
An official website of the United States government
The .gov means it’s official. Federal government websites often end in .gov or .mil. Before sharing sensitive information, make sure you’re on a federal government site.
The site is secure. The https:// ensures that you are connecting to the official website and that any information you provide is encrypted and transmitted securely.
- Publications
- Account settings
Preview improvements coming to the PMC website in October 2024. Learn More or Try it out now .
- Advanced Search
- Journal List
- Am J Pharm Educ
- v.73(1); 2009 Feb 19
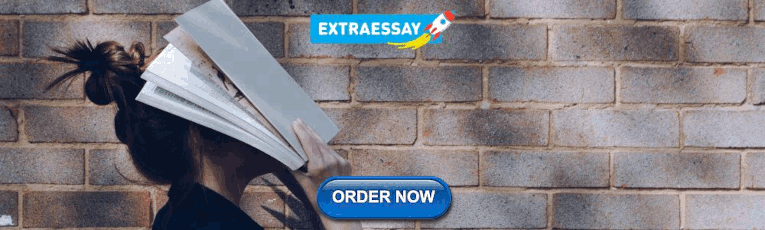
Learning Styles: A Review of Theory, Application, and Best Practices
Much pedagogical research has focused on the concept of “learning styles.” Several authors have proposed that the ability to typify student learning styles can augment the educational experience. As such, instructors might tailor their teaching style so that it is more congruent with a given student's or class of students' learning style. Others have argued that a learning/teaching style mismatch encourages and challenges students to expand their academic capabilities. Best practice might involve offering courses that employ a variety of teaching styles. Several scales are available for the standardization of learning styles. These scales employ a variety of learning style descriptors and are sometimes criticized as being measures of personality rather than learning style. Learning styles may become an increasingly relevant pedagogic concept as classes increase in size and diversity. This review will describe various learning style instruments as well as their potential use and limitations. Also discussed is the use of learning style theory in various concentrations including pharmacy.
INTRODUCTION
The diversity of students engaged in higher education continues to expand. Students come to colleges with varied ethnic and cultural backgrounds, from a multitude of training programs and institutions, and with differing learning styles. 1 Coupled with this increase in diversification has been a growth in distance education programs and expansions in the types of instructional media used to deliver information. 2 , 3 These changes and advances in technology have led many educators to reconsider traditional, uniform instruction methods and stress the importance of considering student learning styles in the design and delivery of course content. 4 - 5 Mismatches between an instructor's style of teaching and a student's method of learning have been cited as potential learning obstacles within the classroom and as a reason for using a variety of teaching modalities to deliver instruction. 6 - 8 The concept of using a menu of teaching modalities is based on the premise that at least some content will be presented in a manner suited to every type of learner within a given classroom or course. Some research has focused on profiling learning types so that instructors have a better understanding of the cohort of students they are educating. 7 - 8 This information can be used to guide the selection of instruction modalities employed in the classroom. Limited research has also focused on describing and characterizing composite learning styles and patterns for students in various concentrations of study (eg, medicine, engineering). 5 , 6 , 9 This review will describe the potential utility and limitations in assessing learning styles.
LEARNING STYLES
A benchmark definition of “learning styles” is “characteristic cognitive, effective, and psychosocial behaviors that serve as relatively stable indicators of how learners perceive, interact with, and respond to the learning environment. 10 Learning styles are considered by many to be one factor of success in higher education. Confounding research and, in many instances, application of learning style theory has begat the myriad of methods used to categorize learning styles. No single commonly accepted method currently exists, but alternatively several potential scales and classifications are in use. Most of these scales and classifications are more similar than dissimilar and focus on environmental preferences, sensory modalities, personality types, and/or cognitive styles. 11 Lack of a conceptual framework for both learning style theory and measurement is a common and central criticism in this area. In 2004 the United Kingdom Learning and Skills Research Center commissioned a report intended to systematically examine existing learning style models and instruments. In the commission report, Coffield et al identified several inconsistencies in learning style models and instruments and cautioned educators with regards to their use. 12 The authors also outlined a suggested research agenda for this area.
Alternatively, many researchers have argued that knowledge of learning styles can be of use to both educators and students. Faculty members with knowledge of learning styles can tailor pedagogy so that it best coincides with learning styles exhibited by the majority of students. 4 Alternatively, students with knowledge of their own preferences are empowered to use various techniques to enhance learning, which in turn may impact overall educational satisfaction. This ability is particularly critical and useful when an instructor's teaching style does not match a student's learning style. Compounding the issue of learning styles in the classroom has been the movement in many collegiate environments to distance and/or asynchronous education. 2 , 3 This shift in educational modality is inconsistent with the learning models with which most older students and adult learners are accustomed from their primary and high school education. 3 , 13 , 14 Alternatively, environmental influences and more widespread availability of technological advances (eg, personal digital assistants, digital video, the World Wide Web, wireless Internet) may make younger generations of students more comfortable with distance learning. 15 - 17
LEARNING STYLES INSTRUMENTS
As previously stated, several models and measures of learning styles have been described in the literature. Kolb proposed a model involving a 4-stage cyclic structure that begins with a concrete experience, which lends to a reflective observation and subsequently an abstract conceptualization that allows for active experimentation. 18 Kolb's model is associated with the Learning Style Inventory instrument (LSI). The LSI focuses on learner's preferences in terms of concrete versus abstract, and action versus reflection. Learners are subsequently described as divergers, convergers, assimilators, or accommodators.
Honey and Mumford developed an alternative instrument known as the Learning Style Questionnaire (LSQ). 6 Presumably, the LSQ has improved validity and predictive accuracy compared to the LSI. The LSQ describes 4 distinct types of learners: activists (learn primarily by experience), reflectors (learn from reflective observation), theorists (learn from exploring associations and interrelationships), and pragmatics (learn from doing or trying things with practical outcomes). The LSQ has been more widely used and studied in management and business settings and its applicability to academia has been questioned. 6 An alternative to the LSQ, the Canfield Learning Style Inventory (CLSI) describes learning styles along 4 dimensions. 19 These dimensions include conditions for learning, area of interest, mode of learning, and conditions for performance. Analogous to the LSQ, applicability of the CLSI to academic settings has been questioned. Additionally, some confusion surrounding scoring and interpretation of certain result values also exists.
Felder and Silverman introduced a learning style assessment instrument that was specifically designed for classroom use and was first applied in the context of engineering education. 20 The instrument consists of 44 short items with a choice between 2 responses to each sentence. Learners are categorized in 4 dichotomous areas: preference in terms of type and mode of information perception (sensory or intuitive; visual or verbal), approaches to organizing and processing information (active or reflective), and the rate at which students progress towards understanding (sequential or global). The instrument associated with the model is known as the Index of Learning Survey (ILS). 21 The ILS is based on a 44-item questionnaire and outputs a preference profile for a student or an entire class. The preference profile is based on the 4 previously defined learning dimensions. The ILS has several advantages over other instruments including conciseness and ease of administration (in both a written and computerized format). 20 , 21 No published data exist with regards to the use of the ILS in populations of pharmacy students or pharmacists. Cook described a study designed to examine the reliability of the ILS for determining learning styles among a population of internal medicine residents. 20 The researchers administered the ILS twice and the Learning Style Type Indicator (LSTI) once to 138 residents (86 men, 52 women). The LSTI has been previously compared to the ILS by several investigators. 8 , 19 Cook found that the Cronbach's alpha scores for the ILS and LSTI ranged from 0.19 to 0.69. They preliminarily concluded that the ILS scores were reliable and valid among this cohort of residents, particularly within the active-reflective and sensing-intuitive domains. In a separate study, Cook et al attempted to evaluate convergence and discrimination among the ILS, LSI, and another computer-based instrument known as the Cognitive Styles Analysis (CSA). 11 The cohort studied consisted of family medicine and internal medicine residents as well as first- and third-year medical students. Eighty-nine participants completed all 3 instruments, and responses were analyzed using calculated Pearson's r and Cronbach's α. The authors found that the ILS active-reflective and sensing-intuitive scores as well as the LSI active-reflective scores were valid in determining learning styles. However, the ILS sequential-global domain failed to correlate well with other instruments and may be flawed, at least in this given population. The authors advised the use of caution when interpreting scores without a strong knowledge of construct definitions and empirical evidence.
Several other instruments designed to measure personality indexes or psychological types may overlap and describe learning styles in nonspecific fashions. One example of such an indicator is the Myers-Briggs Index. 6 While some relation between personality indexes and learning styles may exist, the use of instruments intended to describe personality to characterize learning style has been criticized by several authors. Therefore, the use of these markers to measure learning styles is not recommended. 6 The concept of emotional intelligence is another popular way to characterize intellect and learning capacity but similarly should not be misconstrued as an effective means of describing learning styles. 23
Several authors have proposed correlations between culture and learning styles. 6 , 24 This is predicated on the concept that culture influences environmental perceptions which, in turn, to some degree determine the way in which information is processed and organized. The storage, processing, and assimilation methods for information contribute to how new knowledge is learned. Culture also plays a role in conditioning and reinforcing learning styles and partially explains why teaching methods used in certain parts of the world may be ineffective or less effective when blindly transplanted to another locale. 6 , 24 Teachers should be aware of this phenomenon and the influence it has on the variety of learning styles that are present in classrooms. This is especially true in classrooms that have a large contingency of international students. Such classrooms are becoming increasingly common as more and more schools expand their internationalization efforts. 25
The technological age may also be influencing the learning styles of younger students and emerging generations of learners. The Millennial Generation has been described as more technologically advanced than their Generation X counterparts, with higher expectations for the use of computer-aided media in the classroom. 15 , 16 , 26 Younger students are accustomed to enhanced visual images associated with various computer- and television-based games and game systems. 16 , 26 Additionally, video technology is increasingly becoming “transportable” in the way of mobile computing, MP3 devices, personal digital video players, and other technologies. 26 All of these advances have made visual images more pervasive and common within industrialized nations.
APPLYING LEARNING STYLES TO THE CLASSROOM
As class sizes increase, so do the types and numbers of student learning styles. Also, as previously mentioned, internationalization and changes in the media culture may affect the spectrum of classroom learning styles as well. 24 , 25 Given the variability in learning styles that may exist in a classroom, some authors suggested that students should adapt their learning styles to coincide with a given instruction style. 6 , 27 This allows instructors to dictate the methods used to instruct in the classroom. This approach also allows instructors to “teach from their strengths,” with little consideration to other external factors such as learning style of students. While convenient, this unilateral approach has been criticized for placing all of the responsibility for aligning teaching and learning on the student. When the majority of information is presented in formats that are misaligned with learning styles, students may spend more time manipulating material than they do in comprehending and applying the information. Additionally, a unilaterally designed classroom may reinforce a “do nothing” approach among faculty members. 6 Alternatively, a teaching style-learning style mismatch might challenge students to adjust, grow intellectually, and learn in more integrated ways. However, it may be difficult to predict which students have the baseline capacity to adjust, particularly when significant gaps in knowledge of a given subject already exist or when the learner is a novice to the topic being instructed. 6 , 27 This might be especially challenging within professional curricula where course load expectations are significant.
Best practice most likely involves a teaching paradigm which addresses and accommodates multiple dimensions of learning styles that build self-efficacy. 27 Instructing in a way that encompasses multiple learning styles gives the teacher an opportunity to reach a greater extent of a given class, while also challenging students to expand their range of learning styles and aptitudes at a slower pace. This may avoid lost learning opportunities and circumvent unnecessary frustration from both the teacher and student. For many instructors, multi-style teaching is their inherent approach to learning, while other instructors more commonly employ unilateral styles. Learning might be better facilitated if instructors were cognizant of both their teaching styles and the learning styles of their students. An understanding and appreciation of a given individual's teaching style requires self-reflection and introspection and should be a component of a well-maintained teaching portfolio. Major changes or modifications to teaching styles might not be necessary in order to effectively create a classroom atmosphere that addresses multiple learning styles or targets individual ones. However, faculty members should be cautious to not over ambitiously, arbitrarily, or frivolously design courses and activities with an array of teaching modalities that are not carefully connected, orchestrated, and delivered.
Novice learners will likely be more successful when classrooms, either by design or by chance, are tailored to their learning style. However, the ultimate goal is to instill within students the skills to recognize and react to various styles so that learning is maximized no matter what the environment. 28 This is an essential skill for an independent learner and for students in any career path.
Particular consideration of learning styles might be given to asynchronous courses and other courses where a significant portion of time is spent online. 29 As technology advances and classroom sizes in many institutions become increasingly large, asynchronous instruction is becoming more pervasive. In many instances, students who have grown accustomed to technological advances may prefer asynchronous courses. Online platforms may inherently affect learning on a single dimension (visual or auditory). Most researchers who have compared the learning styles of students enrolled in online versus traditional courses have found no correlations between the learning styles and learning outcomes of cohorts enrolled in either course type. Johnson et al compared learning style profiles to student satisfaction with either online or face-to-face study groups. 30 Forty-eight college students participated in the analysis. Learning styles were measured using the ILS. Students were surveyed with regard to their satisfaction with various study group formats. These results were then correlated to actual performance on course examinations. Active and visual learners demonstrated a significant preference for face-to-face study groups. Alternatively, students who were reflective learners demonstrated a preference for online groups. Likely due to the small sample size, none of these differences achieved statistical significance. The authors suggest that these results are evidence for courses employing hybrid teaching styles that reach as many different students as possible. Cook et al studied 121 internal medicine residents and also found no association (p > 0.05) between ILS-measured learning styles and preferences for learning formats (eg, Web-based versus paper-based learning modules). 31 Scores on assessment questions related to learning modules administered to the residents were also not statistically correlated with learning styles.
Cook et al examined the effectiveness of adapting Web-based learning modules to a given learner's style. 32 The investigators created 2 versions of a Web-based instructional module on complementary and alternative medications. One version of the modules directed the learner to “active” questions that provided learners immediate and comprehensive feedback, while the other version involved “reflective” questions that directed learners back to the case content for answers. Eighty-nine residents were randomly matched or mismatched based on their active-reflective learning styles (as determined by ILS) to either the “active” or “reflective” test version. Posttest scores for either question type among mismatched subjects did not differ significantly ( p = 0.97), suggesting no interaction between learning styles and question types. The authors concluded from this small study that learning styles had no influence on learning outcomes. The study was limited in its lack of assessment of baseline knowledge, motivation, or other characteristics. Also, the difficulty of the assessment may not have been sufficient enough to distinguish a difference and/or “mismatched” learners may have automatically adapted to the information they received regardless of type.
STUDIES OF PHARMACY STUDENTS
There are no published studies that have systematically examined the learning styles of pharmacy students. Pungente et al collected some learning styles data as part of a study designed to evaluate how first-year pharmacy students' learning styles influenced preferences toward different activities associated with problem-based learning (PBL). 33 One hundred sixteen first-year students completed Kolb's LSI. Learning styles were then matched to responses from a survey designed to assess student preferences towards various aspects of PBL. The majority of students were classified by the LSI as being accommodators (36.2%), with a fairly even distribution of styles among remaining students (19.8% assimilators, 22.4% convergers, 21.6% divergers). There was a proportional distribution of learning styles among a convenience sample of pharmacy students. Divergers were the least satisfied with the PBL method of instruction, while convergers demonstrated the strongest preference for this method of learning. The investigators proposed that the next step might be to correlate learning styles and PBL preferences with actual academic success.
Limited research correlating learning styles to learning outcomes has hampered the application of learning style theory to actual classroom settings. Complicating research is the plethora of different learning style measurement instruments available. Despite these obstacles, efforts to better define and utilize learning style theory is an area of growing research. A better knowledge and understanding of learning styles may become increasingly critical as classroom sizes increase and as technological advances continue to mold the types of students entering higher education. While research in this area continues to grow, faculty members should make concentrated efforts to teach in a multi-style fashion that both reaches the greatest extent of students in a given class and challenges all students to grow as learners.
2.2 Approaches to Research
Learning objectives.
- Describe the different research methods used by psychologists
- Discuss the strengths and weaknesses of case studies, naturalistic observation, surveys, and archival research
- Compare longitudinal and cross-sectional approaches to research
There are many research methods available to psychologists in their efforts to understand, describe, and explain behavior and the cognitive and biological processes that underlie it. Some methods rely on observational techniques. Other approaches involve interactions between the researcher and the individuals who are being studied—ranging from a series of simple questions to extensive, in-depth interviews—to well-controlled experiments.
Each of these research methods has unique strengths and weaknesses, and each method may only be appropriate for certain types of research questions. For example, studies that rely primarily on observation produce incredible amounts of information, but the ability to apply this information to the larger population is somewhat limited because of small sample sizes. Survey research, on the other hand, allows researchers to easily collect data from relatively large samples. While this allows for results to be generalized to the larger population more easily, the information that can be collected on any given survey is somewhat limited and subject to problems associated with any type of self-reported data. Some researchers conduct archival research by using existing records. While this can be a fairly inexpensive way to collect data that can provide insight into a number of research questions, researchers using this approach have no control on how or what kind of data was collected. All of the methods described thus far are correlational in nature. This means that researchers can speak to important relationships that might exist between two or more variables of interest. However, correlational data cannot be used to make claims about cause-and-effect relationships.
Correlational research can find a relationship between two variables, but the only way a researcher can claim that the relationship between the variables is cause and effect is to perform an experiment. In experimental research, which will be discussed later in this chapter, there is a tremendous amount of control over variables of interest. While this is a powerful approach, experiments are often conducted in very artificial settings. This calls into question the validity of experimental findings with regard to how they would apply in real-world settings. In addition, many of the questions that psychologists would like to answer cannot be pursued through experimental research because of ethical concerns.
Clinical or Case Studies
In 2011, the New York Times published a feature story on Krista and Tatiana Hogan, Canadian twin girls. These particular twins are unique because Krista and Tatiana are conjoined twins, connected at the head. There is evidence that the two girls are connected in a part of the brain called the thalamus, which is a major sensory relay center. Most incoming sensory information is sent through the thalamus before reaching higher regions of the cerebral cortex for processing.
Link to Learning
To learn more about Krista and Tatiana, watch this New York Times video about their lives.
The implications of this potential connection mean that it might be possible for one twin to experience the sensations of the other twin. For instance, if Krista is watching a particularly funny television program, Tatiana might smile or laugh even if she is not watching the program. This particular possibility has piqued the interest of many neuroscientists who seek to understand how the brain uses sensory information.
These twins represent an enormous resource in the study of the brain, and since their condition is very rare, it is likely that as long as their family agrees, scientists will follow these girls very closely throughout their lives to gain as much information as possible (Dominus, 2011).
In observational research, scientists are conducting a clinical or case study when they focus on one person or just a few individuals. Indeed, some scientists spend their entire careers studying just 10–20 individuals. Why would they do this? Obviously, when they focus their attention on a very small number of people, they can gain a tremendous amount of insight into those cases. The richness of information that is collected in clinical or case studies is unmatched by any other single research method. This allows the researcher to have a very deep understanding of the individuals and the particular phenomenon being studied.
If clinical or case studies provide so much information, why are they not more frequent among researchers? As it turns out, the major benefit of this particular approach is also a weakness. As mentioned earlier, this approach is often used when studying individuals who are interesting to researchers because they have a rare characteristic. Therefore, the individuals who serve as the focus of case studies are not like most other people. If scientists ultimately want to explain all behavior, focusing attention on such a special group of people can make it difficult to generalize any observations to the larger population as a whole. Generalizing refers to the ability to apply the findings of a particular research project to larger segments of society. Again, case studies provide enormous amounts of information, but since the cases are so specific, the potential to apply what’s learned to the average person may be very limited.
Naturalistic Observation
If you want to understand how behavior occurs, one of the best ways to gain information is to simply observe the behavior in its natural context. However, people might change their behavior in unexpected ways if they know they are being observed. How do researchers obtain accurate information when people tend to hide their natural behavior? As an example, imagine that your professor asks everyone in your class to raise their hand if they always wash their hands after using the restroom. Chances are that almost everyone in the classroom will raise their hand, but do you think hand washing after every trip to the restroom is really that universal?
This is very similar to the phenomenon mentioned earlier in this chapter: many individuals do not feel comfortable answering a question honestly. But if we are committed to finding out the facts about hand washing, we have other options available to us.
Suppose we send a classmate into the restroom to actually watch whether everyone washes their hands after using the restroom. Will our observer blend into the restroom environment by wearing a white lab coat, sitting with a clipboard, and staring at the sinks? We want our researcher to be inconspicuous—perhaps standing at one of the sinks pretending to put in contact lenses while secretly recording the relevant information. This type of observational study is called naturalistic observation : observing behavior in its natural setting. To better understand peer exclusion, Suzanne Fanger collaborated with colleagues at the University of Texas to observe the behavior of preschool children on a playground. How did the observers remain inconspicuous over the duration of the study? They equipped a few of the children with wireless microphones (which the children quickly forgot about) and observed while taking notes from a distance. Also, the children in that particular preschool (a “laboratory preschool”) were accustomed to having observers on the playground (Fanger, Frankel, & Hazen, 2012).
It is critical that the observer be as unobtrusive and as inconspicuous as possible: when people know they are being watched, they are less likely to behave naturally. If you have any doubt about this, ask yourself how your driving behavior might differ in two situations: In the first situation, you are driving down a deserted highway during the middle of the day; in the second situation, you are being followed by a police car down the same deserted highway ( Figure 2.7 ).
It should be pointed out that naturalistic observation is not limited to research involving humans. Indeed, some of the best-known examples of naturalistic observation involve researchers going into the field to observe various kinds of animals in their own environments. As with human studies, the researchers maintain their distance and avoid interfering with the animal subjects so as not to influence their natural behaviors. Scientists have used this technique to study social hierarchies and interactions among animals ranging from ground squirrels to gorillas. The information provided by these studies is invaluable in understanding how those animals organize socially and communicate with one another. The anthropologist Jane Goodall , for example, spent nearly five decades observing the behavior of chimpanzees in Africa ( Figure 2.8 ). As an illustration of the types of concerns that a researcher might encounter in naturalistic observation, some scientists criticized Goodall for giving the chimps names instead of referring to them by numbers—using names was thought to undermine the emotional detachment required for the objectivity of the study (McKie, 2010).
The greatest benefit of naturalistic observation is the validity , or accuracy, of information collected unobtrusively in a natural setting. Having individuals behave as they normally would in a given situation means that we have a higher degree of ecological validity, or realism, than we might achieve with other research approaches. Therefore, our ability to generalize the findings of the research to real-world situations is enhanced. If done correctly, we need not worry about people or animals modifying their behavior simply because they are being observed. Sometimes, people may assume that reality programs give us a glimpse into authentic human behavior. However, the principle of inconspicuous observation is violated as reality stars are followed by camera crews and are interviewed on camera for personal confessionals. Given that environment, we must doubt how natural and realistic their behaviors are.
The major downside of naturalistic observation is that they are often difficult to set up and control. In our restroom study, what if you stood in the restroom all day prepared to record people’s hand washing behavior and no one came in? Or, what if you have been closely observing a troop of gorillas for weeks only to find that they migrated to a new place while you were sleeping in your tent? The benefit of realistic data comes at a cost. As a researcher you have no control of when (or if) you have behavior to observe. In addition, this type of observational research often requires significant investments of time, money, and a good dose of luck.
Sometimes studies involve structured observation. In these cases, people are observed while engaging in set, specific tasks. An excellent example of structured observation comes from Strange Situation by Mary Ainsworth (you will read more about this in the chapter on lifespan development). The Strange Situation is a procedure used to evaluate attachment styles that exist between an infant and caregiver. In this scenario, caregivers bring their infants into a room filled with toys. The Strange Situation involves a number of phases, including a stranger coming into the room, the caregiver leaving the room, and the caregiver’s return to the room. The infant’s behavior is closely monitored at each phase, but it is the behavior of the infant upon being reunited with the caregiver that is most telling in terms of characterizing the infant’s attachment style with the caregiver.
Another potential problem in observational research is observer bias . Generally, people who act as observers are closely involved in the research project and may unconsciously skew their observations to fit their research goals or expectations. To protect against this type of bias, researchers should have clear criteria established for the types of behaviors recorded and how those behaviors should be classified. In addition, researchers often compare observations of the same event by multiple observers, in order to test inter-rater reliability : a measure of reliability that assesses the consistency of observations by different observers.
Often, psychologists develop surveys as a means of gathering data. Surveys are lists of questions to be answered by research participants, and can be delivered as paper-and-pencil questionnaires, administered electronically, or conducted verbally ( Figure 2.9 ). Generally, the survey itself can be completed in a short time, and the ease of administering a survey makes it easy to collect data from a large number of people.
Surveys allow researchers to gather data from larger samples than may be afforded by other research methods . A sample is a subset of individuals selected from a population , which is the overall group of individuals that the researchers are interested in. Researchers study the sample and seek to generalize their findings to the population.
There is both strength and weakness of the survey in comparison to case studies. By using surveys, we can collect information from a larger sample of people. A larger sample is better able to reflect the actual diversity of the population, thus allowing better generalizability. Therefore, if our sample is sufficiently large and diverse, we can assume that the data we collect from the survey can be generalized to the larger population with more certainty than the information collected through a case study. However, given the greater number of people involved, we are not able to collect the same depth of information on each person that would be collected in a case study.
Another potential weakness of surveys is something we touched on earlier in this chapter: People don't always give accurate responses. They may lie, misremember, or answer questions in a way that they think makes them look good. For example, people may report drinking less alcohol than is actually the case.
Any number of research questions can be answered through the use of surveys. One real-world example is the research conducted by Jenkins, Ruppel, Kizer, Yehl, and Griffin (2012) about the backlash against the US Arab-American community following the terrorist attacks of September 11, 2001. Jenkins and colleagues wanted to determine to what extent these negative attitudes toward Arab-Americans still existed nearly a decade after the attacks occurred. In one study, 140 research participants filled out a survey with 10 questions, including questions asking directly about the participant’s overt prejudicial attitudes toward people of various ethnicities. The survey also asked indirect questions about how likely the participant would be to interact with a person of a given ethnicity in a variety of settings (such as, “How likely do you think it is that you would introduce yourself to a person of Arab-American descent?”). The results of the research suggested that participants were unwilling to report prejudicial attitudes toward any ethnic group. However, there were significant differences between their pattern of responses to questions about social interaction with Arab-Americans compared to other ethnic groups: they indicated less willingness for social interaction with Arab-Americans compared to the other ethnic groups. This suggested that the participants harbored subtle forms of prejudice against Arab-Americans, despite their assertions that this was not the case (Jenkins et al., 2012).
Archival Research
Some researchers gain access to large amounts of data without interacting with a single research participant. Instead, they use existing records to answer various research questions. This type of research approach is known as archival research . Archival research relies on looking at past records or data sets to look for interesting patterns or relationships.
For example, a researcher might access the academic records of all individuals who enrolled in college within the past ten years and calculate how long it took them to complete their degrees, as well as course loads, grades, and extracurricular involvement. Archival research could provide important information about who is most likely to complete their education, and it could help identify important risk factors for struggling students ( Figure 2.10 ).
In comparing archival research to other research methods, there are several important distinctions. For one, the researcher employing archival research never directly interacts with research participants. Therefore, the investment of time and money to collect data is considerably less with archival research. Additionally, researchers have no control over what information was originally collected. Therefore, research questions have to be tailored so they can be answered within the structure of the existing data sets. There is also no guarantee of consistency between the records from one source to another, which might make comparing and contrasting different data sets problematic.
Longitudinal and Cross-Sectional Research
Sometimes we want to see how people change over time, as in studies of human development and lifespan. When we test the same group of individuals repeatedly over an extended period of time, we are conducting longitudinal research. Longitudinal research is a research design in which data-gathering is administered repeatedly over an extended period of time. For example, we may survey a group of individuals about their dietary habits at age 20, retest them a decade later at age 30, and then again at age 40.
Another approach is cross-sectional research. In cross-sectional research , a researcher compares multiple segments of the population at the same time. Using the dietary habits example above, the researcher might directly compare different groups of people by age. Instead a group of people for 20 years to see how their dietary habits changed from decade to decade, the researcher would study a group of 20-year-old individuals and compare them to a group of 30-year-old individuals and a group of 40-year-old individuals. While cross-sectional research requires a shorter-term investment, it is also limited by differences that exist between the different generations (or cohorts) that have nothing to do with age per se, but rather reflect the social and cultural experiences of different generations of individuals make them different from one another.
To illustrate this concept, consider the following survey findings. In recent years there has been significant growth in the popular support of same-sex marriage. Many studies on this topic break down survey participants into different age groups. In general, younger people are more supportive of same-sex marriage than are those who are older (Jones, 2013). Does this mean that as we age we become less open to the idea of same-sex marriage, or does this mean that older individuals have different perspectives because of the social climates in which they grew up? Longitudinal research is a powerful approach because the same individuals are involved in the research project over time, which means that the researchers need to be less concerned with differences among cohorts affecting the results of their study.
Often longitudinal studies are employed when researching various diseases in an effort to understand particular risk factors. Such studies often involve tens of thousands of individuals who are followed for several decades. Given the enormous number of people involved in these studies, researchers can feel confident that their findings can be generalized to the larger population. The Cancer Prevention Study-3 (CPS-3) is one of a series of longitudinal studies sponsored by the American Cancer Society aimed at determining predictive risk factors associated with cancer. When participants enter the study, they complete a survey about their lives and family histories, providing information on factors that might cause or prevent the development of cancer. Then every few years the participants receive additional surveys to complete. In the end, hundreds of thousands of participants will be tracked over 20 years to determine which of them develop cancer and which do not.
Clearly, this type of research is important and potentially very informative. For instance, earlier longitudinal studies sponsored by the American Cancer Society provided some of the first scientific demonstrations of the now well-established links between increased rates of cancer and smoking (American Cancer Society, n.d.) ( Figure 2.11 ).
As with any research strategy, longitudinal research is not without limitations. For one, these studies require an incredible time investment by the researcher and research participants. Given that some longitudinal studies take years, if not decades, to complete, the results will not be known for a considerable period of time. In addition to the time demands, these studies also require a substantial financial investment. Many researchers are unable to commit the resources necessary to see a longitudinal project through to the end.
Research participants must also be willing to continue their participation for an extended period of time, and this can be problematic. People move, get married and take new names, get ill, and eventually die. Even without significant life changes, some people may simply choose to discontinue their participation in the project. As a result, the attrition rates, or reduction in the number of research participants due to dropouts, in longitudinal studies are quite high and increases over the course of a project. For this reason, researchers using this approach typically recruit many participants fully expecting that a substantial number will drop out before the end. As the study progresses, they continually check whether the sample still represents the larger population, and make adjustments as necessary.
As an Amazon Associate we earn from qualifying purchases.
This book may not be used in the training of large language models or otherwise be ingested into large language models or generative AI offerings without OpenStax's permission.
Want to cite, share, or modify this book? This book uses the Creative Commons Attribution License and you must attribute OpenStax.
Access for free at https://openstax.org/books/psychology/pages/1-introduction
- Authors: Rose M. Spielman, Kathryn Dumper, William Jenkins, Arlene Lacombe, Marilyn Lovett, Marion Perlmutter
- Publisher/website: OpenStax
- Book title: Psychology
- Publication date: Dec 8, 2014
- Location: Houston, Texas
- Book URL: https://openstax.org/books/psychology/pages/1-introduction
- Section URL: https://openstax.org/books/psychology/pages/2-2-approaches-to-research
© Feb 9, 2022 OpenStax. Textbook content produced by OpenStax is licensed under a Creative Commons Attribution License . The OpenStax name, OpenStax logo, OpenStax book covers, OpenStax CNX name, and OpenStax CNX logo are not subject to the Creative Commons license and may not be reproduced without the prior and express written consent of Rice University.
- Open supplemental data
- Reference Manager
- Simple TEXT file
People also looked at
Original research article, learning scientific observation with worked examples in a digital learning environment.
- 1 Department Educational Sciences, Chair for Formal and Informal Learning, Technical University Munich School of Social Sciences and Technology, Munich, Germany
- 2 Aquatic Systems Biology Unit, TUM School of Life Sciences, Technical University of Munich, Freising, Germany
Science education often aims to increase learners’ acquisition of fundamental principles, such as learning the basic steps of scientific methods. Worked examples (WE) have proven particularly useful for supporting the development of such cognitive schemas and successive actions in order to avoid using up more cognitive resources than are necessary. Therefore, we investigated the extent to which heuristic WE are beneficial for supporting the acquisition of a basic scientific methodological skill—conducting scientific observation. The current study has a one-factorial, quasi-experimental, comparative research design and was conducted as a field experiment. Sixty two students of a German University learned about scientific observation steps during a course on applying a fluvial audit, in which several sections of a river were classified based on specific morphological characteristics. In the two experimental groups scientific observation was supported either via faded WE or via non-faded WE both presented as short videos. The control group did not receive support via WE. We assessed factual and applied knowledge acquisition regarding scientific observation, motivational aspects and cognitive load. The results suggest that WE promoted knowledge application: Learners from both experimental groups were able to perform the individual steps of scientific observation more accurately. Fading of WE did not show any additional advantage compared to the non-faded version in this regard. Furthermore, the descriptive results reveal higher motivation and reduced extraneous cognitive load within the experimental groups, but none of these differences were statistically significant. Our findings add to existing evidence that WE may be useful to establish scientific competences.
1 Introduction
Learning in science education frequently involves the acquisition of basic principles or generalities, whether of domain-specific topics (e.g., applying a mathematical multiplication rule) or of rather universal scientific methodologies (e.g., performing the steps of scientific observation) ( Lunetta et al., 2007 ). Previous research has shown that worked examples (WE) can be considered particularly useful for developing such cognitive schemata during learning to avoid using more cognitive resources than necessary for learning successive actions ( Renkl et al., 2004 ; Renkl, 2017 ). WE consist of the presentation of a problem, consecutive solution steps and the solution itself. This is especially advantageous in initial cognitive skill acquisition, i.e., for novice learners with low prior knowledge ( Kalyuga et al., 2001 ). With growing knowledge, fading WE can lead from example-based learning to independent problem-solving ( Renkl et al., 2002 ). Preliminary work has shown the advantage of WE in specific STEM domains like mathematics ( Booth et al., 2015 ; Barbieri et al., 2021 ), but less studies have investigated their impact on the acquisition of basic scientific competencies that involve heuristic problem-solving processes (scientific argumentation, Schworm and Renkl, 2007 ; Hefter et al., 2014 ; Koenen et al., 2017 ). In the realm of natural sciences, various basic scientific methodologies are employed to acquire knowledge, such as experimentation or scientific observation ( Wellnitz and Mayer, 2013 ). During the pursuit of knowledge through scientific inquiry activities, learners may encounter several challenges and difficulties. Similar to the hurdles faced in experimentation, where understanding the criteria for appropriate experimental design, including the development, measurement, and evaluation of results, is crucial ( Sirum and Humburg, 2011 ; Brownell et al., 2014 ; Dasgupta et al., 2014 ; Deane et al., 2014 ), scientific observation additionally presents its own set of issues. In scientific observation, e.g., the acquisition of new insights may be somewhat incidental due to spontaneous and uncoordinated observations ( Jensen, 2014 ). To address these challenges, it is crucial to provide instructional support, including the use of WE, particularly when observations are carried out in a more self-directed manner.
For this reason, the aim of the present study was to determine the usefulness of digitally presented WE to support the acquisition of a basic scientific methodological skill—conducting scientific observations—using a digital learning environment. In this regard, this study examined the effects of different forms of digitally presented WE (non-faded vs. faded) on students’ cognitive and motivational outcomes and compared them to a control group without WE. Furthermore, the combined perspective of factual and applied knowledge, as well as motivational and cognitive aspects, represent further value added to the study.
2 Theoretical background
2.1 worked examples.
WE have been commonly used in the fields of STEM education (science, technology, engineering, and mathematics) ( Booth et al., 2015 ). They consist of a problem statement, the steps to solve the problem, and the solution itself ( Atkinson et al., 2000 ; Renkl et al., 2002 ; Renkl, 2014 ). The success of WE can be explained by their impact on cognitive load (CL) during learning, based on assumptions from Cognitive Load Theory ( Sweller, 2006 ).
Learning with WE is considered time-efficient, effective, and superior to problem-based learning (presentation of the problem without demonstration of solution steps) when it comes to knowledge acquisition and transfer (WE-effect, Atkinson et al., 2000 ; Van Gog et al., 2011 ). Especially WE can help by reducing the extraneous load (presentation and design of the learning material) and, in turn, can lead to an increase in germane load (effort of the learner to understand the learning material) ( Paas et al., 2003 ; Renkl, 2014 ). With regard to intrinsic load (difficulty and complexity of the learning material), it is still controversially discussed if it can be altered by instructional design, e.g., WE ( Gerjets et al., 2004 ). WE have a positive effect on learning and knowledge transfer, especially for novices, as the step-by-step presentation of the solution requires less extraneous mental effort compared to problem-based learning ( Sweller et al., 1998 ; Atkinson et al., 2000 ; Bokosmaty et al., 2015 ). With growing knowledge, WE can lose their advantages (due to the expertise-reversal effect), and scaffolding learning via faded WE might be more successful for knowledge gain and transfer ( Renkl, 2014 ). Faded WE are similar to complete WE, but fade out solution steps as knowledge and competencies grow. Faded WE enhance near-knowledge transfer and reduce errors compared to non-faded WE ( Renkl et al., 2000 ).
In addition, the reduction of intrinsic and extraneous CL by WE also has an impact on learner motivation, such as interest ( Van Gog and Paas, 2006 ). Um et al. (2012) showed that there is a strong positive correlation between germane CL and the motivational aspects of learning, like satisfaction and emotion. Gupta (2019) mentions a positive correlation between CL and interest. Van Harsel et al. (2019) found that WE positively affect learning motivation, while no such effect was found for problem-solving. Furthermore, learning with WE increases the learners’ belief in their competence in completing a task. In addition, fading WE can lead to higher motivation for more experienced learners, while non-faded WE can be particularly motivating for learners without prior knowledge ( Paas et al., 2005 ). In general, fundamental motivational aspects during the learning process, such as situational interest ( Lewalter and Knogler, 2014 ) or motivation-relevant experiences, like basic needs, are influenced by learning environments. At the same time, their use also depends on motivational characteristics of the learning process, such as self-determined motivation ( Deci and Ryan, 2012 ). Therefore, we assume that learning with WE as a relevant component of a learning environment might also influence situational interest and basic needs.
2.1.1 Presentation of worked examples
WE are frequently used in digital learning scenarios ( Renkl, 2014 ). When designing WE, the application via digital learning media can be helpful, as their content can be presented in different ways (video, audio, text, and images), tailored to the needs of the learners, so that individual use is possible according to their own prior knowledge or learning pace ( Mayer, 2001 ). Also, digital media can present relevant information in a timely, motivating, appealing and individualized way and support learning in an effective and needs-oriented way ( Mayer, 2001 ). The advantages of using digital media in designing WE have already been shown in previous studies. Dart et al. (2020) presented WE as short videos (WEV). They report that the use of WEV leads to increased student satisfaction and more positive attitudes. Approximately 90% of the students indicated an active learning approach when learning with the WEV. Furthermore, the results show that students improved their content knowledge through WEV and that they found WEV useful for other courses as well.
Another study ( Kay and Edwards, 2012 ) presented WE as video podcasts. Here, the advantages of WE regarding self-determined learning in terms of learning location, learning time, and learning speed were shown. Learning performance improved significantly after use. The step-by-step, easy-to-understand explanations, the diagrams, and the ability to determine the learning pace by oneself were seen as beneficial.
Multimedia WE can also be enhanced with self-explanation prompts ( Berthold et al., 2009 ). Learning from WE with self-explanation prompts was shown to be superior to other learning methods, such as hypertext learning and observational learning.
In addition to presenting WE in different medial ways, WE can also comprise different content domains.
2.1.2 Content and context of worked examples
Regarding the content of WE, algorithmic and heuristic WE, as well as single-content and double-content WE, can be distinguished ( Reiss et al., 2008 ; Koenen et al., 2017 ; Renkl, 2017 ). Algorithmic WE are traditionally used in the very structured mathematical–physical field. Here, an algorithm with very specific solution steps is to learn, for example, in probability calculation ( Koenen et al., 2017 ). In this study, however, we focus on heuristic double-content WE. Heuristic WE in science education comprise fundamental scientific working methods, e.g., conducting experiments ( Koenen et al., 2017 ). Furthermore, double-content WE contain two learning domains that are relevant for the learning process: (1) the learning domain describes the primarily to be learned abstract process or concept, e.g., scientific methodologies like observation (see section 2.2), while (2) the exemplifying domain consists of the content that is necessary to teach this process or concept, e.g., mapping of river structure ( Renkl et al., 2009 ).
Depending on the WE content to be learned, it may be necessary for learning to take place in different settings. This can be in a formal or informal learning setting or a non-formal field setting. In this study, the focus is on learning scientific observation (learning domain) through river structure mapping (exemplary domain), which takes place with the support of digital media in a formal (university) setting, but in an informal context (nature).
2.2 Scientific observation
Scientific observation is fundamental to all scientific activities and disciplines ( Kohlhauf et al., 2011 ). Scientific observation must be clearly distinguished from everyday observation, where observation is purely a matter of noticing and describing specific characteristics ( Chinn and Malhotra, 2001 ). In contrast to this everyday observation, scientific observation as a method of knowledge acquisition can be described as a rather complex activity, defined as the theory-based, systematic and selective perception of concrete systems and processes without any fundamental manipulation ( Wellnitz and Mayer, 2013 ). Wellnitz and Mayer (2013) described the scientific observation process via six steps: (1) formulation of the research question (s), (2) deduction of the null hypothesis and the alternative hypothesis, (3) planning of the research design, (4) conducting the observation, (5) analyzing the data, and (6) answering the research question(s) on this basis. Only through reliable and qualified observation, valid data can be obtained that provide solid scientific evidence ( Wellnitz and Mayer, 2013 ).
Since observation activities are not trivial and learners often observe without generating new knowledge or connecting their observations to scientific explanations and thoughts, it is important to provide support at the related cognitive level, so that observation activities can be conducted in a structured way according to pre-defined criteria ( Ford, 2005 ; Eberbach and Crowley, 2009 ). Especially during field-learning experiences, scientific observation is often spontaneous and uncoordinated, whereby random discoveries result in knowledge gain ( Jensen, 2014 ).
To promote successful observing in rather unstructured settings like field trips, instructional support for the observation process seems useful. To guide observation activities, digitally presented WE seem to be an appropriate way to introduce learners to the individual steps of scientific observation using concrete examples.
2.3 Research questions and hypothesis
The present study investigates the effect of digitally presented double-content WE that supports the mapping of a small Bavarian river by demonstrating the steps of scientific observation. In this analysis, we focus on the learning domain of the WE and do not investigate the exemplifying domain in detail. Distinct ways of integrating WE in the digital learning environment (faded WE vs. non-faded WE) are compared with each other and with a control group (no WE). The aim is to examine to what extent differences between those conditions exist with regard to (RQ1) learners’ competence acquisition [acquisition of factual knowledge about the scientific observation method (quantitative data) and practical application of the scientific observation method (quantified qualitative data)], (RQ2) learners’ motivation (situational interest and basic needs), and (RQ3) CL. It is assumed that (Hypothesis 1), the integration of WE (faded and non-faded) leads to significantly higher competence acquisition (factual and applied knowledge), significantly higher motivation and significantly lower extraneous CL as well as higher germane CL during the learning process compared to a learning environment without WE. No differences between the conditions are expected regarding intrinsic CL. Furthermore, it is assumed (Hypothesis 2) that the integration of faded WE leads to significantly higher competence acquisition, significantly higher motivation, and lower extraneous CL as well as higher germane CL during the learning processes compared to non-faded WE. No differences between the conditions are expected with regard to intrinsic CL.
The study took place during the field trips of a university course on the application of a fluvial audit (FA) using the German working aid for mapping the morphology of rivers and their floodplains ( Bayerisches Landesamt für Umwelt, 2019 ). FA is the leading fluvial geomorphological tool for application to data collection contiguously along all watercourses of interest ( Walker et al., 2007 ). It is widely used because it is a key example of environmental conservation and monitoring that needs to be taught to students of selected study programs; thus, knowing about the most effective ways of learning is of high practical relevance.
3.1 Sample and design
3.1.1 sample.
The study was conducted with 62 science students and doctoral students of a German University (age M = 24.03 years; SD = 4.20; 36 females; 26 males). A total of 37 participants had already conducted a scientific observation and would rate their knowledge in this regard at a medium level ( M = 3.32 out of 5; SD = 0.88). Seven participants had already conducted an FA and would rate their knowledge in this regard at a medium level ( M = 3.14 out of 5; SD = 0.90). A total of 25 participants had no experience at all. Two participants had to be excluded from the sample afterward because no posttest results were available.
3.1.2 Design
The study has a 1-factorial quasi-experimental comparative research design and is conducted as a field experiment using a pre/posttest design. Participants were randomly assigned to one of three conditions: no WE ( n = 20), faded WE ( n = 20), and non-faded WE ( n = 20).
3.2 Implementation and material
3.2.1 implementation.
The study started with an online kick-off meeting where two lecturers informed all students within an hour about the basics regarding the assessment of the structural integrity of the study river and the course of the field trip days to conduct an FA. Afterward, within 2 weeks, students self-studied via Moodle the FA following the German standard method according to the scoresheets of Bayerisches Landesamt für Umwelt (2019) . This independent preparation using the online presented documents was a necessary prerequisite for participation in the field days and was checked in the pre-testing. The preparatory online documents included six short videos and four PDF files on the content, guidance on the German protocol of the FA, general information on river landscapes, information about anthropogenic changes in stream morphology and the scoresheets for applying the FA. In these sheets, the river and its floodplain are subdivided into sections of 100 m in length. Each of these sections is evaluated by assessing 21 habitat factors related to flow characteristics and structural variability. The findings are then transferred into a scoring system for the description of structural integrity from 1 (natural) to 7 (highly modified). Habitat factors have a decisive influence on the living conditions of animals and plants in and around rivers. They included, e.g., variability in water depth, stream width, substratum diversity, or diversity of flow velocities.
3.2.2 Materials
On the field trip days, participants were handed a tablet and a paper-based FA worksheet (last accessed 21st September 2022). 1 This four-page assessment sheet was accompanied by a digital learning environment presented on Moodle that instructed the participants on mapping the water body structure and guided the scientific observation method. All three Moodle courses were identical in structure and design; the only difference was the implementation of the WE. Below, the course without WE are described first. The other two courses have an identical structure, but contain additional WE in the form of learning videos.
3.2.3 No worked example
After a short welcome and introduction to the course navigation, the FA started with the description of a short hypothetical scenario: Participants should take the role of an employee of an urban planning office that assesses the ecomorphological status of a small river near a Bavarian city. The river was divided into five sections that had to be mapped separately. The course was structured accordingly. At the beginning of each section, participants had to formulate and write down a research question, and according to hypotheses regarding the ecomorphological status of the river’s section, they had to collect data in this regard via the mapping sheet and then evaluate their data and draw a conclusion. Since this course serves as a control group, no WE videos supporting the scientific observation method were integrated. The layout of the course is structured like a book, where it is not possible to scroll back. This is important insofar as the participants do not have the possibility to revisit information in order to keep the conditions comparable as well as distinguishable.
3.2.4 Non-faded worked example
In the course with no-faded WE, three instructional videos are shown for each of the five sections. In each of the three videos, two steps of the scientific observation method are presented so that, finally, all six steps of scientific observation are demonstrated. The mapping of the first section starts after the general introduction (as described above) with the instruction to work on the first two steps of scientific observation: the formulation of a research question and hypotheses. To support this, a video of about 4 min explains the features of scientific sound research questions and hypotheses. To this aim, a practical example, including explanations and tips, is given regarding the formulation of research questions and hypotheses for this section (e.g., “To what extent does the building development and the closeness of the path to the water body have an influence on the structure of the water body?” Alternative hypothesis: It is assumed that the housing development and the closeness of the path to the water body have a negative influence on the water body structure. Null hypothesis: It is assumed that the housing development and the closeness of the path to the watercourse have no negative influence on the watercourse structure.). Participants should now formulate their own research questions and hypotheses, write them down in a text field at the end of the page, and then skip to the next page. The next two steps of scientific observation, planning and conducting, are explained in a short 4-min video. To this aim, a practical example including explanations and tips is given regarding planning and conducting scientific for this section (e.g., “It’s best to go through each evaluation category carefully one by one that way you are sure not to forget anything!”). Now, participants were asked to collect data for the first section using their paper-based FA worksheet. Participants individually surveyed the river and reported their results in the mapping sheet by ticking the respective boxes in it. After collecting this data, they returned to the digital learning environment to learn how to use these data by studying the last two steps of scientific observation, evaluation, and conclusion. The third 4-min video explained how to evaluate and interpret collected data. For this purpose, a practical example with explanations and tips is given regarding evaluating and interpreting data for this section (e.g., “What were the individual points that led to the assessment? Have there been points that were weighted more than others? Remember the introduction video!”). At the end of the page, participants could answer their before-stated research questions and hypotheses by evaluating their collected data and drawing a conclusion. This brings participants to the end of the first mapping section. Afterward, the cycle begins again with the second section of the river that has to be mapped. Again, participants had to conduct the steps of scientific observation, guided by WE videos, explaining the steps in slightly different wording or with different examples. A total of five sections are mapped, in which the structure of the learning environment and the videos follow the same procedure.
3.2.5 Faded worked example
The digital learning environment with the faded WE follow the same structure as the version with the non-faded WE. However, in this version, the information in the WE videos is successively reduced. In the first section, all three videos are identical to the version with the non-faded WE. In the second section, faded content was presented as follows: the tip at the end was omitted in all three videos. In the third section, the tip and the practical example were omitted. In the fourth and fifth sections, no more videos were presented, only the work instructions.
3.3 Procedure
The data collection took place on four continuous days on the university campus, with a maximum group size of 15 participants on each day. The students were randomly assigned to one of the three conditions (no WE vs. faded WE vs. non-faded WE). After a short introduction to the procedure, the participants were handed the paper-based FA worksheet and one tablet per person. Students scanned the QR code on the first page of the worksheet that opened the pretest questionnaire, which took about 20 min to complete. After completing the questionnaire, the group walked for about 15 min to the nearby small river that was to be mapped. Upon arrival, there was first a short introduction to the digital learning environment and a check that the login (via university account on Moodle) worked. During the next 4 h, the participants individually mapped five segments of the river using the cartography worksheet. They were guided through the steps of scientific observation using the digital learning environment on the tablet. The results of their scientific observation were logged within the digital learning environment. At the end of the digital learning environment, participants were directed to the posttest via a link. After completing the test, the tablets and mapping sheets were returned. Overall, the study took about 5 h per group each day.
3.4 Instruments
In the pretest, sociodemographic data (age and gender), the study domain and the number of study semesters were collected. Additionally, the previous scientific observation experience and the estimation of one’s own ability in this regard were assessed. For example, it was asked whether scientific observation had already been conducted and, if so, how the abilities were rated on a 5-point scale from very low to very high. Preparation for the FA on the basis of the learning material was assessed: Participants were asked whether they had studied all six videos and all four PDF documents, with the response options not at all, partially, and completely. Furthermore, a factual knowledge test about scientific observation and questions about self-determination theory was administered. The posttest used the same knowledge test, and additional questions on basic needs, situational interest, measures of CL and questions about the usefulness of the WE. All scales were presented online, and participants reached the questionnaire via QR code.
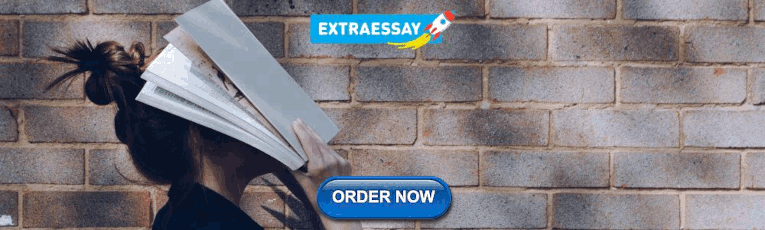
3.4.1 Scientific observation competence acquisition
For the factual knowledge (quantitative assessment of the scientific observation competence), a single-choice knowledge test with 12 questions was developed and used as pre- and posttest with a maximum score of 12 points. It assesses the learners’ knowledge of the scientific observation method regarding the steps of scientific observation, e.g., formulating research questions and hypotheses or developing a research design. The questions are based on Wahser (2008 , adapted by Koenen, 2014 ) and adapted to scientific observation: “Although you are sure that you have conducted the scientific observation correctly, an unexpected result turns up. What conclusion can you draw?” Each question has four answer options (one of which is correct) and, in addition, one “I do not know” option.
For the applied knowledge (quantified qualitative assessment of the scientific observation competence), students’ scientific observations written in the digital learning environment were analyzed. A coding scheme was used with the following codes: 0 = insufficient (text field is empty or includes only insufficient key points), 1 = sufficient (a research question and no hypotheses or research question and inappropriate hypotheses are stated), 2 = comprehensive (research question and appropriate hypothesis or research question and hypotheses are stated, but, e.g., incorrect null hypothesis), 3 = very comprehensive (correct research question, hypothesis and null hypothesis are stated). One example of a very comprehensive answer regarding the research question and hypothesis is: To what extent does the lack of riparian vegetation have an impact on water body structure? Hypothesis: The lack of shore vegetation has a negative influence on the water body structure. Null hypothesis: The lack of shore vegetation has no influence on the water body structure. Afterward, a sum score was calculated for each participant. Five times, a research question and hypotheses (steps 1 and 2 in the observation process) had to be formulated (5 × max. 3 points = 15 points), and five times, the research questions and hypotheses had to be answered (steps 5 and 6 in the observation process: evaluation and conclusion) (5 × max. 3 points = 15 points). Overall, participants could reach up to 30 points. Since the observation and evaluation criteria in data collection and analysis were strongly predetermined by the scoresheet, steps 3 and 4 of the observation process (planning and conducting) were not included in the analysis.
All 600 cases (60 participants, each 10 responses to code) were coded by the first author. For verification, 240 cases (24 randomly selected participants, eight from each course) were cross-coded by an external coder. In 206 of the coded cases, the raters agreed. The cases in which the raters did not agree were discussed together, and a solution was found. This results in Cohen’s κ = 0.858, indicating a high to very high level of agreement. This indicates that the category system is clearly formulated and that the individual units of analysis could be correctly assigned.
3.4.2 Self-determination index
For the calculation of the self-determination index (SDI-index), Thomas and Müller (2011) scale for self-determination was used in the pretest. The scale consists of four subscales: intrinsic motivation (five items; e.g., I engage with the workshop content because I enjoy it; reliability of alpha = 0.87), identified motivation (four items; e.g., I engage with the workshop content because it gives me more options when choosing a career; alpha = 0.84), introjected motivation (five items; e.g., I engage with the workshop content because otherwise I would have a guilty feeling; alpha = 0.79), and external motivation (three items, e.g., I engage with the workshop content because I simply have to learn it; alpha = 0.74). Participants could indicate their answers on a 5-point Likert scale ranging from 1 = completely disagree to 5 = completely agree. To calculate the SDI-index, the sum of the self-determined regulation styles (intrinsic and identified) is subtracted from the sum of the external regulation styles (introjected and external), where intrinsic and external regulation are scored two times ( Thomas and Müller, 2011 ).
3.4.3 Motivation
Basic needs were measured in the posttest with the scale by Willems and Lewalter (2011) . The scale consists of three subscales: perceived competence (four items; e.g., during the workshop, I felt that I could meet the requirements; alpha = 0.90), perceived autonomy (five items; e.g., during the workshop, I felt that I had a lot of freedom; alpha = 0.75), and perceived autonomy regarding personal wishes and goals (APWG) (four items; e.g., during the workshop, I felt that the workshop was how I wish it would be; alpha = 0.93). We added all three subscales to one overall basic needs scale (alpha = 0.90). Participants could indicate their answers on a 5-point Likert scale ranging from 1 = completely disagree to 5 = completely agree.
Situational interest was measured in the posttest with the 12-item scale by Lewalter and Knogler (2014 ; Knogler et al., 2015 ; Lewalter, 2020 ; alpha = 0.84). The scale consists of two subscales: catch (six items; e.g., I found the workshop exciting; alpha = 0.81) and hold (six items; e.g., I would like to learn more about parts of the workshop; alpha = 0.80). Participants could indicate their answers on a 5-point Likert scale ranging from 1 = completely disagree to 5 = completely agree.
3.4.4 Cognitive load
In the posttest, CL was used to examine the mental load during the learning process. The intrinsic CL (three items; e.g., this task was very complex; alpha = 0.70) and extraneous CL (three items; e.g., in this task, it is difficult to identify the most important information; alpha = 0.61) are measured with the scales from Klepsch et al. (2017) . The germane CL (two items; e.g., the learning session contained elements that supported me to better understand the learning material; alpha = 0.72) is measured with the scale from Leppink et al. (2013) . Participants could indicate their answers on a 5-point Likert scale ranging from 1 = completely disagree to 5 = completely agree.
3.4.5 Attitudes toward worked examples
To measure how effective participants rated the WE, we used two scales related to the WE videos as instructional support. The first scale from Renkl (2001) relates to the usefulness of WE. The scale consists of four items (e.g., the explanations were helpful; alpha = 0.71). Two items were recoded because they were formulated negatively. The second scale is from Wachsmuth (2020) and relates to the participant’s evaluation of the WE. The scale consists of nine items (e.g., I always did what was explained in the learning videos; alpha = 0.76). Four items were recoded because they were formulated negatively. Participants could indicate their answers on a 5-point Likert scale ranging from 1 = completely disagree to 5 = completely agree.
3.5 Data analysis
An ANOVA was used to calculate if the variable’s prior knowledge and SDI index differed between the three groups. However, as no significant differences between the conditions were found [prior factual knowledge: F (2, 59) = 0.15, p = 0.865, η 2 = 0.00 self-determination index: F (2, 59) = 0.19, p = 0.829, η 2 = 0.00], they were not included as covariates in subsequent analyses.
Furthermore, a repeated measure, one-way analysis of variance (ANOVA), was conducted to compare the three treatment groups (no WE vs. faded WE vs. non-faded WE) regarding the increase in factual knowledge about the scientific observation method from pretest to posttest.
A MANOVA (multivariate analysis) was calculated with the three groups (no WE vs. non-faded WE vs. faded WE) as a fixed factor and the dependent variables being the practical application of the scientific observation method (first research question), situational interest, basic needs (second research question), and CL (third research question).
Additionally, to determine differences in applied knowledge even among the three groups, Bonferroni-adjusted post-hoc analyses were conducted.
The descriptive statistics between the three groups in terms of prior factual knowledge about the scientific observation method and the self-determination index are shown in Table 1 . The descriptive statistics revealed only small, non-significant differences between the three groups in terms of factual knowledge.

Table 1 . Means (standard deviations) of factual knowledge tests (pre- and posttest) and self-determination index for the three different groups.
The results of the ANOVA revealed that the overall increase in factual knowledge from pre- to posttest just misses significance [ F (1, 57) = 3.68, p = 0.060, η 2 = 0 0.06]. Furthermore, no significant differences between the groups were found regarding the acquisition of factual knowledge from pre- to posttest [ F (2, 57) = 2.93, p = 0.062, η 2 = 0.09].
An analysis of the descriptive statistics showed that the largest differences between the groups were found in applied knowledge (qualitative evaluation) and extraneous load (see Table 2 ).
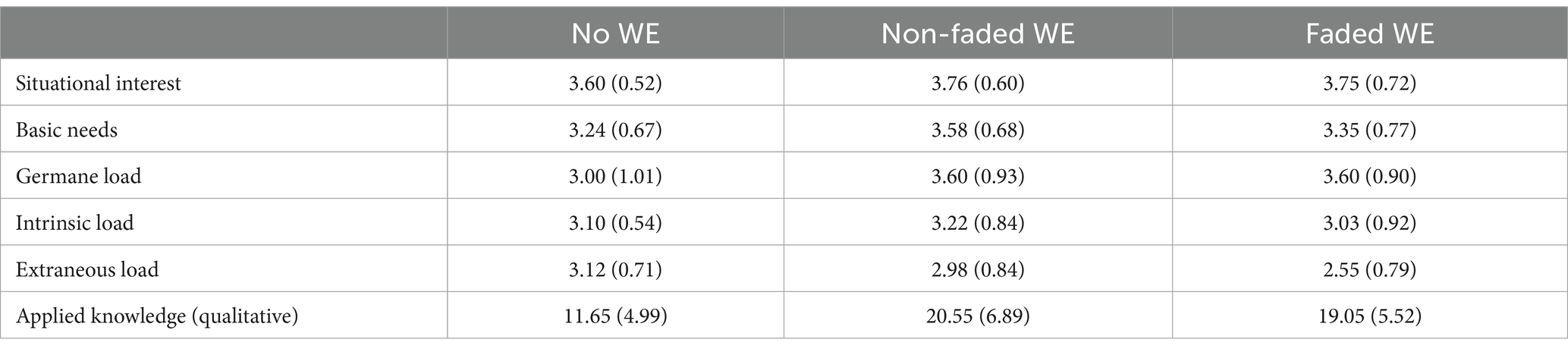
Table 2 . Means (standard deviations) of dependent variables with the three different groups.
Results of the MANOVA revealed significant overall differences between the three groups [ F (12, 106) = 2.59, p = 0.005, η 2 = 0.23]. Significant effects were found for the application of knowledge [ F (2, 57) = 13.26, p = <0.001, η 2 = 0.32]. Extraneous CL just missed significance [ F (2, 57) = 2.68, p = 0.065, η 2 = 0.09]. There were no significant effects for situational interest [ F (2, 57) = 0.44, p = 0.644, η 2 = 0.02], basic needs [ F (2, 57) = 1.22, p = 0.302, η 2 = 0.04], germane CL [ F (2, 57) = 2.68, p = 0.077, η 2 = 0.09], and intrinsic CL [ F (2, 57) = 0.28, p = 0.757, η 2 = 0.01].
Bonferroni-adjusted post hoc analysis revealed that the group without WE had significantly lower scores in the evaluation of the applied knowledge than the group with non-faded WE ( p = <0.001, M diff = −8.90, 95% CI [−13.47, −4.33]) and then the group with faded WE ( p = <0.001, M diff = −7.40, 95% CI [−11.97, −2.83]). No difference was found between the groups with faded and non-faded WE ( p = 1.00, M diff = −1.50, 95% CI [−6.07, 3.07]).
The descriptive statistics regarding the perceived usefulness of WE and participants’ evaluation of the WE revealed that the group with the faded WE rated usefulness slightly higher than the participants with non-faded WE and also reported a more positive evaluation. However, the results of a MANOVA revealed no significant overall differences [ F (2, 37) = 0.32, p = 0.732, η 2 = 0 0.02] (see Table 3 ).

Table 3 . Means (standard deviations) of dependent variables with the three different groups.
5 Discussion
This study investigated the use of WE to support students’ acquisition of science observation. Below, the research questions are answered, and the implications and limitations of the study are discussed.
5.1 Results on factual and applied knowledge
In terms of knowledge gain (RQ1), our findings revealed no significant differences in participants’ results of the factual knowledge test both across all three groups and specifically between the two experimental groups. These results are in contradiction with related literature where WE had a positive impact on knowledge acquisition ( Renkl, 2014 ) and faded WE are considered to be more effective in knowledge acquisition and transfer, in contrast to non-faded WE ( Renkl et al., 2000 ; Renkl, 2014 ). A limitation of the study is the fact that the participants already scored very high on the pretest, so participation in the intervention would likely not yield significant knowledge gains due to ceiling effects ( Staus et al., 2021 ). Yet, nearly half of the students reported being novices in the field prior to the study, suggesting that the difficulty of some test items might have been too low. Here, it would be important to revise the factual knowledge test, e.g., the difficulty of the distractors in further study.
Nevertheless, with regard to application knowledge, the results revealed large significant differences: Participants of the two experimental groups performed better in conducting scientific observation steps than participants of the control group. In the experimental groups, the non-faded WE group performed better than the faded WE group. However, the absence of significant differences between the two experimental groups suggests that faded and non-faded WE used as double-content WE are suitable to teach applied knowledge about scientific observation in the learning domain ( Koenen, 2014 ). Furthermore, our results differ from the findings of Renkl et al. (2000) , in which the faded version led to the highest knowledge transfer. Despite the fact that the non-faded WE performed best in our study, the faded version of the WE was also appropriate to improve learning, confirming the findings of Renkl (2014) and Hesser and Gregory (2015) .
5.2 Results on learners’ motivation
Regarding participants’ motivation (RQ2; situational interest and basic needs), no significant differences were found across all three groups or between the two experimental groups. However, descriptive results reveal slightly higher motivation in the two experimental groups than in the control group. In this regard, our results confirm existing literature on a descriptive level showing that WE lead to higher learning-relevant motivation ( Paas et al., 2005 ; Van Harsel et al., 2019 ). Additionally, both experimental groups rated the usefulness of the WE as high and reported a positive evaluation of the WE. Therefore, we assume that even non-faded WE do not lead to over-instruction. Regarding the descriptive tendency, a larger sample might yield significant results and detect even small effects in future investigations. However, because this study also focused on comprehensive qualitative data analysis, it was not possible to evaluate a larger sample in this study.
5.3 Results on cognitive load
Finally, CL did not vary significantly across all three groups (RQ3). However, differences in extraneous CL just slightly missed significance. In descriptive values, the control group reported the highest extrinsic and lowest germane CL. The faded WE group showed the lowest extrinsic CL and a similar germane CL as the non-faded WE group. These results are consistent with Paas et al. (2003) and Renkl (2014) , reporting that WE can help to reduce the extraneous CL and, in return, lead to an increase in germane CL. Again, these differences were just above the significance level, and it would be advantageous to retest with a larger sample to detect even small effects.
Taken together, our results only partially confirm H1: the integration of WE (both faded and non-faded WE) led to a higher acquisition of application knowledge than the control group without WE, but higher factual knowledge was not found. Furthermore, higher motivation or different CL was found on a descriptive level only. The control group provided the basis for comparison with the treatment in order to investigate if there is an effect at all and, if so, how large the effect is. This is an important point to assess whether the effort of implementing WE is justified. Additionally, regarding H2, our results reveal no significant differences between the two WE conditions. We assume that the high complexity of the FA could play a role in this regard, which might be hard to handle, especially for beginners, so learners could benefit from support throughout (i.e., non-faded WE).
In addition to the limitations already mentioned, it must be noted that only one exemplary topic was investigated, and the sample only consisted of students. Since only the learning domain of the double-content WE was investigated, the exemplifying domain could also be analyzed, or further variables like motivation could be included in further studies. Furthermore, the influence of learners’ prior knowledge on learning with WE could be investigated, as studies have found that WE are particularly beneficial in the initial acquisition of cognitive skills ( Kalyuga et al., 2001 ).
6 Conclusion
Overall, the results of the current study suggest a beneficial role for WE in supporting the application of scientific observation steps. A major implication of these findings is that both faded and non-faded WE should be considered, as no general advantage of faded WE over non-faded WE was found. This information can be used to develop targeted interventions aimed at the support of scientific observation skills.
Data availability statement
The raw data supporting the conclusions of this article will be made available by the authors, without undue reservation.
Ethics statement
Ethical approval was not required for the study involving human participants in accordance with the local legislation and institutional requirements. Written informed consent to participate in this study was not required from the participants in accordance with the national legislation and the institutional requirements.
Author contributions
ML: Writing – original draft. SM: Writing – review & editing. JP: Writing – review & editing. JG: Writing – review & editing. DL: Writing – review & editing.
The author(s) declare that no financial support was received for the research, authorship, and/or publication of this article.
Conflict of interest
The authors declare that the research was conducted in the absence of any commercial or financial relationships that could be construed as a potential conflict of interest.
Publisher’s note
All claims expressed in this article are solely those of the authors and do not necessarily represent those of their affiliated organizations, or those of the publisher, the editors and the reviewers. Any product that may be evaluated in this article, or claim that may be made by its manufacturer, is not guaranteed or endorsed by the publisher.
Supplementary material
The Supplementary material for this article can be found online at: https://www.frontiersin.org/articles/10.3389/feduc.2024.1293516/full#supplementary-material
1. ^ https://www.lfu.bayern.de/wasser/gewaesserstrukturkartierung/index.htm
Atkinson, R. K., Derry, S. J., Renkl, A., and Wortham, D. (2000). Learning from examples: instructional principles from the worked examples research. Rev. Educ. Res. 70, 181–214. doi: 10.3102/00346543070002181
Crossref Full Text | Google Scholar
Barbieri, C. A., Booth, J. L., Begolli, K. N., and McCann, N. (2021). The effect of worked examples on student learning and error anticipation in algebra. Instr. Sci. 49, 419–439. doi: 10.1007/s11251-021-09545-6
Bayerisches Landesamt für Umwelt. (2019). Gewässerstrukturkartierung von Fließgewässern in Bayern – Erläuterungen zur Erfassung und Bewertung. (Water structure mapping of flowing waters in Bavaria - Explanations for recording and assessment) . Available at: https://www.bestellen.bayern.de/application/eshop_app000005?SID=1020555825&ACTIONxSESSxSHOWPIC(BILDxKEY:%27lfu_was_00152%27,BILDxCLASS:%27Artikel%27,BILDxTYPE:%27PDF%27)
Google Scholar
Berthold, K., Eysink, T. H., and Renkl, A. (2009). Assisting self-explanation prompts are more effective than open prompts when learning with multiple representations. Instr. Sci. 37, 345–363. doi: 10.1007/s11251-008-9051-z
Bokosmaty, S., Sweller, J., and Kalyuga, S. (2015). Learning geometry problem solving by studying worked examples: effects of learner guidance and expertise. Am. Educ. Res. J. 52, 307–333. doi: 10.3102/0002831214549450
Booth, J. L., McGinn, K., Young, L. K., and Barbieri, C. A. (2015). Simple practice doesn’t always make perfect. Policy Insights Behav. Brain Sci. 2, 24–32. doi: 10.1177/2372732215601691
Brownell, S. E., Wenderoth, M. P., Theobald, R., Okoroafor, N., Koval, M., Freeman, S., et al. (2014). How students think about experimental design: novel conceptions revealed by in-class activities. Bioscience 64, 125–137. doi: 10.1093/biosci/bit016
Chinn, C. A., and Malhotra, B. A. (2001). “Epistemologically authentic scientific reasoning” in Designing for science: implications from everyday, classroom, and professional settings . eds. K. Crowley, C. D. Schunn, and T. Okada (Mahwah, NJ: Lawrence Erlbaum), 351–392.
Dart, S., Pickering, E., and Dawes, L. (2020). Worked example videos for blended learning in undergraduate engineering. AEE J. 8, 1–22. doi: 10.18260/3-1-1153-36021
Dasgupta, A., Anderson, T. R., and Pelaez, N. J. (2014). Development and validation of a rubric for diagnosing students’ experimental design knowledge and difficulties. CBE Life Sci. Educ. 13, 265–284. doi: 10.1187/cbe.13-09-0192
PubMed Abstract | Crossref Full Text | Google Scholar
Deane, T., Nomme, K. M., Jeffery, E., Pollock, C. A., and Birol, G. (2014). Development of the biological experimental design concept inventory (BEDCI). CBE Life Sci. Educ. 13, 540–551. doi: 10.1187/cbe.13-11-0218
Deci, E. L., and Ryan, R. M. (2012). Self-determination theory. In P. A. M. LangeVan, A. W. Kruglanski, and E. T. Higgins (Eds.), Handbook of theories of social psychology , 416–436.
Eberbach, C., and Crowley, K. (2009). From everyday to scientific observation: how children learn to observe the Biologist’s world. Rev. Educ. Res. 79, 39–68. doi: 10.3102/0034654308325899
Ford, D. (2005). The challenges of observing geologically: third graders’ descriptions of rock and mineral properties. Sci. Educ. 89, 276–295. doi: 10.1002/sce.20049
Gerjets, P., Scheiter, K., and Catrambone, R. (2004). Designing instructional examples to reduce intrinsic cognitive load: molar versus modular presentation of solution procedures. Instr. Sci. 32, 33–58. doi: 10.1023/B:TRUC.0000021809.10236.71
Gupta, U. (2019). Interplay of germane load and motivation during math problem solving using worked examples. Educ. Res. Theory Pract. 30, 67–71.
Hefter, M. H., Berthold, K., Renkl, A., Riess, W., Schmid, S., and Fries, S. (2014). Effects of a training intervention to foster argumentation skills while processing conflicting scientific positions. Instr. Sci. 42, 929–947. doi: 10.1007/s11251-014-9320-y
Hesser, T. L., and Gregory, J. L. (2015). Exploring the Use of Faded Worked Examples as a Problem Solving Approach for Underprepared Students. High. Educ. Stud. 5, 36–46.
Jensen, E. (2014). Evaluating children’s conservation biology learning at the zoo. Conserv. Biol. 28, 1004–1011. doi: 10.1111/cobi.12263
Kalyuga, S., Chandler, P., Tuovinen, J., and Sweller, J. (2001). When problem solving is superior to studying worked examples. J. Educ. Psychol. 93, 579–588. doi: 10.1037/0022-0663.93.3.579
Kay, R. H., and Edwards, J. (2012). Examining the use of worked example video podcasts in middle school mathematics classrooms: a formative analysis. Can. J. Learn. Technol. 38, 1–20. doi: 10.21432/T2PK5Z
Klepsch, M., Schmitz, F., and Seufert, T. (2017). Development and validation of two instruments measuring intrinsic, extraneous, and germane cognitive load. Front. Psychol. 8:1997. doi: 10.3389/fpsyg.2017.01997
Knogler, M., Harackiewicz, J. M., Gegenfurtner, A., and Lewalter, D. (2015). How situational is situational interest? Investigating the longitudinal structure of situational interest. Contemp. Educ. Psychol. 43, 39–50. doi: 10.1016/j.cedpsych.2015.08.004
Koenen, J. (2014). Entwicklung und Evaluation von experimentunterstützten Lösungsbeispielen zur Förderung naturwissenschaftlich experimenteller Arbeitsweisen . Dissertation.
Koenen, J., Emden, M., and Sumfleth, E. (2017). Naturwissenschaftlich-experimentelles Arbeiten. Potenziale des Lernens mit Lösungsbeispielen und Experimentierboxen. (scientific-experimental work. Potentials of learning with solution examples and experimentation boxes). Zeitschrift für Didaktik der Naturwissenschaften 23, 81–98. doi: 10.1007/s40573-017-0056-5
Kohlhauf, L., Rutke, U., and Neuhaus, B. J. (2011). Influence of previous knowledge, language skills and domain-specific interest on observation competency. J. Sci. Educ. Technol. 20, 667–678. doi: 10.1007/s10956-011-9322-3
Leppink, J., Paas, F., Van der Vleuten, C. P., Van Gog, T., and Van Merriënboer, J. J. (2013). Development of an instrument for measuring different types of cognitive load. Behav. Res. Methods 45, 1058–1072. doi: 10.3758/s13428-013-0334-1
Lewalter, D. (2020). “Schülerlaborbesuche aus motivationaler Sicht unter besonderer Berücksichtigung des Interesses. (Student laboratory visits from a motivational perspective with special attention to interest)” in Handbuch Forschen im Schülerlabor – theoretische Grundlagen, empirische Forschungsmethoden und aktuelle Anwendungsgebiete . eds. K. Sommer, J. Wirth, and M. Vanderbeke (Münster: Waxmann-Verlag), 62–70.
Lewalter, D., and Knogler, M. (2014). “A questionnaire to assess situational interest – theoretical considerations and findings” in Poster Presented at the 50th Annual Meeting of the American Educational Research Association (AERA) (Philadelphia, PA)
Lunetta, V., Hofstein, A., and Clough, M. P. (2007). Learning and teaching in the school science laboratory: an analysis of research, theory, and practice. In N. Lederman and S. Abel (Eds.). Handbook of research on science education , Mahwah, NJ: Lawrence Erlbaum, 393–441.
Mayer, R. E. (2001). Multimedia learning. Cambridge University Press.
Paas, F., Renkl, A., and Sweller, J. (2003). Cognitive load theory and instructional design: recent developments. Educ. Psychol. 38, 1–4. doi: 10.1207/S15326985EP3801_1
Paas, F., Tuovinen, J., van Merriënboer, J. J. G., and Darabi, A. (2005). A motivational perspective on the relation between mental effort and performance: optimizing learner involvement in instruction. Educ. Technol. Res. Dev. 53, 25–34. doi: 10.1007/BF02504795
Reiss, K., Heinze, A., Renkl, A., and Groß, C. (2008). Reasoning and proof in geometry: effects of a learning environment based on heuristic worked-out examples. ZDM Int. J. Math. Educ. 40, 455–467. doi: 10.1007/s11858-008-0105-0
Renkl, A. (2001). Explorative Analysen zur effektiven Nutzung von instruktionalen Erklärungen beim Lernen aus Lösungsbeispielen. (Exploratory analyses of the effective use of instructional explanations in learning from worked examples). Unterrichtswissenschaft 29, 41–63. doi: 10.25656/01:7677
Renkl, A. (2014). “The worked examples principle in multimedia learning” in Cambridge handbook of multimedia learning . ed. R. E. Mayer (Cambridge University Press), 391–412.
Renkl, A. (2017). Learning from worked-examples in mathematics: students relate procedures to principles. ZDM 49, 571–584. doi: 10.1007/s11858-017-0859-3
Renkl, A., Atkinson, R. K., and Große, C. S. (2004). How fading worked solution steps works. A cognitive load perspective. Instr. Sci. 32, 59–82. doi: 10.1023/B:TRUC.0000021815.74806.f6
Renkl, A., Atkinson, R. K., and Maier, U. H. (2000). “From studying examples to solving problems: fading worked-out solution steps helps learning” in Proceeding of the 22nd Annual Conference of the Cognitive Science Society . eds. L. Gleitman and A. K. Joshi (Mahwah, NJ: Erlbaum), 393–398.
Renkl, A., Atkinson, R. K., Maier, U. H., and Staley, R. (2002). From example study to problem solving: smooth transitions help learning. J. Exp. Educ. 70, 293–315. doi: 10.1080/00220970209599510
Renkl, A., Hilbert, T., and Schworm, S. (2009). Example-based learning in heuristic domains: a cognitive load theory account. Educ. Psychol. Rev. 21, 67–78. doi: 10.1007/s10648-008-9093-4
Schworm, S., and Renkl, A. (2007). Learning argumentation skills through the use of prompts for self-explaining examples. J. Educ. Psychol. 99, 285–296. doi: 10.1037/0022-0663.99.2.285
Sirum, K., and Humburg, J. (2011). The experimental design ability test (EDAT). Bioscene 37, 8–16.
Staus, N. L., O’Connell, K., and Storksdieck, M. (2021). Addressing the ceiling effect when assessing STEM out-of-school time experiences. Front. Educ. 6:690431. doi: 10.3389/feduc.2021.690431
Sweller, J. (2006). The worked example effect and human cognition. Learn. Instr. 16, 165–169. doi: 10.1016/j.learninstruc.2006.02.005
Sweller, J., Van Merriënboer, J. J. G., and Paas, F. (1998). Cognitive architecture and instructional design. Educ. Psychol. Rev. 10, 251–295. doi: 10.1023/A:1022193728205
Thomas, A. E., and Müller, F. H. (2011). “Skalen zur motivationalen Regulation beim Lernen von Schülerinnen und Schülern. Skalen zur akademischen Selbstregulation von Schüler/innen SRQ-A [G] (überarbeitete Fassung)” in Scales of motivational regulation in student learning. Student academic self-regulation scales SRQ-A [G] (revised version). Wissenschaftliche Beiträge aus dem Institut für Unterrichts- und Schulentwicklung Nr. 5 (Klagenfurt: Alpen-Adria-Universität)
Um, E., Plass, J. L., Hayward, E. O., and Homer, B. D. (2012). Emotional design in multimedia learning. J. Educ. Psychol. 104, 485–498. doi: 10.1037/a0026609
Van Gog, T., Kester, L., and Paas, F. (2011). Effects of worked examples, example-problem, and problem- example pairs on novices’ learning. Contemp. Educ. Psychol. 36, 212–218. doi: 10.1016/j.cedpsych.2010.10.004
Van Gog, T., and Paas, G. W. C. (2006). Optimising worked example instruction: different ways to increase germane cognitive load. Learn. Instr. 16, 87–91. doi: 10.1016/j.learninstruc.2006.02.004
Van Harsel, M., Hoogerheide, V., Verkoeijen, P., and van Gog, T. (2019). Effects of different sequences of examples and problems on motivation and learning. Contemp. Educ. Psychol. 58, 260–275. doi: 10.1002/acp.3649
Wachsmuth, C. (2020). Computerbasiertes Lernen mit Aufmerksamkeitsdefizit: Unterstützung des selbstregulierten Lernens durch metakognitive prompts. (Computer-based learning with attention deficit: supporting self-regulated learning through metacognitive prompts) . Chemnitz: Dissertation Technische Universität Chemnitz.
Wahser, I. (2008). Training von naturwissenschaftlichen Arbeitsweisen zur Unterstützung experimenteller Kleingruppenarbeit im Fach Chemie (Training of scientific working methods to support experimental small group work in chemistry) . Dissertation
Walker, J., Gibson, J., and Brown, D. (2007). Selecting fluvial geomorphological methods for river management including catchment scale restoration within the environment agency of England and Wales. Int. J. River Basin Manag. 5, 131–141. doi: 10.1080/15715124.2007.9635313
Wellnitz, N., and Mayer, J. (2013). Erkenntnismethoden in der Biologie – Entwicklung und evaluation eines Kompetenzmodells. (Methods of knowledge in biology - development and evaluation of a competence model). Z. Didaktik Naturwissensch. 19, 315–345.
Willems, A. S., and Lewalter, D. (2011). “Welche Rolle spielt das motivationsrelevante Erleben von Schülern für ihr situationales Interesse im Mathematikunterricht? (What role does students’ motivational experience play in their situational interest in mathematics classrooms?). Befunde aus der SIGMA-Studie” in Erziehungswissenschaftliche Forschung – nachhaltige Bildung. Beiträge zur 5. DGfE-Sektionstagung “Empirische Bildungsforschung”/AEPF-KBBB im Frühjahr 2009 . eds. B. Schwarz, P. Nenninger, and R. S. Jäger (Landau: Verlag Empirische Pädagogik), 288–294.
Keywords: digital media, worked examples, scientific observation, motivation, cognitive load
Citation: Lechner M, Moser S, Pander J, Geist J and Lewalter D (2024) Learning scientific observation with worked examples in a digital learning environment. Front. Educ . 9:1293516. doi: 10.3389/feduc.2024.1293516
Received: 13 September 2023; Accepted: 29 February 2024; Published: 18 March 2024.
Reviewed by:
Copyright © 2024 Lechner, Moser, Pander, Geist and Lewalter. This is an open-access article distributed under the terms of the Creative Commons Attribution License (CC BY) . The use, distribution or reproduction in other forums is permitted, provided the original author(s) and the copyright owner(s) are credited and that the original publication in this journal is cited, in accordance with accepted academic practice. No use, distribution or reproduction is permitted which does not comply with these terms.
*Correspondence: Miriam Lechner, [email protected]
- Bipolar Disorder
- Therapy Center
- When To See a Therapist
- Types of Therapy
- Best Online Therapy
- Best Couples Therapy
- Best Family Therapy
- Managing Stress
- Sleep and Dreaming
- Understanding Emotions
- Self-Improvement
- Healthy Relationships
- Student Resources
- Personality Types
- Verywell Mind Insights
- 2023 Verywell Mind 25
- Mental Health in the Classroom
- Editorial Process
- Meet Our Review Board
- Crisis Support
What Is the Psychology of Learning?
Learning in psychology is based on a person's experiences
Kendra Cherry, MS, is a psychosocial rehabilitation specialist, psychology educator, and author of the "Everything Psychology Book."
:max_bytes(150000):strip_icc():format(webp)/IMG_9791-89504ab694d54b66bbd72cb84ffb860e.jpg)
James Lacy, MLS, is a fact-checker and researcher.
:max_bytes(150000):strip_icc():format(webp)/James-Lacy-1000-73de2239670146618c03f8b77f02f84e.jpg)
The psychology of learning focuses on a range of topics related to how people learn and interact with their environments.
Are you preparing for a big test in your psychology of learning class? Or are you just interested in a review of learning and behavioral psychology topics? This learning study guide offers a brief overview of some of the major learning issues including behaviorism, classical, and operant conditioning .
Let's learn a bit more about the psychology of learning.
Definition of Learning in Psychology
Learning can be defined in many ways, but most psychologists would agree that it is a relatively permanent change in behavior that results from experience. During the first half of the 20th century, the school of thought known as behaviorism rose to dominate psychology and sought to explain the learning process. Behaviorism sought to measure only observable behaviors.
3 Types of Learning in Psychology
Behavioral learning falls into three general categories.
Classical Conditioning
Classical conditioning is a learning process in which an association is made between a previously neutral stimulus and a stimulus that naturally evokes a response.
For example, in Pavlov's classic experiment , the smell of food was the naturally occurring stimulus that was paired with the previously neutral ringing of the bell. Once an association had been made between the two, the sound of the bell alone could lead to a response.
For example, if you don't know how to swim and were to fall into a pool, you'd take actions to avoid the pool.
Operant Conditioning
Operant conditioning is a learning process in which the probability of a response occurring is increased or decreased due to reinforcement or punishment. First studied by Edward Thorndike and later by B.F. Skinner , the underlying idea behind operant conditioning is that the consequences of our actions shape voluntary behavior.
Skinner described how reinforcement could lead to increases in behaviors where punishment would result in decreases. He also found that the timing of when reinforcements were delivered influenced how quickly a behavior was learned and how strong the response would be. The timing and rate of reinforcement are known as schedules of reinforcement .
For example, your child might learn to complete their homework because you reward them with treats and/or praise.
Observational Learning
Observational learning is a process in which learning occurs through observing and imitating others. Albert Bandura's social learning theory suggests that in addition to learning through conditioning, people also learn through observing and imitating the actions of others.
Basic Principles of Social Learning Theory
As demonstrated in his classic Bobo Doll experiments, people will imitate the actions of others without direct reinforcement. Four important elements are essential for effective observational learning: attention, motor skills, motivation, and memory.
For example, a teen's older sibling gets a speeding ticket, with the unpleasant results of fines and restrictions. The teen then learns not to speed when they take up driving.
The three types of learning in psychology are classical conditioning, operant conditioning, and observational learning.
History of the Psychology of Learning
One of the first thinkers to study how learning influences behavior was psychologist John B. Watson , who suggested in his seminal 1913 paper Psychology as the Behaviorist Views It that all behaviors are a result of the learning process. Psychology, the behaviorists believed, should be the scientific study of observable, measurable behavior. Watson's work included the famous Little Albert experiment in which he conditioned a small child to fear a white rat.
Behaviorism dominated psychology for much of the early 20th century. Although behavioral approaches remain important today, the latter part of the century was marked by the emergence of humanistic psychology, biological psychology, and cognitive psychology .
Other important figures in the psychology of learning include:
- Edward Thorndike
- Ivan Pavlov
- B.F. Skinner
- Albert Bandura
A Word From Verywell
The psychology of learning encompasses a vast body of research that generally focuses on classical conditioning, operant conditioning, and observational learning. As the field evolves, it continues to have important implications for explaining and motivating human behavior.
By Kendra Cherry, MSEd Kendra Cherry, MS, is a psychosocial rehabilitation specialist, psychology educator, and author of the "Everything Psychology Book."

Teacher effectiveness for students with and without disabilities
Research has often focused on how teachers and educators can best instruct and accommodate students with disabilities. However, are the methods used to teach students with disabilities effective and inclusive for all students? Michigan State University researchers are some of the first to answer that question.
Faculty and doctoral students from across MSU, including from the College of Social Science and the College of Education, offer some of the first findings on differentiating the effectiveness of instruction for students with and without disabilities.
Their study suggests that to help schools make decisions that are best for student outcomes, policymakers may want to consider teacher quality measures that look separately at these student groups.
Published in the journal Educational Evaluation and Policy Analysis, this research presents a major breakthrough in understanding how to best measure achievement for both students with and without disabilities.
"Most students with disabilities spend most of their school day in general education classrooms, but many teachers indicate they receive insufficient training and preparedness to educate these students," said Scott Imberman, study author and professor in the Department of Economics in the College of Social Science and the College of Education. "We thought that through the use of statistical measures of teacher quality, we could identify which teachers are more effective teachers with these students and how much general education teachers' ability to instruct these students varies."
It's important that students with disabilities have access to high-quality teachers, and not all teachers receive the necessary training and skills to support those students. They also can struggle more with certain subjects, such as math. Student success outcomes are also often determined by how the entire class performs rather than how individual students perform.
When it comes to evaluating the success of all students, numerical measures known as value-added measures, or VAM, are typically used. However, these measures often do not distinguish between evaluating students with and without disabilities.
The MSU research team created a study using data from the Los Angeles Unified School District due to the large number of enrollees and students with disabilities. They created two specific value-added measures -- one for evaluating the effectiveness for teachers instructing students with disabilities and the other for students without disabilities.
They found that some of the best-performing teachers for students without disabilities have lower value-added scores for students with disabilities. Similarly, they noted that top-performing teachers for students with disabilities have lower value-added scores for students without disabilities. This means that some students who may appear to be matched with a high-quality teacher could actually be better off with other teachers.
The bigger inequity, according to Imberman, is that although "some general education teachers do have specialized skills that make them more effective for students with disabilities, our case study in Los Angeles suggested disabled students are typically not matched to these teachers."
While the results do not identify how to better match teachers with students with disabilities, they do raise the point to schools and policymakers to explore how both groups of students and, especially those with disabilities, can have better academic gains. It is also necessary that educators, especially those who have been teaching longer, receive the appropriate training to support students with disabilities.
"We hope that our methods can be used in the future to help school officials better match students with disabilities to the teachers who are best equipped to instruct them and better assess which teachers might need additional training in educating disabled students," Imberman said.
In addition to Imberman, the research team included Katharine Strunk, dean of the University of Pennsylvania's Graduate School of Education; Nathan Jones, associate professor in the Special Education program at Boston University Wheelock College of Education and Human Development; W. Jesse Wood, senior analyst at Abt Associates; Neil Filosa, doctoral student in the MSU Department of Economics; and Ijun Lai, researcher with Mathematica.
- Educational Psychology
- K-12 Education
- Learning Disorders
- Educational Policy
- Education and Employment
- STEM Education
- Poverty and Learning
- Special education
- Intellectual giftedness
- Developmental disability
- Learning disability
- Collaboration
- Early childhood education
- Hyperactivity
- Asperger syndrome
Story Source:
Materials provided by Michigan State University . Note: Content may be edited for style and length.
Journal Reference :
- W. Jesse Wood, Ijun Lai, Neil R. Filosa, Scott A. Imberman, Nathan D. Jones, Katharine O. Strunk. Are Effective Teachers for Students With Disabilities Effective Teachers for All? Educational Evaluation and Policy Analysis , 2023; DOI: 10.3102/01623737231214555
Cite This Page :
Explore More
- Could Tardigrade Proteins Slow Aging in Humans?
- Supermassive Black Holes Awakened
- Beethoven's Genes: Low Beat Synchronization
- Common Household Chemicals Threat to Brain?
- Tiniest 'Starquake' Ever Detected
- Amazing Archive of Ancient Human Brains
- Night-Time Light and Stroke Risk
- Toward Secure Quantum Communication Globally
- Artificial Nanofluidic Synapses: Memory
- 49 New Galaxies Discovered in Under Three Hours
Trending Topics
Strange & offbeat.
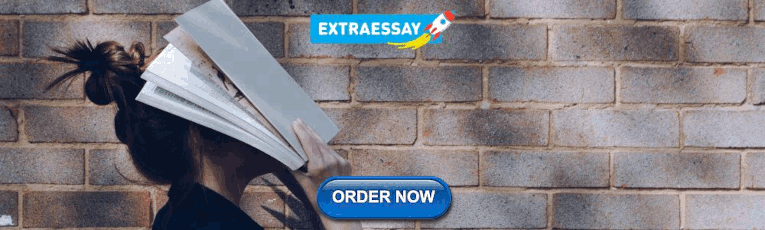
IMAGES
VIDEO
COMMENTS
people learn comes from research in many different disciplines. This chapter of the Teaching Guide introduces three central learning theories, as well as relevant research from the fields of neuroscience, anthropology, cognitive science, psychology, and education. In This Section Overview of Learning Theories Behaviorism Cognitive Constructivism
The term learning styles refers to the view that different people learn information in different ways. In recent decades, the concept of learning styles has steadily gained influence. In this article, we describe the intense interest and discussion that the concept of learning styles has elicited among professional educators at all levels of the educational system.
Research Methods in Psychology AP A Han dbook s in Psychology VOLUME Research Designs: Quantitative, Qualitative, Neuropsychological, and Biological SECOND EDITION Harris Cooper, Editor-in-Chief Marc N. Coutanche, Linda M. McMullen, A. T. Panter, sychological Association. Not for further distribution.
Some of the main theories of learning include: Behavioral learning theory. Cognitive learning theory. Constructivist learning theory. Social learning theory. Experiential learning theory. This article explores these learning theories, including how each one explains the learning process.
Psychology research can usually be classified as one of three major types. 1. Causal or Experimental Research. When most people think of scientific experimentation, research on cause and effect is most often brought to mind. Experiments on causal relationships investigate the effect of one or more variables on one or more outcome variables.
Olivia Guy-Evans, MSc. Research methods in psychology are systematic procedures used to observe, describe, predict, and explain behavior and mental processes. They include experiments, surveys, case studies, and naturalistic observations, ensuring data collection is objective and reliable to understand and explain psychological phenomena.
Stephany Duany Rea. Human Arenas (2023) Research on the psychology of learning has highlighted straightforward ways of enhancing learning. However, effective learning strategies are underused by ...
In the case of operant conditioning, behavior is modified by its consequence. Human beings constantly react and adapt to their environment by learning through conditioning, frequently ...
The Scientific Method in Psychology Research. The steps of the scientific method in psychology research are: Make an observation. Ask a research question and make predictions about what you expect to find. Test your hypothesis and gather data. Examine the results and form conclusions. Report your findings.
The psychology of learning refers broadly to theory and research derived from different types of learning, including classical conditioning, operant conditioning, and observational learning (modeling). Applied behavior analysis draws primarily on operant conditioning as the basis for developing interventions.
Introduction. Psychology is an ever-growing and popular field (Gough and Lyons, 2016; Clay, 2017).Due to this growth and the need for science-based research to base health decisions on (Perestelo-Pérez, 2013), the use of research methods in the broad field of psychology is an essential point of investigation (Stangor, 2011; Aanstoos, 2014).Research methods are therefore viewed as important ...
The five main methods of psychological research are: Experimental research: This method involves manipulating one or more independent variables to observe their effect on one or more dependent variables while controlling for other variables. The goal is to establish cause-and-effect relationships between variables.
These three fields—learning, instruction, and assessment—come together in educational psychology: the scientific study of how the instructional environment and the characteristics of the learner interact to produce cognitive growth in the learner. Fig. 2. Three components of educational psychology. Full size image.
LEARNING STYLES. A benchmark definition of "learning styles" is "characteristic cognitive, effective, and psychosocial behaviors that serve as relatively stable indicators of how learners perceive, interact with, and respond to the learning environment. 10 Learning styles are considered by many to be one factor of success in higher education. . Confounding research and, in many instances ...
Psychological research encompasses a variety of types, each serving a unique purpose and contributing to our understanding of human behavior and mental processes. Drawing from "Unit-4" of our reference PDF, this blog aims to explore the different types of research in psychology, highlighting their distinct characteristics and applications.
Figure 4. Ivan Pavlov's research on the digestive system of dogs unexpectedly led to his discovery of the learning process now known as classical conditioning. Pavlov came to his conclusions about how learning occurs completely by accident. Pavlov was a physiologist, not a psychologist.
Learning Objectives By the end of this section, you will be able to: Describe the different research methods used by psychologists; Discuss the strengths and weaknesses of case studies, naturalistic observation, surveys, and archival research; Compare longitudinal and cross-sectional approaches to research
The different types of theories all play important roles in psychological research. Exercises Practice: Find an empirical research report in a professional journal, identify a theory that the researchers present, and then describe the theory in terms of its formality (informal vs. formal), scope (broad vs. narrow), and theoretical approach ...
Psychologists typically use different research methods to conduct experiments. Their research involves studying subjects, making observations, and reaching conclusions. Learning more about different research methods in the field of psychology can help you choose a suitable option for your experiments and make you a better researcher.
Qualitative Research. Non-numerical evidence, usually examined in its raw form. Used when a researcher wants to understand people's opinions, idiosyncratic responses to an event, motivations, or underlying reasons for actions or decisions. Learn about the Types of Qualitative Research Methods. link will open in a new window
Understanding the four main branches of psychology—clinical, cognitive, developmental, and social—provides valuable insights into human behavior and mental processes. The foundational knowledge gained from a bachelor's degree program in psychology lays the groundwork for a career in psychology or other industries.
The research, described in the March 12 online edition of Proceedings of the National Academy of Sciences (PNAS), suggests that varying what we study and spacing out our learning over time can ...
Random assignment is a method for assigning participants in a sample to the different conditions, and it is an important element of all experimental research in psychology and other fields too. In its strictest sense, random assignment should meet two criteria. One is that each participant has an equal chance of being assigned to each condition ...
Science education often aims to increase learners' acquisition of fundamental principles, such as learning the basic steps of scientific methods. Worked examples (WE) have proven particularly useful for supporting the development of such cognitive schemas and successive actions in order to avoid using up more cognitive resources than are necessary. Therefore, we investigated the extent to ...
The psychology of learning encompasses a vast body of research that generally focuses on classical conditioning, operant conditioning, and observational learning. As the field evolves, it continues to have important implications for explaining and motivating human behavior. By Kendra Cherry, MSEd. Kendra Cherry, MS, is a psychosocial ...
Emotional change is an emerging common factor for explaining outcome in psychotherapy. Its measurement is becoming increasingly important for advancing and integrating psychotherapy research. The aim in the special series of 2023 Journal of Psychotherapy Integration was to provide an integrative roadmap for psychotherapy researchers to advance the field of emotional change: The authors believe ...
Although previous research has demonstrated that the acquisition of mathematical skills requires support from multiple cognitive abilities, the associations between cognitive precursors in different domains and mathematics at different hierarchical levels among primary school children are not well understood. This study explores the cognitive mechanisms underlying primary school children's ...
Educational Evaluation and Policy Analysis, 2023; DOI: 10.3102/01623737231214555. Michigan State University. "Teacher effectiveness for students with and without disabilities." ScienceDaily ...
On Friday, more than 80 biologists and A.I. experts signed a call for the technology to be regulated so that it cannot be used to create new biological weapons. Such a concern might apply to new ...
Alternative Writing Assignment - PART 1 Research Strategies: Naturalistic Observation Methods - For this strategy, the person who is conducting research creates observations of individuals in their own natural environments. When it comes to different types of organizational and social settings, this method is commonly used, and it also has similar sources coming from anthropology and the study ...