Multiple-Hazard Zone Case Study ( Edexcel A Level Geography )
Revision note.
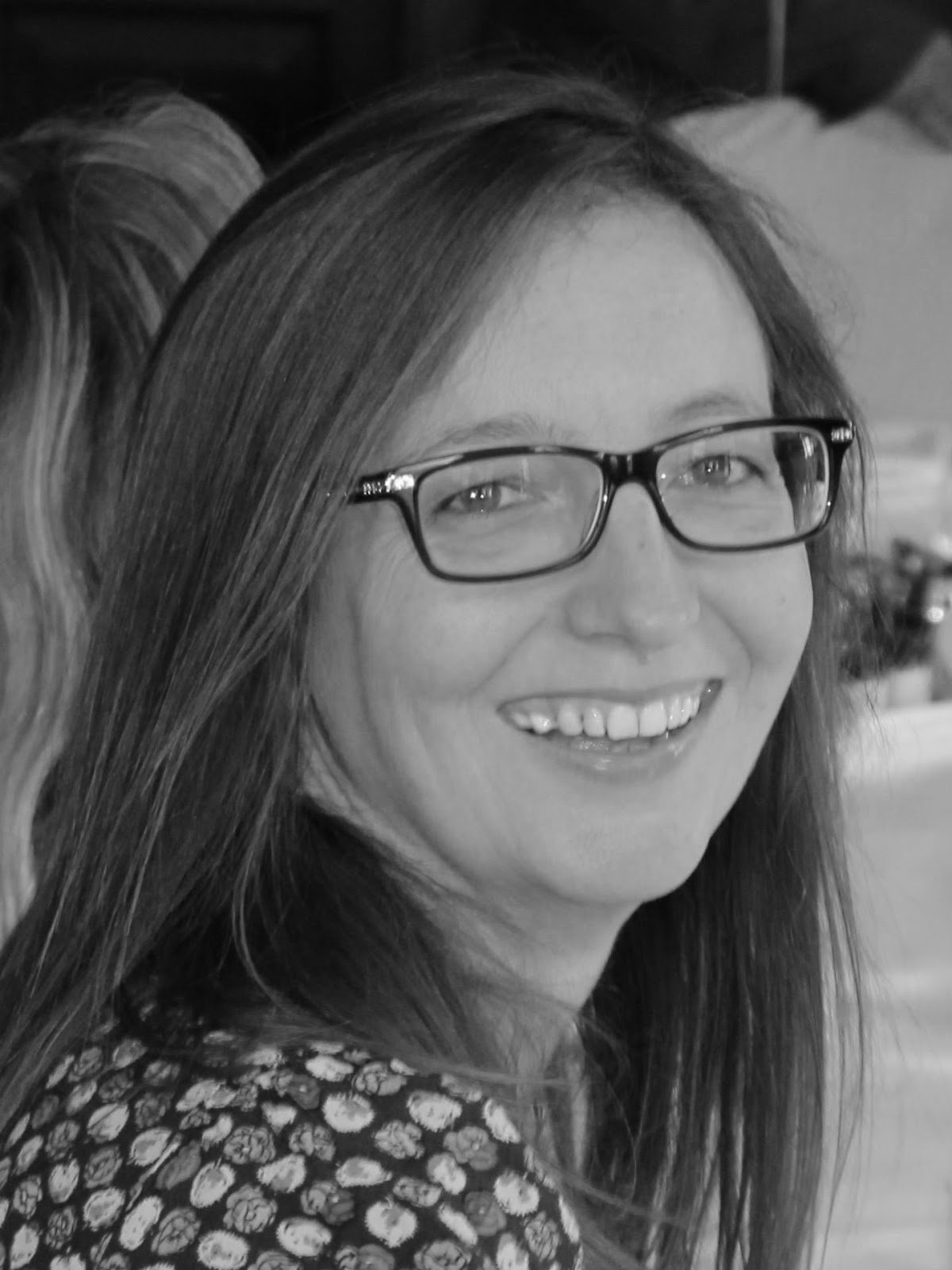
Geography Lead
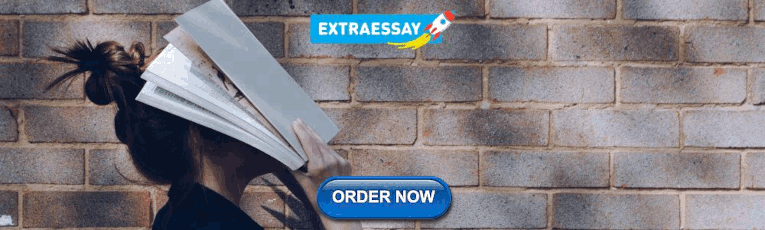
Multiple-Hazard Zone Case Study
- There are many areas in the world which are vulnerable to multiple hazards
- Dealing with a new hazard event whilst still recovering from a previous hazard event
- Issues with development due to the costs of recovering from multiple hazard events
The Philippines
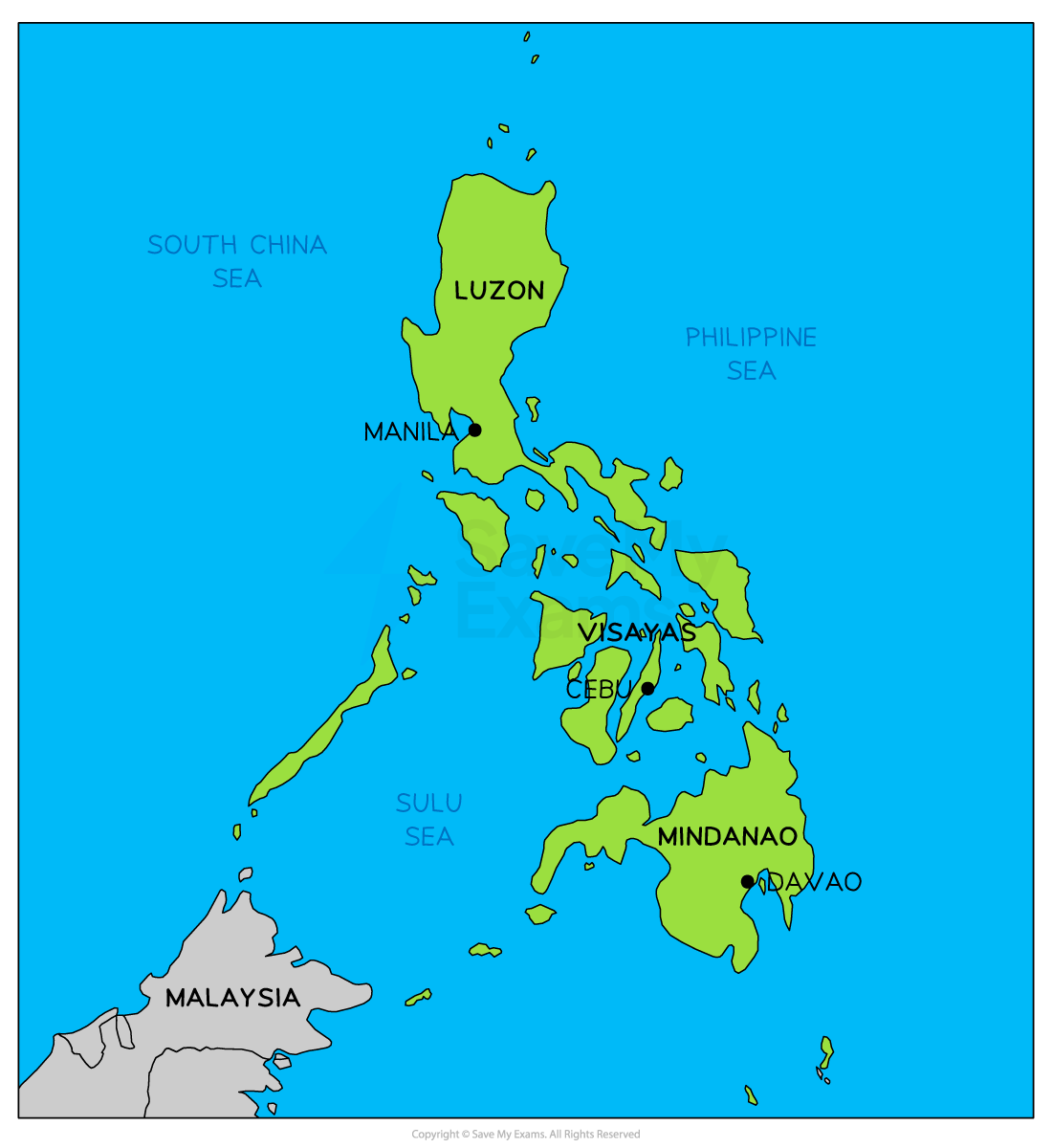
Map of the Philippines
- The Philippines is an island nation situated in Southeast Asia in the Western Pacific Ocean
- It is an archipelago consisting of over 7,600 islands
- The capital is Manila
- The population is estimated at 115.6 million (2022) with a population density of 385.2 people per km 2
- Almost 50% of the population is urban
- An estimated 74% of the population and 60% of the land area is vulnerable to hazard events
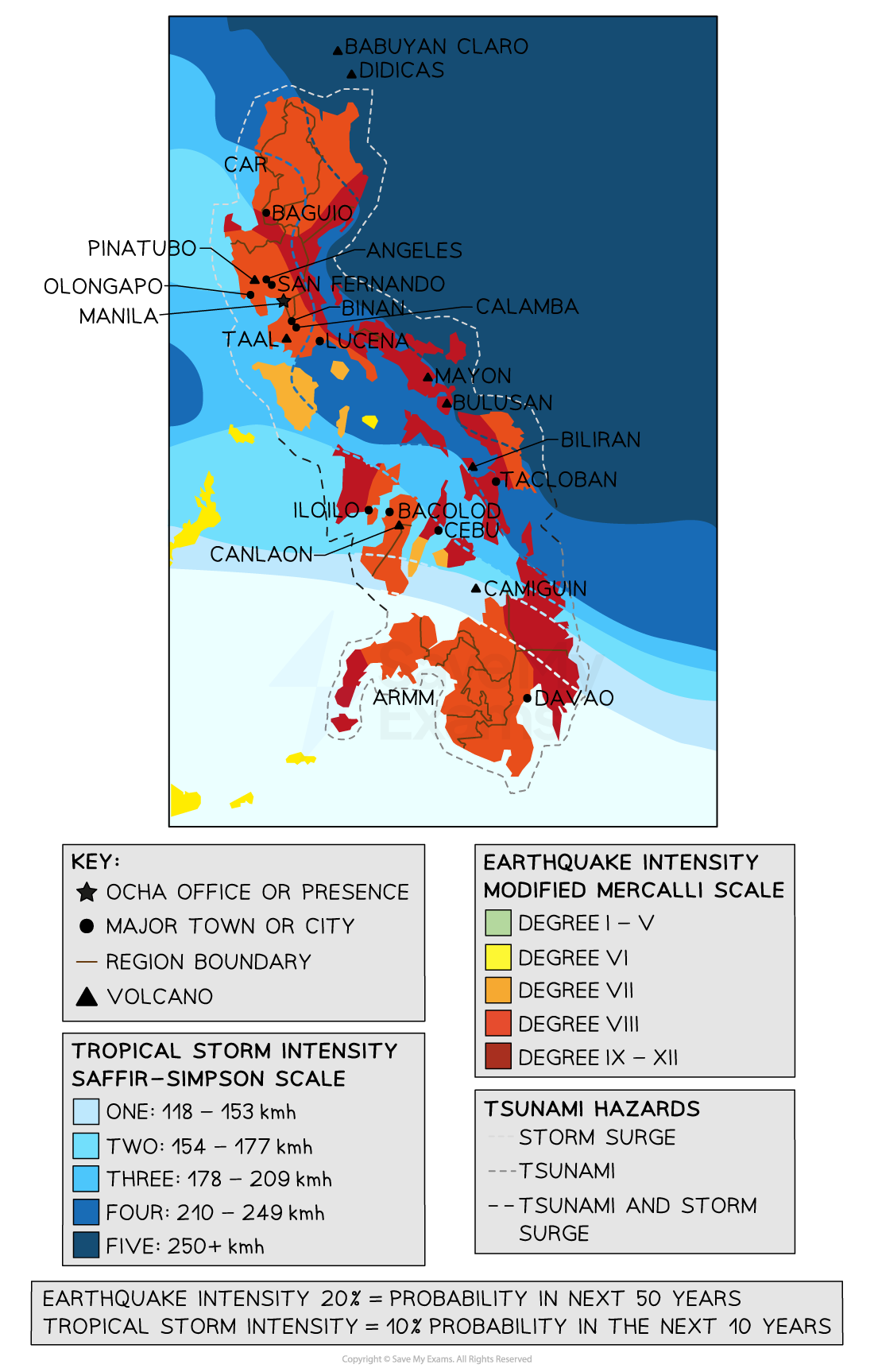
Risk of hazard events
- In 2019 and 2020 the Philippines experienced 4 typhoons, the eruption of the Taal volcano and a number of earthquakes
Tectonic activity
- The Philippines are located at the boundaries of a number of major and minor plate boundaries
- It is on the western edge of the Pacific Ring of Fire
- These are mainly convergent boundaries leading to both volcanoes and earthquakes
Volcanoes and earthquakes
- There are 24 active volcanoes on the islands
- The eruption of Mount Pinatubo in 1991 was until recently the largest eruption in the last 100 years
- Approximately 2.81 million people live within 30km of the Taal volcano
- Mount Mayon has erupted over 50 times in the last 500 years
- The location in the Western Pacific makes the Philippines vulnerable to tsunami
- Tsunami may result from both volcanic eruptions and earthquakes
- The worst tsunami in recorded history occurred in 1976 when tsunami waves of up to 5 meters struck Lebak in the southwest of the Philippines
- The Philippines is located between 5 o and 20 o north of the equator and lies in the tropical cyclone zone
- The most active months are June to September
- The islands are affected by between 15-20 typhoons a year
- Wind speeds reached 315km/h and 6,500 people were killed
Landslides
- The mountainous landscape of the Philippines means that landslides are common
- Earthquakes, volcanic eruptions and typhoons can all trigger landslides
Vulnerability
- Increased urbanisation has led to high population densities in cities such as Manila
- The population is mostly coastal as inland areas are mountainous, this increases the vulnerability to storm surges, typhoons and tsunami
- Deforestation increases the risks of landslides as there is less interception and more surface runoff
- More settlements have been built in areas of high risk, Angeles was built near Mount Pinatubo before the eruption in 1991
- The GDP per capita is US$3,460 compared to the USA which is US$70,248
You've read 0 of your 10 free revision notes
Get unlimited access.
to absolutely everything:
- Downloadable PDFs
- Unlimited Revision Notes
- Topic Questions
- Past Papers
- Model Answers
- Videos (Maths and Science)
Join the 100,000 + Students that ❤️ Save My Exams
the (exam) results speak for themselves:
Did this page help you?
Author: Bridgette
After graduating with a degree in Geography, Bridgette completed a PGCE over 25 years ago. She later gained an MA Learning, Technology and Education from the University of Nottingham focussing on online learning. At a time when the study of geography has never been more important, Bridgette is passionate about creating content which supports students in achieving their potential in geography and builds their confidence.
Please enable JavaScript.
Coggle requires JavaScript to display documents.
The Philippines - Multi-hazard Case Study (Earthquakes (Earthquakes are…
- Its geographical position on the globe means its highly prone to natural disasters. The Philippines is on the western rim of the 'Pacific Ring of Fire' which brings both earthquakes and volcanic activity .
- The Philippines is a country off the mainland southeast Asia consisting of over 7,000 islands . It is home to over 100 million people .
- It is also situated in an area where tropical storms come from across the Pacific to southeast Asia, this makes the Philippines the most exposed country in the world. Tropical storms bring a large amount of flooding and the threat of landslides and lahars .
- Earthquakes are fairly common occurrence in the Philippines. Between 2000 and 2013 , there were 17 earthquakes with a minimum magnitude of 5.6 . These caused numerous deaths and damaged a large number of buildings.
- In 1976 , just off Mindanao , there was an earthquake of magnitude 7.9 which caused tsunamis and inundated the western shore of Mindanao, killing up to 8,000 people .
- In 1990 , in Luzon , there was an earthquake of magnitude 7.8 which killed over 1,600 people and caused widespread ground rupturing and soil liquefaction .
- In 2013 , a magnitude 7.2 earthquake hit Bohol and killed over 200 and injured over 800 people . It caused damaged to tens of thousands of buildings .
- Some of the most devastating in recent years are:
- The Philippines has a number of active volcanoes on its islands. Opinions vary, but 23 active volcanoes is reasonable.
- Among these are three with a history of violent eruptions:
- Mount Pinatubo erupted violently in 1991 , being the second largest eruption on the planet. Pinatubo can be very deadly as 500,000 people live in a 40km radius . Early warnings and evacuations saved around 5,000 people in 1991 . The eruption was so violent it threw gasses, ash and steam into the upper atmosphere , this caused the global temperature to decrease half a degree for two years . The eruption was then followed by Typhoon Yunya , the heavy rainfall along with the volcanic ash created very deadly lahars and the final death toll was around 850 .
- The Mayon volcano is the most active , having erupted almost 50 times in the past 400 years . Its most destructive eruption was in 1812 , its most recent eruption was in 2014 . Mayon is widely known for its lahars , with volcanic ash mixing with the heavy rainfall from the typhoons to produce rivers of mud .
- The Taal volcano is one of the most active in the country with over 33 eruptions noted on historical record. All of these eruptions came from a volcano which sits in the middle of a crater lake . This crater lake formed from an e xplosion from prehistoric times which formed the caldera of the Taal . In the historical record, Taal has been responsible for approximately 5,000-6,000 deaths due to the densely populated areas that are close by.
- Tropical storms cross the country every year, bringing with them the risk of flooding, landslides and lahars if combined with a volcanic eruption.
- It is tropical storms and the hazards that come along with them which cause the highest number of deaths from natural hazards in the Philippines. The most fatalities come from the storm surges .
- An example of a Typhoon in the Philippines would be Typhoon Haiyan of Novemember 2013.
- 78.7% of mortality losses came from tropical storms , with the seconds highest killer being earthquakes , but only having a percentage of 7.9% .
- 79% of economic losses also came from tropical storms , however, the second highest is flooding. Flooding caused an economic loss of 17.3% .
Can we help you find something?
Gtranslate custom.
- Publications
- Responding to Multiple Disasters: Case Study on the Philippine Disaster Resilience Foundation Emergency Operations Center and Response to Super Typhoon Goni and Vamco during the COVID-19 Pandemic
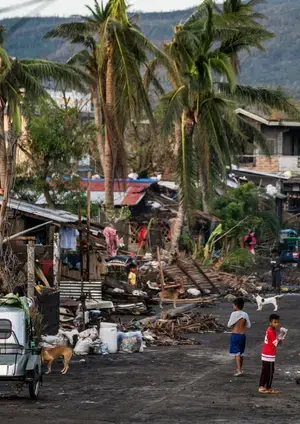
- Philippines
- Responding to Multiple Disasters: Case Study on the Philippine Disaster Resilience Foundation Emergency Operations Center and Re 1.12 MB
As COVID-19 continued to threaten business operations and health conditions, the Philippines had to also deal with Super Typhoon Goni (known locally as Rolly) and Super Typhoon Vamco (known locally as Ulysses) in the last quarter of 2020.
As a leading private sector network managing and reducing disaster risks and assisting affected areas, the Philippine Disaster Resilience Foundation (PDRF), a CBi Member Network, has been working closely with the Government and UN organizations. This case study draws on the PDRF experience around the triple whammy scenario in 2020 descibed above and answers the question "How does the Philippine Disaster Resilience Foundation EOC respond to multiple disaster scenarios?"
Content Search
Philippines
REAP Country Case Studies: Philippines - 9 December 2021
Attachments.

The Philippines is one of the largest island groups in the world with 7,107 islands and islets (OCD-DND, 208). The country is exposed to multiple hazards - at least 60 per cent of the total land area is exposed to multiple hazards, and 74 per cent of the population is vulnerable to impacts (GFDRR, 2017; IFRC, 2020). The country is situated on the typhoon belt of the western pacific and located within the Circum-Pacific Belt, also known as the Ring of Fires. On average, about 20 tropical cyclones enter the Philippines waters each year, with approximately eight or nine making landfall (ESCAP/WMO, 2009; Bankoff, 2003), causing the greatest damage.
Other hazards which are increasing in frequency include floods, landslides, tsunamis and wildfires, all of which are occurring in frequency. Of these, hydro-meteorological events accounted for over 80 percent of the natural disasters in the country during the last half-century (UNDRR, 2019). All the main hydrometeorological hazards in the country (typhoons, storm surges, drought, sea-level rise, and tsunamis) are directly influenced by climate change and are, therefore, expected to continue to exacerbate in terms of intensity, frequency, and unpredictability over the coming years (IFRC, 2020). According to the Philippine Atmospheric Geophysical and Astronomical Services Administration (PAGASA), the intensity of tropical cyclones entering the Philippines area of responsibility (PAR)1 had been increasing between 1951 and 2015. In recent years, the country has been struck by some of the strongest ever recorded in the world, such as Typhoon Haiyan in 2013. Other slow-onset phenomena like the El Niño Southern Oscillation in 2015, which affected seven million individuals across 43 provinces and was the strongest since 1950, can disrupt the economy and livelihoods (IFRC, 2020).
Related Content
Philippines: ifrc network mid-year report, january - june 2023 (14 december 2023).
Philippines + 1 more
Philippines Assistance Overview, September 2023
Philippines annual country report 2022 - country strategic plan 2018 - 2023, philippines: 2023 ifrc network country plan (maaph001).
- International
- Education Jobs
- Schools directory
- Resources Education Jobs Schools directory News Search
The Philippines: multi-hazardous environment case study
Subject: Geography
Age range: 16+
Resource type: Assessment and revision

Last updated
25 July 2019
- Share through email
- Share through twitter
- Share through linkedin
- Share through facebook
- Share through pinterest
Revision notes on The Philippines as a multi-hazardous environment for AQA’s A Level Geography course, focusing on major hazards (earthquakes, volcanoes, tropical storms, landslides and tsunamis), vulnerability and management.
Tes paid licence How can I reuse this?
Your rating is required to reflect your happiness.
It's good to leave some feedback.
Something went wrong, please try again later.
This resource hasn't been reviewed yet
To ensure quality for our reviews, only customers who have purchased this resource can review it
Report this resource to let us know if it violates our terms and conditions. Our customer service team will review your report and will be in touch.
Not quite what you were looking for? Search by keyword to find the right resource:

- Sign Up for Free Sign Up
Multi-Hazard Environment: The Philippines
Description.
- philippines
- multi hazard environment
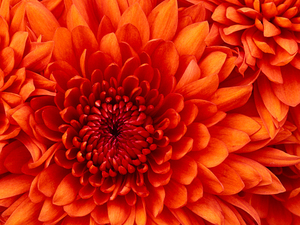
over 7 years ago | ||
Resource summary
- off the mainland of SE Asia
- Consists of an archipelago of 7,000 islands
- Brings earthquakes and volcanoes
- Most exposed country in the world to typhoons
- In past 2 decades has experienced over 300 natural disasters
- Severe Bohol earthquake
- 7 typhoons, including Haiyan
- 8 tropical storms
- Volcanic eruptions
- Cost to economy: 0.5% of national GDP
- Most exposed in world to typhoons
- Top - down approach to administration. Most vulnerable thus not catered for
- Consists of over 7000 islands - too much responsibility falls to local government where money is not always wisely spent.
- One government official: 'resources are stretched; even before we could recover from one disaster, here is the next one.'
- Environmental degradation - deforestation = rapid runoff = flooding and landslides
- Fatalistic attitude - 'God's Will'
- No land use planning - flimsy buildings
- Clear link between poverty and vulnerability
- Have relied upon a reactive approach rather than mitigation or avoidance
- Failed to produce land use planning
- A focus on post - disaster relief and short term preparedness such as evacuation rather than long term mitigation
- Little local initiative - not community based administartion system,. but rather a top - down one. No emphasis on the most vulnerable.
- reduce impacts of hazards by encouraging people to collaborate in protecting their lives and resources
- Cooperation with government bodies to gain financial support for mitigation measures and ensure the programmes have long term sustainability
- train local volunteers in disaster management
- Dissemination of information to whole community
- UN claims Philippines has some of the best risk reduction laws in the world - but most are still on paper
- Recent government legislature: 70% of disaster spending must be used on long - term plans
- leads to tsunamis, fires, landslides and human crime such as looting
- 2013 Bohol: 7.2 magnitude, killed over 200, damage to tens of thousands of buildings
- Guinyangan fault - most destructive, minimum of 7.0 Richter Scale earthquakes
- Approx. 23 active volcanoes on its islands
- Lead to lahars
- Islands formed by a combination of folding at the boundary. Volcanoes formed from rising magma.
- Last eruption 2014
- Most destructive: 1814
- Volcanic ash mixes with heavy rainfall from tropical storms to produce rivers of mud
- early warnings saved 5000
- Followed by Typhoon Yunya - final death toll 850
- 500,000 live within 40km of it
- So violent that ash and gas ejected into upper atmosphere and affected global temps. for at least 2 years - avg. global temp down 0.5 degrees Celsius
- Develop in Pacific and move westwards over the islands
- Typhoon Haiyan
- Risk of flooding, landslides and lahars (if combined with a volcano)
- Mostly from storm surges
- Around 10 typhoons a year
Want to create your own Mind Maps for free with GoConqr? Learn more .
Skip to content
Get Revising
Join get revising, already a member.

Philippines - multiple hazard zone
- Case studies
- Created by: izzyyyy.
- Created on: 20-02-24 10:23
- group of 7,000 islands in theSouth China Sea in South East Asia - islands are at latitudes 5–20°N of the equator.
- vulnerable population of 98 million people who are at risk from a variety of hazards, including 11 million people in the densely populated coastally situated capital Manila
- one of the most disaster-prone countries ? situated on a major plate boundary ? north and east coast faces the Pacific ? tropical monsoon climate is experienced there and subject to heavy rain ?lies in SE of Asia’s typhoon belt
- average population density is high with 240 people per square km, with Manila having a density of up to 2,000 people per square km.
- creates the Manila Ocean trench
- 37 volcanoes, 23 active
- near to a destructive plate boundary whereby the dense oceanic crust of the Philippine Plate is being subducted beneath the continental crust of the Eurasian Plate.
- islands were formed by a combination of folding at the boundary, and volcanos formed from magma that has risen to the surface from the Mantle below from the subducted Philippine plate.
- The eruption of Mount Pinatubo in June 1991, which killed 500 people, and caused crop failure due to the falling ash (from the eruption).
- e Philippine Plate and the Eurasian Plate can become locked together as the Philippine Plate is being subducted, which can cause the buildup and sudden release of pressure.
- can also occur at Fault Lines in the area, where the plate has cracked under pressure.
- e.g. 1990 Earthquake on Luzon Island, magnitude 7.8, killing 1500 people
- triggered by tectonic activity in the area from Earthquakes
- can also be caused by heavy levels of rainfall which lead to cliff saturation if they fall in areas with a steep gradient
- e.g. 2006 Landslide on Leyte Island after 10 days of heavy rain, which buried a village and killed hundreds of people subsequently
- situated in the latitudes between 520 degrees North of the Equator, so is vulnerable to Typhoons developing in the West of the Pacific Ocean, where Ocean surface temperature is above 26 degrees celsius and the Coriolis effect is strong enough to rotate the low pressure system
- e.g.1 Typhoon Xangsane in 2006, which swept across Manila and the surrounding densely populated area, destroying homes with torrential rain and subsequent flooding/landslides
- e.g. in 1976 a submarine earthquake of magnitude 7.9 caused a Tsunami of 45m high which hit the coastline of the Moro Gulf on the Southern Island of Mindanao, damaging 14 buildings in Zamboanga City.
- Between 1990 and 2012, droughts caused economic damage of US $64.5 million.
- Flash flooding between 1990 and 2012 affected 5,700,690 people. There were economic losses of US $1 billion.
- economic deprivation/poverty = low capacity to cope, as they can’t afford to build stable hazard proof infrastructure, provide special educational programmes to citizens, provide mitigation disasters kits
- Landslide disaster risk has increased as the pressures of an increasing population have led to deforestation of upland areas for agriculture, which reduces interception (increased lag time) and water table capacity (decreasing potential levels of infiltration)
- Settlements have been built in vulnerable areas e.g. Angeles near Mount Pinatubo when it was classified as dormant (not erupted since 1830) before eruption in 1991
- ? National disaster coordinating council? Philippine Atmospheric, Geophysical and astronomical services? Philippine Institute of Volcanology and Seismology (PHIVOLCS)
- ? Land use planning e.g. preventing building in high-risk areas ? Building regulations ? Structural programme of defences e.g. building embankments to reduce floodrisk in the case of tsunamis and tropical storms ? 70% of disaster spending must be used on long-term plans
- various programmeat community level e.g. train local volunteers in disaster management and cooperating with the government for financial support, ensuring sustainability and identifying risk through hazard mapping
- 10,710 hectares were damaged by wildfires from Jan to Jun 1995. Most plantation forests were burned March is the peak of the fire season. There were 104 fires with 4,127 hectares damaged
No comments have yet been made
Similar Geography resources:
Philippines Case Study- Multiple Hazard Zones 2.0 / 5 based on 1 rating
Hazard Trends 0.0 / 5
1.7 Tectonic Hazards 0.0 / 5
Phillipines 0.0 / 5
Tectonics - definitions and examples 0.0 / 5
Tectonics EQ3 0.0 / 5
Techtonics 0.0 / 5
tectonics case studies!! 0.0 / 5
Disaster Trends and Multiple Hazard Zones 0.0 / 5
tectonic hazards and processes 5.0 / 5 based on 1 rating
Advertisement
Risk Assessment of Multi-Hazards in Hangzhou: A Socioeconomic and Risk Mapping Approach Using the CatBoost-SHAP Model
- Open access
- Published: 22 August 2024
- Volume 15 , pages 640–656, ( 2024 )
Cite this article
You have full access to this open access article
- Bofan Yu 1 ,
- Jiaxing Yan 1 ,
- Yunan Li 1 &
- Huaixue Xing 2
294 Accesses
Explore all metrics
As the global push for sustainable urban development progresses, this study, set against the backdrop of Hangzhou City, one of China’s megacities, addressed the conflict between urban expansion and the occurrence of urban geological hazards. Focusing on the predominant geological hazards troubling Hangzhou—urban road collapse, land subsidence, and karst collapse—we introduced a Categorical Boosting-SHapley Additive exPlanations (CatBoost-SHAP) model. This model not only demonstrates strong performance in predicting the selected typical urban hazards, with area under the curve (AUC) values reaching 0.92, 0.92, and 0.94, respectively, but also, through the incorporation of the explainable model SHAP, visually presents the prediction process, the interrelations between evaluation factors, and the weight of each factor. Additionally, the study undertook a multi-hazard evaluation, producing a susceptibility zoning map for multiple hazards, while performing tailored analysis by integrating economic and population density factors of Hangzhou. This research enables urban decision makers to transcend the “black box” limitations of machine learning, facilitating informed decision making through strategic resource allocation and scheduling based on economic and demographic factors of the study area. This approach holds the potential to offer valuable insights for the sustainable development of cities worldwide.
Similar content being viewed by others
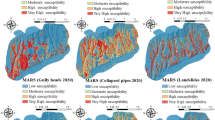
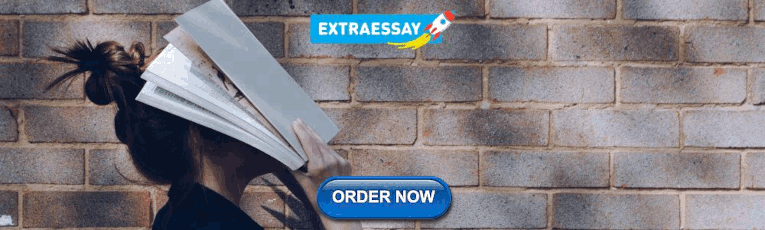
Analytical techniques for mapping multi-hazard with geo-environmental modeling approaches and UAV images
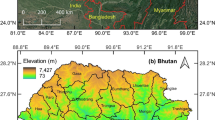
Multi-hazard zoning for national scale population risk mapping: a pilot study in Bhutan Himalaya
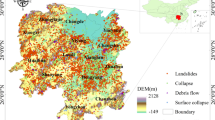
Temporal and spatial pattern analysis and susceptibility assessment of geological hazards in Hunan Province of China from 2015 to 2022
Explore related subjects.
- Artificial Intelligence
Avoid common mistakes on your manuscript.
1 Introduction
The concept of urban sustainability was first proposed by the Brundtland Commission in 1987 (Redclift 1989 ), and since then, it has been widely adopted and integrated into urban planning strategies worldwide. However, as urban areas expand and populations increase, cities face the growing challenge of balancing this development with the mitigation of geological hazards (Zhou and Zhao 2013 ; Chen et al. 2022 ; Lu et al. 2022 ). The confluence of rapid urbanization and the inherent vulnerabilities to seismic, hydrological, and subsurface events creates a paradox, and the very high density of infrastructure that characterize urban growth can amplify the risks of such hazards (Chen et al. 2012 ; Zhang and Li 2020 ). Addressing this conflict is not only critical for safeguarding human life and property but also for the resilience and continuity of urban progress in harmony with the environment (Godschalk 2003 ; Etinay et al. 2018 ; Gu et al. 2018 ). As countries worldwide endeavor to construct sustainable cities, China’s urban areas are also actively embracing the principles of sustainable development (Liu et al. 2014 ; Wang and He 2015 ). Hangzhou City is one of China’s 10 megacities, with a permanent population exceeding 10 million, a per capita GDP on par with developed nations (Zhou et al. 2022 ), and was named China’s Happiest City for 17 consecutive years. However, according to the survey data from the Zhejiang Geological Survey, as of 2022, Hangzhou had identified 1055 geological hazard sites, and three types of hazards are widely distributed in its urban areas. Urban road collapses have caused the most casualties, land subsidence is the most widespread and has resulted in the largest economic losses, while karst collapses appeared the earliest and caused significant challenges for Hangzhou in the past when technology was not yet advanced. Urban road collapse is caused by the formation and expansion of underground voids, often resulting from groundwater erosion, drainage system leaks, or underground construction activities (Wang et al. 2022 ). These collapses jeopardize road safety, disrupt residents’ mobility, and can lead to significant property losses, sometimes resulting in casualties (Wang and Xu 2022 ; Wang et al. 2023 ). Karst collapse occurs when soluble rocks like limestone are dissolved by solution processes, creating underground voids that, when large enough, cause the surface to lose support and collapse (Papadopoulou-Vrynioti et al. 2013 ; Gutiérrez et al. 2014 ). Such collapses are common in karst geological areas and threaten the stability of buildings and infrastructure (De Waele et al. 2011 ). Land subsidence is typically caused by excessive groundwater extraction, overloading of the land, or changes in geological structures, which, over time, can damage urban infrastructure, affecting the efficiency of surface water discharge and urban drainage systems (Hu et al. 2004 ; Bagheri-Gavkosh et al. 2021 ; Shirzaei et al. 2021 ).
Susceptibility mapping is a critical component in evaluating and predicting geological hazards (Sun 2024 ; Yan et al. 2024 ; Yu et al. 2024 ), which integrates geological environmental conditions with factors that cause and trigger hazards, aiming to forecast the likelihood of geological disasters in specific areas. For susceptibility mapping of urban areas, considering that multi-hazards are crucial, the concept of multi-hazard analysis, a term used by the United Nations in sustainable development and Agenda 21 for risk reduction and disaster management (Gray 1990 ), becomes vital for better regional planning, as it allows for a comprehensive understanding of the interplay between different hazards, enabling a thorough assessment of geological safety risks in a given area. Over the past decades, scientific research on multi-hazards has become more common, yet remains a challenge for twentyfirst century researchers due to the complexity of integrating diverse datasets, the need for advanced modeling techniques, and the difficulty in predicting the combined effects of multiple hazards (Shi et al. 2016 ; Pourghasemi et al. 2020 ; Shi et al. 2020 ; Alikaei et al. 2023 ). Due to the complexity of urban regions and the smaller, more dispersed nature of disaster points, knowledge-driven methods, particularly analytical hierarchy process (AHP), have been commonly used in such evaluations (Wu et al. 2018 ; Wei et al. 2021 ; Haghiri et al. 2024 ). However, as the demand for more accurate disaster assessments grows, the limitations of the AHP method, notably its reliance on the subjective judgment of experts for weighting factors, become increasingly apparent (Ishizaka and Labib 2009 ; Munier et al. 2021 ). This has led researchers to explore data-driven models, which have been noted for their broad application in assessing the susceptibility of large-scale geological disasters like landslides and floods (Li et al. 2023 ; Liu et al. 2023 ). Unlike traditional models, data-driven models can handle more complex datasets and ensure prediction accuracy through extensive data training (Jordan and Mitchell 2015 ; Sharifani and Amini 2023 ). Decision tree models, as an essential part of supervised learning, are favored for their ability to visually represent decision processes and ensure model stability (Kotsiantis 2013 ; Costa and Pedreira 2023 ). Among these models, CatBoost is particularly effective at handling categorical data without extensive preprocessing. Unlike other models such as XGBoost, its unique algorithm for dealing with categorical features prevents overfitting and improves prediction accuracy. Additionally, CatBoost uses ordered boosting to reduce target leakage and enhance generalization. Known for its fast training speed and efficiency with large datasets, CatBoost proves superior for our binary classification problem. These advantages enable more accurate and reliable predictions in assessing small-scale geological hazards in Hangzhou (Hancock and Khoshgoftaar 2020 ). Meanwhile, in geological evaluations, outcomes often represent just one aspect. The evaluation process and subsequent actions hold more significance in practical applications, leading to the underutilization of data-driven models in real-world scenarios due to their “black box” nature (Castelvecchi 2016 ; Rudin 2019 ). Thus, explainable models have become a crucial link between data-driven models and real-world decision making. Within the realm of explainable AI (XAI), SHAP (SHapley Additive exPlanations) emerges as a key tool (Van den Broeck et al. 2022 ). It treats each model feature as a player in a “game,” revealing the relationships between features or between features and the model (Marcílio and Eler 2020 ), enhancing the reliability of machine learning and facilitating urban decision makers in making informed plans. Although the application of machine learning interpretability model in urban hazard assessment has seen some exploration, it remains a relatively uncharted territory with significant potential for further development.
Our study introduced a novel CatBoost-SHAP model for the assessment of multi-hazards within the plain area of Hangzhou, marking its first application in evaluating urban geological hazards. The integration of the SHAP interpretability model into the multi-hazard analysis helps enhance the transparency and understanding of the model’s decision-making process, thus facilitating a deeper insight into the factors that influence geological hazards, which is designed to aid urban planners and policymakers by providing a comprehensive and interpretable tool for better informed decision making in urban development. In conclusion, by integrating SHAP-based multi-hazard susceptibility assessments with analyses of urban economic conditions and population dynamics, policymakers can more efficiently distribute resources and govern various sectors in harmony with the city’s development objectives. This approach also provides a replicable model of reference for other major cities around the world.
2 Background
In this section, we provide an overview of the study area and the geological hazard background. We also introduce the evaluation indicators selected for the susceptibility assessment. The data used in this study include two parts. The first part consists of evaluation indicators and the status of hazards, which were derived from the internal documents of the Hangzhou Urban Geological Safety Survey Project Footnote 1 by the China Geological Survey, Nanjing Center, and they are currently unavailable to the public. The second part consists of publicly accessible data, such as zoning and economic and population statistics for Hangzhou City. Although we obtained these data through collaboration with the Geological Survey of Zhejiang Province, they are also accessible via the Hangzhou City government website. Footnote 2
2.1 Study Area Overview
Hangzhou is in the eastern part of China, in the northern region of Zhejiang Province (Fig. 1 a). As of 2023, it has a permanent population of 12.522 million and its gross domestic product (GDP) has reached RMB 2005.9 billion yuan. The Hangzhou Plain area (Fig. 1 b), situated in the northeastern part of the city, covers a total area of 4381.3 m 2 , accounting for 26.4% of the city’s total area. Despite its size, this area contributes over 50% of Hangzhou’s GDP.
2.2 Geological Hazards
In this section, we describe the three primary geological hazards in the study area, encompassing their distribution characteristics, the nature of the hazards, and their impacts on the study region. This section provides a detailed overview of each hazard type, illustrating where these hazards are most prevalent and discussing their specific attributes and consequences.
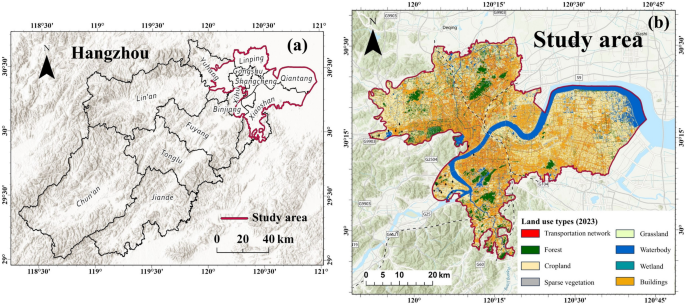
a Geographical location and district divisions of Hangzhou. b Location and land use of the study area
2.2.1 Land Subsidence
Between 2010 and 2021, the area in Hangzhou that experienced a cumulative land subsidence of more than 100 mm was approximately 8.4 km 2 , accounting for about 0.3% of the total area in the city. Moreover, over 80% of the total subsidence area in Hangzhou occurred within the study area. These subsidence areas are widely distributed within the study region, causing an average annual loss of over USD 1 billion. According to the Geological Survey of Zhejiang Province, the main areas affected include Xiaoshan District, Qiantang District, Linping District, and Xihu District, with the highest recorded subsidence reaching 127.5 mm within Xiaoshan District. The Hangzhou Plain is influenced by complex ancient topography and geomorphology, as well as drastic changes in ancient climate, multiple fluctuations in the sea level, and neotectonic movements since the Quaternary period. The region’s Quaternary sediments have undergone multiple cycles of accumulation and erosion, developing a complex set of terrestrial and marine-terrestrial interfacial sedimentary strata. The status of cumulative land subsidence of the study area is shown in Fig. 2 .
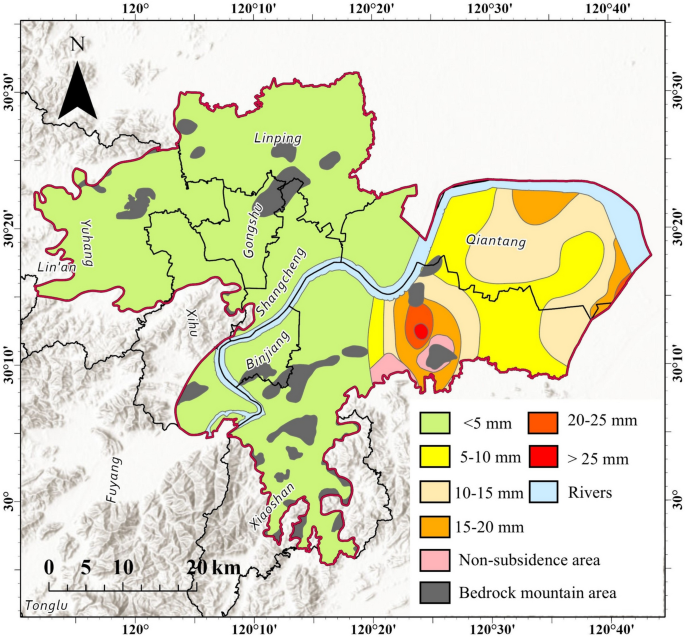
Current land subsidence map of the study area (2023)
2.2.2 Urban Road Collapse
Road collapses in Hangzhou City are primarily located in the Binjiang, Xihu, and Xiaoshan Districts. Over the past 20 years, there have been a total of 14 collapse incidents in these areas, resulting in 6 deaths and over USD 300 million in economic losses. Within the study area, several serious urban road collapse incidents have occurred. For instance, on 29 December 2020, a sudden road collapse occurred on the pedestrian path of the Qingfeng New Village section of Xixi Road in Xihu District, covering an area of about 25 m 2 and resulting in two fatalities. These areas are widely distributed with silty soil, sandy soil, soft soil, and hidden rivers, along with widespread artificial fill, making the geological conditions complex. Based on the analysis of 14 road collapse cases, the collapse depth was generally within the fill range or within 3 m of the boundary between the fill layer and the underlying strata, with a fill thickness of generally 1–5 m, and a collapse depth of generally 1–3 m. Artificial fill and silty soil are the main geological factors leading to road collapses in Hangzhou. The spatial distribution of road collapses is significantly correlated with the distribution of sandy soil layers, artificial fill, and underground utilities. The urban road collapses of the study area are shown in Fig. 3 .
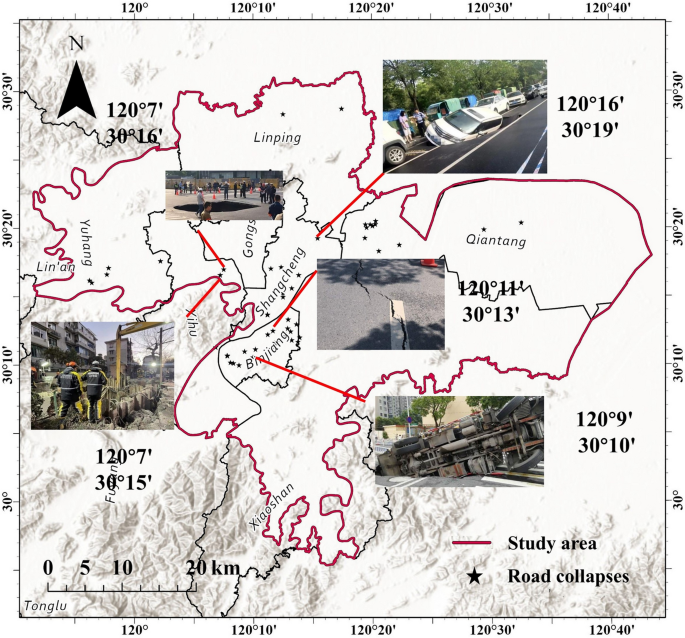
Urban road collapse map and field photos. The source of the field photographs is the internal documents of the Hangzhou Urban Geological Safety Survey Project (Project No. DD20190281).
2.2.3 Karst Collapse
Since records began in 1964, Hangzhou City has experienced 26 instances of karst collapse of varying sizes, primarily located in the Xihu area, with a total of 14 collapses. These incidents resulted in one fatality, two injuries, and caused uneven land subsidence, cracking, building damage, and building collapse, leading to significant economic losses. The occurrence of karst collapses is closely related to human activities such as water extraction, underground construction, and drainage. Geologically, the development of underground karst, with calcium oxide content generally above 45%, and limestone that is easily eroded by surface water and groundwater contribute to the complexity of hydrogeological conditions. Human engineering activities such as subway and tunnel construction are a major cause of karst collapse in the study area. The status of karst collapses of the study area is shown in Fig. 4 .
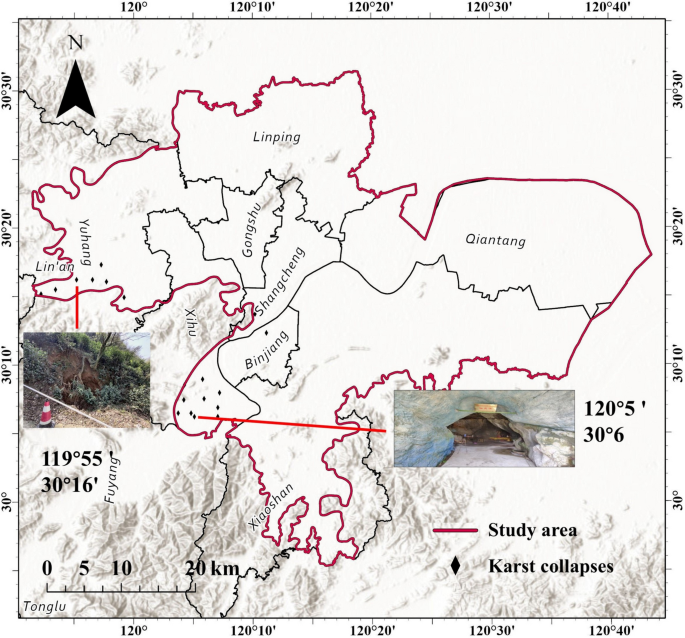
Karst collapse map and field photos. The source of the field photographs is the internal documents of the Hangzhou Urban Geological Safety Survey Project (Project No. DD20190281).
2.3 Evaluation Indicators
Following a comprehensive inspection of urban hazards in the study area by the Zhejiang Geological Survey and the China Geological Survey, Nanjing Center, the research identified that the urban geological hazards in the study area are primarily related to marine and river-lake soft soils, the abundance of groundwater, rainfall, and the disturbance of groundwater levels and strata by human engineering activities. Consequently, the 10 factors presented in Table 1 and Fig. 5 were selected as evaluation indicators for susceptibility mapping in this study. The ten factors were categorized into three groups based on their characteristics: hydrological conditions, geological conditions, and human activity intensities. Hydrological conditions include groundwater richness, the burial depth of the underground confined water level, and rainfall, which influence ground stability by altering underground waterflow and pressure (Miller and Sias 1998 ; Machowski et al. 2016 ). Geological conditions encompass factors that affect soil and rock stability, such as the burial depth of the top layer of saturated silty sand soil, thickness of the surface fill layer, karst and limestone distribution, thickness of the soft soil layer, and subsidence rate. These factors are directly related to the potential for land collapse or subsidence (Cui et al. 2015 ). Human activity intensities, primarily represented by the density of major linear projects, affect geological stability through increased ground load or alterations in underground water dynamics (Al-Qubatee et al. 2022 ; Zhang and Wang 2022 ). Table 2 presents the factors associated with different hazards and their categories.
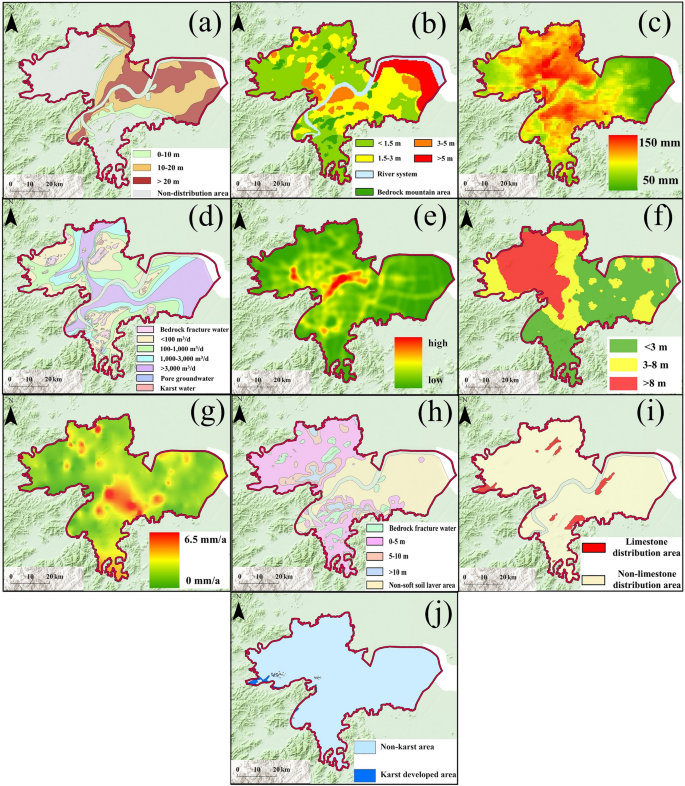
Multi-hazard risk evaluation indicators: a Burial depth of the top layer of saturated silty sand soil; b thickness of the surface fill layer; c rainfall; d groundwater richness; e major linear project density; f burial depth of the underground confined water level; g subsidence rate; h soft soil layer thickness; i limestone distribution; j Karst distribution
3 Methodology
In this section, we introduce the principles and advantages of the CatBoost model, as well as the parameters we used for training the model. We also explain the principles of the SHAP interpretability method.
3.1 Categorical Boosting (CatBoost) Model
Categorical Boosting distinguishes itself as an innovative gradient boosting algorithm, specifically engineered to enhance the handling of categorical data with unparalleled efficiency and accuracy (Prokhorenkova et al. 2018 ). This algorithm, developed in 2018, is achieved through a distinctive ensemble of decision trees constructed sequentially, utilizing an additive approach to progressively correct the residuals from preceding trees. The principles of the CatBoost algorithm are illustrated in Fig. 6 . Unique to CatBoost are two pivotal innovations: ordered boosting and direct categorical feature processing. Ordered boosting diverges from traditional boosting methods by leveraging a randomly selected data subset at each iteration for gradient learning, effectively minimizing overfitting and thereby augmenting the model’s generalizability (Hancock and Khoshgoftaar 2020 ; Ibrahim et al. 2020 ). Concurrently, CatBoost’s native processing of categorical variables circumvents the need for one-hot encoding, employing a specialized algorithm that calculates statistics on categorical features in relation to the target variable. The predictive model in CatBoost is formalized as Eq. 1 :
where \(\hat{y}_{i}\) denotes the predicted outcome for the \(i\) -th instance, \(f_{k}\) represents the decision function of the \(k\) -th tree, and \(k\) is the total number of trees in the ensemble. The objective function of CatBoost integrates a loss component and a regularization term to effectively control the model’s complexity, which is shown as Eq. 2 :
where \(l\) signifies the loss function, measuring the discrepancy between predicted values \(\hat{y}_{i}\) and actual targets \(y_{i}\) , and \(\Omega\) denotes the regularization term, imposing penalties on the model’s complexity to avert overfitting.
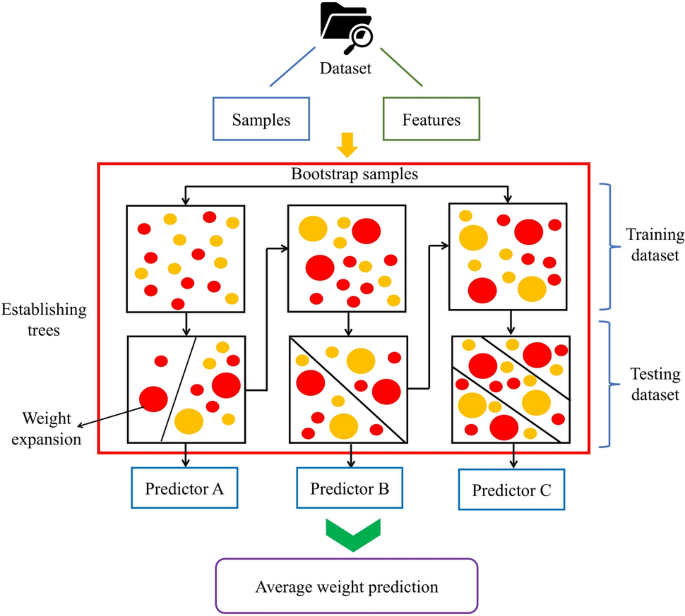
Schematic diagram of the CatBoost model
Before training the model, it is essential to meticulously prepare the data, ensuring that it is appropriately organized and clean (Brownlee 2020 ). Moreover, dividing the data into training and testing sets is imperative. The training set is used to train the model, allowing it to learn the underlying patterns within the data. In contrast, the testing set serves as a separate, unbiased dataset to evaluate the model’s performance and generalizability to unseen data. This division helps in minimizing overfitting and maximizing the model’s ability to make accurate predictions on new data (Storkey 2009 ; Medar et al. 2017 ). In our study, 70% of the data was allocated as the training set, with the remaining portion serving as the test set, which is a standard practice in machine learning for assessing various geological hazards (Tehrany et al. 2014 ). The evaluation indicators extracted using ArcGIS 10.8 software were delineated into “target” and “features,” where the target refers to hazard samples and the features are the evaluation factors. Within the framework of the training set, the model is tasked with discerning the intricate correlations between these “target” and “features” to facilitate accurate predictions (Bishop 2006 ). Distinct regions are annotated with binary values, 1 or 0, indicative of severe and mild urban geological hazards, respectively. Consequently, the model evaluates the probability of each area within the study domain being identified as severely impacted by hazards (denoted by a value of 1). The derived probabilities are then employed in susceptibility mapping, an established method for assessing susceptibility to geological hazards (Ganesh et al. 2023 ; Lyu and Yin 2023 ). During model training, to ensure reliable comparisons, the CatBoost model was configured with 100 trees (weak learners), and the maximum depth of each tree was limited to 3 to avert overfitting. The learning rate was fixed at 0.1. The prediction mechanism of the ensemble model aligned with that of the individual models.
3.2 SHapley Additive exPlanations (SHAP)
In the realm of machine learning, understanding the influence of individual features on model predictions is paramount. SHapley Additive exPlanations (SHAP), a method grounded in cooperative game theory, serves as a pivotal tool for this purpose. It employs the Shapley value concept to equitably attribute the outcome of a collaborative effort among features in a predictive model. SHapley Additive exPlanations elucidates how each feature contributes to shifting the model’s prediction away from a baseline value, typically the mean of the target values in the training set. The consistency principle is central to SHAP’s approach, which ensures that any change in a model’s dependency on a feature is accurately reflected in the SHAP values. This makes SHAP a reliable method for detailing the importance and effect of features on model predictions.
The computation of SHAP values considers the marginal contribution of a feature by examining all possible combinations of features. This is formally represented as Eq. 3 :
where \(F\) is the set of all features, \(S\) represents a subset of these features excluding feature \(j\) , \(f\left( S \right)\) is the model’s prediction with the subset \(S\) , and \(f\left( {S \cup \left\{ j \right\}} \right)\) is the prediction when feature \(j\) is included. This formula assesses the average impact of adding feature \(j\) to different combinations of features, highlighting its unique contribution.
This section presents the results from our analyses. In Sect. 4.1 , we demonstrate the process of evaluating model accuracy using the receiver operating characteristic (ROC) curves. In Sect. 4.2 , we display the susceptibility maps for individual hazards and multi-hazards in the study area. In Sect. 4.3 , we present the results and analysis of model interpretation using SHAP plots. In Sect. 4.4 , we provide a further analysis of the susceptibility results in relation to the current economic and demographic conditions of the study area.
4.1 Accuracy Assessment
After completing the predictions, the model’s accuracy was assessed using the area under the curve-receiver operating characteristic (ROC-AUC) metric, which serves as a pivotal metric for evaluating and comparing the efficacy of predictive models in geological hazards analysis (Pham et al. 2020 ; Wang et al. 2020 ). The AUC represents the integral of the ROC curve, offering a single scalar value between 0 and 1 to quantify model discrimination capacity, that is, how well the model can differentiate between positive and negative instances without being tied to a specific classification threshold. Ideal performance is indicated by an AUC of 1, implying perfect separation of classes, whereas an AUC of 0.5 suggests no better accuracy than random guessing. For this study, the ROC-AUC curve is shown in Fig. 7 . As indicated by the curve, the model demonstrates excellent performance in predicting all three types of hazards.
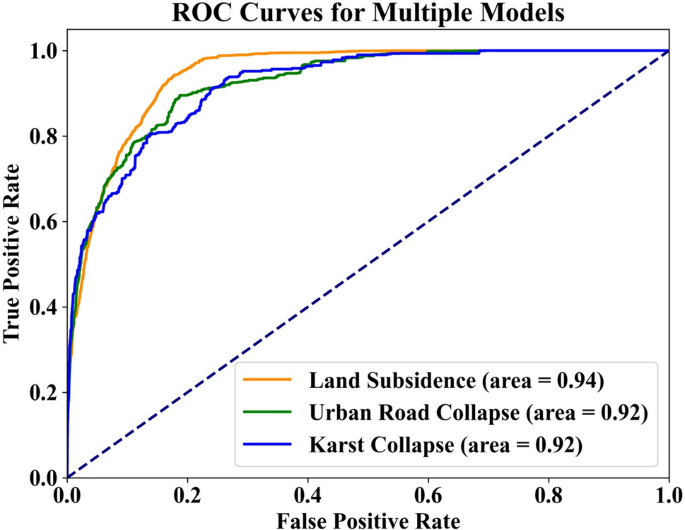
Area under the curve-receiver operating characteristic (ROC-AUC) curve
4.2 Susceptibility Mapping
Following the completion of model predictions, the training and testing datasets were combined. Subsequently, all datapoints were collected and integrated into the ArcGIS 10.8 software, facilitating the generation of susceptibility maps for the entire area under investigation. The likelihood of subsidence areas being allocated a value of 1 (within a range of 0 to 1) underwent reclassification via the equal interval method. This process delineated the study area into four distinct susceptibility categories: low (0–0.25), moderate (0.25–0.5), high (0.5–0.75), and very high susceptibility (0.75–1). In the single-hazard susceptibility map, Qiantang and Xiaoshan Districts are primarily susceptible to land subsidence. Binjiang, Xihu, and Xiaoshan Districts are mainly susceptible to road collapse, while karst areas, specifically West Lake District, along with sporadic distribution in other limestone-developed areas, are susceptible to karst collapse. The susceptibility mapping results show a high degree of overlap with the distribution of hazard points. The susceptibility mapping outcomes for each type of hazard are depicted in Fig. 8 , which shows the specific susceptibility zones and hazard incidents. Table 2 includes their respective shares of the total study area and further details.
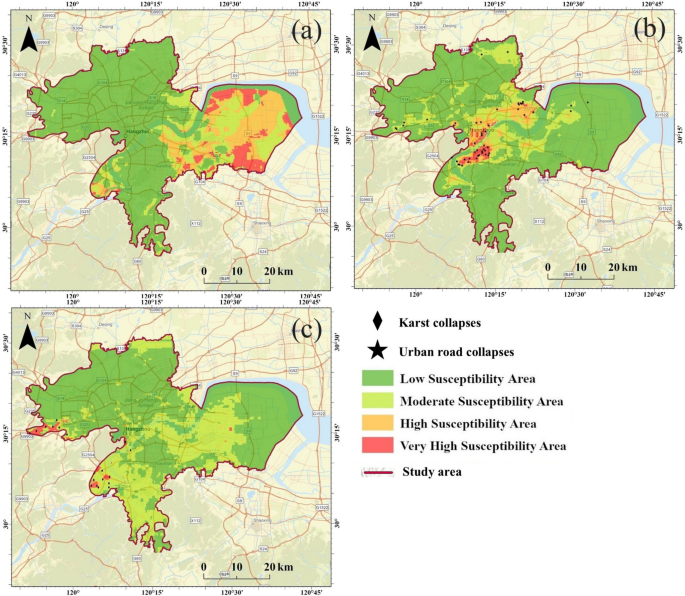
Susceptibility maps. a Land subsidence; b road collapse; c Karst collapse.
Although the susceptibility map of a single hazard can effectively show the likelihood of different areas in the study area being affected by a particular hazard, from the perspective of the city as a whole, the same area may be subjected to multiple hazards. For urban decision makers, zoning the city based on the hazard characteristics of different areas can save resources while facilitating efficient governance. To obtain a comprehensive susceptibility map of the study area, based on the susceptibility classification discussed earlier, we compiled data on areas of high and very high susceptibility, overlaying the susceptibility scenarios of different hazards. According to the analysis, 73.71% of the study area does not fall within the high susceptibility zones for urban geological hazards, with only a low proportion of small-scale hazards such as collapses. The detailed comprehensive susceptibility zoning map and the proportion of different conditions are presented in Fig. 9 .
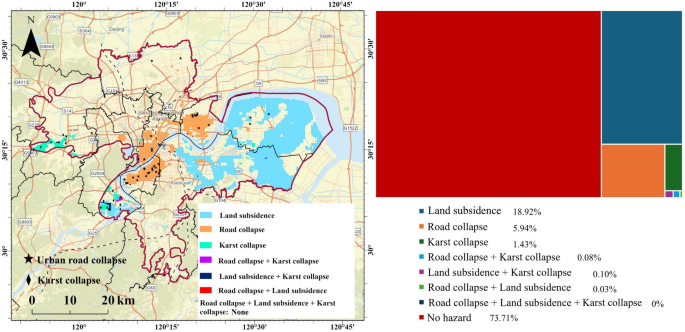
A multi-hazard susceptibility map of Hangzhou City
4.3 Model Interpretability
The SHAP plots, as a way to showcase SHAP’s interpretability, come in three varieties—force, summary, and decision plots—each offering a unique way to visualize and understand the data and the model’s behavior without delving into their specific functions (Yang et al. 2022 ). The force plot (Fig. 10 ), presented from the micro perspective of a specific sample point, visualizes how different features impact the model’s prediction of land subsidence, road collapse, and karst collapse. The length and direction of each arrow represent the strength and direction of each feature’s effect. A feature with a red arrow contributes to an increase in the prediction value, while a blue arrow denotes a feature that decreases the prediction value. For example, in the case of land subsidence, the contribution of “Rainfall” (c) corresponds to the portion indicated by the black curly brace in Fig. 10 . When the contribution of a feature is negligible, the program may not label its contribution on the graph. For instance, this is the case for “Groundwater richness” (d) in land subsidence, as well as “Rainfall” (c) and “Burial depth of the underground confined water level” (f) in karst collapse. Each plot starts with a base value (the average prediction over the dataset), and the combined effect of all the features leads to the final prediction ( f ( x )) at the end of the plot. Each sample point has a different f ( x ). To interpret the plot, one observes the balance of red and blue arrows, their lengths, and the final prediction point relative to the base value, which together convey the cumulative effect of all features for the specific prediction. Also, the numbers indicated below the factors in the figure represent the transformed or standardized values of the sample data used in the model’s prediction process and do not have a specific inherent meaning.
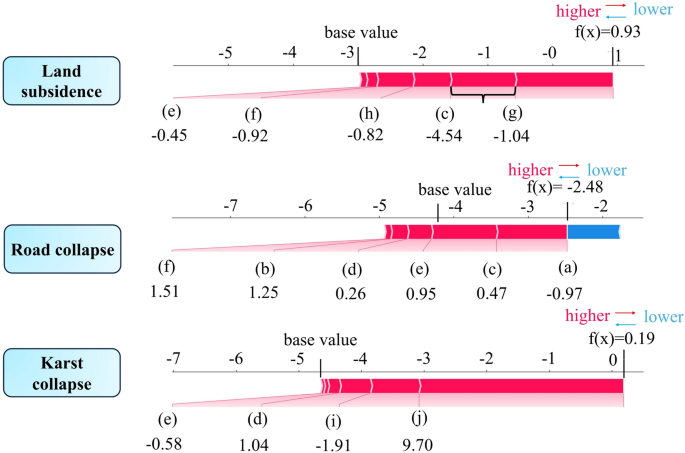
SHapley Additive exPlanations (SHAP) force plot of the test dataset. a Burial depth of the top layer of saturated silty sand soil; b thickness of the surface fill layer; c rainfall; d groundwater richness; e major linear project density; f burial depth of the underground confined water level; g subsidence rate; h soft soil layer thickness; i limestone distribution; j Karst distribution
Figure 10 shows that in the land subsidence analysis of this sample, each relevant factor contributed positively to the model’s prediction, especially “Subsidence rate” (g). In the road collapse analysis, all relevant factors, except for “Burial depth of the top layer of saturated silty sand soil” (a), contributed positively. Finally, in the karst collapse analysis, all relevant factors, particularly “Karst distribution” (j), made significant contributions to the model’s prediction.
Unlike the force plot that analyzes the individual prediction of a specific sample, the summary plot in Fig. 11 displays the distribution and average impact of each feature across all observations. On the vertical axis, the order of the factors from top to bottom represents a decreasing importance of factors in the model’s prediction. The horizontal axis shows the SHAP values for each sample point, with each point representing a sample. Wider sections along the horizontal axis indicate a higher concentration of samples. In terms of the color of the points, the redder the color, the higher the feature value; the bluer the color, the lower the feature value. For example, in the land subsidence analysis, overall, “Subsidence rate” (g) contributes the most to the model’s prediction, while “Major linear project density” (e) contributes the least. In the road collapse analysis, there is a large concentration of low feature values (near −1) for “Major linear project density” (e), and the maximum feature value for this factor is around 2, which indicates relatively high feature values.

SHapley Additive exPlanations (SHAP) summary plot of the test dataset. a Burial depth of the top layer of saturated silty sand soil; b thickness of the surface fill layer; c rainfall; d groundwater richness; e major linear project density; f burial depth of the underground confined water level; g subsidence rate; h soft soil layer thickness; i limestone distribution; j Karst distribution
The decision plot in Fig. 12 captures the influence of discriminative features on the model’s predictions across 500 individual samples for land subsidence, road collapse, and karst collapse scenarios. Each line traces a journey from a baseline value, without any feature impact, to one where all six relevant features have shaped the outcome, that is, the final prediction value (the features selected for each type of hazard are shown in Table 1 ). The vertical axis ranks the factors from top to bottom based on their overall contribution to the model’s prediction, consistent with the vertical axis of the summary plot. The color of each line corresponds to the magnitude of the final prediction value—the redder the line, the higher the value; the bluer the line, the lower the value. For example, in Line 1 of the road collapse scenario, the line is purple, corresponding to a final prediction value of approximately − 4, with significant fluctuations due to the influence of “Rainfall” on this sample. These plots illustrate the cumulative contribution of factors to the model’s output, with the final position of each line on the right signifying the integrated effect of the factors.
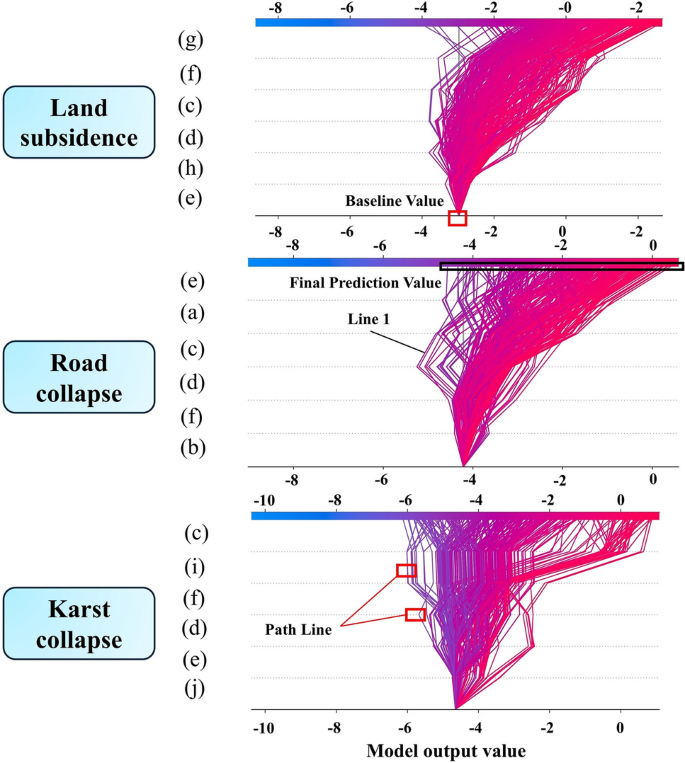
SHapley Additive exPlanations (SHAP) decision plot of the test dataset. a Burial depth of the top layer of saturated silty sand soil; b thickness of the surface fill layer; c rainfall; d groundwater richness; e major linear project density; f burial depth of the underground confined water level; g subsidence rate; h soft soil layer thickness; i limestone distribution; j Karst distributionn.
Baseline Value: The starting point of every path line in the graph, which represents the model’s expected output without any input features; Path Line: Each line represents the prediction process for a data point, starting from the baseline value and ending at the actual output value of the model; Final Prediction Value: The endpoint of each line, indicating the model’s final predicted output after considering all features.
4.4 Socioeconomic Comprehensive Mapping
Figure 13 shows Hangzhou’s per capita GDP in 2023 and population density. Integrating urban development levels into multi-hazard management can yield superior outcomes. This method ensures that resources are allocated efficiently, focusing on areas where the impact of disasters on human lives and economic assets is likely to be greatest. Such an approach aligns more closely with the principles of sustainable development, which call for the harmonious progression of socioeconomic factors, population, and the environment.
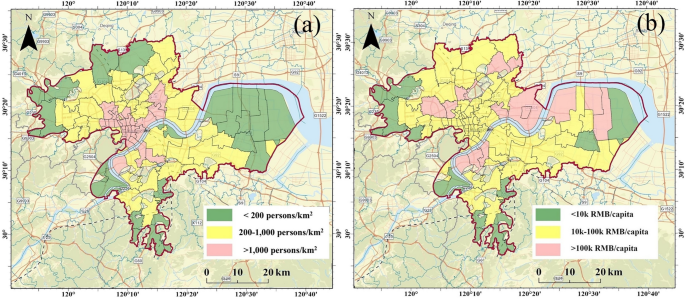
Status of population density and GDP in Hangzhou, 2023. a Population density (persons/km 2 ); b GDP (RMB yuan per capita).
In our research, we overlaid the comprehensive assessment results of urban geological hazards with per capita GDP and population density, given that GDP and population density may not always be directly proportional (Hou et al. 2016 ; Pradhan et al. 2023 ). According to our mapping outcomes, Binjiang and Shangcheng Districts not only exhibit high population density and per capita GDP but also show a high susceptibility to urban road collapse, necessitating a prioritized allocation of resources towards monitoring and mitigation efforts in these areas. Meanwhile, along the banks of the Qiantang River in Qiantang District, despite the lower population density, the area’s highly developed economy calls for significant attention to land subsidence prevention and control. Further specifics are presented in Fig. 14 .
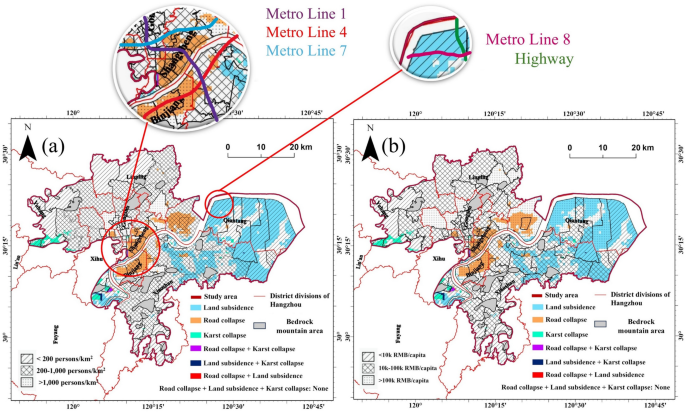
Susceptibility map of multiple hazards combined with population density and GDP data in Hangzhou City in 2023. a Multiple hazards and population density (persons/km 2 ); b Multiple hazards and GDP (RMB yuan per capita). Hazard and population/GDP details, and metro lines and railway are also shown in the enlarged maps of Binjiang and Shangcheng Districts and Qiantang District.
5 Discussion
In this study, we proposed a CatBoost-SHAP model tailored for the Hangzhou Plain area, which is characterized by complex geological conditions, significant human activity impact, and dispersed hazard zones. During the prediction phase, the CatBoost algorithm demonstrated exceptional performance with AUC values of 0.92, 0.92, and 0.94 for three distinct types of hazards, approaching the perfect accuracy mark of 1. However, despite the high AUC scores, the underlying principles of the model’s judgments remained obscure, making it challenging for urban decision makers to effectively manage and mitigate risks based on the analysis. To address this, our research integrated the well-developed SHAP interpretability model. Through the examination of various SHAP visualizations and the factor weight distribution graph in Fig. 15 , we identified that hydrological factors such as “Rainfall” and “Burial depth of the underground confined water level” universally and significantly influence the occurrence of urban geological hazards. The model’s predictive analysis also exhibited variability, likely due to dispersed data distribution, necessitating further in-depth discussion in future research. Notably, “Thickness of the soft soil layer,” a unique factor in road collapse scenarios, held a substantial weight in the analysis, aligning with the primary cause of road subsidence in Hangzhou being the inadequate bearing capacity of the surface fill soil. This correlation further validates the accuracy of our study and analysis. The proposed CatBoost-SHAP model excels in prediction and offers reasonable explanations for its outputs, demonstrating its application potential. As research progresses, our team will continue to advocate for its broader application.
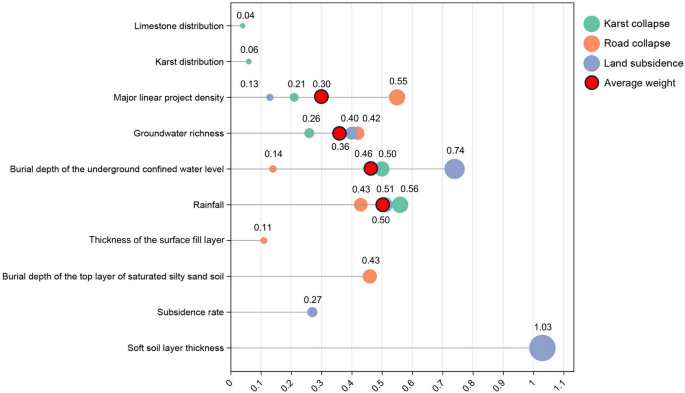
Factor weight chart based on SHapley Additive exPlanations (SHAP) values
Our research also provides new directions for disaster prevention in Hangzhou City. Specifically, for areas like Binjiang and Qiantang Districts, where urban road collapse is prevalent, further monitoring of underground voids is recommended. For the significantly impactful factor of “Soft soil layer thickness,” further borehole data collection in road collapse-prone areas is advised to gain clearer insights into the stratigraphic conditions. Regarding hydrological conditions and other factors examined in the study, the construction of a groundwater monitoring network and further expansion of the land subsidence monitoring network are necessary to provide technical support for the smart city construction of Hangzhou City. To address the issue of karst collapse, it is crucial to enhance the identification of hidden adverse geological conditions such as concealed karst and ancient river channels, especially around the West Lake area, by intensifying geophysical exploration efforts.
This research, based on geological data and simulations, aimed to guide further geological investigations with its accurate results. It reaffirmed the directive role of integrating geological science with computer technology in actual sustainable urban construction, offering valuable experience for cities worldwide facing similar challenges with multiple urban geological hazards. This translation emphasizes the practical application of interdisciplinary approaches in enhancing urban sustainability, reflecting the significance of tailored geological and technological interventions in mitigating the risks associated with urban development.
6 Conclusion
To align urban construction for sustainable development with the safety of people’s lives and property in Hangzhou City, this study introduced a CatBoost-SHAP model for multi-geological hazards assessment in the plain area of the city. This marks the first application of the well-known CatBoost model in the field of dispersed geological hazards in urban areas, where it has demonstrated exceptional performance with AUC values of 0.92, 0.92, and 0.94 for three types of hazards. Upon validating the accuracy of the results, a susceptibility map for each hazard was created, along with a multi-hazard susceptibility map for the study area. The comparison of hazard point distribution and the mapping results also confirmed the effectiveness of our approach.
The primary aim of this research was to apply our findings to support sustainable development initiatives in Hangzhou City. To assist decision makers in urban planning and to increase the credibility of the research, we innovatively incorporated the SHAP interpretability model. By opening the “black box” of the CatBoost, the impact and contribution of each factor to the modeling results were clearly determined. This undoubtedly boosts the confidence of urban policymakers in applying machine learning.
By overlaying the susceptibility of multi-hazards with the urban economic and population situation, decision makers can allocate resources and manage different regions more effectively in line with urban development. We believe that this approach has significant implications for balancing economic development, social inclusivity, and environmental protection in the process of sustainable urban development.
Project No. DD20190281.
https://tjj.hangzhou.gov.cn/ .
Alikaei, S., M. Rahmani, F. Jamalabadi, M.E. Akdogan, and S. Khoshnevis. 2023. Multi-hazard-based land use planning in isolated area; Learning from the experience of Pule-Khumri City, Afghanistan. Sustainable Cities and Society 99: Article 104873.
Al-Qubatee, W., F.A. Hasan, H. Ritzema, G. Nasher, and P. Hellegers. 2022. Natural and human-induced drivers of groundwater depletion in Wadi Zabid, Tihama coastal plain, Yemen. Journal of Environmental Planning and Management 65(14): 2609–2630.
Article Google Scholar
Bagheri-Gavkosh, M., S.M. Hosseini, B. Ataie-Ashtiani, Y. Sohani, H. Ebrahimian, F. Morovat, and S. Ashrafi. 2021. Land subsidence: A global challenge. Science of the Total Environment 778: Article 146193.
Bishop, C.M. 2006. Pattern recognition and machine learning . New York: Springer.
Google Scholar
Brownlee, J. 2020. Data preparation for machine learning: Data cleaning, feature selection, and data transforms in Python. https://github.com/aaaastark/Data-Scientist-Books/blob/main/Data%20Preparation%20for%20Machine%20Learning%20Data%20Cleaning%2C%20Feature%20Selection%2C%20and%20Data%20Transforms%20in%20Python%20by%20Jason%20Brownlee%20(z-lib.org).pdf . Accessed 15 Jun 2024.
Castelvecchi, D. 2016. Can we open the black box of AI?. Nature 538(7623): 21–23.
Chen, F., H. Lin, Y. Zhang, and Z. Lu. 2012. Ground subsidence geo-hazards induced by rapid urbanization: Implications from InSAR observation and geological analysis. Natural Hazards and Earth System Sciences 12(4): 935–942.
Chen, Y., J. Song, S. Zhong, Z. Liu, and W. Gao. 2022. Effect of destructive earthquake on the population-economy-space urbanization at county level – A case study on Dujiangyan County, China. Sustainable Cities and Society 76: Article 103345.
Costa, V.G., and C.E. Pedreira. 2023. Recent advances in decision trees: An updated survey. Artificial Intelligence Review 56(5): 4765–4800.
Cui, Z.-D., J.-Q. Yang, and L. Yuan. 2015. Land subsidence caused by the interaction of high-rise buildings in soft soil areas. Natural Hazards 79(2): 1199–1217.
De Waele, J., F. Gutiérrez, M. Parise, and L. Plan. 2011. Geomorphology and natural hazards in karst areas: A review. Geomorphology 134(1–2): 1–8.
Etinay, N., C. Egbu, and V. Murray. 2018. Building urban resilience for disaster risk management and disaster risk reduction. Procedia Engineering 212(6): 575–582.
Ganesh, B., S. Vincent, S. Pathan, and S.R. Garcia Benitez. 2023. Machine learning based landslide susceptibility mapping models and GB-SAR based landslide deformation monitoring systems: Growth and evolution. Remote Sensing Applications: Society and Environment 29(19): Article 100905.
Godschalk, D.R. 2003. Urban hazard mitigation: Creating resilient cities. Natural Hazards Review 4(3): 136–143.
Gray, M.A. 1990. The United Nations Environment Programme: An assessment. Environmental Law 20(2): Article 291.
Gu, H., S. Du, B. Liao, J. Wen, C. Wang, R. Chen, and B. Chen. 2018. A hierarchical pattern of urban social vulnerability in Shanghai, China and its implications for risk management. Sustainable Cities and Society 41: 170–179.
Gutiérrez, F., M. Parise, J. De Waele, and H. Jourde. 2014. A review on natural and human-induced geohazards and impacts in karst. Earth-Science Reviews 138: 61–88.
Haghiri, M., N. Raeisi, R. Azizi, K. Shabani, and M. Ghadiri. 2024. Evaluation of karst aquifer development and karst water resource potential using fuzzy logic model (FAHP) and analysis hierarchy process (AHP): A case study, North of Iran. Carbonates and Evaporites 39(2). https://doi.org/10.1007/s13146-024-00925-w .
Hancock, J.T., and T.M. Khoshgoftaar. 2020. CatBoost for big data: An interdisciplinary review. Journal of Big Data 7(1): Article 94.
Hou, J., J. Lv, X. Chen, and S. Yu. 2016. China’s regional social vulnerability to geological disasters: Evaluation and spatial characteristics analysis. Natural Hazards 84(1): 97–111.
Hu, R.L., Z.Q. Yue, L.C. Wang, and S.J. Wang. 2004. Review on current status and challenging issues of land subsidence in China. Engineering Geology 76(1–2): 65–77.
Ibrahim, A.A., R.L. Ridwan, M.M. Muhammed, R.O. Abdulaziz, and G.A. Saheed. 2020. Comparison of the CatBoost classifier with other machine learning methods. International Journal of Advanced Computer Science and Applications 11(11): 738–748.
Ishizaka, A., and A. Labib. 2009. Analytic hierarchy process and expert choice: Benefits and limitations. OR Insight 22(4): 201–220.
Jordan, M.I., and T.M. Mitchell. 2015. Machine learning: Trends, perspectives, and prospects. Science 349(6245): 255–260.
Article CAS Google Scholar
Kotsiantis, S.B. 2013. Decision trees: A recent overview. Artificial Intelligence Review 39(4): 261–283.
Li, Y., F.B. Osei, T. Hu, and A. Stein. 2023. Urban flood susceptibility mapping based on social media data in Chengdu City, China. Sustainable Cities and Society 88: Article 104307.
Liu, H., G. Zhou, R. Wennersten, and B. Frostell. 2014. Analysis of sustainable urban development approaches in China. Habitat International 41: 24–32.
Liu, S., L. Wang, W. Zhang, Y. He, and S. Pijush. 2023. A comprehensive review of machine learning-based methods in landslide susceptibility mapping. Geological Journal 58(6): 2283–2301.
Lu, H., X. Lu, L. Jiao, and Y. Zhang. 2022. Evaluating urban agglomeration resilience to disaster in the Yangtze Delta city group in China. Sustainable Cities and Society 76: Article 103464.
Lyu, H.-M., and Z.-Y. Yin. 2023. An improved MCDM combined with GIS for risk assessment of multi-hazards in Hong Kong. Sustainable Cities and Society 91: Article 104427.
Machowski, R., M.A. Rzetala, M. Rzetala, and M. Solarski. 2016. Geomorphological and hydrological effects of subsidence and land use change in industrial and urban areas. Land Degradation & Development 27(7): 1740–1752.
Marcílio, W.E., and D.M. Eler. 2020. From explanations to feature selection: Assessing SHAP values as feature selection mechanism. In Proceedings of the 2020 33rd SIBGRAPI Conference on Graphics, Patterns and Images (SIBGRAPI) , 7–10 November 2020, Porto de Galinhas, Brazil, 340–347.
Medar, R., V.S. Rajpurohit, and B. Rashmi. 2017. Impact of training and testing data splits on accuracy of time series forecasting in machine learning. In Proceedings of the 2017 International Conference on Computing, Communication, Control and Automation (ICCUBEA) , 17–18 August 2017, Pune, India, 1–6.
Miller, D.J., and J. Sias. 1998. Deciphering large landslides: Linking hydrological, groundwater and slope stability models through GIS. Hydrological Processes 12(6): 923–941.
Munier, N., E. Hontoria, N. Munier, and E. Hontoria. 2021. Shortcomings of the AHP method. In Uses and limitations of the AHP method: A non-mathematical and rational analysis , ed. N. Munier, and E. Hontoria, 41–90. Cham: Springer.
Papadopoulou-Vrynioti, K., G.D. Bathrellos, H.D. Skilodimou, G. Kaviris, and K. Makropoulos. 2013. Karst collapse susceptibility mapping considering peak ground acceleration in a rapidly growing urban area. Engineering Geology 158: 77–88.
Pham, B.T., T. Nguyen-Thoi, C. Qi, T. Van Phong, J. Dou, L.S. Ho, H. Van Le, and I. Prakash. 2020. Coupling RBF neural network with ensemble learning techniques for landslide susceptibility mapping. Catena 195: Article 104805.
Pourghasemi, H.R., A. Gayen, M. Edalat, M. Zarafshar, and J.P. Tiefenbacher. 2020. Is multi-hazard mapping effective in assessing natural hazards and integrated watershed management?. Geoscience Frontiers 11(4): 1203–1217.
Pradhan, B., S. Lee, A. Dikshit, and H. Kim. 2023. Spatial flood susceptibility mapping using an explainable artificial intelligence (XAI) model. Geoscience Frontiers 14(6): Article 101625.
Prokhorenkova, L., G. Gusev, A. Vorobev, A.V. Dorogush, and A. Gulin. 2018. CatBoost: Unbiased boosting with categorical features. In Proceedings of the 32nd Conference on Neural Information Processing Systems (NeurIPS 2018) , 3–8 December 2018, Montréal, Canada. https://proceedings.neurips.cc/paper_files/paper/2018/file/14491b756b3a51daac41c24863285549-Paper.pdf . Accessed 13 Jul 2023.
Redclift, M. 1989. The environmental consequences of Latin America’s agricultural development: Some thoughts on the Brundtland Commission report. World Development 17(3): 365–377.
Rudin, C. 2019. Stop explaining black box machine learning models for high stakes decisions and use interpretable models instead. Nature Machine Intelligence 1(5): 206–215.
Sharifani, K., and M. Amini. 2023. Machine learning and deep learning: A review of methods and applications. World Information Technology and Engineering Journal 10(7): 3897–3904.
Shi, P., X. Yang, W. Xu, and J. Wang. 2016. Mapping global mortality and affected population risks for multiple natural hazards. International Journal of Disaster Risk Science 7(1): 54–62.
Shi, P., T. Ye, Y. Wang, T. Zhou, W. Xu, J. Du, J. Wang, and N. Li et al. 2020. Disaster risk science: A geographical perspective and a research framework. International Journal of Disaster Risk Science 11(4): 426–440.
Shirzaei, M., J. Freymueller, T.E. Törnqvist, D.L. Galloway, T. Dura, and P.S.J. Minderhoud. 2021. Measuring, modelling and projecting coastal land subsidence. Nature Reviews Earth & Environment 2(1): 40–58.
Storkey, A. 2009. When training and test sets are different: Characterizing learning transfer . In Dataset shift in machine learning , ed. J. Quiñonero-Candela, M. Sugiyama, A. Schwaighofer, and N.D. Lawrence. Cambridge, MA: The MIT Press.
Sun, D. 2024. Land subsidence susceptibility mapping in urban settlements using time-series PS-InSAR and random forest model. Gondwana Research 125: 406–424.
Tehrany, M.S., B. Pradhan, and M.N. Jebur. 2014. Flood susceptibility mapping using a novel ensemble weights-of-evidence and support vector machine models in GIS. Journal of Hydrology 512: 332–343.
Van den Broeck, G., A. Lykov, M. Schleich, and D. Suciu. 2022. On the tractability of SHAP explanations. Journal of Artificial Intelligence Research 74: 851–886.
Wang, Y., S. Li, X. Liu, J. Zhang, and W. Cheng. 2020. Comparative study of landslide susceptibility mapping with different recurrent neural networks. Computers & Geosciences 138: Article 104445.
Wang, K., J. Zhang, G. Gao, J. Qiu, Y. Zhong, C. Guo, W. Zhao, K. Tang, and X. Su. 2022. Causes, risk analysis, and countermeasures of urban road collapse in China from 2019 to 2020. Journal of Performance of Constructed Facilities 36(6): Article 04022054.
Wang, Y., Y. Qiao, W. Deng, F. Wang, W. Bai, J. Jiang, J. Liu, S. Xu, et al. 2023. Construction of an urban road collapse risk assessment model and its case study in Guangzhou. In Computational and experimental simulations in engineering: Proceedings of the International Conference on Computational & Experimental Engineering and Sciences 2023 , ed. S. Li, 269–290. Cham: Springer.
Wang, J., and D. He. 2015. Sustainable urban development in China: Challenges and achievements. Mitigation and Adaptation Strategies for Global Change 20(5): 665–682.
Wang, X.-W., and Y.-S. Xu. 2022. Investigation on the phenomena and influence factors of urban ground collapse in China. Natural Hazards 113(1): 1–33.
Wei, A., D. Li, Y. Zhou, Q. Deng, and L. Yan. 2021. A novel combination approach for karst collapse susceptibility assessment using the analytic hierarchy process, catastrophe, and entropy model. Natural Hazards 105(1): 405–430.
Wu, Y., X. Jiang, Z. Guan, W. Luo, and Y. Wang. 2018. AHP-based evaluation of the karst collapse susceptibility in Tailai Basin, Shandong Province, China. Environmental Earth Sciences 77: 1–14.
Yan, G., D. Lu, S. Li, S. Liang, L. Xiong, and G. Tang. 2024. Optimizing slope unit-based landslide susceptibility mapping using the priority-flood flow direction algorithm. Catena 235: Article 107657.
Yang, Y., Y. Yuan, Z. Han, and G. Liu. 2022. Interpretability analysis for thermal sensation machine learning models: An exploration based on the SHAP approach. Indoor Air 32(2): Article e12984.
Yu, L., Y. Wang, and B. Pradhan. 2024. Enhancing landslide susceptibility mapping incorporating landslide typology via stacking ensemble machine learning in Three Gorges Reservoir, China. Geoscience Frontiers 15(4): Article 101802.
Zhang, Z., and Y. Li. 2020. Coupling coordination and spatiotemporal dynamic evolution between urbanization and geological hazards - A case study from China. Science of the Total Environment 728: Article 138825.
Zhang, H., and Z. Wang. 2022. Human activities and natural geographical environment and their interactive effects on sudden geologic hazard: A perspective of macro-scale and spatial statistical analysis. Applied Geography 143: Article 102711.
Zhou, Y., T. Wu, and Y. Wang. 2022. Urban expansion simulation and development-oriented zoning of rapidly urbanising areas: A case study of Hangzhou. Science of the Total Environment 807: Article 150813.
Zhou, N.-Q., and S. Zhao. 2013. Urbanization process and induced environmental geological hazards in China. Natural Hazards 67: 797–810.
Download references
Acknowledgments
This research is supported by the China Geological Survey, Nanjing Center, Zhejiang Geological Survey, and China University of Geosciences (Wuhan). The work described in this article was funded by the Laboratory of Geological Safety of Underground Space in Coastal Cities, Ministry of Natural Resources (Project No. BHKF2022Z02), and the China Geological Survey, Nanjing Center (Project No. DD20190281).
Author information
Authors and affiliations.
The Institute of Geological Survey of China University of Geosciences (Wuhan), China University of Geosciences (Wuhan), Wuhan, 430074, China
Bofan Yu, Jiaxing Yan & Yunan Li
China Geological Survey, Nanjing Center, Nanjing, 210016, China
Huaixue Xing
You can also search for this author in PubMed Google Scholar
Corresponding author
Correspondence to Huaixue Xing .
Rights and permissions
Open Access This article is licensed under a Creative Commons Attribution 4.0 International License, which permits use, sharing, adaptation, distribution and reproduction in any medium or format, as long as you give appropriate credit to the original author(s) and the source, provide a link to the Creative Commons licence, and indicate if changes were made. The images or other third party material in this article are included in the article's Creative Commons licence, unless indicated otherwise in a credit line to the material. If material is not included in the article's Creative Commons licence and your intended use is not permitted by statutory regulation or exceeds the permitted use, you will need to obtain permission directly from the copyright holder. To view a copy of this licence, visit http://creativecommons.org/licenses/by/4.0/ .
Reprints and permissions
About this article
Yu, B., Yan, J., Li, Y. et al. Risk Assessment of Multi-Hazards in Hangzhou: A Socioeconomic and Risk Mapping Approach Using the CatBoost-SHAP Model. Int J Disaster Risk Sci 15 , 640–656 (2024). https://doi.org/10.1007/s13753-024-00578-2
Download citation
Accepted : 12 August 2024
Published : 22 August 2024
Issue Date : August 2024
DOI : https://doi.org/10.1007/s13753-024-00578-2
Share this article
Anyone you share the following link with will be able to read this content:
Sorry, a shareable link is not currently available for this article.
Provided by the Springer Nature SharedIt content-sharing initiative
- Hangzhou city
- Multi-hazard risk assessment
- Machine learning
- Machine learning interpretability
- Socioeconomic analysis
- Urban sustainability development
- Find a journal
- Publish with us
- Track your research
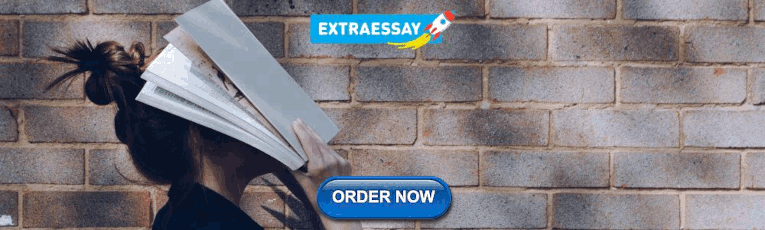
COMMENTS
Revision notes on 1.3.2 Multiple-Hazard Zone Case Study for the Edexcel A Level Geography syllabus, written by the Geography experts at Save My Exams.
The Philippines - Multi-hazard Case Study. Its geographical position on the globe means its highly prone to natural disasters. The Philippines is on the western rim of the 'Pacific Ring of Fire' which brings both earthquakes and volcanic activity. The Philippines is a country off the mainland southeast Asia consisting of over 7,000 islands.
The Philippines Hazard Hotspot Case StudyThe. Philippines Hazard Hotspot Case Study The Philippines is a group of 7000 islands in. he South China Sea in South East Asia. It has a vulnerable population of 98 million people who are at risk from a variety of hazards, including 11 million people in the densely populated coastally s.
This case study draws on the PDRF experience around the triple whammy scenario in 2020 descibed above and answers the question "How does the Philippine Disaster Resilience Foundation EOC respond to multiple disaster scenarios?"
The Philippines is one of the largest island groups in the world with 7,107 islands and islets (OCD-DND, 208). The country is exposed to multiple hazards - at least 60 per cent of the total land ...
Study with Quizlet and memorize flashcards containing terms like What and where are the Philippines?, Why is the area naturally vulnerable to hazards?, When did Mt Pinatubo erupt? and more.
Lesson 12 of 18. Includes: Practise question starter with model answer. Multiple hazard zones. Vanuatu recap quiz. The Philippines case study. How hazards become disasters: Hydrometeorological disasters: Tropical storms, flooding, landslides. Volcanic disasters: eruptions, pyroclastic flow, mudslides.
A case study booklet for the Phillipines - this meets the requirement for the multi-hazardous case study element for the Natural Hazards unit. It gives pupils a structured way to research and develop their case study notes.
Revision notes on The Philippines as a multi-hazardous environment for AQA's A Level Geography course, focusing on major hazards (earthquakes, volcanoes, tropical storms, landslides and tsunamis), vulnerability and management.
Study with Quizlet and memorize flashcards containing terms like What boundary does it sit on? (Philippines), What ocean does it's northern and eastern coast face? Why is this a problem? (Philippines), What other problems does it's position cause? (Philippines) and more.
Study with Quizlet and memorize flashcards containing terms like Where is the Philippines?, How many people live in the Philippines?, How large is the Philippines economy? and more.
Take a look at our interactive learning Mind Map about Multi-Hazard Environment: The Philippines, or create your own Mind Map using our free cloud based Mind Map maker.
The Philippines - multi-hazard environment The Philippines is a group of 7,000 islands in the South China Sea in South East Asia. The islands are at latitudes 5-20°N of the equator. It has a vulnerable population of 98 million people who are at risk from a variety of hazards, including 11 million people in the densely populated coastally situated megacity of Manila, the capital.
Multiple Hazard Zones - The Philippines. Consists of 7,000 islands (many are very small) spread across the typhoon belt. Its northern and eastern coasts face the pacific which is the worlds most TSUNAMI -PRONE ocean. The Philippines lies of the boundary between the Philippine and Eurasian Plate boundary. The Eurasian plate is forced beneath the ...
Study with Quizlet and memorize flashcards containing terms like What is a Multiple Hazard Zone?, What is the Philippines' Population Size?, GDP per Capita: and more.
1.7C - Multiple Hazard Zones. The concept of a multiple-hazard zone, and how linked hydrometeorological hazards sometimes contribute to a tectonic disaster. (Philippines) Multiple hazard zones are places where two or more natural hazards occur, and in some cases interact to produce complex disasters. Examples are California, Indonesia and Japan.
Philippines - multiple hazard zone. background. group of 7,000 islands in theSouth China Sea in South East Asia - islands are at latitudes 5-20°N of the equator. ... Philippines Case Study- Multiple Hazard Zones. 2.0 / 5 based on 1 rating. Hazard Trends. 0.0 / 5. 1.7 Tectonic Hazards. 0.0 / 5. Phillipines. 0.0 / 5. Tectonics - definitions ...
multiple hazard zones what is the effect of being a multiple hazard/disaster hotspot? The hazards often occur as part of a complex web, making their impact greater and more complex to manage. The frequency of events does not always make the impact easier to manage, although in
Study with Quizlet and memorize flashcards containing terms like a disaster hotspot..., Greatest risk areas around the world, Multi Hazard Zone Case Study and more.
As the global push for sustainable urban development progresses, this study, set against the backdrop of Hangzhou City, one of China's megacities, addressed the conflict between urban expansion and the occurrence of urban geological hazards. Focusing on the predominant geological hazards troubling Hangzhou—urban road collapse, land subsidence, and karst collapse—we introduced a ...
Case study of a multi-hazardous environment beyond the UK to illustrate and analyse the nature of the hazards and the social, economic and environmental risks presented, and how human qualities and responses such as resilience, adaptation, mitigation and management contribute to its continuing human occupation. Case study at a local scale of a specified place in a hazardous setting to ...
PHILIPPINES MULTIPLE HAZARD ZONE case study. Physical geographical factors. Click the card to flip 👆. -Lies on convergent plate boundary producing earthquakes + volcanoes. - is located in Pacific Ocean so very prone to tsunamis. - lies within a major typhoon belt. - has 22 active volcanoes so destructive volcanic eruptions. - landslides ...
Study with Quizlet and memorize flashcards containing terms like Why is The Philippines subject to both tectonic and hydromet hazards ?, How many recorded disasters in last 30 years?, How many countries /10 of most risked cities are the Philippines ones? and more.