The Four Types of Research Design — Everything You Need to Know

Updated: December 11, 2023
Published: January 18, 2023
When you conduct research, you need to have a clear idea of what you want to achieve and how to accomplish it. A good research design enables you to collect accurate and reliable data to draw valid conclusions.
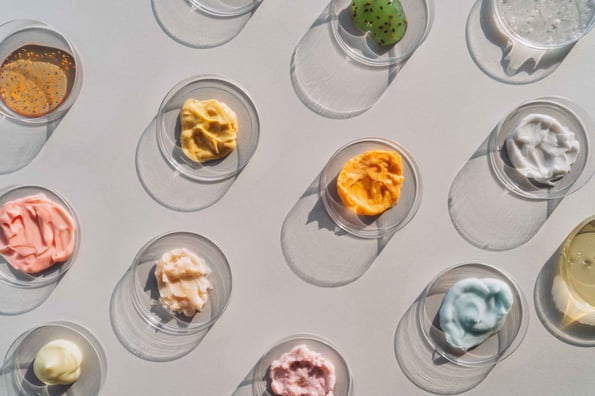
In this blog post, we'll outline the key features of the four common types of research design with real-life examples from UnderArmor, Carmex, and more. Then, you can easily choose the right approach for your project.
Table of Contents
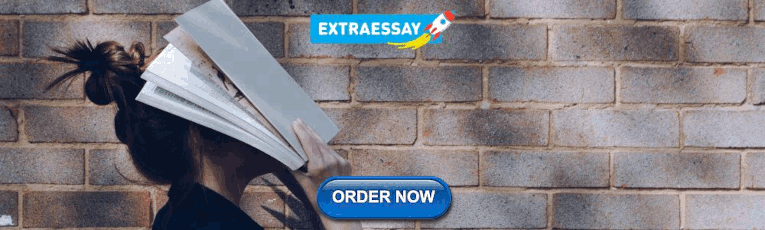
What is research design?
The four types of research design, research design examples.
Research design is the process of planning and executing a study to answer specific questions. This process allows you to test hypotheses in the business or scientific fields.
Research design involves choosing the right methodology, selecting the most appropriate data collection methods, and devising a plan (or framework) for analyzing the data. In short, a good research design helps us to structure our research.
Marketers use different types of research design when conducting research .
There are four common types of research design — descriptive, correlational, experimental, and diagnostic designs. Let’s take a look at each in more detail.
Researchers use different designs to accomplish different research objectives. Here, we'll discuss how to choose the right type, the benefits of each, and use cases.
Research can also be classified as quantitative or qualitative at a higher level. Some experiments exhibit both qualitative and quantitative characteristics.
.png)
Free Market Research Kit
5 Research and Planning Templates + a Free Guide on How to Use Them in Your Market Research
- SWOT Analysis Template
- Survey Template
- Focus Group Template
You're all set!
Click this link to access this resource at any time.
Experimental
An experimental design is used when the researcher wants to examine how variables interact with each other. The researcher manipulates one variable (the independent variable) and observes the effect on another variable (the dependent variable).
In other words, the researcher wants to test a causal relationship between two or more variables.
In marketing, an example of experimental research would be comparing the effects of a television commercial versus an online advertisement conducted in a controlled environment (e.g. a lab). The objective of the research is to test which advertisement gets more attention among people of different age groups, gender, etc.
Another example is a study of the effect of music on productivity. A researcher assigns participants to one of two groups — those who listen to music while working and those who don't — and measure their productivity.
The main benefit of an experimental design is that it allows the researcher to draw causal relationships between variables.
One limitation: This research requires a great deal of control over the environment and participants, making it difficult to replicate in the real world. In addition, it’s quite costly.
Best for: Testing a cause-and-effect relationship (i.e., the effect of an independent variable on a dependent variable).
Correlational
A correlational design examines the relationship between two or more variables without intervening in the process.
Correlational design allows the analyst to observe natural relationships between variables. This results in data being more reflective of real-world situations.
For example, marketers can use correlational design to examine the relationship between brand loyalty and customer satisfaction. In particular, the researcher would look for patterns or trends in the data to see if there is a relationship between these two entities.
Similarly, you can study the relationship between physical activity and mental health. The analyst here would ask participants to complete surveys about their physical activity levels and mental health status. Data would show how the two variables are related.
Best for: Understanding the extent to which two or more variables are associated with each other in the real world.
Descriptive
Descriptive research refers to a systematic process of observing and describing what a subject does without influencing them.
Methods include surveys, interviews, case studies, and observations. Descriptive research aims to gather an in-depth understanding of a phenomenon and answers when/what/where.
SaaS companies use descriptive design to understand how customers interact with specific features. Findings can be used to spot patterns and roadblocks.
For instance, product managers can use screen recordings by Hotjar to observe in-app user behavior. This way, the team can precisely understand what is happening at a certain stage of the user journey and act accordingly.
Brand24, a social listening tool, tripled its sign-up conversion rate from 2.56% to 7.42%, thanks to locating friction points in the sign-up form through screen recordings.
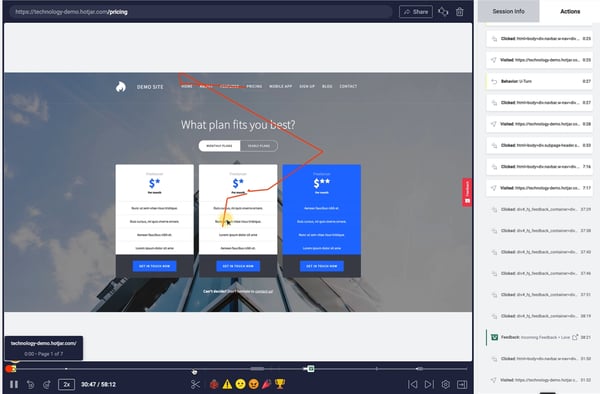
Carma Laboratories worked with research company MMR to measure customers’ reactions to the lip-care company’s packaging and product . The goal was to find the cause of low sales for a recently launched line extension in Europe.
The team moderated a live, online focus group. Participants were shown w product samples, while AI and NLP natural language processing identified key themes in customer feedback.
This helped uncover key reasons for poor performance and guided changes in packaging.
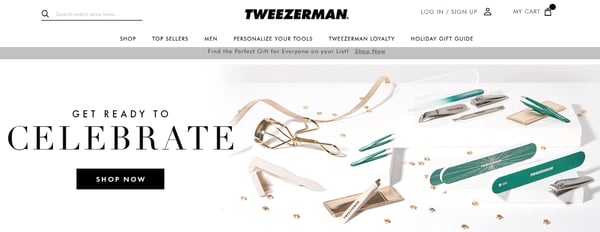
Research Design 101
Everything You Need To Get Started (With Examples)
By: Derek Jansen (MBA) | Reviewers: Eunice Rautenbach (DTech) & Kerryn Warren (PhD) | April 2023
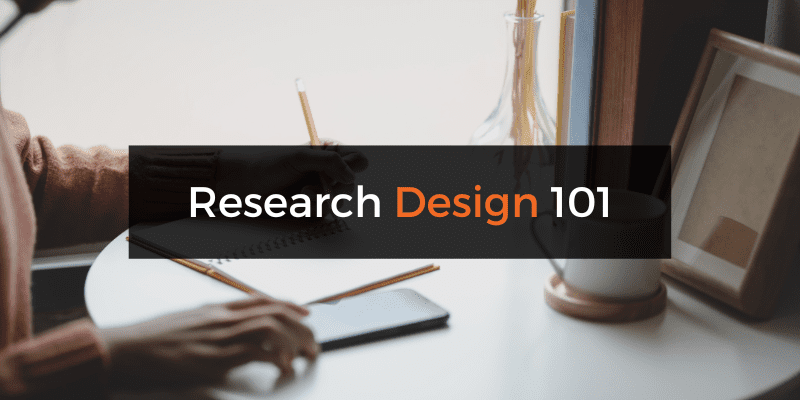
Navigating the world of research can be daunting, especially if you’re a first-time researcher. One concept you’re bound to run into fairly early in your research journey is that of “ research design ”. Here, we’ll guide you through the basics using practical examples , so that you can approach your research with confidence.
Overview: Research Design 101
What is research design.
- Research design types for quantitative studies
- Video explainer : quantitative research design
- Research design types for qualitative studies
- Video explainer : qualitative research design
- How to choose a research design
- Key takeaways
Research design refers to the overall plan, structure or strategy that guides a research project , from its conception to the final data analysis. A good research design serves as the blueprint for how you, as the researcher, will collect and analyse data while ensuring consistency, reliability and validity throughout your study.
Understanding different types of research designs is essential as helps ensure that your approach is suitable given your research aims, objectives and questions , as well as the resources you have available to you. Without a clear big-picture view of how you’ll design your research, you run the risk of potentially making misaligned choices in terms of your methodology – especially your sampling , data collection and data analysis decisions.
The problem with defining research design…
One of the reasons students struggle with a clear definition of research design is because the term is used very loosely across the internet, and even within academia.
Some sources claim that the three research design types are qualitative, quantitative and mixed methods , which isn’t quite accurate (these just refer to the type of data that you’ll collect and analyse). Other sources state that research design refers to the sum of all your design choices, suggesting it’s more like a research methodology . Others run off on other less common tangents. No wonder there’s confusion!
In this article, we’ll clear up the confusion. We’ll explain the most common research design types for both qualitative and quantitative research projects, whether that is for a full dissertation or thesis, or a smaller research paper or article.
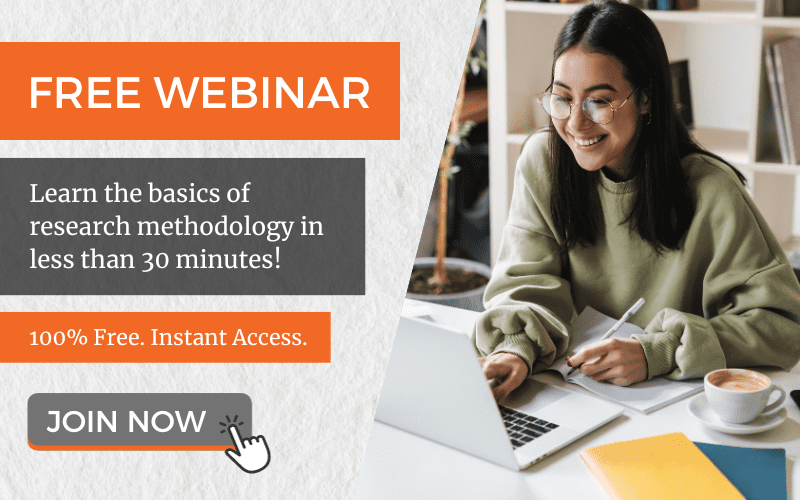
Research Design: Quantitative Studies
Quantitative research involves collecting and analysing data in a numerical form. Broadly speaking, there are four types of quantitative research designs: descriptive , correlational , experimental , and quasi-experimental .
Descriptive Research Design
As the name suggests, descriptive research design focuses on describing existing conditions, behaviours, or characteristics by systematically gathering information without manipulating any variables. In other words, there is no intervention on the researcher’s part – only data collection.
For example, if you’re studying smartphone addiction among adolescents in your community, you could deploy a survey to a sample of teens asking them to rate their agreement with certain statements that relate to smartphone addiction. The collected data would then provide insight regarding how widespread the issue may be – in other words, it would describe the situation.
The key defining attribute of this type of research design is that it purely describes the situation . In other words, descriptive research design does not explore potential relationships between different variables or the causes that may underlie those relationships. Therefore, descriptive research is useful for generating insight into a research problem by describing its characteristics . By doing so, it can provide valuable insights and is often used as a precursor to other research design types.
Correlational Research Design
Correlational design is a popular choice for researchers aiming to identify and measure the relationship between two or more variables without manipulating them . In other words, this type of research design is useful when you want to know whether a change in one thing tends to be accompanied by a change in another thing.
For example, if you wanted to explore the relationship between exercise frequency and overall health, you could use a correlational design to help you achieve this. In this case, you might gather data on participants’ exercise habits, as well as records of their health indicators like blood pressure, heart rate, or body mass index. Thereafter, you’d use a statistical test to assess whether there’s a relationship between the two variables (exercise frequency and health).
As you can see, correlational research design is useful when you want to explore potential relationships between variables that cannot be manipulated or controlled for ethical, practical, or logistical reasons. It is particularly helpful in terms of developing predictions , and given that it doesn’t involve the manipulation of variables, it can be implemented at a large scale more easily than experimental designs (which will look at next).
That said, it’s important to keep in mind that correlational research design has limitations – most notably that it cannot be used to establish causality . In other words, correlation does not equal causation . To establish causality, you’ll need to move into the realm of experimental design, coming up next…
Need a helping hand?
Experimental Research Design
Experimental research design is used to determine if there is a causal relationship between two or more variables . With this type of research design, you, as the researcher, manipulate one variable (the independent variable) while controlling others (dependent variables). Doing so allows you to observe the effect of the former on the latter and draw conclusions about potential causality.
For example, if you wanted to measure if/how different types of fertiliser affect plant growth, you could set up several groups of plants, with each group receiving a different type of fertiliser, as well as one with no fertiliser at all. You could then measure how much each plant group grew (on average) over time and compare the results from the different groups to see which fertiliser was most effective.
Overall, experimental research design provides researchers with a powerful way to identify and measure causal relationships (and the direction of causality) between variables. However, developing a rigorous experimental design can be challenging as it’s not always easy to control all the variables in a study. This often results in smaller sample sizes , which can reduce the statistical power and generalisability of the results.
Moreover, experimental research design requires random assignment . This means that the researcher needs to assign participants to different groups or conditions in a way that each participant has an equal chance of being assigned to any group (note that this is not the same as random sampling ). Doing so helps reduce the potential for bias and confounding variables . This need for random assignment can lead to ethics-related issues . For example, withholding a potentially beneficial medical treatment from a control group may be considered unethical in certain situations.
Quasi-Experimental Research Design
Quasi-experimental research design is used when the research aims involve identifying causal relations , but one cannot (or doesn’t want to) randomly assign participants to different groups (for practical or ethical reasons). Instead, with a quasi-experimental research design, the researcher relies on existing groups or pre-existing conditions to form groups for comparison.
For example, if you were studying the effects of a new teaching method on student achievement in a particular school district, you may be unable to randomly assign students to either group and instead have to choose classes or schools that already use different teaching methods. This way, you still achieve separate groups, without having to assign participants to specific groups yourself.
Naturally, quasi-experimental research designs have limitations when compared to experimental designs. Given that participant assignment is not random, it’s more difficult to confidently establish causality between variables, and, as a researcher, you have less control over other variables that may impact findings.
All that said, quasi-experimental designs can still be valuable in research contexts where random assignment is not possible and can often be undertaken on a much larger scale than experimental research, thus increasing the statistical power of the results. What’s important is that you, as the researcher, understand the limitations of the design and conduct your quasi-experiment as rigorously as possible, paying careful attention to any potential confounding variables .
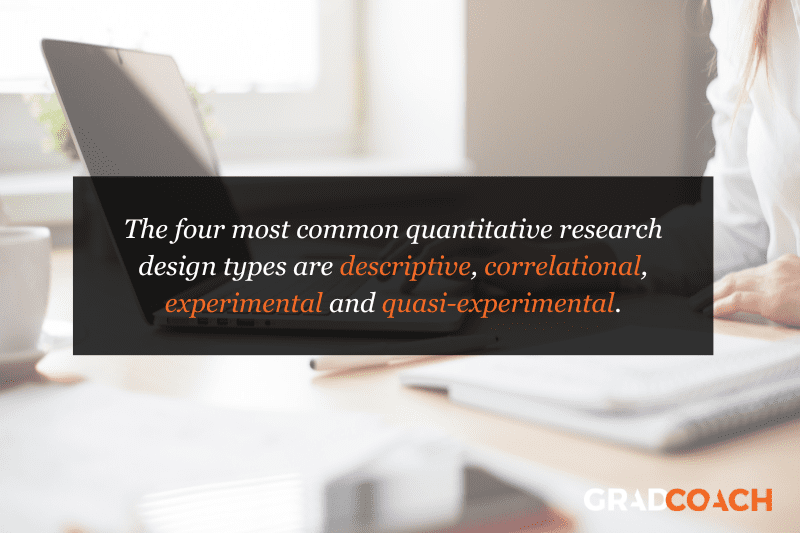
Research Design: Qualitative Studies
There are many different research design types when it comes to qualitative studies, but here we’ll narrow our focus to explore the “Big 4”. Specifically, we’ll look at phenomenological design, grounded theory design, ethnographic design, and case study design.
Phenomenological Research Design
Phenomenological design involves exploring the meaning of lived experiences and how they are perceived by individuals. This type of research design seeks to understand people’s perspectives , emotions, and behaviours in specific situations. Here, the aim for researchers is to uncover the essence of human experience without making any assumptions or imposing preconceived ideas on their subjects.
For example, you could adopt a phenomenological design to study why cancer survivors have such varied perceptions of their lives after overcoming their disease. This could be achieved by interviewing survivors and then analysing the data using a qualitative analysis method such as thematic analysis to identify commonalities and differences.
Phenomenological research design typically involves in-depth interviews or open-ended questionnaires to collect rich, detailed data about participants’ subjective experiences. This richness is one of the key strengths of phenomenological research design but, naturally, it also has limitations. These include potential biases in data collection and interpretation and the lack of generalisability of findings to broader populations.
Grounded Theory Research Design
Grounded theory (also referred to as “GT”) aims to develop theories by continuously and iteratively analysing and comparing data collected from a relatively large number of participants in a study. It takes an inductive (bottom-up) approach, with a focus on letting the data “speak for itself”, without being influenced by preexisting theories or the researcher’s preconceptions.
As an example, let’s assume your research aims involved understanding how people cope with chronic pain from a specific medical condition, with a view to developing a theory around this. In this case, grounded theory design would allow you to explore this concept thoroughly without preconceptions about what coping mechanisms might exist. You may find that some patients prefer cognitive-behavioural therapy (CBT) while others prefer to rely on herbal remedies. Based on multiple, iterative rounds of analysis, you could then develop a theory in this regard, derived directly from the data (as opposed to other preexisting theories and models).
Grounded theory typically involves collecting data through interviews or observations and then analysing it to identify patterns and themes that emerge from the data. These emerging ideas are then validated by collecting more data until a saturation point is reached (i.e., no new information can be squeezed from the data). From that base, a theory can then be developed .
As you can see, grounded theory is ideally suited to studies where the research aims involve theory generation , especially in under-researched areas. Keep in mind though that this type of research design can be quite time-intensive , given the need for multiple rounds of data collection and analysis.
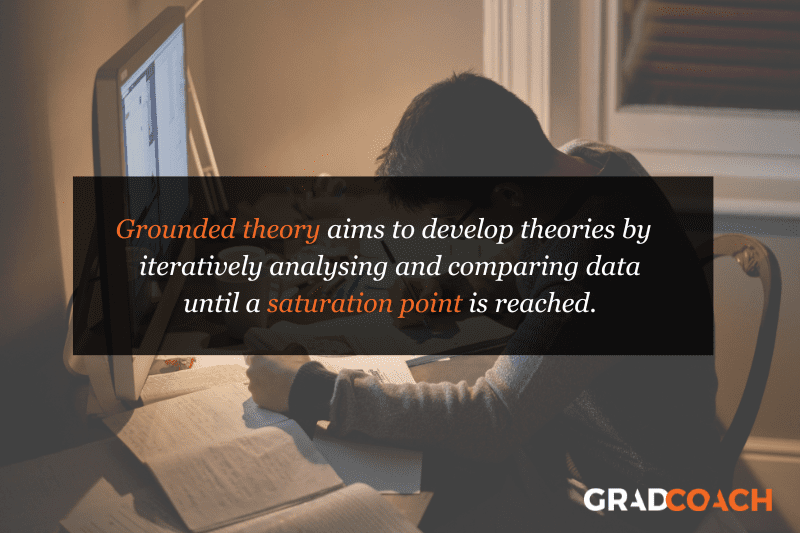
Ethnographic Research Design
Ethnographic design involves observing and studying a culture-sharing group of people in their natural setting to gain insight into their behaviours, beliefs, and values. The focus here is on observing participants in their natural environment (as opposed to a controlled environment). This typically involves the researcher spending an extended period of time with the participants in their environment, carefully observing and taking field notes .
All of this is not to say that ethnographic research design relies purely on observation. On the contrary, this design typically also involves in-depth interviews to explore participants’ views, beliefs, etc. However, unobtrusive observation is a core component of the ethnographic approach.
As an example, an ethnographer may study how different communities celebrate traditional festivals or how individuals from different generations interact with technology differently. This may involve a lengthy period of observation, combined with in-depth interviews to further explore specific areas of interest that emerge as a result of the observations that the researcher has made.
As you can probably imagine, ethnographic research design has the ability to provide rich, contextually embedded insights into the socio-cultural dynamics of human behaviour within a natural, uncontrived setting. Naturally, however, it does come with its own set of challenges, including researcher bias (since the researcher can become quite immersed in the group), participant confidentiality and, predictably, ethical complexities . All of these need to be carefully managed if you choose to adopt this type of research design.
Case Study Design
With case study research design, you, as the researcher, investigate a single individual (or a single group of individuals) to gain an in-depth understanding of their experiences, behaviours or outcomes. Unlike other research designs that are aimed at larger sample sizes, case studies offer a deep dive into the specific circumstances surrounding a person, group of people, event or phenomenon, generally within a bounded setting or context .
As an example, a case study design could be used to explore the factors influencing the success of a specific small business. This would involve diving deeply into the organisation to explore and understand what makes it tick – from marketing to HR to finance. In terms of data collection, this could include interviews with staff and management, review of policy documents and financial statements, surveying customers, etc.
While the above example is focused squarely on one organisation, it’s worth noting that case study research designs can have different variation s, including single-case, multiple-case and longitudinal designs. As you can see in the example, a single-case design involves intensely examining a single entity to understand its unique characteristics and complexities. Conversely, in a multiple-case design , multiple cases are compared and contrasted to identify patterns and commonalities. Lastly, in a longitudinal case design , a single case or multiple cases are studied over an extended period of time to understand how factors develop over time.
As you can see, a case study research design is particularly useful where a deep and contextualised understanding of a specific phenomenon or issue is desired. However, this strength is also its weakness. In other words, you can’t generalise the findings from a case study to the broader population. So, keep this in mind if you’re considering going the case study route.
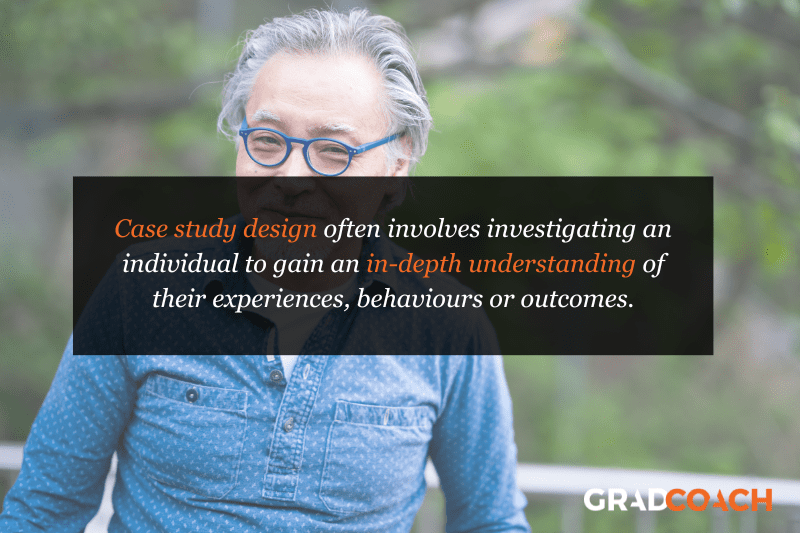
How To Choose A Research Design
Having worked through all of these potential research designs, you’d be forgiven for feeling a little overwhelmed and wondering, “ But how do I decide which research design to use? ”. While we could write an entire post covering that alone, here are a few factors to consider that will help you choose a suitable research design for your study.
Data type: The first determining factor is naturally the type of data you plan to be collecting – i.e., qualitative or quantitative. This may sound obvious, but we have to be clear about this – don’t try to use a quantitative research design on qualitative data (or vice versa)!
Research aim(s) and question(s): As with all methodological decisions, your research aim and research questions will heavily influence your research design. For example, if your research aims involve developing a theory from qualitative data, grounded theory would be a strong option. Similarly, if your research aims involve identifying and measuring relationships between variables, one of the experimental designs would likely be a better option.
Time: It’s essential that you consider any time constraints you have, as this will impact the type of research design you can choose. For example, if you’ve only got a month to complete your project, a lengthy design such as ethnography wouldn’t be a good fit.
Resources: Take into account the resources realistically available to you, as these need to factor into your research design choice. For example, if you require highly specialised lab equipment to execute an experimental design, you need to be sure that you’ll have access to that before you make a decision.
Keep in mind that when it comes to research, it’s important to manage your risks and play as conservatively as possible. If your entire project relies on you achieving a huge sample, having access to niche equipment or holding interviews with very difficult-to-reach participants, you’re creating risks that could kill your project. So, be sure to think through your choices carefully and make sure that you have backup plans for any existential risks. Remember that a relatively simple methodology executed well generally will typically earn better marks than a highly-complex methodology executed poorly.
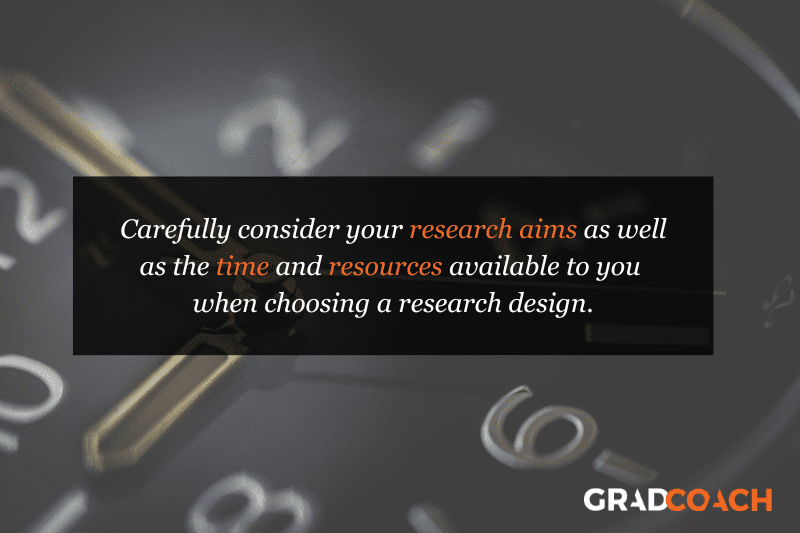
Recap: Key Takeaways
We’ve covered a lot of ground here. Let’s recap by looking at the key takeaways:
- Research design refers to the overall plan, structure or strategy that guides a research project, from its conception to the final analysis of data.
- Research designs for quantitative studies include descriptive , correlational , experimental and quasi-experimenta l designs.
- Research designs for qualitative studies include phenomenological , grounded theory , ethnographic and case study designs.
- When choosing a research design, you need to consider a variety of factors, including the type of data you’ll be working with, your research aims and questions, your time and the resources available to you.
If you need a helping hand with your research design (or any other aspect of your research), check out our private coaching services .
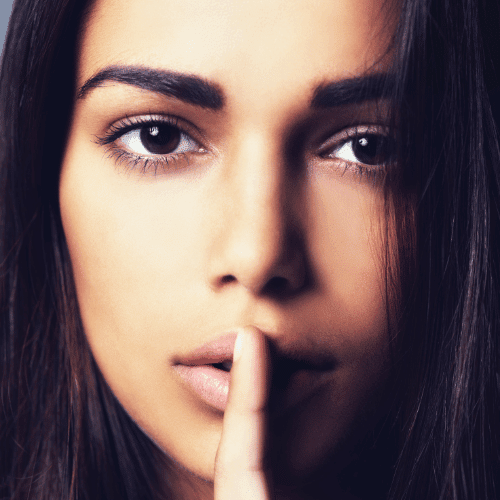
Psst... there’s more!
This post was based on one of our popular Research Bootcamps . If you're working on a research project, you'll definitely want to check this out ...
You Might Also Like:
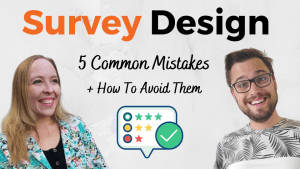
10 Comments
Is there any blog article explaining more on Case study research design? Is there a Case study write-up template? Thank you.
Thanks this was quite valuable to clarify such an important concept.
Thanks for this simplified explanations. it is quite very helpful.
This was really helpful. thanks
Thank you for your explanation. I think case study research design and the use of secondary data in researches needs to be talked about more in your videos and articles because there a lot of case studies research design tailored projects out there.
Please is there any template for a case study research design whose data type is a secondary data on your repository?
This post is very clear, comprehensive and has been very helpful to me. It has cleared the confusion I had in regard to research design and methodology.
This post is helpful, easy to understand, and deconstructs what a research design is. Thanks
how to cite this page
Thank you very much for the post. It is wonderful and has cleared many worries in my mind regarding research designs. I really appreciate .
how can I put this blog as my reference(APA style) in bibliography part?
Submit a Comment Cancel reply
Your email address will not be published. Required fields are marked *
Save my name, email, and website in this browser for the next time I comment.
- Print Friendly
Have a language expert improve your writing
Run a free plagiarism check in 10 minutes, automatically generate references for free.
- Knowledge Base
- Methodology
Research Design | Step-by-Step Guide with Examples
Published on 5 May 2022 by Shona McCombes . Revised on 20 March 2023.
A research design is a strategy for answering your research question using empirical data. Creating a research design means making decisions about:
- Your overall aims and approach
- The type of research design you’ll use
- Your sampling methods or criteria for selecting subjects
- Your data collection methods
- The procedures you’ll follow to collect data
- Your data analysis methods
A well-planned research design helps ensure that your methods match your research aims and that you use the right kind of analysis for your data.
Table of contents
Step 1: consider your aims and approach, step 2: choose a type of research design, step 3: identify your population and sampling method, step 4: choose your data collection methods, step 5: plan your data collection procedures, step 6: decide on your data analysis strategies, frequently asked questions.
- Introduction
Before you can start designing your research, you should already have a clear idea of the research question you want to investigate.
There are many different ways you could go about answering this question. Your research design choices should be driven by your aims and priorities – start by thinking carefully about what you want to achieve.
The first choice you need to make is whether you’ll take a qualitative or quantitative approach.
Qualitative research designs tend to be more flexible and inductive , allowing you to adjust your approach based on what you find throughout the research process.
Quantitative research designs tend to be more fixed and deductive , with variables and hypotheses clearly defined in advance of data collection.
It’s also possible to use a mixed methods design that integrates aspects of both approaches. By combining qualitative and quantitative insights, you can gain a more complete picture of the problem you’re studying and strengthen the credibility of your conclusions.
Practical and ethical considerations when designing research
As well as scientific considerations, you need to think practically when designing your research. If your research involves people or animals, you also need to consider research ethics .
- How much time do you have to collect data and write up the research?
- Will you be able to gain access to the data you need (e.g., by travelling to a specific location or contacting specific people)?
- Do you have the necessary research skills (e.g., statistical analysis or interview techniques)?
- Will you need ethical approval ?
At each stage of the research design process, make sure that your choices are practically feasible.
Prevent plagiarism, run a free check.
Within both qualitative and quantitative approaches, there are several types of research design to choose from. Each type provides a framework for the overall shape of your research.
Types of quantitative research designs
Quantitative designs can be split into four main types. Experimental and quasi-experimental designs allow you to test cause-and-effect relationships, while descriptive and correlational designs allow you to measure variables and describe relationships between them.
With descriptive and correlational designs, you can get a clear picture of characteristics, trends, and relationships as they exist in the real world. However, you can’t draw conclusions about cause and effect (because correlation doesn’t imply causation ).
Experiments are the strongest way to test cause-and-effect relationships without the risk of other variables influencing the results. However, their controlled conditions may not always reflect how things work in the real world. They’re often also more difficult and expensive to implement.
Types of qualitative research designs
Qualitative designs are less strictly defined. This approach is about gaining a rich, detailed understanding of a specific context or phenomenon, and you can often be more creative and flexible in designing your research.
The table below shows some common types of qualitative design. They often have similar approaches in terms of data collection, but focus on different aspects when analysing the data.
Your research design should clearly define who or what your research will focus on, and how you’ll go about choosing your participants or subjects.
In research, a population is the entire group that you want to draw conclusions about, while a sample is the smaller group of individuals you’ll actually collect data from.
Defining the population
A population can be made up of anything you want to study – plants, animals, organisations, texts, countries, etc. In the social sciences, it most often refers to a group of people.
For example, will you focus on people from a specific demographic, region, or background? Are you interested in people with a certain job or medical condition, or users of a particular product?
The more precisely you define your population, the easier it will be to gather a representative sample.
Sampling methods
Even with a narrowly defined population, it’s rarely possible to collect data from every individual. Instead, you’ll collect data from a sample.
To select a sample, there are two main approaches: probability sampling and non-probability sampling . The sampling method you use affects how confidently you can generalise your results to the population as a whole.
Probability sampling is the most statistically valid option, but it’s often difficult to achieve unless you’re dealing with a very small and accessible population.
For practical reasons, many studies use non-probability sampling, but it’s important to be aware of the limitations and carefully consider potential biases. You should always make an effort to gather a sample that’s as representative as possible of the population.
Case selection in qualitative research
In some types of qualitative designs, sampling may not be relevant.
For example, in an ethnography or a case study, your aim is to deeply understand a specific context, not to generalise to a population. Instead of sampling, you may simply aim to collect as much data as possible about the context you are studying.
In these types of design, you still have to carefully consider your choice of case or community. You should have a clear rationale for why this particular case is suitable for answering your research question.
For example, you might choose a case study that reveals an unusual or neglected aspect of your research problem, or you might choose several very similar or very different cases in order to compare them.
Data collection methods are ways of directly measuring variables and gathering information. They allow you to gain first-hand knowledge and original insights into your research problem.
You can choose just one data collection method, or use several methods in the same study.
Survey methods
Surveys allow you to collect data about opinions, behaviours, experiences, and characteristics by asking people directly. There are two main survey methods to choose from: questionnaires and interviews.
Observation methods
Observations allow you to collect data unobtrusively, observing characteristics, behaviours, or social interactions without relying on self-reporting.
Observations may be conducted in real time, taking notes as you observe, or you might make audiovisual recordings for later analysis. They can be qualitative or quantitative.
Other methods of data collection
There are many other ways you might collect data depending on your field and topic.
If you’re not sure which methods will work best for your research design, try reading some papers in your field to see what data collection methods they used.
Secondary data
If you don’t have the time or resources to collect data from the population you’re interested in, you can also choose to use secondary data that other researchers already collected – for example, datasets from government surveys or previous studies on your topic.
With this raw data, you can do your own analysis to answer new research questions that weren’t addressed by the original study.
Using secondary data can expand the scope of your research, as you may be able to access much larger and more varied samples than you could collect yourself.
However, it also means you don’t have any control over which variables to measure or how to measure them, so the conclusions you can draw may be limited.
As well as deciding on your methods, you need to plan exactly how you’ll use these methods to collect data that’s consistent, accurate, and unbiased.
Planning systematic procedures is especially important in quantitative research, where you need to precisely define your variables and ensure your measurements are reliable and valid.
Operationalisation
Some variables, like height or age, are easily measured. But often you’ll be dealing with more abstract concepts, like satisfaction, anxiety, or competence. Operationalisation means turning these fuzzy ideas into measurable indicators.
If you’re using observations , which events or actions will you count?
If you’re using surveys , which questions will you ask and what range of responses will be offered?
You may also choose to use or adapt existing materials designed to measure the concept you’re interested in – for example, questionnaires or inventories whose reliability and validity has already been established.
Reliability and validity
Reliability means your results can be consistently reproduced , while validity means that you’re actually measuring the concept you’re interested in.
For valid and reliable results, your measurement materials should be thoroughly researched and carefully designed. Plan your procedures to make sure you carry out the same steps in the same way for each participant.
If you’re developing a new questionnaire or other instrument to measure a specific concept, running a pilot study allows you to check its validity and reliability in advance.
Sampling procedures
As well as choosing an appropriate sampling method, you need a concrete plan for how you’ll actually contact and recruit your selected sample.
That means making decisions about things like:
- How many participants do you need for an adequate sample size?
- What inclusion and exclusion criteria will you use to identify eligible participants?
- How will you contact your sample – by mail, online, by phone, or in person?
If you’re using a probability sampling method, it’s important that everyone who is randomly selected actually participates in the study. How will you ensure a high response rate?
If you’re using a non-probability method, how will you avoid bias and ensure a representative sample?
Data management
It’s also important to create a data management plan for organising and storing your data.
Will you need to transcribe interviews or perform data entry for observations? You should anonymise and safeguard any sensitive data, and make sure it’s backed up regularly.
Keeping your data well organised will save time when it comes to analysing them. It can also help other researchers validate and add to your findings.
On their own, raw data can’t answer your research question. The last step of designing your research is planning how you’ll analyse the data.
Quantitative data analysis
In quantitative research, you’ll most likely use some form of statistical analysis . With statistics, you can summarise your sample data, make estimates, and test hypotheses.
Using descriptive statistics , you can summarise your sample data in terms of:
- The distribution of the data (e.g., the frequency of each score on a test)
- The central tendency of the data (e.g., the mean to describe the average score)
- The variability of the data (e.g., the standard deviation to describe how spread out the scores are)
The specific calculations you can do depend on the level of measurement of your variables.
Using inferential statistics , you can:
- Make estimates about the population based on your sample data.
- Test hypotheses about a relationship between variables.
Regression and correlation tests look for associations between two or more variables, while comparison tests (such as t tests and ANOVAs ) look for differences in the outcomes of different groups.
Your choice of statistical test depends on various aspects of your research design, including the types of variables you’re dealing with and the distribution of your data.
Qualitative data analysis
In qualitative research, your data will usually be very dense with information and ideas. Instead of summing it up in numbers, you’ll need to comb through the data in detail, interpret its meanings, identify patterns, and extract the parts that are most relevant to your research question.
Two of the most common approaches to doing this are thematic analysis and discourse analysis .
There are many other ways of analysing qualitative data depending on the aims of your research. To get a sense of potential approaches, try reading some qualitative research papers in your field.
A sample is a subset of individuals from a larger population. Sampling means selecting the group that you will actually collect data from in your research.
For example, if you are researching the opinions of students in your university, you could survey a sample of 100 students.
Statistical sampling allows you to test a hypothesis about the characteristics of a population. There are various sampling methods you can use to ensure that your sample is representative of the population as a whole.
Operationalisation means turning abstract conceptual ideas into measurable observations.
For example, the concept of social anxiety isn’t directly observable, but it can be operationally defined in terms of self-rating scores, behavioural avoidance of crowded places, or physical anxiety symptoms in social situations.
Before collecting data , it’s important to consider how you will operationalise the variables that you want to measure.
The research methods you use depend on the type of data you need to answer your research question .
- If you want to measure something or test a hypothesis , use quantitative methods . If you want to explore ideas, thoughts, and meanings, use qualitative methods .
- If you want to analyse a large amount of readily available data, use secondary data. If you want data specific to your purposes with control over how they are generated, collect primary data.
- If you want to establish cause-and-effect relationships between variables , use experimental methods. If you want to understand the characteristics of a research subject, use descriptive methods.
Cite this Scribbr article
If you want to cite this source, you can copy and paste the citation or click the ‘Cite this Scribbr article’ button to automatically add the citation to our free Reference Generator.
McCombes, S. (2023, March 20). Research Design | Step-by-Step Guide with Examples. Scribbr. Retrieved 14 May 2024, from https://www.scribbr.co.uk/research-methods/research-design/
Is this article helpful?
Shona McCombes
- Skip to main content
- Skip to primary sidebar
- Skip to footer
- QuestionPro

- Solutions Industries Gaming Automotive Sports and events Education Government Travel & Hospitality Financial Services Healthcare Cannabis Technology Use Case NPS+ Communities Audience Contactless surveys Mobile LivePolls Member Experience GDPR Positive People Science 360 Feedback Surveys
- Resources Blog eBooks Survey Templates Case Studies Training Help center

Home Market Research Research Tools and Apps
Research Design: What it is, Elements & Types
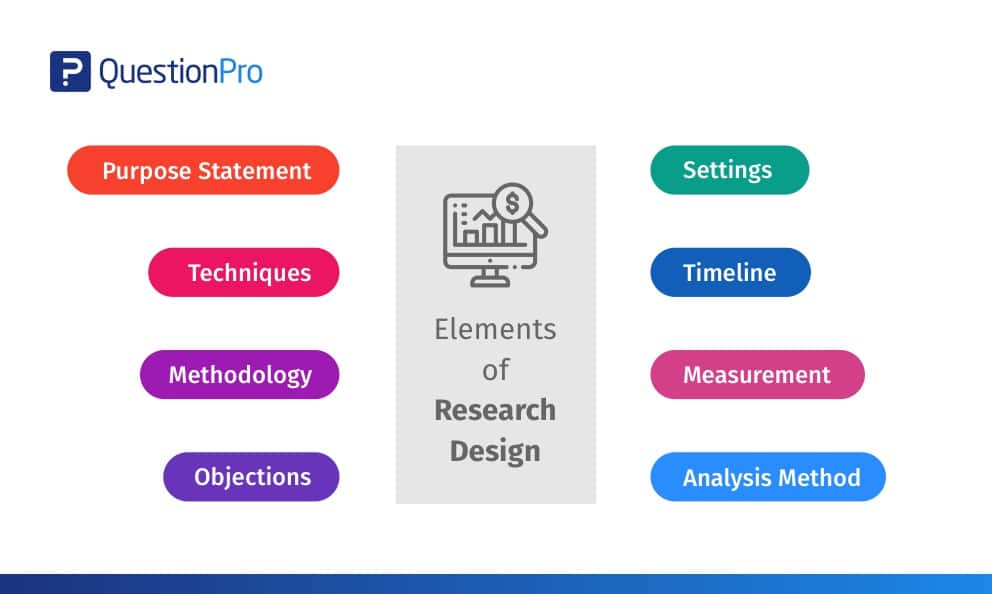
Can you imagine doing research without a plan? Probably not. When we discuss a strategy to collect, study, and evaluate data, we talk about research design. This design addresses problems and creates a consistent and logical model for data analysis. Let’s learn more about it.
What is Research Design?
Research design is the framework of research methods and techniques chosen by a researcher to conduct a study. The design allows researchers to sharpen the research methods suitable for the subject matter and set up their studies for success.
Creating a research topic explains the type of research (experimental, survey research , correlational , semi-experimental, review) and its sub-type (experimental design, research problem , descriptive case-study).
There are three main types of designs for research:
- Data collection
- Measurement
- Data Analysis
The research problem an organization faces will determine the design, not vice-versa. The design phase of a study determines which tools to use and how they are used.
The Process of Research Design
The research design process is a systematic and structured approach to conducting research. The process is essential to ensure that the study is valid, reliable, and produces meaningful results.
- Consider your aims and approaches: Determine the research questions and objectives, and identify the theoretical framework and methodology for the study.
- Choose a type of Research Design: Select the appropriate research design, such as experimental, correlational, survey, case study, or ethnographic, based on the research questions and objectives.
- Identify your population and sampling method: Determine the target population and sample size, and choose the sampling method, such as random , stratified random sampling , or convenience sampling.
- Choose your data collection methods: Decide on the data collection methods , such as surveys, interviews, observations, or experiments, and select the appropriate instruments or tools for collecting data.
- Plan your data collection procedures: Develop a plan for data collection, including the timeframe, location, and personnel involved, and ensure ethical considerations.
- Decide on your data analysis strategies: Select the appropriate data analysis techniques, such as statistical analysis , content analysis, or discourse analysis, and plan how to interpret the results.
The process of research design is a critical step in conducting research. By following the steps of research design, researchers can ensure that their study is well-planned, ethical, and rigorous.
Research Design Elements
Impactful research usually creates a minimum bias in data and increases trust in the accuracy of collected data. A design that produces the slightest margin of error in experimental research is generally considered the desired outcome. The essential elements are:
- Accurate purpose statement
- Techniques to be implemented for collecting and analyzing research
- The method applied for analyzing collected details
- Type of research methodology
- Probable objections to research
- Settings for the research study
- Measurement of analysis
Characteristics of Research Design
A proper design sets your study up for success. Successful research studies provide insights that are accurate and unbiased. You’ll need to create a survey that meets all of the main characteristics of a design. There are four key characteristics:
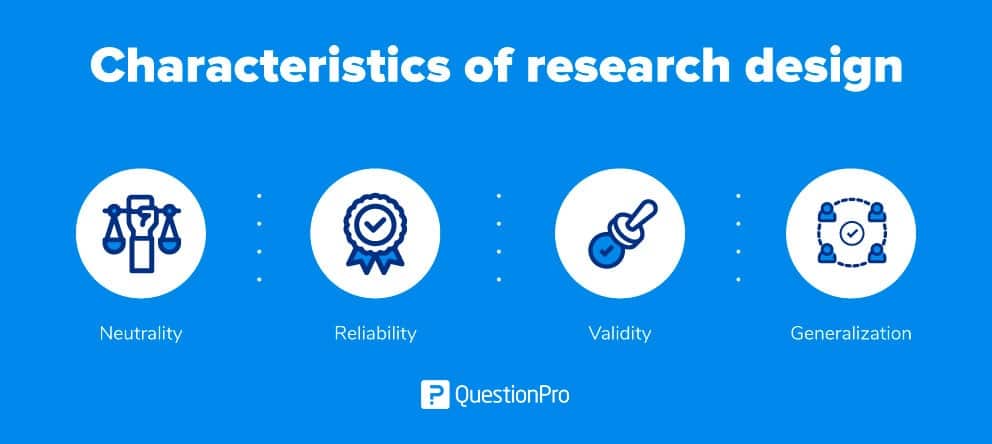
- Neutrality: When you set up your study, you may have to make assumptions about the data you expect to collect. The results projected in the research should be free from research bias and neutral. Understand opinions about the final evaluated scores and conclusions from multiple individuals and consider those who agree with the results.
- Reliability: With regularly conducted research, the researcher expects similar results every time. You’ll only be able to reach the desired results if your design is reliable. Your plan should indicate how to form research questions to ensure the standard of results.
- Validity: There are multiple measuring tools available. However, the only correct measuring tools are those which help a researcher in gauging results according to the objective of the research. The questionnaire developed from this design will then be valid.
- Generalization: The outcome of your design should apply to a population and not just a restricted sample . A generalized method implies that your survey can be conducted on any part of a population with similar accuracy.
The above factors affect how respondents answer the research questions, so they should balance all the above characteristics in a good design. If you want, you can also learn about Selection Bias through our blog.
Research Design Types
A researcher must clearly understand the various types to select which model to implement for a study. Like the research itself, the design of your analysis can be broadly classified into quantitative and qualitative.
Qualitative research
Qualitative research determines relationships between collected data and observations based on mathematical calculations. Statistical methods can prove or disprove theories related to a naturally existing phenomenon. Researchers rely on qualitative observation research methods that conclude “why” a particular theory exists and “what” respondents have to say about it.
Quantitative research
Quantitative research is for cases where statistical conclusions to collect actionable insights are essential. Numbers provide a better perspective for making critical business decisions. Quantitative research methods are necessary for the growth of any organization. Insights drawn from complex numerical data and analysis prove to be highly effective when making decisions about the business’s future.
Qualitative Research vs Quantitative Research
Here is a chart that highlights the major differences between qualitative and quantitative research:
In summary or analysis , the step of qualitative research is more exploratory and focuses on understanding the subjective experiences of individuals, while quantitative research is more focused on objective data and statistical analysis.
You can further break down the types of research design into five categories:
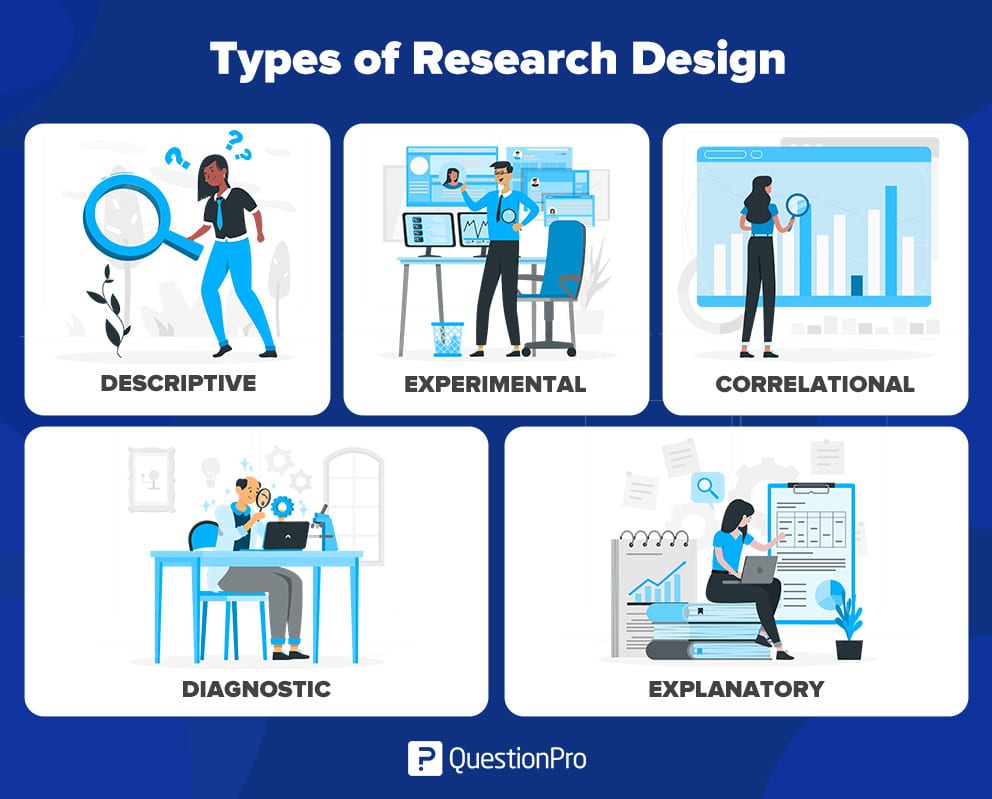
1. Descriptive: In a descriptive composition, a researcher is solely interested in describing the situation or case under their research study. It is a theory-based design method created by gathering, analyzing, and presenting collected data. This allows a researcher to provide insights into the why and how of research. Descriptive design helps others better understand the need for the research. If the problem statement is not clear, you can conduct exploratory research.
2. Experimental: Experimental research establishes a relationship between the cause and effect of a situation. It is a causal research design where one observes the impact caused by the independent variable on the dependent variable. For example, one monitors the influence of an independent variable such as a price on a dependent variable such as customer satisfaction or brand loyalty. It is an efficient research method as it contributes to solving a problem.
The independent variables are manipulated to monitor the change it has on the dependent variable. Social sciences often use it to observe human behavior by analyzing two groups. Researchers can have participants change their actions and study how the people around them react to understand social psychology better.
3. Correlational research: Correlational research is a non-experimental research technique. It helps researchers establish a relationship between two closely connected variables. There is no assumption while evaluating a relationship between two other variables, and statistical analysis techniques calculate the relationship between them. This type of research requires two different groups.
A correlation coefficient determines the correlation between two variables whose values range between -1 and +1. If the correlation coefficient is towards +1, it indicates a positive relationship between the variables, and -1 means a negative relationship between the two variables.
4. Diagnostic research: In diagnostic design, the researcher is looking to evaluate the underlying cause of a specific topic or phenomenon. This method helps one learn more about the factors that create troublesome situations.
This design has three parts of the research:
- Inception of the issue
- Diagnosis of the issue
- Solution for the issue
5. Explanatory research : Explanatory design uses a researcher’s ideas and thoughts on a subject to further explore their theories. The study explains unexplored aspects of a subject and details the research questions’ what, how, and why.
Benefits of Research Design
There are several benefits of having a well-designed research plan. Including:
- Clarity of research objectives: Research design provides a clear understanding of the research objectives and the desired outcomes.
- Increased validity and reliability: To ensure the validity and reliability of results, research design help to minimize the risk of bias and helps to control extraneous variables.
- Improved data collection: Research design helps to ensure that the proper data is collected and data is collected systematically and consistently.
- Better data analysis: Research design helps ensure that the collected data can be analyzed effectively, providing meaningful insights and conclusions.
- Improved communication: A well-designed research helps ensure the results are clean and influential within the research team and external stakeholders.
- Efficient use of resources: reducing the risk of waste and maximizing the impact of the research, research design helps to ensure that resources are used efficiently.
A well-designed research plan is essential for successful research, providing clear and meaningful insights and ensuring that resources are practical.
QuestionPro offers a comprehensive solution for researchers looking to conduct research. With its user-friendly interface, robust data collection and analysis tools, and the ability to integrate results from multiple sources, QuestionPro provides a versatile platform for designing and executing research projects.
Our robust suite of research tools provides you with all you need to derive research results. Our online survey platform includes custom point-and-click logic and advanced question types. Uncover the insights that matter the most.
FREE TRIAL LEARN MORE
MORE LIKE THIS
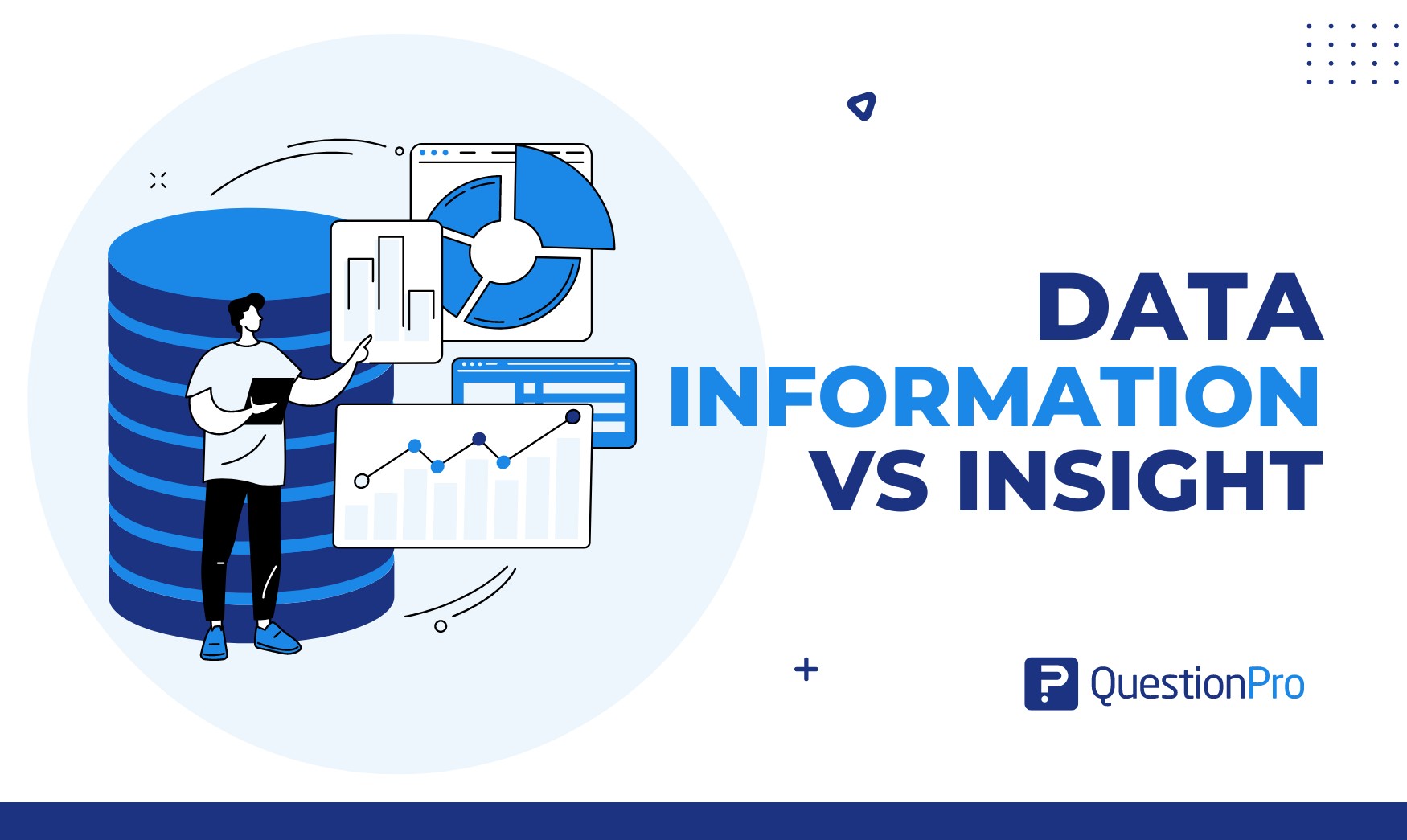
Data Information vs Insight: Essential differences
May 14, 2024
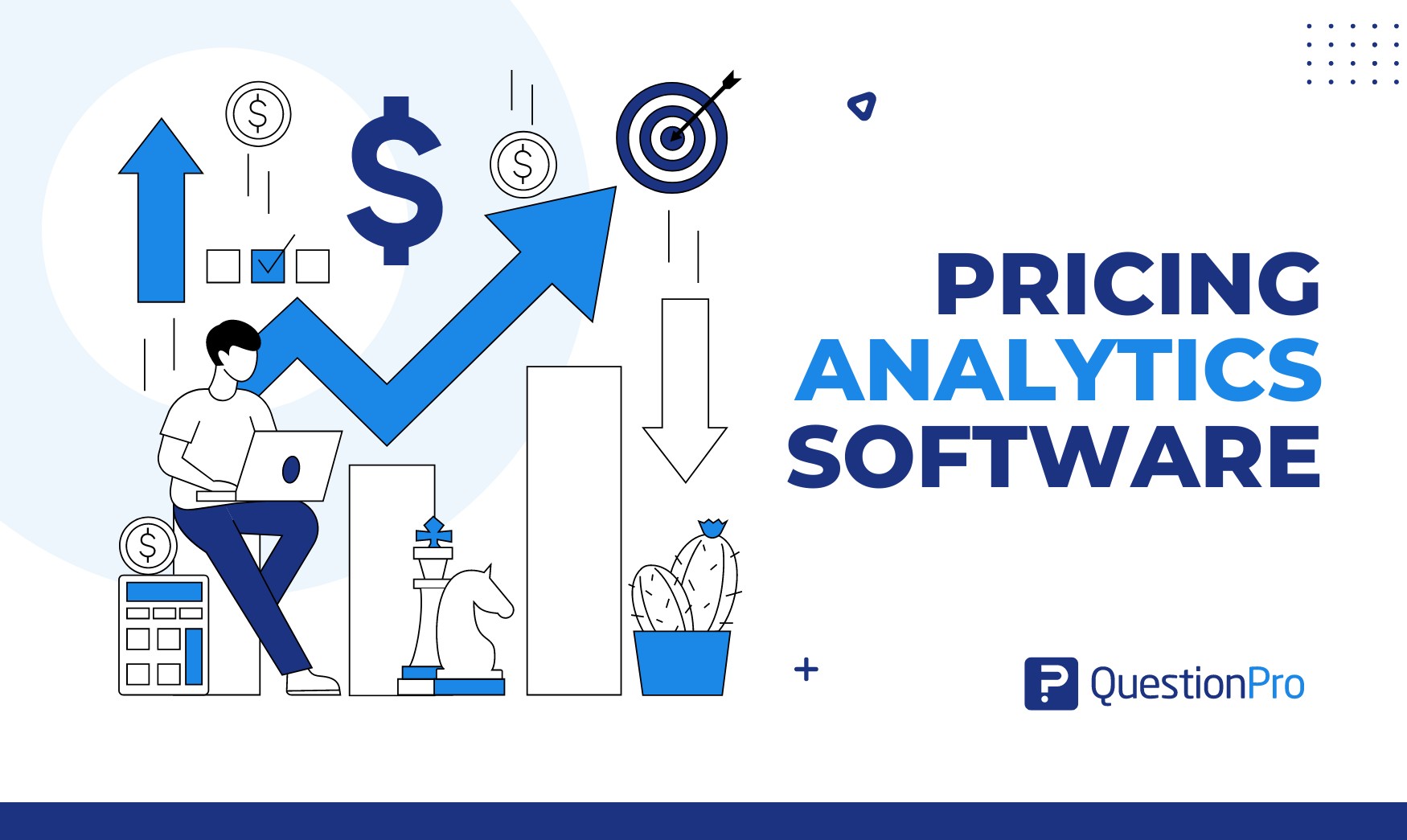
Pricing Analytics Software: Optimize Your Pricing Strategy
May 13, 2024
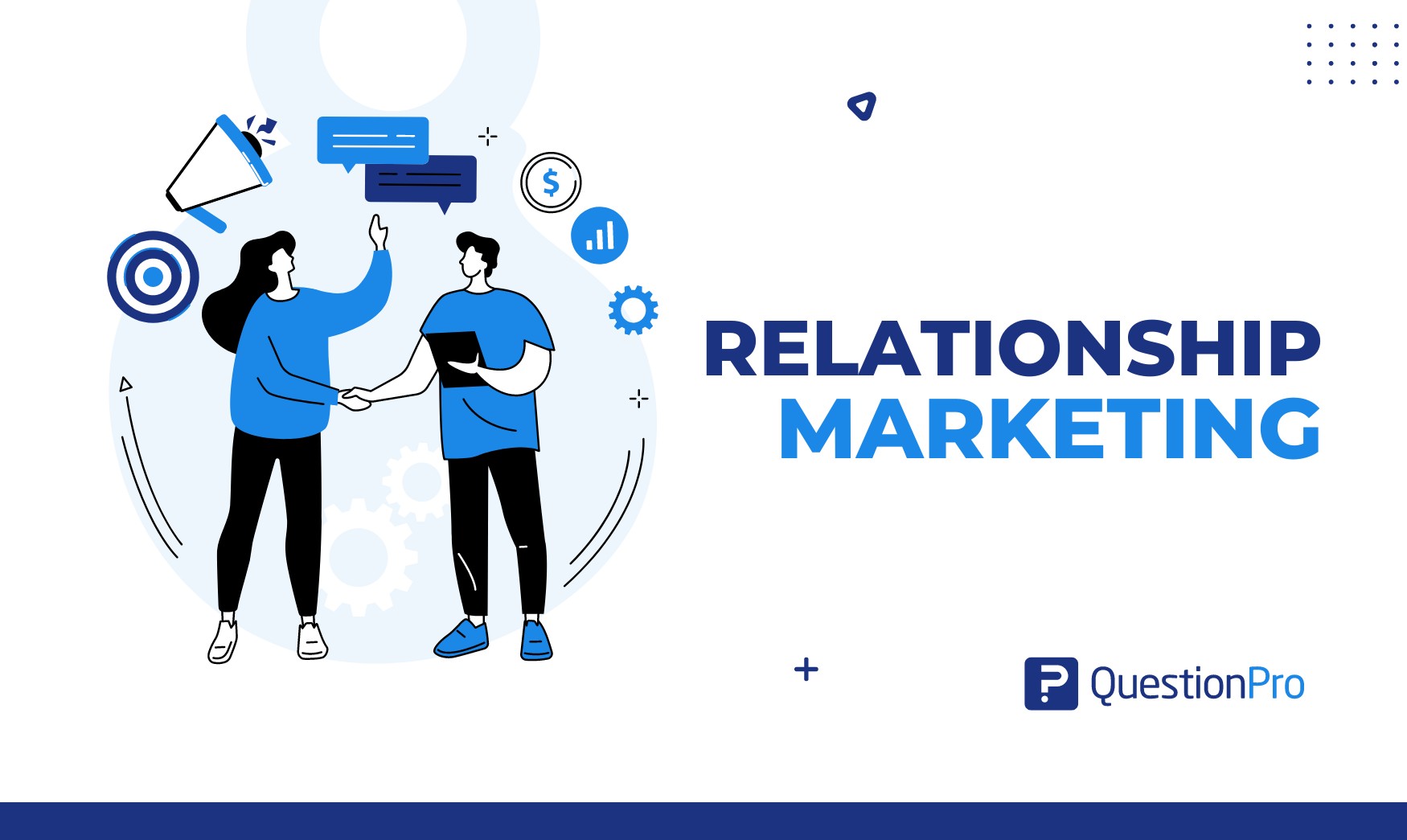
Relationship Marketing: What It Is, Examples & Top 7 Benefits
May 8, 2024
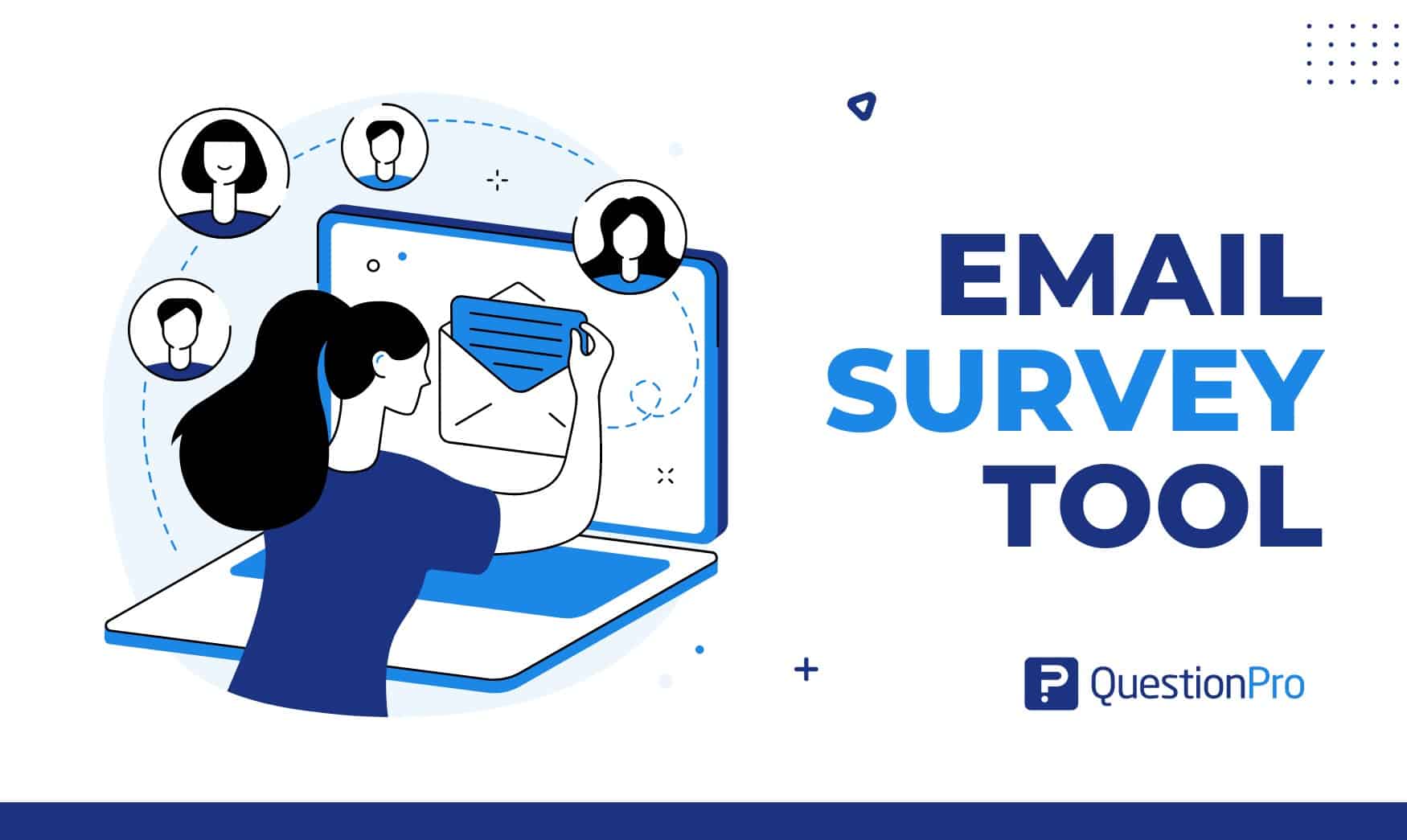
The Best Email Survey Tool to Boost Your Feedback Game
May 7, 2024
Other categories
- Academic Research
- Artificial Intelligence
- Assessments
- Brand Awareness
- Case Studies
- Communities
- Consumer Insights
- Customer effort score
- Customer Engagement
- Customer Experience
- Customer Loyalty
- Customer Research
- Customer Satisfaction
- Employee Benefits
- Employee Engagement
- Employee Retention
- Friday Five
- General Data Protection Regulation
- Insights Hub
- Life@QuestionPro
- Market Research
- Mobile diaries
- Mobile Surveys
- New Features
- Online Communities
- Question Types
- Questionnaire
- QuestionPro Products
- Release Notes
- Research Tools and Apps
- Revenue at Risk
- Survey Templates
- Training Tips
- Uncategorized
- Video Learning Series
- What’s Coming Up
- Workforce Intelligence
Diagnostic Trials
- Living reference work entry
- First Online: 04 November 2021
- Cite this living reference work entry
- Madhu Mazumdar 3 ,
- Xiaobo Zhong 4 &
- Bart Ferket 4
610 Accesses
The term diagnostic trial is generally used in two different ways. A diagnostic trial type I describes studies that evaluate accuracy of diagnostic tests in detecting disease or its severity. Primary endpoints for these studies are generally test accuracy outcomes measured in terms of sensitivity, specificity, positive predictive value, negative predictive value, and area under the receiver operating characteristics curves. Although establishing an accurate diagnosis or excluding disease is a critical first step to manage a health problem, medical decision-makers generally rely on a larger evidence base of empirical data that includes how tests impact patient health outcomes, such as morbidity, mortality, functional status, and quality of life. Therefore, the diagnostic trial type II evaluates the value of test results to guide or determine treatment decisions within a broader management strategy. Typically, differences in diagnostic accuracy result in differences in delivery of treatment, and ultimately affect disease prognosis and patient outcomes. As such, in the diagnostic trial type II , the downstream consequences of tests followed by treatment decisions are evaluated together in a joint construct. These diagnostic randomized clinical trials or test-treatment trials are considered the gold standard of proof for the clinical effectiveness or clinical utility of diagnostic tests. In this chapter, we define the variety of accuracy measures used for assessing diagnostic tests, summarize guidance on sample size calculation, and bring attention to the importance of more accurate reporting of study results.
This is a preview of subscription content, log in via an institution to check access.
Access this chapter
Institutional subscriptions
Ahmed HU, El-Shater Bosaily A, Brown LC, Gabe R, Kaplan R, Parmar MK, Collaco-Moraes Y et al (2017) Diagnostic accuracy of multi-parametric MRI and TRUS biopsy in prostate cancer (PROMIS): a paired validating confirmatory study. Lancet 389(10071):815–822. https://doi.org/10.1016/s0140-6736(16)32401-1
Article Google Scholar
Beam CA (1992) Strategies for improving power in diagnostic radiology research. AJR Am J Roentgenol 159(3):631–637. https://doi.org/10.2214/ajr.159.3.1503041
Begg CB, Greenes RA (1983) Assessment of diagnostic tests when disease verification is subject to selection bias. Biometrics 39(1):207–215
Article MathSciNet Google Scholar
Bossuyt PM, Reitsma JB, Linnet K, Moons KG (2012) Beyond diagnostic accuracy: the clinical utility of diagnostic tests. Clin Chem 58(12):1636–1643. https://doi.org/10.1373/clinchem.2012.182576
Braga LH, Farrokhyar F, Bhandari M (2012) Confounding: what is it and how do we deal with it? Can J Surg 55(2):132–138. https://doi.org/10.1503/cjs.036311
Bruni L, Barrionuevo-Rosas L, Albero G, Serrano B, Mena M, Gómez D, Muñoz J, Bosch FX, de Sanjosé S (2014) Human papillomavirus and related diseases report. L’Hospitalet de Llobregat: ICO Information Centre on HPV and Cancer
Google Scholar
Cardoso F, Piccart-Gebhart M, Van’t Veer L, Rutgers E (2007) The MINDACT trial: the first prospective clinical validation of a genomic tool. Mol Oncol 1(3):246–251. https://doi.org/10.1016/j.molonc.2007.10.004
Colli A, Fraquelli M, Casazza G, Conte D, Nikolova D, Duca P, Thorlund K, Gluud C (2014) The architecture of diagnostic research: from bench to bedside--research guidelines using liver stiffness as an example. Hepatology 60(1):408–418. https://doi.org/10.1002/hep.26948
de Groot JA, Bossuyt PM, Reitsma JB, Rutjes AW, Dendukuri N, Janssen KJ, Moons KG (2011) Verification problems in diagnostic accuracy studies: consequences and solutions. BMJ 343:d4770. https://doi.org/10.1136/bmj.d4770
Douglas PS, Hoffmann U, Patel MR, Mark DB, Al-Khalidi HR, Cavanaugh B, Cole J et al (2015) Outcomes of anatomical versus functional testing for coronary artery disease. N Engl J Med 372(14):1291–1300. https://doi.org/10.1056/NEJMoa1415516
Faraggi D, Reiser B (2002) Estimation of the area under the ROC curve. Stat Med 21(20):3093–3106. https://doi.org/10.1002/sim.1228
Ferrante di Ruffano L, Dinnes J, Taylor-Phillips S, Davenport C, Hyde C, Deeks JJ (2017) Research waste in diagnostic trials: a methods review evaluating the reporting of test-treatment interventions. BMC Med Res Methodol 17(1):32. https://doi.org/10.1186/s12874-016-0286-0
Ferrante di Ruffano L, Hyde CJ, McCaffery KJ, Bossuyt PM, Deeks JJ (2012) Assessing the value of diagnostic tests: a framework for designing and evaluating trials. BMJ 344:e686. https://doi.org/10.1136/bmj.e686
Fosgate GT (2009) Practical sample size calculations for surveillance and diagnostic investigations. J Vet Diagn Investig 21(1):3–14. https://doi.org/10.1177/104063870902100102
Flahault A, Cadilhac M, Thomas G (2005) Sample size calculation should be performed for design accuracy in diagnostic test studies. J Clin Epidemiol 58(8):859–862. https://doi.org/10.1016/j.jclinepi.2004.12.009
Glasziou PP, Cole BF, Gelber RD, Hilden J, Simes RJ (1998) Quality adjusted survival analysis with repeated quality of life measures. Stat Med 17(11):1215–1229. https://doi.org/10.1002/(sici)1097-0258(19980615)17:11<1215::aid-sim844>3.0.co;2-y
Gluud C, Gluud LL (2005) Evidence based diagnostics. BMJ 330(7493):724–726
Hajian-Tilaki KO, Hanley JA, Joseph L, Collet JP (1997) A comparison of parametric and nonparametric approaches to ROC analysis of quantitative diagnostic tests. Med Decis Mak 17(1):94–102. https://doi.org/10.1177/0272989x9701700111
Harel O, Zhou XH (2006) Multiple imputation for correcting verification bias. Stat Med 25(22):3769–3786. https://doi.org/10.1002/sim.2494
Henrichs J, Verfaille V, Jellema P, Viester L, Pajkrt E, Wilschut J, van der Horst HE, Franx A, de Jonge A (2019) Effectiveness of routine third trimester ultrasonography to reduce adverse perinatal outcomes in low risk pregnancy (the IRIS study): nationwide, pragmatic, multicentre, stepped wedge cluster randomised trial. BMJ 367:l5517. https://doi.org/10.1136/bmj.l5517
Hooper R, Díaz-Ordaz K, Takeda A, Khan K (2013) Comparing diagnostic tests: trials in people with discordant test results. Stat Med 32(14):2443–2456. https://doi.org/10.1002/sim.5676
Huchko MJ, Sneden J, Zakaras JM, Smith-McCune K, Sawaya G, Maloba M, Bukusi EA, Cohen CR (2015) A randomized trial comparing the diagnostic accuracy of visual inspection with acetic acid to visual inspection with Lugol’s iodine for cervical cancer screening in HIV-infected women. PLoS One 10(4):e0118568. https://doi.org/10.1371/journal.pone.0118568
Huang EP, Lin FI, Shankar LK (2017) Beyond correlations, sensitivities, and specificities: a roadmap for demonstrating utility of advanced imaging in oncology treatment and clinical trial design. Acad Radiol 24(8):1036–1049. https://doi.org/10.1016/j.acra.2017.03.002
Hu ZD (2016) STARD guideline in diagnostic accuracy tests: perspective from a systematic reviewer. Ann Transl Med 4(3):46. https://doi.org/10.3978/j.issn.2305-5839.2016.01.03
Hu ZD, Wei TT, Yang M, Ma N, Tang QQ, Qin BD, Fu HT, Zhong RQ (2015) Diagnostic value of osteopontin in ovarian cancer: a meta-analysis and systematic review. PLoS One 10(5):e0126444. https://doi.org/10.1371/journal.pone.0126444
Kearon C, Ginsberg JS, Douketis J, Turpie AG, Bates SM, Lee AY, Crowther MA et al (2006) An evaluation of D-dimer in the diagnosis of pulmonary embolism: a randomized trial. Ann Intern Med 144(11):812–821. https://doi.org/10.7326/0003-4819-144-11-200606060-00007
Kosinski AS, Barnhart HX (2003) A global sensitivity analysis of performance of a medical diagnostic test when verification bias is present. Stat Med 22(17):2711–2721. https://doi.org/10.1002/sim.1517
Korevaar DA, van Enst WA, Spijker R, Bossuyt PM, Hooft L (2014) Reporting quality of diagnostic accuracy studies: a systematic review and meta-analysis of investigations on adherence to STARD. Evid Based Med 19(2):47–54. https://doi.org/10.1136/eb-2013-101637
Korevaar DA, Wang J, van Enst WA, Leeflang MM, Hooft L, Smidt N, Bossuyt PM (2015) Reporting diagnostic accuracy studies: some improvements after 10 years of STARD. Radiology 274(3):781–789. https://doi.org/10.1148/radiol.14141160
Kumar R, Indrayan A (2011) Receiver operating characteristic (ROC) curve for medical researchers. Indian Pediatr 48(4):277–287. https://doi.org/10.1007/s13312-011-0055-4
Li J, Fine J (2004) On sample size for sensitivity and specificity in prospective diagnostic accuracy studies. Stat Med 23(16):2537–2550. https://doi.org/10.1002/sim.1836
Liu A, Schisterman EF, Mazumdar M, Hu J (2005) Power and sample size calculation of comparative diagnostic accuracy studies with multiple correlated test results. Biom J 47(2):140–150. https://doi.org/10.1002/bimj.200410094
Article MathSciNet MATH Google Scholar
Lijmer JG, Mol BW, Heisterkamp S, Bonsel GJ, Prins MH, van der Meulen JH, Bossuyt PM (1999) Empirical evidence of design-related bias in studies of diagnostic tests. JAMA 282(11):1061–1066. https://doi.org/10.1001/jama.282.11.1061
Lijmer JG, Bossuyt PM (2009) Various randomized designs can be used to evaluate medical tests. J Clin Epidemiol 62(4):364–373. https://doi.org/10.1016/j.jclinepi.2008.06.017
Lu B, Gatsonis C (2013) Efficiency of study designs in diagnostic randomized clinical trials. Stat Med 32(9):1451–1466. https://doi.org/10.1002/sim.5655
Mark DB, Federspiel JJ, Cowper PA, Anstrom KJ, Hoffmann U, Patel MR, Davidson-Ray L et al (2016) Economic outcomes with anatomical versus functional diagnostic testing for coronary artery disease. Ann Intern Med 165(2):94–102. https://doi.org/10.7326/m15-2639
McClish DK (1989) Analyzing a portion of the ROC curve. Med Decis Mak 9(3):190–195. https://doi.org/10.1177/0272989x8900900307
Metz CE (1978) Basic principles of ROC analysis. Semin Nucl Med 8(4):283–298. https://doi.org/10.1016/s0001-2998(78)80014-2
Majeed H, Amir E (2018) EQUATOR-Oncology: reducing the latitude of cancer trial design and reporting: Nature Publishing Group
Mustafa RA, Wiercioch W, Cheung A, Prediger B, Brozek J, Bossuyt P, Garg AX, Lelgemann M, Büehler D, Schünemann HJ (2017) Decision making about healthcare-related tests and diagnostic test strategies. Paper 2: a review of methodological and practical challenges. J Clin Epidemiol 92:18–28. https://doi.org/10.1016/j.jclinepi.2017.09.003
Modic MT, Obuchowski NA, Ross JS, Brant-Zawadzki MN, Grooff PN, Mazanec DJ, Benzel EC (2005) Acute low back pain and radiculopathy: MR imaging findings and their prognostic role and effect on outcome. Radiology 237(2):597–604. https://doi.org/10.1148/radiol.2372041509
NCSS. PASS (Power Analysis and Sample Size) Software 2018
Network, Equator (2017) EQUATOR Network: what we do and how we are organised 2016
Newby DE, Adamson PD, Berry C, Boon NA, Dweck MR, Flather M, Forbes J et al (2018) Coronary CT angiography and 5-year risk of myocardial infarction. N Engl J Med 379(10):924–933. https://doi.org/10.1056/NEJMoa1805971
Obuchowski NA (1998) Sample size calculations in studies of test accuracy. Stat Methods Med Res 7(4):371–392. https://doi.org/10.1177/096228029800700405
Ogilvie JC, Douglas Creelman C (1968) Maximum-likelihood estimation of receiver operating characteristic curve parameters. J Math Psychol 5(3):377–391
Obuchowski NA, Bullen JA (2018) Receiver operating characteristic (ROC) curves: review of methods with applications in diagnostic medicine. Phys Med Biol 63(7):07tr01. https://doi.org/10.1088/1361-6560/aab4b1
Park SH, Goo JM, Jo CH (2004). Receiver operating characteristic (ROC) curve: practical review for radiologists. Korean J Radiol 5(1):11–18. https://doi.org/10.3348/kjr.2004.5.1.11
Pepe MS (2003) The statistical evaluation of medical tests for classification and prediction. Medicine
Sackett DL, Haynes RB (2002) The architecture of diagnostic research. BMJ 324(7336):539–541. https://doi.org/10.1136/bmj.324.7336.539
Simel DL, Samsa GP, Matchar DB (1991) Likelihood ratios with confidence: sample size estimation for diagnostic test studies. J Clin Epidemiol 44(8):763–770. https://doi.org/10.1016/0895-4356(91)90128-v
Steinberg DM, Fine J, Chappell R (2009) Sample size for positive and negative predictive value in diagnostic research using case-control designs. Biostatistics 10(1):94–105. https://doi.org/10.1093/biostatistics/kxn018
Article MATH Google Scholar
Sun F, Schoelles KM, Coates VH (2013) Assessing the utility of genetic tests. J Ambul Care Manage 36(3):222–232. https://doi.org/10.1097/JAC.0b013e318295d7e3
Swets JA (1986) Indices of discrimination or diagnostic accuracy: their ROCs and implied models. Psychol Bull 99(1):100–117
Thompson IM, Ankerst DP, Chen C, Scott Lucia M, Goodman PJ, Crowley JJ, Parnes HL, Coltman CA (2005) Operating characteristics of prostate-specific antigen in men with an initial PSA level of 3.0 ng/ml or lower. JAMA 294(1):66–70
Trevethan R (2019) Response: commentary: sensitivity, specificity, and predictive values: foundations, Pliabilities, and pitfalls in research and practice. Front Public Health 7:408. https://doi.org/10.3389/fpubh.2019.00408
van Oudenaarde K, Swart NM, Bloem JL, Bierma-Zeinstra SMA, Algra PR, Bindels PJE, Koes BW et al (2018) General practitioners referring adults to MR imaging for knee pain: a randomized controlled trial to assess cost-effectiveness. Radiology 288(1):170–176. https://doi.org/10.1148/radiol.2018171383
Whiting P, Rutjes AW, Reitsma JB, Glas AS, Bossuyt PM, Kleijnen J (2004) Sources of variation and bias in studies of diagnostic accuracy: a systematic review. Ann Intern Med 140(3):189–202. https://doi.org/10.7326/0003-4819-140-3-200402030-00010
Walsh SJ (1997) Limitations to the robustness of binormal ROC curves: effects of model misspecification and location of decision thresholds on bias, precision, size and power. Stat Med 16(6):669–679. https://doi.org/10.1002/(sici)1097-0258(19970330)16:6<669::aid-sim489>3.0.co;2-q
Zhou X-H, McClish DK, Obuchowski NA (2009) Statistical methods in diagnostic medicine. John Wiley & Sons
Download references
Author information
Authors and affiliations.
Director of Institute for Healthcare Delivery Science, Mount Sinai Health System, New York, USA
Madhu Mazumdar
Ichan School of Medicine at Mount Sinai, New York, NY, USA
Xiaobo Zhong & Bart Ferket
You can also search for this author in PubMed Google Scholar
Corresponding author
Correspondence to Madhu Mazumdar .
Editor information
Editors and affiliations.
Samuel Oschin Comprehensive Cancer Insti, WEST HOLLYWOOD, CA, USA
Steven Piantadosi
Bloomberg School of Public Health, Johns Hopkins Center for Clinical Trials Bloomberg School of Public Health, Baltimore, MD, USA
Curtis L. Meinert
Section Editor information
Statistician, MRC Clinical Trials Unit at UCL, Institute of Clinical Trials and Methodology, London, UK
Babak Choodari-Oskooei
MRC Clinical Trials Unit and Institute of Clinical Trials and Methodology, University College of London, London, England
Mahesh Parmar
The Johns Hopkins Center for Clinical Trials and Evidence Synthesis, Johns Hopkins University, Baltimore, MD, USA
Brigham and Women’s Hospital, Harvard Medical School, Boston, MA, USA
Rights and permissions
Reprints and permissions
Copyright information
© 2021 Springer Nature Switzerland AG
About this entry
Cite this entry.
Mazumdar, M., Zhong, X., Ferket, B. (2021). Diagnostic Trials. In: Piantadosi, S., Meinert, C.L. (eds) Principles and Practice of Clinical Trials. Springer, Cham. https://doi.org/10.1007/978-3-319-52677-5_281-1
Download citation
DOI : https://doi.org/10.1007/978-3-319-52677-5_281-1
Received : 18 January 2021
Accepted : 03 February 2021
Published : 04 November 2021
Publisher Name : Springer, Cham
Print ISBN : 978-3-319-52677-5
Online ISBN : 978-3-319-52677-5
eBook Packages : Springer Reference Mathematics Reference Module Computer Science and Engineering
- Publish with us
Policies and ethics
- Find a journal
- Track your research

An official website of the United States government
The .gov means it’s official. Federal government websites often end in .gov or .mil. Before sharing sensitive information, make sure you’re on a federal government site.
The site is secure. The https:// ensures that you are connecting to the official website and that any information you provide is encrypted and transmitted securely.
- Publications
- Account settings
Preview improvements coming to the PMC website in October 2024. Learn More or Try it out now .
- Advanced Search
- Journal List

Diagnostic test evaluation methodology: A systematic review of methods employed to evaluate diagnostic tests in the absence of gold standard – An update
Chinyereugo m. umemneku chikere.
1 Institute of Health & Society, Faculty of Medical Sciences Newcastle University, Newcastle upon Tyne, England, United Kingdom
Kevin Wilson
2 School of Mathematics, Statistics and Physics, Newcastle University, Newcastle upon Tyne, England, United Kingdom
Sara Graziadio
3 National Institute for Health Research, Newcastle In Vitro Diagnostics Co-operative, Newcastle upon Tyne Hospitals National Health Services Foundation Trust, Newcastle upon Tyne, England, United Kingdom
A. Joy Allen
4 National Institute for Health Research, Newcastle In Vitro Diagnostics Co-operative, Newcastle University, Newcastle upon Tyne, England, United Kingdom
Associated Data
All relevant data are within the manuscript and its supporting information files.
To systematically review methods developed and employed to evaluate the diagnostic accuracy of medical test when there is a missing or no gold standard.
Study design and settings
Articles that proposed or applied any methods to evaluate the diagnostic accuracy of medical test(s) in the absence of gold standard were reviewed. The protocol for this review was registered in PROSPERO (CRD42018089349).
Identified methods were classified into four main groups: methods employed when there is a missing gold standard; correction methods (which make adjustment for an imperfect reference standard with known diagnostic accuracy measures); methods employed to evaluate a medical test using multiple imperfect reference standards; and other methods, like agreement studies, and a mixed group of alternative study designs. Fifty-one statistical methods were identified from the review that were developed to evaluate medical test(s) when the true disease status of some participants is unverified with the gold standard. Seven correction methods were identified and four methods were identified to evaluate medical test(s) using multiple imperfect reference standards. Flow-diagrams were developed to guide the selection of appropriate methods.
Various methods have been proposed to evaluate medical test(s) in the absence of a gold standard for some or all participants in a diagnostic accuracy study. These methods depend on the availability of the gold standard, its’ application to the participants in the study and the availability of alternative reference standard(s). The clinical application of some of these methods, especially methods developed when there is missing gold standard is however limited. This may be due to the complexity of these methods and/or a disconnection between the fields of expertise of those who develop (e.g. mathematicians) and those who employ the methods (e.g. clinical researchers). This review aims to help close this gap with our classification and guidance tools.
Introduction
Before a new medical test can be introduced into clinical practice, it should be evaluated for analytical validity (does the test work in the laboratory?), clinical validity (does the test work in the patient population of interest?) and clinical utility (is the test useful–can it lead to improvement in health outcomes?) [ 1 , 2 ]. Clinical validity studies, also called diagnostic accuracy studies, evaluate the test’s accuracy in discriminating between patients with or without the target condition (disease) [ 3 ]. The characteristics of the test (e.g. sensitivity and specificity) may inform what role the index test (the new test under evaluation) plays in the diagnostic pathway; is it a triage, add-on or replacement test? [ 4 ] Sensitivity (the proportion of participants correctly identified by the index test as having the target condition e.g. those with the disease) and specificity (the proportion of participants correctly identified by the index as not having the target condition) [ 5 – 7 ] are basic measures of the diagnostic accuracy of a test. Other common measures are predictive values, likelihood values, overall accuracy [ 8 , 9 ], receiver operating characteristic (ROC) curve, area under the ROC curve (AUROC) [ 10 ], ROC surface, and volume under the ROC surface (VUS) [ 11 – 13 ]. These measures are obtained by comparing the index test results with the results of the best currently available test for diagnosing the same target condition in the same participants; both tests are supposedly applied to all participants of the study [ 14 ]. The test employed as the benchmark to evaluate the index test is called the reference standard [ 15 ]. The reference standard could be a gold standard (GS), with sensitivity and specificity equal to 100%. This means that the gold standard perfectly discriminates between participants with or without the target conditions and provides unbiased estimates of the diagnostic accuracy measure of the index test as describe in Fig 1 . The term “bias” in this review is defined as the difference between the estimated value and the true value of the parameter of interest [ 16 ].

It is also expected that when evaluating the diagnostic accuracy of a medical test, the participants undertake both the index and reference tests within a short time-period if not simultaneously. This is to avoid biases caused by changes in their true disease status, which can also affect the diagnostic accuracy of the index test.
In addition to the common aforementioned diagnostic accuracy measures, there are other ways to evaluate the test performance of an index test. These include studies of agreement or concordance [ 17 ] between the index test and the reference standard and test positivity (or negativity) rate; that is the proportion of diagnostic tests that are positive (or negative) to the target condition [ 18 ].
In practice, there are deviations from the classical method ( Fig 1 ). These deviations are:
- Scenarios where the gold standard is not applied to all participants in the study (i.e. there is a missing gold standard) because it is expensive, or invasive, or patients do not consent to it, or the clinicians decided not to give the gold test to some patients for medical reasons [ 19 , 20 ]. Evaluating the new test using data only from participants whose disease status was confirmed with the gold standard can produce work-up or verification bias [ 21 ].
- Scenarios where the reference standard is not a gold standard (i.e. it is an imperfect reference standard) because it has a misclassification error or because there is no generally accepted reference standard for the target condition. Using an imperfect reference standard produces reference standard bias [ 22 , 23 ].
Several methods have been developed and used to evaluate the test performance of a medical test in these two scenarios.
Reviews of some of these methods have been undertaken previously. The reviews by Zhou [ 24 ], Alonzo [ 25 ] and the report by Naaktgeboren et al [ 26 ] focused on methods when the gold standard or reference standard is not applied to all participants in the study; Van Smeden et al [ 27 ] and Collins and Huynh [ 28 ] focused on the latent class models (LCMs); and Hui and Zhou [ 29 ], Trikalinos and Balion [ 30 ] and Enoe et al [ 31 ] focused on methods employed when the reference standard is imperfect. Zaki et al [ 32 ] focused on the agreement between medical tests whose results are reported as a continuous response. Branscum et al [ 33 ] focused on Bayesian approaches; and the reviews by Walsh [ 23 ], Rutjes et al [ 14 ] and Reitsma et al [ 34 ] focused around methods for evaluating diagnostic tests when there is a missing or imperfect reference standard.
The existing comprehensive reviews on this topic were published about 11 years ago [ 14 , 34 ]; knowledge, ideas, and research in this field has evolved significantly since then. Several new methods have been proposed and some existing methods have been modified. It is also possible that some previously identified methods may now be obsolete. Therefore, one of the aims of this systematic review is to review new and existing methods employed to evaluate the test performance of medical test(s) in the absence of gold standard for all or some of the participants in the study. It also aims to provide easy to use tools (flow-diagrams) for the selection of methods to consider when evaluating medical tests when sub-sample of the participants do not undergo the gold standard. The review builds upon the earlier reviews by Rutjes et al and Reitsma et al [ 14 , 34 ]. This review sought to identify methods developed to evaluate a medical test with continuous results in the presence of verification bias and when the diagnostic outcome (disease status) is classified into three or more groups (e.g. diseased, intermediate and non-diseased). This is a gap identified in the review conducted by Alonzo [ 25 ] in 2014.
The subsequent sections discuss the method employed to undertake the review, the results, the discussion of the findings and guidance to researchers involved in test accuracy studies.
Methodology
A protocol for this systematic review was developed, peer-reviewed and registered on PROSPERO (CRD42018089349).
Eligibility criteria
The review includes methodological articles (that is papers that proposed or developed a method) and application articles (that is papers where any of the proposed methods) were applied.
- Articles published in English language in a peer-reviewed journal.
- Articles that focus on evaluating the diagnostic accuracy of new (index) test when there is a missing gold standard, no gold standard or imperfect reference standard.
- Articles that assumed that the reference standard was a gold standard and the gold standard was applied to all participants in the study.
- Books, dissertations, thesis, conference abstracts, and articles not published in a peer reviewed journal.
- Systematic reviews and meta-analyses of the diagnostic accuracy of medical test(s) for a target condition (disease) in the absence of gold standard for some or all of the participants. However, individual articles included in these reviews that met the inclusion criteria were included.
Search strategies and selection of articles
The PRISMA statement [ 35 ] was used as a guideline when conducting this systematic review. The PRISMA checklist for this review, S1 Checklist , is included as one of the supplementary materials. The following bibliographic databases were searched: EMBASE, MEDLINE, SCOPUS, WILEY online library (which includes Cochrane library, EBM), PSYCINFO, Web of Science, and CINAHL. The details of the search strategies at reported in the S1 Appendix . The search dates were from January 2005 –February 2019. This is because, this review is an update of a review by Rutjes et al and Reitsma et al whose searched up to 2005. However, original methodological articles that proposed and described a method to evaluate medical test(s) when there is a missing or no gold standard published before 2005 were also included in the review. These original articles were identified by "snowballing" [ 36 ] from the references of some articles. All articles obtained from the electronic databases were imported to Endnote X8.0.2. The selection of articles to be included in this review were done by three people (CU, AJA, and KW). The sifting process was in two-stages: by title and abstract and then by full text against the inclusion and exclusion criteria. Any discrepancies between reviewers were resolved in a group meeting.
Data synthesis
A data collection form was developed for this review ( S1 Data ), which was piloted on seven studies and remodified to fit the purpose of this review. Information extracted from the included articles were synthesized narratively.
A total of 6127 articles were identified; 5472 articles were left after removing the duplicated articles; 5071 articles were excluded after sifting by title and abstract; 401 articles went forward to full text assessment; and a total of 209 articles were included in the review. The search and selection procedure are depicted using the PRISMA [ 35 ] flow-diagram ( Fig 2 ).

The articles included in this review used a wide variety of different study designs, like cross-sectional studies, retrospective studies, cohort studies, prospective studies and simulation studies.
The identified methods were categorized into four groups based on the availability and/or application of the gold standard to the participants in the study. These group are:
- Group 1: Methods employed when there is a missing gold standard.
- Group 2: Correction methods which adjust for using an imperfect reference standard whose diagnostic accuracy is known.
- Group 3: Methods employed when using multiple imperfect reference standards.
- Group 4: “other methods” . This group includes methods like study of agreement, test positivity rate, and considering alternative study design like validation.
Methods in groups 2, 3 and 4 are employed when there is no gold standard to evaluate the diagnostic accuracy of the index test; while methods in group 1 are employed when there is a gold standard to evaluate the diagnostic accuracy of the index test(s). However, the gold standard is applied to only a sub-sample of the participants.
A summary of all methods identified in the review, their key references and the clinical applications of these methods are reported on Table 1 .
LCA: latent class analysis; CRS is composite reference standard. ROC is receiver operating characteristics; NGS is no gold standard
Methods employed when gold standard is missing
Fifty-one statistical methods were identified from the review that were developed to evaluate the diagnostic accuracy of index test(s) when the true disease status of some participants is not verified with the gold standard. These methods are divided into two subgroups:

- Differential verification approach : Participants whose disease status was not verified with the gold standard could undergo another reference standard (that is imperfect or less invasive than the gold standard [ 84 ]) to ascertain their disease status. This is known as differential verification [ 200 ]. Differential verification has been explored Alonzo et al, De Groot et al and Naaktgeboren et al [ 200 – 202 ]. They discussed the bias associated with differential verification, and how results using this approach could be presented. There are three identified statistical methods in this group. They are: a Bayesian latent class approach proposed by De Groot et al [ 82 ], a Bayesian method proposed by Lu et al [ 203 ] and a ROC approach proposed by Glueck et al [ 16 ]. These three methods aim to simultaneously adjust for differential verification bias and reference standard bias that arises from using an alternative reference standard (i.e. imperfect reference standard) for participants whose true disease status was not verified with the gold standard.
Correction methods
This group includes algebraic methods developed to correct the estimated sensitivity and specificity of the index test when the sensitivity and specificity of the imperfect reference standard is known. There are seven statistical methods in this group described in five different articles [ 91 – 95 ]. The methods by Emerson et al [ 95 ] does not estimate a single value for sensitivity or specificity, unlike the other correction methods [ 91 – 94 ] but produces an upper bound value and a lower bound value for the sensitivity and specificity of the index test. These bounded values are used to explain the uncertainty around the estimated sensitivity and specificity of the index test.
Methods with multiple imperfect reference standards
A gold standard or accurate information about the diagnostic accuracy of the imperfect reference standard are often not available to evaluate the index test. In these situations, multiple imperfect reference standards can be employed to evaluate the index test. Methods in this group include:
- Discrepancy analysis : this compares the index test with an imperfect reference standard. Participants with discordant results undergo another imperfect test, called the resolver test, to ascertain their disease status. Discrepancy analysis is typically not recommended because it produces biased estimates [ 100 , 204 ]. Modifications of this approach have been proposed [ 18 , 101 , 136 ]. In these, some of the participants with concordant responses (true positives and true negatives) are sampled to undertake the resolver test alongside participants with discordant responses (false negative–FN and false positive–FP). However, further research is needed to explore if these modified approaches are adequate to remove or reduce the potential bias.
- Latent class analysis (LCA) : The test performance of all the tests employed in the study are evaluated simultaneously using probabilistic models with the basic assumption that the disease status is latent or unobserved. There are frequentist LCAs and Bayesian LCAs. The frequentist LCAs use only the data from the participants in the study to estimate the diagnostic accuracy measures of the tests; while the Bayesian LCAs employ external information (e.g. expert opinion or estimates from previous research study) on the diagnostic accuracy measures of the tests evaluated in additional to the empirical data obtained from participants within the study. The LCAs assume that the tests (new test and reference standards) are either conditionally independent given the true disease status or the tests are conditionally dependent. To model the conditional dependence among the tests, various latent class model (LCM) with different dependence structure have been developed such as the Log-linear LCM [ 102 ], Probit LCM [ 103 ], extended log-linear and Probit LCM [ 108 ], Gaussian Random Effect LCM [ 105 ] and two-crossed random effect LCM [ 107 ] among others. However, some studies [ 205 ],[ 206 ] have shown that latent class models with different conditional dependence structures produce different estimates of sensitivities and specificities and each model still has a good fit. Thus, further research could be carried out to explore if each of the conditional dependence LCM are case specific.
- Construct composite reference standard : this method combines results from multiple imperfect tests (excluding the index test) with a predetermined rule to construct a reference standard that is used to evaluate the index test. By excluding the index test as part of the composite reference standard, incorporation bias can be avoided [ 131 ]. A novel method identified under the composite reference standard is the “dual composite reference standard” proposed by Tang et al [ 134 ].
- Panel or consensus diagnosis : this method uses the decision from a panel of experts to ascertain the disease status of each participant, which is then used to evaluate the index test.
Other methods
This group includes methods that fit the inclusion criteria but could not be placed into the other three groups. They include study of agreement, test positivity rate and the use of an alternative study design such as analytical validation. Study of agreement and test positivity rate are best used as exploratory tools alongside other methods [ 152 , 178 ] because they are not robust enough to assess the diagnostic ability of the medical test. Validation of a medical test cut across different disciplines in medicine such as psychology, laboratory or experimental medicine. With this approach, the medical test is assessed based on what it is designed to do [ 191 ]. Other designs include case-control designs (where the participants are known to have or not have the target condition) [ 207 , 208 ], laboratory based studies or experimental studies which are undertaken with the aim to evaluate the analytical sensitivity and specificity of the index test [ 190 , 209 , 210 ].
Guidance to researchers
The guidance flowchart ( Fig 5 ) is a modification and extension of the guidance for researchers flow-diagram developed by Reitsma et al [ 34 ].

Since, evaluating the accuracy measures of the index test is the focus of any diagnostic accuracy study, the flowchart starts with asking the first question “Is there a gold standard to evaluate the index test?” Following the responses from each question box (not bold); methods are suggested (bold boxes at the bottom of the flowchart) to guide clinical researchers, test evaluators, and researchers as to the different methods to consider.
Although, this review aims to provide up-to-date approaches that have been proposed or employed to evaluate the diagnostic accuracy of an index test in the absence of a gold standard for some or all of the participants in the accuracy study; some things researchers can consider when designing an accuracy study aside from the aim of their studies, are outlined in Box 1 ([ 26 , 211 – 218 ]).
Box 1: Suggestions when designing a diagnostic accuracy study.
- Design a protocol : The protocol describes every step of the study. It states the problem and how it will be addressed.
- Selection of participants from target population : The target population determines the criteria for including participants in the study. Also, the population is important in selecting the appropriate setting for the study.
- Selection of appropriate reference standard: The reference standard should diagnose same target condition as the index test. The choice of reference standard (gold or non-gold) determines the methods to apply when evaluating the index test (see Fig 5 ).
- Sample size : Having adequate sample size is necessary to make precise inference from the statistical analysis that will be carried out. Studies that discuss the appropriate sample size to consider when planning test accuracy are [ 211 – 215 ].
- Selection of accuracy measure to estimate : The researchers need to decide which accuracy measures they wish to estimate, and this is often determined by the test’s response (binary or continuous).
- Anticipate and eliminate possible bias : multiple forms of bias may exist [ 26 , 216 – 218 ]. Exploring how to avoid or adjust for these bias (if they are unavoidable) is important.
- Validation of results : Is validation of the results from the study on an independent sample feasible? Validation ensures an understanding of the reproducibility, strengths, and limitations of the study.
Some guidelines and tools have been developed to assist in designing, conducting and reporting diagnostic accuracy studies such as the STARD [ 219 – 223 ] guidelines, GATE [ 224 ] framework, QUADAS [ 225 ] tools; which can aid the design of a robust test accuracy study.
This review sought to identify and review new and existing methods employed to evaluate the diagnostic accuracy of a medical test in the absence of gold standard. The identified methods are classified into four main groups based on the availability and/or the application of the gold standard on the participants in the study. The four groups are: methods employed when only a sub-sample of the participants have their disease status verified with the gold standard (group 1); correction methods (group 2); methods using multiple imperfect reference standards (group 3) and other methods (group 4) such as study of agreement, test positivity rate and alternative study designs like validation.
In this review additional statistical methods have been identified that were not included in the earlier reviews on this topic by Reitsma et al [ 34 ] and Alonzo [ 25 ]. A list of all the methods identified in this review are presented in the supplementary material ( S1 Supplementary Information ). This includes a brief description of the methods and a discussion of their strengths and weaknesses and any identified case studies where the methods have been clinically applied. Only a small number of the methods we have identified have applied clinically and published [ 38 , 63 ]. This may be due to the complexity of these methods (in terms of application and interpretation of results), and/or a disconnection between the fields of expertise of those who develop (e.g. mathematicians or statisticians) and those who employ the methods (e.g. clinical researchers). For example, the publication of such method in specialist statistical journals may not be readily accessible to clinical researchers designing the study. In order to close this gap, two flow-diagrams (Figs (Figs3 3 and and4) 4 ) were constructed in addition to the modified guidance flowchart, ( Fig 5 ) as guidance tools to aid clinical researchers and test evaluators in the choice of methods to consider when evaluating medical test in the absence of gold standard. Also, an R package ( bcROCsurface ) and an interactive web application (Shiny app) that estimates the ROC surface and VUS in the presence of verification bias have been developed by To Duc [ 78 ] to help bridge the gap.
One of the issues not addressed in this current review was on methods that evaluate the differences in diagnostic accuracy of two or more tests in the presence of verification bias. Some published articles that consider this issue are Nofuentes and Del Castillo [ 226 – 230 ], Marin-Jimenez and Nofuentes [ 231 ], Harel and Zhou [ 232 ] and Zhou and Castelluccio [ 233 ]. This review also did not consider methods employed to estimate the time-variant sensitivity and specificity of diagnostic test in absence of a gold standard. This issue has recently been addressed by Wang et al [ 234 ].
In terms of the methodology, a limitation of this review is the exclusion of books, dissertations, thesis, conference abstract and articles not published in English language (such as the review by Masaebi et al [ 235 ] which was published in 2019), which could imply that there could still be some methods not identified by this review.
Regarding the methods identified in this review, further research could be carried to explore the different modification to the discrepancy analysis approaches to understand if these modifications reduce or remove the potential bias. In addition, further research is needed to determine if the different methods developed to evaluate an index test in the presence of verification bias are robust methods. Given the large numbers of statistical methods that have been developed especially to evaluate medical tests when there is a missing gold standard and the complexity of some of these methods; more interactive web application (e.g. Shiny package in R [ 236 ]) could be developed to implement these methods in addition to the Shiny app developed by To Duc [ 78 ] and Lim et al [ 237 ]. The development of such interactive web tools will expedite the clinical applications of these developed methods and help bridge the gap between the method developers and the clinical researchers or tests evaluators who are the end users of these methods.
Various methods have been proposed and applied in the evaluation of medical tests when there is a missing gold standard result for some participants, or no gold standard at all. These methods depend on the availability of the gold standard, its application to all or subsample of participants in the study, the availability of alternative reference standard(s), and underlying assumption(s) made with respect to the index test(s) and / or participants in the study.
Knowing the appropriate method to employ when analysing the data from participants of a diagnostic accuracy studies in the absence of gold standard, help to make statistically robust inference on the accuracy of the index test. This, in addition to data on cost-effectiveness, utility and usability of the test will support clinicians, policy makers and stake holders to decide the adoption of the new test in practice or not.
Supporting information
S1 checklist, s1 appendix, s1 supplementary information, acknowledgments.
The authors will like to acknowledge Professor Patrick Bossuyt from the Department of Clinical Epidemiology and Biostatistics, Academic Medical centre, University of Amsterdam, the Netherlands, for giving the consent to update his review, reviewing the protocol and his continued advice throughout this work. Also we will like to acknowledge the authors of the previous review, Dr Anne Rutjes in University of Bern, Switzerland; Professor Johannes Reitsma in the Department of Epidemiology, Julius Center Research Program Methodology UMC Utrecht, The Netherlands; Professor Arri Coomarasamy in the College of Medical and Dental Sciences, University of Birmingham, UK; and Professor Khalid Saeed Khan in Queen Mary, University of London for the guidance flowchart which was modified and extended. AJA, SG, and LV are supported by the National Institute for Health Research (NIHR) Newcastle In Vitro Diagnostics Co-operative. The views expressed are those of the authors and not necessarily those of the NIHR or the Department of Health and Social Care.
Funding Statement
This work is supported by the Newcastle University Research Excellence; the School of Mathematics, Statistics and Physics Newcastle University; the Institute of Health & Society Newcastle University; and the National Institute for Health Research (NIHR) [NIHR Newcastle In Vitro Diagnostics Co-operative]. The view and opinions expressed are those of the authors and do not necessary reflect those of the NIHR Newcastle In Vitro Diagnostics Co-operative, Newcastle University and Newcastle upon Tyne NHS Foundation Trust, the NHS or Newcastle Research Academy. The views expressed are those of the authors and not necessarily those of the NIHR, the NHS or the Department of Health and Social Care. The funders had no role in study design, data collection and analysis, decision to publish, or preparation of the manuscript.
Data Availability
- Survey Software The world’s leading omnichannel survey software
- Online Survey Tools Create sophisticated surveys with ease.
- Mobile Offline Conduct efficient field surveys.
- Text Analysis
- Close The Loop
- Automated Translations
- NPS Dashboard
- CATI Manage high volume phone surveys efficiently
- Cloud/On-premise Dialer TCPA compliant Cloud & on-premise dialer
- IVR Survey Software Boost productivity with automated call workflows.
- Analytics Analyze survey data with visual dashboards
- Panel Manager Nurture a loyal community of respondents.
- Survey Portal Best-in-class user friendly survey portal.
- Voxco Audience Conduct targeted sample research in hours.

Find the best survey software for you! (Along with a checklist to compare platforms)
Get Buyer’s Guide
- 100+ question types
- Drag-and-drop interface
- Skip logic and branching
- Multi-lingual survey
- Text piping
- Question library
- CSS customization
- White-label surveys
- Customizable ‘Thank You’ page
- Customizable survey theme
- Reminder send-outs
- Survey rewards
- Social media
- Website surveys
- Correlation analysis
- Cross-tabulation analysis
- Trend analysis
- Real-time dashboard
- Customizable report
- Email address validation
- Recaptcha validation
- SSL security
Take a peek at our powerful survey features to design surveys that scale discoveries.
Download feature sheet.
- Hospitality
- Financial Services
- Academic Research
- Customer Experience
- Employee Experience
- Product Experience
- Market Research
- Social Research
- Data Analysis
Explore Voxco
Need to map Voxco’s features & offerings? We can help!
Watch a Demo
Download Brochures
Get a Quote
- NPS Calculator
- CES Calculator
- A/B Testing Calculator
- Margin of Error Calculator
- Sample Size Calculator
- CX Strategy & Management Hub
- Market Research Hub
- Patient Experience Hub
- Employee Experience Hub
- Market Research Guide
- Customer Experience Guide
- The Voxco Guide to Customer Experience
- NPS Knowledge Hub
- Survey Research Guides
- Survey Template Library
- Webinars and Events
- Feature Sheets
- Try a sample survey
- Professional services
Find the best customer experience platform
Uncover customer pain points, analyze feedback and run successful CX programs with the best CX platform for your team.
Get the Guide Now

We’ve been avid users of the Voxco platform now for over 20 years. It gives us the flexibility to routinely enhance our survey toolkit and provides our clients with a more robust dataset and story to tell their clients.
VP Innovation & Strategic Partnerships, The Logit Group
- Client Stories
- Voxco Reviews
- Why Voxco Research?
- Careers at Voxco
- Vulnerabilities and Ethical Hacking
Explore Regional Offices
- Cloud/On-premise Dialer TCPA compliant Cloud on-premise dialer
- Predictive Analytics
- Customer 360
- Customer Loyalty
- Fraud & Risk Management
- AI/ML Enablement Services
- Credit Underwriting
Get Buyer’s Guide
- SMS surveys
- Banking & Financial Services
- Retail Solution
- Risk Management
- Customer Lifecycle Solutions
- Net Promoter Score
- Customer Behaviour Analytics
- Customer Segmentation
- Data Unification
Explore Voxco
Watch a Demo
Download Brochures
- CX Strategy & Management Hub
- Blogs & White papers
- Case Studies

VP Innovation & Strategic Partnerships, The Logit Group
- Why Voxco Intelligence?
- Our clients
- Client stories
- Featuresheets
Explore the Elements of Research Design
SHARE THE ARTICLE ON
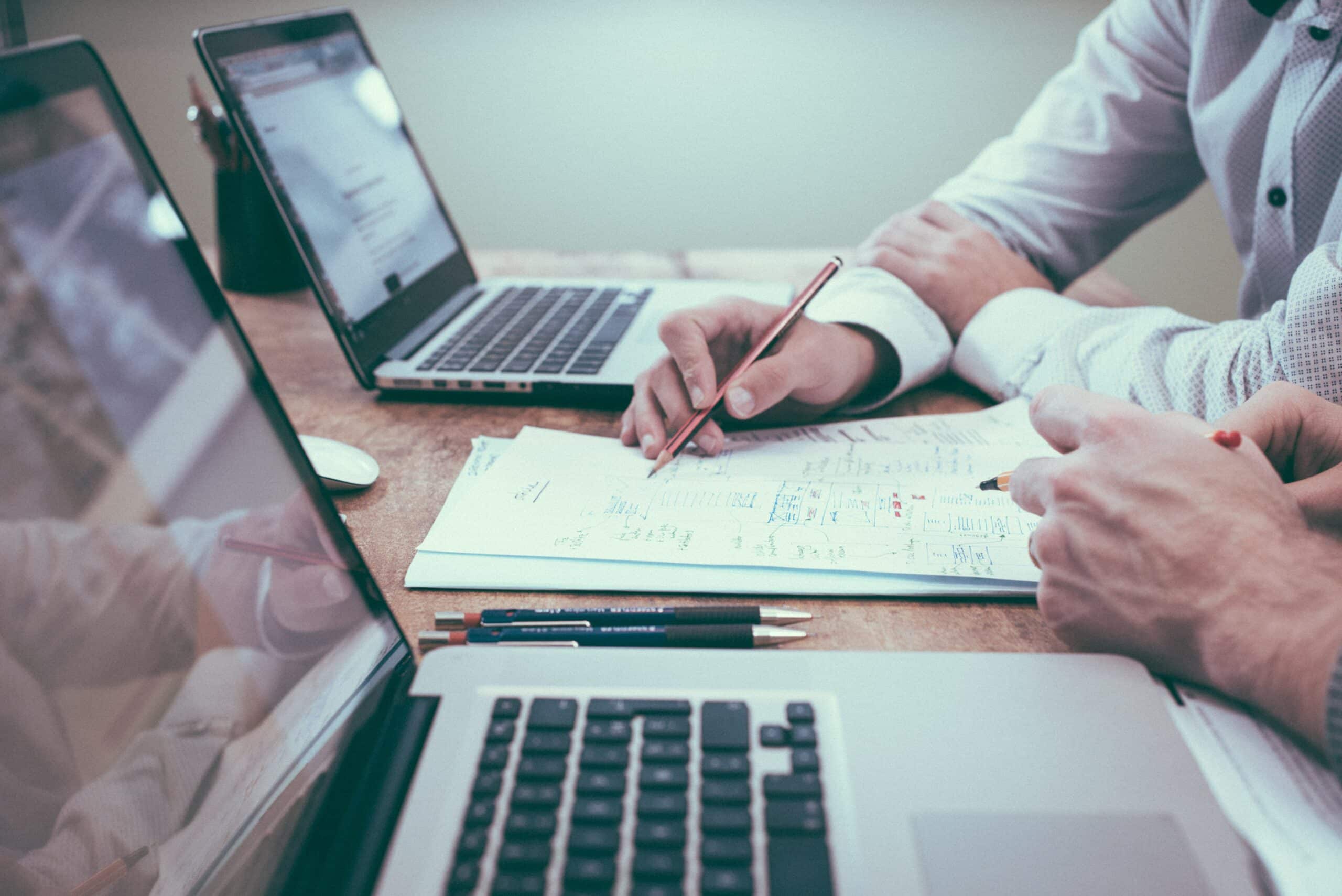
Research design is a blueprint for your entire research process. It helps you seamlessly navigate through the complexities of sampling, data collection, analysis, and interpretation. Whether you are venturing into the world of social sciences or conducting market research, understanding the elements and intricacies of the methodologies behind research will help you conduct the study with more clarity and confidence.
In this blog, we’ll explore the characteristics and types of research methodology to help you understand how to design your research process.
What is research design?
According to the definition of research design, it refers to the framework of market research methods and techniques that are chosen by a researcher. The design that is chosen by the researchers allow them to utilise the methods that are suitable for the study and to set up their studies successfully in the future as well.
Research design offers a variety of options. It can be qualitative, quantitative, or mixed. Under these designs, researchers can choose from various research methods such as experimental studies, surveys, correlational studies, or quasi-experimental review studies. There are also sub-types of research methods including experimental design, defining research problems, and descriptive studies.
Research designs are influenced by the research problem a company chooses to work on. This problem serves as the determining factor in the choice of research design, highlighting the logical sequence of steps in conducting a research study.
The market research study’s design phase is when the researchers determine the tools to be used and how they will be used. Good research usually ensures minimum levels of bias in the data collection method to improve both the internal and external validity of the research. The desired outcome of experimental research is to have a design that will result in the least amount of error in the study.
Read how Voxco helped Modus Research increase research efficiency with Voxco Online, CATI, IVR, and panel systems.
What are the elements of research design.
Some essential elements of research designs are highlighted below:
1. Research purpose:
A research design cannot be decided without an accurate purpose or problem statement.
2. Appropriate sampling:
This includes determining the appropriate sampling methods, correct sample size, and key characteristics of the population. Tools like a market research panel can simplify this step by giving you access to vetted and willing survey participants.
3. Data collection methods:
The process of gathering data from the participants is also a critical element. This includes selecting what data to collect, the right mode of data collection, and the tools used for the purpose. Voxco offers three modes of data collection – online , CATI , and mobile-offline .
4. Data analysis:
Research designs include data analysis and interpretation. This element includes deciding which statistical method to use to analyze the data to mitigate any error or bias in research results.
5. Types of methodology:
This step includes determining the best among the several types of research methodology. Different research designs require different settings for the conduction of a study.
6. Setting up time frame:
Another element is to outline the general timeline it will take to conduct a study using different research methods.
7. Integrity:
Using an accurate research design will help your study be successful. Research studies that are successful and include the least amount of error provide important insights that are free of bias.
8. Ethical considerations:
It must also ensure adhering to ethical considerations such as informed consent, confidentiality, and anonymity.
What are the main characteristics of research design?
To better understand how you can design your own research process, let’s take a look at the main characteristics of the subject.
01. Neutrality before research initiation:
When you are planning to study a phenomenon, you may have an assumption about the kind of data you are expecting to collect. However, the results you find from the study should not be driven by bias and must be neutral. In order to understand the opinions on the obtained results, you can discuss it with multiple people and consider the points made by individuals who agree with the results obtained.
02. Reliability of research design:
When you replicate an already conducted market research , you expect similar results. Decide the type of research questions you are going to ask through your surveys and define that in your research design. This will help set a standard for the results. Only if your design is reliable it will help you obtain the expected results.
03. Validity of insights:
You need to ensure that the survey questionnaire you are using is valid. Validity refers to the fact that the research tool you use measures what it purports to measure. Only valid tools will help researchers in gathering accurate results for their study.
04. Generalizability of research findings:
The outcome of your research design should be generalizable to a wider population. Good research design findings are generalizable to everyone, and they indicate that if your survey were to be replicated on any subgroup of the population, it would yield similar results.
A good research design balances all the above characteristics. Researchers must also understand the different research design types to choose from. This understanding will help them implement the most accurate research design for their study.
See how easily you can create, test, distribute, and design the surveys.
- 50+ question types
- Advanced logic
- White-label
- Auto-translations in 100+ languages
What are the different types of research design?
Broadly, there are two types of research design types:
- Qualitative research design
- Quantitative research design
Quantitative Research Design:
Quantitative research is the process of collecting and analyzing numerical data. It is generally used to find patterns, averages, predictions, and cause-effect relationships between the variables being studied. It is also used to generalize the results of a particular study to the population in consideration.
Quantitative research is widely used in science, both in the natural and social sciences. It provides actionable insights that are essential for company growth.
Qualitative Research Design:
Qualitative research is a method used for market research that aims to obtain data through open-ended questions and conversations with the intended consumers.
This method aims to establish not only “what” people think but also “how” they came to that opinion and “why” they think so.
What are the subtypes of research design?
We can further explore research design in five sub-types based on the objective, methodology, and focus.
01. Descriptive research design
Descriptive research refers to the methods that describe the characteristics of the variables under study. This methodology focuses on answering questions relating to “what” than the “why” of the research subject. The primary focus of descriptive research is to simply describe the nature of the demographics under the study instead of focusing on the “why”.
Descriptive research is called an observational research method, as none of the variables in the study are influenced during the research process. If the problem is unclear enough to conduct a descriptive analysis, researchers can use exploratory research methods first.
02. Experimental research design
Experimental research , also called experimentation, is conducted using a scientific approach with two or more variables. The first variable is a constant that can be manipulated to see the differences caused by the second variable. Most studies using quantitative research methods are experimental in nature.
Experimental research helps you in gathering the necessary data for you to make better decisions about your proposed hypothesis. The success of experimental research usually confirms that the change observed in the variable under study is solely based on the manipulation of the independent variable.
Experimental research design is the most practical and accurate kind of research method that helps establish causation. This research design is used in social sciences to understand and observe human behavior. The behavior is observed by placing humans in two groups so that researchers can make comparisons.
03. Correlational research design
A correlation refers to an association or a relationship between two entities.
Correlational research studies how one entity impacts the other and what are changes are observed when either one of them changes. This research method is carried out to understand naturally occurring relationships between variables.
Hence, at least two groups are required to conduct correlational quantitative research successfully. The variables in this study are not under the researcher’s control; the researcher is simply trying to establish whether or not a relationship between two variables exists.
Since correlational studies only explain whether there is a relationship between two groups, they do not establish causation. Thus, it is not recommended to draw conclusions solely based on correlational studies; just because two variables are in sync does not mean they are interrelated or that one variable is causing the changes in the other variable!
A numeric correlation coefficient determines the strength of the relationship between two variables and ranges from -1 to +1. If the correlation coefficient obtained is -1, it indicates a perfect negative relationship between the two variables, i.e., as one variable increases (age), the other variable decreases (purchase of sports products).
If the correlation coefficient of a study is found to be +1, it indicates a perfect positive relationship between the two variables, whereas one variable increases (age) and the other variable also increases (purchasing beauty-enhancing products).
04. Diagnostic research design
In a diagnostic research design, the researcher is trying to evaluate the cause of a specific problem or phenomenon.
This research design is used to understand more in detail the factors that are creating problems in the company. Diagnostic research design includes three steps:
Step -1: The inception of the issue – When did the issue arise? In what situations is the issue more evident?
Step -2: Diagnosis of the issue – What is the underlying cause of the issue? What is influencing the issue to worsen?
Step -3: Solution for the issue – What is working in curing the issue? Under what situations does the problem seem to become less evident?
05. Explanatory research design
Explanatory research design uses the ideas and thoughts of a researcher on one subject to be the guiding point for future studies, it is also used in exploring theories further. The research focuses on explaining the unexplored patterns of phenomena and elaborates on the details pertaining to the research questions such as; what, why, and how.
Voxco helps the top 50 MR firms & 450+ global brands gather omnichannel feedback, measure sentiment, uncover insights, and act on them.
See how voxco can enhance your research efficiency..
A clear research design provides a direction guiding your process with a clear objective and questions to investigate the topic of interest. Research design ensures the validity and reliability of the research findings and confirms that one can replicate the result even for future research. An appropriately created and executed research design helps you draw meaningful conclusions.
Explore Voxco Survey Software
+ Omnichannel Survey Software
+ Online Survey Software
+ CATI Survey Software
+ IVR Survey Software
+ Market Research Tool
+ Customer Experience Tool
+ Product Experience Software
+ Enterprise Survey Software

Ad Recall Survey Questions
Ad Recall Survey Questions Voxco is trusted by 450+ Global Brands in 40+ countries See what question types are possible with a sample survey! Try
Data Integrity and why does it matter?
Data Integrity and why does it matter? SHARE THE ARTICLE ON Share on facebook Share on twitter Share on linkedin Table of Contents Introduction As
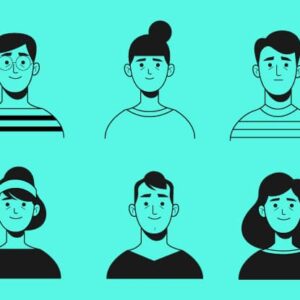
Gender Survey Questions
Crafting Inclusive Gender Survey Questions SHARE THE ARTICLE ON Table of Contents A survey questionnaire is one of the ways that enables you to connect
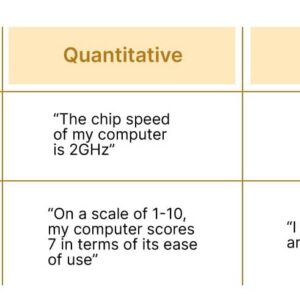
Understanding and Implementing Customer Experience programs
Understanding and Implementing Customer Experience programs Free Download: Enhance NPS® Scores using our NPS® Survey Templates Download Now SHARE THE ARTICLE ON Customer sentiment has
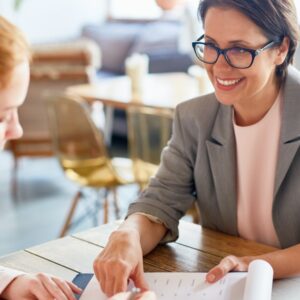
Voxco partners with Zamplia to launch Voxco Audience
Voxco Audience Announcement SHARE THE ARTICLE ON Share on facebook Share on twitter Share on linkedin Voxco, a leading provider of Omnichannel Survey Software, has
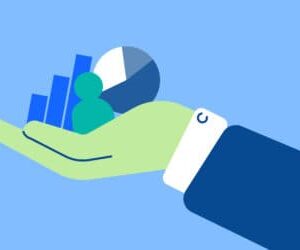
Humanizing Market Research
Humanizing Market Research Process: Connect With Your Audience SHARE THE ARTICLE ON Table of Contents When dealing with products, services, and enterprises, it can be
We use cookies in our website to give you the best browsing experience and to tailor advertising. By continuing to use our website, you give us consent to the use of cookies. Read More
Looking for the best research tools?
Voxco offers the best online & offline survey research tools!

- Basics of Research Process
- Methodology
- Research Design: Definition, Types, Characteristics & Study Examples
- Speech Topics
- Basics of Essay Writing
- Essay Topics
- Other Essays
- Main Academic Essays
- Research Paper Topics
- Basics of Research Paper Writing
- Miscellaneous
- Chicago/ Turabian
- Data & Statistics
- Admission Writing Tips
- Admission Advice
- Other Guides
- Student Life
- Studying Tips
- Understanding Plagiarism
- Academic Writing Tips
- Basics of Dissertation & Thesis Writing

- Essay Guides
- Research Paper Guides
- Formatting Guides
- Admission Guides
- Dissertation & Thesis Guides
Research Design: Definition, Types, Characteristics & Study Examples

Table of contents

Use our free Readability checker
A research design is the blueprint for any study. It's the plan that outlines how the research will be carried out. A study design usually includes the methods of data collection, the type of data to be gathered, and how it will be analyzed. Research designs help ensure the study is reliable, valid, and can answer the research question.
Behind every groundbreaking discovery and innovation lies a well-designed research. Whether you're investigating a new technology or exploring a social phenomenon, a solid research design is key to achieving reliable results. But what exactly does it means, and how do you create an effective one? Stay with our paper writers and find out:
- Detailed definition
- Types of research study designs
- How to write a research design
- Useful examples.
Whether you're a seasoned researcher or just getting started, understanding the core principles will help you conduct better studies and make more meaningful contributions.
What Is a Research Design: Definition
Research design is an overall study plan outlining a specific approach to investigating a research question . It covers particular methods and strategies for collecting, measuring and analyzing data. Students are required to build a study design either as an individual task or as a separate chapter in a research paper , thesis or dissertation .
Before designing a research project, you need to consider a series aspects of your future study:
- Research aims What research objectives do you want to accomplish with your study? What approach will you take to get there? Will you use a quantitative, qualitative, or mixed methods approach?
- Type of data Will you gather new data (primary research), or rely on existing data (secondary research) to answer your research question?
- Sampling methods How will you pick participants? What criteria will you use to ensure your sample is representative of the population?
- Data collection methods What tools or instruments will you use to gather data (e.g., conducting a survey , interview, or observation)?
- Measurement What metrics will you use to capture and quantify data?
- Data analysis What statistical or qualitative techniques will you use to make sense of your findings?
By using a well-designed research plan, you can make sure your findings are solid and can be generalized to a larger group.
Research design example
You are going to investigate the effectiveness of a mindfulness-based intervention for reducing stress and anxiety among college students. You decide to organize an experiment to explore the impact. Participants should be randomly assigned to either an intervention group or a control group. You need to conduct pre- and post-intervention using self-report measures of stress and anxiety.
What Makes a Good Study Design?
To design a research study that works, you need to carefully think things through. Make sure your strategy is tailored to your research topic and watch out for potential biases. Your procedures should be flexible enough to accommodate changes that may arise during the course of research.
A good research design should be:
- Clear and methodologically sound
- Feasible and realistic
- Knowledge-driven.
By following these guidelines, you'll set yourself up for success and be able to produce reliable results.
Research Study Design Structure
A structured research design provides a clear and organized plan for carrying out a study. It helps researchers to stay on track and ensure that the study stays within the bounds of acceptable time, resources, and funding.
A typical design includes 5 main components:
- Research question(s): Central research topic(s) or issue(s).
- Sampling strategy: Method for selecting participants or subjects.
- Data collection techniques: Tools or instruments for retrieving data.
- Data analysis approaches: Techniques for interpreting and scrutinizing assembled data.
- Ethical considerations: Principles for protecting human subjects (e.g., obtaining a written consent, ensuring confidentiality guarantees).
Research Design Essential Characteristics
Creating a research design warrants a firm foundation for your exploration. The cost of making a mistake is too high. This is not something scholars can afford, especially if financial resources or a considerable amount of time is invested. Choose the wrong strategy, and you risk undermining your whole study and wasting resources.
To avoid any unpleasant surprises, make sure your study conforms to the key characteristics. Here are some core features of research designs:
- Reliability Reliability is stability of your measures or instruments over time. A reliable research design is one that can be reproduced in the same way and deliver consistent outcomes. It should also nurture accurate representations of actual conditions and guarantee data quality.
- Validity For a study to be valid , it must measure what it claims to measure. This means that methodological approaches should be carefully considered and aligned to the main research question(s).
- Generalizability Generalizability means that your insights can be practiced outside of the scope of a study. When making inferences, researchers must take into account determinants such as sample size, sampling technique, and context.
- Neutrality A study model should be free from personal or cognitive biases to ensure an impartial investigation of a research topic. Steer clear of highlighting any particular group or achievement.
Key Concepts in Research Design
Now let’s discuss the fundamental principles that underpin study designs in research. This will help you develop a strong framework and make sure all the puzzles fit together.
Primary concepts
Types of Approaches to Research Design
Study frameworks can fall into 2 major categories depending on the approach to compiling data you opt for. The 2 main types of study designs in research are qualitative and quantitative research. Both approaches have their unique strengths and weaknesses, and can be utilized based on the nature of information you are dealing with.
Quantitative Research
Quantitative study is focused on establishing empirical relationships between variables and collecting numerical data. It involves using statistics, surveys, and experiments to measure the effects of certain phenomena. This research design type looks at hard evidence and provides measurements that can be analyzed using statistical techniques.
Qualitative Research
Qualitative approach is used to examine the behavior, attitudes, and perceptions of individuals in a given environment. This type of study design relies on unstructured data retrieved through interviews, open-ended questions and observational methods.
If you need your study done yesterday, leave StudyCrumb a “ write my research paper for me ” notice and have your project completed by experts.
Types of Research Designs & Examples
Choosing a research design may be tough especially for the first-timers. One of the great ways to get started is to pick the right design that will best fit your objectives. There are 4 different types of research designs you can opt for to carry out your investigation:
- Experimental
- Correlational
- Descriptive
- Diagnostic/explanatory.
Below we will go through each type and offer you examples of study designs to assist you with selection.
1. Experimental
In experimental research design , scientists manipulate one or more independent variables and control other factors in order to observe their effect on a dependent variable. This type of research design is used for experiments where the goal is to determine a causal relationship.
Its core characteristics include:
- Randomization
- Manipulation
- Replication.
A pharmaceutical company wants to test a new drug to investigate its effectiveness in treating a specific medical condition. Researchers would randomly assign participants to either a control group (receiving a placebo) or an experimental group (receiving the new drug). They would rigorously control all variables (e.g, age, medical history) and manipulate them to get reliable results.
2. Correlational
Correlational study is used to examine the existing relationships between variables. In this type of design, you don’t need to manipulate other variables. Here, researchers just focus on observing and measuring the naturally occurring relationship.
Correlational studies encompass such features:
- Data collection from natural settings
- No intervention by the researcher
- Observation over time.
A research team wants to examine the relationship between academic performance and extracurricular activities. They would observe students' performance in courses and measure how much time they spend engaging in extracurricular activities.
3. Descriptive
Descriptive research design is all about describing a particular population or phenomenon without any interruption. This study design is especially helpful when we're not sure about something and want to understand it better.
Descriptive studies are characterized by such features:
- Random and convenience sampling
- Observation
- No intervention.
A psychologist wants to understand how parents' behavior affects their child's self-concept. They would observe the interaction between children and their parents in a natural setting. Gathered information will help her get an overview of this situation and recognize some patterns.
4. Diagnostic
Diagnostic or explanatory research is used to determine the cause of an existing problem or a chronic symptom. Unlike other types of design, here scientists try to understand why something is happening.
Among essential hallmarks of explanatory studies are:
- Testing hypotheses and theories
- Examining existing data
- Comparative analysis.
A public health specialist wants to identify the cause of an outbreak of water-borne disease in a certain area. They would inspect water samples and records to compare them with similar outbreaks in other areas. This will help to uncover reasons behind this accident.
How to Design a Research Study: Step-by-Step Process
When designing your research don't just jump into it. It's important to take the time and do things right in order to attain accurate findings. Follow these simple steps on how to design a study to get the most out of your project.
1. Determine Your Aims
The first step in the research design process is figuring out what you want to achieve. This involves identifying your research question, goals and specific objectives you want to accomplish. Think whether you want to explore a specific issue or develop a new theory? Setting your aims from the get-go will help you stay focused and ensure that your study is driven by purpose.
Once you are clear with your goals, you need to decide on the main approach. Will you use qualitative or quantitative methods? Or perhaps a mixture of both?
2. Select a Type of Research Design
Choosing a suitable design requires considering multiple factors, such as your research question, data collection methods, and resources. There are various research design types, each with its own advantages and limitations. Think about the kind of data that would be most useful to address your questions. Ultimately, a well-devised strategy should help you gather accurate data to achieve your objectives.
3. Define Your Population and Sampling Methods
To design a research project, it is essential to establish your target population and parameters for selecting participants. First, identify a cohort of individuals who share common characteristics and possess relevant experiences.
For instance, if you are researching the impact of social media on mental health, your population could be young adults aged 18-25 who use social media frequently.
With your population in mind, you can now choose an optimal sampling method. Sampling is basically the process of narrowing down your target group to only those individuals who will participate in your study. At this point, you need to decide on whether you want to randomly choose the participants (probability sampling) or set out any selection criteria (non-probability sampling).
To examine the influence of social media on mental well-being, we will divide a whole population into smaller subgroups using stratified random sampling . Then, we will randomly pick participants from each subcategory to make sure that findings are also true for a broader group of young adults.
4. Decide on Your Data Collection Methods
When devising your study, it is also important to consider how you will retrieve data. Depending on the type of design you are using, you may deploy diverse methods. Below you can see various data collection techniques suited for different research designs.
Data collection methods in various studies
Additionally, if you plan on integrating existing data sources like medical records or publicly available datasets, you want to mention this as well.
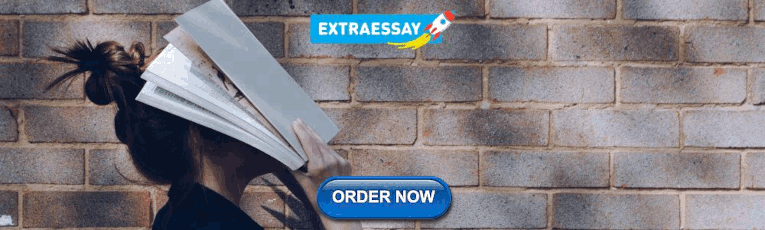
5. Arrange Your Data Collection Process
Your data collection process should also be meticulously thought out. This stage involves scheduling interviews, arranging questionnaires and preparing all the necessary tools for collecting information from participants. Detail how long your study will take and what procedures will be followed for recording and analyzing the data.
State which variables will be studied and what measures or scales will be used when assessing each variable.
Measures and scales
Measures and scales are tools used to quantify variables in research. A measure is any method used to collect data on a variable, while a scale is a set of items or questions used to measure a particular construct or concept. Different types of scales include nominal, ordinal, interval, or ratio , each of which has distinct properties
Operationalization
When working with abstract information that needs to be quantified, researchers often operationalize the variable by defining it in concrete terms that can be measured or observed. This allows the abstract concept to be studied systematically and rigorously.
Operationalization in study design example
If studying the concept of happiness, researchers might operationalize it by using a scale that measures positive affect or life satisfaction. This allows us to quantify happiness and inspect its relationship with other variables, such as income or social support.
Remember that research design should be flexible enough to adjust for any unforeseen developments. Even with rigorous preparation, you may still face unexpected challenges during your project. That’s why you need to work out contingency plans when designing research.
6. Choose Data Analysis Techniques
It’s impossible to design research without mentioning how you are going to scrutinize data. To select a proper method, take into account the type of data you are dealing with and how many variables you need to analyze.
Qualitative data may require thematic analysis or content analysis.
Quantitative data, on the other hand, could be processed with more sophisticated statistical analysis approaches such as regression analysis, factor analysis or descriptive statistics.
Finally, don’t forget about ethical considerations. Opt for those methods that minimize harm to participants and protect their rights.
Research Design Checklist
Having a checklist in front of you will help you design your research flawlessly.
Bottom Line on Research Design & Study Types
Designing a research project involves making countless decisions that can affect the quality of your work. By planning out each step and selecting the best methods for data collection and analysis, you can ensure that your project is conducted professionally.
We hope this article has helped you to better understand the research design process. If you have any questions or comments, ping us in the comments section below.

Entrust us your study and we will find the best research paper writer to complete your project. Count on a unique work and fast turnaround.

Joe Eckel is an expert on Dissertations writing. He makes sure that each student gets precious insights on composing A-grade academic writing.
You may also like

- checkbox I clearly defined my research question and its significance.
- checkbox I considered crucial factors such as the nature of my study, type of required data and available resources to choose a suitable design.
- checkbox A sample size is sufficient to provide statistically significant results.
- checkbox My data collection methods are reliable and valid.
- checkbox Analysis methods are appropriate for the type of data I will be gathering.
- checkbox My research design protects the rights and privacy of my participants.
- checkbox I created a realistic timeline for research, including deadlines for data collection, analysis, and write-up.
- checkbox I considered funding sources and potential limitations.
For more advanced studies, you can even combine several types. Mixed-methods research may come in handy when exploring complex phenomena that cannot be adequately captured by one method alone.
FAQ About Research Study Designs
1. what is a study design.
Study design, or else called research design, is the overall plan for a project, including its purpose, methodology, data collection and analysis techniques. A good design ensures that your project is conducted in an organized and ethical manner. It also provides clear guidelines for replicating or extending a study in the future.
2. What is the purpose of a research design?
The purpose of a research design is to provide a structure and framework for your project. By outlining your methodology, data collection techniques, and analysis methods in advance, you can ensure that your project will be conducted effectively.
3. What is the importance of research designs?
Research designs are critical to the success of any research project for several reasons. Specifically, study designs grant:
- Clear direction for all stages of a study
- Validity and reliability of findings
- Roadmap for replication or further extension
- Accurate results by controlling for potential bias
- Comparison between studies by providing consistent guidelines.
By following an established plan, researchers can be sure that their projects are organized, ethical, and reliable.
4. What are the 4 types of study designs?
There are generally 4 types of study designs commonly used in research:
- Experimental studies: investigate cause-and-effect relationships by manipulating the independent variable.
- Correlational studies: examine relationships between 2 or more variables without intruding them.
- Descriptive studies: describe the characteristics of a population or phenomenon without making any inferences about cause and effect.
- Explanatory studies: intended to explain causal relationships.
- Privacy Policy

Home » Descriptive Research Design – Types, Methods and Examples
Descriptive Research Design – Types, Methods and Examples
Table of Contents

Descriptive Research Design
Definition:
Descriptive research design is a type of research methodology that aims to describe or document the characteristics, behaviors, attitudes, opinions, or perceptions of a group or population being studied.
Descriptive research design does not attempt to establish cause-and-effect relationships between variables or make predictions about future outcomes. Instead, it focuses on providing a detailed and accurate representation of the data collected, which can be useful for generating hypotheses, exploring trends, and identifying patterns in the data.
Types of Descriptive Research Design
Types of Descriptive Research Design are as follows:
Cross-sectional Study
This involves collecting data at a single point in time from a sample or population to describe their characteristics or behaviors. For example, a researcher may conduct a cross-sectional study to investigate the prevalence of certain health conditions among a population, or to describe the attitudes and beliefs of a particular group.
Longitudinal Study
This involves collecting data over an extended period of time, often through repeated observations or surveys of the same group or population. Longitudinal studies can be used to track changes in attitudes, behaviors, or outcomes over time, or to investigate the effects of interventions or treatments.
This involves an in-depth examination of a single individual, group, or situation to gain a detailed understanding of its characteristics or dynamics. Case studies are often used in psychology, sociology, and business to explore complex phenomena or to generate hypotheses for further research.
Survey Research
This involves collecting data from a sample or population through standardized questionnaires or interviews. Surveys can be used to describe attitudes, opinions, behaviors, or demographic characteristics of a group, and can be conducted in person, by phone, or online.
Observational Research
This involves observing and documenting the behavior or interactions of individuals or groups in a natural or controlled setting. Observational studies can be used to describe social, cultural, or environmental phenomena, or to investigate the effects of interventions or treatments.
Correlational Research
This involves examining the relationships between two or more variables to describe their patterns or associations. Correlational studies can be used to identify potential causal relationships or to explore the strength and direction of relationships between variables.
Data Analysis Methods
Descriptive research design data analysis methods depend on the type of data collected and the research question being addressed. Here are some common methods of data analysis for descriptive research:
Descriptive Statistics
This method involves analyzing data to summarize and describe the key features of a sample or population. Descriptive statistics can include measures of central tendency (e.g., mean, median, mode) and measures of variability (e.g., range, standard deviation).
Cross-tabulation
This method involves analyzing data by creating a table that shows the frequency of two or more variables together. Cross-tabulation can help identify patterns or relationships between variables.
Content Analysis
This method involves analyzing qualitative data (e.g., text, images, audio) to identify themes, patterns, or trends. Content analysis can be used to describe the characteristics of a sample or population, or to identify factors that influence attitudes or behaviors.
Qualitative Coding
This method involves analyzing qualitative data by assigning codes to segments of data based on their meaning or content. Qualitative coding can be used to identify common themes, patterns, or categories within the data.
Visualization
This method involves creating graphs or charts to represent data visually. Visualization can help identify patterns or relationships between variables and make it easier to communicate findings to others.
Comparative Analysis
This method involves comparing data across different groups or time periods to identify similarities and differences. Comparative analysis can help describe changes in attitudes or behaviors over time or differences between subgroups within a population.
Applications of Descriptive Research Design
Descriptive research design has numerous applications in various fields. Some of the common applications of descriptive research design are:
- Market research: Descriptive research design is widely used in market research to understand consumer preferences, behavior, and attitudes. This helps companies to develop new products and services, improve marketing strategies, and increase customer satisfaction.
- Health research: Descriptive research design is used in health research to describe the prevalence and distribution of a disease or health condition in a population. This helps healthcare providers to develop prevention and treatment strategies.
- Educational research: Descriptive research design is used in educational research to describe the performance of students, schools, or educational programs. This helps educators to improve teaching methods and develop effective educational programs.
- Social science research: Descriptive research design is used in social science research to describe social phenomena such as cultural norms, values, and beliefs. This helps researchers to understand social behavior and develop effective policies.
- Public opinion research: Descriptive research design is used in public opinion research to understand the opinions and attitudes of the general public on various issues. This helps policymakers to develop effective policies that are aligned with public opinion.
- Environmental research: Descriptive research design is used in environmental research to describe the environmental conditions of a particular region or ecosystem. This helps policymakers and environmentalists to develop effective conservation and preservation strategies.
Descriptive Research Design Examples
Here are some real-time examples of descriptive research designs:
- A restaurant chain wants to understand the demographics and attitudes of its customers. They conduct a survey asking customers about their age, gender, income, frequency of visits, favorite menu items, and overall satisfaction. The survey data is analyzed using descriptive statistics and cross-tabulation to describe the characteristics of their customer base.
- A medical researcher wants to describe the prevalence and risk factors of a particular disease in a population. They conduct a cross-sectional study in which they collect data from a sample of individuals using a standardized questionnaire. The data is analyzed using descriptive statistics and cross-tabulation to identify patterns in the prevalence and risk factors of the disease.
- An education researcher wants to describe the learning outcomes of students in a particular school district. They collect test scores from a representative sample of students in the district and use descriptive statistics to calculate the mean, median, and standard deviation of the scores. They also create visualizations such as histograms and box plots to show the distribution of scores.
- A marketing team wants to understand the attitudes and behaviors of consumers towards a new product. They conduct a series of focus groups and use qualitative coding to identify common themes and patterns in the data. They also create visualizations such as word clouds to show the most frequently mentioned topics.
- An environmental scientist wants to describe the biodiversity of a particular ecosystem. They conduct an observational study in which they collect data on the species and abundance of plants and animals in the ecosystem. The data is analyzed using descriptive statistics to describe the diversity and richness of the ecosystem.
How to Conduct Descriptive Research Design
To conduct a descriptive research design, you can follow these general steps:
- Define your research question: Clearly define the research question or problem that you want to address. Your research question should be specific and focused to guide your data collection and analysis.
- Choose your research method: Select the most appropriate research method for your research question. As discussed earlier, common research methods for descriptive research include surveys, case studies, observational studies, cross-sectional studies, and longitudinal studies.
- Design your study: Plan the details of your study, including the sampling strategy, data collection methods, and data analysis plan. Determine the sample size and sampling method, decide on the data collection tools (such as questionnaires, interviews, or observations), and outline your data analysis plan.
- Collect data: Collect data from your sample or population using the data collection tools you have chosen. Ensure that you follow ethical guidelines for research and obtain informed consent from participants.
- Analyze data: Use appropriate statistical or qualitative analysis methods to analyze your data. As discussed earlier, common data analysis methods for descriptive research include descriptive statistics, cross-tabulation, content analysis, qualitative coding, visualization, and comparative analysis.
- I nterpret results: Interpret your findings in light of your research question and objectives. Identify patterns, trends, and relationships in the data, and describe the characteristics of your sample or population.
- Draw conclusions and report results: Draw conclusions based on your analysis and interpretation of the data. Report your results in a clear and concise manner, using appropriate tables, graphs, or figures to present your findings. Ensure that your report follows accepted research standards and guidelines.
When to Use Descriptive Research Design
Descriptive research design is used in situations where the researcher wants to describe a population or phenomenon in detail. It is used to gather information about the current status or condition of a group or phenomenon without making any causal inferences. Descriptive research design is useful in the following situations:
- Exploratory research: Descriptive research design is often used in exploratory research to gain an initial understanding of a phenomenon or population.
- Identifying trends: Descriptive research design can be used to identify trends or patterns in a population, such as changes in consumer behavior or attitudes over time.
- Market research: Descriptive research design is commonly used in market research to understand consumer preferences, behavior, and attitudes.
- Health research: Descriptive research design is useful in health research to describe the prevalence and distribution of a disease or health condition in a population.
- Social science research: Descriptive research design is used in social science research to describe social phenomena such as cultural norms, values, and beliefs.
- Educational research: Descriptive research design is used in educational research to describe the performance of students, schools, or educational programs.
Purpose of Descriptive Research Design
The main purpose of descriptive research design is to describe and measure the characteristics of a population or phenomenon in a systematic and objective manner. It involves collecting data that describe the current status or condition of the population or phenomenon of interest, without manipulating or altering any variables.
The purpose of descriptive research design can be summarized as follows:
- To provide an accurate description of a population or phenomenon: Descriptive research design aims to provide a comprehensive and accurate description of a population or phenomenon of interest. This can help researchers to develop a better understanding of the characteristics of the population or phenomenon.
- To identify trends and patterns: Descriptive research design can help researchers to identify trends and patterns in the data, such as changes in behavior or attitudes over time. This can be useful for making predictions and developing strategies.
- To generate hypotheses: Descriptive research design can be used to generate hypotheses or research questions that can be tested in future studies. For example, if a descriptive study finds a correlation between two variables, this could lead to the development of a hypothesis about the causal relationship between the variables.
- To establish a baseline: Descriptive research design can establish a baseline or starting point for future research. This can be useful for comparing data from different time periods or populations.
Characteristics of Descriptive Research Design
Descriptive research design has several key characteristics that distinguish it from other research designs. Some of the main characteristics of descriptive research design are:
- Objective : Descriptive research design is objective in nature, which means that it focuses on collecting factual and accurate data without any personal bias. The researcher aims to report the data objectively without any personal interpretation.
- Non-experimental: Descriptive research design is non-experimental, which means that the researcher does not manipulate any variables. The researcher simply observes and records the behavior or characteristics of the population or phenomenon of interest.
- Quantitative : Descriptive research design is quantitative in nature, which means that it involves collecting numerical data that can be analyzed using statistical techniques. This helps to provide a more precise and accurate description of the population or phenomenon.
- Cross-sectional: Descriptive research design is often cross-sectional, which means that the data is collected at a single point in time. This can be useful for understanding the current state of the population or phenomenon, but it may not provide information about changes over time.
- Large sample size: Descriptive research design typically involves a large sample size, which helps to ensure that the data is representative of the population of interest. A large sample size also helps to increase the reliability and validity of the data.
- Systematic and structured: Descriptive research design involves a systematic and structured approach to data collection, which helps to ensure that the data is accurate and reliable. This involves using standardized procedures for data collection, such as surveys, questionnaires, or observation checklists.
Advantages of Descriptive Research Design
Descriptive research design has several advantages that make it a popular choice for researchers. Some of the main advantages of descriptive research design are:
- Provides an accurate description: Descriptive research design is focused on accurately describing the characteristics of a population or phenomenon. This can help researchers to develop a better understanding of the subject of interest.
- Easy to conduct: Descriptive research design is relatively easy to conduct and requires minimal resources compared to other research designs. It can be conducted quickly and efficiently, and data can be collected through surveys, questionnaires, or observations.
- Useful for generating hypotheses: Descriptive research design can be used to generate hypotheses or research questions that can be tested in future studies. For example, if a descriptive study finds a correlation between two variables, this could lead to the development of a hypothesis about the causal relationship between the variables.
- Large sample size : Descriptive research design typically involves a large sample size, which helps to ensure that the data is representative of the population of interest. A large sample size also helps to increase the reliability and validity of the data.
- Can be used to monitor changes : Descriptive research design can be used to monitor changes over time in a population or phenomenon. This can be useful for identifying trends and patterns, and for making predictions about future behavior or attitudes.
- Can be used in a variety of fields : Descriptive research design can be used in a variety of fields, including social sciences, healthcare, business, and education.
Limitation of Descriptive Research Design
Descriptive research design also has some limitations that researchers should consider before using this design. Some of the main limitations of descriptive research design are:
- Cannot establish cause and effect: Descriptive research design cannot establish cause and effect relationships between variables. It only provides a description of the characteristics of the population or phenomenon of interest.
- Limited generalizability: The results of a descriptive study may not be generalizable to other populations or situations. This is because descriptive research design often involves a specific sample or situation, which may not be representative of the broader population.
- Potential for bias: Descriptive research design can be subject to bias, particularly if the researcher is not objective in their data collection or interpretation. This can lead to inaccurate or incomplete descriptions of the population or phenomenon of interest.
- Limited depth: Descriptive research design may provide a superficial description of the population or phenomenon of interest. It does not delve into the underlying causes or mechanisms behind the observed behavior or characteristics.
- Limited utility for theory development: Descriptive research design may not be useful for developing theories about the relationship between variables. It only provides a description of the variables themselves.
- Relies on self-report data: Descriptive research design often relies on self-report data, such as surveys or questionnaires. This type of data may be subject to biases, such as social desirability bias or recall bias.
About the author
Muhammad Hassan
Researcher, Academic Writer, Web developer
You may also like

Questionnaire – Definition, Types, and Examples

Case Study – Methods, Examples and Guide

Observational Research – Methods and Guide

Quantitative Research – Methods, Types and...

Qualitative Research Methods

Explanatory Research – Types, Methods, Guide
- Open access
- Published: 15 May 2024
Alzheimer blood biomarkers: practical guidelines for study design, sample collection, processing, biobanking, measurement and result reporting
- Xuemei Zeng 1 ,
- Yijun Chen 2 ,
- Anuradha Sehrawat 1 ,
- Jihui Lee 1 ,
- Tara K. Lafferty 1 ,
- Julia Kofler 3 ,
- Sarah B. Berman 4 ,
- Robert A. Sweet 1 , 4 ,
- Dana L. Tudorascu 1 ,
- William E. Klunk 1 ,
- Milos D. Ikonomovic 1 , 4 , 5 ,
- Anna Pfister 6 , 7 ,
- Henrik Zetterberg 6 , 7 , 8 , 9 , 10 , 11 ,
- Beth E. Snitz 4 ,
- Anne D. Cohen 1 ,
- Victor L. Villemagne 1 ,
- Tharick A. Pascoal 1 , 4 ,
- M. llyas Kamboh 12 ,
- Oscar I. Lopez 4 ,
- Kaj Blennow 6 , 7 &
- Thomas K. Karikari ORCID: orcid.org/0000-0003-1422-4358 1 , 6
Molecular Neurodegeneration volume 19 , Article number: 40 ( 2024 ) Cite this article
16 Altmetric
Metrics details
Alzheimer’s disease (AD), the most common form of dementia, remains challenging to understand and treat despite decades of research and clinical investigation. This might be partly due to a lack of widely available and cost-effective modalities for diagnosis and prognosis. Recently, the blood-based AD biomarker field has seen significant progress driven by technological advances, mainly improved analytical sensitivity and precision of the assays and measurement platforms. Several blood-based biomarkers have shown high potential for accurately detecting AD pathophysiology. As a result, there has been considerable interest in applying these biomarkers for diagnosis and prognosis, as surrogate metrics to investigate the impact of various covariates on AD pathophysiology and to accelerate AD therapeutic trials and monitor treatment effects. However, the lack of standardization of how blood samples and collected, processed, stored analyzed and reported can affect the reproducibility of these biomarker measurements, potentially hindering progress toward their widespread use in clinical and research settings. To help address these issues, we provide fundamental guidelines developed according to recent research findings on the impact of sample handling on blood biomarker measurements. These guidelines cover important considerations including study design, blood collection, blood processing, biobanking, biomarker measurement, and result reporting. Furthermore, the proposed guidelines include best practices for appropriate blood handling procedures for genetic and ribonucleic acid analyses. While we focus on the key blood-based AD biomarkers for the AT(N) criteria (e.g., amyloid-beta [Aβ]40, Aβ42, Aβ42/40 ratio, total-tau, phosphorylated-tau, neurofilament light chain, brain-derived tau and glial fibrillary acidic protein), we anticipate that these guidelines will generally be applicable to other types of blood biomarkers. We also anticipate that these guidelines will assist investigators in planning and executing biomarker research, enabling harmonization of sample handling to improve comparability across studies.
Alzheimer’s disease (AD), the most common form of dementia, poses significant economic and social burden on affected individuals, as well as their families, caregivers, communities, and healthcare systems worldwide [ 1 ]. An estimated excess of 50 million people are living with AD globally, including 6.7 million in the United States. By 2050, these numbers are expected to rise to 152 million and 13.8 million, respectively [ 2 , 3 ]. Neuropathologically, AD is characterized by two hallmark lesions in the brain; amyloid-β (Aβ) plaques and tau neurofibrillary tangles [ 4 , 5 ]. Most individuals with brain pathophysiological evidence of AD clinically show progressive cognitive impairment [ 6 ]. Unfortunately, despite decades of research and numerous clinical trials, AD remains difficult to treat, with only a few FDA-approved drugs available for treatment [ 1 ]. Among them, lecanemab, donanemab and aducanumab, humanized antibodies designed to reduce the amyloid plaque burden, are the only ones expected to provide disease-modifying therapy [ 7 , 8 , 9 ]. The other drugs are palliative treatments that reduce the symptoms temporarily but are not directed toward preventing or slowing disease progression.
The slow pace of AD drug development is partly due to a lack of accessible and cost-effective biomarkers for participant enrollment and stratification in clinical trials. The National Institute on Aging and the Alzheimer’s Association (NIA-AA) research framework recommends the use of biomarker criteria for amyloid pathology, tau pathology, and neurodegeneration [AT(N)] for a biological definition of AD [ 10 ]. However, these assessments are currently performed using expensive, time-consuming, and sometimes invasive procedures with limited global accessibility such as magnetic resonance imaging (MRI), positron emission tomography (PET) scans, and/or cerebrospinal fluid (CSF) biomarkers [ 11 , 12 , 13 ], which are unsuitable for large-scale clinical applications and population screenings. It has been estimated that screening a single participant for AD clinical trials with PET and MRI could cost at least US $8,000 [ 14 ]. Given the typically high screen-failure rate (percentage of screened participants not meeting the enrollment criteria), it is not surprising that participant screening may cost 50–70% of total per-participant costs [ 14 ]. These costs could be prohibitive for large-scale clinical trials. In terms of clinical applications, imaging all patients with suspected cognitive impairment due to AD using PET and MRI would be difficult to achieve due to the low throughput and the limited availability of the specialized facilities and expertise needed to administer and interpret these tests [ 13 ].
To address this issue, there is a growing need to develop less invasive, more cost-effective, and scalable biomarkers that can reliably identify AD pathology. Blood-based AD biomarkers are a desirable choice due to availability of blood specimens through routine clinical practice and research programs. In clinical trials, blood biomarkers have already shown utility as pre-screening measures to streamline the identification and inclusion of individuals who fit pre-defined criteria for biological evidence of disease [ 15 , 16 ]. Importantly, clinical prescriptions of the recent FDA-approved anti-amyloid drugs require prior confirmation of brain amyloidosis. However, since amyloid PET and CSF Aβ42/Aβ 40 assessments are not feasible in many hospital settings, blood biomarkers would be very useful proxies.
The development of such biomarkers has been hindered by the extreme complexity of the blood proteome, low biomarker abundance, and signal dilution from peripheral tissues. However, significant advances have been achieved in the past decade, benefiting partly from the development of ultra-sensitive immunoassays and high-performance mass spectrometry technology platforms [ 17 , 18 , 19 , 20 ]. Blood-based biomarkers with high potential of providing accurate assessment of the AT(N) criteria include the Aβ42/40 ratio for amyloid pathology, phosphorylated tau (p-tau) for tau pathology, and neurofilament light-chain (NfL) and brain-derived tau for neurodegeneration/axonal injury [ 11 , 21 ]. In addition, plasma glial fibrillary acidic protein (GFAP), an indicator of reactive astrogliosis often associated with brain Aβ plaques, has also been proposed as an early marker for amyloid pathology [ 22 , 23 , 24 , 25 ].
The anticipated next stage in the development of highly sensitive and specific blood biomarkers for AD is to employ them in real-world settings for clinical diagnosis, population studies, and eligibility screening for therapeutic trials. However, a major challenge facing the field is the need for increased standardization of collection, processing, and storage procedures. Another important challenge is the need for agreed-upon procedures to monitor and maintain long-term stability in the biomarker measurements, especially since none of the blood-based AD biomarkers in use currently has certified reference measurement procedures. These obstacles must be overcome before blood-based biomarkers can be effectively adopted in clinical and research-based settings, and these measurements can be appropriately harmonized. A survey of studies across fifteen centers revealed variations in sample processing, such as the time of day for collection, fasting status, time from collection to centrifugation, the temperature at various steps, and centrifugation parameters [ 26 ]. The lack of standardization can introduce measurement variations, reducing clinical reliability and making it challenging to compare results across laboratories and establish clinical thresholds. These variations can be introduced at three phases: preanalytical, analytical, and post-analytical. The advancement of analytical technologies, such as automation in sample preparation, has dramatically reduced errors in the analytical phase. It is now thought that the preanalytical stage is the most error-prone phase (over 60%), followed by the post-analytical phase (over 20%) [ 27 ].
Decades of research have led to the development of a consensus protocol for standardized collection and biobanking of CSF-based AD biomarkers [ 28 ], which has been key to the much improved inter-laboratory reproducibility of the core CSF biomarkers in recent years [ 29 ]. Blood-based AD biomarkers often have smaller effect sizes than the corresponding CSF biomarkers (with the exception of GFAP) [ 25 ], possibly due to signal attenuation caused by counterpart proteins secreted or biomarkers sequestered by peripheral tissues and the increased biological complexity of blood [ 13 , 30 ] (further discussed below). Therefore, minimizing preanalytical variations is even more critical for blood-based AD biomarkers. O’Bryant et al., in 2015, proposed a set of guidelines to standardize blood sample collection [ 31 ]. Yet, the evidence evaluating defined preanalytical factors was limited at that time. Since then, many research studies have been published, and an updated evidence-based plasma handling standardized operating procedure (SOP) was proposed in 2022 by Verberk et al. [ 26 ]. However, this SOP was limited to preanalytical factors and was directed at experienced blood biomarker laboratories and scientists. As blood biomarkers become more widely available and simplified commercial technologies get increasingly accessible, fundamental guidelines that: (1) take into account the preanalytical, analytical, and post-analytical pipeline; (2) accommodate the needs of investigators new to the blood biomarker space; and (3) are applicable to both traditional (blood collection and assessments in a clinical setting) and non-traditional (home collections, population-based evaluations, resource-limited settings) environments, are needed.
Standardization of blood AD biomarker research across sites, studies and investigators must consider several steps, including study design, blood collection, blood processing, biobanking, biomarker measurement, and result reporting (Fig. 1 ). This review aims to expand on earlier guidelines by employing the evidence base from recent research findings and our own experiences to provide a detailed description of the general considerations associated with each of these steps. Furthermore, we present an easy-to-follow SOP to aid in the design and implementation of high-quality blood-based AD biomarker research projects, covering the preanalytical, analytical, and post-analytical phases. It is worth noting that although we focus on the key blood-based AD biomarkers for the AT(N) criteria (e.g., Aβ40, Aβ42, Aβ42/40 ratio, total-tau (t-tau), p-tau, NfL, brain-derived tau [BD-tau] and GFAP), we anticipate that these guidelines will generally be applicable to other types of blood biomarkers as well as discovery proteomic investigations.
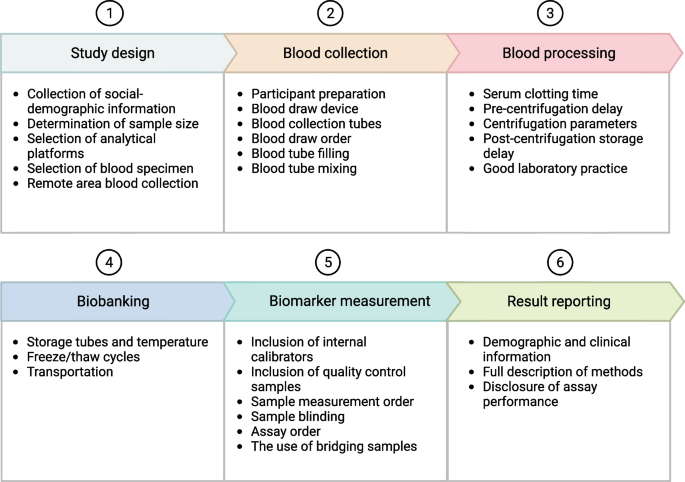
Proposed general workflow for conducting high-quality blood AD biomarker research and the important considerations associated with each step. The major steps are divided into six, namely study design, blood collection, blood processing, biobanking, biomarker measurement and results reporting. The important considerations under each step are listed in the Figure. Detailed discussions are provided in the text
Considerations during study design
Item 1: collection of sociodemographic, lifestyle, and health information.
Numerous sociodemographic, lifestyle, health, and environmental factors have been associated with an increased risk of AD [ 32 , 33 , 34 ]. For example, age, education, sex, race, creatinine levels, concomitant medication (including anti-amyloid therapies), medical history, and comorbidities such as diabetes, hypertension, impaired kidney function, liver diseases, and cardiovascular diseases have been found to be significantly associated with blood AD biomarkers levels in some research cohorts compared with unaffected controls [ 7 , 35 , 36 , 37 , 38 , 39 , 40 , 41 , 42 , 43 ]. Additionally, conditions such as pregnancy, menopausal symptoms and hormone therapy use are critical as biomarker changes have been reported [ 44 , 45 ] but require further investigation. While it may not be feasible to include all demographic and clinical information, we recommend recording as much information as possible during subject recruitment and through the medical record extraction to prevent over-/under-interpretation of results and to enable adjustment of reference ranges according to population characteristics if needed. Importantly, the collection, processing, storage, and application of personal data must conform with the prevailing ethical guidelines and legal frameworks in the countries concerned. For example, there is the General Data Protection Regulation (GDPR) in Europe and Health Insurance Portability and Accountability Act of 1996 (HIPAA) in the United States.
Item 2: sample size consideration
Sample size planning is critical in studies evaluating the diagnostic and prognostic capabilities of blood AD biomarkers and the impact of covariates. Insufficient sample size reduces the power to detect true significance. In contrast, excess sample size may magnify the importance of clinically non-meaningful differences, increase costs, prolong the study, and potentially expose more participants to needless evaluations [ 46 ]. Blood AD biomarkers often have smaller effect sizes than CSF biomarkers due to the expected dilution effect from peripheral tissues. Aβ42/40 ratio, for example, has reduced difference between Aβ+ vs. Aβ- individuals from ~ 40% in CSF to ~ 10% in blood [ 13 , 25 , 30 ]. Similarly, p-tau fold change is comparatively decreased from 166% in CSF to 85.6% in the blood [ 13 ], despite plasma p-tau having larger effect sizes than plasma Aβ42/40 ratio when compared directly [ 25 , 47 ]. Therefore, blood AD biomarker studies will require larger sample sizes than CSF biomarker studies. To determine the optimal sample size, one must consider factors such as expected effect size, population variance, desirable type I and type II error rates, participant dropout rate for longitudinal studies, and adjustment of covariates [ 46 ]. It is important to note that sample size calculation can become challenging for complex studies and will require statisticians’ advice during study design.
Item 3: selection of analytical platforms
Blood AD biomarkers tend to be near or below the detection limit of traditional enzyme-linked immunosorbent assays (ELISAs). However, in the past decade, many technologies have emerged that have significantly accelerated research in this area [ 18 , 19 , 48 , 49 , 50 , 51 , 52 , 53 , 54 , 55 , 56 , 57 , 58 , 59 , 60 , 61 , 62 ]. Among them, Single molecule array (Simoa), mass spectrometry, as well as immunoassay technologies available on platforms such as the Elecsys and Cobas systems from Roche Diagnostics, Meso Scale Discovery (MSD), and immunomagnetic reduction (MagQu) are the most used. Recently, the Lumipulse G system, widely used for running electrochemiluminescence (ECL) immunoassays in CSF samples for research and in vitro diagnostic purposes, has also moved into the blood biomarker space, with assays now available for plasma Aβ peptides, NfL, p-tau181 and p-tau217 [ 63 ]. In addition, Ella, a novel platform for running multi-analyte automated microfluidic immunoassays (Simple Plex™) has now entered the AD biomarker field, with assays available for plasma NfL [ 64 , 65 ]. Emerging platforms include Nano Mosaic and NULISA. We have summarized in Table 1 the commonly used platforms for the key AD blood biomarkers, but the list is not meant to be exhaustive. For more detailed descriptions, readers should refer to recent review articles [ 20 , 66 , 67 , 68 ] and references therein.
Extensive studies have been conducted to examine associations of blood biomarkers with AD pathology and compare the performance of different platforms. While a comprehensive review of the literature is beyond the scope of this work, a brief summary of the key findings is provided below:
Amyloid plaques, one of the primary pathological features of AD, consist mainly of amyloid beta peptides [ 77 , 78 ]. While CSF Aβ42/40 has been used in clinical settings to assess brain Aβ plaques, the association of blood Aβ42/40 with AD pathologies has been controversial [ 79 , 80 ]. Several immunoassays and MS assays are available to measure blood Aβ peptides [ 20 ], but overall, there is low inter-platform reproducibility [ 75 , 81 ]. MS assays generally exhibit superior predictive power for brain Aβ compared to immunoassays, possibly due to higher specificity obtained through MS assays [ 81 ].
CSF t-tau is a biomarker for neurodegeneration or neuronal injury [ 82 ]. However, plasma t-tau shows low correlation with CSF t-tau due to potential contamination with tau from peripheral sources [ 83 , 84 ]. Improved plasma t-tau assays have been reported recently [ 85 , 86 ]. In addition, recently developed Simoa assay targeting brain-derived tau showed a better correlation with CSF t-tau and improved biomarker performance [ 21 ].
CSF p-tau is a biomarker for neurofibrillary tangles [ 15 , 87 , 88 ]. Despite their low abundance in the blood, several assays are available to measure p-tau species in the blood [ 13 , 89 , 90 , 91 , 92 ]. Unlike plasma Aβ assays, p-tau assays exhibited overall strong inter-platform concordance [ 20 , 93 , 94 , 95 , 96 ]. P-tau181, p-tau217, and p-tau231 are the most widely studied p-tau species. P-tau212 is a new marker recently reported [ 97 ]. Different p-tau species might increase at different stages of the AD continuum [ 91 , 98 ]. Unlike their CSF counterparts, blood p-tau exhibits better association with Aβ plaques rather than neurofibrillary tangles.
GFAP is a biomarker for reactive astrogliosis [ 99 ], a cellular response often associated with brain Aβ plaque pathology in AD [ 100 ]. Plasma GFAP positively correlated with Aβ burden and tau pathology in AD [ 101 , 102 ]. Plasma GFAP level may be impacted by non-AD brain injuries and is an FDA-approved biomarker for detecting intracranial lesions after brain injury [ 103 ].
Neuronal damage/injury leads to elevated secretion of NfL into the extracellular space [ 104 ]. Although non-AD specific, NfL is an excellent biomarker for neurodegeneration to monitor the disease progression of AD patients [ 105 , 106 ]. Head-to-head comparison of Simoa and Ella assays in a multiple sclerosis cohort demonstrated a strong correlation between the platforms [ 64 , 65 ]. Plasma/serum brain-derived tau showed stronger specificity to AD pathophysiology versus related non-AD disorders.
Item 4: selection of blood specimen
Both plasma and serum have been utilized for measuring AD biomarkers. Studies comparing AD biomarker levels in serum and plasma have shown that some analytes, including Aβ peptides, t-tau, BD-tau and multiple p-tau species, are present at lower levels in serum, possibly due to a loss from clot trapping [ 26 , 107 , 108 , 109 , 110 , 111 ]. This makes it more challenging to measure such biomarkers in serum, especially for individuals whose biomarker levels are close to the lower detection limit. Nonetheless, biomarkers such as p-tau231, p-tau181 and BD-tau have been shown to have equivalent diagnostic accuracies in plasma and serum [ 21 , 89 , 107 , 109 , 111 ]. P-tau217 [ 112 ] and p-tau212 [ 97 ] are currently measurable in plasma but not serum. It is important to note that the choice of the blood specimen depends on the overall research objectives and sample availability. For example, serum may be a better choice for studies that evaluate the integrity of the blood-brain barrier since the CSF/serum albumin ratio is a well-established indicator of blood-CSF barrier function [ 113 ]. Additionally, serum is more widely used in hospital systems, with more clinical tests using serum instead of plasma as the specimen, according to the Mayo Clinic 2023 Test Catalog [ 114 ].
On the other hand, many research cohorts collect plasma instead of serum. Ethylenediaminetetraacetic acid (EDTA), heparin, and citrate are the most commonly used anticoagulants in clinics for plasma collection [ 115 ]. EDTA is the most universal in AD biomarker research [ 26 ]. Several studies have suggested that citrate plasma has lower levels of several biomarkers, including Aβ peptides, NfL, GFAP, and t-tau, compared with EDTA and heparin plasma [ 116 , 117 ]. However, studies comparing heparin vs. EDTA have generated mixed results. For example, one study found most biomarkers to be more abundant in heparin plasma than EDTA plasma [ 107 ], while another found higher levels of t-tau and p-tau181 but similar levels of Aβ40 and Aβ42 in heparin compared with EDTA plasma samples [ 118 ]. Rózga et al., on the other hand, reported that the levels of t-tau were significantly lower in heparin plasma [ 116 ].
Regardless of the type of blood specimen chosen, it is important to use the same type of specimen throughout the study. Although biomarkers may show a similar trend in different specimens, they are not necessarily linearly correlated in samples from all individuals. For example, despite strong correlations and similar diagnostic accuracy between paired serum and plasma p-tau levels, Kac et al. observed larger disagreements in samples with lower p-tau concentrations [ 109 ].
Item 5: blood collection from remote areas, under-resourced settings, or home care
Advanced laboratory equipment, such as ultra-low temperature freezers and centrifuges, are typically required for the processing of traditional venipuncture-based blood specimen. This can create significant obstacles for community-based studies utilizing home sampling, as well as for studies in remote areas, where access to such equipment may be limited. In addition, venipuncture may be difficult and painful for individuals with small or fragile veins [ 119 ]. To overcome these challenges, some research initiatives have explored alternative blood collection methods. For instance, Walter et al. compared Aβ40 and Aβ42 levels in conventional venous blood vs. capillary blood collected by finger insertion using microvettes. They found a good correlation between the two specimen types, despite slightly lower levels in capillary blood [ 120 ]. Similarly, Lombardi et al. and Simrén et al. investigated the use of dried blood spots (DBS) and dried plasma spots (DPS) for NfL measurement and found that NfL levels in both DBS and DPS samples correlated strongly with those in EDTA plasma samples with a stronger correlation observed for DPS samples [ 121 , 122 ].
Considerations during blood collection
Item 1: preparation of participants for the blood draw.
Studies examining the impact of pre-blood draw activities of participants, such as fasting, physical exercise, medication use, and the time of day for blood collection, are limited. However, preliminary evidence shows that some of these factors can impact blood biomarker levels. For example, Rózga et al. found that the levels of Aβ40 and Aβ42 were 5–9% higher in blood samples collected in the afternoon compared with those collected in the morning, with the opposite trend noted for t-tau [ 116 ]. Meyer et al. found significantly higher Aβ40 and Aβ42 levels in non-fasting blood when measured with Simoa assays [ 123 ]. Signal variation from plasma samples obtained on consecutive days from the same individual or within a cohort, which allows for the evaluation of short term fluctuation, have also been reported [ 124 ].
To minimize potential bias arising from these effects, we recommend pre-defining the participant preparation protocol upfront and following it throughout the study. Ideally, blood should be drawn at the same time of the day for all participants with the same fasting status throughout the study. If practical in the population under study, fasting blood samples may be more reliable in general. It is also important to record information such as blood draw time, fasting status, date and time of last meal, hours of sleep the previous night, pre-blood draw exercise activity, and medication use, to facilitate downstream interpretation of results.
Item 2: blood draw devices
Blood can be collected from participants in various ways, including venipuncture using an evacuated system (Vacutainer®), a syringe, butterfly needles, intravenous (IV) catheters, fingersticks, or heelsticks. The choice of blood draw methods depends on factors such as patient characteristics, the type of tests to be performed, and the preference and experience of clinical staff. Venipuncture using a needle and vacutainer tubes are the most used for routine blood draw. It is important to note that the devices used for the blood draw may influence the blood sample quality. For example, IV catheters and smaller-bore needles are sometimes used for patients with hard-to-access veins or when multiple blood draws over an extended period are needed. However, both have been found to have a higher hemolysis rate [ 125 , 126 ]. Additionally, lubricant coating and needle material, if released into the blood, can potentially contaminate the specimens, which have been shown to affect antigen-antibody binding in some immunoassays [ 127 ]. There is a lack of published research on the impact of blood draw devices on blood AD biomarker levels, aside from the type of blood collection tubes used (described below). To harmonize the blood collection procedures across different labs, O’Bryant et al. recommended using 21-gauge needles for blood draw in adults [ 31 ]. When possible, new straight needle venipuncture is preferred over the IV start. Any variation from standard procedures should be carefully documented.
Item 3: blood collection tubes
Most biomarker studies use evacuated tube systems for blood collection. There are many different brands of evacuated tube systems available, including BD’s Vacutainer®, Sarstedt’s Monovette®, and Greiner Bio-One’s VACUETTE®. Different blood collection tubes vary in materials, shape, size, additives used, and safety features. The color of the tube closure typically indicates the additives. We have listed some commonly used blood collection tubes and their intended clinical applications in Fig. 2 . Various components of the evacuated tubes, such as their surface coating, stopper materials, stopper lubricants, gel separators, and additives, can interfere with clinical laboratory assays and are potential sources for preanalytical variation [ 115 , 127 , 128 ]. For instance, gel separators – inert gels used as barriers for better separation of serum or plasma from cells/clots after centrifugation – have been shown to absorb blood constituents and interfere with various clinical tests for therapeutic drug monitoring [ 129 ]. It is worth noting that several studies indicated that AD biomarkers, especially Aβ peptides in the CSF matrix, may exhibit varying absorption rates to tube walls based on the materials they are made of. Specifically, there is a higher overall loss when polystyrene tubes are utilized [ 130 , 131 , 132 ]. Pre-treating the tubes with detergent Tween-20 might mitigate the absorption [ 133 , 134 ]. However, currently, there is no evidence to suggest that the primary materials used in blood tubes have any effect on AD biomarker levels [ 116 , 135 ]. To reduce the risk of variability caused by using different types of tubes, we suggest using the same brand of tubes consistently throughout the studies and limiting the number of lots to as few as possible.
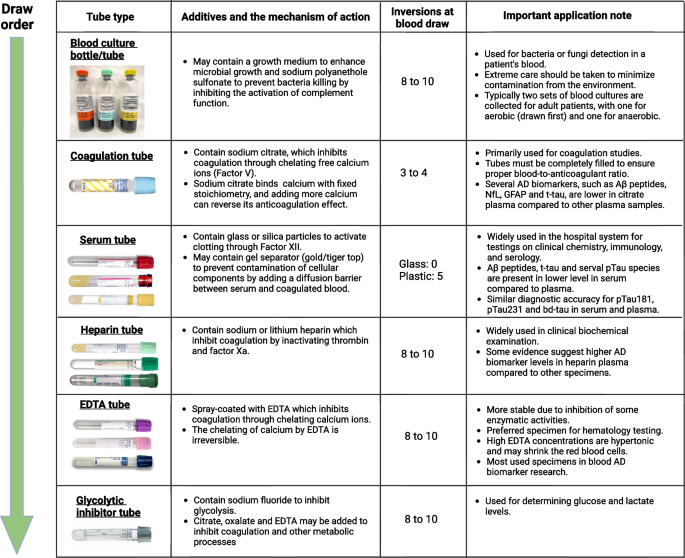
Commonly used human blood collection tubes, their draw order, additives, and important application notes. The numbers of inversions are based on BD’s recommendations for Vacutainer® tubes
Item 4: blood draw order
Different blood collection tubes contain distinctive additives. To minimize the impact of additive cross-contamination when collecting samples from a single blood draw into different types of tubes, the Clinical and Laboratory Standards Institute [ 136 ] CLSI; https://clsi.org/ ) recommended a specific blood draw order (as shown in Fig. 2 ): (1) blood culture tube or bottle; (2) sodium citrate tube; (3) serum tube; (4) heparin tube; (5) EDTA tube; (6) sodium fluoride/potassium oxalate glycolytic inhibitor tube. Following the recommended blood draw order is crucial to avoid carry-over additives that may result in inaccurate results. For example, if an EDTA tube is drawn before a serum tube, some of the EDTA may carry over into the serum tube and interfere with the coagulation.
Item 5: blood collection tube filling height
Some collection tubes, such as those for plasma collection, contain spray-coated or liquid additives. Therefore, different tube filling heights may cause variations in the blood-to-additive ratio, potentially influencing the protein composition. In support of this, Rózga et al. found a lower level of plasma t-tau when K 2 -EDTA tubes were filled only to 50% compared to 100% [ 116 ]. Therefore, to minimize variation, we recommend adhering to manufacturers’ recommendations for blood volume filling to maintain a consistent additive-to-blood ratio among all samples.
Item 6: proper mixing of blood samples
For blood collection tubes containing additives, it is crucial to gently invert the tubes immediately after the blood draw to ensure proper mixing of the additive with the blood. Failure to do so may result in non-homogenous samples and the formation of microclots or residual fibrins that can obstruct the sample probe of analytical instruments. The number of required inversions varies by tube type. We suggest following the manufacturer’s guidelines for the mixing. The recommended number of inversions for BD’s Vacutainer® tubes is listed in Fig. 2 .
Considerations during blood processing
Both serum and plasma are liquid components derived from blood after separating the blood cells, typically through centrifugation. The main difference is that serum is collected from clotted blood, while plasma is collected without clotting through anti-coagulants, thus retaining the clotting factors. Apart from the variations mentioned above for blood collection, several factors during blood processing, including pre-centrifugation delay time, centrifugation conditions, post-centrifugation storage delay, and temperature at various processing steps, may also contribute to the pre-analytical variation of the resulting blood specimens. In the following sections, we summarize current research findings on the impact of these variables and provide general guidelines for blood processing. Additionally, we have included a detailed step-by-step SOP for collecting plasma from EDTA tubes in Additional file 1 .
Item 1: serum clotting time
Harmonizing SOPs for serum collection can be challenging, partly because of the difficulty in setting the optimal clotting time. Insufficient clotting may lead to the formation of residual fibrin, which may clog the biomarker-measuring instruments [ 137 ]. In contrast, prolonged clotting may lead to cell lysis, resulting in serum contamination with cellular components [ 138 ]. The ideal clotting time varies not only by tube type but also by patient characteristics. Plain red top tubes (glass with no additive or plastic coated with silica as clot activator) and serum separator tubes (SST; gold top or tiger top) with clot activators and gel separators are commonly used in the clinics for serum collection. BD Diagnostic recommends a 30-min clotting time for SST and a 60-min clotting time for the red top tubes. Patients with certain diseases, such as liver diseases and multiple myeloma, or those on anticoagulant therapy, may require a longer clotting time. It has therefore been recommended that blood samples should be left to sit upright at room temperature for at least 30 min but no more than 60 min to allow clots to form and minimize the interference of blood cell lysis [ 31 ].
Item 2: pre-centrifugation delay time
Several studies have investigated the impact of pre-centrifugation delay on AD blood biomarker measurements. A long delay has been associated with a more significant decrease in biomarker levels. This impact can be mitigated by storing blood at 4 °C rather than room temperature prior to centrifugation [ 26 , 116 , 120 ]. Among the AD blood biomarkers, Aβ peptides are particularly susceptible to loss from pre-centrifugation delay. Their levels drop in a time-dependent manner when stored at room temperature [ 139 ]. To minimize interference of blood cell lysis and protein degradation, it is recommended completing the whole process within 2 h [ 31 ] and, if not feasible, keeping the blood refrigerated for no more than 24 h [ 26 ]. However, the shorter the pre-centrifugation delay, the better for all the blood biomarkers.
Item 3: centrifugation settings, including speed, time, and temperature
Optimal centrifugation settings are crucial for obtaining high-quality serum/plasma samples. Prolonged or excessive-speed centrifugation may cause blood cell lysis, while centrifugation that is too short and/or at an insufficient speed may result in incomplete separation of serum/plasma from blood cells [ 140 ]. Centrifugation settings may vary by blood collection tube type. For example, coagulated tubes require longer centrifugation than plasma tubes to ensure complete serum separation from the clot (CLSI H21). According to a recent survey [ 26 ] the common practice in the blood AD biomarker field is to centrifuge for 5–15 min at 1500–3000 xg. However, there are still very limited studies evaluating the impact of centrifugation parameters on AD blood biomarker measurements. A preliminary investigation found no significant difference between room temperature and 4 °C centrifugation for most AD biomarkers except t-tau, whose abundance was lower with 4 °C centrifugation [ 26 ].
Item 4: post-centrifugation storage delay
Post-centrifugation delay may also contribute to a decrease in biomarker abundance, although the rate of decline appears slower than during pre-centrifugation delay [ 26 , 120 ]. Similarly, keeping samples on wet ice while waiting for storage has been found to greatly mitigate the impact of storage delay.
Item 5: good laboratory practice (GLP)
Adhering to GLP during blood processing is crucial for ensuring the safety, quality, and integrity of research studies. Below are some key practices that should be followed:
All blood samples and associated collection devices should be considered potentially infectious, and proper personal protective equipment (PPE) should always be used to minimize exposure risk.
To protect the confidentiality of research participants, personal information should not be included on specimen labels. To avoid sample mix-up, all tubes should be clearly labeled, preferably using printed labels or barcodes rather than handwritten ones. This labeling should be done in advance of the participants’ visits for blood collection.
Good pipetting skills are essential for ensuring high sample quality. When pipetting plasma/serum from the blood collection tubes, gently draw the liquid from the top and gradually move the pipette down with the liquid. It is important to avoid disturbing the buffy coat and the cell layers in the plasma tubes and clots in the serum tubes. If allowed, leave the bottom ~ 10% of plasma/serum behind to prevent cross-layer contamination.
If plasma/serum samples are to be aliquoted into more than one tube, it is important to transfer them from the blood collection tubes to a second, intermediary tube (such as low protein binding conical tubes) after centrifugation. Before aliquoting, the samples should be mixed by inverting the conical intermediary tube or pipetting up and down multiple times to ensure homogeneity. Direct aliquoting from the blood collection tubes right after centrifugation may lead to heterogeneity among aliquots due to the impact of centrifugation forces.
Hemolysis significantly deteriorates sample quality and is the primary cause of unusable specimens for clinical assays [ 140 ]. Therefore, samples should be inspected for signs of hemolysis which may impact the assay results. We recommend using a quick reference chart (such as the CDC Hemolysis Reference Palette) to record the hemolysis scale during specimen collection and checking the influence of hemolysis during data analysis.
Item 6: general procedures for serum collection
Figure 3 A illustrates the general procedures for collecting serum. We recommend collecting at least 5 ml of blood, yielding approximately 2.5 ml of serum to ensure sufficient specimens for multianalyte measurement. To minimize the impact of freeze/thaw, samples should be aliquoted. Both plain red-top tubes and serum separator tubes are commonly used for serum collection. Other than glass plain red top tubes, all other tube types require five inversions immediately after the blood draw to mix the blood with clot activators. Below are the general procedures for serum collection.
CRITICAL: If not using the glass red top tubes, phlebotomists should gently invert the blood tubes 5 times immediately after blood draw.
CRITICAL: Place the filled blood collection tubes upright at room temperature for 30 to 60 min to allow the clot to form.
CRITICAL: If the blood is not centrifuged immediately after the clotting time (30 to 60 min at room temperature), the tubes should be refrigerated (4 °C) for no longer than 2 h.
Centrifuge clotted tubes balanced by weight for 10 min at 1500 to 2000 × g at 4 °C.
Use the disposable transfer pipette to transfer the serum (top layer) to a 15 mL conical tube (or 50 mL conical tube if collecting 30 to 100 mL of blood). Be careful not to disturb the clot containing red blood cells, white blood cells, platelets, etc.
If more than one tube is collected, combine the serum samples from all tubes into the same conical tube.
Gently invert the conical tube 8–10 times to mix. Aliquot 250 μl to 1 ml into labeled microtubes or cryovials with O-ring-sealed screw leads. Residual aliquots can be saved and pooled as QC samples for repeated analysis.
Store all aliquots upright in a specimen box in an -80 °C or colder freezer.
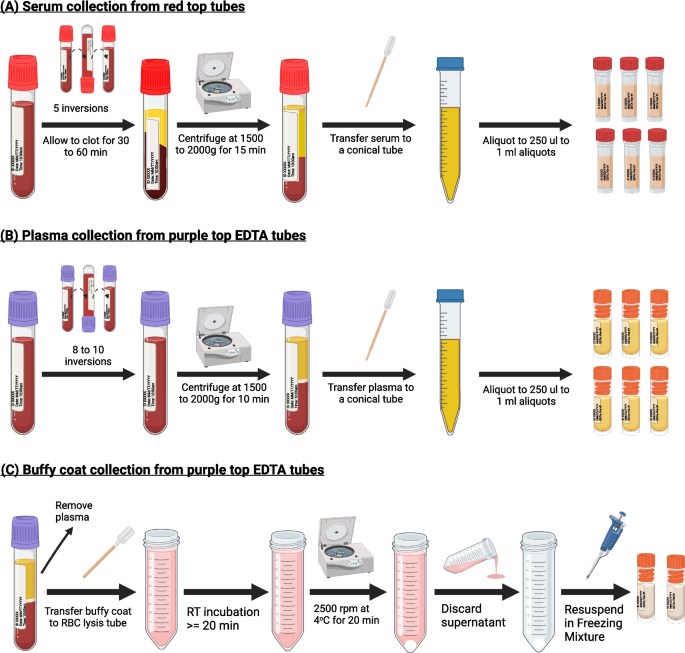
General procedures for serum ( A ), plasma ( B ), and buffy coat ( C ) collection from whole blood
Item 7: general procedures for plasma collection
Figure 3 B depicts the general procedures for collecting plasma. We recommend using EDTA or heparin tubes for blood collection, filling the tubes until the vacuum is exhausted, and following the procedures below.
CRITICAL: Immediately after blood collection, gently invert/mix (180-degree turns) the EDTA tubes 8–10 times. Place the tubes upright on a rack until centrifugation.
CRITICAL: It is advisable to store collected blood at 4 °C instead of at room temperature before centrifugation. Blood samples should be centrifuged within 2 h of blood collection to minimize degradation of AD biomarkers.
CRITICAL: In case of unavoidable prolonged centrifugation delay, place blood samples in the refrigerator for no more than 24 h. Avoid direct contact of blood tubes with ice to minimize cell lysis.
Centrifuge balanced blood collection tubes for 10 min at 1500 to 2000 × g at 4 °C.
Use the disposable transfer pipette to transfer the plasma (top layer) to a 15 mL conical tube (or 50 mL conical tube if collecting 30 to 100 mL of blood). Be careful not to disturb the buffy coat layer (the whitish layer in the middle) and the red blood cell layer (the red layer at the bottom).
If more than one tube is collected, combine the plasma samples from all blood collection tubes into the same conical tube.
Item 8: general procedures for buffy coat collection
Buffy coat contains most of the white blood cells and platelets of the anti-coagulated blood and is useful for a variety of clinical applications including genomic/genetic analysis. The remaining blood fractions after plasma collection can be used to collect buffy coat. To ensure high quality DNA, we recommend further enriching the buffy coats using a hypotonic solution to lyse the residual red blood cells. Figure 3 C illustrates the general procedures for buffy coat collection.
Note: Prepare the following reagents ahead of time and store them at 4 °C.
Ammonium chloride solution: 7.72 g/L
Ammonium bicarbonate solution: 0.79 g/L
Freezing mixture: TriPotassium Citrate: 17.8 g, Sodium Phosphate, monobasic: 2.4 g, Sodium Phosphate, dibasic: 2.8 g, Glycerin (Glycerol): 400 ml; bring volume to 1 L with distilled water.
Freshly prepare RBC lysis buffer by combining 45 ml ammonium chloride solution and 5 ml ammonium bicarbonate solution.
After removing the plasma (top layer) from the EDTA or heparin tubes, use another transfer pipette to draw the buffy coat (the whitish layer on top of the RBC layer) and place into the RBC lysis buffer tube (50 ml).
Mix by pipetting up and down to separate any leftover cells from within transfer pipette.
Cap the 50 ml tubes with lysis buffer + buffy coat and gently invert several times to mix.
Incubate at room temp for at least 20 min.
Add 10% bleach or Cavicide to the used blood tubes (lower layer with RBC) with leftover blood in them; discard in an appropriate biohazard bag.
After 20 min incubation, centrifuge 50 ml tubes at 4 °C for 20 min at 2500 rpm
After centrifuging, a white pellet will be visible at the bottom of the tube.
If no pellet is visible, centrifuge for an additional 20 min.
If pellet is visible, pour the red supernatant into a beaker filled with 10% bleach or Cavicide.
Let pellet dry (approximately 10–20 min).
Add 1 ml of freezing mixture to pellet. [ Freezing Mixture: TriPotassium Citrate: 17.8 g, Sodium Phosphate, monobasic: 2.4 g, Sodium Phosphate, dibasic: 2.8 g, Glycerin (Glycerol): 400 ml; bring volume to 1 L with distilled water].
Gently mix to break the pellet into single cell suspension.
Transfer whole (cells + freezing mix) into cryotubes.
Store at -80 °C for subsequent DNA isolation for genetic studies.
Item 9: detailed step-by-step SOP for blood collection and processing
We have combined the points discussed above and our own experiences to develop an SOP for the handling of blood for biomarker measurements (see Additional file 1 ). This SOP, which was primarily developed to streamline handling procedures for neurodegenerative disease cohort studies at the University of Pittsburgh Alzheimer’s Disease Research Center and ancillary centers and studies, can also be used by other investigators including those who are new to blood biomarker studies. Importantly, the SOP is adapted to allow for the concurrent processing of whole blood into plasma/serum for protein biomarker evaluation, and buffy coat for genetic/genomic studies. Moreover, the design of the SOP enables multiple tube aliquots to be collected and stored, and residual volumes pooled for quality control (QC) purposes.
Considerations for biobanking
Item 1: storage tubes and temperature.
To minimize analyte loss caused by adherence to the tube surfaces, it is recommended to use low protein binding microcentrifuge tubes or cryovials to store serum or plasma samples. For long-term storage, tubes with O-ring-sealed screws should be used to prevent evaporation. To prevent degradation, samples should be stored at ultra-low temperature freezers (-80 °C) or liquid N 2 tanks instead of -20 °C freezers. If feasible, aliquots of the same sample should be stored in separate freezers to prevent complete loss in the case of a freezer failure.
Item 2: freeze/thaw cycles
Several studies have investigated the impact of freeze/thaw cycles on blood biomarkers [ 107 , 116 , 141 , 142 , 143 ]. The overall results suggest that plasma AD biomarkers are stable for at least three freeze/thaw cycles. However, serum AD biomarkers may be more sensitive to freeze/thaw cycles. For example, serum Aβ40 level significantly decreased after any freeze/thaw cycle, while serum Aβ42 level showed a significant decrease at the third freeze-thaw cycle [ 107 ]. Therefore, to minimize the impact of freeze/thaw cycles, we recommend aliquoting samples in adequate volume, limiting the total number of freeze/thaw cycles to as few as possible, but never more than three.
Item 3: transportation
To transport samples to different facilities, it is recommended to use abundant dry ice sufficient to last at least 24 h post the expected delivery time to avoid sample thawing due to delivery delays. If possible, use a courier that replenishes dry ice mid-way, and avoid weekend delivery. It is worth noting that dry ice inside storage tubes may change the pH of the specimen during thawing and potentially influence the assays [ 144 ]. Therefore, samples may be stored free of dry ice in -80 °C freezers for at least 24 h before thawing for measurement. To provide guidance to readers, we have compiled a list of recommended practices during blood transportation, created based on issues regularly encountered at a major biomarker laboratory.
Include an accurate sample list (including accurate volumes). Ideally, provide a Microsoft Excel sheet (or similar) with columns for sample IDs, their corresponding volumes, and their location in the box. Adding a pictorial illustration of sample arrangements in the boxes is also helpful.
Inform the receiving laboratory ahead of time so that they will keep a lookout and be able to receive and store them in good time. The receiving lab may need to find freezer space before your samples arrive. Therefore, it is important that they are informed ahead of time.
Ensure that the package contains an adequate amount of dry ice. For long-distance transportation that spans multiple days, choose a reliable company that can refill the dry ice midway.
Print labels using a computer, rather than handwriting them, to ensure better legibility.
Use tubes with caps that do not become loose accidentally.
Sort the samples in the order you want them analyzed. If you are unsure of the order, there are two main rules:
If your samples are in groups: randomize them, so that all groups are represented in all analytical runs.
If you have longitudinal samples, keep all samples from the same participant together and in the order in which they were collected. Ensure your sample coding reflects this ordering.
Do the final sorting of the samples BEFORE you send them. It might take longer and be too time-consuming for the receiving lab to do it.
Considerations during biomarker measurements
The quality of laboratory and clinical assays has greatly improved due to advances in instrument technology, particularly the use of automated equipment, which has led to higher reproducibility through standardized procedures. However, analytical errors/variations from various sources may still occur and render results unusable or confound study findings. Common sources of analytical errors/variations include instrument malfunction, operators’ failure to follow procedures, inherent batch-to-batch variation of the assay, and matrix interference. To minimize errors/variations, it is important to use good process controls and follow good laboratory practices. Below, we outline several considerations that are critical for ensuring high-quality results and monitoring/maintaining the long-term stability of the AD blood biomarker measurements.
Item 1: preparation of samples for measurement
All blood specimens should be considered potential biohazards and handled with appropriate PPE. To ensure accurate measurement, it is crucial to homogenize samples before measurement. Samples should be completely thawed and mixed thoroughly. To minimize the impact of particulates that could clot cartridges or sample probes in the instrumentation, samples should be centrifuged before dispensing into a measurement container. It is also important to minimize the bubbles during pipetting. Any remaining samples should be promptly returned to the -80 °C freezer to minimize protein degradation. Place a dot on the tube lid with a permanent marker after each freeze-thaw cycle and keep track of the total number of cycles in updated inventory of samples. It is also recommended to keep samples with the same number of freeze-thaw cycles together and separate from the original, un-thawed samples.
Item 2: inclusion of calibrators
Despite significant technological advances, batch-to-batch variation remains an inherent issue in clinical chemistry. Contributing factors may include variations in instrument performance, changes in reagents and consumables, operator variability, etc. To address this issue, calibrators should be included in every batch to help correct for batch effects. Some platforms, such as Lumipulse, have been built to circumvent this issue such that there is an internal, manufacturer-provided calibration curve against which all sample results are plotted. However, this approach only works if the manufacturer can adjust all reagent batches to perform equivalently to the initial batch used to generate the built-in curve. The acceptance criteria for the calibration curve must be predefined to ensure accuracy and consistency. Although these criteria may vary depending on the platform used, certain parameters such as the regression coefficient, the variance between predicted and actual concentrations, and the repeatability for each calibrator should be considered.
Item 3: inclusion of QC samples
QC samples play a critical role in process control and should be included in every analytical run to evaluate assay performance and address potential errors. At a minimum, QC samples should be run in duplicates at the beginning and end of each analytical run. These samples should be selected in a way that they cover a range of concentrations across the standard curve or the typical range of concentrations for the measured sample values. It is typically advisable to use use three QC samples – low, average, and high concentrations relative to the assay standard curve. For optimal results, the same lot of QC samples should be used over time to help detect system or operator performance changes. Whenever possible, QC samples should be the same specimen types as the test samples. When switching between batches of QC samples, bridging is recommended – the relative comparison of QC samples to adjust the biomarker distribution on one plate to the other. These factors can then be applied to all samples measured on each plate/run to normalize the entire dataset. In Fig. 4 , we present a hypothetical example of a cohort consisting of 280 samples that were analyzed across four plates for Biomarker X. As shown in Fig. 4 A, the mean and overall data distribution of the uncorrected results from plates 1 and 2 appear similar, while plates 3 and 4 show a noticeable shift (simulation datasets in Additional file 2 ). However, after normalization using the plate-specific scaling factors based on QC readings, as shown in Fig. 4 B, the results from all four plates become more comparable.
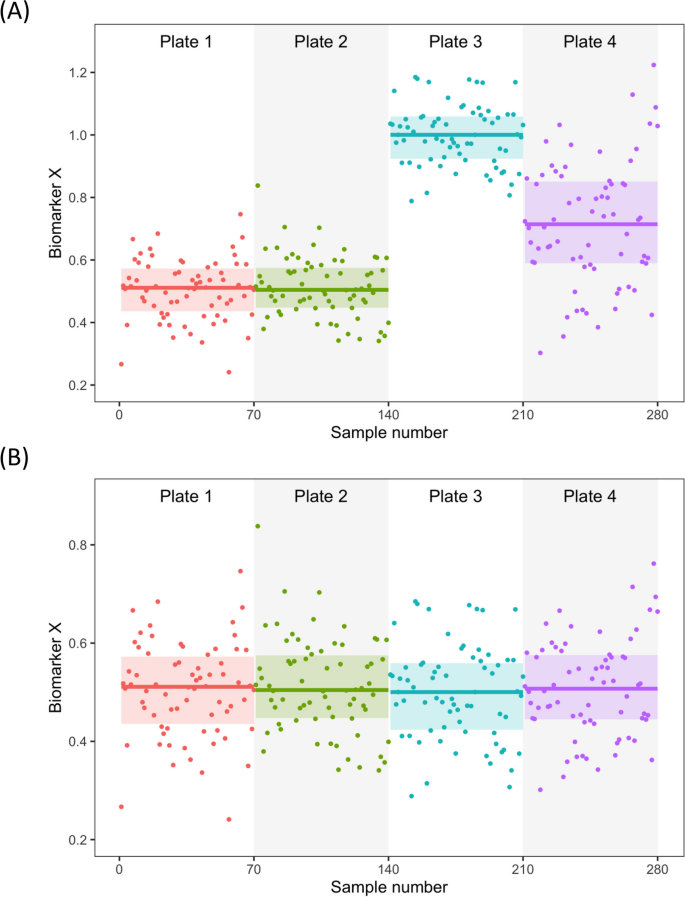
Visualization of data generated from measuring a hypothetical biomarker on 280 samples spread across four plates. The figure shows the results before ( A ) and after ( B ) adjustment based on the signals generated for identical aliquots of the same quality control samples measured on each plate, assuming all other key variables remain the same
Similar to calibrators, a predefined set of criteria should be applied to determine whether a run passes or fails. Key parameters to consider include the deviation from target values established through prior repeated analyses and the reproducibility of the QC measurements. An article by Schindler et al. [ 145 ] which tracked CSF assay performances over approximately a decade sheds light on this issue and potential corrective factors.
Item 4: sample measurement order
Batch-to-batch variation and within-batch run order effects can bias results, leading to erroneous conclusions. One way to address these issues is to randomize the order of sample analysis. There are various methods of randomization, including simple randomization, block randomization, stratified randomization, and covariate adaptive randomization [ 146 ]. Simple randomization involves using a single random sequence and is easy to implement, for example, by using the random number generator in Excel. However, simple randomization may result in an unequal distribution of groups and may not be suitable for studies with a small sample size ( n < 200). Block randomization addresses this issue by randomly assigning an equal number of samples from each group to each block and is more appropriate for small-size studies. For studies with multiple covariates, stratified randomization or covariate adaptive randomization may be necessary to control the potential uneven distribution of covariates. Additionally, for longitudinal measures, we recommend measuring all time points for the same participant simultaneously to minimize the impact of batch variation.
Item 5: sample blinding
Knowledge of grouping information may bias the analysis results and taint the data. Whenever possible, scientists performing the measurements and the analysts/statisticians who work with the ensuing data should be blinded from grouping allocation until measurement results have been finalized. A third party should perform the randomization to generate sample analysis orders.
Item 6: assay order
If multiple biomarkers are to be measured from the same aliquots, it is best practice to start with the biomarker most sensitive to degradation/denaturation from repeated freeze/thaw cycles. For example, among the core AD blood biomarkers, Aβ40 and Aβ42 tend to be most prone to degradation and should therefore be measured first, if possible, followed by t-tau, p-tau, BD-tau and finally GFAP and NfL. In addition, using multiplexed assays, if available, can help minimize the number of freeze/thaw cycles required.
Item 7: the use of bridging samples
For discovery research, it is recommended to process all samples at the same time with the same batch of reagents. However, in cases where it is unavoidable to change the reagent lots or run the analysis at different times or in various laboratories, harmonization can be achieved by repeating the measurement for bridging samples. Ideally, bridging samples should be from the same sample cohort and cover the full range of values of the study samples. The bridging samples are analyzed using the same reagents and consumables for each batch, allowing the determination of batch-specific normalization factors. The same approach described in Fig. 4 for normalizing run data from different plates is applicable here to between-batch normalization.
Item 8: longitudinal samples
Longitudinal studies, which involve continuous tracking of the same individuals over time, are commonly employed to assess the influence of various interventions or risk factors on disease outcomes. In contrast to cross-sectional studies, which capture snapshots of participants at a single point in time, longitudinal studies typically require an extended duration to collect specimens for the entire study. In some cases, researchers may be able to wait until all samples are collected to run them simultaneously. However, there are instances where running batches of samples along the way becomes necessary to gain preliminary insights into the data. To minimize the impact of temporal variation, proper control measures must be implemented to monitor and maintain the long-term stability of measurements. Several practical guidelines, proposed by Palmqvist et al. for AD CSF biomarkers, can be adopted for blood AD biomarkers [ 78 ]. These include controlling preanalytical variables, following good laboratory practices, utilizing calibrators and QC samples, and assessing the batch effect through batch-bridging. Ideally, one would like to use the same QCs throughout a longitudinal study. However, if this is not possible, it is important to perform batch-bridging and re-run samples from the previous analysis round to normalize runs. Therefore, it is crucial to properly plan for the longitudinal studies, including stocking up reagents (including QC and bridging samples) and consumables for the entire study if shelf life is allowed. While most blood AD biomarker research has been conducted in research settings, there is an increasing number of studies being initiated to evaluate the use of these biomarkers in real clinical practice, where specimens are continuously analyzed. Maintaining measurement stability becomes even more important in these real-world settings.
Considerations for result reporting
Item 1: demographic and clinical information.
To minimize potential bias caused by demographic and clinical factors, it is important to include the distribution of available demographic and clinical characteristics among groups in the report, along with their associated statistical findings. These information help identify potential moderating variables and adjust for their effect in the analysis. Additionally, including this information in the report allows better comparison with results from different studies.
Item 2: full description of methods
To facilitate the coherent pooling of data in future meta-analyses, it is important to include detailed descriptions of blood collection/handling protocols and laboratory assay protocols in the Method section of peer-reviewed publications. In addition, as preanalytical variables, such as blood draw devices and parameters during blood processing, may interfere with AD biomarker measurements, it is important to describe these variables and to indicated if they were changed during the study.
Item 3: disclosure of assay performance
It is important to include assay performance in the publication to enable other researchers to evaluate the quality of the reported results. Multiple attributes can be useful for assessing assay performance, such as calibration curves’ R -value, linearity range, the limit of detection, and limit of quantification, as well as QC performance measures such as repeatability and intermediate precision. Moreover, the reports should also disclose if plate adjustments, such as with the use of bridging samples or QC samples, have been performed to account for batch variation.
Unresolved issues
Certified reference materials.
A major source of confusion in the field is that blood biomarker assays for the same analyte (e.g., plasma p-tau181 or p-tau217) from various sources return very different numerical values in terms of concentrations. We have sought to explain the analytical reasons behind this phenomenon – that it is often due to the use of different calibrators as well as variability of signals from the technical platforms [ 13 ]. However, the issue limits the comparability of assay results across platforms, which might become even more acute once cutpoints are generated. The use of certified reference materials could provide a solution, by providing a focal point for comparing and calibrating the standards used in the different assays. An example of the use of certified reference materials in mitigating between-assay bias was demonstrated by the application by Boulo S et al. in CSF samples [ 147 ].
Real world applications
Despite excellent performances of blood biomarkers demonstrated in many publications, we should be mindful that the results have mostly come from highly selected research cohorts that additionally have prior confirmation of pathology using CSF or neuroimaging biomarkers or even sometimes both. As we venture into applying blood biomarkers as potential first line screeners in clinical practice and therapeutic trials, it is important to note that this might be the real “test drive” for the biomarkers. As such, their performances may not be as stellar as observed for multiple reasons. This includes the much lower prevalence of AD pathophysiology in the community compared with the research cohorts, and the high heterogeneity of diseases presented in the clinics contrary to those included in defined cohorts. Moreover, such heterogenous cases would not benefit from prior categorization using CSF and neuroimaging biomarkers as often done for the existing cohorts that have recorded high accuracies.
Clinical use of blood biomarkers is much anticipated. However, it is often forgotten that one of the fundamental factors that need addressing prior to this happening is the generation and validation of assay thresholds. Such threshold points would not only be based on the detection of amyloid and/or tau pathology but would additionally investigate potential clinical scenarios such as if and how the set cutpoints respond to given demographic and clinical variables.
The blood AD biomarker research field has experienced rapid growth in recent years, with numerous studies highlighting the potential of blood-based AD biomarkers in supporting clinical decision-making and accelerating therapeutic development. We have outlined in this review general guidelines for the various steps involved in blood AD biomarker measurement, to promote good laboratory practices to minimize analytical errors and facilitate the development of standardized protocols that can improve reproducibility and enable cross-validation across different research centers. Table 2 provides a summary of the important preanalytical, analytical and post-analytical factors to consider in AD blood biomarker research. However, further research is needed to fully understand the impact of preanalytical and analytical variables, and these guidelines should be updated as new research findings become available. Finally, we provide an adaptable SOP that can be applied to blood collection, processing, and downstream handling in biomarker evaluations.
Availability of data and materials
All the data are included in the manuscript and the supporting information.
Abbreviations
Amyloid-beta
- Alzheimer’s disease
Brain-derived tau
Cerebrospinal fluid
Dried blood spot
Dried plasma spot
Electrochemiluminescence
Ethylenediaminetetraacetic acid
Enzyme-linked immunosorbent assay
General Data Protection Regulation
Glial fibrillary acidic protein
Good laboratory practice
Health Insurance Portability and Accountability Act of 1996
Interdigitated microelectrode sensor
Immunomagnetic reduction
Immunoprecipitation coupled with mass spectrometry
Intravenous
Magnetic resonance imaging
Mass spectrometry
Meso Scale Discovery
Neurofilament light-chain
Phosphorylated tau
Positron emission tomography
Personal protective equipment
Quality control
Single-molecule array
Standardized operating procedure
Serum separator tubes
Alzheimer’s Association. 2023. Alzheimer’s disease facts and figures. Alzheimers Dement. 2023;19:1598–695.
Hebert LE, Weuve J, Scherr PA, Evans DA. Alzheimer disease in the United States (2010–2050) estimated using the 2010 census. Neurology. 2013;80:1778–83.
Article PubMed PubMed Central Google Scholar
Patterson C. World Alzheimer Report 2018: The state of the art of dementia research; New frontiers. London: Alzheimer’s Disease International; 2018.
Alzheimer A, Stelzmann RA, Schnitzlein HN, Murtagh FR. An English translation of Alzheimer’s 1907 paper, “Uber eine eigenartige Erkankung der Hirnrinde.” Clin Anat. 1995;8:429–31.
Article CAS PubMed Google Scholar
DeTure MA, Dickson DW. The neuropathological diagnosis of Alzheimer’s disease. Mol Neurodegener. 2019;14:32.
Tarawneh R, Holtzman DM. The clinical problem of symptomatic Alzheimer disease and mild cognitive impairment. Cold Spring Harb Perspect Med. 2012;2:a006148.
van Dyck CH, Swanson CJ, Aisen P, Bateman RJ, Chen C, Gee M, Kanekiyo M, Li D, Reyderman L, Cohen S, et al. Lecanemab in early Alzheimer’s disease. N Engl J Med. 2022;388:9–21.
Article PubMed Google Scholar
Budd Haeberlein S, Aisen PS, Barkhof F, Chalkias S, Chen T, Cohen S, Dent G, Hansson O, Harrison K, von Hehn C, et al. Two randomized phase 3 studies of aducanumab in early Alzheimer’s disease. J Prev Alzheimers Dis. 2022;9:197–210.
CAS PubMed Google Scholar
Reardon S. FDA approves Alzheimer’s drug lecanemab amid safety concerns. Nature. 2023;613:227–8.
Jack CR Jr, Bennett DA, Blennow K, Carrillo MC, Dunn B, Haeberlein SB, Holtzman DM, Jagust W, Jessen F, Karlawish J, et al. NIA-AA research framework: toward a biological definition of Alzheimer’s disease. Alzheimers Dement. 2018;14:535–62.
Alawode DOT, Heslegrave AJ, Ashton NJ, Karikari TK, Simrén J, Montoliu-Gaya L, Pannee J, O’Connor A, Weston PSJ, Lantero-Rodriguez J, et al. Transitioning from cerebrospinal fluid to blood tests to facilitate diagnosis and disease monitoring in Alzheimer’s disease. J Intern Med. 2021;290:583–601.
Article CAS PubMed PubMed Central Google Scholar
Balogun WG, Zetterberg H, Blennow K, Karikari TK. Plasma biomarkers for neurodegenerative disorders: ready for prime time? Curr Opin Psychiatry. 2023;36(2):112.
Karikari TK, Ashton NJ, Brinkmalm G, Brum WS, Benedet AL, Montoliu-Gaya L, Lantero-Rodriguez J, Pascoal TA, Suárez-Calvet M, Rosa-Neto P, et al. Blood phospho-tau in Alzheimer disease: analysis, interpretation, and clinical utility. Nat Rev Neurol. 2022;18:400–18.
Malzbender K, Lavin-Mena L, Hughes L, Bose N, Goldman D, Patel D. Key Barriers to Clinical Trials for Alzheimer’s Disease. Leonard D., editor. Los Angeles, CA, USA: Schaeffer Center for Health Policy and Economics; 2020.
Moscoso A, Karikari TK, Grothe MJ, Ashton NJ, Lantero-Rodriguez J, Snellman A, Zetterberg H, Blennow K, Schöll M. CSF biomarkers and plasma p-tau181 as predictors of longitudinal tau accumulation: Implications for clinical trial design. Alzheimers Dement. 2022;18:2614–26.
Cullen NC, Leuzy A, Janelidze S, Palmqvist S, Svenningsson AL, Stomrud E, Dage JL, Mattsson-Carlgren N, Hansson O. Plasma biomarkers of Alzheimer’s disease improve prediction of cognitive decline in cognitively unimpaired elderly populations. Nat Commun. 2021;12:3555.
Rissin DM, Kan CW, Campbell TG, Howes SC, Fournier DR, Song L, Piech T, Patel PP, Chang L, Rivnak AJ, et al. Single-molecule enzyme-linked immunosorbent assay detects serum proteins at subfemtomolar concentrations. Nat Biotechnol. 2010;28:595–9.
Nakamura A, Kaneko N, Villemagne VL, Kato T, Doecke J, Doré V, Fowler C, Li Q-X, Martins R, Rowe C, et al. High performance plasma amyloid-β biomarkers for Alzheimer’s disease. Nature. 2018;554:249–54.
Ovod V, Ramsey KN, Mawuenyega KG, Bollinger JG, Hicks T, Schneider T, Sullivan M, Paumier K, Holtzman DM, Morris JC, et al. Amyloid β concentrations and stable isotope labeling kinetics of human plasma specific to central nervous system amyloidosis. Alzheimers Dement. 2017;13:841–9.
Chong JR, Ashton NJ, Karikari TK, Tanaka T, Schöll M, Zetterberg H, Blennow K, Chen CP, Lai MKP. Blood-based high sensitivity measurements of beta-amyloid and phosphorylated tau as biomarkers of Alzheimer’s disease: a focused review on recent advances. J Neurol Neurosurg Psychiatry. 2021;92:1231–41.
Gonzalez-Ortiz F, Turton M, Kac PR, Smirnov D, Premi E, Ghidoni R, Benussi L, Cantoni V, Saraceno C, Rivolta J, et al. Brain-derived tau: a novel blood-based biomarker for Alzheimer’s disease-type neurodegeneration. Brain. 2023;146:1152–65.
Pereira JB, Janelidze S, Smith R, Mattsson-Carlgren N, Palmqvist S, Zetterberg H, Stomrud E, Ashton NJ, Blennow K, Hansson O. Plasma glial fibrillary acidic protein is an early marker of Aβ pathology in Alzheimer’s disease. medRxiv. 2021:2021.2004.2011.21255152.
Osborn LM, Kamphuis W, Wadman WJ, Hol EM. Astrogliosis: an integral player in the pathogenesis of Alzheimer’s disease. Prog Neurobiol. 2016;144:121–41.
Bellaver B, Povala G, Ferreira PCL, Ferrari-Souza JP, Leffa DT, Lussier FZ, Benedet AL, Ashton NJ, Triana-Baltzer G, Kolb HC, et al. Astrocyte reactivity influences amyloid-β effects on tau pathology in preclinical Alzheimer’s disease. Nat Med. 2023;29(7):1775–81.
Benedet AL, Brum WS, Hansson O, Karikari TK, Zimmer ER, Zetterberg H, Blennow K, Ashton NJ. The accuracy and robustness of plasma biomarker models for amyloid PET positivity. Alzheimers Res Ther. 2022;14:26.
Verberk IMW, Misdorp EO, Koelewijn J, Ball AJ, Blennow K, Dage JL, Fandos N, Hansson O, Hirtz C, Janelidze S, et al. Characterization of pre-analytical sample handling effects on a panel of Alzheimer’s disease–related blood-based biomarkers: Results from the Standardization of Alzheimer’s Blood Biomarkers (SABB) working group. Alzheimers Dement. 2022;18:1484–97.
Plebani M. Exploring the iceberg of errors in laboratory medicine. Clin Chim Acta. 2009;404:16–23.
Teunissen CE, Petzold A, Bennett JL, Berven FS, Brundin L, Comabella M, Franciotta D, Frederiksen JL, Fleming JO, Furlan R, et al. A consensus protocol for the standardization of cerebrospinal fluid collection and biobanking. Neurology. 2009;73:1914–22.
Gobom J, Parnetti L, Rosa-Neto P, Vyhnalek M, Gauthier S, Cataldi S, Lerch O, Laczo J, Cechova K, Clarin M, et al. Validation of the LUMIPULSE automated immunoassay for the measurement of core AD biomarkers in cerebrospinal fluid. Clin Chem Lab Med. 2022;60:207–19.
Schindler SE, Bollinger JG, Ovod V, Mawuenyega KG, Li Y, Gordon BA, Holtzman DM, Morris JC, Benzinger TLS, Xiong C, et al. High-precision plasma β-amyloid 42/40 predicts current and future brain amyloidosis. Neurology. 2019;93:e1647–59.
O’Bryant SE, Gupta V, Henriksen K, Edwards M, Jeromin A, Lista S, Bazenet C, Soares H, Lovestone S, Hampel H, et al. Guidelines for the standardization of preanalytic variables for blood-based biomarker studies in Alzheimer’s disease research. Alzheimers Dement. 2015;11:549–60.
Armstrong RA. Risk factors for Alzheimer’s disease. Folia Neuropathol. 2019;57:87–105.
Article Google Scholar
Henderson AS. The risk factors for Alzheimer’s disease: a review and a hypothesis. Acta Psychiatr Scand. 1988;78:257–75.
Killin LOJ, Starr JM, Shiue IJ, Russ TC. Environmental risk factors for dementia: a systematic review. BMC Geriatr. 2016;16:175.
Mielke MM, Dage JL, Frank RD, Algeciras-Schimnich A, Knopman DS, Lowe VJ, Bu G, Vemuri P, Graff-Radford J, Jack CR Jr, Petersen RC. Performance of plasma phosphorylated tau 181 and 217 in the community. Nat Med. 2022;28:1398–405.
Toledo JB, Vanderstichele H, Figurski M, Aisen PS, Petersen RC, Weiner MW, Jack CR, Jagust W, Decarli C, Toga AW, et al. Factors affecting Aβ plasma levels and their utility as biomarkers in ADNI. Acta Neuropathol. 2011;122:401.
Schindler SE, Karikari TK, Ashton NJ, Henson RL, Yarasheski KE, West T, Meyer MR, Kirmess KM, Li Y, Saef B, et al. Effect of race on prediction of brain amyloidosis by plasma Aβ42/Aβ40, phosphorylated tau, and neurofilament light. Neurology. 2022;99:e245–57.
Syrjanen JA, Campbell MR, Algeciras-Schimnich A, Vemuri P, Graff-Radford J, Machulda MM, Bu G, Knopman DS, Jack CR Jr, Petersen RC, Mielke MM. Associations of amyloid and neurodegeneration plasma biomarkers with comorbidities. Alzheimers Dement. 2022;18:1128–40.
Arvanitakis Z, Lucas JA, Younkin LH, Younkin SG, Graff-Radford NR. Serum creatinine levels correlate with plasma amyloid β protein. Alzheimer Dis Assoc Disord. 2002;16:187–90.
Blasko I, Kemmler G, Krampla W, Jungwirth S, Wichart I, Jellinger K, Tragl KH, Fischer P. Plasma amyloid beta protein 42 in non-demented persons aged 75 years: effects of concomitant medication and medial temporal lobe atrophy. Neurobiol Aging. 2005;26:1135–43.
Chatterjee P, Pedrini S, Ashton NJ, Tegg M, Goozee K, Singh AK, Karikari TK, Simrén J, Vanmechelen E, Armstrong NJ, et al. Diagnostic and prognostic plasma biomarkers for preclinical Alzheimer’s disease. Alzheimers Dement. 2022;18:1141–54.
Lopez OL, Chang Y, Ives DG, Snitz BE, Fitzpatrick AL, Carlson MC, Rapp SR, Williamson JD, Tracy RP, DeKosky ST, Kuller LH. Blood amyloid levels and risk of dementia in the Ginkgo Evaluation of Memory Study (GEMS): a longitudinal analysis. Alzheimers Dement. 2019;15:1029–38.
Pontecorvo MJ, Lu M, Burnham SC, Schade AE, Dage JL, Shcherbinin S, Collins EC, Sims JR, Mintun MA. Association of donanemab treatment with exploratory plasma biomarkers in early symptomatic Alzheimer disease: a secondary analysis of the TRAILBLAZER-ALZ randomized clinical trial. JAMA Neurol. 2022;79:1250–9.
Depypere H, Vergallo A, Lemercier P, Lista S, Benedet A, Ashton N, Cavedo E, Zetterberg H, Blennow K, Vanmechelen E, et al. Menopause hormone therapy significantly alters pathophysiological biomarkers of Alzheimer’s disease. Alzheimers Dement. 2023;19:1320–30.
Thurston RC, Maki P, Chang Y, Wu M, Aizenstein HJ, Derby CA, Karikari TK. Menopausal vasomotor symptoms and plasma Alzheimer disease biomarkers. Am J Obstet Gynecol. 2023. https://doi.org/10.1016/j.ajog.2023.11.002 .
Sakpal TV. Sample size estimation in clinical trial. Perspect Clin Res. 2010;1:67–9.
PubMed PubMed Central Google Scholar
Milà-Alomà M, Ashton NJ, Shekari M, Salvadó G, Ortiz-Romero P, Montoliu-Gaya L, Benedet AL, Karikari TK, Lantero-Rodriguez J, Vanmechelen E, et al. Plasma p-tau231 and p-tau217 as state markers of amyloid-β pathology in preclinical Alzheimer’s disease. Nat Med. 2022;28:1797–801.
Fandos N, Pérez-Grijalba V, Pesini P, Olmos S, Bossa M, Villemagne VL, Doecke J, Fowler C, Masters CL, Sarasa M. Plasma amyloid β 42/40 ratios as biomarkers for amyloid β cerebral deposition in cognitively normal individuals. Alzheimers Dement (Amst). 2017;8:179–87.
De Meyer S, Schaeverbeke JM, Verberk IMW, Gille B, De Schaepdryver M, Luckett ES, Gabel S, Bruffaerts R, Mauroo K, Thijssen EH, et al. Comparison of ELISA- and SIMOA-based quantification of plasma Aβ ratios for early detection of cerebral amyloidosis. Alzheimers Res Ther. 2020;12:162.
Doecke JD, Pérez-Grijalba V, Fandos N, Fowler C, Villemagne VL, Masters CL, Pesini P, Sarasa M. Total Aβ(42)/Aβ(40) ratio in plasma predicts amyloid-PET status, independent of clinical AD diagnosis. Neurology. 2020;94:e1580–91.
Vogelgsang J, Shahpasand-Kroner H, Vogelgsang R, Streit F, Vukovich R, Wiltfang J. Multiplex immunoassay measurement of amyloid-β42 to amyloid-β40 ratio in plasma discriminates between dementia due to Alzheimer’s disease and dementia not due to Alzheimer’s disease. Exp Brain Res. 2018;236:1241–50.
Palmqvist S, Janelidze S, Stomrud E, Zetterberg H, Karl J, Zink K, Bittner T, Mattsson N, Eichenlaub U, Blennow K, Hansson O. Performance of fully automated plasma assays as screening tests for Alzheimer disease-related β-amyloid status. JAMA Neurol. 2019;76:1060–9.
Wilson DH, Rissin DM, Kan CW, Fournier DR, Piech T, Campbell TG, Meyer RE, Fishburn MW, Cabrera C, Patel PP, et al. The Simoa HD-1 analyzer: a novel fully automated digital immunoassay analyzer with single-molecule sensitivity and multiplexing. J Lab Autom. 2016;21:533–47.
Keshavan A, Pannee J, Karikari TK, Rodriguez JL, Ashton NJ, Nicholas JM, Cash DM, Coath W, Lane CA, Parker TD, et al. Population-based blood screening for preclinical Alzheimer’s disease in a British birth cohort at age 70. Brain. 2021;144:434–49.
Aldo P, Marusov G, Svancara D, David J, Mor G. Simple Plex(™): a novel multi-analyte, automated microfluidic immunoassay platform for the detection of human and mouse cytokines and chemokines. Am J Reprod Immunol. 2016;75:678–93.
Kaneko N, Nakamura A, Washimi Y, Kato T, Sakurai T, Arahata Y, Bundo M, Takeda A, Niida S, Ito K, et al. Novel plasma biomarker surrogating cerebral amyloid deposition. Proc Jpn Acad Ser B Phys Biol Sci. 2014;90:353–64.
Kirmess KM, Meyer MR, Holubasch MS, Knapik SS, Hu Y, Jackson EN, Harpstrite SE, Verghese PB, West T, Fogelman I, et al. The PrecivityAD™ test: accurate and reliable LC-MS/MS assays for quantifying plasma amyloid beta 40 and 42 and apolipoprotein E proteotype for the assessment of brain amyloidosis. Clin Chim Acta. 2021;519:267–75.
Hong C-Y, Wu CC, Chiu YC, Yang SY, Horng HE, Yang HC. Magnetic susceptibility reduction method for magnetically labeled immunoassay. Appl Phys Lett. 2006;88:212512.
Chieh JJ, Yang SY, Jian ZF, Wang WC, Horng HE, Yang HC, Hong C-Y. Hyper-high-sensitivity wash-free magnetoreduction assay on biomolecules using high-Tc superconducting quantum interference devices. J Appl Phys. 2008;103:014703.
Yang Y-H, Situmeang RFV, Ong PA. Can blood amyloid levels be used as a biomarker for Alzheimer’s disease? Brain Sci Adv. 2021;7:17–25.
Lue L-F, Kuo Y-M, Sabbagh M. Advance in plasma AD core biomarker development: current findings from immunomagnetic reduction-based SQUID technology. Neurol Ther. 2019;8:95–111.
Kim Y, Yoo YK, Kim HY, Roh JH, Kim J, Baek S, Lee JC, Kim HJ, Chae M-S, Jeong D, et al. Comparative analyses of plasma amyloid-β levels in heterogeneous and monomerized states by interdigitated microelectrode sensor system. Sci Adv. 2019;5:eaav1388.
Wilson EN, Young CB, Ramos Benitez J, Swarovski MS, Feinstein I, Vandijck M, Le Guen Y, Kasireddy NM, Shahid M, Corso NK, et al. Performance of a fully-automated Lumipulse plasma phospho-tau181 assay for Alzheimer’s disease. Alzheimers Res Ther. 2022;14:172.
Gauthier A, Viel S, Perret M, Brocard G, Casey R, Lombard C, Laurent-Chabalier S, Debouverie M, Edan G, Vukusic S, et al. Comparison of SimoaTM and EllaTM to assess serum neurofilament-light chain in multiple sclerosis. Ann Clin Transl Neurol. 2021;8:1141–50.
Truffi M, Garofalo M, Ricciardi A, Cotta Ramusino M, Perini G, Scaranzin S, Gastaldi M, Albasini S, Costa A, Chiavetta V, et al. Neurofilament-light chain quantification by Simoa and Ella in plasma from patients with dementia: a comparative study. Sci Rep. 2023;13:4041.
Ding XL, Tuo QZ, Lei P. An introduction to ultrasensitive assays for plasma tau detection. J Alzheimers Dis. 2021;80:1353–62.
Lue LF, Guerra A, Walker DG. Amyloid beta and tau as Alzheimer’s disease blood biomarkers: promise from new technologies. Neurol Ther. 2017;6:25–36.
Li D, Mielke MM. An update on blood-based markers of Alzheimer’s disease using the SiMoA platform. Neurol Ther. 2019;8:73–82.
Barthélemy NR, Horie K, Sato C, Bateman RJ. Blood plasma phosphorylated-tau isoforms track CNS change in Alzheimer’s disease. J Exp Med. 2020;217(11):e20200861.
Jang H, Kim JS, Lee HJ, Kim C-H, Na DL, Kim HJ, Allué JA, Sarasa L, Castillo S, Pesini P, et al. Performance of the plasma Aβ42/Aβ40 ratio, measured with a novel HPLC-MS/MS method, as a biomarker of amyloid PET status in a DPUK-KOREAN cohort. Alzheimers Res Ther. 2021;13:179.
Montoliu-Gaya L, Benedet AL, Tissot C, Vrillon A, Ashton NJ, Brum WS, Lantero-Rodriguez J, Stevenson J, Nilsson J, Sauer M, et al. Mass spectrometric simultaneous quantification of tau species in plasma shows differential associations with amyloid and tau pathologies. Nat Aging. 2023;3:661–9.
Palmqvist S, Stomrud E, Cullen N, Janelidze S, Manuilova E, Jethwa A, Bittner T, Eichenlaub U, Suridjan I, Kollmorgen G, et al. An accurate fully automated panel of plasma biomarkers for Alzheimer’s disease. Alzheimers Dement. 2023;19:1204–15.
Feng W, Beer JC, Hao Q, Ariyapala IS, Sahajan A, Komarov A, Cha K, Moua M, Qiu X, Xu X, et al. NULISA: a proteomic liquid biopsy platform with attomolar sensitivity and high multiplexing. Nat Commun. 2023;14:7238.
Yang S-Y, Chiu M-J, Chen T-F, Horng H-E. Detection of plasma biomarkers using immunomagnetic reduction: a promising method for the early diagnosis of Alzheimer’s disease. Neurol Ther. 2017;6:37–56.
Pannee J, Shaw LM, Korecka M, Waligorska T, Teunissen CE, Stoops E, Vanderstichele HMJ, Mauroo K, Verberk IMW, Keshavan A, et al. The global Alzheimer’s Association round robin study on plasma amyloid β methods. Alzheimers Dement (Amst). 2021;13:e12242.
Korecka M, Shaw LM. Mass spectrometry-based methods for robust measurement of Alzheimer’s disease biomarkers in biological fluids. J Neurochem. 2021;159:211–33.
Palmqvist S, Zetterberg H, Mattsson N, Johansson P, Minthon L, Blennow K, Olsson M, Hansson O. Detailed comparison of amyloid PET and CSF biomarkers for identifying early Alzheimer disease. Neurology. 2015;85:1240–9.
Palmqvist S, Zetterberg H, Blennow K, Vestberg S, Andreasson U, Brooks DJ, Owenius R, Hägerström D, Wollmer P, Minthon L, Hansson O. Accuracy of brain amyloid detection in clinical practice using cerebrospinal fluid β-amyloid 42: a cross-validation study against amyloid positron emission tomography. JAMA Neurol. 2014;71:1282–9.
Qu Y, Ma Y-H, Huang Y-Y, Ou Y-N, Shen X-N, Chen S-D, Dong Q, Tan L, Yu J-T. Blood biomarkers for the diagnosis of amnestic mild cognitive impairment and Alzheimer’s disease: a systematic review and meta-analysis. Neurosci Biobehav Rev. 2021;128:479–86.
Koychev I, Jansen K, Dette A, Shi L, Holling H. Blood-based ATN biomarkers of Alzheimer’s disease: a meta-analysis. J Alzheimers Dis. 2021;79:177–95.
Janelidze S, Teunissen CE, Zetterberg H, Allué JA, Sarasa L, Eichenlaub U, Bittner T, Ovod V, Verberk IMW, Toba K, et al. Head-to-head comparison of 8 plasma amyloid-β 42/40 assays in Alzheimer disease. JAMA Neurol. 2021;78:1375–82.
Mattsson N, Insel PS, Palmqvist S, Portelius E, Zetterberg H, Weiner M, Blennow K, Hansson O. Initiative tAsDN: cerebrospinal fluid tau, neurogranin, and neurofilament light in Alzheimer’s disease. EMBO Mol Med. 2016;8:1184–96.
Zetterberg H, Wilson D, Andreasson U, Minthon L, Blennow K, Randall J, Hansson O. Plasma tau levels in Alzheimer’s disease. Alzheimers Res Ther. 2013;5:9.
Mattsson N, Zetterberg H, Janelidze S, Insel PS, Andreasson U, Stomrud E, Palmqvist S, Baker D, Tan Hehir CA, Jeromin A, et al. Plasma tau in Alzheimer disease. Neurology. 2016;87:1827–35.
Chen Z, Mengel D, Keshavan A, Rissman RA, Billinton A, Perkinton M, Percival-Alwyn J, Schultz A, Properzi M, Johnson K, et al. Learnings about the complexity of extracellular tau aid development of a blood-based screen for Alzheimer’s disease. Alzheimers Dement. 2019;15:487–96.
Snellman A, Lantero-Rodriguez J, Emeršič A, Vrillon A, Karikari TK, Ashton NJ, Gregorič Kramberger M, Čučnik S, Paquet C, Rot U, et al. N-terminal and mid-region tau fragments as fluid biomarkers in neurological diseases. Brain. 2022;145:2834–48.
Ashton NJ, Benedet AL, Pascoal TA, Karikari TK, Lantero-Rodriguez J, Brum WS, Mathotaarachchi S, Therriault J, Savard M, Chamoun M, et al. Cerebrospinal fluid p-tau231 as an early indicator of emerging pathology in Alzheimer’s disease. eBioMedicine. 2022;76:103836.
Karikari TK, Emeršič A, Vrillon A, Lantero-Rodriguez J, Ashton NJ, Kramberger MG, Dumurgier J, Hourregue C, Čučnik S, Brinkmalm G, et al. Head-to-head comparison of clinical performance of CSF phospho-tau T181 and T217 biomarkers for Alzheimer’s disease diagnosis. Alzheimers Dement. 2021;17:755–67.
Karikari TK, Pascoal TA, Ashton NJ, Janelidze S, Benedet AL, Rodriguez JL, Chamoun M, Savard M, Kang MS, Therriault J, et al. Blood phosphorylated tau 181 as a biomarker for Alzheimer’s disease: a diagnostic performance and prediction modelling study using data from four prospective cohorts. Lancet Neurol. 2020;19:422–33.
Karikari TK, Benedet AL, Ashton NJ, Lantero Rodriguez J, Snellman A, Suárez-Calvet M, Saha-Chaudhuri P, Lussier F, Kvartsberg H, Rial AM, et al. Diagnostic performance and prediction of clinical progression of plasma phospho-tau181 in the Alzheimer’s Disease Neuroimaging Initiative. Mol Psychiatry. 2021;26:429–42.
Ashton NJ, Pascoal TA, Karikari TK, Benedet AL, Lantero-Rodriguez J, Brinkmalm G, Snellman A, Schöll M, Troakes C, Hye A, et al. Plasma p-tau231: a new biomarker for incipient Alzheimer’s disease pathology. Acta Neuropathol. 2021;141:709–24.
Palmqvist S, Janelidze S, Quiroz YT, Zetterberg H, Lopera F, Stomrud E, Su Y, Chen Y, Serrano GE, Leuzy A, et al. Discriminative accuracy of plasma phospho-tau217 for Alzheimer disease vs other neurodegenerative disorders. JAMA. 2020;324:772–81.
Mielke MM, Frank RD, Dage JL, Jeromin A, Ashton NJ, Blennow K, Karikari TK, Vanmechelen E, Zetterberg H, Algeciras-Schimnich A, et al. Comparison of plasma phosphorylated tau species with amyloid and tau positron emission tomography, neurodegeneration, vascular pathology, and cognitive outcomes. JAMA Neurol. 2021;78:1108–17.
Ashton NJ, Puig-Pijoan A, Milà-Alomà M, Fernández-Lebrero A, García-Escobar G, González-Ortiz F, Kac PR, Brum WS, Benedet AL, Lantero-Rodriguez J, et al. Plasma and CSF biomarkers in a memory clinic: Head-to-head comparison of phosphorylated tau immunoassays. Alzheimers Dement. 2023;19:1913–24.
Bayoumy S, Verberk IMW, den Dulk B, Hussainali Z, Zwan M, van der Flier WM, Ashton NJ, Zetterberg H, Blennow K, Vanbrabant J, et al. Clinical and analytical comparison of six Simoa assays for plasma P-tau isoforms P-tau181, P-tau217, and P-tau231. Alzheimers Res Ther. 2021;13:198.
Janelidze S, Bali D, Ashton NJ, Barthélemy NR, Vanbrabant J, Stoops E, Vanmechelen E, He Y, Dolado AO, Triana-Baltzer G, et al. Head-to-head comparison of 10 plasma phospho-tau assays in prodromal Alzheimer’s disease. Brain. 2023;146:1592–601.
Kac PR, González-Ortiz F, Emeršič A, Dulewicz M, Koutarapu S, Turton M, An Y, Smirnov D, Kulczyńska-Przybik A, Varma V, et al. Plasma p-tau212: antemortem diagnostic performance and prediction of autopsy verification of Alzheimer’s disease neuropathology. medRxiv. 2023:2023.2012.2011.23299806.
Ashton NJ, Janelidze S, Mattsson-Carlgren N, Binette AP, Strandberg O, Brum WS, Karikari TK, González-Ortiz F, Di Molfetta G, Meda FJ, et al. Differential roles of Aβ42/40, p-tau231 and p-tau217 for Alzheimer’s trial selection and disease monitoring. Nat Med. 2022;28:2555–62.
Yang Z, Wang KK. Glial fibrillary acidic protein: from intermediate filament assembly and gliosis to neurobiomarker. Trends Neurosci. 2015;38:364–74.
Monterey MD, Wei H, Wu X, Wu JQ. The many faces of astrocytes in Alzheimer’s disease. Front Neurol. 2021;12:619626.
Chatterjee P, Pedrini S, Stoops E, Goozee K, Villemagne VL, Asih PR, Verberk IMW, Dave P, Taddei K, Sohrabi HR, et al. Plasma glial fibrillary acidic protein is elevated in cognitively normal older adults at risk of Alzheimer’s disease. Transl Psychiatry. 2021;11:27.
Benedet AL, Milà-Alomà M, Vrillon A, Ashton NJ, Pascoal TA, Lussier F, Karikari TK, Hourregue C, Cognat E, Dumurgier J, et al. Differences between plasma and cerebrospinal fluid glial fibrillary acidic protein levels across the Alzheimer disease continuum. JAMA Neurol. 2021;78:1471–83.
Papa L, Ladde JG, O’Brien JF, Thundiyil JG, Tesar J, Leech S, Cassidy DD, Roa J, Hunter C, Miller S, et al. Evaluation of glial and neuronal blood biomarkers compared with clinical decision rules in assessing the need for computed tomography in patients with mild traumatic brain injury. JAMA Netw Open. 2022;5:e221302.
Sjögren M, Blomberg M, Jonsson M, Wahlund LO, Edman A, Lind K, Rosengren L, Blennow K, Wallin A. Neurofilament protein in cerebrospinal fluid: a marker of white matter changes. J Neurosci Res. 2001;66:510–6.
Gaiottino J, Norgren N, Dobson R, Topping J, Nissim A, Malaspina A, Bestwick JP, Monsch AU, Regeniter A, Lindberg RL, et al. Increased neurofilament light chain blood levels in neurodegenerative neurological diseases. PLoS One. 2013;8:e75091.
Ashton NJ, Janelidze S, Al Khleifat A, Leuzy A, van der Ende EL, Karikari TK, Benedet AL, Pascoal TA, Lleó A, Parnetti L, et al. A multicentre validation study of the diagnostic value of plasma neurofilament light. Nat Commun. 2021;12:3400.
Ashton NJ, Suárez-Calvet M, Karikari TK, Lantero-Rodriguez J, Snellman A, Sauer M, Simrén J, Minguillon C, Fauria K, Blennow K, Zetterberg H. Effects of pre-analytical procedures on blood biomarkers for Alzheimer’s pathophysiology, glial activation, and neurodegeneration. Alzheimers Dement (Amst). 2021;13:e12168.
O’Connell GC, Alder ML, Webel AR, Moore SM. Neuro biomarker levels measured with high-sensitivity digital ELISA differ between serum and plasma. Bioanalysis. 2019;11:2087–94.
Kac PR, Gonzalez-Ortiz F, Simrén J, Dewit N, Vanmechelen E, Zetterberg H, Blennow K, Ashton NJ, Karikari TK. Diagnostic value of serum versus plasma phospho-tau for Alzheimer’s disease. Alzheimers Res Ther. 2022;14:65.
Jonaitis EM, Zetterberg H, Koscik RL, Betthauser TJ, Van Hulle CA, Hogan K, Hegge L, Kollmorgen G, Suridjan I, Gleason CE, et al. Crosswalk study on blood collection-tube types for Alzheimer’s disease biomarkers. Alzheimers Dement. 2022;14:e12266.
Google Scholar
Gonzalez-Ortiz F, Dias A, Turton M, Magalhães R, Kac PR, Correia M, Harrison P, Zetterberg H, Maia LF, Blennow K, Karikari TK. Preanalytical stability of plasma/serum brain-derived tau. Alzheimers Dement. 2023;19(10):4764–70.
Gonzalez-Ortiz F, Ferreira PCL, González-Escalante A, Montoliu-Gaya L, Ortiz-Romero P, Kac PR, Turton M, Kvartsberg H, Ashton NJ, Zetterberg H, et al. A novel ultrasensitive assay for plasma p-tau217: performance in individuals with subjective cognitive decline and early Alzheimer’s disease. Alzheimers Dement. 2023. https://doi.org/10.1002/alz.13525 .
Tumani H, Hegen H. CSF albumin: albumin CSF/serum ratio (marker for blood-CSF barrier function). 2015. pp. 111–114.
Mayo Clinic Test Catalog. https://www.mayocliniclabs.com/en/test-catalog .
Bowen RA, Remaley AT. Interferences from blood collection tube components on clinical chemistry assays. Biochem Med (Zagreb). 2014;24:31–44.
Rózga M, Bittner T, Batrla R, Karl J. Preanalytical sample handling recommendations for Alzheimer’s disease plasma biomarkers. Alzheimers Dement (Amst). 2019;11:291–300.
van Lierop Z, Verberk IMW, van Uffelen KWJ, Koel-Simmelink MJA, In ‘t Veld L, Killestein J, Teunissen CE. Pre-analytical stability of serum biomarkers for neurological disease: neurofilament-light, glial fibrillary acidic protein and contactin-1. Clin Chem Lab Med. 2022;60:842–50.
Jiang L, Ding X, Wang W, Yang X, Li T, Lei P. Head-to-head comparison of different blood collecting tubes for quantification of Alzheimer’s disease biomarkers in plasma. Biomolecules. 2022;12:1194.
Walsh G. Difficult peripheral venous access: recognizing and managing the patient at risk. J Assoc Vasc Access. 2008;13:198–203.
Walter M, Wiltfang J, Vogelgsang J. Pre-analytical sampling and storage conditions of amyloid-β peptides in venous and capillary blood. J Alzheimers Dis. 2020;78:529–35.
Lombardi V, Carassiti D, Giovannoni G, Lu CH, Adiutori R, Malaspina A. The potential of neurofilaments analysis using dry-blood and plasma spots. Sci Rep. 2020;10:97.
Simrén J, Ashton NJ, Blennow K, Zetterberg H. Blood neurofilament light in remote settings: alternative protocols to support sample collection in challenging pre-analytical conditions. Alzheimers Dement. 2021;13:e12145.
Meyer P-F, Ashton NJ, Karikari TK, Strikwerda-Brown C, Köbe T, Gonneaud J, Pichet Binette A, Ozlen H, Yakoub Y, Simrén J, et al. Plasma p-tau231, p-tau181, PET biomarkers, and cognitive change in older adults. Ann Neurol. 2022;91:548–60.
Brum WS, Ashton NJ, Simrén J, di Molfetta G, Karikari TK, Benedet AL, Zimmer ER, Lantero-Rodriguez J, Montoliu-Gaya L, Jeromin A, et al. Biological variation estimates of Alzheimer’s disease plasma biomarkers in healthy individuals. Alzheimers Dement. 2024;20:1284–97.
Heyer NJ, Derzon JH, Winges L, Shaw C, Mass D, Snyder SR, Epner P, Nichols JH, Gayken JA, Ernst D, Liebow EB. Effectiveness of practices to reduce blood sample hemolysis in EDs: a laboratory medicine best practices systematic review and meta-analysis. Clin Biochem. 2012;45:1012–32.
Dugan L, Leech L, Speroni KG, Corriher J. Factors affecting hemolysis rates in blood samples drawn from newly placed IV sites in the emergency department. J Emerg Nurs. 2005;31:338–45.
Bowen RA, Hortin GL, Csako G, Otañez OH, Remaley AT. Impact of blood collection devices on clinical chemistry assays. Clin Biochem. 2010;43:4–25.
Bowen RA, Sattayapiwat A, Gounden V, Remaley AT. Blood collection tube-related alterations in analyte concentrations in quality control material and serum specimens. Clin Biochem. 2014;47:150–7.
Schrapp A, Mory C, Duflot T, Pereira T, Imbert L, Lamoureux F. The right blood collection tube for therapeutic drug monitoring and toxicology screening procedures: standard tubes, gel or mechanical separator? Clin Chim Acta. 2019;488:196–201.
Lewczuk P, Beck G, Esselmann H, Bruckmoser R, Zimmermann RD, Fiszer M, Bibl M, Maler JM, Kornhuber J, Wiltfang J. Effect of sample collection tubes on cerebrospinal fluid concentrations of tau proteins and amyloid β peptides. Clin Chem. 2006;52:332–4.
Perret-Liaudet A, Pelpel M, Tholance Y, Dumont B, Vanderstichele H, Zorzi W, ElMoualij B, Schraen S, Moreaud O, Gabelle A, et al. Cerebrospinal fluid collection tubes: a critical issue for Alzheimer disease diagnosis. Clin Chem. 2012;58:787–9.
Bjerke M, Portelius E, Minthon L, Wallin A, Anckarsäter H, Anckarsäter R, Andreasen N, Zetterberg H, Andreasson U, Blennow K. Confounding factors influencing amyloid Beta concentration in cerebrospinal fluid. Int J Alzheimers Dis. 2010;2010:986310.
Pica-Mendez AM, Tanen M, Dallob A, Tanaka W, Laterza OF. Nonspecific binding of Aβ42 to polypropylene tubes and the effect of Tween-20. Clin Chim Acta. 1833;2010:411.
Toombs J, Paterson RW, Schott JM, Zetterberg H. Amyloid-beta 42 adsorption following serial tube transfer. Alzheimers Res Ther. 2014;6:5.
Lachno DR, Vanderstichele H, De Groote G, Kostanjevecki V, De Meyer G, Siemers ER, Willey MB, Bourdage JS, Konrad RJ, Dean RA. The influence of matrix type, diurnal rhythm and sample collection and processing on the measurement of plasma β-amyloid isoforms using the INNO-BIA plasma Aβ forms multiplex assay. J Nutr Health Aging. 2009;13:220–5.
(CLSI). CaLSI: collection, transport, and processing of blood specimens for testing plasma-based coagulation assays and molecular hemostasis assays; approved guideline—fourth edition. CLSI document H21–A4. Wayne: Clinical and Laboratory Standards Institute; 2017.
Reichstein E. The importance of preanalytical factors in immunodiagnostic testing. EJIFCC. 2003;14:124–7.
Timms JF, Arslan-Low E, Gentry-Maharaj A, Luo Z, T’Jampens D, Podust VN, Ford J, Fung ET, Gammerman A, Jacobs I, Menon U. Preanalytic influence of sample handling on SELDI-TOF serum protein profiles. Clin Chem. 2007;53:645–56.
Sunde AL, Alsnes IV, Aarsland D, Ashton NJ, Tovar-Rios DA, De Santis G, Blennow K, Zetterberg H, Kjosavik SR. Preanalytical stability of plasma biomarkers for Alzheimer’s disease pathology. Alzheimers Dement. 2023;15:e12439.
Lippi G, Blanckaert N, Bonini P, Green S, Kitchen S, Palicka V, Vassault AJ, Plebani M. Haemolysis: an overview of the leading cause of unsuitable specimens in clinical laboratories. Clin Chem Lab Med. 2008;46:764–72.
Keshavan A, Heslegrave A, Zetterberg H, Schott JM. Stability of blood-based biomarkers of Alzheimer’s disease over multiple freeze-thaw cycles. Alzheimers Dement. 2018;10:448–51.
Lierop ZYGJv, Verberk IMW, Uffelen KWJv, Koel-Simmelink MJA, Veld Lit, Killestein J, Teunissen CE. Pre-analytical stability of serum biomarkers for neurological disease: neurofilament-light, glial fibrillary acidic protein and contactin-1. Clin Chem Lab Med. 2022;60:842–850.
Liu HC, Chiu MJ, Lin CH, Yang SY. Stability of plasma amyloid-β 1–40, amyloid-β 1–42, and total tau protein over repeated freeze/thaw cycles. Dement Geriatr Cogn Dis Extra. 2020;10:46–55.
Murphy BM, Swarts S, Mueller BM, van der Geer P, Manning MC, Fitchmun MI. Protein instability following transport or storage on dry ice. Nat Methods. 2013;10:278–9.
Schindler SE, Sutphen CL, Teunissen C, McCue LM, Morris JC, Holtzman DM, Mulder SD, Scheltens P, Xiong C, Fagan AM. Upward drift in cerebrospinal fluid amyloid β 42 assay values for more than 10 years. Alzheimers Dement. 2018;14:62–70.
Kang M, Ragan BG, Park JH. Issues in outcomes research: an overview of randomization techniques for clinical trials. J Athl Train. 2008;43:215–21.
Boulo S, Kuhlmann J, Andreasson U, Brix B, Venkataraman I, Herbst V, Rutz S, Manuilova E, Vandijck M, Dekeyser F, et al. First amyloid β1-42 certified reference material for re-calibrating commercial immunoassays. Alzheimers Dement. 2020;16:1493–503.
Download references
Acknowledgements
R01 AG064877 (MIK), P30 AG066468 (University of Pittsburgh ADRC, OIL as PI), R01 MH116046 (RAS, JK), P01 AG14449 and P01 AG025204 (MDI). HZ is a Wallenberg Scholar supported by grants from the Swedish Research Council (#2022-01018 and #2019-02397), the European Union’s Horizon Europe research and innovation programme under grant agreement No 101053962, Swedish State Support for Clinical Research (#ALFGBG-71320), the Alzheimer Drug Discovery Foundation (ADDF), USA (#201809-2016862), the AD Strategic Fund and the Alzheimer’s Association (#ADSF-21-831376-C, #ADSF-21-831381-C, and #ADSF-21-831377-C), the Bluefield Project, the Olav Thon Foundation, the Erling-Persson Family Foundation, Stiftelsen för Gamla Tjänarinnor, Hjärnfonden, Sweden (#FO2022-0270), the European Union’s Horizon 2020 research and innovation programme under the Marie Skłodowska-Curie grant agreement No 860197 (MIRIADE), the European Union Joint Programme – Neurodegenerative Disease Research (JPND2021-00694), the National Institute for Health and Care Research University College London Hospitals Biomedical Research Centre, and the UK Dementia Research Institute at UCL (UKDRI-1003). KB is supported by the Swedish Research Council (#2017-00915 and #2022-00732), Hjärnfonden, Sweden (#FO2017-0243 and #ALZ2022-0006), and the Swedish state under the agreement between the Swedish government and the County Councils, the ALF-agreement (#ALFGBG-715986 and #ALFGBG-965240). TKK was supported by the NIH (R01 AG083874, U24 AG082930, P30 AG066468, RF1 AG052525-01A1, R01 AG053952-05, R37 AG023651-17, RF1 AG025516-12A1, R01 AG073267-02, R01 AG075336-01, R01 AG072641-02, P01 AG025204-16), the Swedish Research Council (Vetenskåpradet; #2021-03244), the Alzheimer’s Association (#AARF-21-850325), the Swedish Alzheimer Foundation (Alzheimerfonden), the Aina (Ann) Wallströms and Mary-Ann Sjöbloms stiftelsen, and the Emil och Wera Cornells stiftelsen.
Author information
Authors and affiliations.
Department of Psychiatry, School of Medicine, University of Pittsburgh, 3811 O’Hara Street, Pittsburgh, PA, 15213, USA
Xuemei Zeng, Anuradha Sehrawat, Jihui Lee, Tara K. Lafferty, Robert A. Sweet, Dana L. Tudorascu, William E. Klunk, Milos D. Ikonomovic, Anne D. Cohen, Victor L. Villemagne, Tharick A. Pascoal & Thomas K. Karikari
Department of Chemistry, University of Pittsburgh, Pittsburgh, PA, 15213, USA
Department of Pathology, School of Medicine, University of Pittsburgh, Pittsburgh, PA, 15213, USA
Julia Kofler
Department of Neurology, School of Medicine, University of Pittsburgh, Pittsburgh, PA, 15213, USA
Sarah B. Berman, Robert A. Sweet, Milos D. Ikonomovic, Beth E. Snitz, Tharick A. Pascoal & Oscar I. Lopez
Geriatric Research Education and Clinical Center, VA Pittsburgh HS, Pittsburgh, PA, USA
Milos D. Ikonomovic
Department of Psychiatry and Neurochemistry, Institute of Neuroscience and Physiology, The Sahlgrenska Academy, University of Gothenburg, Mölndal, Sweden
Anna Pfister, Henrik Zetterberg, Kaj Blennow & Thomas K. Karikari
Clinical Neurochemistry Laboratory, Sahlgrenska University Hospital, Mölndal, Sweden
Anna Pfister, Henrik Zetterberg & Kaj Blennow
Department of Neurodegenerative Disease, UCL Queen Square Institute of Neurology, London, UK
Henrik Zetterberg
UK Dementia Research Institute at UCL, London, UK
Hong Kong Center for Neurodegenerative Diseases, Hong Kong, China
Department of Medicine, School of Medicine and Public Health, University of Wisconsin-Madison, Madison, WI, USA
Department of Human Genetics, School of Public Health, University of Pittsburgh, Pittsburgh, PA, 15213, USA
M. llyas Kamboh
You can also search for this author in PubMed Google Scholar
Contributions
XZ and TKK contributed to the conception and design of the review. XZ, YC, AS and TKL prepared the first draft of the manuscript, which was critically revised by all authors. All authors contributed to and approved the final version of the manuscript.
Authors’ information
Corresponding author.
Correspondence to Thomas K. Karikari .
Ethics declarations
Ethics approval and consent to participate.
Not applicable.
Consent for publication
Competing interests.
MDI has received research funding from GE Healthcare and Avid Radiopharmaceuticals. HZ has served at scientific advisory boards and/or as a consultant for Abbvie, Acumen, Alector, Alzinova, ALZPath, Annexon, Apellis, Artery Therapeutics, AZTherapies, CogRx, Denali, Eisai, Nervgen, Novo Nordisk, Optoceutics, Passage Bio, Pinteon Therapeutics, Prothena, Red Abbey Labs, reMYND, Roche, Samumed, Siemens Healthineers, Triplet Therapeutics, and Wave, has given lectures in symposia sponsored by Cellectricon, Fujirebio, Alzecure, Biogen, and Roche, and is a co-founder of Brain Biomarker Solutions in Gothenburg AB (BBS), which is a part of the GU Ventures Incubator Program (outside submitted work). KB has served as a consultant and at advisory boards for Acumen, ALZPath, BioArctic, Biogen, Eisai, Lilly, Moleac Pte. Ltd, Novartis, Ono Pharma, Prothena, Roche Diagnostics, and Siemens Healthineers; has served at data monitoring committees for Julius Clinical and Novartis; has given lectures, produced educational materials and participated in educational programs for AC Immune, Biogen, Celdara Medical, Eisai and Roche Diagnostics; and is a co-founder of Brain Biomarker Solutions in Gothenburg AB (BBS), which is a part of the GU Ventures Incubator Program, outside the work presented in this paper. The other authors declare no competing interests.
Additional information
Publisher’s note.
Springer Nature remains neutral with regard to jurisdictional claims in published maps and institutional affiliations.
Supplementary Information
Additional file 1..
Step-by-step blood specimen collection procedures.
Additional file 2.
Simulation datasets used to generate the plots in Fig. 4 .
Rights and permissions
Open Access This article is licensed under a Creative Commons Attribution 4.0 International License, which permits use, sharing, adaptation, distribution and reproduction in any medium or format, as long as you give appropriate credit to the original author(s) and the source, provide a link to the Creative Commons licence, and indicate if changes were made. The images or other third party material in this article are included in the article's Creative Commons licence, unless indicated otherwise in a credit line to the material. If material is not included in the article's Creative Commons licence and your intended use is not permitted by statutory regulation or exceeds the permitted use, you will need to obtain permission directly from the copyright holder. To view a copy of this licence, visit http://creativecommons.org/licenses/by/4.0/ . The Creative Commons Public Domain Dedication waiver ( http://creativecommons.org/publicdomain/zero/1.0/ ) applies to the data made available in this article, unless otherwise stated in a credit line to the data.
Reprints and permissions
About this article
Cite this article.
Zeng, X., Chen, Y., Sehrawat, A. et al. Alzheimer blood biomarkers: practical guidelines for study design, sample collection, processing, biobanking, measurement and result reporting. Mol Neurodegeneration 19 , 40 (2024). https://doi.org/10.1186/s13024-024-00711-1
Download citation
Received : 25 July 2023
Accepted : 13 February 2024
Published : 15 May 2024
DOI : https://doi.org/10.1186/s13024-024-00711-1
Share this article
Anyone you share the following link with will be able to read this content:
Sorry, a shareable link is not currently available for this article.
Provided by the Springer Nature SharedIt content-sharing initiative
- Blood biomarker
- Standardization
- Preanalytical factors
- Neurodegeneration
Molecular Neurodegeneration
ISSN: 1750-1326
- Submission enquiries: Access here and click Contact Us
- General enquiries: [email protected]
- Frontiers in Molecular Biosciences
- Molecular Diagnostics and Therapeutics
- Research Topics
Recent Advances in Breath Analysis: Exploring Exhaled Breath Biomarkers for Disease Diagnostics
Total Downloads
Total Views and Downloads
About this Research Topic
Breathomics is a branch of metabolomics that analyzes various volatile organic compounds (VOCs) from exhaled breath samples. It has been rapidly growing as a non-invasive diagnostic tool to probe or infer the pathogenic or physiological status of the human body, often yielding crucial information for disease diagnostics. Unlike traditional diagnostic methods that often require invasive procedures or complex laboratory analyses, breath analysis offers a simple, cost-effective, and patient-friendly approach that can be easily integrated into routine clinical practice. The non-invasive nature of breath sample collection makes breathomics particularly attractive for disease screening, monitoring, and personalized medicine. Understanding the link between breath molecules and diseases has gained significant advances due to recent developments in more reliable detection techniques and standardized breath sample collection methods. Additionally, the recent rapid progress in algorithm development, including machine learning and artificial intelligence, has unveiled intriguing associations between breath VOCs and diseases that were previously convoluted by multiple factors. With successful clinical applications of gastrointestinal and respiratory diagnostics, breath analysis has expanded to broader fields such as thoracic diseases, neurological disorders, pharmacokinetics, and more. The surge in registered clinical trials employing breath analysis and breathomics underscores the growing significance and potential of this innovative approach in modern healthcare. The objective of this Research Topic is to provide a comprehensive overview of the recent advancements in breathomics, with a particular focus on exploring the association between exhaled breath VOCs and diseases. The aim is to delve deeper into the scientific underpinnings of this emerging field and to assess its potential in modern healthcare. Focusing on the following four areas: • Investigating the mechanism of breath biomarkers and diseases, including assessing unique VOC signatures associated with different diseases, potential VOC profiles for early disease screening, and influences of various factors such as diet, lifestyle, and environmental exposure on breath VOC composition. • Advancements in breath analysis technologies, including novel analytical techniques for the detection and quantification of VOCs in exhaled breath and applications of standardized protocols for breath sample collection, storage, and analysis. • Machine learning and AI-driven data processing in breathomics, including the development of predictive models for disease classification and risk prediction, integration of multi-omics data with breathomics data for comprehensive disease profiling, and validation strategies for assessing the robustness and generalizability of AI-driven models. • Clinical applications of breathomics, including evaluation of breathomics-based diagnostic tools in clinical settings, monitoring disease progression and treatment response through longitudinal breath analysis or pharmacokinetics studies, and exploring the potential of breathomics in personalized medicine and disease screening. Topic editor Meixiu Sun is employed by WIM Spirare Health Technology Limited. The other Topic Editors declare no potential conflicts of interest with regards to the Research.
Keywords : breath analysis, breathomics, volatile organic compounds (VOC), disease diagnostics, machine learning, artificial intelligence
Important Note : All contributions to this Research Topic must be within the scope of the section and journal to which they are submitted, as defined in their mission statements. Frontiers reserves the right to guide an out-of-scope manuscript to a more suitable section or journal at any stage of peer review.
Topic Editors
Topic coordinators, submission deadlines, participating journals.
Manuscripts can be submitted to this Research Topic via the following journals:
total views
- Demographics
No records found
total views article views downloads topic views
Top countries
Top referring sites, about frontiers research topics.
With their unique mixes of varied contributions from Original Research to Review Articles, Research Topics unify the most influential researchers, the latest key findings and historical advances in a hot research area! Find out more on how to host your own Frontiers Research Topic or contribute to one as an author.

Assessing DxGPT: Diagnosing Rare Diseases with Various Large Language Models
- Find this author on Google Scholar
- Find this author on PubMed
- Search for this author on this site
- For correspondence: [email protected]
- Info/History
- Preview PDF
Diagnosing rare diseases is a significant challenge in healthcare, with patients often experiencing long delays and misdiagnoses. The large number of rare diseases and the difficulty for doctors to be familiar with all of them contribute to this problem. Artificial intelligence, particularly large language models (LLMs), has shown promise in improving the diagnostic process by leveraging their extensive knowledge to help doctors navigate the complexities of diagnosing rare diseases.
Foundation 29 presents a comprehensive evaluation of DxGPT, a web-based platform designed to assist healthcare professionals in the diagnostic process for rare diseases. The platform currently utilizes GPT-4, but this study also compares its performance with other large language models, including Claude 3, Gemini 1.5 Pro, Llama, Mistral, Mixtral, and Cohere Command R+. It is crucial to emphasize that DxGPT is not a medical device but rather a decision support tool that aims to aid in clinical reasoning.
This study extends beyond initial synthetic patient cases, incorporating real-world data from the RAMEDIS and Peking Union Medical College Hospital (PUMCH) datasets. The analysis followed two main metrics: Strict Accuracy (P1), how often the first diagnostic suggestion agreed with the real diagnosis, and Top-5 Accuracy (P1 + P5), how often the right diagnosis was in the top five suggestions. The results show a complex picture of diagnostic accuracy, with performance varying significantly across models and datasets:
On the synthetic dataset, closed models like GPT-4, Claude, and Gemini exhibited relatively high accuracy. Open models like Llama 3 and Mixtral performed reasonably well, though lagging behind.
On the RAMEDIS rare disease cases, Claude 3 Opus model demonstrated 55% Strict Accuracy and 70% Top-5 Accuracy, outperforming other closed models. Open models like Llama 3 and Mixtral showed moderate accuracy.
The PUMCH dataset proved challenging for all models, with the highest Strict Accuracy at 59.46% (GPT-4 Turbo 1106) and Top-5 Accuracy at 64.86%.
These findings demonstrate the potential of DxGPT and LLMs in improving diagnostic methods for rare diseases. However, they also emphasize the need for further validation, particularly in real-world clinical settings, and comparison with human expert diagnoses. Successful integration of AI into medical diagnostics will require collaboration between researchers, clinicians, and regulatory bodies to ensure safety, efficacy, and ethical deployment.
Competing Interest Statement
The authors have declared no competing interest.
Funding Statement
Foundation 29 received a grant from Takeda to develop pilot #1 for Global Rare Disease Commission https://www.globalrarediseasecommission.com/Report/ . Pilot #1 is about exploring how to use artificial intelligence for RD diagnosis. Produced work is publically available at www.dx29.ai . Foundation 29 received a grant from GW Pharma to develop www.dx29.ai . This an open source tool and free of charge tool for physicians to accelerate time to diagnosis for patients with rare diseses. Produced work is publically available at www.dx29.ai . Foundation 29 received a grant from UCB Pharma to develop https://dxgpt.app/ . This an open source tool based on GPT-4 Azure OpenAI model and is free of charge tool for physicians and patients to accelerate time to diagnosis for patients with rare diseses. Produced work is publically available at https://dxgpt.app/ and https://github.com/foundation29org/dxgpt_testing . Foundation 29 received a grant from Italfarmaco to develop https://dxgpt.app/ . This an open source tool based on GPT-4 Azure OpenAI model and is free of charge tool for physicians and patients to accelerate time to diagnosis for patients with rare diseses. Produced work is publically available at https://dxgpt.app/ and https://github.com/foundation29org/dxgpt_testing . These grants are not related to any of these pharma's products. This study was funded by all these 4 grants as part of DxGPT development.
Author Declarations
I confirm all relevant ethical guidelines have been followed, and any necessary IRB and/or ethics committee approvals have been obtained.
The details of the IRB/oversight body that provided approval or exemption for the research described are given below:
https://huggingface.co/datasets/chenxz/RareBench
I confirm that all necessary patient/participant consent has been obtained and the appropriate institutional forms have been archived, and that any patient/participant/sample identifiers included were not known to anyone (e.g., hospital staff, patients or participants themselves) outside the research group so cannot be used to identify individuals.
I understand that all clinical trials and any other prospective interventional studies must be registered with an ICMJE-approved registry, such as ClinicalTrials.gov. I confirm that any such study reported in the manuscript has been registered and the trial registration ID is provided (note: if posting a prospective study registered retrospectively, please provide a statement in the trial ID field explaining why the study was not registered in advance).
I have followed all appropriate research reporting guidelines, such as any relevant EQUATOR Network research reporting checklist(s) and other pertinent material, if applicable.
juanjodoolmo{at}foundation29.org
Data Availability
All data produced are available online at: https://huggingface.co/datasets/chenxz/RareBench and https://github.com/foundation29org/dxgpt_testing
https://github.com/foundation29org/dxgpt_testing
View the discussion thread.
Thank you for your interest in spreading the word about medRxiv.
NOTE: Your email address is requested solely to identify you as the sender of this article.

Citation Manager Formats
- EndNote (tagged)
- EndNote 8 (xml)
- RefWorks Tagged
- Ref Manager
- Tweet Widget
- Facebook Like
- Google Plus One
Subject Area
- Health Informatics
- Addiction Medicine (324)
- Allergy and Immunology (627)
- Anesthesia (163)
- Cardiovascular Medicine (2373)
- Dentistry and Oral Medicine (289)
- Dermatology (206)
- Emergency Medicine (379)
- Endocrinology (including Diabetes Mellitus and Metabolic Disease) (836)
- Epidemiology (11770)
- Forensic Medicine (10)
- Gastroenterology (702)
- Genetic and Genomic Medicine (3738)
- Geriatric Medicine (350)
- Health Economics (633)
- Health Informatics (2395)
- Health Policy (933)
- Health Systems and Quality Improvement (896)
- Hematology (341)
- HIV/AIDS (782)
- Infectious Diseases (except HIV/AIDS) (13310)
- Intensive Care and Critical Care Medicine (767)
- Medical Education (365)
- Medical Ethics (104)
- Nephrology (398)
- Neurology (3502)
- Nursing (198)
- Nutrition (525)
- Obstetrics and Gynecology (674)
- Occupational and Environmental Health (664)
- Oncology (1823)
- Ophthalmology (537)
- Orthopedics (219)
- Otolaryngology (287)
- Pain Medicine (232)
- Palliative Medicine (66)
- Pathology (446)
- Pediatrics (1033)
- Pharmacology and Therapeutics (426)
- Primary Care Research (420)
- Psychiatry and Clinical Psychology (3175)
- Public and Global Health (6139)
- Radiology and Imaging (1280)
- Rehabilitation Medicine and Physical Therapy (747)
- Respiratory Medicine (826)
- Rheumatology (379)
- Sexual and Reproductive Health (372)
- Sports Medicine (323)
- Surgery (402)
- Toxicology (50)
- Transplantation (172)
- Urology (145)
Artificial intelligence is being used in healthcare for everything from answering patient questions to assisting with surgeries and developing new pharmaceuticals.
According to Statista , the artificial intelligence (AI) healthcare market, which is valued at $11 billion in 2021, is projected to be worth $187 billion in 2030. That massive increase means we will likely continue to see considerable changes in how medical providers, hospitals, pharmaceutical and biotechnology companies, and others in the healthcare industry operate.
Better machine learning (ML) algorithms, more access to data, cheaper hardware, and the availability of 5G have contributed to the increasing application of AI in the healthcare industry, accelerating the pace of change. AI and ML technologies can sift through enormous volumes of health data—from health records and clinical studies to genetic information—and analyze it much faster than humans.
Healthcare organizations are using AI to improve the efficiency of all kinds of processes, from back-office tasks to patient care. The following are some examples of how AI might be used to benefit staff and patients:
- Administrative workflow: Healthcare workers spend a lot of time doing paperwork and other administrative tasks. AI and automation can help perform many of those mundane tasks, freeing up employee time for other activities and giving them more face-to-face time with patients. For example, generative AI can help clinicians with note-taking and content summarization that can help keep medical records as thoroughly as possible. AI might also help with accurate coding and sharing of information between departments and billing.
- Virtual nursing assistants: One study found that 64% of patients are comfortable with the use of AI for around-the-clock access to answers that support nurses provide. AI virtual nurse assistants—which are AI-powered chatbots, apps, or other interfaces—can be used to help answer questions about medications, forward reports to doctors or surgeons and help patients schedule a visit with a physician. These sorts of routine tasks can help take work off the hands of clinical staff, who can then spend more time directly on patient care, where human judgment and interaction matter most.
- Dosage error reduction: AI can be used to help identify errors in how a patient self-administers medication. One example comes from a study in Nature Medicine , which found that up to 70% of patients don’t take insulin as prescribed. An AI-powered tool that sits in the patient’s background (much like a wifi router) might be used to flag errors in how the patient administers an insulin pen or inhaler.
- Less invasive surgeries: AI-enabled robots might be used to work around sensitive organs and tissues to help reduce blood loss, infection risk and post-surgery pain.
- Fraud prevention: Fraud in the healthcare industry is enormous, at $380 billion/year, and raises the cost of consumers’ medical premiums and out-of-pocket expenses. Implementing AI can help recognize unusual or suspicious patterns in insurance claims, such as billing for costly services or procedures that are not performed, unbundling (which is billing for the individual steps of a procedure as though they were separate procedures), and performing unnecessary tests to take advantage of insurance payments.
A recent study found that 83% of patients report poor communication as the worst part of their experience, demonstrating a strong need for clearer communication between patients and providers. AI technologies like natural language processing (NLP), predictive analytics, and speech recognition might help healthcare providers have more effective communication with patients. AI might, for instance, deliver more specific information about a patient’s treatment options, allowing the healthcare provider to have more meaningful conversations with the patient for shared decision-making.
According to Harvard’s School of Public Health , although it’s early days for this use, using AI to make diagnoses may reduce treatment costs by up to 50% and improve health outcomes by 40%.
One use case example is out of the University of Hawaii , where a research team found that deploying deep learning AI technology can improve breast cancer risk prediction. More research is needed, but the lead researcher pointed out that an AI algorithm can be trained on a much larger set of images than a radiologist—as many as a million or more radiology images. Also, that algorithm can be replicated at no cost except for hardware.
An MIT group developed an ML algorithm to determine when a human expert is needed. In some instances, such as identifying cardiomegaly in chest X-rays, they found that a hybrid human-AI model produced the best results.
Another published study found that AI recognized skin cancer better than experienced doctors. US, German and French researchers used deep learning on more than 100,000 images to identify skin cancer. Comparing the results of AI to those of 58 international dermatologists, they found AI did better.
As health and fitness monitors become more popular and more people use apps that track and analyze details about their health. They can share these real-time data sets with their doctors to monitor health issues and provide alerts in case of problems.
AI solutions—such as big data applications, machine learning algorithms and deep learning algorithms—might also be used to help humans analyze large data sets to help clinical and other decision-making. AI might also be used to help detect and track infectious diseases, such as COVID-19, tuberculosis, and malaria.
One benefit the use of AI brings to health systems is making gathering and sharing information easier. AI can help providers keep track of patient data more efficiently.
One example is diabetes. According to the Centers for Disease Control and Prevention , 10% of the US population has diabetes. Patients can now use wearable and other monitoring devices that provide feedback about their glucose levels to themselves and their medical team. AI can help providers gather that information, store, and analyze it, and provide data-driven insights from vast numbers of people. Using this information can help healthcare professionals determine how to better treat and manage diseases.
Organizations are also starting to use AI to help improve drug safety. The company SELTA SQUARE, for example, is innovating the pharmacovigilance (PV) process , a legally mandated discipline for detecting and reporting adverse effects from drugs, then assessing, understanding, and preventing those effects. PV demands significant effort and diligence from pharma producers because it’s performed from the clinical trials phase all the way through the drug’s lifetime availability. Selta Square uses a combination of AI and automation to make the PV process faster and more accurate, which helps make medicines safer for people worldwide.
Sometimes, AI might reduce the need to test potential drug compounds physically, which is an enormous cost-savings. High-fidelity molecular simulations can run on computers without incurring the high costs of traditional discovery methods.
AI also has the potential to help humans predict toxicity, bioactivity, and other characteristics of molecules or create previously unknown drug molecules from scratch.
As AI becomes more important in healthcare delivery and more AI medical applications are developed, ethical, and regulatory governance must be established. Issues that raise concern include the possibility of bias, lack of transparency, privacy concerns regarding data used for training AI models, and safety and liability issues.
“AI governance is necessary, especially for clinical applications of the technology,” said Laura Craft, VP Analyst at Gartner . “However, because new AI techniques are largely new territory for most [health delivery organizations], there is a lack of common rules, processes, and guidelines for eager entrepreneurs to follow as they design their pilots.”
The World Health Organization (WHO) spent 18 months deliberating with leading experts in ethics, digital technology, law, and human rights and various Ministries of Health members to produce a report that is called Ethics & Governance of Artificial Intelligence for Health . This report identifies ethical challenges to using AI in healthcare, identifies risks, and outlines six consensus principles to ensure AI works for the public’s benefit:
- Protecting autonomy
- Promoting human safety and well-being
- Ensuring transparency
- Fostering accountability
- Ensuring equity
- Promoting tools that are responsive and sustainable
The WHO report also provides recommendations that ensure governing AI for healthcare both maximizes the technology’s promise and holds healthcare workers accountable and responsive to the communities and people they work with.
AI provides opportunities to help reduce human error, assist medical professionals and staff, and provide patient services 24/7. As AI tools continue to develop, there is potential to use AI even more in reading medical images, X-rays and scans, diagnosing medical problems and creating treatment plans.
AI applications continue to help streamline various tasks, from answering phones to analyzing population health trends (and likely, applications yet to be considered). For instance, future AI tools may automate or augment more of the work of clinicians and staff members. That will free up humans to spend more time on more effective and compassionate face-to-face professional care.
When patients need help, they don’t want to (or can’t) wait on hold. Healthcare facilities’ resources are finite, so help isn’t always available instantaneously or 24/7—and even slight delays can create frustration and feelings of isolation or cause certain conditions to worsen.
IBM® watsonx Assistant™ AI healthcare chatbots can help providers do two things: keep their time focused where it needs to be and empower patients who call in to get quick answers to simple questions.
IBM watsonx Assistant is built on deep learning, machine learning and natural language processing (NLP) models to understand questions, search for the best answers and complete transactions by using conversational AI.
Get email updates about AI advancements, strategies, how-tos, expert perspective and more.
See IBM watsonx Assistant in action and request a demo
Get our newsletters and topic updates that deliver the latest thought leadership and insights on emerging trends.
- Open access
- Published: 13 May 2024
Tau- and α-synuclein-targeted gold nanoparticles: applications, opportunities, and future outlooks in the diagnosis and therapy of neurodegenerative diseases
- Andreas Tapia-Arellano 1 , 2 , 3 , 4 ,
- Pablo Cabrera 2 , 3 ,
- Elizabeth Cortés-Adasme 2 , 3 ,
- Ana Riveros 2 , 3 ,
- Natalia Hassan 1 , 3 , 4 &
- Marcelo J. Kogan 2 , 3
Journal of Nanobiotechnology volume 22 , Article number: 248 ( 2024 ) Cite this article
157 Accesses
1 Altmetric
Metrics details
The use of nanomaterials in medicine offers multiple opportunities to address neurodegenerative disorders such as Alzheimer's and Parkinson's disease. These diseases are a significant burden for society and the health system, affecting millions of people worldwide without sensitive and selective diagnostic methodologies or effective treatments to stop their progression. In this sense, the use of gold nanoparticles is a promising tool due to their unique properties at the nanometric level. They can be functionalized with specific molecules to selectively target pathological proteins such as Tau and α-synuclein for Alzheimer’s and Parkinson’s disease, respectively. Additionally, these proteins are used as diagnostic biomarkers, wherein gold nanoparticles play a key role in enhancing their signal, even at the low concentrations present in biological samples such as blood or cerebrospinal fluid, thus enabling an early and accurate diagnosis. On the other hand, gold nanoparticles act as drug delivery platforms, bringing therapeutic agents directly into the brain, improving treatment efficiency and precision, and reducing side effects in healthy tissues. However, despite the exciting potential of gold nanoparticles, it is crucial to address the challenges and issues associated with their use in the medical field before they can be widely applied in clinical settings. It is critical to ensure the safety and biocompatibility of these nanomaterials in the context of the central nervous system. Therefore, rigorous preclinical and clinical studies are needed to assess the efficacy and feasibility of these strategies in patients. Since there is scarce and sometimes contradictory literature about their use in this context, the main aim of this review is to discuss and analyze the current state-of-the-art of gold nanoparticles in relation to delivery, diagnosis, and therapy for Alzheimer’s and Parkinson’s disease, as well as recent research about their use in preclinical, clinical, and emerging research areas.
Graphical Abstract
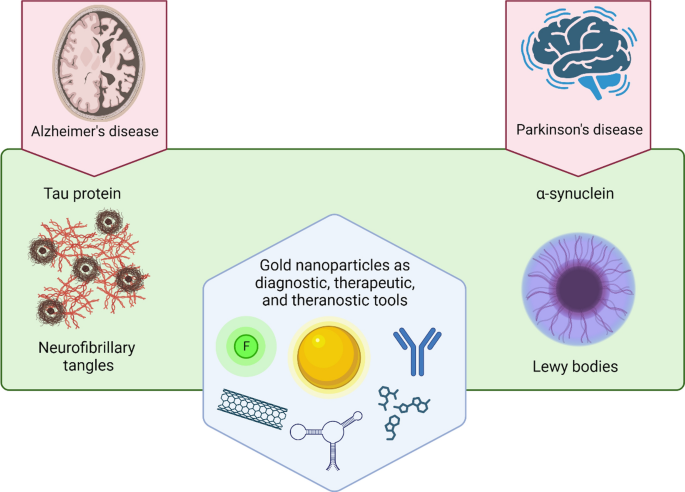
Introduction
Neurodegenerative diseases have become emergent and prevalent illnesses with age, being Alzheimer’s disease (AD) and Parkinson’s disease (PD), two of the most extended disorders [ 1 , 2 ]. Even though these neurodegenerative diseases have different cognitive disabilities, and clinical manifestations [ 3 ], both share a common molecular mechanism: misfolding proteins that underlie the progressive and chronic nature of each disease [ 4 , 5 ]. Alzheimer´s disease (AD) is the most common kind of dementia [ 6 , 7 , 8 ], accompanied by memory loss and dependence on personal daily activities. It is characterized by the presence of two misfolding proteins: amyloid-β peptide (Aβ) and Tau protein, which form highly insoluble aggregates. In this regard, the Aβ peptide aggregates extracellularly, causing synaptic damage, activation of proinflammatory pathways, mitochondrial dysfunction, oxidative stress damage, and neuronal death, promoting the neurodegenerative process and cognitive impairment [ 9 , 10 , 11 , 12 , 13 ].
For over 30 years, Aβ has been considered the mainstream that underlies and dominate the AD research, with extensive literature and clinical trials at the expense of Tau protein [ 14 , 15 ]. Recent studies have shown a preponderant role of Tau protein in the propagation of AD, playing a key interplay with Aβ in the neurodegenerative effect [ 16 , 17 , 18 , 19 ]. In fact, there are more evidence that show the key role of Tau as an active agent, refuting the Amyloid cascade as the main and unique actor of neurodegeneration[ 15 , 20 ], and turning the focus on AD research field. In this sense, Tau protein is a soluble protein predominantly found in neuronal axons and associated with microtubules, playing a fundamental role in microtubule stability under physiological conditions, being relevant from a structural and axonal traffic point of view in neurons [ 21 ]. The loss of Tau protein function has been associated not only with AD, but also with various tauopathies: Pick disease, progressive supranuclear palsy, and corticobasal degeneration [ 22 ]. The human tau gene is localized in chromosome 17 and expresses six isoforms of 351–441 amino acid residues with a molecular weight of 45–65 kDa [ 23 ], being the called 4-R (4R) and 3-R (3R) isoforms mainly located in the axons of an adult brain. Under pathological conditions, 4R and 3R Tau are found to be hyperphosphorylated at threonine and serine residues [ 23 ], producing structural instability and disruptions in neuronal transport processes [ 24 ]. This hyperphosphorylation could also promote an aggregation process forming the neurofibrillary tangles (NFTs) [ 25 ]. Additionally, different intermediate and highly toxic oligomeric species are produced throughout this aggregation process, triggering inflammatory processes and oxidative stress, which ultimately lead to neuronal death [ 24 , 26 ]. Thus, total Tau (T-tau), hyperphosphorylated Tau (P-Tau), and the oligomeric species have been used as key biomarkers for AD [ 27 ] even though they are found at low levels, making their detection difficult in biological fluids such as cerebrospinal fluid (CSF) (low 195 pg/mL or 4.7 pM) [ 28 ].
On the other hand, PD is characterized by motor impairments such as uncontrollable movements and difficulty with balance and coordination [ 5 ]. At the molecular level, there are intracellular lesions called Lewy bodies, which are composed of aggregates of α-synuclein (α-syn) protein [ 29 ]. This protein is part of the synuclein family which consists of three members: α-, β-, and γ-synuclein, that range from 127 to 140 residues [ 30 ]. However, only α-syn is present in the Lewy bodies and, therefore, possesses an attractive clinical perspective. There is no evidence that β- and γ-synuclein are present in the Lewy bodies because they have been demonstrated to fail to assemble into filaments, remaining in a random coil conformation [ 31 ]. α-syn is located in the neuronal presynaptic terminals. It is a small acidic protein of 14 kDa that is found as a monomer in the cytosol or as an α-helix structure in association with phospholipids under physiological conditions. The α-syn protein consists of three different parts: an amphiphilic N-terminal (residues 1–60), a hydrophobic region called NAC (non-amyloid-β component, residues 61–95), and an acidic C-terminal (residues 96–140) [ 32 ]. In this regard, the NAC region is highlighted because it plays an essential role in assembling monomeric α-syn into β-sheet-rich aggregates [ 33 ]. Under pathological conditions, monomers of α-syn exhibit a similar aggregation behavior as Tau, forming various species, including dimers, oligomers, protofibrils, and finally, fibrils, which eventually constitute the Lewy bodies [ 29 ]. Likewise, α-syn oligomers may drive the primary neurotoxicity, playing a critical role in neurodegeneration [ 34 , 35 ]. Even though α-syn oligomers have emerged as potential biomarkers, the current methods have encountered challenges in accurately discriminating their morphology and size to detect them at appropriate concentrations, thus hindering their analysis and study [ 36 , 37 ]. Finally, α-syn can also suffer genetic mutations and post-translational modifications such as phosphorylation, ubiquitination, and oxidation. These modifications have been observed to promote the misfolding process and correlate with disease progression [ 38 ] and, therefore, are being utilized as interesting biomarkers to follow the disease.
Even though the amino acid sequences of Tau and α-syn proteins are different, they both accumulate in pathological conditions, go through the same aggregation process, and generate toxic oligomers involved in the onset of AD and PD, respectively. In this sense, these oligomers possess a transient nature, low concentration in biological samples, and a wide range of different sizes and morphologies, making their analysis, quantification, and detection a remaining challenge in their use as promising biomarkers [ 37 , 39 ]. Therefore, it is mandatory to develop a strategy that detects these species to generate future and potential therapies for AD and PD. Additionally, other species of Tau and α-syn may be used as targets to generate specific biomarkers to discriminate pathological concentrations in biological samples or develop therapies to modify the underlying aggregation processes [ 40 , 41 , 42 ]. Nevertheless, each of these approaches is hindered by factors that prevent the achievement of an effective treatment or diagnosis [ 43 , 44 ], including side effects, the blood–brain barrier (BBB), and the challenges associated with the specificity and sensitivity of the new strategies [ 45 , 46 ]. Overcoming these difficulties is crucial for developing new therapies or early diagnosis.
Nowadays, nanomaterials have acquired a high level of relevance in the nanobiotechnology field, and specifically in biomedicine, due to their several advantages, such as nanometer scale, relative low toxicity, and the capability of crossing biological membranes (including the BBB) [ 47 ]. Among these, gold nanoparticles (GNP) are the most studied and exhibit versatile features such as their high compatibility and stability in relevant biological mediums, tunable shape, and high surface area to be functionalized with different molecules over other kinds of nanomaterials [ 48 , 49 , 50 ]. In this regard, GNP are easily functionalized, becoming them drug carriers to target specific organs [ 51 , 52 ] or specific molecules [ 53 ], enhancing their delivery capabilities compared to other materials (Fig. 1 ) [ 54 ]. Indeed, GNP possess unique properties to increase or amplify signals over other nanomaterials[ 55 , 56 ], such as the surface plasmon resonance (SPR), surface enhanced Raman scattering (SERS), photothermal therapy, and even as a contrast enhancer in X-ray imaging; and thus, facilitating the recognition and sensing of biological molecules in complex samples compared to conventional methods [ 52 , 53 , 57 , 58 , 59 ]. Altogether, GNP have been demonstrated to have a multifunctional use as drug delivery, targeting, amplifiers, and even biomarkers being utilized in therapy, diagnoses, or the combination of both, the so-called theragnostic [ 49 , 60 ]. Thus, GNP have become an interesting tool or complement to enhance conventional methods or techniques in order to increase their sensitivity, specificity, effectiveness, and safety. However, their use in preclinical studies and clinical trials for AD and PD is limited, and in the case of AD, they are mainly restricted to the Aβ peptide. Furthermore, the new therapeutic strategies for AD have shifted to include analyses that consider the Tau protein, and as with the α-syn protein, their relationship with GNP is scarce and has been poorly studied. Thus, both proteins have become an outstanding niche to develop research for new strategies and outlooks.
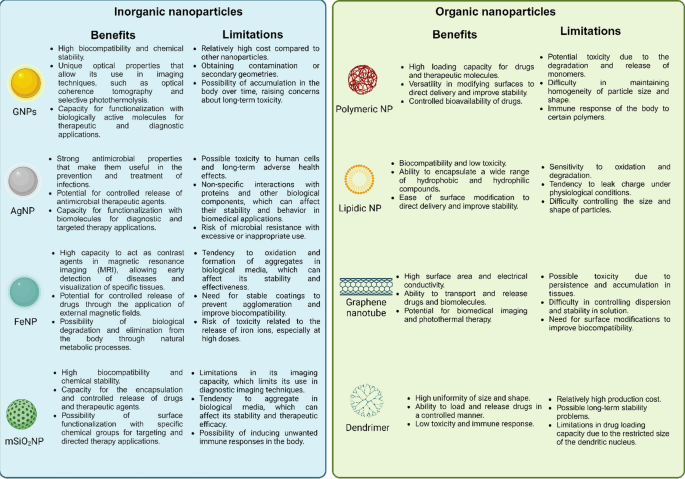
Comparison between the main inorganic and organic nanoparticles composed by different materials. Their benefits and limitations are highlighted to guide their use and potential applications. Created with BioRender.com
This review compiles and focuses on Tau and α-syn proteins as GNP targets, with a special attention to new functionalized nanosystems, how they can reach the central nervous system (CNS), and the detection at low concentrations of different Tau and α-syn species as biomarkers, including the current research in the oligomer field, to generate an early diagnosis. In addition, this review discusses not only in vitro and in vivo assays, state-of-art clinical studies, and FDA approvals, but also highlights the risks and concerns that underlie the uses and applications of GNP, as well as the latest advances and opportunities to generate and drive new methodologies to research the potential use of GNP with Tau and α-syn proteins for therapy, diagnosis, and theragnosis.
Obtention of GNP
GNP have been widely used for electronic, optical, and biological applications including targeted drug delivery. GNP exhibit different geometries (Fig. 2 ), which can influence the interactions at the bio-nano interface, the functionalization degree, the molecular organization of these molecules as well as their chemical state, and even their biological uptake degree [ 61 , 62 ]. Thus, the size and shape are fundamental for the chemical and surface properties, being a key part of the experimental design of a GNP [ 61 , 63 ]. For these reasons, various methods have been developed to modulate these physical properties to obtain different geometries [ 64 ]. In this sense, Gold nanospheres (GNS) are one of the most studied shapes, possessing different synthesis methods such as Turkevich method [ 65 ], which involves citrate as a reducing and stabilizing agent to modulate their size [ 66 , 67 , 68 ]. Another strategy is the Brust-Schiffrin method, which allows to obtain small nanoparticles in the 1–3 nm range [ 69 ], but this synthesis generates a considerable amount of organic solvent used as a stabilizing agent.
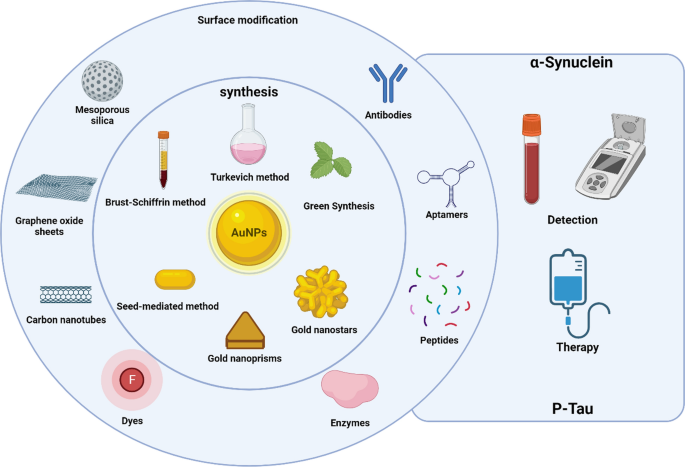
GNP can be obtained by several methods that are shown in the central circle (Turkevich, Brust-Schiffrin, and green synthesis specifically for GNS), obtaining different shapes and sizes that depend on the methodology used. These GNP can also be functionalized with various types of molecules to target α-syn and Tau proteins, making them interesting tools to generate novel strategies to apply in the detection and therapy of PD and AD, respectively. Created with BioRender.com
Currently, the synthesis methods aim to develop a GNS synthesis more environmentally friendly and safe. Green synthesis involves the use of natural sources of chemicals to synthesize GNP [ 70 , 71 ], avoiding the use of toxic chemicals and reducing waste products. These methods are mainly based on the presence of alkaloids and polyphenols, among other elements, from natural extracts, microorganisms, and molecules that allow the reduction of Au 3+ to produce GNP. For example, polyphenols in the aqueous extract of Abutilon Indicum leaves [ 72 ] or alginate [ 73 ], a marine algae polysaccharide, have been used to synthesize GNS. However, these methods exhibit some limitations in polydispersity control because of the variety of molecules present in the extract or microorganism [ 74 , 75 ]. To overcome these issues, the use of purified phytochemicals allows to obtain greater reproducibility, compared to the use of extracts, because there is an understating of which molecules participate as stabilizers and reducing agents [ 76 ]. Curcumin has been used to obtain GNS with promising effects in a human colorectal adenocarcinoma (HT29) cell line model [ 77 ], as well as their antioxidative and antimicrobial properties [ 78 , 79 , 80 ]. Gallic acid is another successful case used to synthesize GNP. This phytochemical is present in tea leaves, fruits, and nuts and has been used to synthesize GNS with a potential application as an antitumoral or anti-aging agent [ 81 , 82 , 83 ].
Anisotropic nanoparticles
Anisotropic nanoparticles are another area of research that has been developed because the shape drives their properties, and it also can be modulate with potential biomedical applications (Fig. 2 ). One of the most studied nanoparticles is gold nanorods (GNR) which can present their plasmon within the first and second biological windows, making them interesting for their applications in biomedicine [ 84 , 85 , 86 ]. The most common synthesis is the seed-mediated method using CTAB as a stabilizer agent [ 86 ], allowing to modulate the plasmon maximum, absorption, and scattering efficiency of the nanosystem [ 87 , 88 ]. Gold nanoprisms (GNPr) also exhibit a plasmon that can be modulated in the biological window [ 89 ], but their synthesis is more friendly using sodium thiosulfate as a reducing and stabilizing agent [ 90 ]. Also, they scatter the light efficiently due to the greater number of hot spots, making them potential candidates as fluorescent probes in imaging [ 91 ]. Gold nanostars (GNSt) are another type of GNP that exhibit more hot spots than GNPr depending on the number of points and length of the arms presented, making them very interesting for their applications in plasmon-enhanced fluorescence (PEF) and surface-enhanced Raman spectroscopy (SERS) [ 91 , 92 ]. Their synthesis is based on the use of HAuCl 4 at basic pH and hydroxylamine as a stabilizer and reducing agent [ 93 ].
Hollow gold nanoparticles (HGNP) are another attractive type of nanoparticle because of their thickness and cavity size can be modified, allowing the maximum absorption to shift in different regions of the spectrum with potential application in photothermal therapy [ 94 , 95 , 96 ]. Also, their cavity can be used to encapsulate drugs, which makes it useful for drug delivery [ 97 ]. Their obtention is by templates over which Au is deposited from a HAuCl 4 solution. Then, these templates are eliminated, and the size and shell thickness depend on the template used as well as the concentration of the added HAuCl 4 solution [ 98 ]. Finally, even though anisotropic GNP exhibits great potential in many different areas, it is noteworthy that their obtention by green methods is still recent. Some issues related to their shape homogeneity and yield need to be improved and encouraged to develop an alternative to traditional chemical methods. For a complete revision see Table 1 , and Ref. [ 99 ] and [ 100 ].
Because the control of morphology, size, and distribution is not trivial [ 48 ], these physical features may modify the biological effect at the nano-bio interface, resulting in different behavior of the nanosystems in in vitro approaches compared to in vivo analysis. Nowadays, efforts are focused on developing smart nanosystems with optimized properties to increase the responsiveness of biological systems [ 61 ]. However, some concerns emerge for their use in biological systems related to their stability and how GNP may interact or behave with the medium that surrounds them in physiological conditions. Also, different synthesis uses organic compounds that are incompatible with their application in biological assays, affecting their potential use in diagnosis and therapy [ 51 , 101 , 102 , 103 ]. To overcome this issue, the surface of GNP can be functionalized easily with different molecules, adding new properties, giving more stability, increasing their biocompatibility and half-life (e.g., plasma), and enhancing their selective delivery to organs.
In this sense, polyethylene glycol (PEG) is the most common way of eliminating toxic stabilizer agents or residues from the surface of GNP by a coating process [ 104 ]. Also, PEG increases the half-life of GNP in the bloodstream due to their hydrophilic nature, conferring water solubility, physiological stability, and biocompatibility to the GNP [ 105 , 106 , 107 ]. Another widely used option is a silica shell, which has been reported to enhance GNP stability and biocompatibility for biomedical applications [ 51 , 108 , 109 ]. Silica coating is chemically inert and allows the encapsulation of different kinds of molecules, such as drugs, dyes, and antibodies, among others, as well as an effective drug release[ 108 ]. Another interesting approach to masking the GNP is the use of endogenous elements present in plasma is. This strategy consists of capping the GNP with serum albumin (SA), which is the most abundant protein in plasma. As reported, these nanosystems exhibit longer circulation times, biocompatibility, and low toxicity, making their use widely accepted in the pharmaceutical industry [ 110 , 111 , 112 ]. Finally, it is noteworthy that green synthesis has been reported to enable the synthesis of GNP as well as their coating, increasing their biocompatibility. Curcumin and Apigenin are highlighted due to their potential use in biomedical applications. Whereas GNP functionalized with curcumin increase their half-life in the bloodstream with potential applications in neurodegenerative diseases[ 113 , 114 ], GNP coated with Apigenin exhibit anti-inflammatory and anti-cancer properties in a photothermal treatment [ 115 ].
GNP delivery to the CNS
Currently, the engineering of GNP to deliver them comprises passive or active strategies for site-specific recognition [ 63 ]. The first strategy is based on nanoparticle accumulation into the brain depending on systemic circulation [ 47 , 116 , 117 ]. However, this mechanism is limited for the renal excretion, reticuloendothelial system, and immune system that eliminate the nanoparticles from bloodstream. Conversely, active targeting comprises the use of biological ligands functionalized onto GNP to recognize specific molecules on the cell surface (Fig. 3 ) [ 118 , 119 ]. In fact, one of the current challenges is to assemble multiple ligands for delivery and targeting in one GNP. The best-case scenario is to design GNP in which the ligand merges both applications into one. Thus, the use of only one ligand is expected to yield a high selectivity for a particular body area, achieving better biocompatibility and ensuring the potential of the nanosystem for diagnosis and therapy.
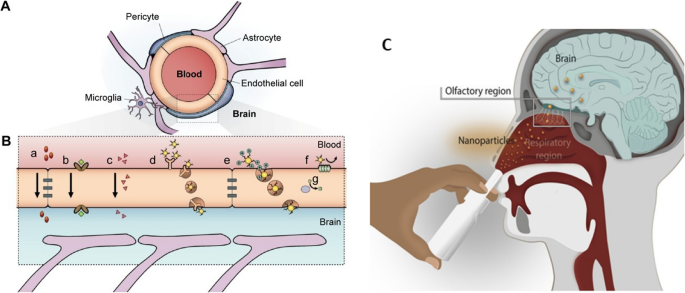
Copyright 2015, with permission from Dove Medical Press Limited. C Anatomical features of the intranasal route involved in nose-to-brain drug delivery. Adapted with permission from Ref. [ 133 ]. Copyright 2022, with permission from Elsevier
Principal pathways to overcome the BBB. A Scheme of the neurovascular unit of BBB constituted of pericytes, astrocytes, and endothelial cells. B The main mechanism of crossing through BBB: a) tight junctions restricting the pass of water-soluble compounds, b) carrier-mediated transport, [c] lipid-soluble agents, d) receptor-mediated endocytosis and transcytosis, e) adsorptive-mediated endocytosis and transcytosis, f) the efflux pump expulses the drugs from the endothelial cells to the blood, g) Cytochrome P450 enzymes. Adapted with permission from Ref. [ 126 ].
Regarding neurodegenerative diseases, the engineering of nanoparticles must consider another factor to achieve an effective therapy, which is that GNP must be able to arrive in the CNS by crossing the BBB [ 120 , 121 ]. This biological barrier controls brain homeostasis through a tight and sophisticated mechanism where layers of endothelial cells limit the entrance of different foreign molecules into the CNS (Fig. 3 a). Thus, the BBB has become a formidable barrier that must be surmounted in the generation of treatment options for neurodegenerative diseases [ 122 , 123 , 124 ]. Several approaches have been designed, but most of them imply a disruption of BBB cells, making them invasive methods that result in deleterious consequences for the cells [ 123 , 125 ]. For this reason, the use of GNP has emerged as a novel alternative because they exhibit different mechanism to cross through the BBB. For example, their internalization can be mediated by endocytosis involving both pinocytosis and phagocytosis mechanisms, but this mechanism results in a widespread organ distribution including kidneys and liver [ 126 , 127 ]. To overcome this issue GNPs can be capped or functionalize with targeting molecules to delivery into the brain [ 128 , 129 , 130 ], being adsorptive-mediated transcytosis (AMT) and receptor-mediated transcytosis (RMT), being RMT the most specific (Fig. 3 b). Peptides Angiopep-2 and CLPFFD-THR functionalized onto GNP are successful examples of RMT [ 131 , 132 ]. The present section describes and summarizes the advances in the field of delivery and targeting, considering the use of GNP with Tau and α-syn proteins for AD and PD, respectively.
A new opportunity: the nose-to-brain delivery
Even though RMT is an interesting strategy, some drawbacks may reduce its effectiveness. Low bioavailability, inadequate permeation, delay in onset of action, and limited presence or saturation of receptors in the BBB are the most common issues. To overcome these limitations, intranasal administration (IN) has emerged as a non-invasive strategy to bypass the BBB, reaching and delivering drugs and nanoparticles directly into the brain (Fig. 3 c) [ 133 ]. Via IN therapy the nanosystems enter by two main pathways: the olfactory and the trigeminal nerves [ 134 , 135 ]. Due to the unique anatomical organization of the olfactory nerves, compounds can circumvent the BBB through two main mechanisms: 1) the neuronal endocytosis, in which compounds are internalized by olfactory neurons of the olfactory epithelium and transported through the axons to reach the olfactory bulb, and then other brain areas; 2) paracellular diffusion, in which compounds can diffuse through intercellular gaps in the olfactory epithelium and channels created between the ensheathing cells surrounding olfactory axons, being externally transported along the neuronal axon and reaching the CSF and olfactory bulb [ 134 , 135 , 136 ]. Regarding GNP, different studies have shown their effective delivery enhances treatment efficacy [ 137 , 138 , 139 , 140 ]. GNP can reach the olfactory bulbs in minutes [ 141 ]. Gallardo-Toledo et al. [ 140 ] analyzed GNS and GNPr functionalized with PEG and the peptide D1 (qshyrhispaqv), as a potential targeting for Aβ and their delivery into the brain. Also, it compared the IN with the intravenous route, demonstrating the major effectiveness of the IN route and reinforcing the idea that IN administration is a promising pathway for the delivery of GNPs into CNS. However, IN delivery has presented some concerns about mucociliary clearance that can easily eliminate the drugs administrated; therefore, some special formulations may be required to decrease this effect. To overcome this issue, GNP surface can be coated to specifically target the olfactory region, exhibiting mucoadhesive properties that allow a major retention and avoiding the mucociliary clearance. In this regard, chitosan emerges as an attractive mucoadhesive polymer due to its cationic nature, biocompatibility, and suitability for high loading of hydrophilic drugs [ 142 ]. For example, Bhumkar et al. loaded insulin in GNP coated with chitosan. After transmucosal administration, they were able to reduce glucose levels on the blood of rats, noting a higher increase in the insulin level after nasal administration compared to oral administration [ 143 ].
GNP and diagnosis
Tau protein is one of the main etiological agents of AD and other tauopathies [ 144 ]. Likewise, one of the key biofluid-based biomarkers for PD is the misfolded and aggregated α-syn protein [ 1 , 145 , 146 ], which is characteristic of Lewy bodies and hallmark of this disease [ 31 , 147 , 148 , 149 ]. In this sense, both α-synuclein and Tau protein can be detected in CSF [ 144 , 146 ], but this process involves the extraction of the sample by lumbar puncture, which is an invasive and painful procedure for the patient. Detection methods are currently limited to ELISA immunoassay and PET imaging (Fig. 4 ) [ 144 ]. The latter method is expensive, requiring access to a cyclotron, and uses radiotracers with a short half-life. In this context, research has been carried out to find new detection and diagnosis forms that are sensitive, economically accessible, and non-invasive [ 150 , 151 , 152 , 153 ]. Currently, researchers are investigating sensitive methodologies that allow the detection of Tau and α-syn in different biofluids. Numerous strategies based on GNP technology have emerged in the search for methodologies that allow the quantitative detection of both proteins (α-syn or Tau) with high sensitivity (for a complete review, see Table 2 ). Most of these biosensors are based on electrochemical methods, SERS, or localized surface plasmon resonance (LSPR) (Fig. 4 ) [ 154 , 155 , 156 ]. In general, these methods are based on immobilization on the surface of the GNP with a selective molecule for the target molecule (antibody, aptamer, etc.) and/or a reporter molecule. This strategy allows an improvement in both the limit of detection (LOD) and sensitivity (Fig. 4 ).
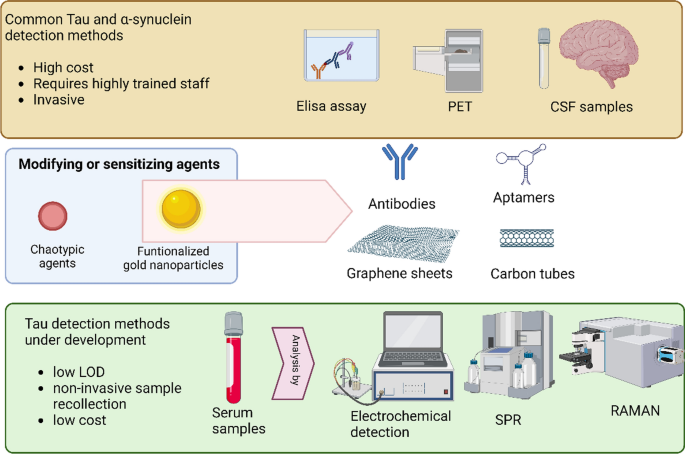
Conventional methods to detect Tau are often expensive, invasive, and require highly trained personnel. However, the use of nanoparticles is enabling the development of new detection methodologies that significantly improve the sensitivity and selectivity of the process. LOD: limit of detection. Created with BioRender.com
GNP designed for α-synuclein diagnosis
In this regard, Zhang et al . [ 155 ] showed that modifying a single-walled carbon nanotube with a gold-nanourchin-conjugated α-syn antibody significantly enhanced the LOD of the biosensor. Specifically, the biosensor displayed a 1000-fold increase in sensitivity, detecting concentrations as low as 1 fM compared to the surface coated solely with antibody, which detected concentrations as low as 1 pM (Fig. 5 ). Another notable immunosensor for α-syn is based on dual signal amplification (enzyme-assisted signal amplification and electrochemical measurement). The sensor is constructed with PAMAM-Au nanocomposites and an immunosensor surface using a horseradish peroxidase-secondary antibody (HRP-Ab2) linked to GNP. This sensor has high analytical performance, sensitivity (LOD: 14.6 pg mL −1 ), and stability [ 156 ]. The approach of using nanocomposites is very interesting since it allows for anchoring larger amounts of target protein with high stability and bioactivity. Furthermore, these detection analyses must exhibit both high sensitivity and high specificity for clinical examination using complex samples such as human plasma and CSF. In this context, there are some reports, such as that of Aminabad et al . [ 157 ], who developed a system based on GNP-modified graphene for bioconjugation with a biotinylated antibody (bioreceptor). Like the previous one, this nanosystem exhibits a synergistic effect between graphene and GNP, resulting in enhanced electrochemical activity. Consequently, it enables the development of a high-sensitivity electrochemical immunosensor (LOD: 4 ng/mL) for human plasma. In addition, there is a very interesting study in which the CSF is measured using a neuro-biosensor system that consists of an electrode with GNP-PGA-modified ITO, with a LOD of 0.135 pg/mL [ 158 ]. Essentially, a concentration of α-syn at the picograms per milliliter (pg/mL) level has been reported in the CSF of healthy individuals; however, it increases to nanograms per milliliter (ng/mL) in PD patients [ 159 ]. Thus, unlike the previous biosensor that detected α-syn in human plasma, this neurobiosensor system can identify and discriminate α-syn in the CSF of both healthy individuals and PD patients with high sensitivity, making it a promising tool for the diagnosis of PD.
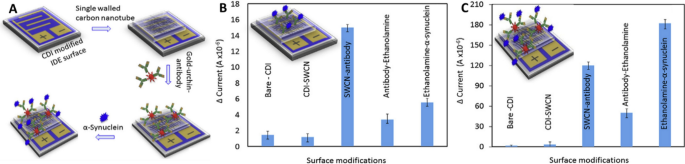
Adapted from Ref. [ 155 ]. Copyright 2020, with permission from Elsevier
The presence of gold nanourchin increases the sensitivity of detection for α-syn. A Schematic representation for detecting α-syn on a single-walled carbon nanotube (SWCN)-modified IDE surface, assisted by goldnanourchin-immobilized antibodies. Detection of 1 nM α-syn using the platform in the absence ( B ) or presence of gold nanourchin ( C ).
Early diagnosis is a key factor to evaluate in neurodegenerative diseases. Since the oligomer species are recognized as the most toxic species that appear in the first stages of the aggregation process, early detection of α-syn oligomer is necessary for PD or other neurodegenerative diseases [ 36 , 160 ]. In recent research studies, aptamers have emerged in the search to identify the oligomers specifically. Aptamers are "artificial antibodies" selected from a randomized group of nucleic acids that are very specific and stable for the target molecule. For example, Wu et al . [ 161 ] successfully designed electrochemiluminescent systems based on GNP-cooper-metal–organic frameworks (NP-Cu-MOFs) with an aptamer as the recognition element for α-syn oligomer detection. In the search for an effective diagnosis of PD, the literature has described that a selective α-syn nitration is a modification associated with oxidative and nitrative damage of neurodegenerative synucleinopathies, and it has been found in biological fluids in patients with PD [ 162 , 163 , 164 ]. This has generated a new challenge for the design of diagnostic systems to detect the different conformations of α-syn. In this context, Zhang et al. [ 165 ] designed an electrochemical immunosensor as a sensitive sandwich assay that utilized supramolecule-mediated GNP composites (GNCs) with anti-α-syn-nitration magnetic nanoparticles (MNPs) as signal amplification tags (LOD: 310 pg mL −1 ). The significance of this work lies in the fact that it investigated the detection of α-syn nitration in diluted serum samples obtained from healthy donors and PD patients, indicating a significant difference between the two study groups and the potential use of this system for clinical applications.
GNP designed for Tau protein diagnosis
On the other hand, Tau protein is a key player in neurodegeneration that poses a diagnostic challenge. Some literature highlights such as Neely et al . [ 166 ], which synthesized GNP functionalized with an anti-Tau antibody (Tau-mab) that can detect up to 1 pg/ml of Tau protein, which is two orders of magnitude lower than the values found in CSF. A similar result was obtained using a monoclonal anti-Tau antibody-coated GNP based on a two-photon scattering assay, in which the sensitivity of the method increased about 16 times [ 166 ]. Kim et al. [ 167 ] investigated the use of guanidine chloride to enhance Tau detection in blood samples. In this case, the researchers demonstrated that the chaotropic agent guanidine chloride improved sensitivity and selectivity compared to traditional methods, preventing the interfering effects of other components in the biological fluid. This same effect was tested by LSPR using PEG-functionalized GNR and anti-Tau antibodies. Therefore, guanidine chloride allows differentiating samples from healthy individuals and patients with AD.
The development of sensitive, non-invasive diagnostic methods for neurodegenerative diseases, such as AD and PD, represents a crucial frontier in medical research. With advancements in systems based on GNP, it is possible to develop innovative approaches to detect biomarkers like Tau protein and α-syn with high sensitivity and specificity. Table 2 describes the main technologies currently developed as sensors to detect α-syn and Tau protein with potential diagnostic applications.
GNP designed for targeted therapies
The therapeutic potential of gold nanoparticles (GNP) in neurodegenerative diseases like Alzheimer's and Parkinson's is being explored. Researchers are leveraging the unique properties of GNPs to develop innovative approaches for more effective diagnosis and treatment, opening up new avenues for theragnosis.
Targeted therapies for α-synuclein
The accumulation of α-syn protein plays a central role in the pathogenesis of PD. Liu et al . [ 189 ] developed a nanosystem consisting of GNP-pDNA-Lipo-NGF-DHA to deliver plasmid DNA (pDNA) to inhibit α-syn expression. Liposomes (Lipo) are the carriers of the GNP-pDNA, and Nerve Growth Factor (NGF) and docosahexaenoic acid (DHA) are the targeting molecules in the nanosystem. NGF has an important role in the maintenance and growth of neurons, which have NGF receptors in their membranes, promoting the entrance of GNP into the neurons [ 190 ]. DHA is a polyunsaturated fatty acid that favors memory and cognitive functions, and it also promotes the movement of GNP across the BBB due to the presence of DHA receptors in the membrane of the BBB. This nanosystem shows effective GNP delivery into the CNS, reducing the overexpression of α-syn and improving motor dysfunction and exploration abilities in a PD mouse model. Hu et al. [ 191 ] developed a similar CTS@GNP-pDNA-NGF nanosystem. In this case, chitosan (CTS) works as a scaffold in which its cationic groups bind to pDNA, inhibiting of α-syn transcription and protecting from enzymatic digestion. This approach demonstrated effective delivery of GNPs to the central nervous system, significantly reducing α-syn overexpression and improving motor behavior in animal models of the disease.
Additionally, Gao et al . [ 192 ] synthesized gold nanoclusters (GNCls) functionalized with N-isobutyryl-L-cysteine (L-NIBC) for the prevention of α-syn fibrillation in vitro. Furthermore, in vivo experiments using mouse PD models showed amelioration of behavior disorders with this nanosystem. The anti-PD effect of the L-NIBC-GNCLs system was evaluated using MPP + lesioned cells, and the in vivo models consisted of MPTP-induced mice, being MPTP a molecule commonly used to induce PD in animal models. The cell studies showed no obvious toxicity and a significant decrease in apoptosis compared to the MPP + control, demonstrating the neuroprotective effect of the GNCls. For the in vivo studies, the MPTP-induced mouse PD models were treated with different doses of the nanosystem administered via intraperitoneal injection. The results showed an improvement in locomotor activity in the mice in the presence of GNCls, significantly increasing the speed and distance traveled, thus, proving the effectiveness of the nanosystem and its delivery (Fig. 6 ).
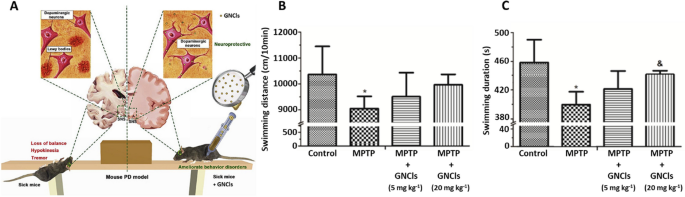
Copyright 2019, with permission from John Elsevier
Treatment of GNP in in vivo transgenic mouse models. A Experimental design of treatment with GNCls in a PD model. Effect of GNCls on motor coordination of mice in a mouse PD model using a swimming test. B Evaluation of swimming distance. C Evaluation of swimming duration. Adapted with permission from Ref. [ 192 ].
Targeted therapies for Tau Protein
Numerous therapeutic studies have focused on Tau protein, which is pivotal in neurodegeneration. Due to the limited studies conducted with GNP, we have included in the review studies involving other nanoparticles and approaches, which may serve as a guide for developing theragnostic using GNP.
In this sense, Sonawane et al . developed two interesting types of protein-capped (PC) metal nanoparticles (PC-F 3 O 4 and PC-CdS) for the inhibition of Tau aggregates, both obtained via biological synthesis with two fungal species ( Funsarium oxysporum and Verticillium sp), showing that PC-CdS inhibited Tau aggregates by 63% and PC-F 3 O 4 by 49% [ 193 ]. Gao et al . loaded Curcumin onto red blood cell membrane-coated PLGA nanoparticles bearing T807 molecules as an imaging agent for positron emission tomography. This nanosystem was able to cross the BBB, and curcumin reduced P-Tau levels and neuronal death both in vitro and in vivo. Vimal et al. [ 194 ] investigated the therapeutic potential of GNP functionalized with PEG in transgenic Tau P301L mutant mice and macaque monkey serum (Fig. 7 ). These nanostructures acted as pseudo-nanochaperones interfering with Tau aggregation and significantly improving neuronal health in transgenic models. Regarding the ex vivo experiment using monkey serum the authors observed that this nanosystem interfering with Tau aggregation. Additionally, Bhattacharyya et al. posited that GNP could act as nanochaperones [ 195 ]. GNP capped with citrate of approximately 5 nm were suggested to allow remodeling of the P301L mutant Tau protein in vitro, modifying its structure and recovering the transport function of the microtubules. Thus, these studies highlight the role of GNP in modulating Tau pathology and maintaining brain function.
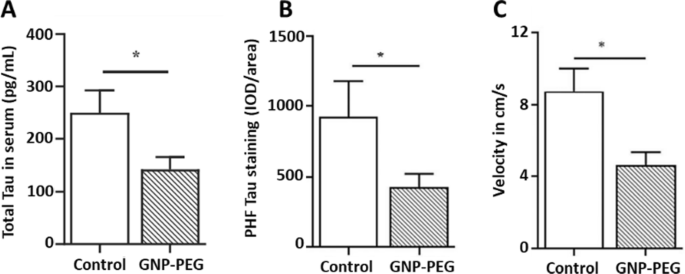
Copyright 2020, with permission from John Wiley and Sons
A Quantification of total Tau concentration in serum samples of control and GNP-PEG-treated tau P301L mice using an ELISA assay after 30 days of treatment. B Quantification of integrated optical densities (IOD) values in control (n = 6) and treated groups (n = 6) for paired helical filament-positive cells using Image-Pro Plus analysis. C Novel object recognition test for the examination of learning and memory, in which the mice exhibited altered velocity. Adapted with permission from Ref. [ 194 ].
Opportunities in therapy
Even though the studies related to the targeting and delivery of Tau protein are scarce, some strategies may be applied or replicated from the research using the amyloid cascade theory, and GNP functionalized with different molecules. Liu et al . [ 6 ] functionalized GNR with the APH (ST0779) enzyme and the scFv (12B4) antibody, which binds to Aβ oligomers and fibrils and can cross the BBB. Javed et al . [ 196 ] also used GNP coated with β-casein, which binds to Aβ and can cross the BBB in zebrafish larvae and adult models via intracardial and cerebrovascular administration. In this sense, Martins et al . [ 197 ] functionalized GNP with Apolipoprotein E3 (ApoE3), which promotes both selective interaction with Aβ aggregates and can also cross the BBB. Altogether, if these approaches are used in a Tau protein context, they allow reaching the CNS.
Another potential strategy to replicate is the use of GNP functionalized with specific D-enantiomeric peptides, which are produced by mirror-image phage display to detect specific targets [ 198 , 199 ]. These peptides exhibit several advantages for their use in biological systems, such as that they are not recognized by proteases, making them more resistant to degradation and increasing their half-life [ 200 ]. Interestingly, these D-peptides can cross the BBB and decrease both the amyloid load and neuroinflammation in transgenic models of AD [ 201 , 202 , 203 , 204 , 205 , 206 , 207 , 208 ]. Various studies have enhanced the inhibitory effect of these peptides on Aβ using GNP across multiple models (in vitro, in vivo, and even ex vivo) [ 84 , 85 , 209 ]. This progress highlights efforts to develop GNP-based systems for delivering targeted molecules, including these peptides, through the BBB for potential noninvasive CNS treatments. In this way, the use of enantiomers emerges as an interesting strategy to use with Tau protein. Recent reports have indicated that D-peptides can target Tau protein with a high inhibitory effect on the Tau aggregation process, and show valuable specificity, low toxicity, and high penetrance into neuron cell cultures [ 210 , 211 , 212 , 213 , 214 ], as was observed, for example, with the d-ttslqmrlyypp sequence [ 213 ]. Similarly, some studies have explored the use of D-peptides to disrupt the α-syn aggregation process. Shaltiel-Karyo et al. [ 215 ] found that β-syn exerts an inhibitory effect on α-syn. They designed a retro-inverso analogue of β-syn with high stability in mouse serum, which inhibited α-syn aggregation in in vitro and in vivo assays. Chemerovski-Glikman et al. [ 216 ] developed a self-assembled cyclic D,L-α-peptide which inhibited α-syn aggregation and even reduced its toxicity and intracellular accumulation. Horsley et al. [ 217 ] compared the effects of a D-peptide and an L-peptide to prevent the α-syn aggregation process, demonstrating that a D-peptide (d-gvlyvgs) was more effective in leading the α-syn monomers into a less aggregation-prone state, alleviating the cytotoxic effects of α-syn aggregates in cell models. Furthermore, these peptides have also exhibited the ability to cross the BBB and high stability in biological fluids, making them a promising strategy to develop new therapeutic agents. Thus, D-peptides open an interesting niche to functionalize them onto GNP as an effective future therapy. Thus, the studies with Aβ peptides can serve as models to follow for generating new treatments, considering the already described benefits as a guide.
Interestingly, since Tau protein is known for its close relationship with the microtubule network, it has also been the subject of interest as a target for treating diseases such as cancer. Ghalandari et al. [ 218 ] proposed the use of GNP or Fe 3 O 4 nanoparticles coated with gold and functionalized with folic acid for photothermal treatment. The results of this study indicated that GNP interact with Tau and tubulin, preventing the formation of long polymers, and triggering apoptosis of malignant cells. Goto et al. [ 219 ] functionalized GNP with anti-Tau and anti-histone H1 antibodies to obtain high-resolution images that allowed the study of intracellular elements for protein localization [ 174 ]. Finally, Chia-Hsiung et al. [ 220 ] presented evidence that Plasmon-Activated Water (PAW) reduces β-amyloid and P-Tau burden in murine models of AD (APP/PS1), improving the behavioral parameters. In this article, the authors used resonantly illuminated GNP, reducing the hydrogen bonds present in the native structure of water and allowing PAW to be obtained. PAW could trap free radicals, which is one of the main mechanisms through which the damage produced in AD would be reduced in the model used. These results emerge as interesting approaches for a field that has not been sufficiently explored, along with their applications in neurodegenerative diseases, allowing the development of potential therapies.
Current clinical trials, GNP, and FDA
Nanomedicine research has expanded greatly in recent years, resulting in potential medical and pharmaceutical tools and systems that are either FDA-approved or in clinical trials. There are currently 537 clinical studies registered on Home-ClinicalTrials.gov referring to nanoparticles, including polymeric, metallic, and lipid nanoparticles, among others. Of these clinical studies, only 7 are clinical trials aimed to neurodegenerative diseases: 4 trials of GNP, 2 of lipid nanoparticles, and 1 of polymeric nanoparticles (Table 3 ). This data shows the huge difference between GNP and other types of nanoparticles, and the inconsistency between the preclinical and clinical studies. There is a large literature related to preclinical assays of GNP, but their clinical translation is scarce [ 221 , 222 ]. In this sense, some concerns support this evidence. One of the reasons is that most research in this field is related to material science, where the main objective is to generate new materials with new properties instead of exploiting the already known materials. For example, the synthesis of GNP is commonly simple, easily scaled up, and tunable, and GNP can be functionalized with different kinds of molecules, drugs, etc., as was above mentioned. However, despite these promising features, clinical studies with these nanostructures are not common. One successful example is the AuroLase Therapy, which was the first FDA-approved inorganic material. AuroLase Therapy is based on gold nanoshells which accumulate preferentially in cancerous tissue and using a near-infrared laser the affected area is irradiated, destroying the cancer cells by photothermal therapy [ 223 ]. Contrarily, among the clinical studies conducted with GNP, only one focused on PD while there are no records of GNP-based treatments for AD (Table 3 ). Although this study is a great advance for nanomedicine in its clinical applications, the use of nanosystems based on GNP for the detection of α-syn or P-Tau in clinical settings has not been reported in spite of the fact that these biomarkers are relevant for PD and AD, respectively.
Another reason points to some concerns related to GNP toxicity and long-term accumulation [ 224 ]. However, GNP have been functionalized with different molecules to decrease their interaction with plasma proteins, increasing their targeting, and thus, showing a reduction in their side effects. For example, one clinical trial analyzed GNP functionalized with a siRNA for gliobastoma [ 225 , 226 ]. This GNP-based therapy exhibited interesting results in patients treated for six months with tolerable side effects (which disappeared at the end of the treatment) being capable of crossing the BBB, promoting tumoral cell apoptosis, and tumor shrinkage. This example shows the potential use of GNP, their specificity, and in which their benefits outweigh the side effects.
In general, these sections collected and highlighted key literature contributing to the engineering of GNP and the development of a critical rational design to generate adequate analysis for targeting, delivery, diagnosis, therapy, or theragnosis. However, there is still another relevant point to address: the cytotoxic effect in elderly patients [ 227 , 228 ]. It is known that their brain homeostatic capacity is reduced, and there is a lack of studies related to this age, requiring a further in-depth exploration of the toxic effect on this people. Thus, it is possible to generate a complete understanding of the GNP function in a physiological context. Strategies such as Nose-to-brain administration or the use of biodegradable nanosystems, such as those synthesized with poly-D-L-lactide-co-glycolide, polylactic acid, poly-e-caprolactone, or even natural materials such as chitosan [ 229 ] may help to minimize the possible toxic effects associated with bioaccumulation (Fig. 8 ) [ 140 , 209 , 230 ], improving their safety. Unfortunately, this research is still incipient and far from being analyzed in clinical trials. However, GNP are highly modifiable structures which their applications keep as potential and promising niches to explore and exploit to develop new therapies. In this sense, due to their tunable GNP nature, research that focuses on GNP behavior and their biological interactions can help to unravel and predict their potential effects and, therefore, raise the knowledge necessary for the rational design of gold nanosystems.
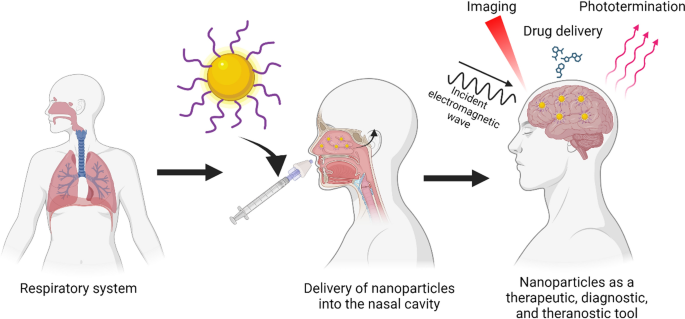
Overview of the ideal type of GNP administration for different applications such as therapy, diagnosis, and theragnosis to reach the CNS for the treatment of neurodegenerative diseases. Created with BioRender.com
Impact of GNP on the aggregation process: relevant issues for a rational design
Interestingly, another function of GNP is acting as chaperones due to their modulation, inhibition, or promotion properties exhibited in the amyloid aggregation process [ 231 ]. As explained above, the aggregation process can produce several species, such as oligomers, which are considered the most toxic species. Targeting the aggregation process using GNP becomes an interesting and potential strategy, not only because there are few reports in the literature regarding α-syn or Tau protein, but also because it is a potential niche to develop different therapeutic strategies for rational design. This has been widely observed and demonstrated using other kinds of amyloid proteins, such as amyloid-β [ 232 , 233 , 234 ] or β-microglobulin [ 235 , 236 , 237 ]. Thus, understanding the molecular mechanisms of the GNP-protein interaction may guide to develop new and alternative strategy to gain control over the aggregation process.
In this regard, one strategy widely used is monitoring the fibrillation process with ThT [ 238 , 239 , 240 ]. In Álvarez et al . [ 241 ], the authors analyzed the effect of different diameters and concentrations of GNS on the kinetics of α-syn, observing all sizes accelerated α-syn kinetics, but only 22 nm GNS were found to be preferentially bound to the fibril surface (Fig. 9 a–c). On the other hand, in presence of GNS functionalized with porphyrin, there was not formation of fibrils, monitored by ThT and AFM [ 242 ]. Indeed, when this sample was analyzed by circular dichroism, α-syn remained in its normal α-helical pattern without the characteristic β-sheet signal, making it a potential therapeutic strategy (Fig. 9 d–f). Another recent study was carried out by Maity et al. [ 243 ] using GNS functionalized with naringenin, a polyphenolic compound obtained mainly from several citrus fruits, showing an inhibitory effect on α-syn kinetics and stabilizing α-syn in its helix-α structure [ 219 ]. Similar results were observed using GNS functionalized with β-boswellic acid, a plant-derived terpenoid, which inhibit the Tau aggregation process [ 244 ].
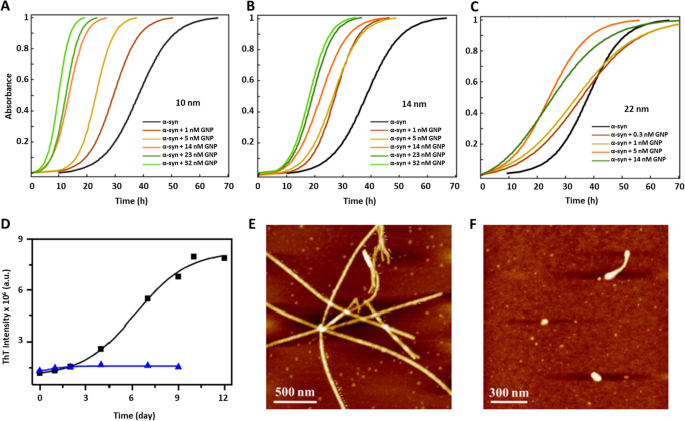
Copyright 2013, American Chemical Society. D Kinetics of α-syn in the absence (black trace) and in the presence of GNS functionalized with porphyrin (blue trace). AFM images of α-syn incubated alone E and in presence of GNS functionalized with porphyrin F after 7 days. Adapted with permission from Ref. [ 242 ]. Copyright 2020, American Chemical Society
Kinetic assays of α-syn. A – C Kinetics of α-syn in the presence of different sizes and concentrations of GNS. Adapted with permission from Ref. [ 241 ].
Another type of GNP analyzed by kinetics assays is GNCls. This GNP have diameters of 2 nm or less, allowing them an efficient urinary excretion compared to larger GNP diminishing their secondary problems [ 245 ]. GNCls also exhibit a strong fluorescence in the visible and near-infrared spectrum and therefore, they have emerged as interesting tools to use in fluorescent bioimaging [ 57 , 246 ]. GNCls functionalized with N-isobutyryl-L-cysteine exhibited an inhibitory effect on the aggregation process of α-syn studied by ThT [ 192 ]. Mahapatra et al . [ 247 ] also studied the capping-charge effect by introducing either a negative charge or an apolar agent on the surface of GNCls. Although both nanosystems decreased the neurotoxic effect of α-syn aggregates, they worked in different ways. Whereas negative GNCls inhibited the aggregation process, producing nonfibrillar aggregates, apolar GNCls accelerated the aggregation process, producing ribbon-like aggregates. Thus, both classes of GNCls guided the toxic aggregates towards less toxic species. Finally, the authors only analyzed the apolar GNCls in in vivo studies, which exhibited an increase in brain delivery and blood–brain barrier (BBB) permeability in a mouse model.
However, although GNP exhibit interesting properties and uses, they have shown different roles in peptide aggregation that could lead to deleterious biological consequences [ 248 ]. Specifically, GNP-protein interactions can alter how proteins behave on the GNP surface, modifying the protein adsorption and orientation and modulating their activity. [ 249 ]. Thus, their potential use requires a detailed analysis to clarify the impact of GNP on biological systems for generating a rational design of GNP. Yang et al . [ 250 ] studied the effect of negatively and positively charged GNP on α-syn orientation. They demonstrated that protein orientation depends on the surface charge: negatively charged citrate-coated GNP interacted with the N-terminal whereas positively charged poly(allylamine hydrochloride)-coated GNP (PAH-GNP) interacted with the C-terminal. These results were accompanied by an increase in the β-sheet content, suggesting that PAH-GNP induce conformational changes resembling the aggregation state of α-syn. A similar result was observed by Lin et al . [ 251 ]. GNP coated with (16-mercaptohexadecyl) trimethylammonium bromide (MTAB), a cationic agent, also promoted N-terminus exposure similar to PAH. However, α-syn interacts with MTAB-coated or PAH-coated GNP in different ways because of their respective intermolecular interactions. While MTAB is unable to create hydrogen bonds with α-syn, PAH contains ammonium ions that facilitate the formation of hydrogen-bonding interactions.
Interestingly, McClain et al . [ 32 ] synthetized GNP coated with sodium dodecyl sulfate (SDS-GNP) to mimic the effect of biological membranes on α-syn conformation. This capping agent presents a negative charge that interacted with the N-terminus of α-syn, resembling the interaction of GNP with citrate. In fact, SDS-GNP were also found to expose the NAC region in a comparable manner to PAH-GNP. These results suggest that the effect of α-syn/GNP interaction could induce a higher α-syn propensity to aggregate depending on surface chemistry, modifying the orientation and adsorption of α-syn onto GNP. Therefore, determining this information becomes mandatory in order to predict and understand GNP behavior and their effects on biological systems and to apply this knowledge to develop future nanomaterials (Fig. 10 ).
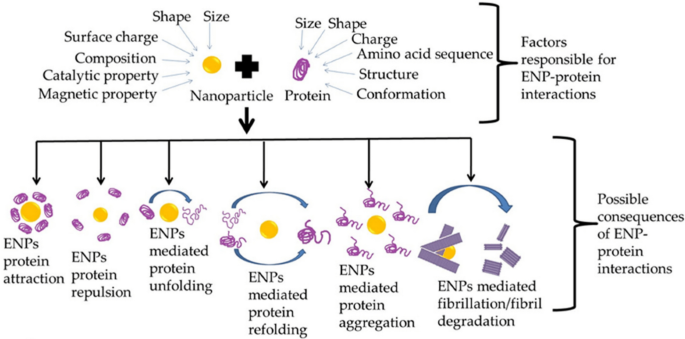
Different functions or consequences of engineering nanoparticles (ENP) interacting with proteins. As a product of this interaction, nanoparticles are capable of unfolding, refolding, promoting protein aggregation, or mediating the fibrillation or defibrillation process. Adapted with permission from Ref. [ 231 ]. 2022, by the authors. Licensee MDPI, Basel, Switzerland. This article is an open access article distributed under the terms and conditions of the Creative Commons Attribution (CC BY) license ( https://creativecommons.org/licenses/by/4.0/ )
Challenges and potential risks of GNP use: neurotoxicity
The explosive development of GNP has raised interesting concerns related to their uses and applications. The surface and geometric design of GNP can modify or alter the biological responses both in in vitro and in vivo studies and in fact, the use of GNP on biological models is not free of limitations, risky factors, or side effects [ 252 , 253 ]. In this regard, current research has been conducted on cytotoxicity analysis, and especially related to neurotoxicity [ 103 , 254 ]. These studies have become relevant because any issue as a result of the use of GNP in the CNS has a major impact due to the low rate of regeneration of the CNS, and considering that the brain is a sensitive organ to the oxidative stress generated by GNP [ 130 , 255 ]. Thus, the neurotoxicity of GNP has become a key parameter to improve and optimize nanostructures in order to design safer and non-toxic GNP for potential therapeutic applications in neurodegenerative diseases [ 256 ].
In fact, GNP have exhibited significant cytotoxic effects, such as generation of oxidative stress, disruption of the lipid bilayer, necrosis/apoptosis, mitochondrial dysfunction, and DNA damage [ 103 ]. These harmful effects are controversial because there is a wide spectrum of studies and analyses using different sizes and shapes of GNP, and there are also differences in the physicochemical nature of GNP coating and the doses or administration routes of GNP [ 103 , 130 , 253 ]. Thus, this wide variety of studies makes it difficult to generalize and point out the effect or behavior of GNP on biological systems, rendering this analysis nontrivial. In this sense, there have been numerous in vitro studies published in the literature describing the neurotoxic effects of GNP, but only are few in vivo studies have been reported [ 103 ]. Because the use and application of GNP have sparked an explosive growing interest in biomedicine, especially in CSN disorders, it is mandatory to disclose the major spectrum of neurotoxic effects that GNP can exhibit. Therefore, this review will concentrate on considering and collecting in vivo , in vitro , and also ex vivo studies that have documented the neurotoxic effects of GNP in order to display the current state-of-art research related to this key parameter.
Nowadays, the most common and widely studied GNP shape is the sphere. Different sizes, treatments, administration routes, coatings, and also the type of animal, their sex, and their age, are all parameters that make it difficult to assemble the results in order to surmise the effect of a particular GNP. Bare GNS with different sizes have been analyzed in in vivo studies, yielding somewhat conflicting findings. GNS with a diameter of 10 or 30 nm have shown neurotoxic effects [ 257 ], while GNS with a diameter of 12.5 or 50 nm have been reported to be non-neurotoxic [ 258 ]. Both studies were carried out with different GNP concentrations, types of animals, and treatments. Similar neurotoxic effects, learning impairment, biochemical changes, and a slight apoptosis and inflammatory response were exhibited in the case of GNS [ 259 , 260 , 261 ] administered either intraperitoneally or by a unilateral stereotactic injection into the cerebral cortex [ 262 , 263 ]. Of note GNS with a diameter of 50 nm injected intravenously showed a non-neurotoxic effect [ 264 ]. Another kind of analysis reported is the ex vivo study using the patch-clamp method on CA1 and CA3 neurons. In this case, stars and spheres were analyzed, but both types of GNP provoked disturbances in neuronal functions similar to those found under pathological conditions [ 265 , 266 ].
Interestingly, GNP can diminish these toxic effects with an appropriate coating [ 130 , 252 ]. Although GNS and GNCls with different coatings have commonly exhibited a non-neurotoxic effect [ 267 , 268 , 269 ], some coatings have exhibited a slight decrease in cell viability in in vitro assays [ 270 ]. Regarding in vivo assays, GNS functionalized with imidazole exhibited a neurotoxic effect, whereas bare GNS did not impact cell viability [ 271 ]. Another example of the apparent contradictory effect of GNS is coating with PEG, which is the most common molecule used to increase GNP stability [ 272 ]. GNS-PEG showed a toxic effect in mice that was reverted by a second functionalization with Trolox, an antioxidant molecule [ 273 ]. However, GNS-PEG exhibited a non-neurotoxic effect compared to the bare GNS in rats [ 274 ]. This non-neurotoxic effect was also observed using different shapes of GNP functionalized with PEG in ex vivo assays [ 275 , 276 ]. Another interesting analysis was carried out using GNS or GNCls with different coatings in a PD-induced model. These studies demonstrated that GNP exhibited a neuroprotective effect, reversing the deleterious damage observed in this disease [ 191 , 192 , 277 ], and showed an anti-neuroinflammatory effect compared to the controls [ 277 ]. This neuroprotective effect was also observed in a transgenic Tau mouse model of AD, in which the presence of GNP improved the cognitive functions of the mice (Fig. 12 ) [ 194 ].
Commonly, the neurotoxic effect of GNP has been evaluated only on neurons from different models through in vitro and in vivo assays, as described above. However, the CNS not only contains neurons but also glial cells, which are closely related to the normal functioning of the brain. There are different kinds of glia with specific functions, but in general, all of them participate in providing a key support system to the neurons [ 278 , 279 ]. Thereby, the evaluation of GNP cytotoxicity both in neuronal models and in glia models allows for a general idea of the impact of GNP on the CNS. Only a few reports of the GNP effect on glia have been reported so far. Leite et al. [ 270 ] analyzed the effect of GNS-PEG on a 3D neural model to precisely determine the cytotoxicity that mimics the in vivo physiological conditions. This novel approach demonstrated that brainspheres were not affected by the different GNP used. Similar results were observed using GNS-PEG in either a human astrocyte cell line or primary cultures [ 275 , 280 ]. This kind of information is key because astrocytes participate in maintaining adequate levels of some neurotransmitters, support the synaptic connection, and consolidate the learning and memory cognitive process [ 281 ]. Another relevant type of cell are microglia, which are the immune cells of the brain. Their activation or death could lead to the generation of neurodegenerative diseases or the loss of homeostasis. GNS with different coatings exhibited a non-cytotoxic effect in different microglial cell lines or primary cultures (Fig. 11 ) [ 267 , 275 , 277 , 282 , 283 ] as well as in in vivo assays analyzing either astrocytes or microglia [ 273 , 284 ]. A similar non-cytotoxic effect was observed in GNCls with different coatings in in vitro assays for astrocytes or microglia [ 268 , 269 , 285 , 286 , 287 ]. Thus, the cytotoxic effect exhibited by GNP highly depends on the coating used, and modulating this parameter allows decreasing or avoiding these side effects in order to design and obtain safer GNP for potential therapies.
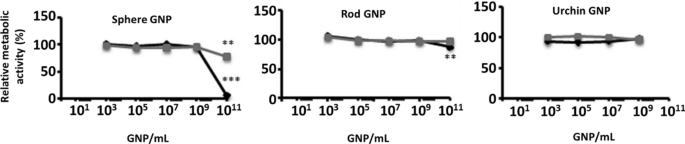
Copyright 2010, American Chemical Society
Cytotoxicity effect of GNP coated with either CTAB or PEG in a microglial (N9) cell line using different concentrations of GNP. Metabolic activity was evaluated using the MTT assay. Adapted with permission from Ref. [ 283 ].
Perspectives on strategies to enhance the analysis of GNP effect: 3D culture and the microfluidic model
GNP are potential tools to carry drugs and treat brain disorders. It is already known that GNP can be evaluated using in vitro cells in 2D cell cultures, which do not resemble the complexity of the in vivo nanoparticle-cell interaction. In this sense, dynamic methodologies highlight as interesting tools to introduce different variables that normally are not considered in static methods, mainly because a dynamic environment produces shear stress, changing the composition and structure of the biomolecules when they interact with nanoparticles [ 288 , 289 , 290 , 291 ]. In this sense, microfluidic (MF) has several advantages over conventional methods by incorporating fluid flow and mechanical forces, bringing a step closer to mimicking the in vivo microenvironment, being an in vivo-like method for studying nano-bio interfaces [ 292 , 293 , 294 , 295 ], for example, emulating the physiological conditions when administering nanoparticles into the bloodstream to reach different targets. In fact, MF corresponds to one of the pioneering techniques to analyze and study hydrodynamic regimes at small scales. This technique utilizes microscale channels to manipulate fluids at the nanoliter scale and suspend objects in a controlled manner [ 296 ], and it has rapidly progressed over the past decade, improving from basic devices to large-scale two-dimensional integration of components, 3D architectures, and nonlinear autoregulatory systems [ 297 ].
Currently, one of the future challenges is to obtain 3D in vitro nervous system models because the 2D cell cultures of neurite networks and in vitro brain cell architecture is limited and does not fully represent the function and complexity of the brain, especially during development and neuronal plasticity. The primary challenge in investigating drug delivery or any kind of molecule in 3D brain models lies in the complex and non-repetitive 3D structure of the brain, as well as its complex integrated circuits. In contrast, the current 2D cell culture models lack the ability to accurately replicate the complexity of the brain. At he moment, neuronal networks arranged in sophisticated 3D matrices or optimum combinations of different cells represent the inception of a groundbreaking generation that has the potential to revolutionize future results [ 298 , 299 ]. Now, the field of bioengineering is striving to emulate the physiological systems with utmost accuracy to study the administration of nanoparticles. Accordingly, 3D in vitro models are the latest and most advanced method in this field. They provide the ability to replicate the physiological conditions seen in vivo [ 298 , 300 ], resulting in improved cell survival, differentiation, and more accurate reproduction of electrical activity [ 270 ]. Lab-on-a-chip (LOC) has been used for neurobiology applications due to the ability of microfluidic channels to approximate the size and flow conditions found in in vivo capillaries, the so called in vivo-like flow. Therefore, studying the delivery of nanoparticles or their impact on specific biological systems is challenging due to dramatic variations in their efficacy across 2D and 3D culture systems [ 301 ].
In fact, there are microfluidic devices that simulate a complex functional and anatomical structure composed of endothelial cells and their BBB, forming tight junctions and constructing a 3D brain spheroid model to study the effect of GNP. Nevertheless, there are few publications describing these models to study the use of GNP [ 293 , 302 ]. For example, Smirnova et al . [ 270 ] studied the effect of GNP functionalized with citrate and PEG using two different human 3D CNS in vitro models. In this work, they fabricated a 3D Lund human mesencephalic spheroid and a human iPSC-derived brain spheroid, called BrainSphere. The BrainSpheres is a multicellular 3D brain model composed of neurons, astrocytes, and oligodendrocytes, forming a complex multicellular model (Fig. 12 ). The comparison between single 3D models and a complex multicellular 3D model was relevant in the study of nanoparticle uptake, morphological and molecular alterations, and cytotoxicity, among others. The results indicated that GNP and their application in the 3D brain spheroid models are well suited to characterize the neurotoxicity of nanoparticles, and that the mixed populations of neural cells within the model allow the presence of glial cells (which participate in brain support). Therefore, GNP neurotoxicity decreased in comparison with the single 3D model, enhancing the comprehensiveness of this study in capturing physiological conditions. In this sense, a similar result was obtained by Palma-Florez et al. [ 292 ]. The authors mimicked the BBB with human astrocytes, pericytes and endothelial cells in a microfluidic device and analyzed a gold nanosystem, GNR-PEG-D1/Ang2, determining its non-cytotoxic effect on the tri-culture and the enhancement of nanosystem permeability (Fig. 13 ).
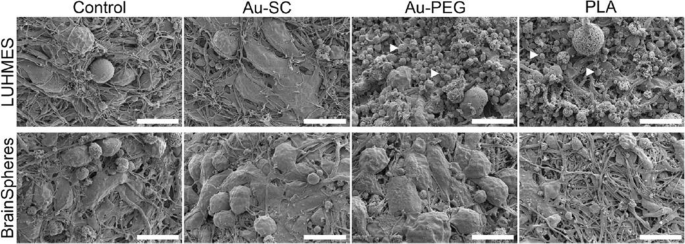
Copyright 2019, Springer Nature
Morphology of 3D LUHMES and BrainSpheres exposed to Au-SC (6 μg/mL), Au-PEG (20 μg/mL) and PLA-NP (20 μg/mL) for 72 h. The control represents untreated spheroids. White arrowheads indicate cell debris. Scale bars: 10 μm. Adapted with permission from Ref. [ 270 ].
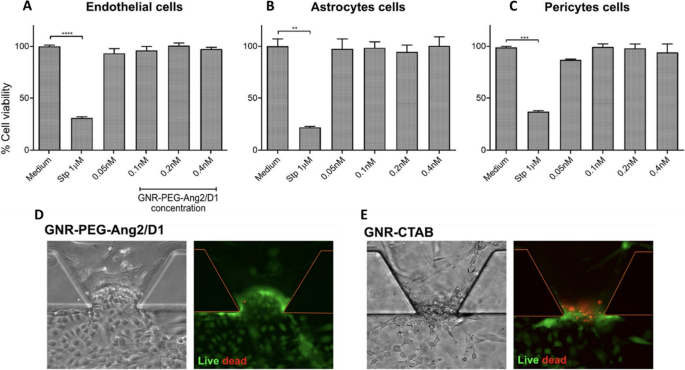
Cytotoxic effect of GNR-PEG-Ang2/D1 on cells that constitute the BBB at three different concentrations. A-C, cytotoxic effect of the nanosystem on each cell line, evaluated separately. D and E, GNR-PEG-Ang2/D1 analyzed on the endothelial barrier in the BBB-on-a-chip. GNR-CTAB was used as a control. Adapted with permission from Ref. [ 292 ]. Copyright, 2023 Springer Nature
Likewise, it is important to mention that one of the key aspects of most protein folding or aggregation studies, as well as studies performed in the presence of inhibitory agents or GNP, are performed in bulk assays. This implies that the degree of freedom possessed by proteins or peptides is greater, so it is uncertain whether these studies truly reflect the behavior of a protein in living organisms [ 303 ]. Indeed, various studies have shown that the use of confined environments alters the kinetics and thermodynamics of protein–protein interactions [ 304 ], even promoting the appearance of β-secondary structures [ 305 ]. Additionally, since in vivo protein folding occurs mainly under rigid confinement characteristics, studies with models in confined spaces should be included in in vitro studies, constituting an interesting and challenging approach to replicate a typical scenario of the in vivo aggregation process [ 306 , 307 , 308 ]. In this sense, obtaining this information is mandatory because, bulk studies have indicated that the modification of the stirring speeds completely alters the aggregation process and morphology of the Aβ42 aggregates. In the same way, studies using microfluidics have shown that modifying the flow inside the microchannels together through a spatial confinement alters the morphology of amyloid aggregates [ 309 , 310 ]. Furthermore, the confinement added to the low retention times achieved in the microchannels takes advantage of the initial stages of the amyloid aggregation process [ 311 , 312 ], which are key steps directly related to the generation of neurotoxic oligomeric species of amyloid aggregates. Thus, the use of microfluidics emerges as a powerful methodology to study the aggregation process of amyloids in the presence of GNP, as well as their effect on the generation of toxic oligomeric species of amyloids. To date, no studies have used microfluidics to investigate the effect of functionalized GNP on the amyloid aggregation process in a confined space using, Tau or α-syn amyloid protein in a dynamic regime. Therefore, for the purpose of future studies, MF research has taken a keen interest in investigating this particular field of study due to the limited exploration of the aggregation process within microfluidic devices.
Conclusions
Plasmonic GNP have gained interest in the biomedical field due to their high versatility and optical and electronic properties. This review highlighted their application in the development of diagnostic tools for PD and AD and described how the selectivity and sensitivity of current methods have been improved. In this sense, their use as delivery therapeutic agents or in vivo imaging has been proposed for the therapy and diagnosis of neurodegenerative diseases, taking advantage of the plasmonic properties of these nanomaterials. However, the research on GNP and their risk factors, neurotoxicity, targeting, selectivity, and sensitivity in the early stages of these diseases in preclinical models is incipient and remains a challenge. Furthermore, the investigation of the interactions between nanoparticles and biological barriers, as well as cellular responses, plays a critical role in developing preclinical and clinical applications of nanomaterials. For these reasons, cutting-edge methodologies such as 3D cultures and microfluidics have emerged as significant innovations by mimicking in vivo biological conditions, thus overcoming the limitations of bulk assays, and providing valuable and representative data for characterizing nanomaterials in relevant biological contexts. Furthermore, using microfluidics and "organ-on-a-chip" platforms highlights the emulation of physiological conditions to reduce the need for animal experimentation and contribute to understanding how GNP can interact with cells and cross the BBB. These advanced approaches are an essential step towards a deeper understanding of nanomaterial-cell interactions and the accurate assessment of their impact on the biological environment. In summary, the use of GNP in the diagnosis, targeting, therapy, and monitoring of neurodegenerative diseases represents an exciting and promising field in biomedical research. Although there are challenges ahead, such as safety, potential intranasal administration, the capping with biodegradable materials, or GNP biodistribution, the positive impact of these nanotools on the quality of patients’ lives and the advance in understanding these complex disorders is undeniable. It is crucial to maintain the collaboration between researchers and experts in various disciplines to make progress in this exciting field and potentially revolutionize the treatment of neurological diseases.
Availability of data and materials
Not applicable.
Abbreviations
Alzheimer’s disease
Blood–brain barrier
Central nervous system
Gold nanoparticles
Neurofibrillary tangles
- Parkinson’s disease
Hyperphosphorylated Tau
α-Synuclein
Gold nanospheres
Gold nanorods
Gold nanoprisms
Gold nanostars
Gold nanoclusters
Polyethylene glycol
Gold nanocomposites
1-Methyl-4-phenyl-1,2,3,6-tetrahydropyridine
Thioflavin-based kinetic assay
Magnetic nanoparticles
Limit od detection
Nerve growth factor
Microfluidics
Emamzadeh FN, Surguchov A. Parkinson’s disease: biomarkers, treatment, and risk factors. Front Neurosci. 2018. https://doi.org/10.3389/fnins.2018.00612 .
Article PubMed PubMed Central Google Scholar
Du X, Wang X, Geng M. Alzheimer’s disease hypothesis and related therapies. Transl Neurodegener. 2018;7:2.
Irvine GB, El-Agnaf OM, Shankar GM, Walsh DM. Protein aggregation in the brain: the molecular basis for Alzheimer’s and Parkinson’s diseases. Mol Med. 2008. https://doi.org/10.2119/2007-00100.Irvine .
Tan SH, Karri V, Tay NWR, Chang KH, Ah HY, Ng PQ, et al. Emerging pathways to neurodegeneration: dissecting the critical molecular mechanisms in Alzheimer’s disease Parkinson’s disease. Biomed Pharmacother. 2019. https://doi.org/10.1016/j.biopha.2018.12.101 .
Article PubMed Google Scholar
Xie A, Gao J, Xu L, Meng D. Shared mechanisms of neurodegeneration in alzheimer’s disease and parkinson’s disease. Biomed Res Int. 2014. https://doi.org/10.1155/2014/648740 .
Liu D, Li W, Jiang X, Bai S, Liu J, Liu X, et al. Using near-infrared enhanced thermozyme and scFv dual-conjugated Au nanorods for detection and targeted photothermal treatment of Alzheimer’s disease. Theranostics. 2019. https://doi.org/10.7150/thno.30649 .
Liu W, Dong X, Liu Y, Sun Y. Photoresponsive materials for intensified modulation of Alzheimer’s amyloid-β protein aggregation: a review. Acta Biomater. 2021. https://doi.org/10.1016/j.actbio.2021.01.018 .
Prince M, Comas-Herrera A, Knapp M, Guerchet M, Karagiannidou M. Improving healthcare for people living with dementia. Coverage, Quality and costs now and in the future. World Alzheimer Report 2016. 2016
Karapetyan G, Fereshetyan K, Harutyunyan H, Yenkoyan K. The synergy of β amyloid 1–42 and oxidative stress in the development of Alzheimer’s disease-like neurodegeneration of hippocampal cells. Sci Rep. 2022. https://doi.org/10.1038/s41598-022-22761-5 .
Yana MH, Wang X, Zhu X. Mitochondrial defects and oxidative stress in Alzheimer disease and Parkinson disease. Free Radic Biol Med. 2013. https://doi.org/10.1016/j.freeradbiomed.2012.11.014 .
Article Google Scholar
Ahmed M, Davis J, Aucoin D, Sato T, Ahuja S, Aimoto S, et al. Structural conversion of neurotoxic amyloid-beta(1–42) oligomers to fibrils. Nat Struct Mol Biol. 2010;17:561–7.
Article CAS PubMed PubMed Central Google Scholar
Zheng H, Koo EH. The amyloid precursor protein: beyond amyloid. Mol Neurodegener. 2006;1:5.
LaFerla FM, Green KN, Oddo S. Intracellular amyloid-beta in Alzheimer’s disease. Nat Rev Neurosci. 2007;8:499–509.
Article CAS PubMed Google Scholar
Liu P-P, Xie Y, Meng X-Y, Kang J-S. History and progress of hypotheses and clinical trials for Alzheimer’s disease. Signal Transduct Target Ther. 2019;4:29. https://doi.org/10.1038/s41392-019-0063-8 .
Silvestro S, Valeri A, Mazzon E. Aducanumab and its effects on tau pathology: is this the turning point of amyloid hypothesis? Int J Mol Sci. 2022;23:2011.
Kametani F, Hasegawa M. Reconsideration of amyloid hypothesis and tau hypothesis in Alzheimer’s disease. Front Neurosci. 2018. https://doi.org/10.3389/fnins.2018.00025 .
Marcatti M, Fracassi A, Montalbano M, Natarajan C, Krishnan B, Kayed R, et al. Aβ/tau oligomer interplay at human synapses supports shifting therapeutic targets for Alzheimer’s disease. Cell Mol Life Sci. 2022;79:222. https://doi.org/10.1007/s00018-022-04255-9 .
Tripathi T, Khan H. Direct interaction between the β-amyloid core and tau facilitates cross-seeding: a novel target for therapeutic intervention. Biochemistry. 2020;59:341–2. https://doi.org/10.1021/acs.biochem.9b01087 .
Weglinski C, Jeans A. Amyloid-β in Alzheimer’s disease—front and centre after all? Neuronal Signal. 2023;7:NS20220086. https://doi.org/10.1042/NS20220086 .
Gulisano W, Maugeri D, Baltrons MA, Fà M, Amato A, Palmeri A, et al. Role of amyloid-β and tau proteins in Alzheimer’s disease: confuting the amyloid cascade. J Alzheimer’s Dis. 2018. https://doi.org/10.3233/JAD-179935 .
Avila J, Lucas JJ, Pérez M, Hernández F. Role of tau protein in both physiological and pathological conditions. Physiol Rev. 2004. https://doi.org/10.1152/physrev.00024.2003 .
Kovacs GG. Chapter 25 Tauopathies. Handb Clin Neurol. 2017;145:355–68.
Pîrşcoveanu DFV, Pirici I, Tudorică V, Bălşeanu TA, Albu VC, Bondari S, et al. Tau protein in neurodegenerative diseases—a review. Roman J Morphol Embryol. 2017;58(4):1141–50.
Google Scholar
Ameri M, Shabaninejad Z, Movahedpour A, Sahebkar A, Mohammadi S, Hosseindoost S, et al. Biosensors for detection of Tau protein as an Alzheimer’s disease marker. Int J Biol Macromol. 2020. https://doi.org/10.1016/j.ijbiomac.2020.06.239 .
Wang Y, Mandelkow E. Tau in physiology and pathology. Nat Rev Neurosci. 2016;17:5–21.
Buée L, Bussière T, Buée-Scherrer V, Delacourte A, Hof PR. Tau protein isoforms, phosphorylation and role in neurodegenerative disorders. Brain Res Rev. 2000. https://doi.org/10.1016/B978-012351830-9/50023-8 .
Molinuevo JL, Ayton S, Batrla R, Bednar MM, Bittner T, Cummings J, et al. Current state of Alzheimer’s fluid biomarkers. Acta Neuropathol. 2018. https://doi.org/10.1007/s00401-018-1932-x .
Sunderland T, Linker G, Mirza N, Putnam KT, Friedman DL, Kimmel LH, et al. Decreased β-amyloid1–42 and increased tau levels in cerebrospinal fluid of patients with Alzheimer disease. JAMA. 2003. https://doi.org/10.1001/jama.289.16.2094 .
Spillantini MG, Schmidt ML, Lee VMY, Trojanowski JQ, Jakes R, Goedert M. α-synuclein in Lewy bodies [8]. Nature. 1997. https://doi.org/10.1038/42166 .
George JM. The synucleins. Genome Biol. 2002;3:1–6.
Goedert M. Alpha-synuclein and neurodegenerative diseases. Nat Rev Neurosci. 2001. https://doi.org/10.1038/35081564 .
McClain SM, Ojoawo AM, Lin W, Rienstra CM, Murphy CJ. Interaction of alpha-synuclein and its mutants with rigid lipid vesicle mimics of varying surface curvature. ACS Nano. 2020. https://doi.org/10.1021/acsnano.0c03420 .
Giasson BI, Murray IVJ, Trojanowski JQ, Lee VMY. A hydrophobic stretch of 12 amino acid residues in the middle of α-synuclein is essential for filament assembly. J Biol Chem. 2001. https://doi.org/10.1074/jbc.M008919200 .
Winner B, Jappelli R, Maji SK, Desplats PA, Boyer L, Aigner S, et al. In vivo demonstration that α-synuclein oligomers are toxic. Proc Natl Acad Sci U S A. 2011. https://doi.org/10.1073/pnas.1100976108 .
Du XY, Xie XX, Liu RT. The role of α-synuclein oligomers in parkinson’s disease. Int J Mol Sci. 2020. https://doi.org/10.3390/ijms21228645 .
Bengoa-Vergniory N, Roberts RF, Wade-Martins R, Alegre-Abarrategui J. Alpha-synuclein oligomers: a new hope. Acta Neuropathol. 2017. https://doi.org/10.1007/s00401-017-1755-1 .
Kulenkampff K, Wolf Perez AM, Sormanni P, Habchi J, Vendruscolo M. Quantifying misfolded protein oligomers as drug targets and biomarkers in Alzheimer and Parkinson diseases. Nat Rev Chem. 2021. https://doi.org/10.1038/s41570-021-00254-9 .
Schmid AW, Fauvet B, Moniatte M, Lashuel HA. Alpha-Synuclein post-Translational modifications as potential biomarkers for parkinson disease and other synucleinopathies. Mol Cell Proteomics. 2013. https://doi.org/10.1074/mcp.R113.032730 .
Schuster J, Funke SA. Methods for the specific detection and quantitation of amyloid-β oligomers in cerebrospinal fluid. JAD. 2016. https://doi.org/10.3233/JAD-151029 .
Ntetsika T, Papathoma PE, Markaki I. Novel targeted therapies for Parkinson’s disease. Mol Med. 2021. https://doi.org/10.1186/s10020-021-00279-2 .
Polanco JC, Li C, Bodea LG, Martinez-Marmol R, Meunier FA, Götz J. Amyloid-β and tau complexity—towards improved biomarkers and targeted therapies. Nat Rev Neurol. 2018. https://doi.org/10.1038/nrneurol.2017.162 .
BulckVan M, Sierra-Magro A, Alarcon-Gil J, Perez-Castillo A, Morales-Garcia JA. Novel approaches for the treatment of alzheimer’s and parkinson’s disease. Int J Mol Sci. 2019;20:719.
Linazasoro G. Recent failures of new potential symptomatic treatments for Parkinson’s disease: Causes and solutions. Movement Disord. 2004. https://doi.org/10.1002/mds.20120 .
Yiannopoulou KG, Anastasiou AI, Zachariou V, Pelidou SH. Reasons for failed trials of disease-modifying treatments for alzheimer disease and their contribution in recent research. Biomedicines. 2019;7:97.
Baldacci F, Mazzucchi S, Della Vecchia A, Giampietri L, Giannini N, Koronyo-Hamaoui M, et al. The path to biomarker-based diagnostic criteria for the spectrum of neurodegenerative diseases. Expert Rev Mol Diagn. 2020. https://doi.org/10.1080/14737159.2020.1731306 .
Obrocki P, Khatun A, Ness D, Senkevich K, Hanrieder J, Capraro F, et al. Perspectives in fluid biomarkers in neurodegeneration from the 2019 biomarkers in neurodegenerative diseases course—a joint PhD student course at University College London and University of Gothenburg. Alz Res Ther. 2020. https://doi.org/10.1186/s13195-020-00586- .
Sela H, Cohen H, Elia P, Zach R, Karpas Z, Zeiri Y. Spontaneous penetration of gold nanoparticles through the blood brain barrier (BBB). J Nanobiotechnology. 2015;13:71.
Hammami I, Alabdallah NM, Jomaa A, Kamoun M. Gold nanoparticles: Synthesis properties and applications. J King Saud Univ Sci. 2021. https://doi.org/10.1016/j.jksus.2021.101560 .
Nejati K, Dadashpour M, Gharibi T, Mellatyar H, Akbarzadeh A. Biomedical applications of functionalized gold nanoparticles: a review. J Clust Sci. 2022. https://doi.org/10.1007/s10876-020-01955-9 .
Dreaden EC, Alkilany AM, Huang X, Murphy CJ, El-Sayed MA. The golden age: gold nanoparticles for biomedicine. Chem Soc Rev. 2012;41:2740–79.
Siddique S, Chow JCL. Gold nanoparticles for drug delivery and cancer therapy. Appl Sci. 2020. https://doi.org/10.3390/app10113824 .
Zhao J, Xu N, Yang X, Ling G, Zhang P. The roles of gold nanoparticles in the detection of amyloid-β peptide for Alzheimer’s disease. Colloids Interface Sci Commun. 2022. https://doi.org/10.1016/j.colcom.2021.100579 .
Sperling RA, Rivera Gil P, Zhang F, Zanella M, Parak WJ. Biological applications of gold nanoparticles. Chem Soc Rev. 2008;37:1896–908.
Mitchell MJ, Billingsley MM, Haley RM, Wechsler ME, Peppas NA, Langer R. Engineering precision nanoparticles for drug delivery. Nat Rev Drug Discov. 2021. https://doi.org/10.1038/s41573-020-0090-8 .
Huang X, El-Sayed MA, GolHuang X, El-Sayed MA. Gold nanoparticles: Optical properties and implementations in cancer diagnosis and photothermal therapy. J Adv Res. 2010. https://doi.org/10.1016/j.jare.2010.02.002d .
Huang X, El-Sayed MA. Gold nanoparticles: optical properties and implementations in cancer diagnosis and photothermal therapy. J Adv Res. 2010. https://doi.org/10.1016/j.jare.2010.02.002 .
Zhang G. Functional gold nanoparticles for sensing applications. Nanotechnol Rev. 2013. https://doi.org/10.1515/ntrev-2012-0088 .
Oyarzún MP, Tapia-Arellano A, Cabrera P, Jara-Guajardo P, Kogan MJ. Plasmonic nanoparticles as optical sensing probes for the detection of alzheimer’s disease. Sensors. 2021. https://doi.org/10.3390/s21062067 .
Saha K, Agasti SS, Kim C, Li X, Rotello VM. Gold nanoparticles in chemical and biological sensing. Chem Rev. 2012. https://doi.org/10.1021/cr2001178 .
Bagheri S, Yasemi M, Safaie-Qamsari E, Rashidiani J, Abkar M, Hassani M, et al. Using gold nanoparticles in diagnosis and treatment of melanoma cancer. Artif Cells Nanomed Biotechnol. 2018. https://doi.org/10.1080/21691401.2018.1430585 .
Gonzalez Solveyra E, Szleifer I. What is the role of curvature on the properties of nanomaterials for biomedical applications? Wiley Interdiscip Rev Nanomed Nanobiotechnol. 2016;8:334–54.
Xie X, Liao J, Shao X, Li Q, Lin Y. The effect of shape on cellular uptake of gold nanoparticles in the forms of stars, rods, and triangles. Sci Rep. 2017;7:3827. https://doi.org/10.1038/s41598-017-04229-z .
Petros RA, Desimone JM. Strategies in the design of nanoparticles for therapeutic applications. Nat Rev Drug Discov. 2010. https://doi.org/10.1038/nrd2591 .
Zare I, Yaraki MT, Speranza G, Najafabadi AH, Shourangiz-Haghighi A, Nik AB, et al. Gold nanostructures: synthesis, properties, and neurological applications. Chem Soc Rev. 2022. https://doi.org/10.1039/D1CS01111A .
Turkevich J, Stevenson PC, Hillier J. A study of the nucleation and growth processes in the synthesis of colloidal gold. Discuss Faraday Soc. 1951. https://doi.org/10.1039/df9511100055 .
Afrapoli ZB, Majidi RF, Negahdari B, Tavoosidana G. ‘Inversed Turkevich’ method for tuning the size of Gold nanoparticles: evaluation the effect of concentration and temperature. Nanomed Res J. 2018;3(4):190–6.
Tran M, DePenning R, Turner M, Padalkar S. Effect of citrate ratio and temperature on gold nanoparticle size and morphology. Mater Res Express. 2016. https://doi.org/10.1088/2053-1591/3/10/105027 .
Yazdani S, Daneshkhah A, Diwate A, Patel H, Smith J, Reul O, et al. Model for gold nanoparticle synthesis: effect of pH and reaction time. ACS Omega. 2021. https://doi.org/10.1021/acsomega.1c01418 .
Slepička P, Kasálková NS, Siegel J, Kolská Z, Švorčík V. Methods of gold and silver nanoparticles preparation. Materials. 2020. https://doi.org/10.3390/ma13010001 .
Santhosh PB, Genova J, Chamati H. Review green synthesis of gold nanoparticles: an eco-friendly approach. Chemistry. 2022. https://doi.org/10.3390/chemistry4020026 .
Rónavári A, Igaz N, Adamecz DI, Szerencsés B, Molnar C, Kónya Z, et al. Green silver and gold nanoparticles: biological synthesis approaches and potentials for biomedical applications. Molecules. 2021;26:844.
Mata R, Nakkala JR, Sadras SR. Polyphenol stabilized colloidal gold nanoparticles from Abutilon indicum leaf extract induce apoptosis in HT-29 colon cancer cells. Colloids Surf B Biointerfaces. 2016. https://doi.org/10.1016/j.colsurfb.2016.03.069 .
Zhao X, Li Z, Deng Y, Zhao Z, Li X, Xia Y. Facile synthesis of gold nanoparticles with alginate and its catalytic activity for reduction of 4-nitrophenol and H2O2 detection. Materials. 2017. https://doi.org/10.3390/ma10050557 .
Cai F, Li S, Huang H, Iqbal J, Wang C, Jiang X. Green synthesis of gold nanoparticles for immune response regulation: mechanisms, applications, and perspectives. J Biomed Mater Res A. 2022;110(2):424–42.
Dhanasekar NN, Rahul GR, Narayanan KB, Raman G, Sakthivel N. Green chemistry approach for the synthesis of gold nanoparticles using the fungus Alternaria sp. J Microbiol Biotechnol. 2015. https://doi.org/10.4014/jmb.1410.10036 .
Zuhrotun A, Oktaviani DJ, Hasanah AN. Biosynthesis of gold and silver nanoparticles using phytochemical compounds. Molecules. 2023. https://doi.org/10.3390/molecules28073240 .
Akbari A, Eshkiki ZS, Mayahi S, Amini SM. In-vitro investigation of curcumin coated gold nanoparticles effect on human colorectal adenocarcinoma cell line. Nanomedicine Res J. 2022;7:66–72.
CAS Google Scholar
Amini SM, Hadighi R, Najm M, Alipour M, Hasanpour H, Vosoogh M, et al. The therapeutic effects of curcumin-coated gold nanoparticle against leishmania major causative agent of zoonotic cutaneous leishmaniasis (ZCL): an in vitro and in vivo study. Curr Microbiol. 2023. https://doi.org/10.1007/s00284-022-03172-1 .
Nambiar S, Osei E, Fleck A, Darko J, Mutsaers AJ, Wettig S. Synthesis of curcumin-functionalized gold nanoparticles and cytotoxicity studies in human prostate cancer cell line. Appl Nanosci. 2018. https://doi.org/10.1007/s13204-018-0728-6 .
Muniyappan N, Pandeeswaran M, Amalraj A. Green synthesis of gold nanoparticles using Curcuma pseudomontana isolated curcumin: Its characterization, antimicrobial, antioxidant and anti- inflammatory activities. Environ Chem Ecotoxicol. 2021;3:117.
Article CAS Google Scholar
Fathy MM, Elfiky AA, Bashandy YS, Hamdy MM, Elgharib AM, Ibrahim IM, et al. An insight into synthesis and antitumor activity of citrate and gallate stabilizing gold nanospheres. Sci Rep. 2023. https://doi.org/10.1038/s41598-023-29821-4 .
Wu YZ, Tsai YY, Chang LS, Chen YJ. Evaluation of gallic acid-coated gold nanoparticles as an anti-aging ingredient. Pharmaceuticals. 2021;14:1071.
Neshastehriz A, Amini SM, Mohammadi A, Mahdavi SR, Mahabadi VP, Akbari A. In-vitro investigation of green synthesized gold nanoparticle’s role in combined photodynamic and radiation therapy of cancerous cells. Adv Nat Sci. 2020;11:045006.
Morales-Zavala F, Arriagada H, Hassan N, Velasco C, Riveros A, Álvarez AR, et al. Peptide multifunctionalized gold nanorods decrease toxicity of β-amyloid peptide in a Caenorhabditis elegans model of Alzheimer’s disease. Nanomedicine. 2017. https://doi.org/10.1016/j.nano.2017.06.013 .
Jara-Guajardo P, Cabrera P, Celis F, Soler M, Berlanga I, Parra-Muñoz N, et al. Gold nanoparticles mediate improved detection of β-amyloid aggregates by fluorescence. Nanomaterials. 2020;10:1–16.
Cabrera P, Jara-Guajardo P, Oyarzún MP, Parra-Muñoz N, Campos A, Soler M, et al. Surface enhanced fluorescence effect improves the in vivo detection of amyloid aggregates. Nanomedicine. 2022;44:102569.
Park K, Drummy LF, Wadams RC, Koerner H, Nepal D, Fabris L, et al. Growth mechanism of gold nanorods. Chem Mater. 2013. https://doi.org/10.1021/cm303659q .
Chhatre A, Thaokar R, Mehra A. Formation of gold nanorods by seeded growth: mechanisms and modeling. Cryst Growth Des. 2018;18(6):3269–82.
Yu X, Wang Z, Cui H, Wu X, Chai W, Wei J, et al. A review on gold nanotriangles: synthesis, self-assembly and their applications. Molecules. 2022. https://doi.org/10.3390/molecules27248766 .
Tapia-Arellano A, Gallardo-Toledo E, Ortiz C, Henríquez J, Feijóo CG, Araya E, et al. Functionalization with PEG/Angiopep-2 peptide to improve the delivery of gold nanoprisms to central nervous system: in vitro and in vivo studies. Mater Sci Eng C. 2021;121:111785.
Gao Y, Wang J, Wang W, Zhao T, Cui Y, Liu P, et al. More symmetrical “hot spots” ensure stronger plasmon-enhanced fluorescence: from Au nanorods to nanostars. Anal Chem. 2021;93:2480–9.
Becerril-Castro IB, Calderon I, Pazos-Perez N, Guerrini L, Schulz F, Feliu N, et al. Gold nanostars: synthesis, optical and SERS analytical properties. Anal Sens. 2022;2:e202200005.
Donoso-González O, Lodeiro L, Aliaga ÁE, Laguna-Bercero MA, Bollo S, Kogan MJ, et al. Functionalization of gold nanostars with cationic β-cyclodextrin-based polymer for drug co-loading and sers monitoring. Pharmaceutics. 2021;13(2):261.
Oldenburg SJ, Averitt RD, Westcott SL, Halas NJ. Nanoengineering of optical resonances. Chem Phys Lett. 1998;288:243–7.
Jaque D, Martínez Maestro L, Del Rosal B, Haro-Gonzalez P, Benayas A, Plaza JL, et al. Nanoparticles for photothermal therapies. Nanoscale. 2014;6:9494–530.
Day ES, Morton JG, West JL. Nanoparticles for thermal cancer therapy. J Biomech Eng. 2009. https://doi.org/10.1115/1.3156800 .
Li Y, He D, Tu J, Wang R, Zu C, Chen Y, et al. The comparative effect of wrapping solid gold nanoparticles and hollow gold nanoparticles with doxorubicin-loaded thermosensitive liposomes for cancer thermo-chemotherapy. Nanoscale. 2018;10:8628–41.
Abdollahi SN, Naderi M, Amoabediny G. Synthesis and characterization of hollow gold nanoparticles using silica spheres as templates. Colloids Surf A Physicochem Eng Asp. 2013;436:1069–75.
Ortiz-Castillo JE, Gallo-Villanueva RC, Madou MJ, Perez-Gonzalez VH. Anisotropic gold nanoparticles: a survey of recent synthetic methodologies. Coord Chem Rev. 2020;425: 213489.
Hassan H, Sharma P, Hasan MR, Singh S, Thakur D, Narang J. Gold nanomaterials—the golden approach from synthesis to applications. Mater Sci Energy Technol. 2022;5:375–90.
Zhang XD, Wu D, Shen X, Liu PX, Yang N, Zhao B, et al. Size-dependent in vivo toxicity of PEG-coated gold nanoparticles. Int J Nanomedicine. 2011. https://doi.org/10.2147/IJN.S21657 .
Jia YP, Shi K, Liao JF, Peng JR, Hao Y, Qu Y, et al. Effects of cetyltrimethylammonium bromide on the toxicity of gold nanorods both in vitro and in vivo: molecular origin of cytotoxicity and inflammation. Small Methods. 2020. https://doi.org/10.1002/smtd.201900799 .
Sani A, Cao C, Cui D. Toxicity of gold nanoparticles (AuNPs): a review. Biochem Biophys Rep. 2021. https://doi.org/10.1016/j.bbrep.2021.100991 .
Howard MD, Jay M, Dziubla TD, Lu X. PEGylation of nanocarrier drug delivery systems: state of the art. J Biomed Nanotechnol. 2008. https://doi.org/10.1166/jbn.2008.021 .
Klibanov AL, Maruyama K, Torchilin VP, Huang L. Amphipathic polyethyleneglycols effectively prolong the circulation time of liposomes. FEBS Lett. 1990. https://doi.org/10.1016/0014-5793(90)81016-H .
Spinelli A, Girelli M, Arosio D, Polito L, Podini P, Martino G, et al. Intracisternal delivery of PEG-coated gold nanoparticles results in high brain penetrance and long-lasting stability. J Nanobiotechnology. 2019;17:1–3.
Navarro JRG, Manchon D, Lerouge F, Blanchard NP, Marotte S, Leverrier Y, et al. Synthesis of PEGylated gold nanostars and bipyramids for intracellular uptake. Nanotechnology. 2012;23: 465602.
Moreira AF, Rodrigues CF, Reis CA, Costa EC, Correia IJ. Gold-core silica shell nanoparticles application in imaging and therapy: a review. Microporous Mesoporous Materials. 2018;270:168–79.
Mamaeva V, Sahlgren C, Lindén M. Mesoporous silica nanoparticles in medicine-recent advances. Adv Drug Deliv Rev. 2013;65(5):689–702.
Bolaños K, Kogan MJ, Araya E. Capping gold nanoparticles with albumin to improve their biomedical properties. Int J Nanomedicine. 2019;14:6387–406.
Elzoghby AO, Samy WM, Elgindy NA. Albumin-based nanoparticles as potential controlled release drug delivery systems. J Controlled Release. 2012. https://doi.org/10.1016/j.jconrel.2011.07.031 .
Matei I, Buta CM, Turcu IM, Culita D, Munteanu C, Ionita G. Formation and stabilization of gold nanoparticles in bovine serum albumin solution. Molecules. 2019;24:3395.
Amini SM, Emami T, Rashidi M, Zrrinnahad H. Curcumin-gold nanoformulation: synthesis, characterizations and biomedical application. Food Biosci. 2024;57:103446.
Mohammadi A, Colagar AH, Khorshidian A, Amini SM. The functional roles of curcumin on astrocytes in neurodegenerative diseases. NeuroImmunoModulation. 2022. https://doi.org/10.1159/000517901 .
Amini SM, Mohammadi E, Askarian-amiri S, Azizi Y, Shakeri-zadeh A, Neshastehriz A. Investigating the in vitro photothermal effect of green synthesized apigenin-coated gold nanoparticle on colorectal carcinoma. IET Nanobiotechnol. 2021. https://doi.org/10.1049/nbt2.12016 .
Hersh AM, Alomari S, Tyler BM. Crossing the blood-brain barrier: advances in nanoparticle technology for drug delivery in neuro-oncology. Int J Mol Sci. 2022;23(8):4153.
Wu D, Chen Q, Chen X, Han F, Chen Z, Wang Y. The blood–brain barrier: structure, regulation, and drug delivery. Sig Transduct Target Ther. 2023. https://doi.org/10.1038/s41392-023-01481-w .
De Bem SG, Muller AP, Machado-De-Ávila RA, Silveira PCL. Advance in the use of gold nanoparticles in the treatment of neurodegenerative diseases: new perspectives. Neural Regen Res. 2021. https://doi.org/10.4103/1673-5374.313040 .
Shukla R, Srivastava V, Sethi A, Ruwali M. Nanomaterial-based drug delivery systems as tools for targeted therapy of neurodegenerative diseases. Nanomed Drug Delivery Neurodegenerative Dis. 2022. https://doi.org/10.1016/B978-0-323-85544-0.00003-4 .
Lockman PR, Mumper RJ, Khan MA, Allen DD. Nanoparticle technology for drug delivery across the blood-brain barrier. Drug Dev Ind Pharm. 2002;28:1–13.
Kumar A, Chaudhary RK, Singh R, Singh SP, Wang SY, Hoe ZY, et al. Nanotheranostic applications for detection and targeting neurodegenerative diseases. Front Neurosci. 2020. https://doi.org/10.3389/fnins.2020.00305 .
Asha Spandana KM, Bhaskaran M, Karri VV, Natarajan J. A comprehensive review of nano drug delivery system in the treatment of CNS disorders. J Drug Deliv Sci Technol. 2020;57:101628.
Rai M, Yadav A. Nanobiotechnology in neurodegenerative diseases. Nanobiotechnol Neurodegenerative Dis. 2019. https://doi.org/10.1007/978-3-030-30930-5 .
Re F, Gregori M, Masserini M. Nanotechnology for neurodegenerative disorders. Maturitas. 2012. https://doi.org/10.1016/j.nano.2012.05.007 .
Meng J, Agrahari V, Youm I. Advances in targeted drug delivery approaches for the central nervous system tumors: the inspiration of nanobiotechnology. J Neuroimmune Pharmacol. 2017. https://doi.org/10.1007/s11481-016-9698-1 .
Velasco-Aguirre C, Morales F, Gallardo-Toledo E, Guerrero S, Giralt E, Araya E, et al. Peptides and proteins used to enhance gold nanoparticle delivery to the brain: preclinical approaches. Int J Nanomedicine. 2015;10:4919–36.
CAS PubMed PubMed Central Google Scholar
Cheng G, Liu Y, Ma R, Cheng G, Guan Y, Chen X, et al. Anti-parkinsonian therapy: strategies for crossing the blood-brain barrier and nano-biological effects of nanomaterials. Nanomicro Lett. 2022;14:105.
Khongkow M, Yata T, Boonrungsiman S, Ruktanonchai UR, Graham D, Namdee K. Surface modification of gold nanoparticles with neuron-targeted exosome for enhanced blood–brain barrier penetration. Sci Rep. 2019;9:8278.
Feng X, Chen A, Zhang Y, Wang J, Shao L, Wei L. Central nervous system toxicity of metallic nanoparticles. Int J Nanomedicine. 2015;2015:4321–40.
Flora SJS. The applications, neurotoxicity, and related mechanism of gold nanoparticles. Neurotoxicity of Nanomaterials and Nanomedicine. 2017.
Prades R, Guerrero S, Araya E, Molina C, Salas E, Zurita E, et al. Delivery of gold nanoparticles to the brain by conjugation with a peptide that recognizes the transferrin receptor. Biomaterials. 2012;33:7194–205.
Habib S, Singh M. Angiopep-2-modified nanoparticles for brain-directed delivery of therapeutics: a review. Polymers (Basel). 2022;14:712.
Formica ML, Real DA, Picchio ML, Catlin E, Donnelly RF, Paredes AJ. On a highway to the brain: a review on nose-to-brain drug delivery using nanoparticles. Appl Mater Today. 2022;29: 101631.
Agrawal M, Saraf S, Saraf S, Antimisiaris SG, Chougule MB, Shoyele SA, et al. Nose-to-brain drug delivery: an update on clinical challenges and progress towards approval of anti-Alzheimer drugs. J Controlled Release. 2018;281:139–77.
Crowe TP, Greenlee MHW, Kanthasamy AG, Hsu WH. Mechanism of intranasal drug delivery directly to the brain. Life Sci. 2018;195:44–52.
Sintov AC, Velasco-Aguirre C, Gallardo-Toledo E, Araya E, Kogan MJ. Metal nanoparticles as targeted carriers circumventing the blood-brain barrier. Int Rev Neurobiol. 2016;130:199–227.
Wang L, Tang S, Yu Y, Lv Y, Wang A, Yan X, et al. Intranasal delivery of temozolomide-conjugated gold nanoparticles functionalized with anti-EphA3 for glioblastoma targeting. Mol Pharm. 2021;18(3):915–27.
Raliya R, Saha D, Chadha TS, Raman B, Biswas P. Non-invasive aerosol delivery and transport of gold nanoparticles to the brain. Sci Rep. 2017;7(1):44718.
Ul Islam S, Shehzad A, Bilal Ahmed M, Lee YS. Intranasal delivery of nanoformulations: a potential way of treatment for neurological disorders. Molecules. 2020;7(1):44718.
Gallardo-Toledo E, Tapia-Arellano A, Celis F, Sinai T, Campos M, Kogan MJ, et al. Intranasal administration of gold nanoparticles designed to target the central nervous system: fabrication and comparison between nanospheres and nanoprisms. Int J Pharm. 2020;590:119957.
Han S, Wang JTW, Yavuz E, Zam A, Rouatbi N, Utami RN, et al. Spatiotemporal tracking of gold nanorods after intranasal administration for brain targeting. J Controlled Release. 2023. https://doi.org/10.1016/j.jconrel.2023.04.022 .
Casettari L, Illum L. Chitosan in nasal delivery systems for therapeutic drugs. Jof Controlled Release. 2014;190:189–200.
Bhumkar DR, Joshi HM, Sastry M, Pokharkar VB. Chitosan reduced gold nanoparticles as novel carriers for transmucosal delivery of insulin. Pharm Res. 2007;24:1415–26.
Pietrzak K, Czarnecka K, Mikiciuk-Olasik E, Szymanski P. New perspectives of Alzheimer disease diagnosis—the most popular and future methods. Med Chem (Los Angeles). 2018;14:34–43.
Fayyad M, Salim S, Majbour N, Erskine D, Stoops E, Mollenhauer B, et al. Parkinson’s disease biomarkers based on α-synuclein. J Neurochem. 2019. https://doi.org/10.1111/jnc.14809 .
Li T, Le W. Biomarkers for Parkinson’s disease: how good are they? Neurosci Bull. 2020;36(2):183–94.
Lee HJ, Bae EJ, Lee SJ. Extracellular α-synuclein-a novel and crucial factor in Lewy body diseases. Nat Rev Neurol. 2014;10:92–8.
Lashuel HA, Overk CR, Oueslati A, Masliah E. The many faces of α-synuclein: from structure and toxicity to therapeutic target. Nat Rev Neurosci. 2013;14:38–48.
Wong YC, Krainc D. α-synuclein toxicity in neurodegeneration: Mechanism and therapeutic strategies. Nat Med. 2017;23(2):1–3.
Leuzy A, Chiotis K, Lemoine L, Gillberg PG, Almkvist O, Rodriguez-Vieitez E, et al. Tau PET imaging in neurodegenerative tauopathies—still a challenge. Mol Psychiatry. 2019;24(8):1112–34.
Cassinelli Petersen G, Roytman M, Chiang GC, Li Y, Gordon ML, Franceschi AM. Overview of tau PET molecular imaging. Curr Opin Neurol. 2022;35(2):230–9.
Paciotti S, Bellomo G, Gatticchi L, Parnetti L. Are we ready for detecting α-synuclein prone to aggregation in patients? The case of “Protein-Misfolding Cyclic Amplification” and “Real-Time Quaking-Induced Conversion” as diagnostic tools. Front Neurol. 2018;9:376477.
Shahnawaz M, Tokuda T, Waraga M, Mendez N, Ishii R, Trenkwalder C, et al. Development of a biochemical diagnosis of Parkinson disease by detection of α-synuclein misfolded aggregates in cerebrospinal fluid. JAMA Neurol. 2017;74(2):163–72.
An Y, Tang L, Jiang X, Chen H, Yang M, Jin L, et al. A photoelectrochemical immunosensor based on au-doped TiO2 nanotube arrays for the detection of α-synuclein. Chemistry. 2010;16:14439–46.
Zhang R, Wang S, Huang X, Yang Y, Fan H, Yang F, et al. Gold-nanourchin seeded single-walled carbon nanotube on voltammetry sensor for diagnosing neurogenerative Parkinson’s disease. Anal Chim Acta. 2020;1094:142–50.
An Y, Jiang X, Bi W, Chen H, Jin L, Zhang S, et al. Sensitive electrochemical immunosensor for α-synuclein based on dual signal amplification using PAMAM dendrimer-encapsulated Au and enhanced gold nanoparticle labels. Biosens Bioelectron. 2012;32:224–30.
Aminabad ED, Mobed A, Hasanzadeh M, Hosseinpour Feizi MA, Safaralizadeh R, Seidi F. Sensitive immunosensing of α-synuclein protein in human plasma samples using gold nanoparticles conjugated with graphene: an innovative immuno-platform towards early stage identification of Parkinson’s disease using point of care (POC) analysis. RSC Adv. 2022;12:4346–57. https://doi.org/10.1039/D1RA06437A .
Sonuç Karaboğa MN, Sezgintürk MK. Cerebrospinal fluid levels of alpha-synuclein measured using a poly-glutamic acid-modified gold nanoparticle-doped disposable neuro-biosensor system. Analyst. 2019;144:611–21. https://doi.org/10.1039/C8AN01279B .
Liu CC, Kanekiyo T, Xu H, Bu G. Apolipoprotein E and Alzheimer disease: risk, mechanisms and therapy. Nat Rev Neurol. 2013;9:106–18.
Zoey FLG, Palanivel M, Padmanabhan P, Gulyás B. Parkinson’s disease: a nanotheranostic approach targeting alpha-synuclein aggregation. Front Cell Dev Biol. 2021. https://doi.org/10.3389/fcell.2021.707441 .
Wu Q, Tan R, Mi X, Tu Y. Electrochemiluminescent aptamer-sensor for alpha synuclein oligomer based on a metal–organic framework. Analyst. 2020;145:2159–67. https://doi.org/10.1039/D0AN00169D .
Giasson BI, Duda JE, Murray IVJ, Chen Q, Souza JM, Hurtig HI, et al. Oxidative damage linked to neurodegeneration by selective α-synuclein nitration in synucleinopathy lesions. Science. 2000;290:985–9. https://doi.org/10.1126/science.290.5493.985 .
Yu Z, Xu X, Xiang Z, Zhou J, Zhang Z, Hu C, et al. Nitrated α-synuclein induces the loss of dopaminergic neurons in the substantia nigra of rats. PLoS ONE. 2010;5:e9956. https://doi.org/10.1371/journal.pone.0009956 .
Kovacs GG, Botond G, Budka H. Protein coding of neurodegenerative dementias: The neuropathological basis of biomarker diagnostics. Acta Neuropathol. 2010;119:389–408.
Zhang ZH, Hu J, Chen Q, Chen J, Hu X, Koh K, et al. The magnetic-nanoparticle-assisted sensitive detection of nitrated α-syn in blood based on a sensitizing electrochemical layer. Nanoscale. 2021;13:8107–17.
Neely A, Perry C, Varisli B, Singh AK, Arbneshi T, Senapati D, et al. Ultrasensitive and highly selective detection of alzheimer’s disease biomarker using two-photon rayleigh scattering properties of gold nanoparticle. ACS Nano. 2009. https://doi.org/10.1021/nn900813b .
Kim H, Lee JU, Kim S, Song S, Sim SJ. A nanoplasmonic biosensor for ultrasensitive detection of Alzheimer’s disease biomarker using a chaotropic agent. ACS Sens. 2019;4:595–602.
Khatri A, Punjabi N, Ghosh D, Maji SK, Mukherji S. Detection and differentiation of α-Synuclein monomer and fibril by chitosan film coated nanogold array on optical sensor platform. Sens Actuators B Chem. 2018;255:692–700.
Kumar J, Eraña H, López-Martínez E, Claes N, Martín VF, Solís DM, et al. Detection of amyloid fibrils in Parkinson’s disease using plasmonic chirality. Proc Natl Acad Sci. 2018;115:3225–30.
You X, Gopinath SCB, Lakshmipriya T, Li D. High-affinity detection of alpha-synuclein by aptamer-gold conjugates on an amine-modified dielectric surface Buccolieri A, editor. J Anal Methods Chem. 2019;2019:6526850.
Yao T, Wang R, Meng Y, Hun X. Photoelectrochemical sensing of α-synuclein based on a AuNPs/graphdiyne-modified electrode coupled with a nanoprobe. ACS Appl Mater Interfaces. 2021;13:26515–21.
Hun X, Kong X. An enzyme linked aptamer photoelectrochemical biosensor for Tau-381 protein using AuNPs/MoSe2 as sensing material. J Pharm Biomed Anal. 2021;192: 113666.
Razzino CA, Serafín V, Gamella M, Pedrero M, Montero-Calle A, Barderas R, et al. An electrochemical immunosensor using gold nanoparticles-PAMAM-nanostructured screen-printed carbon electrodes for tau protein determination in plasma and brain tissues from Alzheimer patients. Biosens Bioelectron. 2020;163:112238.
Sonuç Karaboga MN, Sezgintürk MK. Analysis of Tau-441 protein in clinical samples using rGO/AuNP nanocomposite-supported disposable impedimetric neuro-biosensing platform: towards Alzheimer’s disease detection. Talanta. 2020;219:121257.
Stegurová L, Dráberová E, Bartos A, Dráber P, Řípová D, Dráber P. Gold nanoparticle-based immuno-PCR for detection of tau protein in cerebrospinal fluid. J Immunol Methods. 2014;406:137–42.
Yang SJ, Lee JU, Jeon MJ, Sim SJ. Highly sensitive surface-enhanced Raman scattering-based immunosensor incorporating half antibody-fragment for quantitative detection of Alzheimer’s disease biomarker in blood. Anal Chim Acta. 2022;1195: 339445.
Zhang X, Liu S, Song X, Wang H, Wang J, Wang Y, et al. Robust and universal sers sensing platform for multiplexed detection of Alzheimer’s disease core biomarkers using PAapt-AuNPs conjugates. ACS Sens. 2019;4:2140–9.
Serafín V, Razzino CA, Gamella M, Pedrero M, Povedano E, Montero-Calle A, et al. Disposable immunoplatforms for the simultaneous determination of biomarkers for neurodegenerative disorders using poly(amidoamine) dendrimer/gold nanoparticle nanocomposite. Anal Bioanal Chem. 2021;413:799–811.
Kim H, Lee JU, Song S, Kim S, Sim SJ. A shape-code nanoplasmonic biosensor for multiplex detection of Alzheimer’s disease biomarkers. Biosens Bioelectron. 2018;101:96–102.
Vestergaard M, Kerman K, Kim DK, Hiep HM, Tamiya E. Detection of Alzheimer’s tau protein using localised surface plasmon resonance-based immunochip. Talanta. 2008;74(4):1038–42.
Tao D, Shui B, Gu Y, Cheng J, Zhang W, Jaffrezic-Renault N, et al. Development of a label-free electrochemical aptasensor for the detection of Tau381 and its preliminary application in AD and non-AD patients’ sera. Biosensors (Basel). 2019;9:84.
Zengin A, Tamer U, Caykara T. A SERS-based sandwich assay for ultrasensitive and selective detection of Alzheimer’s tau protein. Biomacromol. 2013;14:3001–9.
Sinha SS, Jones S, Pramanik A, Ray PC. Nanoarchitecture based SERS for biomolecular fingerprinting and label-free disease markers diagnosis. Acc Chem Res. 2016;49:2725–35.
Maurer V, Frank C, Porsiel JC, Zellmer S, Garnweitner G, Stosch R. Step-by-step monitoring of a magnetic and SERS-active immunosensor assembly for purification and detection of tau protein. J Biophotonics. 2020;13:1–10.
Shui B, Tao D, Cheng J, Mei Y, Jaffrezic-Renault N, Guo Z. A novel electrochemical aptamer-antibody sandwich assay for the detection of tau-381 in human serum. Analyst. 2018;143:3549–54.
Li X, Jiang M, Cheng J, Ye M, Zhang W, Jaffrezic-Renault N, et al. Signal multi-amplified electrochemical biosensor for voltammetric determination of tau-441 protein in biological samples using carbon nanomaterials and gold nanoparticles to hint dementia. Microchim Acta. 2020;187:1–8.
Lu X, Hou X, Tang H, Yi X, Wang J. A high-quality CdSe/CdS/ZnS quantum-dot-based FRET aptasensor for the simultaneous detection of two different alzheimer’s disease core biomarkers. Nanomaterials. 2022;12:4031.
Zhang L, Cao K, Su Y, Hu S, Liang X, Luo Q, et al. Colorimetric and surface-enhanced Raman scattering dual-mode magnetic immunosensor for ultrasensitive detection of blood phosphorylated tau in Alzheimer’s disease. Biosens Bioelectron. 2023;222: 114935.
Liu L, Li M, Xu M, Wang Z, Zeng Z, Li Y, et al. Actively targeted gold nanoparticle composites improve behavior and cognitive impairment in Parkinson’s disease mice. Mater Sci Eng C. 2020;114:111028.
Iulita MF, Bistué Millón MB, Pentz R, Aguilar LF, Do Carmo S, Allard S, et al. Differential deregulation of NGF and BDNF neurotrophins in a transgenic rat model of Alzheimer’s disease. Neurobiol Dis. 2017;108:307–23.
Hu K, Chen X, Chen W, Zhang L, Li J, Ye J, et al. Neuroprotective effect of gold nanoparticles composites in Parkinson’s disease model. Nanomedicine. 2018;14:1123–36.
Gao G, Chen R, He M, Li J, Wang L, Sun T. Gold nanoclusters for Parkinson’s disease treatment. Biomaterials. 2019;194:36–46.
Sonawane SK, Ahmad A, Chinnathambi S. Protein-capped metal nanoparticles inhibit tau aggregation in Alzheimer’s disease. ACS Omega. 2019;4:12833–40.
Vimal SK, Zuo H, Wang Z, Wang H, Long Z, Bhattacharyya S. Self-therapeutic nanoparticle that alters tau protein and ameliorates tauopathy toward a functional nanomedicine to tackle Alzheimer’s. Small. 2020;16(16):1906861.
Bhattacharyya S, Kim K, Teizer W. Remodeling tau and prion proteins using nanochaperons. Adv Biosyst. 2017;1:1700108.
Javed I, Peng G, Xing Y, Yu T, Zhao M, Kakinen A, et al. Inhibition of amyloid beta toxicity in zebrafish with a chaperone-gold nanoparticle dual strategy. Nat Commun. 2019;10:3780.
Martins PAT, Alsaiari S, Julfakyan K, Nie Z, Khashab NM. Self-assembled lipoprotein based gold nanoparticles for detection and photothermal disaggregation of β-amyloid aggregates. Chem Commun. 2017;53(13):2102–5.
Wiesehan K, Willbold D. Mirror-image phage display: aiming at the mirror. ChemBioChem. 2003;4:811–5.
Wiesehan K, Buder K, Linke RP, Patt S, Stoldt M, Unger E, et al. Selection of D-amino-acid peptides that bind to Alzheimer’s disease amyloid peptide Aβ1–42 by mirror image phage display. ChemBioChem. 2003;4:748–53.
Wiesehan K, Stöhr J, Nagel-Steger L, Van Groen T, Riesner D, Willbold D. Inhibition of cytotoxicity and amyloid fibril formation by a D-amino acid peptide that specifically binds to Alzheimer’s disease amyloid peptide. Protein Eng Design Select. 2008;21:241–6.
Van Groen T, Kadish I, Wiesehan K, Funke SA, Willbold D. In vitro and in vivo staining characteristics of small, fluorescent, Aβ42-binding D-enantiomeric peptides in transgenic AD mouse models. ChemMedChem. 2009;4:276–82.
Van Groen T, Wiesehan K, Funke SA, Kadish I, Nagel-Steger L, Willbold D. Reduction of Alzheimer’s disease amyloid plaque load in transgenic mice by D3, a D-enantiomeric peptide identified by mirror image phage display. ChemMedChem. 2008;3:1848–52.
Liu H, Funke SA, Willbold D. Transport of alzheimer disease amyloid-β-binding d-amino acid peptides across an in vitro blood-brain barrier model. Rejuvenation Res. 2010;13:210–3.
Van Groen T, Kadish I, Funke A, Bartnik D, Willbold D. Treatment with Aβ42 binding d-amino acid peptides reduce amyloid deposition and inflammation in APP/PS1 double transgenic mice. Adv Protein Chem Struct Biol. 2012;88:133–52.
Van Groen T, Kadish I, Funke SA, Bartnik D, Willbold D. Treatment with D3 removes amyloid deposits, reduces inflammation, and improves cognition in aged AβPP/PS1 double transgenic mice. JAD. 2013;34:609–20.
Jiang N, Leithold LHE, Post J, Ziehm T, Mauler J, Gremer L, et al. Preclinical pharmacokinetic studies of the tritium labelled D-enantiomeric peptide D3 developed for the treatment of Alzheimer’s disease. PLoS ONE. 2015;10:e0128553.
Bartnik D, Funke SA, Andrei-Selmer LC, Bacher M, Dodel R, Willbold D. Differently selected d-enantiomeric peptides Act on different aβ species. Rejuvenation Res. 2010;13:202–5.
Aileen Funke S, Van Groen T, Kadish I, Bartnik D, Nagel-Steger L, Brener O, et al. Oral treatment with the d-enantiomeric peptide D3 improves the pathology and behavior of alzheimer’s disease transgenic mice. ACS Chem Neurosci. 2010;1:639–48.
Morales-Zavala F, Jara-Guajardo P, Chamorro D, Riveros AL, Chandia-Cristi A, Salgado N, et al. In vivo micro computed tomography detection and decrease in amyloid load by using multifunctionalized gold nanorods: a neurotheranostic platform for Alzheimer’s disease. Biomater Sci. 2021;9:4178–90.
Altendorf T, Gering I, Santiago-Schübel B, Aghabashlou Saisan S, Tamgüney G, Tusche M, et al. Stabilization of monomeric tau protein by All D-enantiomeric peptide ligands as therapeutic strategy for alzheimer’s disease and other tauopathies. Int J Mol Sci. 2023;24(3):2161.
Aillaud I, Funke SA. Tau aggregation inhibiting peptides as potential therapeutics for Alzheimer disease. Cell Mol Neurobiol. 2023;43(3):951–61.
Aillaud I, Kaniyappan S, Chandupatla RR, Ramirez LM, Alkhashrom S, Eichler J, et al. A novel D-amino acid peptide with therapeutic potential (ISAD1) inhibits aggregation of neurotoxic disease-relevant mutant Tau and prevents Tau toxicity in vitro. Alzheimers Res Ther. 2022;14(1):15.
Dammers C, Yolcu D, Kukuk L, Willbold D, Pickhardt M, Mandelkow E, et al. Selection and characterization of tau binding D-enantiomeric peptides with potential for therapy of Alzheimer disease. PLoS ONE. 2016;11:e0167432.
Malhis M, Kaniyappan S, Aillaud I, Chandupatla RR, Ramirez LM, Zweckstetter M, et al. Potent tau aggregation inhibitor D-peptides selected against tau-repeat 2 using mirror image phage display. ChemBioChem. 2021;22(21):3049–59.
Shaltiel-Karyo R, Frenkel-Pinter M, Egoz-Matia N, Frydman-Marom A, Shalev DE, Segal D, et al. Inhibiting α-synuclein oligomerization by stable cell-penetrating β-synuclein fragments recovers phenotype of parkinson’s disease model flies. PLoS ONE. 2010;5(11):e13863.
Chemerovski-Glikman M, Rozentur-Shkop E, Richman M, Grupi A, Getler A, Cohen HY, et al. Self-assembled cyclic d, l-α-peptides as generic conformational inhibitors of the α-synuclein aggregation and toxicity: in vitro and mechanistic studies. Chem A Eur J. 2016. https://doi.org/10.1002/chem.201601830 .
Horsley JR, Jovcevski B, Pukala TL, Abell AD. Designer D-peptides targeting the N-terminal region of α-synuclein to prevent parkinsonian-associated fibrilization and cytotoxicity. Biochim Biophys Acta Proteins Proteom. 2022;1870:140826.
Ghalandari B, Asadollahi K, Shakerizadeh A, Komeili A, Riazi G, Kamrava SK, et al. Microtubule network as a potential candidate for targeting by gold nanoparticle-assisted photothermal therapy. J Photochem Photobiol B. 2019. https://doi.org/10.1016/j.jphotobiol.2019.01.012 .
Goto T, Kasai N, Filip R, Sumitomo K, Nakashima H. Observation of intracellular protein localization area in a single neuron using gold nanoparticles with a scanning electron microscope. Micron. 2019;126:102740.
Cheng CH, Lin KJ, Hong CT, Wu D, Chang HM, Liu CH, et al. Plasmon-activated water reduces amyloid burden and improves memory in animals with Alzheimer’s disease. Sci Rep. 2019. https://doi.org/10.1038/s41598-019-49731-8 .
Yao L, Bojic D, Liu M. Applications and safety of gold nanoparticles as therapeutic devices in clinical trials. J Pharm Anal. 2023.
Zhang R, Kiessling F, Lammers T, Pallares RM. Clinical translation of gold nanoparticles. Drug Deliv Transl Res. 2023;13(2):378–85.
Sung D, Sanchez A, Tward JD. Successful salvage brachytherapy after infusion of gold auroshell nanoshells for localized prostate cancer in a human patient. Adv Radiat Oncol. 2023;8(4):101202.
Balfourier A, Kolosnjaj-Tabi J, Luciani N, Carn F, Gazeau F, Murphy CJ. Gold-based therapy: from past to present. Proc Natl Acad Sci U S A. 2020;117(37):22639–48.
Jensen SA, Day ES, Ko CH, Hurley LA, Luciano JP, Kouri FM, et al. Spherical nucleic acid nanoparticle conjugates as an RNAi-based therapy for glioblastoma. Sci Transl Med. 2013;5(209):209ra152.
Kumthekar P, Ko CH, Paunesku T, Dixit K, Sonabend AM, Bloch O, et al. A first-in-human phase 0 clinical study of RNA interference-based spherical nucleic acids in patients with recurrent glioblastoma. Sci Transl Med. 2021. https://doi.org/10.1126/scitranslmed.abb3945 .
Duan L, Li X, Ji R, Hao Z, Kong M, Wen X, et al. Nanoparticle-based drug delivery systems: an inspiring therapeutic strategy for neurodegenerative diseases. Polymers (Basel). 2023. https://doi.org/10.3390/polym15092196 .
Olaru DG, Olaru A, Kassem GH, Popescu-Drigă MV, Pinoşanu LR, Dumitraşcu DI, et al. Toxicity and health impact of nanoparticles. Basic biology and clinical perspective. Roman J Morphol Embryol. 2019;60:787–92.
Su S, Kang PM. Systemic review of biodegradable nanomaterials in nanomedicine. Nanomaterials. 2020;10:656.
P. Rajput A, Kulkarni M, Pingale PL, Tekade M, Shakya AK, Tekade RK. Understanding the bioaccumulation of pharmaceuticals and personal care products. Essentials of Pharmatoxicology in Drug Research: Toxicity and Toxicodynamics: Volume 1. 2023. https://doi.org/10.1016/B978-0-443-15840-7.00024-5
Jaiswal S, Manhas A, Pandey AK, Priya S, Sharma SK. Engineered nanoparticle-protein interactions influence protein structural integrity and biological significance. Nanomaterials. 2022;12(7):1214.
Kim Y, Park JH, Lee H, Nam JM. How do the size, charge and shape of nanoparticles affect amyloid β aggregation on brain lipid bilayer? Sci Rep. 2016;6:19548.
Sanati M, Khodagholi F, Aminyavari S, Ghasemi F, Gholami M, Kebriaeezadeh A, et al. Impact of gold nanoparticles on amyloid β-induced alzheimer’s disease in a rat animal model: involvement of STIM proteins. ACS Chem Neurosci. 2019;10:2299–309.
Tapia-Arellano A, Gallardo-Toledo E, Celis F, Rivera R, Moglia I, Campos M, et al. The curvature of gold nanoparticles influences the exposure of amyloid-β and modulates its aggregation process. Mater Sci Eng C. 2021;128: 112269.
Brancolini G, Toroz D, Corni S. Can small hydrophobic gold nanoparticles inhibit β2- microglobulin fibrillation? Nanoscale. 2014;6(14):7903–11.
Cantarutti C, Raimondi S, Brancolini G, Corazza A, Giorgetti S, Ballico M, et al. Citrate-stabilized gold nanoparticles hinder fibrillogenesis of a pathological variant of β2-microglobulin. Nanoscale. 2017;9(11):3941–51.
Cantarutti C, Raj G, Fogolari F, Giorgetti S, Corazza A, Bellotti V, et al. Interference of citrate-stabilized gold nanoparticles with β2-microglobulin oligomeric association. Chem Commun. 2018;54(43):5422–5.
Arosio P, Vendruscolo M, Dobson CM, Knowles TP. Chemical kinetics for drug discovery to combat protein aggregation diseases. Trends Pharmacol Sci. 2014;35:127–35.
Arosio P, Knowles TP, Linse S. On the lag phase in amyloid fibril formation. Phys Chem Chem Phys. 2015;17:7606–18.
Arosio P, Michaels TC, Linse S, Månsson C, Emanuelsson C, Presto J, et al. Kinetic analysis reveals the diversity of microscopic mechanisms through which molecular chaperones suppress amyloid formation. Nat Commun. 2016;7:10948.
Álvarez YD, Fauerbach JA, Pellegrotti JV, Jovin TM, Jares-Erijman EA, Stefani FD. Influence of gold nanoparticles on the kinetics of α-synuclein aggregation. Nano Lett. 2013;13:6156–63.
Bera K, Mondal A, Pal U, Maiti NC. Porphyrin-armored gold nanospheres modulate the secondary structure of α-synuclein and arrest its fibrillation. J Phys Chem C. 2020;124:6418–34.
Maity A, Mondal A, Kundu S, Shome G, Misra R, Singh A, et al. Naringenin-functionalized gold nanoparticles and their role in α-synuclein stabilization. Langmuir. 2023;39:7231–48. https://doi.org/10.1021/acs.langmuir.2c03259 .
Gharb M, Nouralishahi A, Riazi A, Riazi G. Inhibition of tau protein aggregation by a chaperone-like β-boswellic acid conjugated to gold nanoparticles. ACS Omega. 2022;7:30347–58.
Yang G, Phua SZF, Bindra AK, Zhao Y. Degradability and clearance of inorganic nanoparticles for biomedical applications. Adv Mater. 2019;31(10):1805730.
van de Looij SM, Hebels ER, Viola M, Hembury M, Oliveira S, Vermonden T. Gold nanoclusters: imaging, therapy, and theranostic roles in biomedical applications. Bioconjug Chem. 2022;33(1):4–23.
Mahapatra A, Sarkar S, Biswas SC, Chattopadhyay K. Modulation of α-synuclein fibrillation by ultrasmall and biocompatible gold nanoclusters. ACS Chem Neurosci. 2020;11(20):3442–54.
Sivanesan S, Rajeshkumar S. Gold nanoparticles in diagnosis and treatment of Alzheimer’s disease. Nanobiotechnol Neurodegenerative Dis. 2019.
Yang JA, Johnson BJ, Wu S, Woods WS, George JM, Murphy CJ. Study of wild-type α-synuclein binding and orientation on gold nanoparticles. Langmuir. 2013;29:4603–15.
Yang JA, Lin W, Woods WS, George JM, Murphy CJ. α-Synuclein’s adsorption, conformation, and orientation on cationic gold nanoparticle surfaces seeds global conformation change. J Phys Chem B. 2014;118:3559–71.
Lin W, Insley T, Tuttle MD, Zhu L, Berthold DA, Král P, et al. Control of protein orientation on gold nanoparticles. J Phys Chem C. 2015;119:21035–43.
Vinod C, Jena S. Nano-neurotheranostics: impact of nanoparticles on neural dysfunctions and strategies to reduce toxicity for improved efficacy. Front Pharmacol. 2021;12:612692.
Báez DF, Gallardo-Toledo E, Oyarzún MP, Araya E, Kogan MJ. The influence of size and chemical composition of silver and gold nanoparticles on in vivo toxicity with potential applications to central nervous system diseases. Int J Nanomedicine. 2021;16:2187–201.
Sun H, Jia J, Jiang C, Zhai S. Gold nanoparticle-induced cell death and potential applications in nanomedicine. Int J Mol Sci. 2018;19(3):754.
Bencsik A, Lestaevel P, Guseva CI. Nano- and neurotoxicology: an emerging discipline. Prog Neurobiol. 2018;160:45–63.
Teleanu DM, Chircov C, Grumezescu AM, Volceanov A, Teleanu RI. Impact of nanoparticles on brain health: an up to date overview. J Clin Med. 2018;7:490.
Cardoso E, Rezin GT, Zanoni ET, de Souza NF, Leffa DD, Damiani AP, et al. Acute and chronic administration of gold nanoparticles cause DNA damage in the cerebral cortex of adult rats. Mutat Res. 2014;766:25–30.
Lasagna-Reeves C, Gonzalez-Romero D, Barria MA, Olmedo I, Clos A, Sadagopa Ramanujam VM, et al. Bioaccumulation and toxicity of gold nanoparticles after repeated administration in mice. Biochem Biophys Res Commun. 2010;393:649–55.
Chen YS, Hung YC, Lin LW, Liau I, Hong MY, Huang GS. Size-dependent impairment of cognition in mice caused by the injection of gold nanoparticles. Nanotechnology. 2010;21:485102.
Noor NA, Fahmy HM, Mourad IM. Evaluation of the potential neurotoxicity of gold nanoparticles in the different rat brain regions. IJSBAR. 2016;30:114–29.
Siddiqi NJ, Abdelhalim MAK, El-Ansary AK, Alhomida AS, Ong WY. Identification of potential biomarkers of gold nanoparticle toxicity in rat brains. J Neuroinflammation. 2012. https://doi.org/10.1186/1742-2094-9-123 .
Lee U, Yoo CJ, Kim YJ, Yoo YM. Cytotoxicity of gold nanoparticles in human neural precursor cells and rat cerebral cortex. J Biosci Bioeng. 2016. https://doi.org/10.1016/j.jbiosc.2015.07.004 .
Yoo C-J, Lee U, Kim Y-J, Park J, Yoo Y-M. Dose-dependent cytotoxicity of gold nanoparticles on human neural progenitor cells and rat brain. J Nanosci Nanotechnol. 2019. https://doi.org/10.1166/jnn.2019.16547 .
Pannerselvam B, Devanathadesikan V, Alagumuthu TS, Kanth SV, Thangavelu KP. Assessment of in-vivo biocompatibility evaluation of phytogenic gold nanoparticles on Wistar albino male rats. IET Nanobiotechnol. 2020. https://doi.org/10.1049/iet-nbt.2019.0116 .
Jung S, Bang M, Kim BS, Lee S, Kotov NA, Kim B, et al. Intracellular gold nanoparticles increase neuronal excitability and aggravate seizure activity in the mouse brain. PLoS ONE. 2014;9:e91360.
Salinas K, Kereselidze Z, DeLuna F, Peralta XG, Santamaria F. Transient extracellular application of gold nanostars increases hippocampal neuronal activity. J Nanobiotechnology. 2014;12:1–7.
Stojiljković A, Kuehni-Boghenbor K, Gaschen V, Schüpbach G, Mevissen M, Kinnear C, et al. High-content analysis of factors affecting gold nanoparticle uptake by neuronal and microglial cells in culture. Nanoscale. 2016;8:16650–61.
Xiao L, Wei F, Zhou Y, Anderson GJ, Frazer DM, Lim YC, et al. Dihydrolipoic acid-gold nanoclusters regulate microglial polarization and have the potential to alter neurogenesis. Nano Lett. 2020;20(1):478–95.
Yuan Q, Yao Y, Zhang X, Yuan J, Sun B, Gao X. The gold nanocluster protects neurons directly or via inhibiting cytotoxic secretions of microglia cell. J Nanosci Nanotechnol. 2018;19(4):1986–95.
Leite PEC, Pereira MR, Harris G, Pamies D, Dos Santos LMG, Granjeiro JM, et al. Suitability of 3D human brain spheroid models to distinguish toxic effects of gold and poly-lactic acid nanoparticles to assess biocompatibility for brain drug delivery. Part Fibre Toxicol. 2019;16:1–20.
Imperatore R, Carotenuto G, Di Grazia MA, Ferrandino I, Palomba L, Mariotti R, et al. Imidazole-stabilized gold nanoparticles induce neuronal apoptosis: an in vitro and in vivo study. J Biomed Mater Res A. 2015;103(4):1436–46.
Manson J, Kumar D, Meenan BJ, Dixon D. Polyethylene glycol functionalized gold nanoparticles: the influence of capping density on stability in various media. Gold Bull. 2011;44:99–105. https://doi.org/10.1007/s13404-011-0015-8 .
Zhang X, Guo X, Kang X, Yang H, Guo W, Guan L, et al. Surface functionalization of pegylated gold nanoparticles with antioxidants suppresses nanoparticle-induced oxidative stress and neurotoxicity. Chem Res Toxicol. 2020;33(5):1195–205.
Pereira MC, Adewale OB, Roux S, Cairncross L, Davids H. Biochemical assessment of the neurotoxicity of gold nanoparticles functionalized with colorectal cancer-targeting peptides in a rat model. Hum Exp Toxicol. 2021;40(11):1962–73.
Ji J, Moquin A, Bertorelle F, Chang P, Antoine R, Luo J, et al. Organotypic and primary neural cultures as models to assess effects of different gold nanostructures on glia and neurons. Nanotoxicology. 2019;13(3):285–304.
Tuna BG, Yesilay G, Yavuz Y, Yilmaz B, Culha M, Maharramov A, et al. Electrophysiological effects of polyethylene glycol modified gold nanoparticles on mouse hippocampal neurons. Heliyon. 2020;6:e05824.
Xue J, Liu T, Liu Y, Jiang Y, Seshadri VDD, Mohan SK, et al. Neuroprotective effect of biosynthesised gold nanoparticles synthesised from root extract of Paeonia moutan against Parkinson disease—in vitro & in vivo model. J Photochem Photobiol B. 2019;200:111635.
Sancho L, Contreras M, Allen NJ. Glia as sculptors of synaptic plasticity. Neurosci Res. 2021;167:17–29.
Allen NJ, Lyons DA. Glia as architects of central nervous system formation and function. Science. 2018;362(6411):181–5.
Mytych J, Lewinska A, Zebrowski J, Wnuk M. Gold nanoparticles promote oxidant-mediated activation of NF-κB and 53BP1 recruitment-based adaptive response in human astrocytes. Biomed Res Int. 2015. https://doi.org/10.1155/2015/304575 .
Kofuji P, Araque A. Astrocytes and behavior. Annu Rev Neurosci. 2021;44:49–67.
Park SY, Yi EH, Kim Y, Park G. Anti-neuroinflammatory effects of ephedra sinica stapf extract-capped gold nanoparticles in microglia. Int J Nanomedicine. 2019;14:2861–77.
Hutter E, Boridy S, Labrecque S, Lalancette-Hébert M, Kriz J, Winnik FM, et al. Microglial response to gold nanoparticles. ACS Nano. 2010;4:2595–606.
Lira-Diaz E, Gonzalez-Pedroza MG, Vasquez C, Morales-Luckie RA, Gonzalez-Perez O. Gold nanoparticles produce transient reactive gliosis in the adult brain. Neurosci Res. 2021;170:76–86.
Gran ER, Bertorelle F, Fakhouri H, Antoine R, Perić Bakulić M, Sanader Maršić Ž, et al. Size and ligand effects of gold nanoclusters in alteration of organellar state and translocation of transcription factors in human primary astrocytes. Nanoscale. 2021;13:3173–83.
Maysinger D, Sanader Maršić Ž, Gran ER, Shobo A, MacAiran JR, Zhang I, et al. Insights into the impact of gold nanoclusters Au10SG10on human microglia. ACS Chem Neurosci. 2022;13:464–76.
Sobska J, Waszkielewicz M, Podleśny-Drabiniok A, Olesiak-Banska J, Krężel W, Matczyszyn K. Gold nanoclusters display low immunogenic effect in microglia cells. Nanomaterials. 2021;11:1066.
Weiss ACG, Kempe K, Förster S, Caruso F. Microfluidic examination of the “hard” biomolecular corona formed on engineered particles in different biological milieu. Biomacromol. 2018;19:2580–94. https://doi.org/10.1021/acs.biomac.8b00196 .
Palchetti S, Pozzi D, Capriotti AL, BarberaLa G, Chiozzi RZ, Digiacomo L, et al. Influence of dynamic flow environment on nanoparticle-protein corona: from protein patterns to uptake in cancer cells. Colloids Surf B Biointerfaces. 2017;153:263–71.
Braun NJ, DeBrosse MC, Hussain SM, Comfort KK. Modification of the protein corona–nanoparticle complex by physiological factors. Mater Sci Eng C. 2016;64:34–42.
Palchetti S, Colapicchioni V, Digiacomo L, Caracciolo G, Pozzi D, Capriotti AL, et al. The protein corona of circulating PEGylated liposomes. Biochimica et Biophysica Acta (BBA) Biomembranes. 2016;1858:189–96.
Palma-Florez S, López-Canosa A, Moralez-Zavala F, Castaño O, Kogan MJ, Samitier J, et al. BBB-on-a-chip with integrated micro-TEER for permeability evaluation of multi-functionalized gold nanorods against Alzheimer’s disease. J Nanobiotechnology. 2023;21:115.
Fan Y, Xu C, Deng N, Gao Z, Jiang Z, Li X, et al. Understanding drug nanocarrier and blood-brain barrier interaction based on a microfluidic microphysiological model. Lab Chip. 2023;23:1935–44.
Ziółkowska K, Kwapiszewski R, Brzózka Z. Microfluidic devices as tools for mimicking the in vivo environment. New J Chem. 2011;35(5):979–90.
Valencia PM, Farokhzad OC, Karnik R, Langer R. Microfluidic technologies for accelerating the clinical translation of nanoparticles. Nat Nanotechnol. 2012;7:93–112.
Whitesides GM. The origins and the future of microfluidics. Nature. 2006;442:368–73.
Shepherd SJ, Issadore D, Mitchell MJ. Microfluidic formulation of nanoparticles for biomedical applications. Biomaterials. 2021;274: 120826.
Alépée N, Bahinski A, Daneshian M, De Wever B, Fritsche E, Goldberg A, et al. State-of-the-art of 3D cultures (organs-on-a-chip) in safety testing and pathophysiology. Altex. 2014. https://doi.org/10.14573/altex.1406111 .
Prasanna P, Rathee S, Rahul V, Mandal D, Goud MSC, Yadav P, et al. Microfluidic platforms to unravel mysteries of Alzheimer’s disease: How far have we come? Life. 2021;11:1022.
Pamies D, Hartung T, Hogberg HT. Biological and medical applications of a brain-on-a-chip. Exp Biol Med. 2014;239:1096–107.
Hartung T. 3D—a new dimension of in vitro research. Adv Drug Deliv Rev. 2014;69:vi.
Sokolova V, Nzou G, van der Meer SB, Ruks T, Heggen M, Loza K, et al. Ultrasmall gold nanoparticles (2 nm) can penetrate and enter cell nuclei in an in vitro 3D brain spheroid model. Acta Biomater. 2020;111:349–62.
Simpson LW, Good TA, Leach JB. Protein folding and assembly in confined environments: Implications for protein aggregation in hydrogels and tissues. Biotechnol Adv. 2020;42:107573.
Ellis RJ. Protein folding: importance of the anfinsen cage. Curr Biol. 2003. https://doi.org/10.1016/j.cub.2003.10.051 .
Javidpour L, Sahimi M. Confinement in nanopores can destabilize α-helix folding proteins and stabilize the β structures. J Chem Phys. 2011;135:125101.
Gospodarczyk W, Kozak M. Microchip circulation drastically accelerates amyloid aggregation of 1–42 β-amyloid peptide from felis catus. ACS Chem Neurosci. 2017;8:2558–67.
Park J, Lee BK, Jeong GS, Hyun JK, Lee CJ, Lee SH. Three-dimensional brain-on-a-chip with an interstitial level of flow and its application as an in vitro model of Alzheimer’s disease. Lab Chip. 2015;15:141–50.
Gospodarczyk W, Kozak M. The severe impact of in vivo-like microfluidic flow and the influence of gemini surfactants on amyloid aggregation of hen egg white lysozyme. RSC Adv. 2017;7:10973–84.
Foderá V, Pagliara S, Otto O, Keyser UF, Donald AM. Microfluidics reveals a flow-induced large-scale polymorphism of protein aggregates. J Phys Chem Lett. 2012;3:2803–7.
Lee JS, Um E, Park JK, Park CB. Microfluidic self-assembly of insulin monomers into amyloid fibrils on a solid surface. Langmuir. 2008;24:7068–71.
Shammas SL, Garcia GA, Kumar S, Kjaergaard M, Horrocks MH, Shivji N, et al. A mechanistic model of tau amyloid aggregation based on direct observation of oligomers. Nat Commun. 2015. https://doi.org/10.1038/ncomms8025 .
Horrocks MH, Tosatto L, Dear AJ, Garcia GA, Iljina M, Cremades N, et al. Fast flow microfluidics and single-molecule fluorescence for the rapid characterization of α-synuclein oligomers. Anal Chem. 2015. https://doi.org/10.1021/acs.analchem.5b01811 .
Download references
Acknowledgements
The authors would like to acknowledge The National Research and Development Agency of Chile (ANID), depending on the Ministry of Science, Technology, Knowledge, and Innovation.
This work was funded by Fondecyt Postdoctoral Fellowship 3220583, ANID doctoral scholarships 202110617 and 21200403, Millenium Nucleus in NanobioPhysics (N2BP) N°NCN2021_021, Fondecyt 1230830 and 1211482, and Fondap 15130011.
Author information
Authors and affiliations.
Instituto Universitario de Investigación y Desarrollo Tecnológico (IDT), Universidad Tecnológica Metropolitana, Santiago, Chile
Andreas Tapia-Arellano & Natalia Hassan
Facultad de Cs. Qcas. y Farmacéuticas, Universidad de Chile, Santiago, Chile
Andreas Tapia-Arellano, Pablo Cabrera, Elizabeth Cortés-Adasme, Ana Riveros & Marcelo J. Kogan
Advanced Center for Chronic Diseases (ACCDis), Santiago, Chile
Andreas Tapia-Arellano, Pablo Cabrera, Elizabeth Cortés-Adasme, Ana Riveros, Natalia Hassan & Marcelo J. Kogan
Millenium Nucleus in NanoBioPhysics, Valparaíso, Chile
You can also search for this author in PubMed Google Scholar
Contributions
ATA, NH and MJK conceived the topic and drew up the outline. ATA, PC, ECA, AR and NH wrote and revised the original draft. ATA and MJK reviewed and edited the draft. ATA, NH and MJK provided funding for the project. All authors read and approved the final manuscript .
Corresponding authors
Correspondence to Andreas Tapia-Arellano , Natalia Hassan or Marcelo J. Kogan .
Ethics declarations
Ethics approval and consent to participate, consent for publication.
All authors gave their consent for publication.
Competing interests
The authors declare that they have no competing interests.
Additional information
Publisher's note.
Springer Nature remains neutral with regard to jurisdictional claims in published maps and institutional affiliations.
Rights and permissions
Open Access This article is licensed under a Creative Commons Attribution 4.0 International License, which permits use, sharing, adaptation, distribution and reproduction in any medium or format, as long as you give appropriate credit to the original author(s) and the source, provide a link to the Creative Commons licence, and indicate if changes were made. The images or other third party material in this article are included in the article's Creative Commons licence, unless indicated otherwise in a credit line to the material. If material is not included in the article's Creative Commons licence and your intended use is not permitted by statutory regulation or exceeds the permitted use, you will need to obtain permission directly from the copyright holder. To view a copy of this licence, visit http://creativecommons.org/licenses/by/4.0/ . The Creative Commons Public Domain Dedication waiver ( http://creativecommons.org/publicdomain/zero/1.0/ ) applies to the data made available in this article, unless otherwise stated in a credit line to the data.
Reprints and permissions
About this article
Cite this article.
Tapia-Arellano, A., Cabrera, P., Cortés-Adasme, E. et al. Tau- and α-synuclein-targeted gold nanoparticles: applications, opportunities, and future outlooks in the diagnosis and therapy of neurodegenerative diseases. J Nanobiotechnol 22 , 248 (2024). https://doi.org/10.1186/s12951-024-02526-0
Download citation
Received : 02 February 2024
Accepted : 02 May 2024
Published : 13 May 2024
DOI : https://doi.org/10.1186/s12951-024-02526-0
Share this article
Anyone you share the following link with will be able to read this content:
Sorry, a shareable link is not currently available for this article.
Provided by the Springer Nature SharedIt content-sharing initiative
- Alzheimer's disease
- Protein misfolding
- Theragnosis
Journal of Nanobiotechnology
ISSN: 1477-3155
- Submission enquiries: [email protected]
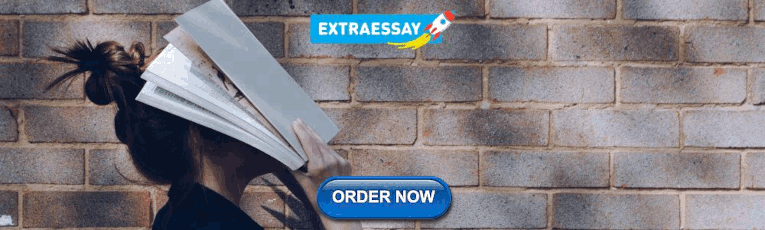
IMAGES
VIDEO
COMMENTS
A research design is a strategy for answering your research question using empirical data. Creating a research design means making decisions about: Your overall research objectives and approach. Whether you'll rely on primary research or secondary research. Your sampling methods or criteria for selecting subjects. Your data collection methods.
Diagnostic research designs: an introductory overview Madhukar Pai, MD, PhD Associate Professor of Epidemiology, McGill University Montreal, Canada Email: [email protected] Classical EBM approach to diagnosis: compute sens/spec, LRs, and work out the post-test probabilities… Disease ruled IN Disease ruled OUT Disease not ruled in or out
The diagnostic research design consists of three steps: Inception, which includes data collection and problem definition. Diagnostics, which comprises data analysis, hypothesis testing, and setting objectives. ... Research Design Examples. Let's explore how leading brands employ different types of research design. In most cases, companies ...
Research design refers to the overall plan, structure or strategy that guides a research project, from its conception to the final analysis of data. Research designs for quantitative studies include descriptive, correlational, experimental and quasi-experimenta l designs. Research designs for qualitative studies include phenomenological ...
Diagnostic research is conducted to improve diagnostic procedures and tests with the aim of improving diagnostic accuracy. Prognostic research mainly aims to identify and quantify factors that dictate the prognosis in patients [ 1 ]. Research conducted in pathology follows two general design strategies; the research is either descriptive where ...
Bayes' theory •Bayes' Theorem is a simple mathematical formula used for calculating conditional probabilities •every test is done with a certain probability of disease - degree of suspicion [pre-test or prior probability] •the probability of disease after the test result is the post-test or posterior probability pre-test
Ranganathan P. Understanding Research Study Designs. Indian J Crit Care Med 2019;23 (Suppl 4):S305-S307. Keywords: Clinical trials as topic, Observational studies as topic, Research designs. We use a variety of research study designs in biomedical research. In this article, the main features of each of these designs are summarized. Go to:
The study design used to answer a particular research question depends on the nature of the question and the availability of resources. In this article, which is the first part of a series on "study designs," we provide an overview of research study designs and their classification. The subsequent articles will focus on individual designs.
Table of contents. Step 1: Consider your aims and approach. Step 2: Choose a type of research design. Step 3: Identify your population and sampling method. Step 4: Choose your data collection methods. Step 5: Plan your data collection procedures. Step 6: Decide on your data analysis strategies.
Designing Diagnostic Studies13. Introduction. Research and investigation are important components of the practice of pathology. Pathologists are mainly involved in two types of clinical research: diagnostic or prognostic. Diagnostic research is conducted to improve diagnostic procedures and tests with the aim of improving diagnostic accuracy.
For example, one monitors the influence of an independent variable such as a price on a dependent variable such as customer satisfaction or brand loyalty. It is an efficient research method as it contributes to solving a problem. ... Diagnostic research: In diagnostic design, the researcher is looking to evaluate the underlying cause of a ...
Types of Research Designs Compared | Guide & Examples. Published on June 20, 2019 by Shona McCombes.Revised on June 22, 2023. When you start planning a research project, developing research questions and creating a research design, you will have to make various decisions about the type of research you want to do.. There are many ways to categorize different types of research.
Types of Diagnostic Design. Diagnostic design analysis is classified into quantitative and qualitative categories. Cross-Sectional Study. A cross-sectional study is prevalent in medical research, social science, and biology. This study approach examines data from a population or a representative population sample at a specific point in time.
Assessment of Diagnostic Accuracy of Single Test. Establishing an accurate diagnosis is a critical first step in the management of a health problem; type I diagnostic trials or diagnostic accuracy studies attempt to answer this question. To design a type I diagnostic trial, investigators must recruit subjects with and without the index disease and obtain valid and precise information about the ...
A good research design draws an outcome that can be applied to a large set of people and is not limited to sample size or the research group. Neutrality. ... Diagnostic design. In diagnostic research, the design strives to explore the reason behind an issue and find solutions to solve it. This type of research design tries to solve the problems ...
Results. Identified methods were classified into four main groups: methods employed when there is a missing gold standard; correction methods (which make adjustment for an imperfect reference standard with known diagnostic accuracy measures); methods employed to evaluate a medical test using multiple imperfect reference standards; and other methods, like agreement studies, and a mixed group of ...
04. Diagnostic research design. In a diagnostic research design, the researcher is trying to evaluate the cause of a specific problem or phenomenon. This research design is used to understand more in detail the factors that are creating problems in the company. Diagnostic research design includes three steps:
Understanding the Descriptive/ Diagnostic Research Design. This Power Point Presentation has been made while referring to the research books written by eminent, renowned and expert authors as mentioned in the references section. The purpose of this Presentation is to help the research students in developing an insight about The Descriptive ...
Abstract. Much of clinical research is aimed at assessing causality. However, clinical research can also address the value of new medical tests, which will ultimately be used for screening for ...
It's the plan that outlines how the research will be carried out. A study design usually includes the methods of data collection, the type of data to be gathered, and how it will be analyzed. Research designs help ensure the study is reliable, valid, and can answer the research question. Behind every groundbreaking discovery and innovation lies ...
A good diagnostic research design is essential for conducting successful research. It ensures that the research is conducted in a systematic and rigorous manner. It also ensures that the results are valid and reliable. Diagnostic Research Design Example. Here is an example of a diagnostic research design for a study on low student achievement ...
As discussed earlier, common research methods for descriptive research include surveys, case studies, observational studies, cross-sectional studies, and longitudinal studies. Design your study: Plan the details of your study, including the sampling strategy, data collection methods, and data analysis plan.
Research design is the plan, structure and strategy and investigation concaved so as to obtain search question and control variance" (Borwankar, 1995). ... For example, you can experiment with ...
Alzheimer's disease (AD), the most common form of dementia, remains challenging to understand and treat despite decades of research and clinical investigation. This might be partly due to a lack of widely available and cost-effective modalities for diagnosis and prognosis. Recently, the blood-based AD biomarker field has seen significant progress driven by technological advances, mainly ...
At NYU Langone's Multi-Omics Study Design and Data Integration Resource (MOSDIR), we are dedicated to developing novel statistical methods and customized statistical approaches that are tailored to your specific study and research needs. From study conception to data interpretation, MOSDIR is here to guide you through every step of your multi ...
The Multi-Omics Study Design and Data Integration Resource is located in Manhattan at 180 Madison Avenue, fourth floor. To contact our director, Huilin Li, PhD, please email [email protected]. To submit an inquiry, please complete our request form.
Breathomics is a branch of metabolomics that analyzes various volatile organic compounds (VOCs) from exhaled breath samples. It has been rapidly growing as a non-invasive diagnostic tool to probe or infer the pathogenic or physiological status of the human body, often yielding crucial information for disease diagnostics. Unlike traditional diagnostic methods that often require invasive ...
Diagnosing rare diseases is a significant challenge in healthcare, with patients often experiencing long delays and misdiagnoses. The large number of rare diseases and the difficulty for doctors to be familiar with all of them contribute to this problem. Artificial intelligence, particularly large language models (LLMs), has shown promise in improving the diagnostic process by leveraging their ...
One use case example is out of the University of Hawaii, where a research team found that deploying deep learning AI technology can improve breast cancer risk prediction. More research is needed, but the lead researcher pointed out that an AI algorithm can be trained on a much larger set of images than a radiologist—as many as a million or ...
The use of nanomaterials in medicine offers multiple opportunities to address neurodegenerative disorders such as Alzheimer's and Parkinson's disease. These diseases are a significant burden for society and the health system, affecting millions of people worldwide without sensitive and selective diagnostic methodologies or effective treatments to stop their progression. In this sense, the use ...