach is to use the origin-fixation model to determine the likelihood of an observed set of historical language changes. The parameters in this model are obtained from an underlying Wright-Fisher model, so that we may understand—for example—which learning rates, biases and social network structure are more or less well supported by the historical data. As we have argued, our findings do not depend on the detailed structure of the Wright-Fisher model. The crucial component is that a speaker’s behaviour can be represented by an innovation frequency x , and that this is affected by learning from or using language with other members of the speech community over time.
We now compare the likelihood of the empirically attested set of language changes (detailed in S1 Appendix ) under different assumptions on the underlying behaviour of individuals in the respective populations. An appropriate measure for likelihood comparison is the Akaike Information Criterion, corrected for small sample sizes (AIC c , [ 63 ]), as the models we consider have different structures. It is defined as
where k is the number of free parameters in the model, n is the number of observations and L is the likelihood of those n observations, as determined from the origin-fixation model. An observation is the sequence of transitions between different stages of a grammaticalisation cycle over a specified historical time period for a given language, as tabulated in S1 Appendix . The number of observations is therefore the number of languages in the sample (52 for both articles).
The difference in the AIC c value between two models, denoted ΔAIC c , gives a measure of how much the model with the lower AIC c score is preferred over the other. Models with more free parameters (higher k ) can be dispreferred even when the data likelihood increases as a result of increasing parameters. For nested models, this increase is inevitable, but for models with different structures, AIC c remains valid as it is based on general information theoretic principles [ 63 ]. Given two candidate models and a sufficiently large number of observations, e Δ AIC c / 2 provides an estimate of the probability that the model with the higher AIC c better describes the data than that with the lower value. There is some freedom to choose the value of ΔAIC c at which one discards the inferior model. In this work we take a value of around 10 (corresponding to a likelihood ratio of around 150) as indicative of the model with the higher AIC c becoming too implausible to consider further. However since there is some flexibility in this regard, we will generally show the dependence of ΔAIC c on model parameters, so one can gauge the scale of the likelihood differences between models. It is important to note that such model comparisons do not in themselves validate the superior model: for this one needs to consider goodness-of-fit measures as well [ 63 ].
We begin by establishing a baseline against which different individual-level mechanisms of language change will be compared. In this baseline model, language changes occur at the population level as a Poisson process. We emphasise from the outset that this is not an individual-based model of language change: changes in the population occur autonomously without reference to individual speakers. Nevertheless this model helps to illustrate our statistical approach and, as we discuss below, it also provides valuable insights into why particular individual-based mechanisms are found to provide more or less plausible explanations of historical language changes at the population level.
Poisson baseline
In the baseline model, we assume that a change from stage i to stage i + 1 of the cycle occurs as a Poisson process at a constant rate ω i = ω ¯ / ( 4 f i ) in each population, where f i is the fraction of the world’s languages that is currently at stage i of the cycle ( Table 1 ). This factor of f i ensures that the stationary distribution in the baseline model matches the contemporary WALS distribution. This model is equivalent to the origin-fixation model of Fig 1a , with instantaneous fixation ( T F = 0). This model has one free parameter, the mean rate of language change, ω ¯ , which is estimated by maximising the likelihood of the data.
The maximum likelihood value of ω ¯ , the corresponding AIC c , a classical p -value and two goodness-of-fit statistics are presented in Table 3 . The p -value is the probability, within the model, of all possible transitions between stages of the relevant grammaticalisation cycle over the relevant historical period for each language whose likelihood is lower than the transitions that actually occurred. This p -value can be interpreted in the usual way, with a low p -value indicating a likely departure from the model assumptions.
ω ¯ is the maximum likelihood rate of change and AIC c the corrected Akaike information criterion. p is the cumulative probability of events less likely than the observation. Overdispersion measures goodness of fit, with values closer to 1 indicating a better fit. p and overdispersion are estimated from 10 6 Monte Carlo simulations of the process.
By itself, an AIC c score (or differences between them) does not furnish any information about how well a particular model fits the data. To gain an insight into goodness-of-fit, we consider the overdispersion of two random variables X (specified below) which quantifies the extent to which observed deviations of X from their mean values X ¯ within the model are consistent with the expected deviations. For a given observation, the overdispersion is defined as O X = ( X − X ¯ ) 2 / Var ( X ) , that is, the ratio of the observed square deviation to its expected value. If the overdispersion is close to 1, the deviations are as expected, and we conclude that the distribution of X is well-predicted [ 63 ]. For a given language, the two quantities X are: (i) the total number of language changes in the historical period; and (ii) a binary variable that equals 1 if at least one change occurred, or 0 otherwise. We average over all languages in the sample to obtain the single measure that is presented in Table 3 .
The low overdispersion scores suggest that this baseline model provides a good description of changes in the indefinite article, whilst it performs less well for the definite article. A likely source of this difference is the larger number of languages whose definite article changes rapidly compared to the indefinite article, as can be seen from Fig 2 . It is further possible that assumptions made about the data (for example, that the distribution of articles is stationary, that changes in different languages are independent, or, indeed, that the fixation time can be idealised to zero) do not strictly hold. We also remark that the second overdispersion measure is less sensitive than the first: however, it turns out that this is easier to calculate for individual-based models, and we will take a large deviation of this measure from 1 as providing a strong indication of a poor fit to the data.
It is remarkable that this simple model seems to provide a reasonably good fit to the data, particularly in view of an ongoing discussion about the role of population size in language structure and change [ 64 – 67 ] (a point we return to in the Discussion). The Poisson model explicitly assumes that the phenomenological rate of change ω ¯ is constant across all populations, and that each language change is able to propagate rapidly from origination to fixation. These observations suggest that we should expect to find more plausible accounts of historical language change in individual-based models whose emergent population-level dynamics share these properties.
Child-based models of language change
We now examine the constraints on the population-level dynamics of language change that arise from assuming that language change occurs primarily through the process of childhood language acquisition (e.g., [ 2 , 4 , 39 , 54 , 56 , 68 , 69 ]). As noted above, such theories imply that the rate, R , at which a grammar can be updated is once per human generation, which we take to be once every 25 years (i.e, R = 0.04yr −1 ). In the case where learning causes children converge on a single grammar (i.e., categorical use of one of the four article variants), we take ϵ = 1. In the case of variational learners (e.g. [ 4 ]), speakers can entertain mixtures of grammars: this can be realised with ϵ < 1. We consider the categorical case first.
The literature on child-based theories rarely refers to population structure. We therefore begin by assuming that populations are homogeneous: that is, that each child learns from roughly the same number of (cultural) parents, and conversely, that each adult provides linguistic input to roughly the same number of (cultural) offspring. Under these conditions, the emergent origination rates and fixation times in each population depends on a core size that is equal to the population’s actual size (see Methods ). It is therefore necessary for us to estimate the population (speech community) size for each language over the historical period for which empirical data exist. In S1 Appendix , we set out the procedure that we use to estimate the mean population size for each language over its recorded period of change. This is then used as the core population size for that language in our analysis.
This leaves just two unconstrained parameters, the mean rate η ¯ at which innovations arise in individual instances of language learning (the “error” rate, in the child-based model), and the selective bias s in favour of the innovation. Our strategy is to choose the value of η ¯ that maximises the likelihood of the data set given all other parameter settings, and to plot ΔAIC c with respect to the Poisson baseline model as a function of the selection strength s so that we can see where the support for the child-based model is strongest. Here, we treat the individual-based model as the candidate model, so ΔAIC c = AIC c (candidate) − AIC c (baseline) is positive when the evidence supports the baseline model, and negative when the evidence supports the candidate model. The resulting plot is shown in Fig 5 , along with a corresponding plot of the second of the two overdispersion measures considered for the Poisson baseline model.

ΔAIC c (panels a–c) and binary overdispersion (c–f) for negative (a and d) and positive (b, c, d and f) selection strength s within a child-based learning paradigm. The smallest values of both measures (which indicate better fits to the data) are obtained for strong positive selection ( s > 1, highlighted in panels c and f which has a larger vertical scale). The ΔAIC c values are far away from the shaded zone where ΔAIC c ≤ 10 and the evidence in favour of the child-based model starts to become comparable with that of the baseline.
We find that across the entire range of selection strengths s , support for the child-based model is very poor. The greatest plausibility (relative to the Poisson baseline) is obtained where ΔAIC c is smallest: this happens in the limit of infinite selection strength. As can be seen from the rightmost panels of Fig 5 , the values of ΔAIC c in these regions are still rather large, reaching asymyptotes at 204 and 58.4 for definite and indefinite articles, respectively (both to 3 s.f.). This corresponds to the evidence in favour of the candidate model being 10 44 (definite) and 10 13 (indefinite) times smaller than the baseline.
However, this comparison with the Poisson baseline is not entirely fair, as this phenomenological population-level dynamics may not be accessible for any combination of parameters in the individual-based model. For this reason we must also check the goodness-of-fit via the overdispersion measure. Again we find anomalously large values, the asymptotic values being 31300 (definite) and 226 (indefinite), suggesting that the assumptions made about the underlying dynamics of language change are wildly inconsistent with the historical data. Throughout this investigation, we found that ΔAIC c correlates strongly with goodness-of-fit, and so in the rest of this work we show only ΔAIC c , and investigate whether alternative assumptions on the individual-level behaviour are capable of delivering a much smaller ΔAIC c .
To focus this investigation, it is instructive to understand why the empirical data have such a low likelihood (and therewith high ΔAIC c ) within the child-based model. As previously noted, the effective population size (which here, is the same as the actual population size) is of fundamental importance in population genetics models [ 27 ]. When the selection strength, s , is large, each individual innovation is likely to propagate, and the mean origination rate (at the population level) increases linearly with the population size. On the other hand, when the selection strength is small, the origination rate is roughly constant but fixation time T F is proportional to the population size. Since the historical average population sizes in the empirical data set range across six orders of magnitude, then either the origination rate or the fixation time exhibits this wide variation in the child-based model. The fact that the Poisson baseline, which has no dependence on population size at all, apparently provides a much better fit, suggests that individual-based models in which origination rates and fixation times vary more weakly with population size than in the child-based model should be more favoured. Variants of the child-based model in which grammars are probabilistic [ 4 ] do not fall into this class: these have ϵ < 1, which implies a fixation time N / ϵ 2 when s is small. That is, these models are more sensitive to population size than models that allow children to acquire only a single grammar.
Usage-based models of language change
In a usage-based model, a speaker’s grammar may change across their lifespan [ 15 ], in principle in response to every utterance they hear (i.e., up to around 10 7 times a year [ 70 ]). This has the potential to weaken the sensitivity to population size: if a large number of interactions between speakers is required for a change to propagate through the population, then the higher interaction frequency in the usage-based model gives the change a greater chance of going through on the attested historical timescales. However, this effect may be tempered by the fact that the change to each grammar is smaller in each interaction, which has the opposite effect.
To explore the interaction between an increased interaction rate R , and lower impact on the grammar ϵ , it is convenient to work with the memory time T M = 1/( Rϵ ), which is the expected lifetime of a single item of linguistic experience in the speaker’s mind. Considering again the case of homogeneous populations, we compare in Fig 6 the class of usage-based models with no selection ( s = 0) over the reasonable range of R at fixed memory times T M = 1/( Rϵ ) against the baseline model. Note that the dotted parts of the curves correspond to an unphysical parameter value of ϵ > 1. From these ΔAIC c plots, we see that our intuition that an increased interaction rate allows changes to go through more easily is correct. We achieve greater plausibility than the most plausible child-based model when memory times are short, specifically less than one hour. We note that we can approach the plausibility of the Poisson baseline if we allow T M to be as short as one minute.

ΔAIC c in the usage-based model as a function of interaction rate R for the definite (panels a and c) and indefinite (b and d) articles. Along each curve, the memory time T M = 1/( Rϵ ) is held constant. In panels a and b, T M ranges from 25 years (top line) to 1 hour (bottom line). Panels c and d focus on the range of interest where greater plausibility than the child-based model is achieved: the horizontal lines correspond to the s = ∞ asymptotes in Fig 5 . Dotted lines indicate where the usage-based model is unphysical ( ϵ > 1) and the shaded grey region indicates where the fit starts to become comparable to the Poisson baseline (ΔAIC c < 10).
Although shorter memory times in the individual allow for a faster rate of change in the population, the basic property of fixation times being proportional to the population size is unaffected. This is why we find that individual memory times must be very short (perhaps unreasonably so, see Discussion ) to improve on child-based models. Furthermore, there is stronger sensitivity to population size when selection is operating ( s ≠ 0), which leads to lower plausibility gains with respect to the child-based model than in the neutral case ( s = 0). This suggests that one needs to appeal beyond merely shorter memory times to explain the apparently weak effect of population size on article grammaticalisation cycles.
Social network effects
Studies of the Wright-Fisher and related models on heterogeneous networks [ 45 – 47 ] show that these can weaken the effect of population size on characteristic timescales of change. As discussed in the Wright-Fisher model section, above, we model social networks as those with a power law distribution P ( z )∼ z −(1+ ν ) . We recall that the exponent ν controls the heterogeneity of the network, with lower values of ν corresponding to greater heterogeneity: see also Fig 4 . On such networks, the mean fixation time is proportional to an effective population size N e ∼ N 2−2/ ν which is less than the actual size N if 1 < ν < 2 [ 45 – 47 ]. In the context of language change, we can think of N e as measuring the size of a core population who exert much greater influence over the periphery than vice versa. Empirical studies of large networks (like friendship networks) provide some support for this power-law distribution with an exponent ν in the range 1.1 < ν < 1.3 [ 48 – 50 ].
In Fig 7 we examine how the plausibility of both the child- and usage-based models investigated above changes when individual speakers in the model are arranged on complex network structures. This confirms our expectation that models in which timescales of change are less sensitive to population size receive greater support from the data. As previously, the usage-based model provides a more plausible description of language change than the child-based model; moreover, the range of selection strengths and memory times over which a fit comparable to that provided by the Poisson process is much larger than on homogeneous networks.

ΔAIC c for models on heterogeneous social networks for the definite (panels a, c and e) and indefinite (b, d and f) articles as a function of selection strength s . Panels a–d show the effect of different degree exponents ν on the child-based model: panel c and d zoom in on ΔAIC c ≤ 100, showing that plausibility is obtained only for the indefinite article over a limited range of s and ν . Panels e and f show the effect of memory lifetime at fixed ν = 1.2 and ϵ = 1. The horizontal line has the same meaning as in Fig 6 . The dark and light shaded regions correspond to ΔAIC c < 10 and ΔAIC c < 20, respectively, which allows one to see the sensitivity to different evidence thresholds.
We see from Fig 7 that the most plausible models in the space under consideration are those in which selection is relatively weak. This is consistent with recent observations [ 58 – 60 ] that the dynamics of word frequencies appear to be subject to the evolutionary forces of both random drift and selection (i.e., neither is so strong that it dominates the other). Moreover, a number of studies (e.g., [ 46 , 71 , 72 ]) have indicated that heterogeneity tends to lower the barrier to invasion of an infection, mutation or innovation. This possibly points towards a picture whereby the different grammatical structures that are attested cross-linguistically are somewhat similar in their fitness, but may nevertheless replace one another over time in the systematic way that is observed historically due to the manner in which human societies are structured.
The aims of this work were twofold. First, we established how specific assumptions on the way in which individuals learn and use language translate to language change at the population scale. Second, we used historical data for the latter to identify which theories and mechanisms as to how individuals change the language of their speech community have greater empirical support.
Our main result is that if we impose the constraints that arise from assuming that childhood language learning is the driver of language change, there is no combination of the remaining free parameters that provides a good fit to the empirical data. The observed changes are many orders of magnitude more likely in regions of parameter space that correspond to other theories. The reason why the support for the child-based theory is so poor lies in a strong dependence of characteristic timescales at the population level on the underlying population size. If any selective bias in favour of the innovation is weak, the time taken for a change to propagate through a large speech community (the fixation time) is very much longer than the 100 years or so that is seen historically. If selection is strong, changes propagate quickly but then the rate at which successful changes are originated varies strongly with population size. The empirical data apparently show much less sensitivity to population size than the child-based theory implies.
In fact, throughout this work, we have found that the baseline model, which has no dependence on population size, fits the historical data well. One way to construe the baseline model is as changes originating once every 1000 years or so in every population, with changes then propagating rapidly through the population. This suggests that the mechanisms that have stronger empirical support are those that have these characteristics.
We acknowledge that our analysis is based on a single pair of features (the definite and indefinite articles) that are relatively unstable and are correlated. It is due to these correlations that we treated them separately (rather than combining them together into a single likelihood measure, which would assume independence). Nevertheless, comparison of the two articles is informative about how sensitive the analysis is to the details of which languages undergo a specific sequence of changes, as this does vary between the two articles. Overall, we find that it is the overall rate of language change combined with its weak sensitivity to population size that most strongly determines the plausibility of a given individual-based theory.
It is, however, possible that the dynamics of articles are unrepresentative of grammatical features more generally, and that our conclusions therefore do not generalise. We argue that this is unlikely. Regarding overall timescales of change, it is well established, by different analyses [ 31 – 33 ], that articles rank amongst the least stable of grammatical features and that others change more slowly. Basic word order lies at the opposite end of the spectrum, and the lifetime of given word orders have been estimated as ranging from 1000–100000 years [ 73 ]. That is, these most stable structures persist for a timescale that ranges from around the same order of magnitude as articles to two orders of magnitude longer. A quick way to estimate the plausibility of the child-based theory for basic word order from our findings for articles is to consider a generational turnover that is increased by two orders of magnitude (i.e., from 25 years to around 3 months). Here we find a plausible account is possible on sufficiently heterogeneous social networks (see Fig 7 ). This implies that the child-based theory could, at best, account for only the most stable grammatical structures, and does not offer a single explanation for language change that applies across the stability spectrum. The rate of population turnover imposes a fundamental minimum rate of language change which lies above that for unstable features in the child-based account, but potentially below in the usage-based account. Therefore the latter is capable of providing a common explanation for changes across the full stability spectrum.
It is harder to establish whether the weak sensitivity to population size is a feature of other grammatical changes. A detailed record of the history of each feature of interest across many languages is required for a conclusive assessment, data that is difficult to obtain (particularly for more stable features, where greater time depth is required to see a sufficiently large number of changes). However, a number of studies that have directly examined the relationship between population size and various aspects of language structure or change [ 64 – 67 ] have tended to conclude that where there is an effect, it is weak. For example, [ 67 ] reports rates of gain and loss that scale sublinearly with the population size, consistent with the behaviour of Wright-Fisher models on heterogeneous social networks. Moreover, the fact that different methods [ 31 – 33 ] of characterising the stability of a feature with a single metric are broadly consistent suggests that they do not vary significantly over space and time. Indeed, Wichmann and Holman [ 31 ] have argued that the notion of stability is intrinsic to a feature and does not vary geographically. Given these considerations, it seems reasonable to conclude that weak population-size dependence is a generic property of language change, and not peculiar to articles.
We have identified two individual-level mechanisms that may contribute towards such a weak effect of population size on the rate of grammatical change. The first of these is provided for by usage-based accounts of language change which allow individuals to modify their behaviour across their lifespan, not just in the childhood language acquisition period. With more opportunities for individual behaviour to change per unit time, these theories allow changes to propagate through large speech communities more quickly. If the bias towards the innovation (the selection strength, s ) is close to zero and the innovation rate per interaction is also small, changes at the population scale can then occur at roughly the same rate in different speech communities.
In addition to small selection and innovation rates, this mechanism further requires a short memory lifetime in comparison to the lifetime of an individual (days or less, depending on social network structure). Taken at face value, such memory lifetimes may be considered unreasonably short. Here, we advise caution. First, a short memory does not imply that individual speakers are continually changing their behaviour: individual speakers can remain constant in their behaviour for as long as those around them do. If innovations rarely propagate, then most speakers will be exposed to existing conventions and continue to adhere to them, even though during a period of change they may alter their behaviour relatively quickly, albeit in small increments. There is some evidence that such changes can occur in older speakers as well as younger speakers, for example, in a study of Montreal French [ 12 ]. Meanwhile, research on priming [ 74 , 75 ] shows that individual linguistic utterances can affect a speaker’s behaviour in interactions in the very short term before fading away. It would be worth understanding whether such effects could effect more permanent changes, for example, when a change is in progress in a speech community, as this might then imply a shorter effective memory time at the individual level than intuition grounded in everyday experience suggests.
The second mechanism that can reduce the sensitivity of grammatical change to population size are social network effects. Specifically, heterogeneous networks, in which a small number of well-connected speakers interact with a large number of poorly-connected speakers, lead to an effective population size (and therewith a characteristic timescale for change) that increases sublinearly with population size. Since this heterogeneity is a feature of certain social networks (e.g., those relating to phone calls, movie collaborations and social media [ 48 – 50 ]), it is reasonable to assume that this is a property of human social interactions more generally. It is interesting to note that sublinear relationships between rates of change and population sizes have been reported in other empirical studies of language change [ 64 , 67 ]. Heterogeneous social networks offer one possible explanation for this phenomenon. To investigate this possibility further, it would be interesting to obtain more concrete information about the structure of linguistic interactions as well as how these stratify by age. If it were found, for example, that children’s networks are more homogeneous than adult’s, then this would point towards adults playing a key role in propagating an innovation throughout the speech community.
Although our statements about the relationship between individual behaviour and population-level change are grounded in a specific model of individual behaviour, we do not expect them to change if a different model was used. The reason for this is that any model that involves individual agents basing some or all of their future behaviour on that displayed by others (whether through learning or use) is expected to fall into the Wright-Fisher class [ 37 ]. The precise relationship between parameter values in the individual-based model and those in the population-level origin-fixation model may vary between models: however, in any two models with similar memory lifetimes, innovation biases and social network structures would be expected to have the same behaviour at the population scale. In S1 Appendix , we demonstrate this in the case of an extended model in which all properties vary between speakers, in which there is turnover in the population and social networks change over time.
This is not intended to imply that every feasible influence on language change is contained within the Wright-Fisher model used here (at least, at some level of abstraction). For example, we have excluded the possibility of a conformity bias [ 76 , 77 ], wherein speakers suppress minority variants in favour of those in the majority. Such a bias however makes it increasingly difficult for innovations to propagate as the population increases in size, and therefore would be expected to exacerbate the problems of sensitivity to population size. We have also assumed that factors influencing individual linguistic behaviour are constant over space and time. Specifically, social factors like prestige effects have been excluded, and it would be interesting in future work to establish whether these lead more readily to plausible accounts of historical language change.
Supporting information
S1 appendix, funding statement.
The author(s) received no specific funding for this work.
Data Availability

- 5 Things That Cause Languages to Change
You might also enjoy…
- The 3 Hardest Challenges in Learning English
- 7 Reasons the UK is the Best Place to Learn English
After all, what a linguistic looking at the long-term picture might perceive as changes can look to people living through them simply as errors. If you were writing an essay, you wouldn’t start sentences with prepositions, refer to authors whose books you’re referencing by their first names, use “gonna” or “ain’t” or describe an academic’s theory as “awesome”. But these things might all be normal for essay writers in a generation or two. The essays you write today would seem similarly error-laden if you had to submit them fifty years ago, when “fantastic” primarily meant implausible or otherworldly, “hello” was still used as an expression of surprise as well as a greeting, and middle-class children were discouraged by their parents from using an expression as slangy as “hi”. Language also changes at a different rate at different times. Someone living in England in 50 AD wouldn’t be able to understand an Anglo-Saxon from 550 AD, but that Anglo-Saxon probably would be able to have a conversation with their descendant from 1050 AD. In turn, that person from 1050 would find their descendant in 1550 completely incomprehensible. But chances are that someone in 2050 would still be able to understand someone from 1550. After all, we’re still able to follow most of Thomas Cranmer’s “A defence of the true and catholike doctrine of the sacrament of the body and bloud of our sauiour Christ” , which was published in 1550, even if some of the spelling is a little tricky. In both of the periods of significant change in the examples above – 50 AD to 550 AD, and 1050 AD to 1550 AD, language changed as a result of migration and conquest: the arrival of the Anglo-Saxons in the early Middle Ages (which supplanted the native language altogether), and the Norman conquest in 1066 (which made English the language of the underclass). But language doesn’t change solely as a result of violent political turmoil. In this article, we take a look at some of the other things that make us change the way we speak and write.
1. Semantic bleaching

Semantic bleaching isn’t a particularly significant cause of linguistic change, but it is a fun one – and one that’s usually noticeable even when it’s happening. Have you ever heard anyone complaining about how everything gets described in hyperbolic terms these days? Your day is “great”, the film was “amazing”, your dinner is “delicious”, your weekend was “awesome”. You might wonder what’s wrong with having an OK day, going to see an entertaining film, eating a tasty dinner and having a good weekend. Yet didn’t that last sentence sound bland? If someone told you their day had been OK and their weekend was good, might you not wonder if they were actually feeling a bit down, and trying to hide it? The reason for that is semantic bleaching: the process whereby, over time, words lose their force and become less specific and weaker in meaning. There are countless examples: if you go back fifty years, words like “awesome”, “fantastic” and “incredible” meant awe-inspiring or beyond belief; now they just mean “very good.” Even “unbelievable” needs to be qualified – perhaps as “literally unbelievable” – so that it’s not understood to mean “a bit surprising”. In Shakespeare’s Much Ado About Nothing , there’s a moment that’s awkward for modern performances when the villain, Don John, is described as “this naughty man”. When Shakespeare was writing, “naughty” meant “evil” – now it’s just “badly behaved”, with childish connotations at that. Semantic bleaching is also the cause of a difference between US and UK English. US English retains the use of the word “quite” to mean “thoroughly” or “very”, but in the UK, the word has undergone semantic bleaching until it means “a bit”. If someone in the US and someone in the UK are both “quite happy”, the American is a lot happier than the Brit. What makes semantic bleaching an interesting example of how language changes is that it’s intuitive to see how it happens. In cultures that reward boldness and strength of feeling, no one wants to seem half-hearted, but in reaching for the strongest word to describe each situation, we naturally rob words of their force; if everything is “great”, then being “great” can’t be all that significant, and we look for ever-stronger words. Thankfully, this process has been going on for hundreds of years, so we can be confident we won’t run out of alternative ways to express ourselves.
2. Synchronic variation

Synchronic variation sounds like a complicated term, but it’s actually quite simple. It relates to our ability to understand that a word can have different meanings in different contexts. A lot of new words are not entirely new, in that they are not fresh combinations of sounds for that particular language; they’re combinations of sounds repurposed to have a different meaning. That might sound bizarre, but it makes more sense with an example, such as the word “lit”. If you were to hear the sentence “it was lit” out of context, you might not be able to guess the meaning. But if you were to hear your grandmother saying it, you might be able to assume that she means “lit” in the sense of “to light”, and she’s probably talking about a fire or a candle. If you were to hear a friend – or maybe a younger sibling – saying that something was “lit”, you’d understand it in the slang sense of “great”; you might guess that they’re talking about a party that they went to. The two meanings of the word have the same origin ; “lit” comes to mean “excellent” via an older slang sense of “drunk”. It’s easy to see how “everyone got lit”, meaning drunk, might have changed to “everyone was lit”, meaning drunk, excited, enjoying themselves, to “the party was lit”, meaning it was great, whether or not any alcohol was consumed. “Lit” meaning “drunk” is probably metaphorical, such as in the sense of people’s faces lighting up. But despite this shared etymology, you have no problems in understanding that in one context, “lit” could mean literally on fire, and in another, it could mean metaphorically on fire. Confusing as these things might sometimes be for learners of English as a foreign language, in general we have no problem with the idea that a word can have multiple meanings, connected or not. In fact, we deal well enough with this that words can survive with two meanings that are opposites; these are called contronyms. An example is the word “sanction”; it can mean to approve an action, or to oppose a penalty. There are many more such words . Our ability to deal with them gives language flexibility; if a word comes to mean something new, it doesn’t have to battle the old, useful word for its existence – the two words can instead exist side-by-side. And that means that language can change a lot more quickly than might otherwise have been possible.
3. Movement of people

As we noted in at the start of this article, movement of people is the most obvious driver of change to the English language. It was brought to this country through the migration of the Anglo-Saxons, altered through exposure to the Vikings, then the Normans. Crudely speaking, after that migration to Britain gave way to migration from Britain in terms of changing the language; as the British Empire grew and grew, the English language gathered vocabulary from invaded territories. In some cases, these were words for things that didn’t exist in the UK, such as “jungle” from the Hindi jangal , or “wombat” from the Dharug wambad . In others, it was a word for something that did exist, such as “bungalow” and “shampoo” (also both from Hindi). Sometimes the use of a word in English traces the history of Empire to an astonishing degree. The word “wallah”, which is common to several Indian languages and which means the person responsible for a specific thing or business (usually as a suffix – e.g. chai-wallah, the person responsible for the tea), first appears in English-language texts in the 1750s. That’s around the time that the British East India Company began to rule parts of the subcontinent. The word is used increasingly until the First and Second World Wars, when it begins to tail off, dropping down still further from 1947, when India gained independence. This can all be seen in Google’s fascinating ngram viewer ; the colonial history of a subcontinent mapped in a single word. Migration and political differences are also the reason behind many of the differences between American and British English. For instance, take the past tense of “to get” – if you’re British, you might say “got”, and look down on the American “gotten”. But “gotten” is actually the older form; it was in use on both sides of the Atlantic 300 years ago, at which point British people dropped it and Americans preserved it.
4. Simplification
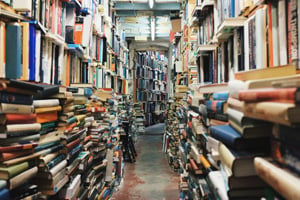
Language doesn’t have to feel the hand of history to cause it to change. Sometimes the factors that cause language to change are much more mundane – such as the desire to make things simpler. One example of this is that we generally prefer things that are easy to say. The word “daughter” comes from the Old English dohtor , in which the ‘h’ sound would have been pronounced – a little like the Dutch dochter . But in English, an “augh’ sound followed by a ‘t’ is tricky to pronounce. In “laughter” it turned into an ‘f’ sound but in ‘daughter’ the ‘gh’ sound vanished altogether, so that we now say “dauter”. In both cases, the spelling is the only thing that remains of the original pronunciation. We simplify our language in other ways, too. For instance, the word “parboil” used to mean to cook something thoroughly or completely. The word comes from the Latin per (thoroughly) and bullire (to boil), but to an English ear it sounds like “part-boil”. Having a word that sounds like it means one thing but actually means another is complicated; so the meaning eventually changed to match the sound of the word. And in some cases, the English language has been deliberately changed to make it easier to spell. When Noah Webster produced his famous dictionary, he changed “humour” to “humor” (which caught on only in American English), “musick” to “music” (which caught on everywhere) and “determine” to “determin” (which didn’t catch on anywhere), alongside dozens of other, similar changes intended to improve a complicated language .
5. Prestige

So if language tends towards simplification, then why is English still so complicated? One reason – among many – for this is that while we sometimes go for the laziest option in our speech and in our writing, that isn’t always the case. One force acting on all languages, where English has had its fair share, is the human desire to sound like people who we think are our superiors – whether that’s people who are higher up on the social ladder, who are better educated, or who we hold up as role models for whatever other reason. The most obvious example of this is the prestige accorded to different languages in the Middle Ages, which we have as a hangover in English even today. For a few hundred years after the Norman invasion, to be truly well-educated, you would be able to speak Latin. As a member of the nobility or gentry, you would speak French. And as a commoner, you would speak English. These divisions are crude and weren’t necessarily reflected on an individual basis, but it remains the case that lower-status words in English have Anglo-Saxon roots, higher-status ones have French roots, and academic language is littered with Latin. One example is the words we use to mean “like a king”: there’s the basic “kingly” (from Old English), “royal” (from French) and “regal” (from Latin). Even now, there’s an implied gradient of sophistication depending on your word choice. The same belief in the superiority of Latin led the spelling and grammar of English being altered to sound more like Latin. Writers of the 16th and 17th centuries added the letter ‘b’ to “doubt” and “debt” to make them resemble their Latin origin, and even more ridiculously, a ‘c’ to “scissors” to resemble the Latin scindere , which – as it turns out – is not actually the root of the word “scissors” at all. But as everyone who’s tried has discovered, once language has changed in a particular way, it’s very hard work to change it back. Images: friends around campfire ; cluttered bookshop ; king ; two vikings talking
How to Write a Process Essay
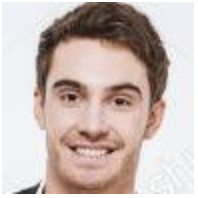
The process essay, also known as the "how-to" essay, is commonly written for people or companies that need tutorials or a set of instructional steps. Whether it's building a robot or cooking a chocolate cake, process essays use a similar format for any variations. They follow a step-by-step style, with the initial step influencing the second, which influences the third, and so on. Each step carries its own importance, and a poor explanation of one step can ruin the entire process. It's important to stay concise and efficient. However, before you begin writing your essay, you should do some small preparations. Let's discover them with our research writing service .
What Is a Process Paper?
A process essay is a type of essay that explains a process step by step and gives guidance for a certain process, working mechanism, procedure, etc. Process essays range from very simple ones, such as instructions for how to ride a bicycle, to more complex ones, such as a chemistry lab report of an oxidative reaction experiment. The goal of a process paper is to give its readers guidance and directions.
Feeling Overwhelmed Writing a Process Essay on Your Own?
Simply send us your paper requirements, choose your paper writer and we’ll get it done fast.
A process paper is characterized, first of all, by explaining a process using a description. Some words that are frequently used in process essays are “further”, “then”, “next”, “first”, “last”, “finally”, and “initially”. It is really important to remember that every process essay includes features, such as:
- clear and straightforward narration - the last thing you want to do is to confuse your reader with complex language and an unorganized thought thread;
- chronological order - avoid skipping steps and shifting them around, as it will result in misunderstanding and frustration for the readers;
- transition words - make sure to separate the next step from the previous ones by using transition words;
- descriptions of the steps - make sure your steps are clear and easy to follow.
There are several types of process essays. The first one is directional - it explains the “how to” for something. It can take on a wide range of subjects, such as how to apply for a credit card, how to get your driver’s license, how to plan a wedding, etc. The outcome of the directional essay should be a result. In the cases of the examples above: a credit card, a driver’s license, or a carefully planned wedding. The other type of process paper is informational - it explains how something works. Here are some examples: how a weather forecast is determined, how a space rocket works, how intermittent fasting changes your body, etc. An informational essay explains something to a reader and does not necessarily end up with a result, like directional does.
Another type of essay that is similar to a process essay is a process analysis essay. The biggest difference is that a process analysis essay not only explains the steps, but also analyses them in depth. It has all of the characteristics of a process essay, although goes into more detail about the causes and consequences of every step.
If you need any additional information for process analysis essays, check out our article: HOW TO WRITE A PROCESS ANALYSIS ESSAY
Writing a process essay is not extremely difficult. By following simple rules and a set of steps, a successful, well-structured essay can be guaranteed.
Prepare The Small Stuff
Here we gathered some small general tips and advice that you should follow throughout your writing process to make sure that all of the expectations of a process paper are met.
- Determine the Audience's Skill Level. It's important to base the level of complexity of the essay on who the readers will be. For example, if you need to teach a friend how to do a simple fix or create a certain tool, then it would be most reasonable to stick to more basic terminology. However, if you are writing an essay for your astrophysics professor about the creation of a black hole in the universe, use more sophisticated and informative terminology.
- Make a List of Materials. Obviously, the creation of anything comes with some prerequisites. Whether it's items or ideas, the importance of knowing the necessities beforehand and having them ready to go is essential. Make sure to place each item in accordance with its importance. The more impactful a part is, the higher up on the list it should be.
- Write out Each Task. In a step-by-step tutorial, each individual task carries some sort of weight. Since an entire process can not be complete if a step is skipped, it's crucial to write out every single step. However, don't go overboard in your explanations. It's not necessary to bring the tutorial to a microscopic level, but each step should be understandable and competent.
If you still have difficulty writing, you can get essay help online from our service.
Process Essay Topics
Choosing a topic for a process paper can be quite challenging. A good place to start is with your passions. If you pick something you are excited about, you can make it interesting for your readers and fun for yourself to write about. If your professor limits you to write a process essay on something you have very little knowledge of, choose a topic that is intriguing and triggers your interest. Then, conduct enough thorough research to make sure you understand everything perfectly before you go ahead and try to explain it to someone else.
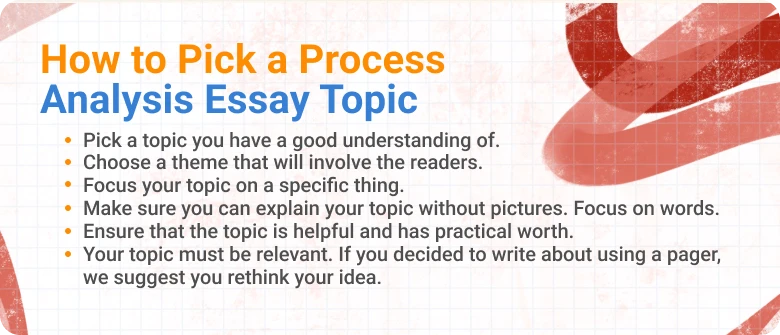
Another very important thing to consider while writing a process essay is your audience. It is highly unlikely that college students are interested in instructions for “How to Get Into Your Dream School” or “How to Pass Your SATs”. Make sure your topic relates to the subject you are studying and you are following your professor’s prompt guidelines.
Here are some ideas that might be of interest for you:
- How to lose weight on a keto diet
- How your immune system fights COVID-19
- How to start selling on Amazon
- How to improve your credit score
- How to decrease your social media usage
- How to apply for unemployment insurance
- How to improve your college performance
- How to open your first bank account
It's important to note that these essay topics are just some common examples used by several college students for their course papers. Feel free to use any one of them if you want, or think of one on your own. Just make sure it's a PROCESS!
Process Essay Outline
Most essay outlines follow the standard scheme: Intro > Body Paragraphs > Conclusion . follow the standard scheme: Intro > Body Paragraphs > Conclusion. A good process essay outline should look like this:
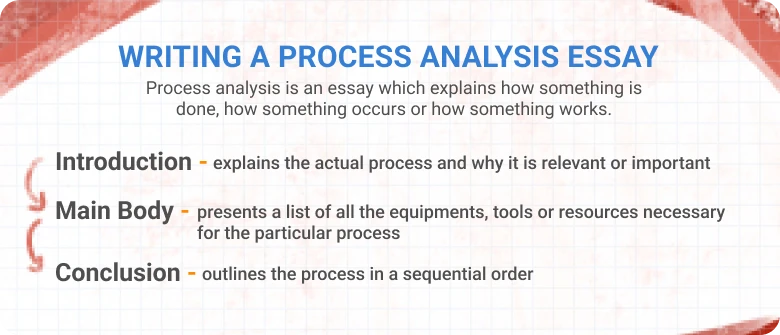
- Introduction — brief your reader on your topic, explain why you have chosen it and how you are planning to approach the explanation of the process.
- Body — the biggest part of your essay that should be divided into paragraphs for easier understanding and structure. Make sure each paragraph is flowing smoothly into the next one with connective words.
Paragraph 1. First step of the process. Explain what the step is, what the best way to perform it is, and how to avoid common mistakes when doing it.
Paragraph 2. The next step of the process (the same as in Paragraph 1). Thoroughly explain what this step is about.
- Conclusion . Here you need to explain why your instructions are valuable. It is your opportunity to persuade your reader(s) that the steps you presented and the process they learned will be useful for them in the future.
Every process is different: some can take a couple of minutes, while others can take months or years to complete. The length of the essay is generally based on the difficulty and number of steps it takes. However, the structure doesn't maneuver.
Introduction
The first thing that you want to do as a writer for your process paper is to help your readers be interested in your individual process. Be descriptive about it, paint a picture for your readers. A joke or a personal reference can be a great attention grabber and can pull your reader right in. For somebody to be keen on approaching your process, they have to express interest in it. Though, it generally goes without saying that many writers ignore this fact. Let's break it down into subsections:
- Give a little bit of historical background. People often want to know the origins of whatever it is that they're working on. Introducing this part of the process helps to intrigue your readers, as well as give them a sense of purpose for the task.
- Create an approximate timeframe. Unfortunately, your readers don't have all day to spend on this one event. In addition to learning about its purpose, people want to know how long the task will take. This way, they can decide how to break up the work. If it's a quick fix, then they can knock it out in one session. However, if it's a large-scale operation, then your readers will obviously have to create their own time schedule.
For example, let’s say that the topic of your essay is “How to Save Money”. You can start the introduction of your process essay by explaining that as a college student, you often find yourself in need of extra money and you are stuck with bad money habits. This will create a good connection with your readers, because almost everyone has been in a situation of needing to be savvy with their finances. Another thing you can mention is the importance of saving money and the multiple opportunities it presents, such as being able to invest it, being able to pay off a credit card debt, or being able to save up to avoid taking out a student loan.
Feeling Overloaded and Stressed?
Our professional writers are ready to help you 24/7!
Body Paragraphs
This is the point in the process essay where you start introducing the step-by-step process your readers will need to take. A lot of the time, it helps to break down each process into subsections. For example, if a step has many parts to it, it would be clever to create a paragraph on its own just for that step. Remember, it's important to keep things smooth and efficient. Break down the body paragraphs in unity with the steps. Let's go into more detail about each step:
Each step should be carefully explained. Every step will vary in length. Think about it: every instruction manual has several steps. Some are more difficult to comprehend or perform than others. For this reason, create your steps and explanations accordingly. You should be able to get a sense of their length and difficulty based on the explanation.
Don't forget to explain the purpose. People don't want orders barked at them aimlessly. Besides just accomplishing a task, people want to learn as they perform.
- Why did they do this?
- What was the purpose of this method?
- Why did we do it this way and not this other way?
To make everything flow smoothly use transitions. Make the steps flow one after another to create a well-structured essay. As you introduce the next step, consider using transition words like “next”, “now”, “then”, “so that”, etc.
Before writing the steps out in full sentences, it is a great idea to create an outline for your body paragraphs. Here is an outline for the body paragraphs of a process essay on “How to Save Money”:
Paragraph 1:
- keep track of your expenses
- organize your collected data
- decide what you can skimp on in your spendings
Paragraph 2:
- create a realistic budget
- check weekly to ensure you are sticking to it
- save 15% of every monthly income
- set a tangible goal for saving, such as a car
These process essay examples use only two body paragraphs, but feel free to include more to ensure a better understanding and cohesive flow for your paper. Although, do not include excessive unnecessary details that clutter your essay and make understanding it even more difficult. While writing your essay, include small brief explanations for each statement. For example, “Even though eating out and grabbing a coffee on your way to class sounds tempting, setting a budget and saving 15% of each of your monthly earnings aside can help you have enough to put down a downpayment on a new car.” Here the reader will understand that there is a direct relationship between each step and the result it is going to give.
Showing the readers that they are learning and not just repeating is one of the most effective ways to lock down their attention and keep them coming back!
After going through every step meticulously and explaining the whole process, a process essay needs a confident conclusion. This paragraph should be short, sweet, and to the point. It's main goal is to accomplish the following tasks:
- Discuss the main result. After the readers have completed the process, they should be left with a final result. It's important that you explain to your readers what the end result will look like, and what can be done with it.
- Restate the process’s general purpose. After completing the task, you obviously would like to know its overall purpose. When your readers feel that they have accomplished a challenge, learned something from it, and have a path to take the result towards, they will be satisfied!
- State your Overall Conclusion. To put a pretty tie around your process essay means that you need to neatly wrap things up! Restate some of the highlightable points as well as the process’s key overall purpose. Make sure that your readers feel accomplished after going through your process, and ensure that you strengthen the necessity of its purpose with a nice concluding sentence!
The conclusion of an essay on “How to Save Money” would explain that the completion of all of the steps will result in saving money that can be used for a specific goal or for rainy day fund purposes. You can mention the importance of every step and briefly repeat some of the key points.
Post-Writing Tips
Here are some final tips to wrap up your writing process. Use them as a checklist for a successful and coherent essay.
- Make sure the work is simple enough to follow. Worst-case-scenario: its author creates a feeling of absolute confusion in the reader’s mind. To avoid this problem, always remember that your readers can be beginners. Do not try to impress them with complicated words or sentences, use simple language to provide clear directions on how to do something. Give as many details as possible, but do it plainly. "Why is he making me do this?" "What was the purpose of this?" "I don't understand this step at all!" If the reader is asking themself these questions, then it's time to do some editing!
- Experiment and try it for yourself or ask a friend. There's no better way to experience success than to actively attempt your process through your own instructions. If everything truly makes sense, then you should have no problem solving the task using your own words. Even better, ask a peer to try it through your words to get an outside point of view.
- Choose the right topic for you and research it well
- Maintain a logical order of steps, make it easy to follow
- Avoid using imperative sentences - you do not want to sound like an Apple TV manual
- Explain terms that are most likely outside of most people’s range of common knowledge
If you have a ready-made essay but need to make significant changes to it, you can use our rewrite my essay service .
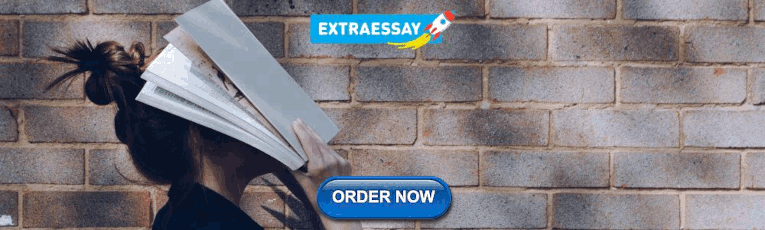
Process Essay Examples
Now that you know all about process essays and how to write them, we have prepared some great essay topic ideas in case you are stuck and cannot choose one:
Building a business from scratch is an intricate process that entails a number of steps. Each of these steps should have specific objectives and measurable outcomes.The following analysis gives the basic steps followed when building any business from scratch.
Saving can be defined as a differed consumption or keeping aside a portion of your income for unexpected future uncertainties or plans. Read for reason and actual steps for saving more money
Read also a thesis statement example from our author. In this article, you can learn something useful for yourself.
Still Need Help?
If you still feel like you could use some help with your process essay, do not hesitate to seek help from our writing service. Our writers specialize in a wide range of essays of different types including creative writing essay , process essays, and would be more than happy to assist you with writing, editing, or direction if you are feeling uneasy. Click the button below and college admission essay writer will process your requests fast.
Related Articles
.webp)
How to write a process essay
- August 25, 2023
Process essays are one of the most common types of essays . It’s simply explaining a process of how to do something.
In this article, we’ll show you how to write a process essay in steps with interactive examples.
Process essay definition
Let’s take a look at the steps outlined below to write a clear and effective process essay.
Choose a topic
You should start by choosing a topic that not only interests you but also attracts your target audience.
Whether it’s brewing the perfect cup of coffee or conquering the art of origami, your topic should be engaging and well-defined. Let’s have a look at topic examples:
- Crafting Exquisite Miniature Bookbindings
- Building a Sustainable Vertical Garden
- Making the Perfect Cup of Coffee
So for this guide, I’ve chosen “Making the Perfect Cup of Coffee”. Now let’s continue with the next steps.
Create a process essay outline
Now that you’ve your topic at hand, it’s time to create an outline to present the steps chronologically. Outline will also help you organize your thoughts and ideas so you won’t get lost during the writing process.
Let’s examine this step with an example of a process essay explaining “Making the Perfect Cup of Coffee”.
Process essay outline example
- Thesis statement
- Provide safety precautions if necessary.
- Address to the reader
- Provide any variations or customization options if applicable.
- End with a memorable concluding thought or call to action.
By presenting the steps in chronological order, your readers can follow the process smoothly.
During this step, just make sure to:
- Expand on each step you outlined earlier.
- Use clear and concise language.
- Make use of bullet points or numbered lists to make the process visually appealing.
After completing the outline, it’s time to write an interesting introduction.
Write an introduction
- Hook the reader's interest with a hook sentence
- Offer a brief overview of the topic and its significance
- Introduce and explain the process with a thesis statement at the end of introduction
Process essay introduction example
Introduction
Now that we have an intro on our hand, you need to tell what materials you need to finish the process.
Write the materials needed for the process
Listing the necessary materials for the process is a best practice for process essays. Typically found just after the introduction, this paragraph is devoted to outlining the necessary materials.
Here, p rioritizing the list is important; the more influential a component is, the higher its position on the list should be.
Example material list for process essay
Body paragraphs
Materials needed
- High-quality coffee beans that align with your flavor preference.
- A grinder for optimal flavor extraction.
- Equipment for brewing methods, such as a pour-over apparatus, a drip coffee maker, a French press, or an espresso machine.
- Fresh and clean water for brewing.
- Optional additives like milk, cream, sugar, flavored syrups, or other preferred elements.
Start writing the process
Right after listing the materials needed, it’s time to start writing the process itself.
When describing your process, be careful not to make it too complicated. To keep your readers on track, use transitional words like “after,” “eventually,” “first,” “then,” and others help you maintain an understandable tone.
Or simply use a 1,2,3, bullet point structure as seen in example below to remind readers of their step during the process.
Body paragraphs - Process writing example
Materials needed ...
- Grind the beans just before brewing for optimal freshness. Use a burr grinder and adjust the coarseness to match your brewing method (coarse for French press, fine for espresso).
- Weigh your coffee grounds using a scale. A standard ratio is 1 to 2 tablespoons of coffee per 6 ounces of water, but adjust to your taste.
- Ensure the water is heated to the ideal temperature, typically between 195-205°F (90-96°C). Water that's too hot or too cold can affect the taste.
- Pay attention to the brewing time. Generally, 4-5 minutes is suitable for most methods, but again, adjust based on your preference.
- After finishing, regularly clean your coffee maker or French press to prevent rancid oils and residue from affecting your coffee's taste.
As seen from the example above, using an imperative language structure is generally preferable. It makes total sense as you’re describing a process in steps and usually don’t need a full sentence structure.
Give tips and supporting details
After explaining the process above, it’s now time to provide tips and supporting details. Here, make use examples, tips, and even warnings if necessary.
In other words, anticipate the questions your readers might have and address them as you go along.
Body paragraphs - Supporting details
Supporting details & tips
- Ensure safe handling of hot water and coffee-making equipment.
- Water that's too hot can result in over-extraction, while water that's too cold won't extract enough flavor.
Write a conclusion
At this step, you simply need to write a conclusion paragraph to end your process essay. First summarize the key points, and restate the process in a concise and short sentence. And finally, finish your process essay by a memorable sentence or a call-to-action.
Process essay conclusion example
Revise and polish your essay.
Now that you’ve written your essay, take a breath, and then come back for some editing. Check for consistency, correct sentence structure, efficient transitions , tense selection , and other linguistic issues that may arise.
If possible, make use of proofreading tools like QuillBot or Grammarly .
- Think about potential reader misunderstandings and address them. If needed, explain what should be avoided.
- Offer explanations for steps that might seem unusual or complicated.
- Define any unfamiliar terms or materials that the reader might not understand. This ensures clarity in your essay.
So you’ve successfully learned how to write a captivating process essay. Remember, practice makes perfect. The more you write, the better you’ll become.
Recently on Tamara Blog
How to write a discussion essay (with steps & examples), writing a great poetry essay (steps & examples), how to write a process essay (steps & examples), writing a common app essay (steps & examples), how to write a synthesis essay (steps & examples), how to write a horror story.
How to Write a Great Process Essay
Otherwise Known as a How-To Essay
CSA-Archive / iStock Vectors / Getty Images
- Writing Essays
- Writing Research Papers
- English Grammar
- M.Ed., Education Administration, University of Georgia
- B.A., History, Armstrong State University
How-to essays , also known as process essays, are much like recipes: They provide instruction for carrying out a procedure or task. You can write a how-to essay about any procedure that you find interesting as long as your topic fits the teacher's assignment.
Start by Brainstorming
The first step in writing your how-to essay is brainstorming. Here are tips to help you:
- Draw a line down the middle of a sheet of paper to make two columns. Label one column "materials" and the other column "steps."
- Write down every item and every step you can think of that will be needed to carry out your task. Don't worry about trying to keep things in order yet. Just empty your head.
- Number your steps on your brainstorming page. Just jot a number beside each item/step. You may need to erase and scribble a few times to get the order right. It's not a neat process.
Create an Outline
First, determine the format required for your essay; ask your teacher if you are unsure. Your essay could contain a numbered list (like the one in the previous section), or it could be written as a standard narrative essay. If you are instructed to write a step-by-step without using numbers, your essay should contain all the elements of any other essay assignment, including an:
- Introductory paragraph : the section that identifies the topic , arouses interest, and prepares the audience or readers for the development of the thesis
- Body : the part of an essay that develops the main idea
- Conclusion : the sentences or paragraphs that bring the essay to a logical end
Regardless of the essay format—whether your teacher allows for numbered paragraphs or sections or simply wants you to craft a narrative report—your outline should center on these three areas.
Creating the Essay
Your introduction will explain why your topic is important or relevant. For example, your paper about "How to Wash a Dog" would explain that dog hygiene is important for your pet's good health.
- Your first body paragraph should contain a list of necessary materials. For example: "The equipment you will need depends somewhat on the size of your dog. At the very minimum, you will need dog shampoo, a large towel, and a container large enough to hold your dog. And, of course, you will need a dog."
- The next paragraphs should contain instructions for following steps in your process, as enumerated in your outline.
- Your summary, or conclusion, explains how your task or process should turn out if it is done correctly. It may also be appropriate to restate the importance of your topic.
Topics to Write About
You may believe that you are not expert enough to write a process essay. This is not so. There are many processes that you go through every day that you can write about, including:
- How to make a perfect paper airplane
- How to dye your hair
- How to wear makeup
- How to survive a weekend with your family
- How to play basketball
- How to play (a popular video game)
The goal in this type of assignment is to show that you can write a well-organized essay and clearly explain to the reader how to do what you are instructing.
- 6 Steps to Writing the Perfect Personal Essay
- How to Write and Format an MBA Essay
- How To Write an Essay
- List of Topics for How-to Essays
- Write an Attention-Grabbing Opening Sentence for an Essay
- How to Write a Response Paper
- How to Write a Narrative Essay or Speech
- What Is Expository Writing?
- How to Write a Great Essay for the TOEFL or TOEIC
- Tips on How to Write an Argumentative Essay
- How to Structure an Essay
- The Five Steps of Writing an Essay
- The Introductory Paragraph: Start Your Paper Off Right
- How to Write a Solid Thesis Statement
- 10 Tips for the SAT Essay
- Writing Cause and Effect Essays for English Learners
Free Paraphrasing Tool
Try our other writing services

Avoid plagiarism in your paraphrased text
People are in love with our paraphrasing tool.
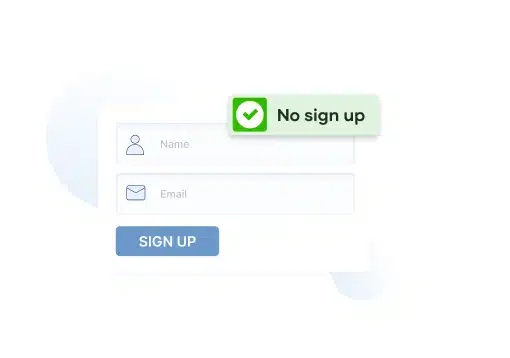
No Signup Needed
You don’t have to register or sign up. Insert your text and get started right away.
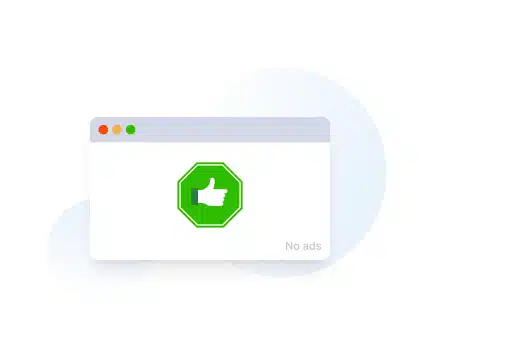
The Paraphraser is Ad-Free
Don’t wait for ads or distractions. The paraphrasing tool is ad-free!
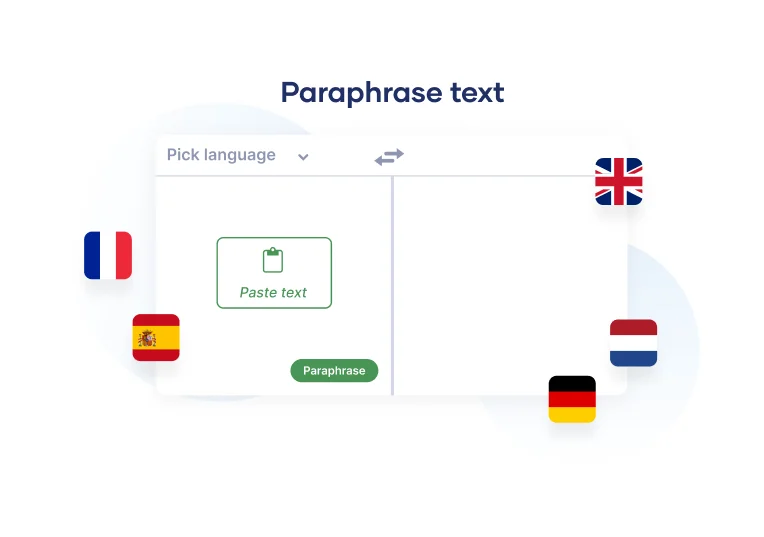
Multi-lingual
Use our paraphraser for texts in different languages.
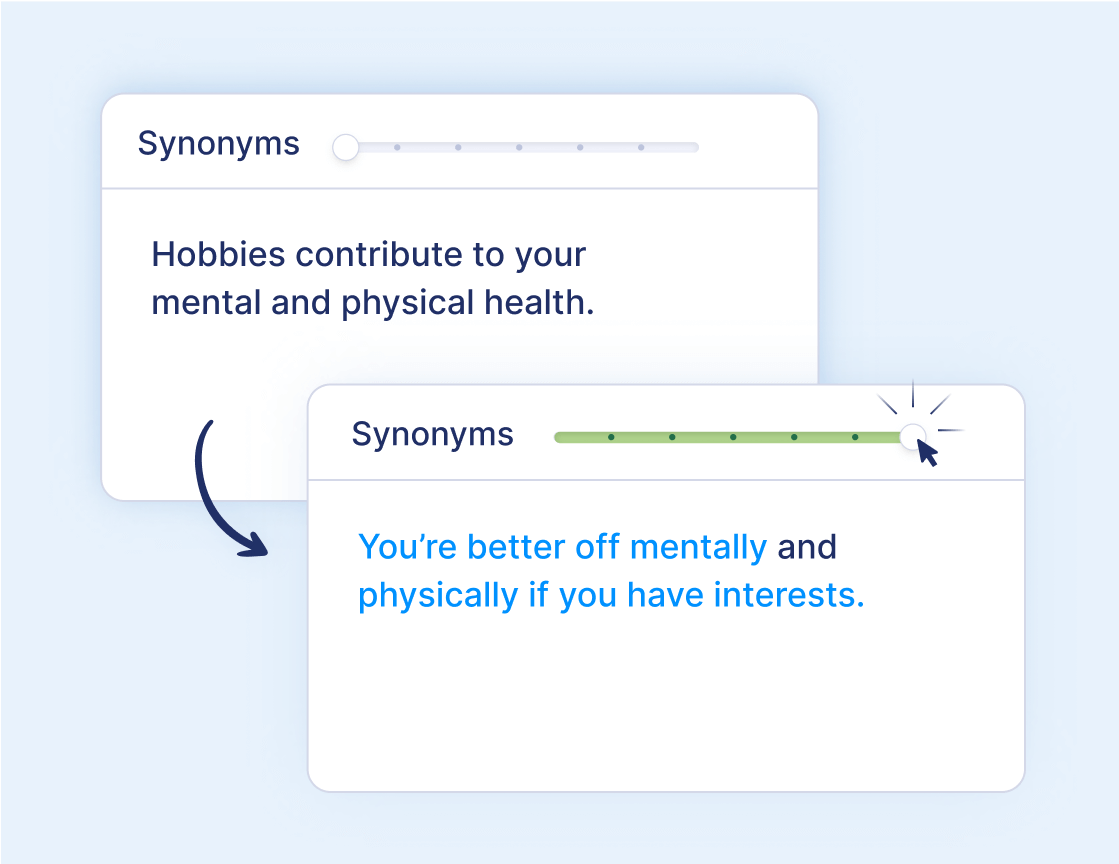
What's a paraphrasing tool?
This AI-powered paraphraser lets you rewrite text in your own words. Use it to paraphrase articles, essays, and other pieces of text. You can also use it to rephrase sentences and find synonyms for individual words. And the best part? It’s all 100% free!
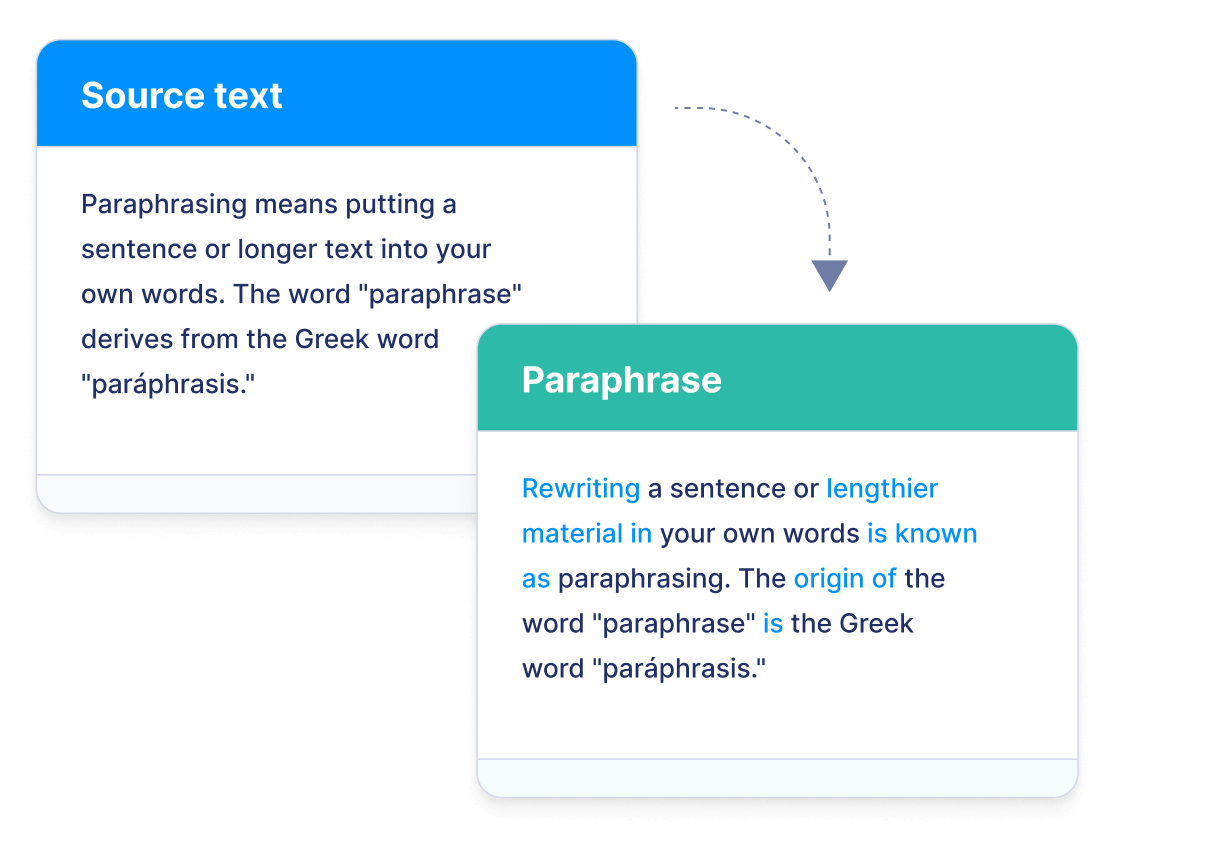
What's paraphrasing?
Paraphrasing involves expressing someone else’s ideas or thoughts in your own words while maintaining the original meaning. Paraphrasing tools can help you quickly reword text by replacing certain words with synonyms or restructuring sentences. They can also make your text more concise, clear, and suitable for a specific audience. Paraphrasing is an essential skill in academic writing and professional communication.
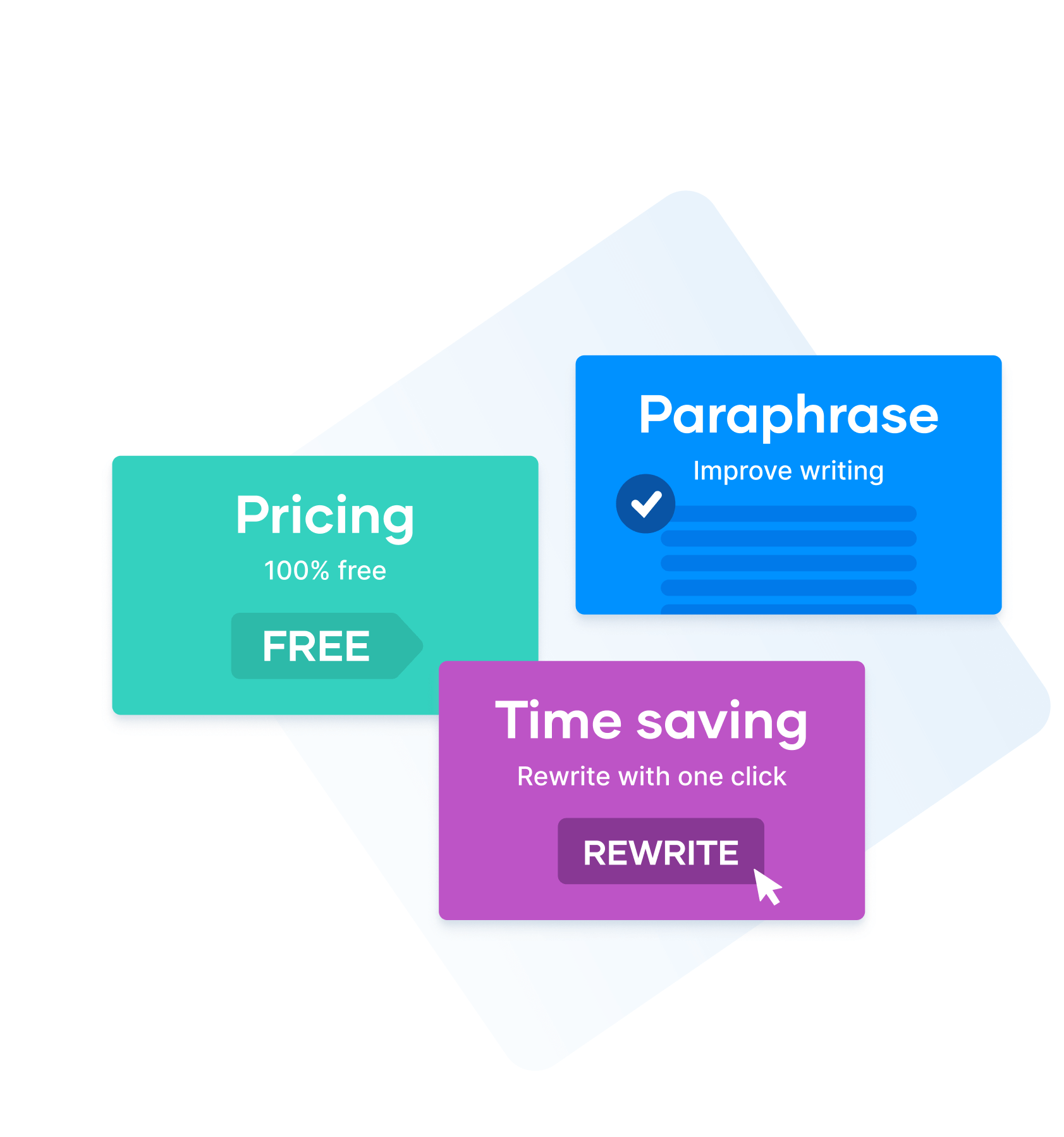
Why use this paraphrasing tool?
- Save time: Gone are the days when you had to reword sentences yourself; now you can rewrite a text or a complete text with one click.
- Improve your writing: Your writing will always be clear and easy to understand. Automatically ensure consistent language throughout.
- Preserve original meaning: Paraphrase without fear of losing the point of your text.
- No annoying ads: We care about the user experience, so we don’t run any ads.
- Accurate: Reliable and grammatically correct paraphrasing.
- No sign-up required: We don’t need your data for you to use our paraphrasing tool.
- Super simple to use: A simple interface even your grandma could use.
- It’s 100% free: No hidden costs, just unlimited use of a free paraphrasing tool.
Features of the paraphrasing tool
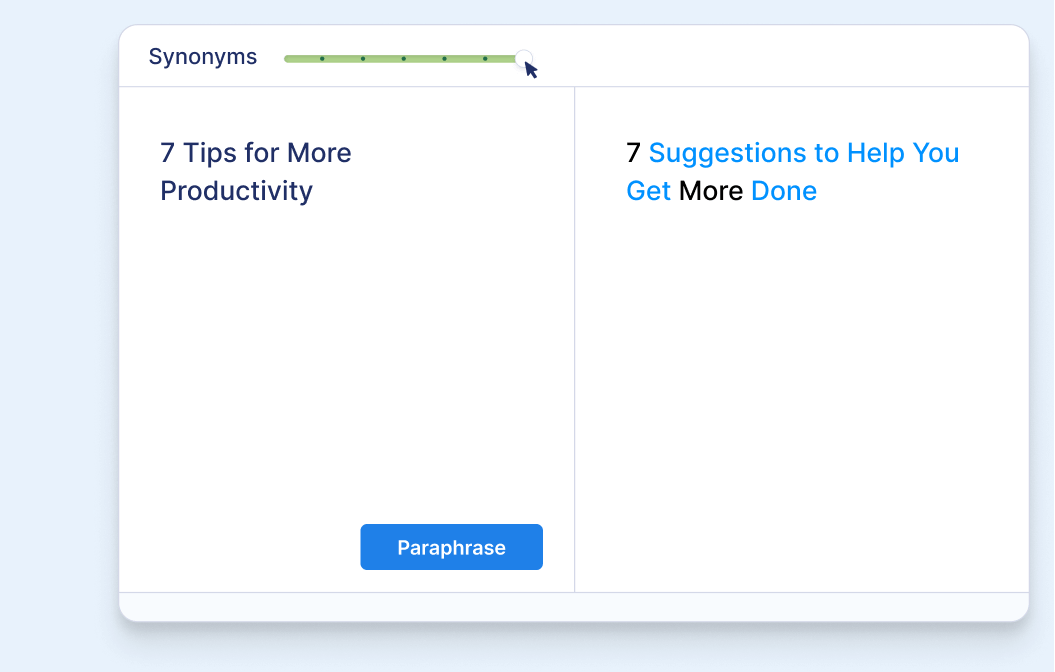
Rephrase individual sentences
With the Scribbr Paraphrasing Tool, you can easily reformulate individual sentences.
- Write varied headlines
- Rephrase the subject line of an email
- Create unique image captions
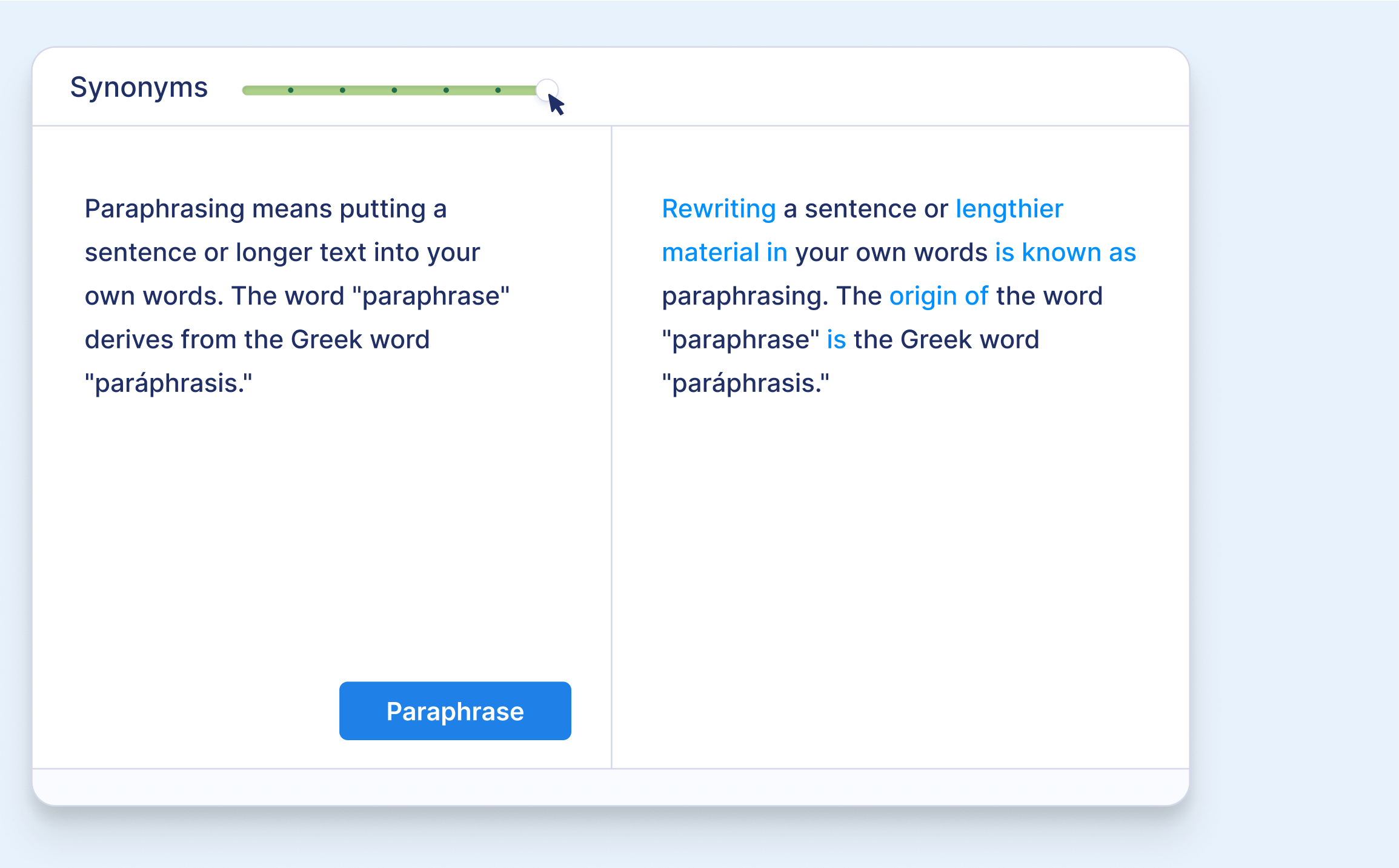
Paraphrase a whole text
Our paraphraser can also help with longer passages (up to 125 words per input). Upload your document or copy your text into the input field.
With one click, you can reformulate the entire text.
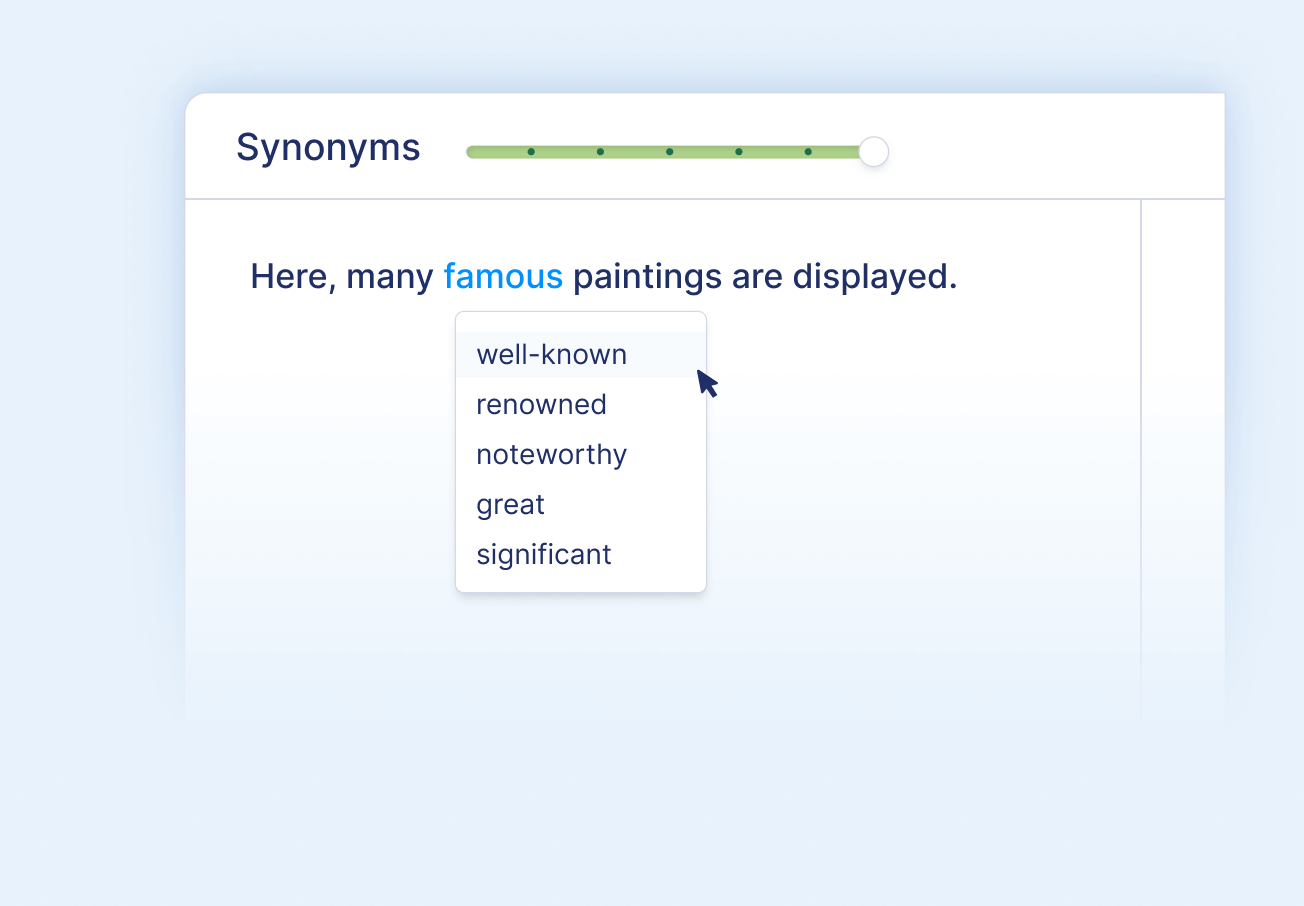
Find synonyms with ease
Simply click on any word to open the interactive thesaurus.
- Choose from a list of suggested synonyms
- Find the synonym with the most appropriate meaning
- Replace the word with a single click
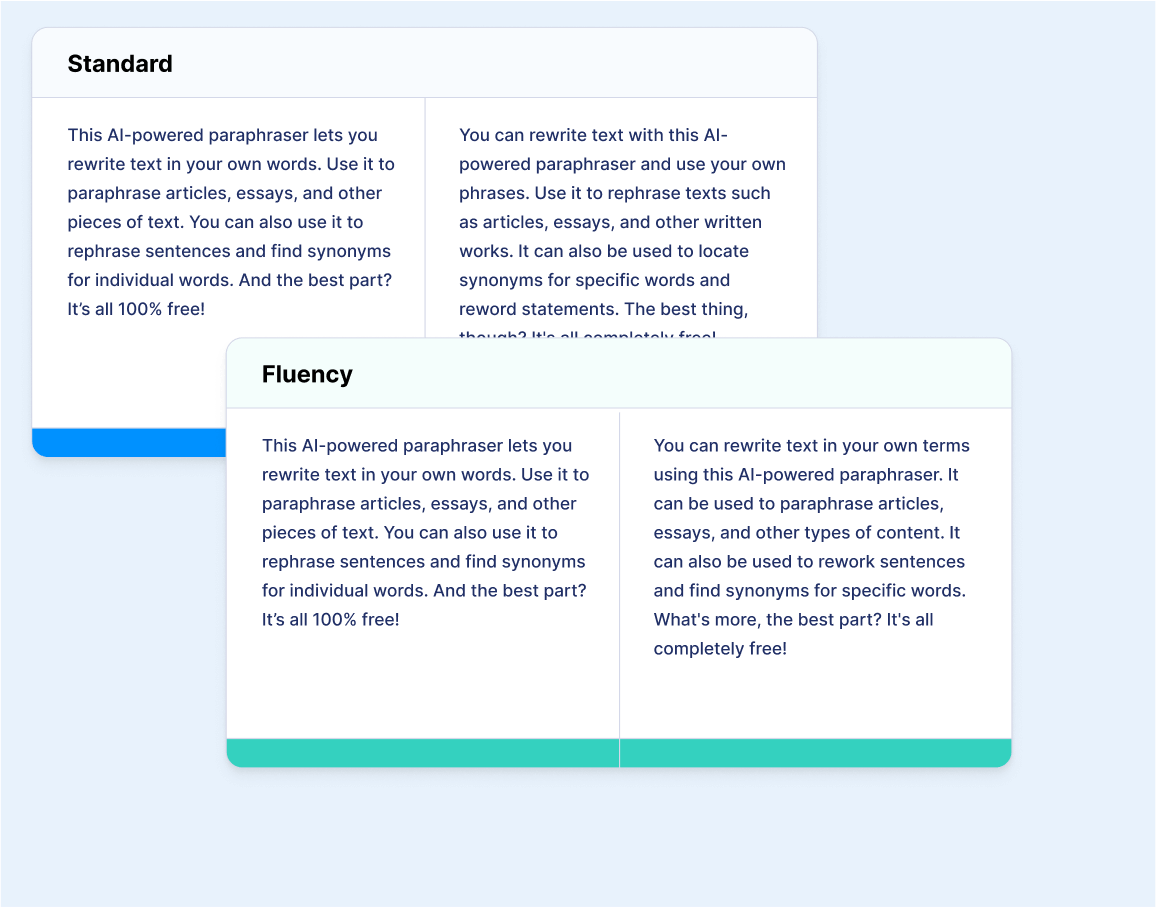
Paraphrase in two ways
- Standard: Offers a compromise between modifying and preserving the meaning of the original text
- Fluency: Improves language and corrects grammatical mistakes.
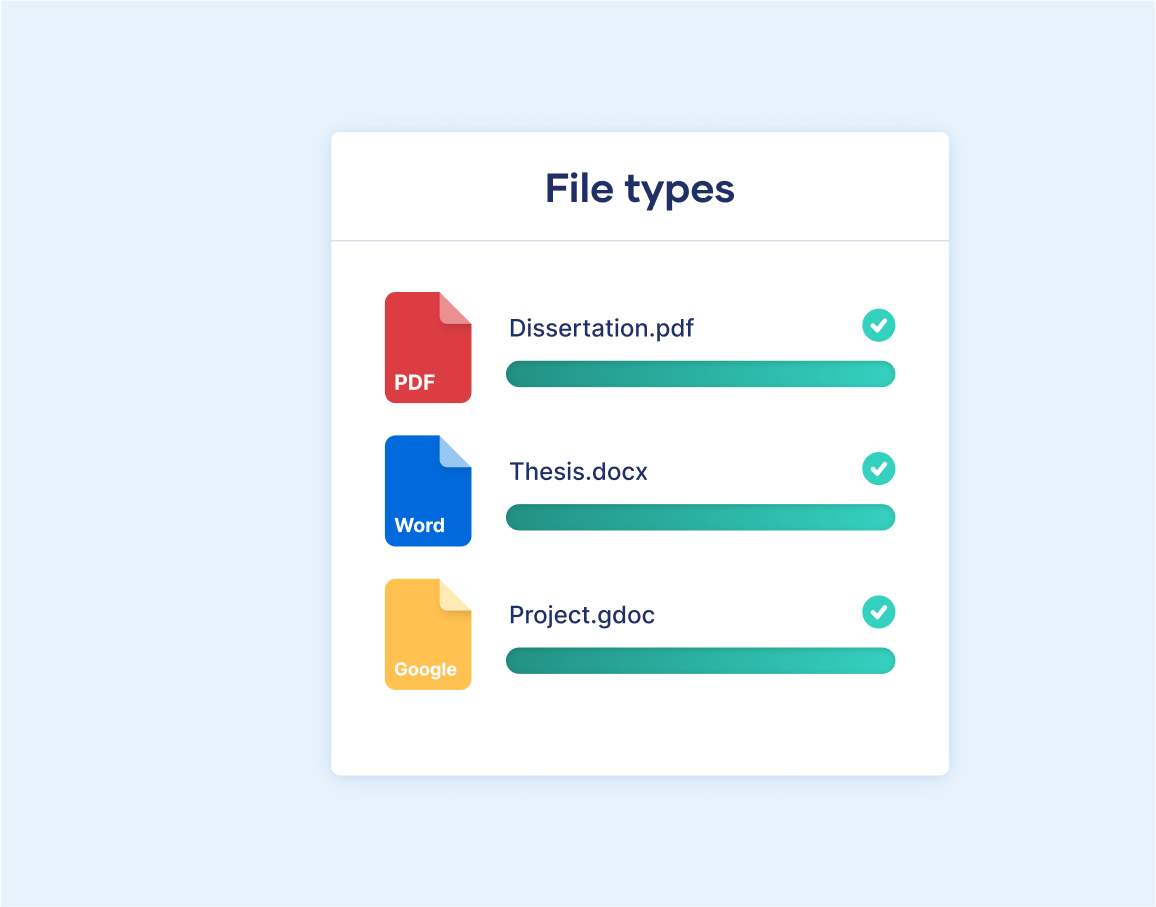
Upload different types of documents
Upload any Microsoft Word document, Google Doc, or PDF into the paraphrasing tool.
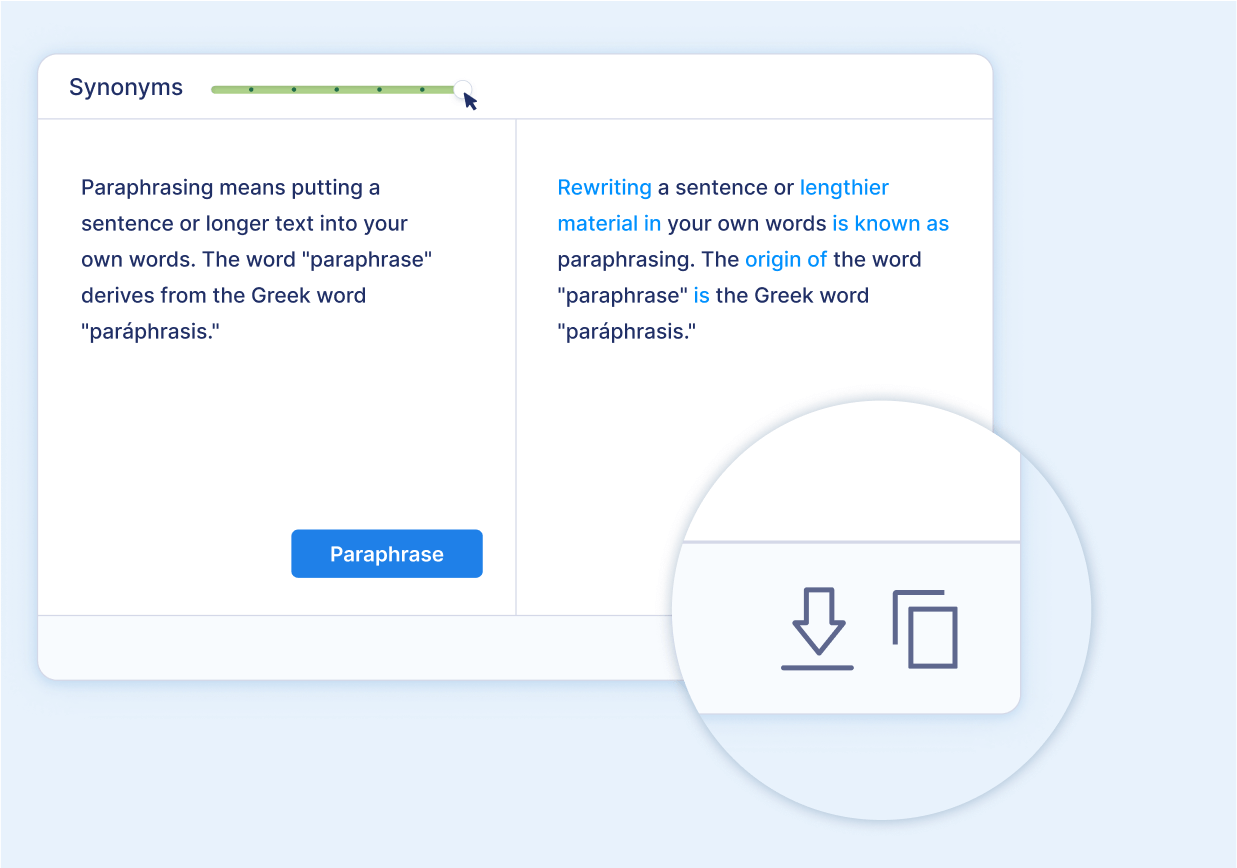
Download or copy your results
After you’re done, you can easily download or copy your text to use somewhere else.
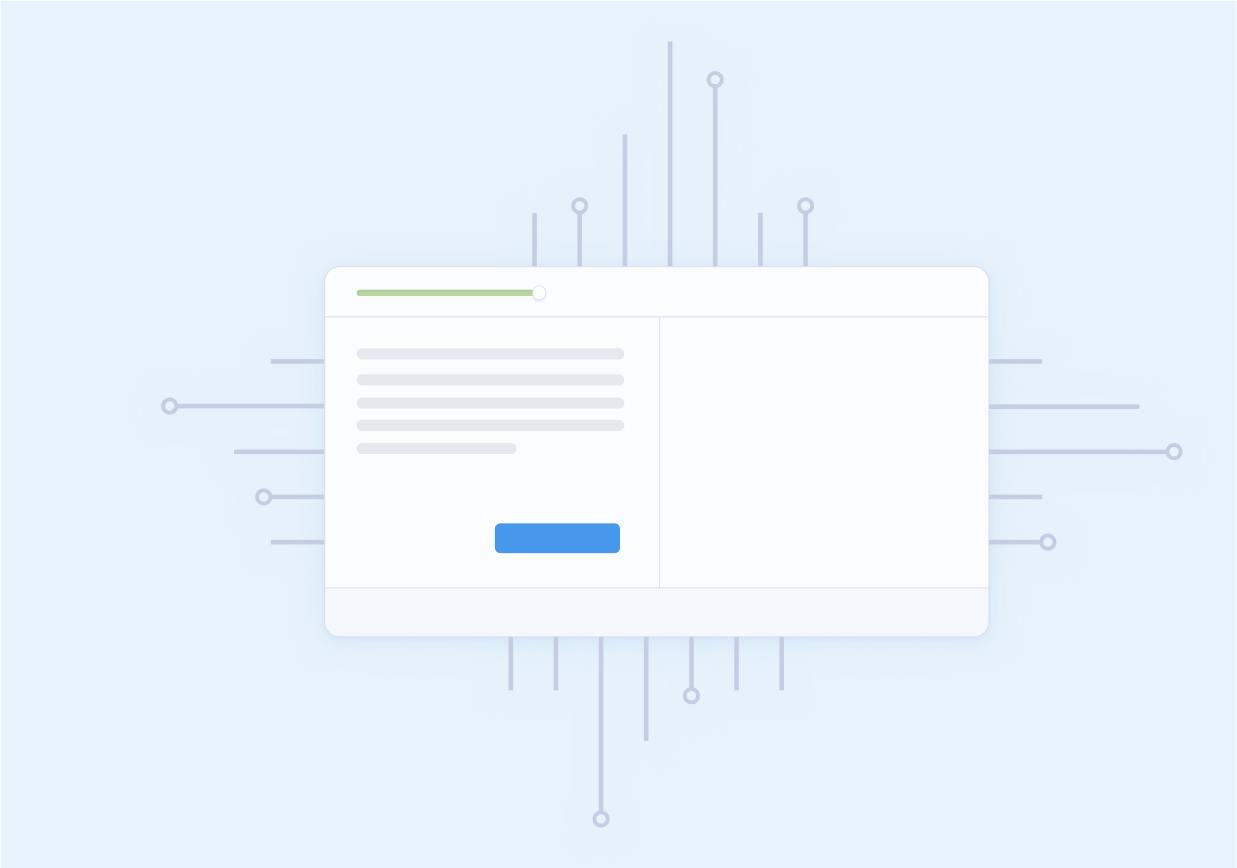
Powered by AI
The paraphrasing tool uses natural language processing to rewrite any text you give it. This way, you can paraphrase any text within seconds.
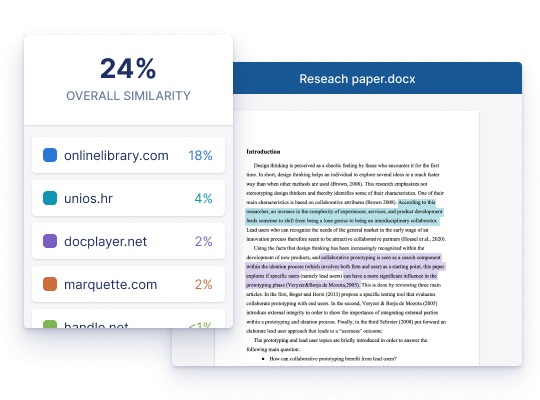
Avoid accidental plagiarism
Want to make sure your document is plagiarism-free? In addition to our paraphrasing tool, which will help you rephrase sentences, quotations, or paragraphs correctly, you can also use our anti-plagiarism software to make sure your document is unique and not plagiarized.
Scribbr’s anti-plagiarism software enables you to:
- Detect plagiarism more accurately than other tools
- Ensure that your paraphrased text is valid
- Highlight the sources that are most similar to your text
Start for free
How does this paraphrasing tool work?
1. put your text into the paraphraser, 2. select your method of paraphrasing, 3. select the quantity of synonyms you want, 4. edit your text where needed, who can use this paraphrasing tool.
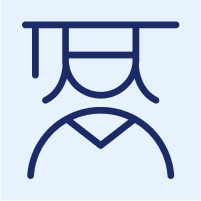
Paraphrasing tools can help students to understand texts and improve the quality of their writing.
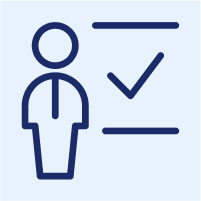
Create original lesson plans, presentations, or other educational materials.
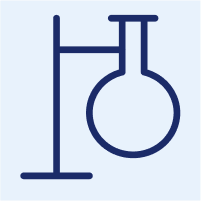
Researchers
Explain complex concepts or ideas to a wider audience.
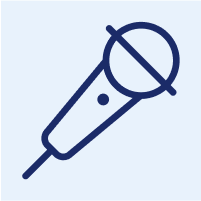
Journalists
Quickly and easily rephrase text to avoid repetitive language.
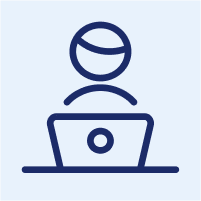
Copywriters
By using a paraphrasing tool, you can quickly and easily rework existing content to create something new and unique.
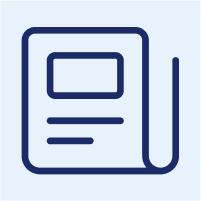
Bloggers can rewrite existing content to make it their own.
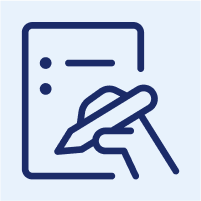
Writers who need to rewrite content, such as adapting an article for a different context or writing content for a different audience.
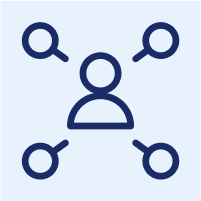
A paraphrasing tool lets you quickly rewrite your original content for each medium, ensuring you reach the right audience on each platform.
The all-purpose paraphrasing tool
The Scribbr Paraphrasing Tool is the perfect assistant in a variety of contexts.
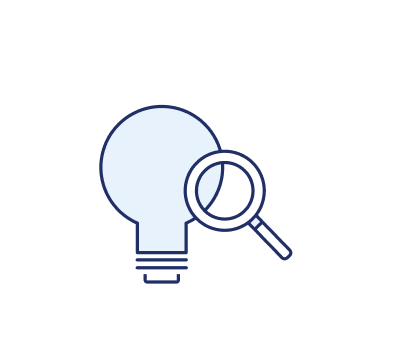
Brainstorming
Writer’s block? Use our paraphraser to get some inspiration.
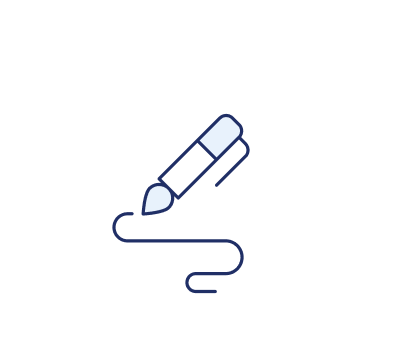
Professional communication
Produce creative headings for your blog posts or PowerPoint slides.
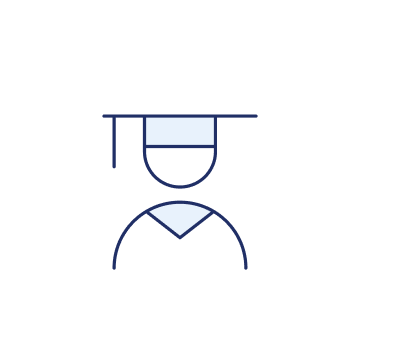
Academic writing
Paraphrase sources smoothly in your thesis or research paper.
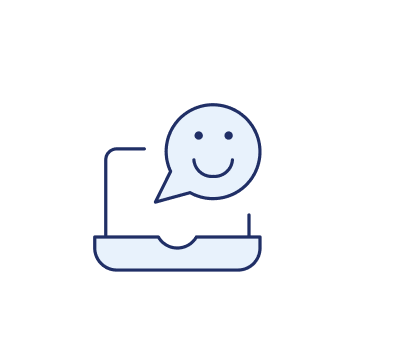
Social media
Craft memorable captions and content for your social media posts.
Paraphrase text online, for free
The Scribbr Paraphrasing Tool lets you rewrite as many sentences as you want—for free.
Write with 100% confidence 👉
Ask our team.
Want to contact us directly? No problem. We are always here for you.
- Email [email protected]
- Start live chat
- Call +1 (510) 822-8066
- WhatsApp +31 20 261 6040
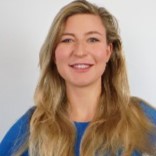
Frequently asked questions
The act of putting someone else’s ideas or words into your own words is called paraphrasing, rephrasing, or rewording. Even though they are often used interchangeably, the terms can mean slightly different things:
Paraphrasing is restating someone else’s ideas or words in your own words while retaining their meaning. Paraphrasing changes sentence structure, word choice, and sentence length to convey the same meaning.
Rephrasing may involve more substantial changes to the original text, including changing the order of sentences or the overall structure of the text.
Rewording is changing individual words in a text without changing its meaning or structure, often using synonyms.
It can. One of the two methods of paraphrasing is called “Fluency.” This will improve the language and fix grammatical errors in the text you’re paraphrasing.
Paraphrasing and using a paraphrasing tool aren’t cheating. It’s a great tool for saving time and coming up with new ways to express yourself in writing. However, always be sure to credit your sources. Avoid plagiarism.
If you don’t properly cite text paraphrased from another source, you’re plagiarizing. If you use someone else’s text and paraphrase it, you need to credit the original source. You can do that by using citations. There are different styles, like APA, MLA, Harvard, and Chicago. Find more information about citing sources here.
Paraphrasing without crediting the original author is a form of plagiarism , because you’re presenting someone else’s ideas as if they were your own.
However, paraphrasing is not plagiarism if you correctly cite the source . This means including an in-text citation and a full reference, formatted according to your required citation style .
As well as citing, make sure that any paraphrased text is completely rewritten in your own words.
Plagiarism means using someone else’s words or ideas and passing them off as your own. Paraphrasing means putting someone else’s ideas in your own words.
So when does paraphrasing count as plagiarism?
- Paraphrasing is plagiarism if you don’t properly credit the original author.
- Paraphrasing is plagiarism if your text is too close to the original wording (even if you cite the source). If you directly copy a sentence or phrase, you should quote it instead.
- Paraphrasing is not plagiarism if you put the author’s ideas completely in your own words and properly cite the source .
Try our services
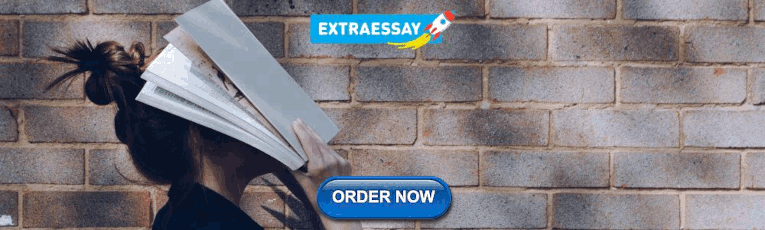
IMAGES
VIDEO
COMMENTS
What most people haven't considered is how technologies affect our language and how these changes are affecting the way we speak and even the way we think. One of the key ways we see this is ...
The English language is no different - but why has it changed over the decades? Some of the main influences on the evolution of languages include: the movement of people across countries and continents, for example, migration and, in previous centuries, colonization. For example, English speakers today would probably be comfortable using the ...
Conclusion. The power of language is undeniable. It serves as the foundation of human communication, influencing our understanding of the world, our interactions with one another, and our engagement with social and political systems. Language is both a reflection of existing power structures and a tool for challenging them.
Beard (2004) as cited in Mantiri [15] explains how political, social, cultural and technological factors affect the language change. The author explains that political factor is caused by foreign ...
Yes, and so is every other human language ! Language is always changing, evolving, and adapting to the needs of its users. This isn't a bad thing; if English hadn't changed since, say, 1950, we wouldn't have words to refer to modems, fax machines, or cable TV. As long as the needs of language users continue to change, so will the language.
The conclusion is that language change in and of itself is neither good nor bad. It can sometimes have beneficial aspects, such as facilitating pronunciation or comprehension, and it can sometimes have detrimental consequences, sometimes creating a greater burden for comprehension and language learning. The mostly negative popular attitudes ...
This essay therefore begins by looking at standardization followed by language expectations and finally the judgment on language use. Standardization of language is the process through which a language has been codified, this process also include grammar and spelling development. It is also through standardization that writing of a language in ...
Language - Evolution, Acquisition, Structure: Every language has a history, and, as in the rest of human culture, changes are constantly taking place in the course of the learned transmission of a language from one generation to another. This is just part of the difference between human culture and animal behaviour. Languages change in all their aspects, in their pronunciation, word forms ...
An essay is a written composition that presents and supports a particular idea, argument, or point of view. It's a way to express your thoughts, share information, and persuade others to see things from your perspective. Essays come in various forms, such as argumentative, persuasive, expository, and descriptive, each serving a unique purpose.
Abstract. Languages emerge and change over time at the population level though interactions between individual speakers. It is, however, hard to directly observe how a single speaker's linguistic innovation precipitates a population-wide change in the language, and many theoretical proposals exist. We introduce a very general mathematical ...
Annotate assignment guidelines in your first language. Create a brainstorm or mind map in a language you feel comfortable working in. Write an outline in any language you know. Translate and talk through an example paper in English to generate ideas. Freewrite in any language and then talk about what you wrote.
The chronological approach (sometimes called the cause-and-effect approach) is probably the simplest way to structure an essay. It just means discussing events in the order in which they occurred, discussing how they are related (i.e. the cause and effect involved) as you go. A chronological approach can be useful when your essay is about a ...
Table of contents. Step 1: Prewriting. Step 2: Planning and outlining. Step 3: Writing a first draft. Step 4: Redrafting and revising. Step 5: Editing and proofreading. Other interesting articles. Frequently asked questions about the writing process.
3. Movement of people. Cultural word-swapping is fluid. As we noted in at the start of this article, movement of people is the most obvious driver of change to the English language. It was brought to this country through the migration of the Anglo-Saxons, altered through exposure to the Vikings, then the Normans.
The process essay, also known as the "how-to" essay, is commonly written for people or companies that need tutorials or a set of instructional steps. Whether it's building a robot or cooking a chocolate cake, process essays use a similar format for any variations. They follow a step-by-step style, with the initial step influencing the second ...
"Writing" is usually understood as the expression of thought. This book redefines "writing" as the thought process itself. Writing is not what you do with thought. Writing is thinking. Better living through interpretation: that's the promise of academic writing, which is a foundational course in most schools because it's a
Hook sentence. Background information. Thesis statement. Step 1: Begin with the basics. Describe the initial steps or preparations required. Explain any tools, materials, or ingredients needed. Provide safety precautions if necessary. Step 2: Break Down the Process. Divide the process into clear, sequential steps.
Paper 2: Language Diversity and Change (7702/2) As detailed in the specification (4.2), the aim of the area of study examined in this paper is to allow students to explore language diversity and change over time. Students will study the key concepts of audience, purpose, genre and mode and will explore language in its wider social, geographical ...
For example, you may write in your process paper: "Making sushi takes 30 minutes of preparation time and 10 minutes of cooking.". The next action is to write a thesis statement. It is one sentence that describes the research problem to be considered in the paper. It can be the last sentence of the introduction.
Start by Brainstorming. The first step in writing your how-to essay is brainstorming. Here are tips to help you: Draw a line down the middle of a sheet of paper to make two columns. Label one column "materials" and the other column "steps." Write down every item and every step you can think of that will be needed to carry out your task.
Save time: Gone are the days when you had to reword sentences yourself; now you can rewrite a text or a complete text with one click. Improve your writing: Your writing will always be clear and easy to understand. Automatically ensure consistent language throughout. Preserve original meaning: Paraphrase without fear of losing the point of your text.
For example, it's easy to create a sizzling sound. Study with Quizlet and memorize flashcards containing terms like Decide if each phrase is an example of standard English or slang. Sort the tiles into the correct categories., Prompt: Write an informative essay explaining what has caused the English spoken today to be different from the English ...