43.2 Developing Critical Thinking
Learning objectives.
By the end of this section, you will be able to:
- Analyze the types of thinking used in nursing
- Recognize when to use the different types of thinking in nursing
- Explore the application of knowledge to thinking in nursing
- Appy Critical Thinking Indicators (CTIs) to decision making
Thinking is something we usually do subconsciously, because we are not usually “thinking about thinking.” However, with the ever-increasing autonomy being afforded to nurses, there is also an increased need for nurses to be able to critically think effectively and intentionally. Being able to critically think helps nurses’ problem solve, generate solutions, and make sound clinical judgments that affect the lives of their patients. Keep reading to learn more about how nurses use critical thinking in practice and how you can develop your own critical thinking skills.
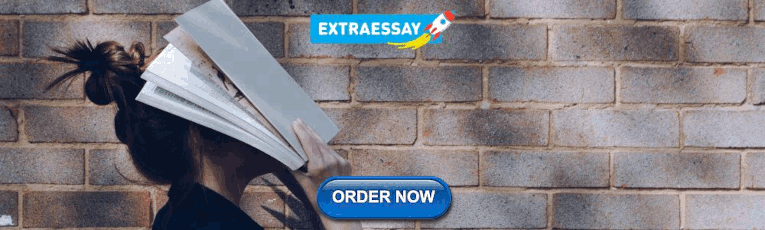
Types of Thinking Used in Nursing
Nurses make decisions while providing patient care by using critical thinking and clinical reasoning. In nursing, critical thinking is a broad term that includes reasoning about clinical issues such as teamwork, collaboration, and streamlining workflow.” On the other hand, clinical reasoning is defined as a complex cognitive process that uses formal and informal thinking strategies to gather and analyze patient information, evaluate the significance of this information, and weigh alternative actions. Each of these types of thinking is described in more detail in the following sections.
Cognitive Thinking
The term cognitive thinking refers to the mental processes and abilities a nurse uses to interpret, analyze, and evaluate information in their practice. Basically, it encompasses how nurses think about the practice decisions they are making. Cognitive thinking and critical thinking go hand in hand because nurses must be able to use their knowledge and mental processes to devise solutions and actions when caring for patients. Using critical thinking means that nurses take extra steps to maintain patient safety and do not just follow orders. It also means the accuracy of patient information is validated and plans for caring for patients are based on their needs, current clinical practice, and research. Critical thinkers possess certain attitudes that foster rational thinking:
- confidence: believing in yourself to complete a task or activity
- curiosity: asking “why” and wanting to know more
- fair-mindedness: treating every viewpoint in an unbiased, unprejudiced way
- independence of thought: thinking on your own
- insight into egocentricity and sociocentricity: thinking of the greater good and not just thinking of yourself. Knowing when you are thinking of yourself (egocentricity) and when you are thinking or acting for the greater good (sociocentricity)
- integrity: being honest and demonstrating strong moral principles
- intellectual humility: recognizing your intellectual limitations and abilities
- interest in exploring thoughts and feelings: wanting to explore different ways of knowing
- nonjudgmental: using professional ethical standards and not basing your judgments on your own personal or moral standards
- perseverance: persisting in doing something despite it being difficult
Cognitive thinking is significant to nursing because it provides a foundation on which nurses can make rapid and accurate decisions in clinical practice. Nurses must be able to think quickly and make informed decisions to promote optimal patient outcomes.
Effective Thinking
To make sound judgments about patient care, nurses must generate alternatives, weigh them against the evidence, and choose the best course of action. The ability to clinically reason develops over time and is based on knowledge and experience. Inductive and deductive reasoning are important critical thinking skills. They help the nurse use clinical judgment when implementing the nursing process. Effective thinking in nursing involves the integration of clinical knowledge and critical thinking to make the best decisions for patients. For example, if a nurse was caring for a patient who presents with hypertension and new-onset left-sided weakness, it is important that the nurse be able to quickly consider potential causes for the weakness and implement immediate stroke protocols. Without the ability to critically think, the nurse may overlook the weakness as being unrelated to the hypertension and not consider the possibility of stroke, leading to a poor patient outcome. Thus, it is imperative that nurses develop effective thinking skills.
Inductive Reasoning
The term inductive reasoning involves noticing cues, making generalizations, and creating hypotheses. Cues are data that fall outside of expected findings and give the nurse a hint or indication of a patient’s potential problem or condition. The nurse organizes these cues into patterns and creates a generalization. A generalization is a judgment formed on the basis of a set of facts, cues, and observations and is similar to gathering pieces of a jigsaw puzzle into patterns until the whole picture becomes clearer. On the basis of generalizations created from patterns of data, the nurse creates a hypothesis regarding a patient problem. Remember, a hypothesis is a proposed explanation for a situation. It attempts to explain the “why” behind the problem that is occurring. If a “why” is identified, then a solution can begin to be explored. No one can draw conclusions without first noticing cues. Paying close attention to a patient, the environment, and interactions with family members is critical for inductive reasoning. As you work to improve your inductive reasoning, begin by first noticing details about the things around you. Be mindful of your five primary senses: the things that you hear, feel, smell, taste, and see. Nurses need strong inductive reasoning patterns and be able to act quickly, especially in emergency situations. They can see how certain objects or events form a pattern (or a generalization) that indicates a common problem.
Consider this example: A nurse assesses a patient who has undergone surgery and finds the surgical incision site is red, warm, and tender to the touch. The nurse recognizes these cues form a pattern of signs of infection and creates a hypothesis that the incision has become infected. The provider is notified of the patient’s change in condition, and a new prescription is received for an antibiotic. This is an example of the use of inductive reasoning in nursing practice.
Deductive Reasoning
Another type of critical thinking is deductive reasoning ; it is referred to as “top-down thinking.” Deductive reasoning relies on using a general standard or rule to create a strategy. Nurses use standards set by their state’s Nurse Practice Act, federal regulations, the American Nursing Association, professional organizations, and their employer to make decisions about patient care and solve problems.
Think about this example: On the basis of research findings, hospital leaders determine patients recover more quickly if they receive adequate rest. The hospital creates a policy for quiet zones at night by initiating no overhead paging, promoting low-speaking voices by staff, and reducing lighting in the hallways. The nurse further implements this policy by organizing care for patients that promotes periods of uninterrupted rest at night. This is an example of deductive thinking, because the intervention is applied to all patients regardless of whether they have difficulty sleeping or not.
Identify the Purpose of Thinking
Rationalizing the purpose of thinking is probably not something you do often, but it is the foundational first step in critical thinking. To effectively use critical thinking in practice, the nurse must first identify the purpose of thinking. For example, the nurse is caring for a patient who presents with fever, tachycardia, and shortness of breath. The patient also has an open, infected wound on the left foot that is not healing. The nurse must recognize that the patient is exhibiting signs and symptoms that may be indicative of an underlying problem. At this point, the nurse must be able to identify that the purpose of thinking with regard to the patient is to consider what might be happening with the patient and formulate a plan of care. This begins the process of critical thinking, which involves several steps: thinking ahead, thinking in action, and reflection on thinking.
Thinking Ahead
Thinking ahead in nursing involves considering what may be going on with the patient to anticipate potential outcomes and complications that may arise. Remember competent nurses are proactive versus reactive. Reactive nursing is letting situations arise and then responding to the change, but proactive nursing is recognizing cues behaviors and patterns that are leading up to a complicated event. Additionally, the nurse will formulate goals of care and must try to anticipate specific needs the patient will have. Considering the patient discussed in the preceding paragraph, the nurse should begin the process of thinking ahead about potential outcomes and complications. The nurse may hypothesize that the patient is starting to develop sepsis from the open wound on the foot so severe sepsis and/or septic shock could be a complication to begin preparing for. The nurse thinks ahead about goals of care for the patient and determines that wound care to prevent infection spread and sepsis is the priority goal at this time.
Thinking in Action
Thinking in action encompasses the thought processes occurring while the nurse is performing interventions. So, if the nurse in our example begins performing wound care, they are thinking about the best dressing to use, how to clean the wound, and if antibiotics should be considered. All of these thoughts are likely occurring as the nurse is providing the care; thus, they are examples of how the nurse is using thinking in action.
Reflection on Thinking
After performing interventions or making decisions, the nurse should reflect on the thinking that occurred. The nurse will use this thinking process to determine if the decision was reactive or responsive. Reactive decision-making involves responding to situations after they have occurred, often in a hurried or unplanned manner. These decisions tend to be impulsive and are driven by immediate needs or crises. Responsive decisions, on the other hand, involve careful deliberation about how to address a situation based on careful consideration of information. In our example, the nurse’s decision appears to have been responsive. The patient was exhibiting some altered vital signs, but nothing indicated that the situation had become emergent yet. The nurse was able to think carefully about the patient’s situation and determine that wound care was the highest priority and begin to implement care in a calm, deliberate manner. In an ideal world, all nursing decisions would be responsive, but in a lot of cases, they must be reactive because of situation severity and medical emergencies.
Application of Knowledge
During the outset of the critical thinking process, nurses must judge whether their knowledge is accurate, complete, factual, timely, and relevant. This can be done by applying knowledge to nursing practice in a multitude of ways, including drawing from past education and experience in nursing and using professional resources and standards. Each of these is discussed in more detail in the following sections.
Knowledge Base
Becoming a nurse requires years of schooling, which contributes to the development of a robust knowledge base. Nurses receive formal education and training that provides them foundational knowledge in anatomy, physiology, pharmacology, and patient care techniques, among many others. Additionally, nurses are required to complete continuing education courses specific to their chosen practice setting, further developing their knowledge base. When applying knowledge in practice, nurses can draw from their knowledge base and make informed decisions about patient care.
Experience in Nursing
Nursing is considered a practice. Nursing practice means we learn from our mistakes and our past experiences and apply this knowledge to our next patient or to the next population we serve. As nurses gain more experience, they can use what they have learned in practice and apply it to new patient situations. Each new encounter with a patient presents unique challenge and learning opportunities that contribute to the development of clinical expertise. Reflecting on these experiences allows nurses to recognize patterns, anticipate patient outcomes, and refine their decision-making processes. Whether they are identifying effective nursing interventions for common conditions, adapting care plans to individual patient needs, or navigating complex situations with compassion, nurses draw upon their accumulated knowledge base from clinical experience to provide high-quality, patient-centered care. Through reflection and continuous learning from past experiences, nurses enhance their clinical skills, ultimately improving patient outcomes.
Professional Resources and Standards
In addition to foundational knowledge bases and experience, nurses can also use professional resources and standards to gain and apply knowledge in practice. Nurses can refer to clinical practice guidelines that have been established by professional organizations and healthcare institutions to help provide a framework for implementing nursing interventions based on the best evidence. By following the guidelines, nurses are ensuring that their care aligns with established standards and promotes optimal patient outcomes. Additionally, nurses should remain up to date about new and emerging research in their practice area, which can be obtained by reading professional journals and publications and attending conferences, workshops, and other trainings. Nurses can use the information learned from these resources to influence practice and ensure the highest standards of care are being performed in their practice setting. By staying informed about the latest developments in nursing and health care, nurses enhance their knowledge base and can adapt their practice to incorporate new evidence and innovations. Along with professional development and staying current with professional practices, nursing students should actively seek and join professional organizations such as critical care nursing or oncology nursing societies because this will lead the student to become expert in that subject and stay relevant with current evidence and practice guidelines.
Clinical Safety and Procedures (QSEN)
Qsen competency: evidence-based practice.
Definition: Providing quality patient care based on up-to-date, theory-derived research and knowledge, rather than personal beliefs, advice, or traditional methods.
Knowledge: The nurse will describe how the strength and relevance of available evidence influences the choice of intervention in provision of patient-centered care.
Skill: The nurse will:
- subscribe to professional journals that produce original research and evidence-based reports related to their specific area of practice
- become familiar with current evidence-based clinical practice topics and guidelines
- assist in creating a work environment that welcomes new evidence into standards of practice
- question the rational for traditional methods of care that result in sub-par outcomes or adverse events
Attitude: The nurse will appreciate the importance of regularly reading relevant professional journals.
Critique of Decision
After determining the best course of action based on the application of knowledge, the nurse can critique the decisions that were made. Specifically, the nurse will use self-reflection to review their actions and thoughts that led them to the decision. The nurse will consider the outcomes of their chosen interventions, reflect on the effectiveness of their approach, and identify areas of improvement. Additionally, the nurse may seek feedback from colleagues to obtain different perspectives about decisions made. Soliciting input from others helps the nurse gain insight and learn from their peers to further inform their future practice. Reflection questions that the nurse may ask themselves to critique their decision include the following:
- Was the patient goal or outcome met?
- Could the intervention have been done differently? Could it have been done better?
- What are alternative decisions that could have been made? What are the merits of each?
Critical Thinking Indicators
Certain behaviors that demonstrate the knowledge, skills, and attitudes that promote critical thinking are called critical thinking indicators (CTIs) . Critical thinking indicators are tangible actions that are performed to assess and improve your thinking skills.
4-Circle CT Model
There are many models and frameworks within nursing and other disciplines that attempt to explain the process of critical thinking. One of the most popular is Alfaro-LeFevre’s 4-Circle CT Model (Alfaro-LeFevre, 2016). This model breaks critical thinking into four components: personal characteristics, intellectual and cognitive abilities, interpersonal abilities and self-management, and technical skills. These four components overlap, forming interconnections in critical thinking.
Link to Learning
Learn more here about the 4-Circle CT Model and see an illustration of it.
Personal Critical Thinking Indicators
Personal CTIs are behaviors that are indicative of critical thinkers. Some of these behaviors that are most relevant to nursing include:
- confidence and resilience: showing ability to reason and learn and overcoming problems
- curiosity and inquisitiveness: asking questions and looking for the “why” behind things
- effective communication: listening well, showing understanding for others thoughts and feelings, and speaking and writing with clarity
- flexibility: changing approaches as needed to obtain the best results
- honesty: looking for the truth and demonstrating integrity while adhering to moral and ethical standards
- self-awareness: being able to identify one’s own knowledge gaps and acknowledge when thinking may be negatively influenced by emotions or self-interests.
Personal Knowledge and Intellectual Skills
Personal knowledge and intellectual skills encompass the knowledge gained from nursing school and clinical experiences. Examples of each of these kinds of skills are listed in Table 43.3 .
Personal Knowledge | Intellectual Skills |
---|---|
Interpersonal and Self-Management Skills
Interpersonal and self-management skills encompass the knowledge and skills needed for effective collaboration. These include:
- addressing conflicts fairly
- advocating for patients, self, and others
- dealing with complaints constructively
- establishing empowered partnerships
- facilitating and navigating change
- fostering positive interpersonal relationships and promoting teamwork
- giving and taking constructive criticism
- leading, motivating, and managing others
- managing stress, time, and energy
- promoting a learning and safety culture
- upholding healthy workplace standards
- using skilled communication in high-stake situations
Technical Skills
Technical skills in nursing refer to the practical abilities and competencies that nurses use in the delivery of patient care. These skills are typically learned through education, training, and hands-on experience. Some common technical skills in nursing include:
- administering medications
- assisting with personal hygiene and activities of daily living
- documentation and charting
- inserting intravenous catheters
- inserting urinary catheters and nasogastric tubes
- performing tracheostomy care
- performing wound care
- taking vital signs
This book may not be used in the training of large language models or otherwise be ingested into large language models or generative AI offerings without OpenStax's permission.
Want to cite, share, or modify this book? This book uses the Creative Commons Attribution License and you must attribute OpenStax.
Access for free at https://openstax.org/books/fundamentals-nursing/pages/1-introduction
- Authors: Christy Bowen, Lindsay Draper, Heather Moore
- Publisher/website: OpenStax
- Book title: Fundamentals of Nursing
- Publication date: Sep 4, 2024
- Location: Houston, Texas
- Book URL: https://openstax.org/books/fundamentals-nursing/pages/1-introduction
- Section URL: https://openstax.org/books/fundamentals-nursing/pages/43-2-developing-critical-thinking
© Aug 20, 2024 OpenStax. Textbook content produced by OpenStax is licensed under a Creative Commons Attribution License . The OpenStax name, OpenStax logo, OpenStax book covers, OpenStax CNX name, and OpenStax CNX logo are not subject to the Creative Commons license and may not be reproduced without the prior and express written consent of Rice University.
This website is intended for healthcare professionals
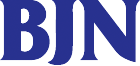
- { $refs.search.focus(); })" aria-controls="searchpanel" :aria-expanded="open" class="hidden lg:inline-flex justify-end text-gray-800 hover:text-primary py-2 px-4 lg:px-0 items-center text-base font-medium"> Search
Search menu
Critical thinking: what it is and why it counts. 2020. https://tinyurl.com/ybz73bnx (accessed 27 April 2021)
Faculty of Intensive Care Medicine. Curriculum for training for advanced critical care practitioners: syllabus (part III). version 1.1. 2018. https://www.ficm.ac.uk/accps/curriculum (accessed 27 April 2021)
Guerrero AP. Mechanistic case diagramming: a tool for problem-based learning. Acad Med.. 2001; 76:(4)385-9 https://doi.org/10.1097/00001888-200104000-00020
Harasym PH, Tsai TC, Hemmati P. Current trends in developing medical students' critical thinking abilities. Kaohsiung J Med Sci.. 2008; 24:(7)341-55 https://doi.org/10.1016/S1607-551X(08)70131-1
Hayes MM, Chatterjee S, Schwartzstein RM. Critical thinking in critical care: five strategies to improve teaching and learning in the intensive care unit. Ann Am Thorac Soc.. 2017; 14:(4)569-575 https://doi.org/10.1513/AnnalsATS.201612-1009AS
Health Education England. Multi-professional framework for advanced clinical practice in England. 2017. https://www.hee.nhs.uk/sites/default/files/documents/multi-professionalframeworkforadvancedclinicalpracticeinengland.pdf (accessed 27 April 2021)
Health Education England, NHS England/NHS Improvement, Skills for Health. Core capabilities framework for advanced clinical practice (nurses) working in general practice/primary care in England. 2020. https://www.skillsforhealth.org.uk/images/services/cstf/ACP%20Primary%20Care%20Nurse%20Fwk%202020.pdf (accessed 27 April 2021)
Health Education England. Advanced practice mental health curriculum and capabilities framework. 2020. https://www.hee.nhs.uk/sites/default/files/documents/AP-MH%20Curriculum%20and%20Capabilities%20Framework%201.2.pdf (accessed 27 April 2021)
Jacob E, Duffield C, Jacob D. A protocol for the development of a critical thinking assessment tool for nurses using a Delphi technique. J Adv Nurs.. 2017; 73:(8)1982-1988 https://doi.org/10.1111/jan.13306
Kohn MA. Understanding evidence-based diagnosis. Diagnosis (Berl).. 2014; 1:(1)39-42 https://doi.org/10.1515/dx-2013-0003
Clinical reasoning—a guide to improving teaching and practice. 2012. https://www.racgp.org.au/afp/201201/45593
McGee S. Evidence-based physical diagnosis, 4th edn. Philadelphia PA: Elsevier; 2018
Norman GR, Monteiro SD, Sherbino J, Ilgen JS, Schmidt HG, Mamede S. The causes of errors in clinical reasoning: cognitive biases, knowledge deficits, and dual process thinking. Acad Med.. 2017; 92:(1)23-30 https://doi.org/10.1097/ACM.0000000000001421
Papp KK, Huang GC, Lauzon Clabo LM Milestones of critical thinking: a developmental model for medicine and nursing. Acad Med.. 2014; 89:(5)715-20 https://doi.org/10.1097/acm.0000000000000220
Rencic J, Lambert WT, Schuwirth L., Durning SJ. Clinical reasoning performance assessment: using situated cognition theory as a conceptual framework. Diagnosis.. 2020; 7:(3)177-179 https://doi.org/10.1515/dx-2019-0051
Examining critical thinking skills in family medicine residents. 2016. https://www.stfm.org/FamilyMedicine/Vol48Issue2/Ross121
Royal College of Emergency Medicine. Emergency care advanced clinical practitioner—curriculum and assessment, adult and paediatric. version 2.0. 2019. https://tinyurl.com/eps3p37r (accessed 27 April 2021)
Young ME, Thomas A, Lubarsky S. Mapping clinical reasoning literature across the health professions: a scoping review. BMC Med Educ.. 2020; 20 https://doi.org/10.1186/s12909-020-02012-9
Advanced practice: critical thinking and clinical reasoning
Sadie Diamond-Fox
Senior Lecturer in Advanced Critical Care Practice, Northumbria University, Advanced Critical Care Practitioner, Newcastle upon Tyne Hospitals NHS Foundation Trust, and Co-Lead, Advanced Critical/Clinical Care Practitioners Academic Network (ACCPAN)
View articles · Email Sadie
Advanced Critical Care Practitioner, South Tees Hospitals NHS Foundation Trust
View articles

Clinical reasoning is a multi-faceted and complex construct, the understanding of which has emerged from multiple fields outside of healthcare literature, primarily the psychological and behavioural sciences. The application of clinical reasoning is central to the advanced non-medical practitioner (ANMP) role, as complex patient caseloads with undifferentiated and undiagnosed diseases are now a regular feature in healthcare practice. This article explores some of the key concepts and terminology that have evolved over the last four decades and have led to our modern day understanding of this topic. It also considers how clinical reasoning is vital for improving evidence-based diagnosis and subsequent effective care planning. A comprehensive guide to applying diagnostic reasoning on a body systems basis will be explored later in this series.
The Multi-professional Framework for Advanced Clinical Practice highlights clinical reasoning as one of the core clinical capabilities for advanced clinical practice in England ( Health Education England (HEE), 2017 ). This is also identified in other specialist core capability frameworks and training syllabuses for advanced clinical practitioner (ACP) roles ( Faculty of Intensive Care Medicine, 2018 ; Royal College of Emergency Medicine, 2019 ; HEE, 2020 ; HEE et al, 2020 ).
Rencic et al (2020) defined clinical reasoning as ‘a complex ability, requiring both declarative and procedural knowledge, such as physical examination and communication skills’. A plethora of literature exists surrounding this topic, with a recent systematic review identifying 625 papers, spanning 47 years, across the health professions ( Young et al, 2020 ). A diverse range of terms are used to refer to clinical reasoning within the healthcare literature ( Table 1 ), which can make defining their influence on their use within the clinical practice and educational arenas somewhat challenging.
Register now to continue reading
Thank you for visiting British Journal of Nursing and reading some of our peer-reviewed resources for nurses. To read more, please register today. You’ll enjoy the following great benefits:
What's included
Limited access to clinical or professional articles
Unlimited access to the latest news, blogs and video content
Signing in with your registered email address

An official website of the United States government
The .gov means it’s official. Federal government websites often end in .gov or .mil. Before sharing sensitive information, make sure you’re on a federal government site.
The site is secure. The https:// ensures that you are connecting to the official website and that any information you provide is encrypted and transmitted securely.
- Publications
- Account settings
- My Bibliography
- Collections
- Citation manager
Save citation to file
Email citation, add to collections.
- Create a new collection
- Add to an existing collection
Add to My Bibliography
Your saved search, create a file for external citation management software, your rss feed.
- Search in PubMed
- Search in NLM Catalog
- Add to Search
Advanced practice: critical thinking and clinical reasoning
Affiliations.
- 1 Advanced Critical Care Practitioner, Newcastle upon Tyne NHS Foundation Trust / Senior Lecturer in Advanced Critical Care Practice, Department of Nursing, Midwifery and Health, Northumbria University.
- 2 Advanced Critical Care Practitioner, South Tees Hospitals NHS Foundation Trust.
- PMID: 33983801
- DOI: 10.12968/bjon.2021.30.9.526
Clinical reasoning is a multi-faceted and complex construct, the understanding of which has emerged from multiple fields outside of healthcare literature, primarily the psychological and behavioural sciences. The application of clinical reasoning is central to the advanced non-medical practitioner (ANMP) role, as complex patient caseloads with undifferentiated and undiagnosed diseases are now a regular feature in healthcare practice. This article explores some of the key concepts and terminology that have evolved over the last four decades and have led to our modern day understanding of this topic. It also considers how clinical reasoning is vital for improving evidence-based diagnosis and subsequent effective care planning. A comprehensive guide to applying diagnostic reasoning on a body systems basis will be explored later in this series.
Keywords: Advanced practice; Clinical reasoning; Consultation; Critical thinking; Diagnostic accuracy.
PubMed Disclaimer
Similar articles
- Teaching strategies of clinical reasoning in advanced nursing clinical practice: A scoping review. Giuffrida S, Silano V, Ramacciati N, Prandi C, Baldon A, Bianchi M. Giuffrida S, et al. Nurse Educ Pract. 2023 Feb;67:103548. doi: 10.1016/j.nepr.2023.103548. Epub 2023 Jan 17. Nurse Educ Pract. 2023. PMID: 36708638 Review.
- Undertaking consultations and clinical assessments at advanced level. Diamond-Fox S. Diamond-Fox S. Br J Nurs. 2021 Feb 25;30(4):238-243. doi: 10.12968/bjon.2021.30.4.238. Br J Nurs. 2021. PMID: 33641390
- [Informing the clinical reasoning of advanced practice students with nursing theories]. Garry-Bruneau M, Clerens K, Le Dévic L, Perret M, Gastinel A, Dallaire C. Garry-Bruneau M, et al. Soins. 2024 Jul-Aug;69(887):41-44. doi: 10.1016/j.soin.2024.05.013. Epub 2024 Jun 25. Soins. 2024. PMID: 39019516 French.
- Advanced Nurse Practitioners' (Emergency) perceptions of their role, positionality and professional identity: A narrative inquiry. Kerr L, Macaskill A. Kerr L, et al. J Adv Nurs. 2020 May;76(5):1201-1210. doi: 10.1111/jan.14314. Epub 2020 Feb 23. J Adv Nurs. 2020. PMID: 32017199
- Teaching Clinical Reasoning and Critical Thinking: From Cognitive Theory to Practical Application. Richards JB, Hayes MM, Schwartzstein RM. Richards JB, et al. Chest. 2020 Oct;158(4):1617-1628. doi: 10.1016/j.chest.2020.05.525. Epub 2020 May 22. Chest. 2020. PMID: 32450242 Review.
- Clinical learning opportunity in public academic hospitals: A concept analysis. Motsaanaka MN, Makhene A, Ndawo G. Motsaanaka MN, et al. Health SA. 2022 Oct 26;27:1920. doi: 10.4102/hsag.v27i0.1920. eCollection 2022. Health SA. 2022. PMID: 36337451 Free PMC article.
- Search in MeSH
LinkOut - more resources
Full text sources, other literature sources.
- scite Smart Citations
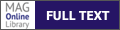
- Citation Manager
NCBI Literature Resources
MeSH PMC Bookshelf Disclaimer
The PubMed wordmark and PubMed logo are registered trademarks of the U.S. Department of Health and Human Services (HHS). Unauthorized use of these marks is strictly prohibited.
Shaping Clinical Reasoning
- First Online: 02 January 2023
Cite this chapter
- Rita Payan-Carreira 3 , 4 &
- Joana Reis 4 , 5
Part of the book series: Integrated Science ((IS,volume 12))
1228 Accesses
Clinical reasoning is at the core of all health-related professions, and it is long recognized as a critical skill for clinical practice. Yet, it is difficult to characterize it, as clinical reasoning combines different high-thinking abilities. Also, it is not content that is historically taught or learned in a particular subject. But clinical reasoning became increasingly visible when this competency is explicitly stated in the curricula of educational programs in health-related professions. Teaching and learning an abstract concept such as clinical reasoning in complement to the core knowledge and the procedural competencies expected from healthcare professionals raises some concerns regarding its implementation, the best way to do it, and how to assess it. This book chapter intends to discuss the need to invest in the development of clinical reasoning skills in the health-related graduation programme. It addresses some of the pedagogical and theoretical frameworks for fostering high-level reasoning and problem-solving skills in the clinical areas and the effectiveness and success of different pedagogic activities to develop and shape clinical reasoning throughout the curriculum.
Graphical Abstract/Art Performance
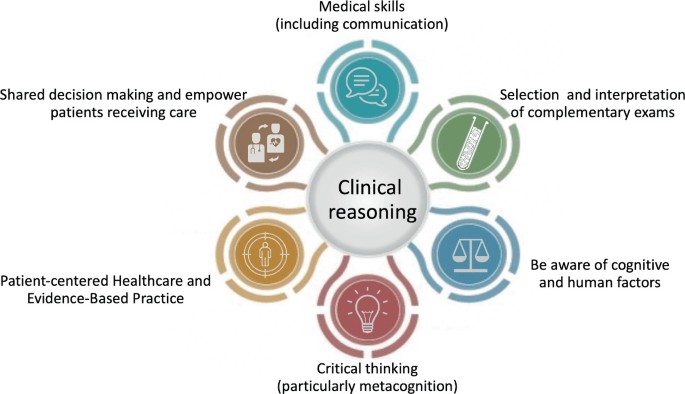
The elements involved in clinical reasoning.
As medical educators […] we know that medical knowledge and competence is developmental; however, habits of the mind – behavior and practical – and wisdom are achieved through deliberate practice that can be achieved throughout medical school and with further refinement of medical skills during the clinical years […] Allison A. Vanderbilt et al. [ 1 ]
This is a preview of subscription content, log in via an institution to check access.
Access this chapter
Subscribe and save.
- Get 10 units per month
- Download Article/Chapter or eBook
- 1 Unit = 1 Article or 1 Chapter
- Cancel anytime
- Available as PDF
- Read on any device
- Instant download
- Own it forever
- Available as EPUB and PDF
- Compact, lightweight edition
- Dispatched in 3 to 5 business days
- Free shipping worldwide - see info
- Durable hardcover edition
Tax calculation will be finalised at checkout
Purchases are for personal use only
Institutional subscriptions
Similar content being viewed by others
Developing Clinical Reasoning Capabilities
Ready to reason: integration of clinical education and basic science improves medical students’ self-assessed clinical reasoning before clerkships.
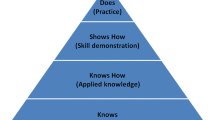
Assessing Clinical Reasoning: Targeting the Higher Levels of the Pyramid
Vanderbilt AA, Perkins SQ, Muscaro MK et al (2017) Creating physicians of the 21st century: assessment of the clinical years. Adv Med Educ Pract 8:395
Article Google Scholar
Higgs J, Jones MA (2008) Clinical decision making and multiple problem spaces. In: Higgs J, Jensen G, Loftus S, Christensen N (eds) Clinical reasoning in the health professions. Elsevier, Amsterdam, pp 3–17
Google Scholar
Young ME, Thomas A, Lubarsky S et al (2020) Mapping clinical reasoning literature across the health professions: a scoping review. BMC Med Educ 20:1–11
Daly P (2018) A concise guide to clinical reasoning. J Eval Clin Pract 24:966–972
Simmons B (2010) Clinical reasoning: concept analysis. J Adv Nurs 66:1151–1158
Chin-Yee B, Upshur R (2018) Clinical judgement in the era of big data and predictive analytics. J Eval Clin Pract 24:638–645
Faucher C (2011) Differentiating the elements of clinical thinking. Optom Educ 36(3):140–145
Young M, Thomas A, Lubarsky S et al (2018) Drawing boundaries: the difficulty in defining clinical reasoning. Acad Med 93:990–995
Payan-Carreira R, Cruz G, Papathanasiou IV et al (2019) The effectiveness of critical thinking instructional strategies in health professions education: a systematic review. Stud High Educ 44:829–843
Gummesson C, Sundén A, Fex A (2018) Clinical reasoning as a conceptual framework for interprofessional learning: a literature review and a case study. Phys Ther Rev 23:29–34
Connor DM, Durning SJ, Rencic JJ (2020) Clinical reasoning as a core competency. Acad Med 95(8):1166–1171
May SA (2013) Clinical reasoning and case-based decision making: the fundamental challenge to veterinary educators. J Vet Med Educ 40:200–209
Evans JSB, Stanovich KE (2013) Dual-process theories of higher cognition: advancing the debate. Perspect Psychol Sci 8:223–241
Evans JSBT (2008) Dual-processing accounts of reasoning, judgment, and social cognition. Annu Rev Psychol 59:255–278
Ten Cate O (2017) Introduction. In: Ten Cate O, Custers EJFM, Durning SJ (eds) Principles and practice of case-based clinical reasoning education: a method for preclinical students. Springer Nature, pp 3–19
Croskerry P (2009) A universal model of diagnostic reasoning. Acad Med 84:1022–1028
Evans JSB (2019) Reflections on reflection: the nature and function of type 2 processes in dual-process theories of reasoning. Think Reason 25:383–415
Melnikoff DE, Bargh JA (2018) The mythical number two. Trends Cogn Sci 22:280–293
Handley SJ, Trippas D (2015) Dual processes and the interplay between knowledge and structure: a new parallel processing model. In: Psychology of learning and motivation. Elsevier, pp 33–58
Houdé O (2019) 3-system theory of the cognitive brain: a post-Piagetian approach to cognitive development. Routledge, pp 100–119
Braude HD (2017) 13 clinical reasoning and knowing. In: The Bloomsbury companion to contemporary philosophy of medicine. Bloomsbury Academic, pp 323–342
Medina MS, Castleberry AN, Persky AM (2017) Strategies for improving learner metacognition in health professional education. Am J Pharm Educ 81(4):78
Makary MA, Daniel M (2016) Medical error—the third leading cause of death in the US. The BMJ 353:i2139
LaRochelle JS, Dong T, Durning SJ (2015) Preclerkship assessment of clinical skills and clinical reasoning: the longitudinal impact on student performance. Mil Med 180:43–46
Clapper TC, Ching K (2020) Debunking the myth that the majority of medical errors are attributed to communication. Med Educ 54:74–81
Okafor N, Payne VL, Chathampally Y et al (2016) Using voluntary reports from physicians to learn from diagnostic errors in emergency medicine. Emerg Med J 33:245–252
Braun LT, Zwaan L, Kiesewetter J et al (2017) Diagnostic errors by medical students: results of a prospective qualitative study. BMC Med Educ 17:191. https://doi.org/10.1186/s12909-017-1044-7
Norman GR, Monteiro SD, Sherbino J et al (2017) The causes of errors in clinical reasoning: cognitive biases, knowledge deficits, and dual process thinking. Acad Med 92:23–30
Deming M, Mark A, Nyemba V et al (2019) Cognitive biases and knowledge deficits leading to delayed recognition of cryptococcal meningitis. IDCases 18:e00588. https://doi.org/10.1016/j.idcr.2019.e00588
Article CAS Google Scholar
Royce CS, Hayes MM, Schwartzstein RM (2019) Teaching critical thinking: a case for instruction in cognitive biases to reduce diagnostic errors and improve patient safety. Acad Med 94:187–194
Wellbery C (2011) Flaws in clinical reasoning: a common cause of diagnostic error. Am Fam Physician 84:1042–1048
Amey L, Donald KJ, Teodorczuk A (2017) Teaching clinical reasoning to medical students. Br J Hosp Med 78:399–401
Stuart S, Hartig J, Willett L (2017) The importance of framing. J Gen Intern Med 32:706–710
Rylander M, Guerrasio J (2016) Heuristic errors in clinical reasoning. Clin Teach 13:287–290
O’sullivan E, Schofield S (2018) Cognitive bias in clinical medicine. J R College Physicians Edinburgh 48:225–232
Delany C, Golding C (2014) Teaching clinical reasoning by making thinking visible: an action research project with allied health clinical educators. BMC Med Educ 14:20. https://doi.org/10.1186/1472-6920-14-20
Elen J, Jiang L, Huyghe S et al (2019) Promoting critical thinking in European higher education institutions: towards an educational protocol. Vila Real: UTAD available at: https://repositorio.utad.pt/bitstream/10348/9227/1/CRITHINKEDU%20O4%20(ebook)_FINA
Miller GE (1990) The assessment of clinical skills/competence/performance. Acad Med 65:S63–S67
Payan-Carreira R, Dominguez C, Monteiro MJ, da Conceição Rainho M (2016) Application of the adapted FRISCO framework in case-based learning activities. Revista Lusófona de Educação 173–189
Hawkins D, Elder L, Paul R (2019) The thinker’s guide to clinical reasoning: based on critical thinking concepts and tools. Rowman & Littlefield
Keir JE, Saad SL, Davin L (2018) Exploring tutor perceptions and current practices in facilitating diagnostic reasoning in preclinical medical students: Implications for tutor professional development needs. MedEdPublish 7:(2). Available at: https://researchonline.nd.edu.au/cgi/viewcontent.cgi?article=1942&context=med_article
Henard F, Roseveare D (2012) Fostering quality teaching in higher education: policies and practices. An IMHE Guide for Higher Education Institutions (OCDE publishing) 7–11. Available at http://supporthere.org/sites/default/files/qt_policies_and_practices_1.pdf
Barrett JL, Denegar CR, Mazerolle SM (2018) Challenges facing new educators: expanding teaching strategies for clinical reasoning and evidence-based medicine. Athl Train Educ J 13:359–366
Pyrko I, Dӧrfler V, Eden C (2017) Thinking together: what makes communities of practice work? Hum Relations 70:389–409
Payan-Carreira R, Cruz G (2019) Students’ study routines, learning preferences and self-regulation: are they related? In: Tsitouridou M, Diniz AJ, Mikropoulos T (eds) Technology and innovation in learning, teaching and education. TECH-EDU 2018. Communications in computer and information science, vol 993. Springer, Cham. https://doi.org/10.1007/978-3-030-20954-4_14
Linsen A, Elshout G, Pols D et al (2018) Education in clinical reasoning: an experimental study on strategies to foster novice medical students’ engagement in learning activities. Health Prof Educ 4:86–96
Kozlowski D, Hutchinson M, Hurley J et al (2017) The role of emotion in clinical decision making: an integrative literature review. BMC Med Educ 17:255
Iacovides A, Fountoulakis K, Kaprinis S, Kaprinis G (2003) The relationship between job stress, burnout and clinical depression. J Affect Disord 75:209–221
Audétat M-C, Laurin S, Sanche G et al (2013) Clinical reasoning difficulties: a taxonomy for clinical teachers. Med Teach 35:e984–e989. https://doi.org/10.3109/0142159X.2012.733041
Audétat M-C, Laurin S, Dory V et al (2017) Diagnosis and management of clinical reasoning difficulties: part I. Clinical reasoning supervision and educational diagnosis. Med Teach 39:792–796
Kumar DRR, Priyadharshini N, Murugan M, Devi R (2020) Infusing the axioms of clinical reasoning while designing clinical anatomy case vignettes teaching for novice medical students: a randomised cross over study. Anat Cell Biol 53:151
Huhn K, Black L, Christensen N et al (2018) Clinical reasoning: survey of teaching methods and assessment in entry-level physical therapist clinical education. J Phys Ther Educ 32:241–247
Patton N, Christensen N (2019) Pedagogies for teaching and learning clinical reasoning. In: Clinical reasoning in the Health professions. Elsevier, pp 335–344
Bowen JL (2006) Educational strategies to promote clinical diagnostic reasoning. N Engl J Med 355:2217–2225
Ajjawi R, Higgs J (2019) Learning to communicate clinical reasoning. In: Clinical reasoning in the health professions, pp 419–425
Levett-Jones T, Hoffman K, Dempsey J et al (2010) The “five rights” of clinical reasoning: an educational model to enhance nursing students’ ability to identify and manage clinically “at risk” patients. Nurse Educ Today 30:515–520
Gordon M, Findley R (2011) Educational interventions to improve handover in health care: a systematic review. Med Educ 45:1081–1089
Tiruneh DT, Verburgh A, Elen J (2013) Effectiveness of critical thinking instruction in higher education: a systematic review. High Educ Stud 4:1–17
Min Simpkins AA, Koch B, Spear-Ellinwood K, St. John P (2019) A developmental assessment of clinical reasoning in preclinical medical education. Med Educ Online 24:1591257
Tyo MB, McCurry MK (2019) An integrative review of clinical reasoning teaching strategies and outcome evaluation in nursing education. Nurs Educ Perspect 40:11–17
Boulet JR, Durning SJ (2019) What we measure… and what we should measure in medical education. Med Educ 53:86–94
Ten Cate O, Durning SJ (2018) Approaches to assessing the clinical reasoning of preclinical students. In: Principles and practice of case-based clinical reasoning education. Springer, Cham, pp 65–72
Haffer AG, Raingruber BJ (1998) Discovering confidence in clinical reasoning and critical thinking development in baccalaureate nursing students. J Nurs Educ 37:61–70
Dominguez C (Coord. ) (2018) A European collection of the critical thinking skills and dispositions needed in different professional fields for the 21st century. UTAD https://repositorio.utad.pt/bitstream/10348/8319/1/CRITHINKEDU%20O1%20%28ebook%29.p
Download references
Acknowledgements
This work is being developed within the scope of the “Critical Thinking for Successful Jobs–Think4Jobs” project (Ref. Nr. 2020-1-EL01-KA203-078797) funded by the European Commission/EACEA, through the ERASMUS+ Programme. “The European Commission support for the production of this publication does not constitute an endorsement of the contents which reflects the views only of the authors, and the Commission cannot be held responsible for any use which may be made of the information contained therein.”
Author information
Authors and affiliations.
Comprehensive Health Research Centre, Évora, Portugal
Rita Payan-Carreira
Department of Veterinary Medicine, University of Évora, Polo da Mitra [Apartado 94], 7002-774, Évora, Portugal
Rita Payan-Carreira & Joana Reis
Escola Superior Agrária (ESA), Polytechnic Institute of Viana do Castelo, CISAS, Viana do Castelo, Portugal
You can also search for this author in PubMed Google Scholar
Corresponding author
Correspondence to Rita Payan-Carreira .
Editor information
Editors and affiliations.
Universal Scientific Education and Research Network (USERN), Stockholm, Sweden
Nima Rezaei
Rights and permissions
Reprints and permissions
Copyright information
© 2023 The Author(s), under exclusive license to Springer Nature Switzerland AG
About this chapter
Payan-Carreira, R., Reis, J. (2023). Shaping Clinical Reasoning. In: Rezaei, N. (eds) Brain, Decision Making and Mental Health. Integrated Science, vol 12. Springer, Cham. https://doi.org/10.1007/978-3-031-15959-6_9
Download citation
DOI : https://doi.org/10.1007/978-3-031-15959-6_9
Published : 02 January 2023
Publisher Name : Springer, Cham
Print ISBN : 978-3-031-15958-9
Online ISBN : 978-3-031-15959-6
eBook Packages : Behavioral Science and Psychology Behavioral Science and Psychology (R0)
Share this chapter
Anyone you share the following link with will be able to read this content:
Sorry, a shareable link is not currently available for this article.
Provided by the Springer Nature SharedIt content-sharing initiative
- Publish with us
Policies and ethics
- Find a journal
- Track your research
PERSPECTIVE article
Rethinking clinical decision-making to improve clinical reasoning.
- 1 Department of Internal Medicine, National Relevance and High Specialization Hospital Trust ARNAS Civico, Palermo, Italy
- 2 Dipartimento di Promozione della Salute Materno Infantile, Medicina Interna e Specialistica di Eccellenza “G. D’Alessandro” (PROMISE), University of Palermo, Palermo, Italy
Improving clinical reasoning techniques is the right way to facilitate decision-making from prognostic, diagnostic, and therapeutic points of view. However, the process to do that is to fill knowledge gaps by studying and growing experience and knowing some cognitive aspects to raise the awareness of thinking mechanisms to avoid cognitive errors through correct educational training. This article examines clinical approaches and educational gaps in training medical students and young doctors. The authors explore the core elements of clinical reasoning, including metacognition, reasoning errors and cognitive biases, reasoning strategies, and ways to improve decision-making. The article addresses the dual-process theory of thought and the new Default Mode Network (DMN) theory. The reader may consider the article a first-level guide to deepen how to think and not what to think, knowing that this synthesis results from years of study and reasoning in clinical practice and educational settings.
Introduction
Clinical reasoning is based on complex and multifaceted cognitive processes, and the level of cognition is perhaps the most relevant factor that impacts the physician’s clinical reasoning. These topics have inspired considerable interest in the last years ( 1 , 2 ). According to Croskerry ( 3 ) and Croskerry and Norman ( 4 ), over 40 affective and cognitive biases may impact clinical reasoning. In addition, it should not be forgotten that both the processes and the subject matter are complex.
In medicine, there are thousands of known diagnoses, each with different complexity. Moreover, in line with Hammond’s view, a fundamental uncertainty will inevitably fail ( 5 ). Any mistake or failure in the diagnostic process leads to a delayed diagnosis, a misdiagnosis, or a missed diagnosis. The particular context in which a medical decision is made is highly relevant to the reasoning process and outcome ( 6 ).
More recently, there has been renewed interest in diagnostic reasoning, primarily diagnostic errors. Many researchers deepen inside the processes underpinning cognition, developing new universal reasoning and decision-making model: The Dual Process Theory.
This theory has a prompt implementation in medical decision-making and provides a comprehensive framework for understanding the gamma of theoretical approaches taken into consideration previously. This model has critical practical applications for medical decision-making and may be used as a model for teaching decision reasoning. Given this background, this manuscript must be considered a first-level guide to understanding how to think and not what to think, deepening clinical decision-making and providing tools for improving clinical reasoning.
Too much attention to the tip of the iceberg
The New England Journal of Medicine has recently published a fascinating article ( 7 ) in the “Perspective” section, whereon we must all reflect on it. The title is “At baseline” (the basic condition). Dr. Bergl, from the Department of Medicine of the Medical College of Wisconsin (Milwaukee), raised that his trainees no longer wonder about the underlying pathology but are focused solely on solving the acute problem. He wrote that, for many internal medicine teams, the question is not whether but to what extent we should juggle the treatment of critical health problems of patients with care for their coexisting chronic conditions. Doctors are under high pressure to discharge, and then they move patients to the next stage of treatment without questioning the reason that decompensated the clinical condition. Suppose the chronic condition or baseline was not the fundamental goal of our performance. In that case, our juggling is highly inconsistent because we are working on an intermediate outcome curing only the decompensation phase of a disease. Dr. Bergl raises another essential matter. Perhaps equally disturbing, by adopting a collective “base” mentality, we unintentionally create a group of doctors who prioritize productivity rather than developing critical skills and curiosity. We agree that empathy and patience are two other crucial elements in the training process of future internists. Nevertheless, how much do we stimulate all these qualities? Perhaps are not all part of cultural backgrounds necessary for a correct patient approach, the proper clinical reasoning, and balanced communication skills?
On the other hand, a chronic baseline condition is not always the real reason that justifies acute hospitalization. The lack of a careful approach to the baseline and clinical reasoning focused on the patient leads to this superficiality. We are focusing too much on our students’ practical skills and the amount of knowledge to learn. On the other hand, we do not teach how to think and the cognitive mechanisms of clinical reasoning.
Time to rethink the way of thinking and teaching courses
Back in 1910, John Dewey wrote in his book “How We Think” ( 8 ), “The aim of education should be to teach us rather how to think than what to think—rather improve our minds to enable us to think for ourselves than to load the memory with the thoughts of other men.”
Clinical reasoning concerns how to think and make the best decision-making process associated with the clinical practice ( 9 ). The core elements of clinical reasoning ( 10 ) can be summarized in:
1. Evidence-based skills,
2. Interpretation and use of diagnostic tests,
3. Understanding cognitive biases,
4. Human factors,
5. Metacognition (thinking about thinking), and
6. Patient-centered evidence-based medicine.
All these core elements are crucial for the best way of clinical reasoning. Each of them needs a correct learning path to be used in combination with developing the best thinking strategies ( Table 1 ). Reasoning strategies allow us to combine and synthesize diverse data into one or more diagnostic hypotheses, make the complex trade-off between the benefits and risks of tests and treatments, and formulate plans for patient management ( 10 ).
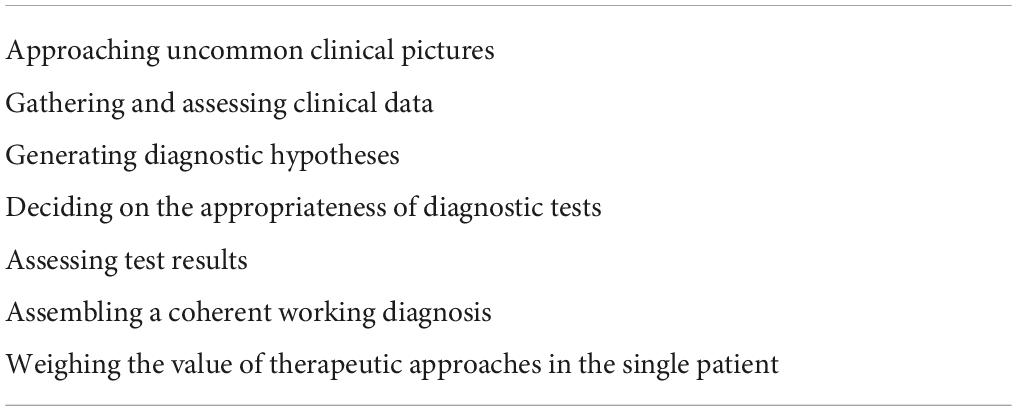
Table 1. Set of some reasoning strategies (view the text for explanations).
However, among the abovementioned core element of clinical reasoning, two are often missing in the learning paths of students and trainees: metacognition and understanding cognitive biases.
Metacognition
We have to recall cognitive psychology, which investigates human thinking and describes how the human brain has two distinct mental processes that influence reasoning and decision-making. The first form of cognition is an ancient mechanism of thought shared with other animals where speed is more important than accuracy. In this case, thinking is characterized by a fast, intuitive way that uses pattern recognition and automated processes. The second one is a product of evolution, particularly in human beings, indicated by an analytical and hypothetical-deductive slow, controlled, but highly consuming way of thinking. Today, the psychology of thinking calls this idea “the dual-process theory of thought” ( 11 – 14 ). The Nobel Prize in Economic Sciences awardee Daniel Kahneman has extensively studied the dichotomy between the two modes of thought, calling them fast and slow thinking. “System 1” is fast, instinctive, and emotional; “System 2” is slower, more deliberative, and more logical ( 15 ). Different cerebral zones are involved: “System 1” includes the dorsomedial prefrontal cortex, the pregenual medial prefrontal cortex, and the ventromedial prefrontal cortex; “System 2” encompasses the dorsolateral prefrontal cortex. Glucose utilization is massive when System 2 is performing ( 16 ). System 1 is the leading way of thought used. None could live permanently in a deliberate, slow, effortful way. Driving a car, eating, and performing many activities over time become automatic and subconscious.
A recent brilliant review of Gronchi and Giovannelli ( 17 ) explores those things. Typically, when a mental effort is required for tasks requiring attention, every individual is subject to a phenomenon called “ego-depletion.” When forced to do something, each one has fewer cognitive resources available to activate slow thinking and thus is less able to exert self-control ( 18 , 19 ). In the same way, much clinical decision-making becomes intuitive rather than analytical, a phenomenon strongly affected by individual differences ( 20 , 21 ). Experimental evidence by functional magnetic resonance imaging and positron emission tomography studies supports that the “resting state” is spontaneously active during periods of “passivity” ( 22 – 25 ). The brain regions involved include the medial prefrontal cortex, the posterior cingulate cortex, the inferior parietal lobule, the lateral temporal cortex, the dorsal medial prefrontal cortex, and the hippocampal formation ( 26 ). Findings reporting high-metabolic activity in these regions at rest ( 27 ) constituted the first clear evidence of a cohesive default mode in the brain ( 28 ), leading to the widely acknowledged introduction of the Default Mode Network (DMN) concept. The DMN contains the medial prefrontal cortex, the posterior cingulate cortex, the inferior parietal lobule, the lateral temporal cortex, the dorsal medial prefrontal cortex, and the hippocampal formation. Lower activity levels characterize the DMN during goal-directed cognition and higher activity levels when an individual is awake and involved in the mental processes requiring low externally directed attention. All that is the neural basis of spontaneous cognition ( 26 ) that is responsible for thinking using internal representations. This paradigm is growing the idea of stimulus-independent thoughts (SITs), defined by Buckner et al. ( 26 ) as “thoughts about something other than events originating from the environment” that is covert and not directed toward the performance of a specific task. Very recently, the role of the DMN was highlighted in automatic behavior (the rapid selection of a response to a particular and predictable context) ( 29 ), as opposed to controlled decision making, suggesting that the DMN plays a role in the autopilot mode of brain functioning.
In light of these premises, everyone can pause to analyze what he is doing, improving self-control to avoid “ego-depletion.” Thus, one can actively switch between one type of thinking and the other. The ability to make this switch makes the physician more performing. In addition, a physician can be trained to understand the ways of thinking and which type of thinking is engaged in various situations. This way, experience and methodology knowledge can energize Systems 1 and 2 and how they interact, avoiding cognitive errors. Figure 1 summarizes all the concepts abovementioned about the Dual Mode Network and its relationship with the DMN.
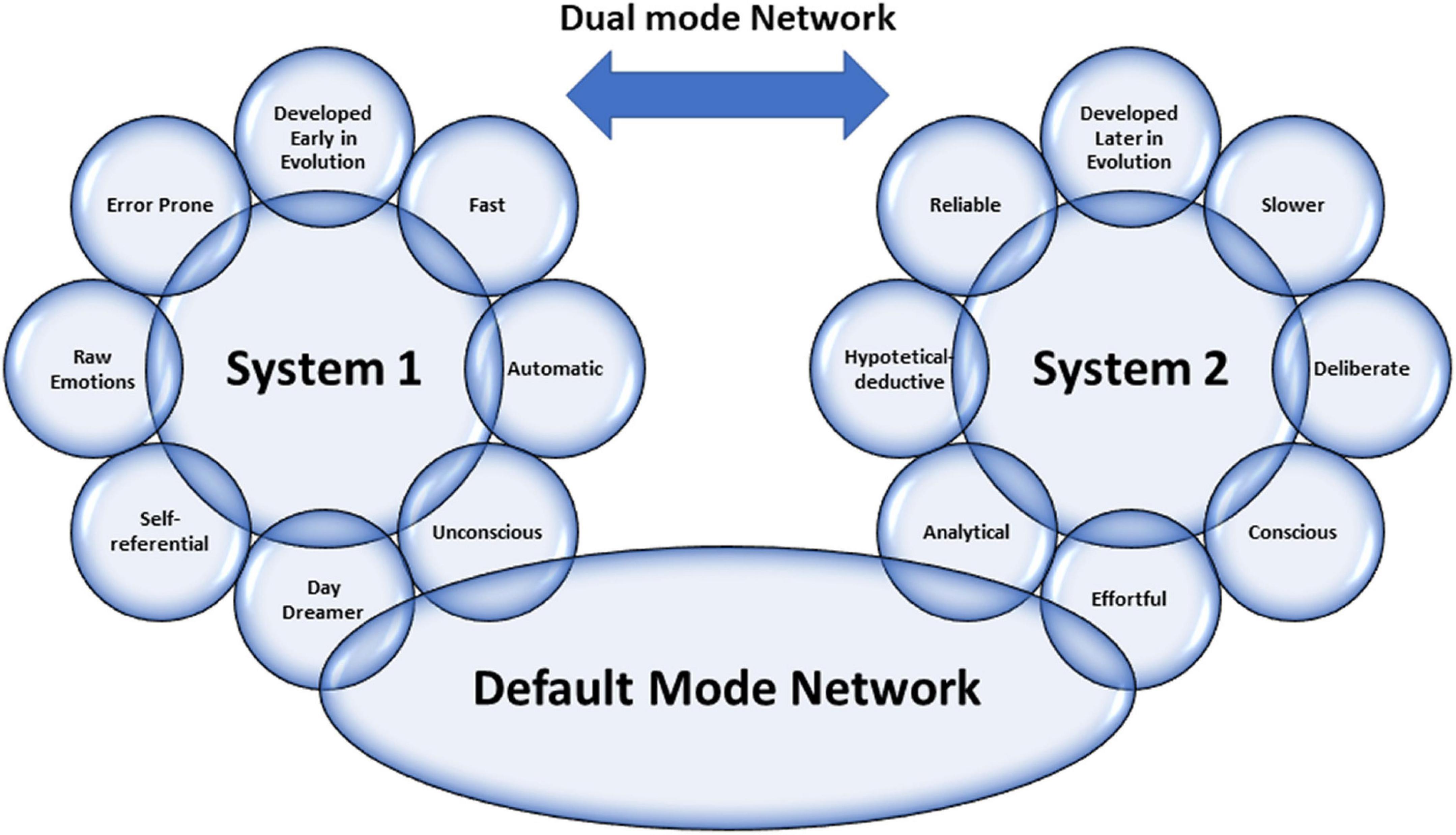
Figure 1. Graphical representation of the characteristics of Dual Mode Network, including the relationship between the two systems by Default Mode Network (view the text for explanations).
Emotional intelligence is another crucial factor in boosting clinical reasoning for the best decision-making applied to a single patient. Emotional intelligence recognizes one’s emotions. Those others label different feelings appropriately and use emotional information to guide thinking and behavior, adjust emotions, and create empathy, adapt to environments, and achieve goals ( 30 ). According to the phenomenological account of Fuchs, bodily perception (proprioception) has a crucial role in understanding others ( 31 ). In this sense, the proprioceptive skills of a physician can help his empathic understanding become elementary for empathy and communication with the patient. In line with Fuchs’ view, empathic understanding encompasses a bodily resonance and mediates contextual knowledge about the patient. For medical education, empathy should help to relativize the singular experience, helping to prevent that own position becomes exclusive, bringing oneself out of the center of one’s own perspective.
Reasoning errors and cognitive biases
Errors in reasoning play a significant role in diagnostic errors and may compromise patient safety and quality of care. A recently published review by Norman et al. ( 32 ) examined clinical reasoning errors and how to avoid them. To simplify this complex issue, almost five types of diagnostic errors can be recognized: no-fault errors, system errors, errors due to the knowledge gap, errors due to misinterpretation, and cognitive biases ( 9 ). Apart from the first type of error, which is due to unavoidable errors due to various factors, we want to mention cognitive biases. They may occur at any stage of the reasoning process and may be linked to intuition and analytical systems. The most frequent cognitive biases in medicine are anchoring, confirmation bias, premature closure, search satisficing, posterior probability error, outcome bias, and commission bias ( 33 ). Anchoring is characterized by latching onto a particular aspect at the initial consultation, and then one refuses to change one’s mind about the importance of the later stages of reasoning. Confirmation bias ignores the evidence against an initial diagnosis. Premature closure leads to a misleading diagnosis by stopping the diagnostic process before all the information has been gathered or verified. Search satisficing blinds other additional diagnoses once the first diagnosis is made posterior probability error shortcuts to the usual patient diagnosis for previously recognized clinical presentations. Outcome bias impinges on our desire for a particular outcome that alters our judgment (e.g., a surgeon blaming sepsis on pneumonia rather than an anastomotic leak). Finally, commission bias is the tendency toward action rather than inaction, assuming that only good can come from doing something (rather than “watching and waiting”). These biases are only representative of the other types, and biases often work together. For example, in overconfidence bias (the tendency to believe we know more than we do), too much faith is placed in opinion instead of gathered evidence. This bias can be augmented by the anchoring effect or availability bias (when things are at the forefront of your mind because you have seen several cases recently or have been studying that condition in particular), and finally by commission bias—with disastrous results.
Novice vs. expert approaches
The reasoning strategies used by novices are different from those used by experts ( 34 ). Experts can usually gather beneficial information with highly effective problem-solving strategies. Heuristics are commonly, and most often successfully, used. The expert has a saved bank of illness scripts to compare and contrast the current case using more often type 1 thinking with much better results than the novice. Novices have little experience with their problems, do not have time to build a bank of illness scripts, and have no memories of previous similar cases and actions in such cases. Therefore, their mind search strategies will be weak, slow, and ponderous. Heuristics are poor and more often unsuccessful. They will consider a more comprehensive range of diagnostic possibilities and take longer to select approaches to discriminate among them. A novice needs specific knowledge and specific experience to become an expert. In our opinion, he also needs special training in the different ways of thinking. It is possible to study patterns, per se as well. It is, therefore, likely to guide the growth of knowledge for both fast thinking and slow one.
Moreover, learning by osmosis has traditionally been the method to move the novice toward expert capabilities by gradually gaining experience while observing experts’ reasoning. However, it seems likely that explicit teaching of clinical reasoning could make this process quicker and more effective. In this sense, an increased need for training and clinical knowledge along with the skill to apply the acquired knowledge is necessary. Students should learn disease pathophysiology, treatment concepts, and interdisciplinary team communication developing clinical decision-making through case-series-derived knowledge combining associative and procedural learning processes such as “Vienna Summer School on Oncology” ( 35 ).
Moreover, a refinement of the training of communicative skills is needed. Improving communication skills training for medical students and physicians should be the university’s primary goal. In fact, adequate communication leads to a correct diagnosis with 76% accuracy ( 36 ). The main challenge for students and physicians is the ability to respond to patients’ individual needs in an empathic and appreciated way. In this regard, it should be helpful to apply qualitative studies through the adoption of a semi-structured or structured interview using face-to-face in-depth interviews and e-learning platforms which can foster interdisciplinary learning by developing expertise for the clinical reasoning and decision-making in each area and integrating them. They could be effective tools to develop clinical reasoning and decision-making competencies and acquire effective communication skills to manage the relationship with patient ( 37 – 40 ).
Clinical reasoning ways
Clinical reasoning is complex: it often requires different mental processes operating simultaneously during the same clinical encounter and other procedures for different situations. The dual-process theory describes how humans have two distinct approaches to decision-making ( 41 ). When one uses heuristics, fast-thinking (system 1) is used ( 42 ). However, complex cases need slow analytical thinking or both systems involved ( 15 , 43 , 44 ). Slow thinking can use different ways of reasoning: deductive, hypothetic-deductive, inductive, abductive, probabilistic, rule-based/categorical/deterministic, and causal reasoning ( 9 ). We think that abductive and causal reasoning need further explanation. Abductive reasoning is necessary when no deductive argument (from general assumption to particular conclusion) nor inductive (the opposite of deduction) may be claimed.
In the real world, we often face a situation where we have information and move backward to the likely cause. We ask ourselves, what is the most plausible answer? What theory best explains this information? Abduction is just a process of choosing the hypothesis that would best explain the available evidence. On the other hand, causal reasoning uses knowledge of medical sciences to provide additional diagnostic information. For example, in a patient with dyspnea, if considering heart failure as a casual diagnosis, a raised BNP would be expected, and a dilated vena cava yet. Other diagnostic possibilities must be considered in the absence of these confirmatory findings (e.g., pneumonia). Causal reasoning does not produce hypotheses but is typically used to confirm or refute theories generated using other reasoning strategies.
Hypothesis generation and modification using deduction, induction/abduction, rule-based, causal reasoning, or mental shortcuts (heuristics and rule of thumbs) is the cognitive process for making a diagnosis ( 9 ). Clinicians develop a hypothesis, which may be specific or general, relating a particular situation to knowledge and experience. This process is referred to as generating a differential diagnosis. The process we use to produce a differential diagnosis from memory is unclear. The hypotheses chosen may be based on likelihood but might also reflect the need to rule out the worst-case scenario, even if the probability should always be considered.
Given the complexity of the involved process, there are numerous causes for failure in clinical reasoning. These can occur in any reasoning and at any stage in the process ( 33 ). We must be aware of subconscious errors in our thinking processes. Cognitive biases are subconscious deviations in judgment leading to perceptual distortion, inaccurate assessment, and misleading interpretation. From an evolutionary point of view, they have developed because, often, speed is more important than accuracy. Biases occur due to information processing heuristics, the brain’s limited capacity to process information, social influence, and emotional and moral motivations.
Heuristics are mind shortcuts and are not all bad. They refer to experience-based techniques for decision-making. Sometimes they may lead to cognitive biases (see above). They are also essential for mental processes, expressed by expert intuition that plays a vital role in clinical practice. Intuition is a heuristic that derives from a natural and direct outgrowth of experiences that are unconsciously linked to form patterns. Pattern recognition is just a quick shortcut commonly used by experts. Alternatively, we can create patterns by studying differently and adequately in a notional way that accumulates information. The heuristic that rules out the worst-case scenario is a forcing mind function that commits the clinician to consider the worst possible illness that might explain a particular clinical presentation and take steps to ensure it has been effectively excluded. The heuristic that considers the least probable diagnoses is a helpful approach to uncommon clinical pictures and thinking about and searching for a rare unrecognized condition. Clinical guidelines, scores, and decision rules function as externally constructed heuristics, usually to ensure the best evidence for the diagnosis and treatment of patients.
Hence, heuristics are helpful mind shortcuts, but the exact mechanisms may lead to errors. Fast-and-frugal tree and take-the-best heuristic are two formal models for deciding on the uncertainty domain ( 45 ).
In the recent times, clinicians have faced dramatic changes in the pattern of patients acutely admitted to hospital wards. Patients become older and older with comorbidities, rare diseases are frequent as a whole ( 46 ), new technologies are growing in a logarithmic way, and sustainability of the healthcare system is an increasingly important problem. In addition, uncommon clinical pictures represent a challenge for clinicians ( 47 – 50 ). In our opinion, it is time to claim clinical reasoning as a crucial way to deal with all complex matters. At first, we must ask ourselves if we have lost the teachings of ancient masters. Second, we have to rethink medical school courses and training ones. In this way, cognitive debiasing is needed to become a well-calibrated clinician. Fundamental tools are the comprehensive knowledge of nature and the extent of biases other than studying cognitive processes, including the interaction between fast and slow thinking. Cognitive debiasing requires the development of good mindware and the awareness that one debiasing strategy will not work for all biases. Finally, debiasing is generally a complicated process and requires lifelong maintenance.
We must remember that medicine is an art that operates in the field of science and must be able to cope with uncertainty. Managing uncertainty is the skill we have to develop against an excess of confidence that can lead to error. Sound clinical reasoning is directly linked to patient safety and quality of care.
Data availability statement
The original contributions presented in this study are included in the article/supplementary material, further inquiries can be directed to the corresponding author.
Author contributions
SC and CA drafted the work and revised it critically. Both authors have approved the submission of the manuscript.
Conflict of interest
The authors declare that the research was conducted in the absence of any commercial or financial relationships that could be construed as a potential conflict of interest.
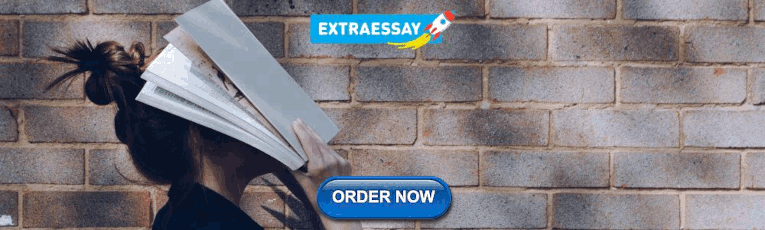
Publisher’s note
All claims expressed in this article are solely those of the authors and do not necessarily represent those of their affiliated organizations, or those of the publisher, the editors and the reviewers. Any product that may be evaluated in this article, or claim that may be made by its manufacturer, is not guaranteed or endorsed by the publisher.
1. Groopman J. How doctors think. New York, NY: Houghton Mifflin Company (2007).
Google Scholar
2. Montgomery K. How doctors think: clinical judgment and the practice of medicine. Oxford: Oxford University Press (2006).
3. Croskerry P. Cognitive and affective dispositions to respond. In: P Croskerry, K Cosby, S Schenkel, R Wears editors. Patient safety in emergency medicine. (Philadelphia, PA: Lippincott, Williams & Wilkins) (2008). p. 219–27.
4. Croskerry P, Norman G. Overconfidence in clinical decision making. Am J Med. (2008) 121:S24–9.
5. Hammond K. Human judgment and social policy: irreducible uncertainty, inevitable error, unavoidable injustice. New York, NY: Oxford University Press (2000).
6. Croskerry P. Context is everything, or: how could I have been that stupid? Healthcare Q. (2009) 12:167–73.
7. Bergl PA. At Baseline. N Engl J Med. (2019) 380:1792–3. doi: 10.1056/NEJMp1900543
PubMed Abstract | CrossRef Full Text | Google Scholar
8. Dewey J. How we think. Boston, MA: D.C. Heath (1933).
9. Cooper N, Frain J. ABC of clinical reasoning sound and rational decision making. Hoboken, NJ: Wiley-Blackwell (2016).
10. Kassirer JP, Kopelman RI. Learning clinical reasoning. Baltimore: Williams & Wilkins (1991).
11. Evans JS. In two minds: dual-process accounts of reasoning. Trends Cogn Sci. (2003) 7:454–9. doi: 10.1016/j.tics.2003.08.012
12. Evans JS. Dual-processing accounts of reasoning, judgment, and social cognition. Annu Rev Psychol. (2008) 59:255–78. doi: 10.1146/annurev.psych.59.103006.093629
13. Osman M. An evaluation of dual-process theories of reasoning. Psychon Bull Rev. (2004) 11:988–1010. doi: 10.3758/bf03196730
14. Evans JS, Stanovich KE. Dual-process theories of higher cognition: advancing the debate. Perspect Psychol Sci. (2013) 8:223–41. doi: 10.1177/1745691612460685
15. Kahneman D. Thinking, Fast and Slow. London: Macmillan (2011).
16. Masicampo EJ, Baumeister RF. Toward a physiology of dual-process reasoning and judgment: Lemonade, willpower, and expensive rule-based analysis. Psychol Sci. (2008) 19:255–60. doi: 10.1111/j.1467-9280.2008.02077.x
17. Gronchi G, Giovannelli F. Dual process theory of thought and default mode network: a possible neural foundation of fast thinking. Front Psychol. (2018) 9:1237. doi: 10.3389/fpsyg.2018.01237
18. Muraven M, Tice DM, Banmeister RE. Self-control as limited resource: regulatory depletion patterns. J Pers Soc Psychol. (1998) 74:774–89. doi: 10.1037//0022-3514.74.3.774
CrossRef Full Text | Google Scholar
19. Baumeister RF, Bratslavsky E, Muraven M, Tice DM. Ego depletion: is the active self a limited resource? J Pers Soc Psychol. (1998) 74:1252–65. doi: 10.1037//0022-3514.74.5.1252
20. Dang CP, Braeken J, Colom R, Ferrer E, Liu C. Why is working memory related to intelligence? Different contributions from storage and processing. Memory. (2014) 22:426–41. doi: 10.1080/09658211.2013.797471
21. Dang HM, Weiss B, Nguyen CM, Tran N, Pollack A. Vietnam as a case example of school-based mental health services in low- and middle-income countries: efficacy and effects of risk status. Sch Psy chol Int. (2017) 38:22–41. doi: 10.1177/0143034316685595
22. Biswal B, Yetkin FZ, Haughton VM, Hyde JS. Functional connectivity in the motor cortex of resting human brain using echo-planar MRI. Magn Reson Med. (1995) 34:537–41. doi: 10.1002/mrm.1910340409
23. Biswal BB. Resting state fMRI: a personal history. Neuroimage. (2012) 62:938–44. doi: 10.1016/j.neuroimage.2012.01.090
24. Bernard JA, Seidler RD, Hassevoort KM, Benson BL, Welsh RC, Wiggins JL, et al. Resting-state cortico-cerebellar functional connectivity networks: a comparison of anatomical and self-organizing map approaches. Front Neuroanat. (2012) 6:31. doi: 10.3389/fnana.2012.00031
25. Snyder AZ, Raichle ME. A brief history of the resting state: the Washington University perspective. Neuroimage. (2012) 62:902–10. doi: 10.1016/j.neuroimage.2012.01.044
26. Buckner RL, Andrews-Hanna JR, Schacter DL. The brain’s default network: anatomy, function, and relevance to disease. Ann N Y Acad Sci. (2008) 1124:1–38. doi: 10.1196/annals.1440.011
27. Raichle ME, MacLeod AM, Snyder AZ, Powers WJ, Gusnard DA, Shulman GL. A default mode of brain function. Proc Natl Acad Sci U.S.A. (2001) 98:676–82. doi: 10.1073/pnas.98.2.676
28. Raichle ME, Snyder AZ. A default mode of brain function: a brief history of an evolving idea. Neuroimage. (2007) 37:1083–90. doi: 10.1016/j.neuroimage.2007.02.041
29. Vatansever D, Menon DK, Stamatakis EA. Default mode contributions to automated information processing. Proc Natl Acad Sci U.S.A. (2017) 114:12821–6. doi: 10.1073/pnas.1710521114
30. Hutchinson M, Hurley J, Kozlowski D, Whitehair L. The use of emotional intelligence capabilities in clinical reasoning and decision-making: a qualitative, exploratory study. J Clin Nurs. (2018) 27:e600–10. doi: 10.1111/jocn.14106
31. Schmidsberger F, Löffler-Stastka H. Empathy is proprioceptive: the bodily fundament of empathy – a philosophical contribution to medical education. BMC Med Educ. (2018) 18:69. doi: 10.1186/s12909-018-1161-y
32. Norman GR, Monteiro SD, Sherbino J, Ilgen JS, Schmidt HG, Mamede S. The causes of errors in clinical reasoning: cognitive biases, knowledge deficits, and dual process thinking. Acad Med. (2017) 92:23–30. doi: 10.1097/ACM.0000000000001421
33. Croskerry P. The importance of cognitive errors in diagnosis and strategies to minimize them. Acad Med. (2003) 78:775–80. doi: 10.1097/00001888-200308000-00003
34. Persky AM, Robinson JD. Moving from novice to expertise and its implications for instruction. Am J Pharm Educ. (2017) 81:6065. doi: 10.5688/ajpe6065
35. Lütgendorf-Caucig C, Kaiser PA, Machacek A, Waldstein C, Pötter R, Löffler-Stastka H. Vienna summer school on oncology: how to teach clinical decision making in a multidisciplinary environment. BMC Med Educ. (2017) 17:100. doi: 10.1186/s12909-017-0922-3
36. Peterson MC, Holbrook JH, Von Hales D, Smith NL, Staker LV. Contributions of the history, physical examination, and laboratory investigation in making medical diagnoses. West J Med. (1992) 156:163–5.
37. Seitz T, Raschauer B, Längle AS, Löffler-Stastka H. Competency in medical history taking-the training physicians’ view. Wien Klin Wochenschr. (2019) 131:17–22. doi: 10.1007/s00508-018-1431-z
38. Lo Monaco M, Mallaci Bocchio R, Natoli G, Scibetta S, Bongiorno T, Argano C, et al. Human relationships in patients’ end-of-life: a qualitative study in a hospice ward. Intern Emerg Med. (2020) 15:975–80. doi: 10.1007/s11739-019-02254-6
39. Turk B, Ertl S, Wong G, Wadowski PP, Löffler-Stastka H. Does case-based blended-learning expedite the transfer of declarative knowledge to procedural knowledge in practice? BMC Med Educ. (2019) 19:447. doi: 10.1186/s12909-019-1884-4
40. Corrao S, Colomba D, Arnone S, Argano C, Di Chiara T, Scaglione R, et al. Improving efficacy of pubmed clinical queries for retrieving scientifically strong studies on treatment. J Am Med Inform Assoc. (2006) 13:485–7. doi: 10.1197/jamia.M2084
41. Houlihan S. Dual-process models of health-related behaviour and cognition: a review of theory. Public Health. (2018) 156:52–9. doi: 10.1016/j.puhe.2017.11.002
42. Gibbons LJ, Stoddart K. ’Fast and frugal heuristics’: clinical decision making in the emergency department. Int Emerg Nurs. (2018) 41:7–12. doi: 10.1016/j.ienj.2018.04.002
43. Brusovansky M, Glickman M, Usher M. Fast and effective: intuitive processes in complex decisions. Psychon Bull Rev. (2018) 25:1542–8. doi: 10.3758/s13423-018-1474-1
44. Hallsson BG, Siebner HR, Hulme OJ. Fairness, fast and slow: a review of dual process models of fairness. Neurosci Biobehav Rev. (2018) 89:49–60. doi: 10.1016/j.neubiorev.2018.02.016
45. Bobadilla-Suarez S, Love BC. Fast or frugal, but not both: decision heuristics under time pressure. J Exp Psychol Learn Mem Cogn. (2018) 44:24–33. doi: 10.1037/xlm0000419
46. Corrao S, Natoli G, Nobili A, Mannucci PM, Pietrangelo A, Perticone F, et al. RePoSI Investigators. comorbidity does not mean clinical complexity: evidence from the RePoSI register. Intern Emerg Med. (2020) 15:621–8. doi: 10.1007/s11739-019-02211-3
47. Corrao S, D’Alia R, Caputo S, Arnone S, Pardo GB, Jefferson T. A systematic approach to medical decision-making of uncommon clinical pictures: a case of ulcerative skin lesions by palm tree thorn injury and a one-year follow-up. Med Inform Internet Med. (2005) 30:203–10. doi: 10.1080/14639230500209104
48. Colomba D, Cardillo M, Raffa A, Argano C, Licata G. A hidden echocardiographic pitfall: the Gerbode defect. Intern Emerg Med. (2014) 9:237–8. doi: 10.1007/s11739-013-1009-8
49. Argano C, Colomba D, Di Chiara T, La Rocca E. Take the wind out your sails: [Corrected] relationship among energy drink abuse, hypertension, and break-up of cerebral aneurysm. Intern Emerg Med. (2012) 7:S9–10. doi: 10.1007/s11739-011-0523-9
50. Corrao S, Amico S, Calvo L, Barone E, Licata G. An uncommon clinical picture: Wellens’ syndrome in a morbidly obese young man. Intern Emerg Med. (2010) 5:443–5. doi: 10.1007/s11739-010-0374-9
Keywords : clinical reasoning, metacognition, cognitive biases, Default Mode Network (DMN), clinical decision making
Citation: Corrao S and Argano C (2022) Rethinking clinical decision-making to improve clinical reasoning. Front. Med. 9:900543. doi: 10.3389/fmed.2022.900543
Received: 20 March 2022; Accepted: 16 August 2022; Published: 08 September 2022.
Reviewed by:
Copyright © 2022 Corrao and Argano. This is an open-access article distributed under the terms of the Creative Commons Attribution License (CC BY) . The use, distribution or reproduction in other forums is permitted, provided the original author(s) and the copyright owner(s) are credited and that the original publication in this journal is cited, in accordance with accepted academic practice. No use, distribution or reproduction is permitted which does not comply with these terms.
*Correspondence: Salvatore Corrao, [email protected] ; [email protected]
Disclaimer: All claims expressed in this article are solely those of the authors and do not necessarily represent those of their affiliated organizations, or those of the publisher, the editors and the reviewers. Any product that may be evaluated in this article or claim that may be made by its manufacturer is not guaranteed or endorsed by the publisher.
- Open access
- Published: 02 September 2024
Clinician perspectives and recommendations regarding design of clinical prediction models for deteriorating patients in acute care
- Robin Blythe ORCID: orcid.org/0000-0002-3643-4332 1 ,
- Sundresan Naicker ORCID: orcid.org/0000-0002-2392-4981 1 ,
- Nicole White ORCID: orcid.org/0000-0002-9292-0773 1 ,
- Raelene Donovan ORCID: orcid.org/0000-0003-0737-7719 2 ,
- Ian A. Scott ORCID: orcid.org/0000-0002-7596-0837 3 , 4 ,
- Andrew McKelliget 2 &
- Steven M McPhail ORCID: orcid.org/0000-0002-1463-662X 1 , 4
BMC Medical Informatics and Decision Making volume 24 , Article number: 241 ( 2024 ) Cite this article
Metrics details
Successful deployment of clinical prediction models for clinical deterioration relates not only to predictive performance but to integration into the decision making process. Models may demonstrate good discrimination and calibration, but fail to match the needs of practising acute care clinicians who receive, interpret, and act upon model outputs or alerts. We sought to understand how prediction models for clinical deterioration, also known as early warning scores (EWS), influence the decision-making of clinicians who regularly use them and elicit their perspectives on model design to guide future deterioration model development and implementation.
Nurses and doctors who regularly receive or respond to EWS alerts in two digital metropolitan hospitals were interviewed for up to one hour between February 2022 and March 2023 using semi-structured formats. We grouped interview data into sub-themes and then into general themes using reflexive thematic analysis. Themes were then mapped to a model of clinical decision making using deductive framework mapping to develop a set of practical recommendations for future deterioration model development and deployment.
Fifteen nurses ( n = 8) and doctors ( n = 7) were interviewed for a mean duration of 42 min. Participants emphasised the importance of using predictive tools for supporting rather than supplanting critical thinking, avoiding over-protocolising care, incorporating important contextual information and focusing on how clinicians generate, test, and select diagnostic hypotheses when managing deteriorating patients. These themes were incorporated into a conceptual model which informed recommendations that clinical deterioration prediction models demonstrate transparency and interactivity, generate outputs tailored to the tasks and responsibilities of end-users, avoid priming clinicians with potential diagnoses before patients were physically assessed, and support the process of deciding upon subsequent management.
Conclusions
Prediction models for deteriorating inpatients may be more impactful if they are designed in accordance with the decision-making processes of acute care clinicians. Models should produce actionable outputs that assist with, rather than supplant, critical thinking.
• This article explored decision-making processes of clinicians using a clinical prediction model for deteriorating patients, also known as an early warning score.
• Our study identified that the clinical utility of deterioration models may lie in their assistance in generating, evaluating, and selecting diagnostic hypotheses, an important part of clinical decision making that is underrepresented in the prediction modelling literature.
• Nurses in particular stressed the need for models that encourage critical thinking and further investigation rather than prescribe strict care protocols.
Peer Review reports
The number of ‘clinical prediction model’ articles published on PubMed has grown rapidly over the past two decades, from 1,918 articles identified with these search terms published in 2002 to 26,326 published in 2022. A clinical prediction model is defined as any multivariable model that provides patient-level estimates of the probability or risk of a disease, condition or future event [ 1 , 2 , 3 ].
Recent systematic and scoping reviews report a lack of evidence that clinical decision support systems based on prediction models are associated with improved patient outcomes once implemented in acute care [ 4 , 5 , 6 , 7 ]. One potential reason may be that some models are not superior to clinical judgment in reducing missed diagnoses or correctly classifying non-diseased patients [ 8 ]. While improving predictive accuracy is important, this appears insufficient for improving patient outcomes, suggesting that more attention should be paid to the process and justification of how prediction models are designed and deployed [ 9 , 10 ].
If model predictions are to influence clinical decision-making, they must not only demonstrate acceptable accuracy, but also be implemented and adopted at scale in clinical settings. This requires consideration of how they are integrated into clinical workflows, how they generate value for users, and how clinicians perceive and respond to their outputs of predicted risks [ 11 , 12 ]. These concepts are tenets of user-centred design, which focuses on building systems based on the needs and responsibilities of those who will use them. User-centred decision support tools can be designed in a variety of ways, but may benefit from understanding the characteristics of the users and the local environment in which tools are implemented, [ 13 ] the nature of the tasks end-users are expected to perform, [ 14 ] and the interface between the user and the tools [ 15 ].
Prediction models for clinical deterioration
A common task for prediction models integrated into clinical decision support systems is in predicting or recognising clinical deterioration, also known as early warning scores. Clinical deterioration is defined as the transition of a patient from their current health state to a worse one that puts them at greater risk of adverse events and death [ 16 ]. Early warning scores were initially designed to get the attention of skilled clinicians when patients began to deteriorate, but have since morphed into complex multivariable prediction models [ 17 ]. As with many other clinical prediction models, early warning scores often fail to demonstrate better patient outcomes once deployed [ 4 , 18 ]. The clinical utility of early warning scores likely rests on two key contextual elements: the presence of uncertainty, both in terms of diagnosis and prognosis, and the potential for undesirable patient outcomes if an appropriate care pathway is delayed or an inappropriate one is chosen [ 19 ].
The overarching goal of this qualitative study was to determine how prediction models for clinical deterioration, or early warning scores, could be better tailored to the needs of end-users to improve inpatient care. This study had three aims. First, to understand the experiences and perspectives of nurses and doctors who use early warning scores. Second, to identify the tasks these clinicians performed when managing deteriorating patients, the decision-making processes that guided these tasks, and how these could be conceptualised schematically. Finally, to address these tasks and needs with actionable, practical recommendations for enhancing future deterioration prediction model development and deployment.
To achieve our study aims, we conducted semi-structured interviews of nurses and doctors at two large, digitally mature hospitals. We first asked clinicians to describe their backgrounds, perspectives, and experience with early warning scores to give context to our analysis. We then examined the tasks and responsibilities of participants and the decision-making processes that guided these tasks using reflexive thematic analysis, an inductive method that facilitated the identification of general themes. We then identified a conceptual decision-making framework from the literature to which we mapped these themes to understand how they may lead to better decision support tools. Finally, we used this framework to formulate recommendations for deterioration prediction model design and deployment. These steps are presented graphically in a flow diagram (Fig. 1 ).
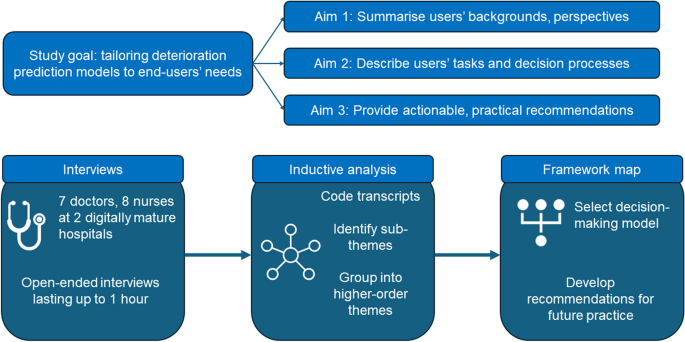
Schema of study goal, aims and methods
The study was conducted at one large tertiary and one medium-sized metropolitan hospital in Brisbane, Australia. The large hospital contained over 1,000 beds, handling over 116,000 admissions and approximately 150,000 deterioration alerts per year in 2019. Over the same period, the medium hospital contained 175 beds, handling over 31,000 admissions and approximately 42,000 deterioration alerts per year. These facilities had a high level of digital maturity, including fully integrated electronic medical records.
Clinical prediction model for deteriorating patients
The deterioration monitoring system used at both hospitals was the Queensland Adult Deterioration Detection System (Q-ADDS) [ 20 , 21 ]. Q-ADDS uses an underlying prediction model to convert patient-level vital signs from a single time of observation into an ordinal risk score describing an adult patient’s risk of acute deterioration. Vital signs collected are respiratory rate (breaths/minute), oxygen flow rate (L/minute), arterial oxygen saturation (percent), blood pressure (mmHg), heart rate (beats/minute), temperature (degrees Celsius), level of consciousness (Alert-Voice-Pain-Unresponsive) and increased or new onset agitation. Increased pain and urine output are collected but not used for score calculation [ 21 ]. The Q-ADDS tool is included in the supplementary material.
Vital signs are entered into the patient’s electronic medical record, either imported from the vital signs monitoring device at the patient’s bedside or from manual entry by nurses. Calculations are made automatically within Q-ADDS to generate an ordinal risk score per patient observation. Scores can be elevated to levels requiring a tiered escalation response if a single vital sign is greatly deranged, or if several observations are deranged by varying degrees. Scores range from 0 to 8+, with automated alerts and escalation protocols ranging from more frequent observations for lower scores to immediate activation of the medical emergency team (MET) at higher scores.
The escalation process for Q-ADDS is highly structured, mandated and well documented [ 21 ]. Briefly, when a patient’s vital signs meet a required alert threshold, the patient’s nurse is required to physically assess the patient and, depending on the level of severity predicted by Q-ADDS, notify the patient’s doctor (escalation). The doctor is then required to be notified of the patient’s Q-ADDS score, potentially review the patient, and discuss any potential changes to care with the nurse. Both nurses and doctors can escalate straight to MET calls or an emergency ‘code blue’ call (requiring cardiopulmonary resuscitation or assisted ventilation) at any time if necessary.
Participant recruitment
Participant recruitment began in February 2022 and concluded in March 2023, disrupted by the COVID-19 pandemic. Eligibility criteria were nurses or doctors at each hospital with direct patient contact who either receive or respond, respectively, to Q-ADDS alerts. An anticipated target sample size of 15 participants was established prior to recruitment, based on expected constraints in recruitment due to clinician workloads and the expected length of interviews relative to their scope, as guided by prior research [ 22 ]. As the analysis plan involved coding interviews iteratively as they were conducted, the main justification for ceasing recruitment was when no new themes relating to the study objectives were generated during successive interviews as the target sample size was approached [ 23 ].
Study information was broadly distributed via email to nurses and doctors in patient-facing roles across hospitals. Nurse unit managers were followed up during regular nursing committee meetings to participate or assist with recruitment within their assigned wards. Doctors were followed up by face-to-face rounding. Snowball sampling, in which participants were encouraged to refer their colleagues for study participation, was employed whenever possible. In all cases, study authors explained study goals and distributed participant consent forms prior to interview scheduling with the explicit proviso that participation was completely voluntary and anonymous to all but two study authors (RB and SN).
Interview process
We used a reflexive framework method to develop an open-ended interview template [ 24 ] that aligned with our study aims. Interview questions were informed by the non-adoption, abandonment, scale-up, spread and sustainability (NASSS) framework [ 25 ]. The NASSS framework relates the end-user perceptions of the technology being evaluated to its value proposition for the clinical situation to which it is being applied. We selected a reflexive method based on the NASSS for our study as we wanted to allow end-users to speak freely about the barriers they faced when using prediction models for clinical deterioration, but did not limit participants to discussing only topics that could fit within the NASSS framework.
Participants were first asked about their background and clinical expertise. They were then invited to share their experiences and perspectives with using early warning scores to manage deteriorating patients. This was used as a segue for participants to describe the primary tasks required of them when evaluating and treating a deteriorating patient. Participants were encouraged to talk through their decision-making process when fulfilling these tasks, and to identify any barriers or obstacles to achieving those tasks that were related to prediction models for deteriorating patients. Participants were specifically encouraged to identify any sources of information that were useful for managing deteriorating patients, including prediction models for other, related disease groups like sepsis, and to think of any barriers or facilitators for making that information more accessible. Finally, participants were invited to suggest ways to improve early warning scores, and how those changes may lead to benefits for patients and clinicians.
As we employed a reflexive methodology to allow clinicians to speak freely about their perspectives and opinions, answers to interview questions were optional and open-ended, allowing participants to discuss relevant tangents. Separate interview guides were developed for nurses and doctors as the responsibilities and information needs of these two disciplines in managing deteriorating patients often differ. Nurses are generally charged with receiving and passing on deterioration alerts, while doctors are generally charged with responding to alerts and making any required changes to patient care plans [ 4 ]. Interview guides are contained in the supplement.
Due to clinician workloads, member checking, a form of post-interview validation in which participants retrospectively confirm their interview answers, was not used. To ensure participants perceived the interviewers as being impartial, two study authors not employed by the hospital network and not involved in direct patient care (RB and SN) were solely responsible for conducting interviews and interrogating interview transcripts. Interviews were recorded and transcribed verbatim, then re-checked for accuracy.
Inductive thematic analysis
Transcripts were analysed using a reflexive thematic methodology informed by Braun and Clarke [ 26 ]. This method was selected because it facilitated exploring the research objectives rather than being restricted to the domains of a specific technology adoption framework, which may limit generalisability [ 27 ]. Interviews were analysed over five steps to identify emergent themes.
Each interview was broken down into segments by RB and SN, where segments corresponded to a distinct opinion.
Whenever appropriate, representative quotes for each distinct concept were extracted.
Segments were grouped into sub-themes.
Sub-themes were grouped into higher-order themes, or general concepts.
Steps 1 through 4 were iteratively repeated by RB and supervised by SN.
As reflexive methods incorporate the experiences and expertise of the analysts, our goal was to extract any sub-themes relevant to the study aims and able to be analysed in the context of early warning scores, prediction models, or decision support tools for clinical deterioration. The concepts explored during this process were not exhaustive, but repeated analysis and re-analysis of participant transcripts helped to ensure all themes could be interpreted in the context of our three study aims: background and perspectives, tasks and decision-making, and recommendations for future practice.
Deductive mapping to a clinical decision-making framework
Once the emergent themes from the inductive analysis were defined, we conducted a brief scan of PubMed for English-language studies that investigated how the design of clinical decision support systems relate to clinical decision-making frameworks. The purpose of this exercise was to identify a framework against which we could map the previously elicited contexts, tasks, and decision-making of end-users in developing a decision-making model that could then be used to support the third aim of formulating recommendations to enhance prediction model development and deployment.
RB and SN then mapped higher-order themes from the inductive analysis to the decision-making model based on whether there was a clear relationship between each theme and a node in the model (see Results).
Recommendations for improving prediction model design were derived by reformatting the inductive themes based on the stated preferences of the participants. These recommendations were then assessed by the remaining authors and the process repeated iteratively until authors were confident that all recommendations were concordant with the decision-making model.
Participant characteristics
Our sample included 8 nurses and 7 doctors of varying levels of expertise and clinical specialties; further information is contained in the supplement. Compared to doctors, nurse participants were generally more experienced, often participating in training or mentoring less experienced staff. Clinical specialities of nurses were diverse, including orthopaedics, cancer services, medical assessment and planning unit, general medicine, and pain management services. Doctor participants ranged from interns with less than a year of clinical experience up to consultant level, including three doctors doing training rotations and two surgical registrars. Clinical specialties of doctors included geriatric medicine, colorectal surgery, and medical education.
Interviews and thematic analysis
Eleven interviews were conducted jointly by RB and SN, one conducted by RB, and three by SN. Interviews were scheduled for up to one hour, with a mean duration of 42 min. Six higher-order themes were identified. These were: added value of more information; communication of model outputs; validation of clinical intuition; capability for objective measurement; over-protocolisation of care; and model transparency and interactivity (Table 1 ). Some aspects of care, including the need for critical thinking and the informational value of discerning trends in patient observations, were discussed in several contexts, making them relevant to more than one higher-order theme.
Added value of other information
Clinicians identified that additional data or variables important for decision making were often omitted from the Q-ADDS digital interface. Such variables included current medical conditions, prescribed medications and prior observations, which were important for interpreting current patient data in the context of their baseline observations under normal circumstances (e.g., habitually low arterial oxygen saturation due to chronic obstructive pulmonary disease) or in response to an acute stimulus (e.g., expected hypotension for next 4 to 8 h while treatment for septic shock is underway).
“The trend is the biggest thing [when] looking at the data , because sometimes people’s observations are deranged forever and it’s not abnormal for them to be tachycardic , whereas for someone else , if it’s new and acute , then that’s a worry.” – Registrar.
Participants frequently emphasised the critical importance of looking at patients holistically, or that patients were more than the sum of the variables used to predict risk. Senior nurses stressed that prediction models were only one part of patient evaluation, and clinicians should be encouraged to incorporate both model outputs and their own knowledge and experiences in decision making rather than trust models implicitly. Doctors also emphasised this holistic approach, adding that they placed more importance on hearing a nurse was concerned for the patient than seeing the model output. Critical thinking about future management was frequently raised in this context, with both nurses and doctors insisting that model predictions and the information required for contextualising risk scores should be communicated together when escalating the patient’s care to more senior clinicians.
Model outputs
Model outputs were discussed in two contexts. First, doctors perceived that ordinal risk scores generated by Q-ADDS felt arbitrary compared to receiving probabilities of a future event, for example cardiorespiratory decompensation, that required a response such as resuscitation or high-level treatment. However, nurses did not wholly embrace probabilities as outputs, instead suggesting that recommendations for how they should respond to different Q-ADDS scores were more important. This difference may reflect the different roles of alert receivers (nurses) and alert responders (doctors).
“[It’s helpful] if you use probabilities… If your patient has a sedation score of 2 and a respiratory rate of 10 , [giving them] a probability of respiratory depression would be helpful. However , I don’t find many clinicians , and certainly beginning practitioners , think in terms of probabilities.” – Clinical nurse consultant.
Second, there was frequent mention of alert fatigue in the context of model outputs. One doctor and two nurses felt there was insufficient leeway for nurses to exercise discretion in responding to risk scores, leading to many unnecessary alert-initiated actions. More nuance in the way Q-ADDS outputs were delivered to clinicians with different roles was deemed important to avoid model alerts being perceived as repetitive and unwarranted. However, three other doctors warned against altering MET call criteria in response to repetitive and seemingly unchanging risk scores and that at-risk patients should, as a standard of care, remain under frequent observation. Frustrations centred more often around rigidly tying repetitive Q-ADDS outputs to certain mandated actions, leading to multiple clinical reviews in a row for a patient whose trajectory was predictable, for example a patient with stable heart failure having a constantly low blood pressure. This led to duplication of nursing effort (e.g., repeatedly checking the blood pressure) and the perception that prediction models were overly sensitive.
“It takes away a lot of nurses’ critical judgement. If someone’s baseline systolic [blood pressure] is 95 [mmHg] , they’re asymptomatic and I would never hear about it previously. We’re all aware that this is where they sit and that’s fine. Now they are required to notify me in the middle of the night , “Just so you know , they’ve dropped to 89 [below an alert threshold of 90mmHg].“” – Junior doctor.
Validation of clinical intuition
Clinicians identified the ability of prediction models to validate their clinical intuition as both a benefit and a hindrance, depending on how outputs were interpreted and acted upon. Junior clinicians appreciated early warning scores giving them more support to escalate care to senior clinicians, as a conversation starter or framing a request for discussion. Clinicians described how assessing the patient holistically first, then obtaining model outputs to add context and validate their diagnostic hypotheses, was very useful in deciding what care should be initiated and when.
“You kind of rule [hypotheses] out… you go to the worst extreme: is it something you need to really be concerned about , especially if their [score] is quite high? You’re thinking of common complications like blood clots , so that presents as tachycardic… I’m thinking of a PE [pulmonary embolism] , then you do the nursing interventions.” – Clinical nurse manager.
While deterioration alerts were often seen as triggers to think about potential causes for deterioration, participants noted that decision making could be compromised if clinicians were primed by model outputs to think of different diagnoses before they had fully assessed the patient at the bedside. Clinicians described the dangers of tunnel vision or, before considering all available clinical information, investigating favoured diagnoses to the exclusion of more likely causes.
“[Diagnosis-specific warnings are] great , [but] that’s one of those things that can lead to a bit of confirmation bias… It’s a good trigger to articulate , “I need to look for sources of infection when I go to escalate"… but then , people can get a little bit sidetracked with that and ignore something more blatant in front of them. I’ve seen people go down this rabbit warren of being obsessed with the “fact” that it was sepsis , but it was something very , very unrelated.” – Nurse educator.
Objective measurement
Clinicians perceived that prediction models were useful as more objective measures of patients’ clinical status that could ameliorate clinical uncertainty or mitigate cognitive biases. In contrast to the risk of confirmation bias arising from front-loading model outputs suggesting specific diagnoses, prediction models could offer a second opinion that could help clinicians recognise opposing signals in noisy data that, in particular, assisted in considering serious diagnoses that shouldn’t be missed (e.g., sepsis), or more frequent and easily treated diagnoses (e.g., dehydration). Prediction models were also useful when they disclosed several small, early changes in patient status that provided an opportunity for early intervention.
“Maybe [the patient has] a low grade fever , they’re a bit tachycardic. Maybe [sepsis] isn’t completely out of the blue for this person. If there was some sort of tool , that said there’s a reasonable chance that they could have sepsis here , I would use that to justify the option of going for blood cultures and maybe a full septic screen. If [I’m indecisive] , that sort of information could certainly push me in that direction.” – Junior doctor.
Clinicians frequently mentioned that prediction models would have been more useful when first starting clinical practice, but become less useful with experience. However, clinicians noted that at any experience level, risk scoring was considered most useful as a triage/prioritisation tool, helping decide which patients to see first, or which clinical concerns to address first.
“[Doctors] can easily triage a patient who’s scoring 4 to 5 versus 1 to 3. If they’re swamped , they can change the escalation process , or triage appropriately with better communication.” – Clinical nurse manager.
Clinicians also stressed that predictions were not necessarily accurate because measurement error or random variation, especially one-off outlier values for certain variables, was a significant contributor to false alerts and inappropriate responses. For example, a single unusually high respiratory rate generated an unusually high risk score, prompting an unnecessary alert.
Over-protocolisation of care
The sentiment most commonly expressed by all experienced nursing participants and some doctors was that nurses were increasingly being trained to solely react to model outputs with fixed response protocols, rather than think critically about what is happening to patients and why. It was perceived that prediction models may actually reduce the capacity for clinicians to process and internalise important information. For example, several nurses observed their staff failing to act on their own clinical suspicions that patients were deteriorating because the risk score had not exceeded a response threshold.
“We’ve had patients on the ward that have had quite a high tachycardia , but it’s not triggering because it’s below the threshold to trigger… [I often need to make my staff] make the clinical decision that they can call the MET anyway , because they have clinical concern with the patient.” – Clinical nurse consultant.
A source of great frustration for many nurses was the lack of critical thinking by their colleagues of possible causes when assessing deteriorating patients. They wanted their staff to investigate whether early warning score outputs or other changes in patient status were caused by simple, easily fixable issues such as fitting the oxygen mask properly and helping the patient sit up to breathe more easily, or whether they indicated more serious underlying pathophysiology. Nurses repeatedly referenced the need for clinicians to always be asking why something was happening, not simply reacting to what was happening.
“[Models should also be] trying to get back to critical thinking. What I’m seeing doesn’t add up with the monitor , so I should investigate further than just simply calling the code.” – Clinical nurse educator.
Model transparency and interactivity
Clinicians frequently requested more transparent and interactive prediction models. These included a desire to receive more training in how prediction models worked and how risk estimates were generated mathematically, and being able to visualise important predictors of deterioration and the absolute magnitude of their effects (effect sizes) in intuitive ways. For example, despite receiving training in Q-ADDS, nurses expressed frustrations that nobody at the hospital seemed to understand how it worked in generating risk scores. Doctors were interested in being able to visualise the relative size and direction of effect of different model variables, potentially using colour-coding, combined with other contextual patient data like current vital sign trends and medications, and presented on one single screen.
The ability to modify threshold values for model variables and see how this impacted risk scores, and what this may then mean for altering MET calling criteria, was also discussed. For example, in an older patient with an acute ischaemic stroke, a persistently high, asymptomatic blood pressure value is an expected bodily response to this acute insult over the first 24–48 h. In the absence of any change to alert criteria, recurrent alerts would be triggered which may encourage overtreatment and precipitous lowering of the blood pressure with potential to cause harm. Altering the criteria to an acceptable or “normal” value for this clinical scenario (i.e. a higher than normal blood pressure) may generate a lower, more patient-centred risk estimate and less propensity to overtreat. This ability to tinker with the model may also enhance understanding of how it works.
“I wish I could alter criteria and see what the score is after that , with another set of observations. A lot of the time… I wonder what they’re sitting at , now that I’ve [altered] the bit that I’m not concerned about… It would be quite helpful to refresh it and have their score refreshed as the new score.” – Junior doctor.
Derivation of the decision-making model
Guided by the responses of our participants regarding their decision-making processes, our literature search identified a narrative review by Banning (2008) that reported previous work by O’Neill et al. (2005) [ 28 , 29 ]. While these studies referred to models of nurse decision-making, we selected a model (Fig. 2 ) that also appropriately described the responses of doctors in our participant group and matched the context of using clinical decision support systems to support clinical judgement. As an example, when clinicians referenced needing to look for certain data points to give context to a patient assessment, this was mapped to nodes relating to “Current patient data,” “Changes to patient status/data,” and “Hypothesis-driven assessment.”
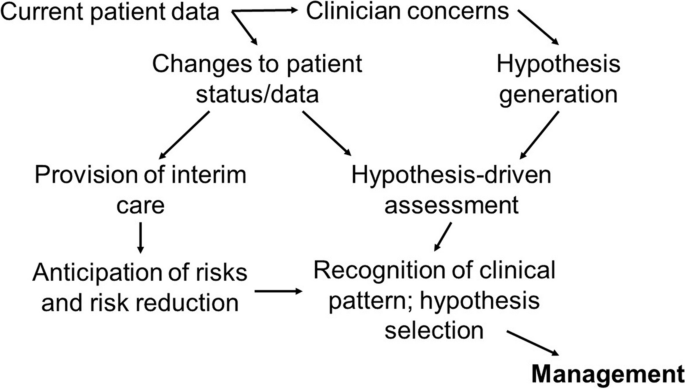
Decision-making model(Adapted from Neill’s clinical decision making framework [2005] and modified by Banning [2006]) with sequential decision nodes
Mapping of themes to decision-making model
The themes from Table 1 were mapped to the nodes in the decision-making model based on close alignment with participant responses (see Fig. 3 ). This mapping is further explained below, where the nodes in the model are described in parentheses.
Value of additional information for decision-making : participants stressed the importance of understanding not only the data going into the prediction model, but also how that data changed over time as trends, and the data that were not included in the model. (Current patient data, changes to patient status/data)
Format, frequency, and relevance of outputs : participants suggested a change in patient data should not always lead to an alert. Doctors, but not necessarily nurses, proposed outputs displayed as probabilities rather than scores, tying model predictions to potential diagnoses or prognoses. (Changes to patient status/data, hypothesis generation)
Using models to validate but not supersede clinical intuition : Depending on the exact timing of model outputs within the pathway of patient assessment, participants found predictions could either augment or hinder the hypothesis generation process. (Hypothesis generation)
Measuring risks objectively : Risk scores can assist with triaging or prioritising patients by urgency or prognostic risk, thereby potentially leading to early intervention to identify and/or prevent adverse events. (Clinician concerns, hypothesis generation)
Supporting critical thinking and reducing over-protocolised care : by acting as triggers for further assessment, participants suggested prediction models can support or discount diagnostic hypotheses, lead to root-cause identification, and facilitate interim cares, for example by ensuring good fit of nasal prongs. (Provision of interim care, hypothesis generation, hypothesis-driven assessment)
Model transparency and interactivity : understanding how prediction models worked, being able to modify or add necessary context to model predictions, and understanding the relative contribution of different predictors could better assist the generation and selection of different hypotheses that may explain a given risk score. (Hypothesis generation, recognition of clinical pattern and hypothesis selection)
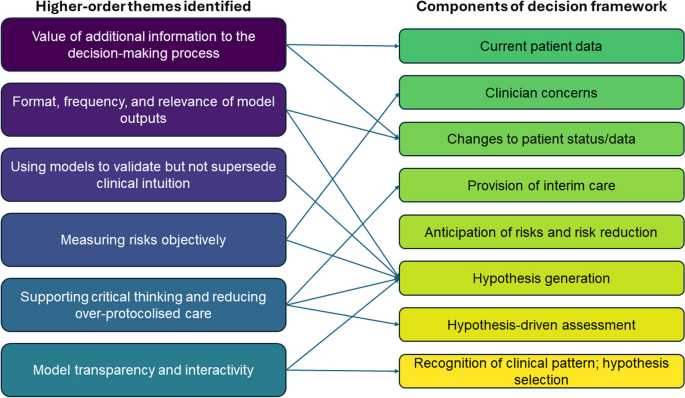
Mapping of the perceived relationships between higher-order themes and nodes in the decision-making model shown in Fig. 2
Recommendations for improving the design of prediction models
Based on the mapping of themes to the decision-making model, we formulated four recommendations for enhancing the development and deployment of prediction models for clinical deterioration.
Improve accessibility and transparency of data included in the model. Provide an interface that allows end-users to see what predictor variables are included in the model, their relative contributions to model outputs, and facilitate easy access to data not included in the model but still relevant for model-informed decisions, e.g., trends of predictor variables over time.
Present model outputs that are relevant to the end-user receiving those outputs, their responsibilities, and the tasks they may be obliged to perform, while preserving the ability of clinicians to apply their own discretionary judgement.
In situations associated with diagnostic uncertainty, avoid tunnel vision from priming clinicians with possible diagnostic explanations based on model outputs, prior to more detailed clinical assessment of the patient.
Support critical thinking whereby clinicians can apply a more holistic view of the patient’s condition, take all relevant contextual factors into account, and be more thoughtful in generating and selecting causal hypotheses.
This qualitative study involving front-line acute care clinicians who respond to early warning score alerts has generated several insights into how clinicians perceive the use of prediction models for clinical deterioration. Clinicians preferred models that facilitated critical thinking, allowed an understanding of the impact of variables included and excluded from the model, provided model outputs specific to the tasks and responsibilities of different disciplines of clinicians, and supported decision-making processes in terms of hypotheses and choice of management, rather than simply responding to alerts in a pre-specified, mandated manner. In particular, preventing prediction models from supplanting critical thinking was repeatedly emphasised.
Reduced staffing ratios, less time spent with patients, greater reliance on more junior workforce, and increasing dependence on automated activation of protocolised management are all pressures that could lead to a decline in clinical reasoning skills. This problem could be exacerbated by adding yet more predictive algorithms and accompanying protocols for other clinical scenarios, which may intensify alert fatigue and disrupt essential clinical care. However, extrapolating our results to areas other than clinical deterioration should be done with caution. An opposing view may be that using prediction models to reduce the burden of routine surveillance may allow redirection of critical thinking skills towards more useful tasks, a question that has not been explored in depth in the clinical informatics literature.
Clinicians expressed interest in models capable of providing causal insights into clinical deterioration. This is neither a function nor capability of most risk prediction models, requiring different assumptions and theoretical frameworks [ 30 ]. Despite this limitation, risk nomograms, visualisations of changes in risk with changes in predictor variables, and other interactive tools for estimating risk may be useful adjuncts for clinical decision-making due to the ease with which input values can be manipulated.
Contributions to the literature
Our research supports and extends the literature on the acceptability of risk prediction models within clinical decision support systems. Common themes in the literature supporting good practices in clinical informatics and which are also reflected in our study include: alert fatigue; the delivery of more relevant contextual information; [ 31 ] the value of patient histories; [ 32 , 33 ] ranking relevant information by clinical importance, including colour-coding; [ 34 , 35 ] not using computerised tools to replace clinical judgement; [ 32 , 36 , 37 ] and understanding the analytic methods underpinning the tool [ 38 ]. One other study has investigated the perspectives of clinicians of relatively simple, rules-based prediction models similar to Q-ADDS. Kappen et al [ 12 ] conducted an impact study of a prediction model for postoperative nausea and vomiting and also found that clinicians frequently made decisions in an intuitive manner that incorporated information both included and absent from prediction models. However, the authors recommended a more directive than assistive approach to model-based recommendations, possibly due to a greater focus on timely prescribing of effective prophylaxis or treatment.
The unique contribution of our study is a better understanding of how clinicians may use prediction models to generate and validate diagnostic hypotheses. The central role of critical thinking and back-and-forth interactions between clinician and model in our results provide a basis for future research using more direct investigative approaches like cognitive task analysis [ 39 ]. Our study has yielded a set of cognitive insights into decision making that can be applied in tandem with statistical best practice in designing, validating and implementing prediction models. [ 19 , 40 , 41 ].
Relevance to machine learning and artificial intelligence prediction models for deterioration
Our results may generalise to prediction models based on machine learning (ML) and artificial intelligence (AI), according to results of several recent studies. Tonekaboni et al [ 42 ] investigated clinician preferences for ML models in the intensive care unit and emergency department using hypothetical scenarios. Several themes appear both in our results and theirs: a need to understand the impact of both included and excluded predictors on model performance; the role of uncertain or noisy data in prediction accuracy; and the influence of trends or patient trajectories in decision making. Their recommendations for more transparent models and the delivery of model outputs designed for the task at hand align closely with ours. The authors’ focus on clinicians’ trust in the model was not echoed by our participants.
Eini-Porat et al [ 43 ] conducted a comprehensive case study of ML models in both adult and paediatric critical care. Their results present several findings supported by our participants despite differences in clinical environments: the value of trends and smaller changes in several vital signs that could cumulatively signal future deterioration; the utility of triage and prioritisation in time-poor settings; and the use of models as triggers for investigating the cause of deterioration.
As ML/AI models proliferate in the clinical deterioration prediction space, [ 44 ] it is important to deeply understand the factors that may influence clinician acceptance of more complex approaches. As a general principle, these methods often strive to input as many variables or transformations of those variables as possible into the model development process to improve predictive accuracy, incorporating dynamic updating to refine model performance. While this functionality may be powerful, highly complex models are not easily explainable, require careful consideration of generalisability, and can prevent clinicians from knowing when a model is producing inaccurate predictions, with potential for patient harm when critical healthcare decisions are being made [ 45 , 46 , 47 ]. Given that our clinicians emphasised the need to understand the model, know which variables are included and excluded, and correctly interpret the format of the output, ML/AI models in the future will need to be transparent in their development and their outputs easily interpretable.
Limitations
The primary limitations of our study were that our sample was drawn from two hospitals with high levels of digital maturity in a metropolitan region of a developed country, with a context specific to clinical deterioration. Our sample of 15 participants may be considered small but is similar to that of other studies with a narrow focus on clinical perspectives [ 42 , 43 ]. All these factors can limit generalisability to other settings or to other prediction models. As described in the methods, we used open-ended interview templates and generated our inductive themes reflexively, which is vulnerable to different types of biases compared to more structured preference elicitation methods with rigidly defined analysis plans. Member checking may have mitigated this bias, but was not possible due to the time required from busy clinical staff.
Our study does not directly deal with methodological issues in prediction model development, [ 41 , 48 ] nor does it provide explicit guidance on how model predictions should be used in clinical practice. Our findings should also not be considered an exhaustive list of concerns clinicians have with prediction models for clinical deterioration, nor may they necessarily apply to highly specialised clinical areas, such as critical care. Our choice of decision making framework was selected because it demonstrated a clear, intuitive causal pathway for model developers to support the clinical decision-making process. However, other, equally valid frameworks may have led to different conclusions, and we encourage more research in this area.
This study elicited clinician perspectives of models designed to predict and manage impending clinical deterioration. Applying these perspectives to a decision-making model, we formulated four recommendations for the design of future prediction models for deteriorating patients: improved transparency and interactivity, tailoring models to the tasks and responsibilities of different end-users, avoiding priming clinicians with diagnostic predictions prior to in-depth clinical review, and finally, facilitating the diagnostic hypothesis generation and assessment process.
Availability of data and materials
Due to privacy concerns and the potential identifiability of participants, interview transcripts are not available. However, interview guides are available in the supplement.
Jenkins DA, Martin GP, Sperrin M, Riley RD, Debray TPA, Collins GS, Peek N. Continual updating and monitoring of clinical prediction models: time for dynamic prediction systems? Diagn Prognostic Res. 2021;5(1):1.
Article Google Scholar
Collins GS, Reitsma JB, Altman DG, Moons KG. Transparent reporting of a multivariable prediction model for individual prognosis or diagnosis (TRIPOD): the TRIPOD statement. BMJ. 2015;350:g7594.
Article PubMed Google Scholar
Moons KG, Altman DG, Reitsma JB, Ioannidis JP, Macaskill P, Steyerberg EW, et al. Transparent reporting of a multivariable prediction model for individual prognosis or diagnosis (TRIPOD): explanation and elaboration. Ann Intern Med. 2015;162(1):W1–73.
Blythe R, Parsons R, White NM, Cook D, McPhail SM. A scoping review of real-time automated clinical deterioration alerts and evidence of impacts on hospitalised patient outcomes. BMJ Qual Saf. 2022;31(10):725–34.
Fahey M, Crayton E, Wolfe C, Douiri A. Clinical prediction models for mortality and functional outcome following ischemic stroke: a systematic review and meta-analysis. PLoS ONE. 2018;13(1):e0185402.
Article PubMed PubMed Central Google Scholar
Fleuren LM, Klausch TLT, Zwager CL, Schoonmade LJ, Guo T, Roggeveen LF, et al. Machine learning for the prediction of sepsis: a systematic review and meta-analysis of diagnostic test accuracy. Intensive Care Med. 2020;46(3):383–400.
White NM, Carter HE, Kularatna S, Borg DN, Brain DC, Tariq A, et al. Evaluating the costs and consequences of computerized clinical decision support systems in hospitals: a scoping review and recommendations for future practice. J Am Med Inform Assoc. 2023;30(6):1205–18.
Sanders S, Doust J, Glasziou P. A systematic review of studies comparing diagnostic clinical prediction rules with clinical judgment. PLoS ONE. 2015;10(6):e0128233.
Abell B, Naicker S, Rodwell D, Donovan T, Tariq A, Baysari M, et al. Identifying barriers and facilitators to successful implementation of computerized clinical decision support systems in hospitals: a NASSS framework-informed scoping review. Implement Sci. 2023;18(1):32.
van der Vegt AH, Campbell V, Mitchell I, Malycha J, Simpson J, Flenady T, et al. Systematic review and longitudinal analysis of implementing Artificial Intelligence to predict clinical deterioration in adult hospitals: what is known and what remains uncertain. J Am Med Inf Assoc. 2024;31(2):509–24.
Davenport T, Kalakota R. The potential for artificial intelligence in healthcare. Future Healthc J. 2019;6(2):94–8.
Kappen TH, van Loon K, Kappen MA, van Wolfswinkel L, Vergouwe Y, van Klei WA, et al. Barriers and facilitators perceived by physicians when using prediction models in practice. J Clin Epidemiol. 2016;70:136–45.
Witteman HO, Dansokho SC, Colquhoun H, Coulter A, Dugas M, Fagerlin A, Giguere AM, Glouberman S, Haslett L, Hoffman A, Ivers N. User-centered design and the development of patient decision aids: protocol for a systematic review. Systematic reviews. 2015;4:1−8.
Zhang J, Norman DA. Representations in distributed cognitive tasks. Cogn Sci. 1994;18(1):87–122.
Johnson CM, Johnson TR, Zhang J. A user-centered framework for redesigning health care interfaces. J Biomed Inf. 2005;38(1):75–87.
Jones D, Mitchell I, Hillman K, Story D. Defining clinical deterioration. Resuscitation. 2013;84(8):1029–34.
Morgan RJ, Wright MM. In defence of early warning scores. Br J Anaesth. 2007;99(5):747–8.
Article CAS PubMed Google Scholar
Smith ME, Chiovaro JC, O’Neil M, Kansagara D, Quinones AR, Freeman M, et al. Early warning system scores for clinical deterioration in hospitalized patients: a systematic review. Annals Am Thorac Soc. 2014;11(9):1454–65.
Baker T, Gerdin M. The clinical usefulness of prognostic prediction models in critical illness. Eur J Intern Med. 2017;45:37–40.
Campbell V, Conway R, Carey K, Tran K, Visser A, Gifford S, et al. Predicting clinical deterioration with Q-ADDS compared to NEWS, between the flags, and eCART track and trigger tools. Resuscitation. 2020;153:28–34.
The Australian Commission on Safety and Quality in Health is the publisher, and the publisher location is Sydney, Australia. https://www.safetyandquality.gov.au/sites/default/files/migrated/35981-ChartDevelopment.pdf .
Vasileiou K, Barnett J, Thorpe S, Young T. Characterising and justifying sample size sufficiency in interview-based studies: systematic analysis of qualitative health research over a 15-year period. BMC Med Res Methodol. 2018;18(1):148.
Hennink MM, Kaiser BN, Marconi VC. Code saturation versus meaning saturation: how many interviews are Enough? Qual Health Res. 2017;27(4):591–608.
Gale NK, Heath G, Cameron E, Rashid S, Redwood S. Using the framework method for the analysis of qualitative data in multi-disciplinary health research. BMC Med Res Methodol. 2013;13(1):1–8.
Greenhalgh T, Wherton J, Papoutsi C, Lynch J, Hughes G, A’Court C, et al. Beyond adoption: a New Framework for Theorizing and evaluating nonadoption, abandonment, and challenges to the Scale-Up, Spread, and sustainability of Health and Care technologies. J Med Internet Res. 2017;19(11):e367.
Braun V, Clarke V. One size fits all? What counts as quality practice in (reflexive) thematic analysis? Qualitative Res Psychol. 2021;18(3):328–52.
Campbell KA, Orr E, Durepos P, Nguyen L, Li L, Whitmore C, et al. Reflexive thematic analysis for applied qualitative health research. Qualitative Rep. 2021;26(6):2011–28.
Google Scholar
Banning M. A review of clinical decision making: models and current research. J Clin Nurs. 2008;17(2):187–95.
O’Neill ES, Dluhy NM, Chin E. Modelling novice clinical reasoning for a computerized decision support system. J Adv Nurs. 2005;49(1):68–77.
Arnold KF, Davies V, de Kamps M, Tennant PWG, Mbotwa J, Gilthorpe MS. Reflection on modern methods: generalized linear models for prognosis and intervention—theory, practice and implications for machine learning. Int J Epidemiol. 2020;49(6):2074–82.
Article PubMed Central Google Scholar
Westerbeek L, Ploegmakers KJ, de Bruijn GJ, Linn AJ, van Weert JCM, Daams JG, et al. Barriers and facilitators influencing medication-related CDSS acceptance according to clinicians: a systematic review. Int J Med Informatics. 2021;152:104506.
Henshall C, Marzano L, Smith K, Attenburrow MJ, Puntis S, Zlodre J, et al. A web-based clinical decision tool to support treatment decision-making in psychiatry: a pilot focus group study with clinicians, patients and carers. BMC Psychiatry. 2017;17(1):265.
Weingart SN, Simchowitz B, Shiman L, Brouillard D, Cyrulik A, Davis RB, et al. Clinicians’ assessments of electronic medication safety alerts in ambulatory care. Arch Intern Med. 2009;169(17):1627–32.
Baysari MT, Zheng WY, Van Dort B, Reid-Anderson H, Gronski M, Kenny E. A late attempt to involve end users in the design of medication-related alerts: Survey Study. J Med Internet Res. 2020;22(3):e14855.
Trafton J, Martins S, Michel M, Lewis E, Wang D, Combs A, et al. Evaluation of the acceptability and usability of a decision support system to encourage safe and effective use of opioid therapy for chronic, noncancer pain by primary care providers. Pain Med. 2010;11(4):575–85.
Wipfli R, Betrancourt M, Guardia A, Lovis C. A qualitative analysis of prescription activity and alert usage in a computerized physician order entry system. Stud Health Technol Inform. 2011;169:940–4.
PubMed Google Scholar
Cornu P, Steurbaut S, De Beukeleer M, Putman K, van de Velde R, Dupont AG. Physician’s expectations regarding prescribing clinical decision support systems in a Belgian hospital. Acta Clin Belg. 2014;69(3):157–64.
Ahearn MD, Kerr SJ. General practitioners’ perceptions of the pharmaceutical decision-support tools in their prescribing software. Med J Australia. 2003;179(1):34–7.
Swaby L, Shu P, Hind D, Sutherland K. The use of cognitive task analysis in clinical and health services research - a systematic review. Pilot Feasibility Stud. 2022;8(1):57.
Steyerberg EW. Applications of prediction models. In: Steyerberg EW, editor. Clinical prediction models. New York: Springer-; 2009. pp. 11–31.
Chapter Google Scholar
Steyerberg EW, Vergouwe Y. Towards better clinical prediction models: seven steps for development and an ABCD for validation. Eur Heart J. 2014;35(29):1925–31.
Tonekaboni S, Joshi S, McCradden MD, Goldenberg A. What Clinicians Want: Contextualizing Explainable Machine Learning for Clinical End Use. In: Doshi-Velez F, Fackler J, Jung K, Kale D, Ranganath R, Wallace B, Wiens J, editors. Proceedings of the 4th Machine Learning for Healthcare Conference; Proceedings of Machine Learning Research: PMLR; 2019;106:359–80.
Eini-Porat B, Amir O, Eytan D, Shalit U. Tell me something interesting: clinical utility of machine learning prediction models in the ICU. J Biomed Inform. 2022;132:104107.
Muralitharan S, Nelson W, Di S, McGillion M, Devereaux PJ, Barr NG, Petch J. Machine learning-based early warning systems for clinical deterioration: systematic scoping review. J Med Internet Res. 2021;23(2):e25187.
Rudin C. Stop Explaining Black Box Machine Learning Models for high stakes decisions and use interpretable models instead. Nat Mach Intell. 2019;1(5):206–15.
Blythe R, Parsons R, Barnett AG, McPhail SM, White NM. Vital signs-based deterioration prediction model assumptions can lead to losses in prediction performance. J Clin Epidemiol. 2023;159:106–15.
Futoma J, Simons M, Panch T, Doshi-Velez F, Celi LA. The myth of generalisability in clinical research and machine learning in health care. Lancet Digit Health. 2020;2(9):e489–92.
Steyerberg EW, Uno H, Ioannidis JPA, van Calster B, Collaborators. Poor performance of clinical prediction models: the harm of commonly applied methods. J Clin Epidemiol. 2018;98:133–43.
Download references
Acknowledgements
We would like to thank the participants who made time in their busy clinical schedules to speak to us and offer their support in recruitment.
This work was supported by the Digital Health Cooperative Research Centre (“DHCRC”). DHCRC is funded under the Commonwealth’s Cooperative Research Centres (CRC) Program. SMM was supported by an NHMRC-administered fellowships (#1181138).
Author information
Authors and affiliations.
Australian Centre for Health Services Innovation and Centre for Healthcare Transformation, School of Public Health and Social Work, Faculty of Health, Queensland University of Technology, 60 Musk Ave, Kelvin Grove, Brisbane, QLD, 4059, Australia
Robin Blythe, Sundresan Naicker, Nicole White & Steven M McPhail
Princess Alexandra Hospital, Metro South Health, Woolloongabba, QLD, Australia
Raelene Donovan & Andrew McKelliget
Queensland Digital Health Centre, Faculty of Medicine, University of Queensland, Brisbane, QLD, Australia
Ian A. Scott
Digital Health and Informatics Directorate, Metro South Health, Woolloongabba, QLD, Australia
Ian A. Scott & Steven M McPhail
You can also search for this author in PubMed Google Scholar
Contributions
RB: conceptualisation, data acquisition, analysis, interpretation, writing. SN: data acquisition, analysis, interpretation, writing. NW: interpretation, writing. RD: data acquisition, interpretation, writing. IS: data acquisition, analysis, interpretation, writing. AM: data acquisition, interpretation, writing. SM: conceptualisation, data acquisition, analysis, interpretation, writing. All authors have approved the submitted version and agree to be accountable for the integrity and accuracy of the work.
Corresponding author
Correspondence to Robin Blythe .
Ethics declarations
Ethics approval and consent to participate.
This study was approved by the Metro South Human Research Ethics Committee (HREC/2022/QMS/84205). Informed consent was obtained prior to interview scheduling, with all participants filling out a participant information and consent form. Consent forms were approved by the ethics committee. Participation was entirely voluntary, and could be withdrawn at any time. All responses were explicitly deemed confidential, with only the first two study authors and the participant privy to the research data. Interviews were then conducted in accordance with Metro South Health and Queensland University of Technology qualitative research regulations. For further information, please contact the corresponding author.
Consent for publication
Not applicable.
Competing interests
The authors declare no competing interests.
Additional information
Publisher’s note.
Springer Nature remains neutral with regard to jurisdictional claims in published maps and institutional affiliations.
Supplementary Information
Supplementary material 1., rights and permissions.
Open Access This article is licensed under a Creative Commons Attribution 4.0 International License, which permits use, sharing, adaptation, distribution and reproduction in any medium or format, as long as you give appropriate credit to the original author(s) and the source, provide a link to the Creative Commons licence, and indicate if changes were made. The images or other third party material in this article are included in the article's Creative Commons licence, unless indicated otherwise in a credit line to the material. If material is not included in the article's Creative Commons licence and your intended use is not permitted by statutory regulation or exceeds the permitted use, you will need to obtain permission directly from the copyright holder. To view a copy of this licence, visit http://creativecommons.org/licenses/by/4.0/ .
Reprints and permissions
About this article
Cite this article.
Blythe, R., Naicker, S., White, N. et al. Clinician perspectives and recommendations regarding design of clinical prediction models for deteriorating patients in acute care. BMC Med Inform Decis Mak 24 , 241 (2024). https://doi.org/10.1186/s12911-024-02647-4
Download citation
Received : 06 September 2023
Accepted : 23 August 2024
Published : 02 September 2024
DOI : https://doi.org/10.1186/s12911-024-02647-4
Share this article
Anyone you share the following link with will be able to read this content:
Sorry, a shareable link is not currently available for this article.
Provided by the Springer Nature SharedIt content-sharing initiative
- Clinical prediction models
- Clinical decision support systems
- Early warning score
- Clinical deterioration
- Clinical decision-making
BMC Medical Informatics and Decision Making
ISSN: 1472-6947
- General enquiries: [email protected]

An official website of the United States government
The .gov means it’s official. Federal government websites often end in .gov or .mil. Before sharing sensitive information, make sure you’re on a federal government site.
The site is secure. The https:// ensures that you are connecting to the official website and that any information you provide is encrypted and transmitted securely.
- Publications
- Account settings
Preview improvements coming to the PMC website in October 2024. Learn More or Try it out now .
- Advanced Search
- Journal List

Clinical Reasoning: A Missing Piece for Improving Evidence-Based Assessment in Psychology
Associated data.
Not applicable.
Clinical reasoning is a foundational component of conducting evidence-based psychological assessments. In spite of its importance, limited attention has been paid to the teaching or measurement of clinical reasoning skills relative to psychological assessment, as well as how clinical reasoning develops or how its efficacy can be measured. Improving clinical reasoning throughout the assessment process, from initial case conceptualization to hypotheses testing, to recommendation writing, has the potential to address commonly noted concerns regarding diagnostic accuracy, as well as the accessibility and utility of psychological reports and recommendations, and will, ultimately, lead to improved outcomes for clients. Consequently, we provide a definition of clinical reasoning in relation to psychological assessment, followed by a critique of graduate training assessment and the current challenges of measuring clinical reasoning in psychology. Lastly, this paper provides suggestions for how to incorporate clinical reasoning throughout the assessment process as a way to answer client questions more effectively and provide meaningful recommendations to improve outcomes.
1. Introduction
Evidence-based assessment (EBA) is a relatively new concept in psychology that emphasizes the theory and research in selecting and using high-quality assessment methods and processes ( Youngstrom and Van Meter 2016 ). Although there are no agreed-upon standards for its application in psychology, there have been some attempts at providing guidelines for EBA, based on the American Psychological Association’s ( American Psychological Association 2006 ) three recommendations for evidence-based psychological practice, including: (a) using the best available research, (b) applying clinical expertise, and (c) attending to patient characteristics, culture, and preferences ( Bornstein 2017 ). Others have noted that EBA requires effective critical thinking and reasoning, which informs all aspects of assessment, from determining the questions and choosing assessment measures to interpreting the results by analyzing information and data within the context of a client ( Dombrowski et al. 2021 ; Victor-Chmil 2013 ; Ward 2019 ). Thus, clinical reasoning supports clinicians who must engage in clinical reasoning during assessment and make diagnostic decisions when presenting client problems in EBA.
The purpose of this paper is to describe the current state of clinical reasoning research in the context of psychological assessment and to propose potential directions for promoting clinical reasoning in assessment practice. This paper will first define the role of clinical reasoning in evidence-based assessment and the research related to this area, outlining some of the contemporary challenges in the training and research related to clinical reasoning in assessment. The second section will summarize the current, albeit limited, literature on how psychologists develop clinical reasoning skills, along with recommendations for extending the research findings on deliberate practice (DP). Finally, this paper will suggest how practitioners might be able to improve their clinical reasoning in assessment contexts, based on the findings of medicine and psychotherapy.
2. The Role of Clinical Reasoning in Evidence-Based ()Assessment
Victor-Chmil ( 2013 ) posited that “ critical thinking is the cognitive processes used for analyzing knowledge” (p. 34) and also that “ clinical reasoning is the cognitive and metacognitive processes for analyzing knowledge relative to a clinical situation or specific patient” ( Victor-Chmil 2013, p. 34 ). Often used interchangeably with other terms such as critical reasoning, clinical reasoning allows psychologists to make sense of a large amount of data as they develop working hypotheses, identify information that supports or refutes those hypotheses, and compare data to diagnostic criteria. Both critical reasoning and clinical reasoning involve intentionally thinking about a problem, testing hypotheses, and generating solutions to the problem ( American Psychological Association n.d. ; Gruppen 2017 ). Critical thinking requires not only attending to the outcome of the process but also attending to the process of thinking, which is often omitted in research on assessment ( Gambrill 2019 ). Because clinical reasoning and critical reasoning are notoriously poorly or inconsistently defined within the literature, and because there is considerable overlap between these two terms, they are considered similar enough that we have used clinical reasoning in this paper, due to its more common use within the broader research literature.
In order to move toward EBA and utilize clinical expertise in this process, it is important to understand the current challenges of implementing EBA ( Ward 2019 ). One challenge is in understanding how clinicians gain and apply the foundational skill of clinical reasoning in psychological assessment ( Dombrowski et al. 2021 ). Reasoning is an under-discussed topic in EBA ( Wright et al. 2022 ) that is used when testing hypotheses related to clients’ functioning within their context, synthesizing and integrating data from multiple sources, and providing diagnoses and meaningful treatment recommendations to improve functioning ( Mash and Hunsley 2005 ; Wright et al. 2022 ; Youngstrom et al. 2015 ; Youngstrom and Van Meter 2016 ). When performed well, clinical reasoning aids psychologists in asking important questions to ensure that consideration is given to how psychologists’ beliefs about clients or their problems influence the assessment process.
Unfortunately, faulty clinical reasoning can lead to misdiagnoses and may harm clients through delayed, insufficient, or inappropriate treatment, which ultimately leads to a lack of faith in psychological services ( Gambrill 2012 ; Wright 2021 ). Currently, there are no available statistics on how faulty clinical reasoning affects the general population because of the difficulty in directly connecting error rates in psychology to negative outcomes ( Gambrill 2012 ). This contrasts with the medical field, where there are considerably more publications on this topic owing to the availability within the medical field of more objective measures of error rates, such as mortality and the length of hospital stays (e.g., Ahmed et al. 2015 ). Specific to psychology, the link between poor critical reasoning and negative client outcomes is largely indirect and has primarily been examined in relation to the common types and sources of errors in both the testing and report-writing processes, largely ignoring the role of critical reasoning in these problems. Because of the important role that psychological assessment can play in improving client functioning, understanding how psychologists think and reason critically throughout the process of assessment and case conceptualization is vital for improving the quality of assessments ( Siegert 1999 ). Additionally, while there have been significant advances in evidence-based treatments, the lack of corresponding attention to EBA is surprising, as treatment selection should be informed by assessment ( Mash and Hunsley 2005 ).
The pursuit of clinical reasoning in assessment is an important goal. The conclusions and diagnostic decisions derived from psychoeducational assessment can have a significant effect on the daily lives of clients. For instance, an understanding of the ecological factors that either support or restrict the success of a student with academic difficulties is critical in determining whether or not the student meets the diagnostic criteria for a learning disability, and identifying the appropriate remediation, learning support at home and school, and accommodations that are specific to that pupil’s educational needs.
3. Examining the Current State of Research Training, Research, and Practice
As Gordon et al. ( 2022 ) aptly remarked, “Clinical reasoning is a topic that often feels familiar (or even obvious) … [however,] this sense of familiarity may be masking important differences in how it is understood, operationalized, and assessed” (p. 109). Indeed, how psychologists engage in clinical reasoning during assessment has largely been neglected in the literature ( Mash and Hunsley 2005 ). In discussing the current state of clinical reasoning in psychology, we have drawn upon research into the technical aspects of test administration ( Oak et al. 2019 ), the use of base rates ( Burns 1990 ), diagnostic accuracy in assessment ( Aspel et al. 1998 ; de Mesquita 1992 ; Watkins 2009 ), and improving report writing ( Nelson 2021 ; Pelco et al. 2009 ; Postal et al. 2018 ), but this body of literature had not addressed how to develop and improve critical reasoning in psychological assessment. Much of the argument of this paper is based on the research of clinical reasoning skills in social work ( Gambrill 2012 , 2019 ), medicine ( Young et al. 2020 ), and psychological therapy ( Miller et al. 2020 ) because clinical reasoning and how to develop and improve clinical reasoning in psychological assessment has largely been ignored. Below, we review what is known about clinical reasoning from the literature, highlighting issues with how it is taught, researched, and, currently, practiced.
3.1. A Focus on Testing Rather than Assessment
One of the challenges in understanding the role of clinical reasoning in assessment has been the commonplace conflation of the terms, “testing” and “assessment”. In training assessment skills, an emphasis on standardized assessment and reducing administrative error in training programs is warranted, as standardized administration requires considerable training, and critical thinking is predicated on quality data. However, paying attention to testing, including choosing appropriate measures with strong psychometric properties and interpreting test scores appropriately, is imperative but it is insufficient to ensure strong clinical reasoning. Testing generally refers to choosing and administrating measures and assessment alignments. Assessment, however, refers to the entire process, from choosing what questions to ask during the initial interview to interpreting all of the data gathered, including but not limited to test scores ( Canivez 2019 ; Suhr 2015 ; Wright 2021 ); the initial steps inform the subsequent hypotheses and guide the assessment process, but they occur prior to test selection, administration, and interpretation ( Ward 2019 ). One problem with most evaluations of assessment skills in training is that there is an emphasis on evaluating the psychometric aspects of assessment and standardized test administration, at the expense of clinical reasoning development ( Mash and Hunsley 2005 ; Wright 2021 ). There is a danger in focusing on the generation of test scores at the expense of clinical reasoning. Psychologists can use psychometrically strong measures and administer them appropriately but will come to poor conclusions if they do not have the clinical reasoning skills to determine what the problem is that is being presented, in order to ask and answer the right questions or to integrate and interpret the resulting data effectively ( Mash and Hunsley 2005 ).
During the psychological assessment process, test scores are an important source of information. Learning the standardized test measures is a complex and time-consuming task that represents an important foundational skill for reducing error and increasing reliability. Error is inherent in testing for various reasons such as client and examiner factors, as well as problematic testing conditions, including incomplete data, time pressures, and complex environments; therefore, it is important to reduce administrative error as much as possible. Unfortunately, despite the focus on a standardized assessment, errors are common. For example, despite the fact that these are learned skills that are a core part of training programs, assessment errors are commonplace, with practitioners often making more errors than students ( Oak et al. 2019 ). This level of difficulty in accurately implementing skills that are essential for assessment contributes to poor clinical reasoning by providing poor-quality data.
3.2. Test-by-Test Reporting
The concern that emphasizing test scores over assessment can lead to weak clinical reasoning is demonstrated by the dominant test-by-test approach used in report writing, which some argue reflects the quality of clinical reasoning ( Pelco et al. 2009 ). It is important that reports are transparent when explaining how the psychologist arrived at their diagnostic conclusions, along with how the assessment process informed the diagnostic decision and recommendations, but test-by-test reports do not make psychologists’ reasoning transparent ( Pelco et al. 2009 ; Wilcox and Schroeder 2015 ). Weak clinical reasoning can contribute to unclear reports that do not support the clients. In this regard, errors in both the assessment and report-writing processes provide indirect evidence of the association between poor clinical reasoning and negative client outcomes.
Along these lines, Wright ( 2021 ) has cogently described the current state of clinical reasoning in assessment: “Psychological assessment has long been a mysterious, intuited process, taught to psychologists in training, test by test, with components of conceptualization, integration, and report writing somewhat tacked onto the end of the process” (p. 3). The test-by-test report style remains the most common technique used by psychologists ( Pelco et al. 2009 ), despite being cited as problematic in the literature ( Postal et al. 2018 ). Test-by-test reports can be a symptom of weak clinical reasoning because psychologists do not integrate other sources of information (e.g., observational data, background information) with the test scores in a meaningful way that will tell a story as to why the clients are struggling, along with the strengths that support them. Meyer et al. ( 2001 ) provided a clear explanation of the role of tests within an assessment, stating that “[T]ests do not think for themselves, nor do they directly communicate with patients. As in the case of a stethoscope, a blood pressure gauge, or an MRI scan, a psychological test is a dumb tool, and the worth of the tool cannot be separated from the sophistication of the clinician who draws inferences from it and then communicates with patients and professionals” (p. 153).
Clinical reasoning is more than interpreting test scores. Test scores should be connected to other information, including how clients attained their scores, error analysis, observation, and reports from selves and others. These additional data support a clear argument for how the conclusions were made. Assessment should also integrate client characteristics and functioning and the contextual aspects of the client’s strengths and challenges, in order to inform interventions ( Wright et al. 2022 ). Unfortunately, when information is segmented into individual sections, and test scores are reported in isolation, it is unclear to the reader why the client is experiencing difficulties, making it difficult to generate useful recommendations ( Wright 2021 ).
The magnitude of this issue is highlighted in Dailor and Jacob ’s ( 2011 ) survey of 208 school psychologists. Of the respondents, 37% read a report within the past year that listed the student’s test scores with no accompanying interpretation; 34% read reports that made recommendations that were unsubstantiated by the data, and 26% read computer-generated reports. Such reports are not useful to readers who depend on them to support clients through follow-up intervention. Limiting the reporting of findings to a list of strengths and weaknesses in the form of test scores reduces the role of the psychologist to that of a psychometrist ( Wright et al. 2022 ). Instead, EBA should utilize an iterative hypothesis-testing and decision-making process that requires well-developed clinical reasoning skills ( Suhr 2015 ; Wright et al. 2022 ).
4. How Do Psychologists Gain Clinical Reasoning Skills?
As a primarily invisible process, identifying how clinical reasoning skills develop through training and experience has been a challenge for both researchers and trainers. This might be the reason why programs spend more time assessing trainee proficiency in test administration than time assessing their broader assessment skills. In addition, there seems to be uncertainty about how or when trainees should learn clinical reasoning skills. Even though clinical reasoning is universally viewed as an important competence outcome by training programs ( Harding 2007 ), programs do not necessarily have a systematic approach to instruction. For instance, there is disagreement as to whether this should be taught in coursework or if it should be acquired through applied experiences such as practica and internship placements. The majority of clinics, schools, and neuropsychologists include assessment in their practice ( Arocha and Patel 1995 ), yet a survey of clinical psychology programs found that less than half of the programs indicated that they teach strategies to improve decision-making and clinical judgment ( Harding 2007 ). This is concerning because it is unlikely that clinical reasoning develops independently, without specific training ( Harding 2007 ). Although the dominant view was once that students acquire these skills unconsciously via clinical experience ( Wright 2021 ), there is growing recognition of the need to explicitly instruct and help trainees to develop accurate clinical reasoning.
Pre-doctoral internships also constitute an opportune period for developing clinical reasoning skills; pre-doctoral internships are generally a time to help students address areas of weakness, in order for them to enter the field with beginning levels of competence. Unfortunately, only 40% of APPIC internship sites offered intensive assessment training for interns ( Krishnamurthy et al. 2004 ). Harding ( 2007 ) noted that this lack of training leads to significant concerns about practitioners’ clinical reasoning because, without instruction in this area, psychologists are not likely to realize that they need to improve their clinical reasoning, and consequently, do not actively work to improve their clinical reasoning as they gain more experience. This poses a significant obstacle to psychologists’ ability to provide EBA ( Cook et al. 2017 ). As suggested by Gambrill ( 2012 ), clinicians are often unaware of the skills that they are lacking without specific feedback. Consequently, the current research suggests that psychologists do not generally receive enough training in clinical reasoning for assessment during their tenure in graduate programs to gain competence in this area.
4.1. Gaining and Measuring Clinical Reasoning
One of the issues with how clinical reasoning in assessment is taught (or not taught), is the limited understanding of what differentiates novices from experts and how much experience or what types of experiences are needed for someone to reach an “expert” level of practice. Researchers have struggled to effectively measure how reasoning develops from novice to expert. There has been an assumption that greater experience results in better clinical reasoning. Practitioners who have more experience should make fewer errors in reasoning and be able to identify what information is important and what legitimately contributes to the overall diagnostic picture. To examine this assumption, some researchers have focused on comparing the differences between experts and novices regarding diagnostic accuracy and reasoning processes.
4.2. Diagnostic Accuracy
In comparing the rates of diagnostic accuracy between less experienced clinicians and more experienced clinicians, the underlying assumption is that if the diagnosis is accurate, the clinical reasoning that proceeded it should be accurate as well. However, evaluating the accuracy of diagnostic decisions provides no information about how clinicians arrive at their conclusions ( Siegert 1999 ). A focus on diagnostic accuracy is similar to an “outcome bias,” which values outcomes over the quality of the process ( Gambrill 2012 , 2019 ). It relegates clinical reasoning to a “black box” where testing information enters and diagnostic conclusions exit, but the transformation process (e.g., clinical reasoning) is a mystery ( Siegert 1999 ; Wright 2021 ).
Similar to the issues discussed earlier with the test-by-test report-writing style, this emphasis on outcome suggests a process that is directed by test scores, which results in minimizing or neglecting the role of the psychologist in taking responsibility for critically interpreting all of the data, not merely the test scores ( Siegert 1999 ). The narrow focus on diagnostic accuracy fails to identify key differences and issues with the questions that psychologists choose to answer, the tools that they use, and the critical reasoning required to make those decisions and integrate and interpret that information to describe client functioning and to make relevant recommendations.
4.3. The Role of Expertise in Clinical Reasoning
Without understanding the clinical reasoning required throughout the assessment process, it is difficult to identify which reasoning practices need to be targeted in training to improve diagnostic accuracy ( Siegert 1999 ). In response, a small body of psychology research has studied the quality of clinical reasoning by examining the reasoning processes of practitioners. As with diagnostic accuracy, much of the literature has compared the processes of less experienced with more experienced practitioners. Within the broader literature, there are mixed findings regarding the effect of experience on the process of clinical reasoning.
A study of therapists found that expert therapists specializing in cognitive-behavioral and psychodynamic approaches generated more comprehensive and complex case conceptualizations than did both experienced therapists and trainees ( Eells et al. 2005 ). A study by Arocha and Patel ( 1995 ) found that when trainees received contradictory information during case conceptualization, they were unsure how to manage it. Rather than adjusting their hypotheses, they tended to either ignore contradictory findings or interpret those findings to fit their initial hypothesis, rather than adjusting their hypothesis ( Arocha and Patel 1995 ). Trainees also rigidly adhered to rules, paying little attention to contextual factors and, consequently, lacked discretionary judgment ( Del Mar et al. 2006 ). Competent psychologists also demonstrated more skill in coping with pressures, having a broader conceptual framework for their planning, and following general standardized procedures.
The relatively sparse corpus of research focused specifically on psychoeducational assessment suggests that experience leads to limited improvements in clinical reasoning ( de Mesquita 1992 ). For example, a study by Aspel et al. ( 1998 ) used a case-based approach to examine the process of clinical reasoning during psychoeducational assessment. Less and more experienced practitioners used similar approaches to the cases and did not change their working hypotheses after reviewing four to five categories of information. In another study, de Mesquita ( 1992 ) found experienced school psychologists, with varying levels of education, who considered similar types and amounts of information and came to similar conclusions as less experienced school psychologists. These two studies highlight the fact that experience does not automatically result in expertise. Education and experience were generally unrelated to diagnostic accuracy, and there was little difference among groups in terms of the amount and type of information reviewed and the number of diagnoses made.
However, when de Mesquita ( 1992 ) evaluated the process of clinical reasoning undertaken by practitioners, there were differences between less and more experienced practitioners. Practitioners with more experience required less time to reach an accurate diagnostic decision than did students. More experienced psychologists also generated fewer hypotheses and favored one hypothesis based on previous case experience. de Mesquita proposed that experience alone was not beneficial; instead, it was how well that knowledge was conceptually organized that led to accuracy and efficient reasoning.
Although experience seems to benefit psychologists in some ways, it is unclear how much experience is needed for someone to reach an expert level of practice, or if most practitioners even reach that level. Experience can support improvement, but it does not automatically lead to expertise. In medicine, Haynes et al. ( 2002 ) noted that expertise is not equivalent to experience. Expertise should be judged on one’s knowledge of both the quality of the evidence and skill in interpreting that evidence, considering specific patient circumstances ( Haynes et al. 2002 ). Tracey et al. ( 2014 ) found that practitioners gained confidence in their abilities along with experience, but their level of confidence did not match their performance. In fact, after gaining initial skills, confidence increased much more rapidly than accuracy, so the practitioners believed that they were more accurate than they actually were ( Sanchez and Dunning 2018 ). Furthermore, confidence reduced their motivation to reflect on their skills, identify areas of weakness, and actively work to improve them ( Tracey et al. 2014 ). Without awareness of their limitations, clinicians were likely to continue to make the same mistakes after ten years of practice that they made in their first year because there was no opportunity for self-correction ( Harding 2007 ; Watkins 2009 ). This highlights the importance of separating experience and expertise in understanding the role of clinical reasoning in EBA.
In summary, there is still much uncertainty about how experience and training influence the development of clinical reasoning as trainees move from graduate school to independent practice. The current literature suggests that the profession of psychology has approached clinical reasoning development in an ad hoc way. Relying on practical experiences (i.e., practica) for clinical reasoning development without intentional instruction or opportunities for feedback and reflection has the potential for ineffectual habits to become established, overconfidence to develop in practitioners, and little or no growth over time.
5. Moving Clinical Reasoning Skills from Novice to Expert
Research demonstrates that gaining expertise requires an intentional effort in learning and applying the component skills ( Chow et al. 2015 ; Ericsson 2018 ; Miller et al. 2020 ) rather than acquiring clinical reasoning skills through supervised practice and then continued independent practice, which appears to the primary vehicle for learning clinical reasoning skills in psychology ( Gross et al. 2019 ; Harding 2007 ; Krishnamurthy et al. 2004 ). Consequently, these findings suggest that to gain expertise in clinical reasoning, students require direct instruction and DP rather than simply additional experience. Unfortunately, there is currently no reliable model of assessment for clinical reasoning skills, which makes it difficult to determine where students or psychologists need to improve or how to help them to improve ( Miller et al. 2020 ). As a result, the arguments presented in this section are largely based on research from other areas, and additional research is needed to identify how best these findings might apply to psychoeducational assessment.
Deliberate Practice
A body of research has examined the benefits of DP on expertise development in a variety of fields, including sports, performing arts, and chess ( Ericsson 2018 ). DP requires clearly defining the individual components of the skill to be learned, immediate feedback in performing the skills, repeated practice of the skills, often in solitary settings, and using information from errors to improve performance ( Ericsson 2006 ). In psychology, the outcomes of using DP in assessment have not yet been studied, although it has been successfully applied to psychotherapy practice. The amount of time that psychologists engaged in solitary DP (e.g., reviewing challenging cases, reviewing therapy recordings, writing down reflections and goals) predicted positive client outcomes during psychotherapy ( Chow et al. 2015 ; Clements-Hickman and Reese 2020 ). It was more influential than other psychologist demographic variables, including experience, education, race, gender, and theoretical orientation. It is important to note that in DP, solitary practice is informed by feedback and coaching ( Ericsson 2018 ; McLeod 2021 ; Miller et al. 2020 ). This was the only psychologist activity that predicted client outcomes and demonstrates both the importance of DP and the difference between experience and expertise.
The main components of DP are “(a) individualized learning objectives, (b) use of a coach, (c) feedback, and (d) successive refinement through repetition” ( Miller et al. 2020, p. 39 ). Goal quality is related to performance levels, wherein the weakest performers do not generally engage in goal setting; average performers create goals focused on the desired outcome without setting smaller proximal goals; the highest performers set goals that break down the larger goal into steps that they will take to achieve the final outcome ( Ericsson 2018 ). The research on implementing DP in therapy uses coaching with feedback because coaches are able to see aspects of performance that are often not evident to the psychologist. Beyond the typical requirements of feedback, such as specificity and timeliness, the feedback should focus on improving specific skills rather than on the final product, refining parts of the clinical reasoning process one step at a time, which leads to better performance in the long run ( Miller et al. 2020 ). One challenge with this process, especially for practicing psychologists, is that implementing changes will result in some failures due to the learning process. This requires a willingness to experience short-term failure in order to improve over the long term ( Miller et al. 2020 ). Instead of focusing solely on how to assess, DP would direct attention to developing the psychologists’ clinical reasoning ( Miller et al. 2020 ). This process of DP has not yet been applied to assessment, but its success in therapy suggests that it is worth exploring this process in the context of assessment.
As with other practices, DP requires intentionality. Miller et al. ( 2020 ) offer suggestions for incorporating DP, including scheduling time for it, and protecting it by removing other distractions (e.g., emails or booking another meeting during that time). Taking time every week to jot down notes about what was learned through clinical practice, including successes as well as mistakes that were made and what contributed to them, is one example of an intention DP. Research is needed to determine how to effectively incorporate DP into clinical reasoning during assessment because it is an environment providing limited feedback ( Lillienfeld and Basterfield 2020 ; Tracey et al. 2014 ). One strategy to improve the awareness of accuracy is to record and monitor one’s diagnostic accuracy and utility over time ( Kleinmuntz 1990 ); unfortunately, psychologists rarely receive this type of feedback from their psychological assessments ( Mash and Hunsley 2005 ), and there is generally a low to moderate level of diagnostic agreement between clinicians ( Rettew et al. 2009 ), making it exceedingly difficult for them to implement this strategy. More work is needed to find effective ways for psychologists to elicit feedback that they can use to inform their evaluations of their assessment practices.
One study found that explicitly teaching medical students how to engage in DP increased their planning and the structure of their work, as well as their performance on clinical exams ( Duvivier et al. 2011 ). However, instruction was only as effective as the student’s engagement with the process and required training in the self-assessment of weaknesses. Not surprisingly, students who were more accurate in their self-assessments performed better than students who were less accurate in their self-assessments ( Duvivier et al. 2011 ).
6. Recommendations for Improving Clinical Reasoning
The first recommendation for improving clinical reasoning is to seek feedback throughout the assessment process and after the assessment is over. The nature of brief assessment relationships requires that psychologists intentionally and effortfully seek out this feedback ( Siegert 1999 ). As noted in the work on DP in therapy, it is necessary to seek out negative feedback in order to identify areas of growth, which is necessary to improve practice ( Miller et al. 2020 ). Mental health professionals often fail to acknowledge the uncertainty inherent in the assessment process ( Gambrill 2012 ). Uncertainty throughout the process is inevitable because psychologists work under time constraints, using information of varying quality and completeness, but the negative impact of uncertainty is greater when psychologists fail to acknowledge that it exists ( Gambrill 2012 ). As a result, professionals often overestimate their effectiveness, and those who are the most experienced are both the most confident and the least likely to be attentive to learning from their mistakes ( Miller et al. 2020 ). In fact, overconfidence is one of the cognitive biases garnering the most research, making it an important area for psychologists to consider in their practice ( Kahneman et al. 2021 ).
6.1. Framing the Assessment
From the outset, psychologists need to create the space and conditions for effective clinical reasoning. Of particular importance is the intentional practice to move away from the narrow framing of a case (e.g., “Does the client have _____ diagnosis?”) because it similarly narrows the hypotheses generated, data collected, and the data that are considered ( Gambrill 2012 ). Heath and Heath ( 2013 ) have argued that when individuals hold one hypothesis, all of their “ego” is invested in it, making it more challenging to actively attempt to disprove it or to pay attention to disconfirming information, increasing the likelihood of engaging in confirmation bias. Putting forth a single hypothesis results in that hypothesis representing them as professionals, making it hard to be open to the possibility that their proposed hypothesis is incorrect. In contrast, developing multiple hypotheses allows the professionals’ egos to be spread across the hypotheses, so as to allow their professional egos to be protected should one or more of their hypotheses be disconfirmed. In order to fully consider multiple hypotheses and to acknowledge the uncertainty inherent in assessment, it may be beneficial to ask what would need to be true for each of them to be the correct diagnosis, making sure to consider those hypotheses in which the psychologist does not initially have much confidence ( Heath and Heath 2013 ).
Opening this space from the outset requires psychologists to reflect on their own assumptions about the client, referral question, and their goals versus client goals, in order to take steps to minimize bias and improve clinical reasoning ( Gambrill 2019 ). It is important for psychologists to identify their assumptions about the client or about the presenting problems so that they can work to move beyond asking questions that reflect their beliefs rather than listening to the actual questions the client would like to have answered ( Gambrill 2012 ). Consideration should also be given to noting potentially negative aspects of the process for clients, including the fact that accessing services may still be challenging after receiving a diagnosis and that recommendations generally require time and effort for the clients and their families ( Heath and Heath 2013 ). This process requires strong listening skills and using motivational interviewing principles to better understand what the client wants to know and the changes to which they are committed in their lives ( Suarez 2011 ). Motivational interviewing has the additional benefit that it can be used to increase client participation and their willingness to engage with later recommendations because it involves the psychologist taking the time to understand client goals and their willingness to make changes; it empowers clients to collaboratively engage in the assessment process ( Suarez 2011 ).
6.2. Data Collection
Addressing cognitive biases in clinical practice is beyond the scope of this paper (see Gambrill 2012 ; 2019 ; Wilcox and Schroeder 2015 ). However, the most frequently noted strategy to improve clinical reasoning is to intentionally and systematically seek out information that could disprove the hypothesis, which relates to confirmation bias ( Kleinmuntz 1990 ). Confirmation bias is a common contributor to making poor decisions because, when psychologists invest time and energy in pursuing a single hypothesis, they also invest their ego in it, which makes it more difficult to let the hypothesis go if there is disconfirming evidence. Humans are good at convincing themselves that they are collecting data in order to make a decision, when they are actually garnering support for the decision that they have already made ( Heath and Heath 2013 ), making it important to take intentional steps to acknowledge and minimize confirmation bias in practice. Over-collecting data increases confidence without decreasing the objective uncertainty ( Gambrill 2012 ).
Many assessment errors are the result of inattention and distraction during the test administration or the overconfidence that, with experience, psychologists can administer the test with less active engagement (e.g., reading test instructions verbatim; Oak et al. 2019 ). As noted above, acknowledging that all psychologists, including ourselves, are at risk of errors, rather than engaging in blind spot bias (e.g., “Others make errors, but I don’t”), is the first step to the increasing awareness of errors and in taking steps to reduce them ( Gambrill 2012 ). It is also important to remember that assessment is more than merely testing ( Suhr 2015 ; Wright 2021 ). Assessment requires choosing measures to answer specific questions related to hypotheses from case conceptualization, actively approaching the data as a detective, attending not only to the psychometric properties of the measures but also attending to contextual and individual factors and the psychology of human behavior, which includes test scores as one source of data among many ( Canivez 2019 ; Suhr 2015 ; Wright 2021 ).
6.3. Interpretation and Decision-Making
Psychologists face pressure to find answers for clients to support them in their difficulties, which can make psychologists feel as though they have to provide definitive answers. Psychologists, however, should beware of extremely high levels of confidence in predictive accuracy ( Kleinmuntz 1990 ); they should, instead, practice humble acknowledgment of the limitations of the data available and of human judgment. In line with the ideals of Socratic ignorance, also known as Socratic wisdom, we should acknowledge the limits of the certainty of our conclusions because, as Popper ( 1996 ) noted, “… in our infinite ignorance, we are all equal” (p. 5). It is important to remember that there is always uncertainty during assessment; failing to acknowledge that uncertainty can increase errors ( Gambrill 2012 ). We should also make sure to attend to contextual factors rather than only focusing on individual factors within the client, such as data from testing ( Gambrill 2012 ). Finally, psychologists should consider documenting their decision-making process at each step, to increase transparency and access to information that could reveal errors, providing the opportunity to learn from them rather than repeat them ( Kahneman et al. 2021 ). Psychologists should consider several questions to ensure that assessment findings are useful for clients, asking themselves: Do these findings and diagnoses help clients to better understand themselves? Do they inform recommendations that the clients are likely to follow? Do these findings make the clients and their families feel empowered ( Nelson 2021 )?
6.4. Considering Base Rates
Base rates represent one available tool to support clinical reasoning and increase diagnostic accuracy. Meehl ( 1957 ) argued that psychologists make more accurate decisions when they use base rates, rather than when they use clinical judgment. Consideration of “the relative frequency of phenomena” or of disorders and behaviors in a population (i.e., base rates; Kamphuis and Finn 2002 ) is important to consider because many psychologists work in clinical settings where almost all clients are presenting with a problem, making it easy to forget what is typical and what is abnormal in a population.
Base rate fallacy or base rate neglect occurs when practitioners do not use base rates when diagnosing; this results in false positives or negatives in the diagnostic decisions ( Koehler 1996 ). Inattention to base rates is more likely to lead to poor decisions when the base rates conflict with other diagnostic information than when the data are in concordance. Koehler ( 1996 ) concluded that decision-makers are often accurate in situations with ample data and when these data are in line with base rates. They are, however, more prone to errors when the base rates are very different from their data. Base rate data can also be challenging due to the complexity of comorbidities that clients present with and the lack of operational definitions of the criteria for disorders ( Ward 2019 ).
When base rate data are available, it is often aggregated (i.e., across the population). This provides the benefit of reducing the bias of individual clinics or psychologists ( Reynolds 2016 ), but it may also obscure actual differences in base rates in a clinical setting as normative-based research sometimes hides individual differences, making them less useful for diagnostic purposes ( Ward 2019 ). In order to effectively use base rates, psychologists need to have information that is specific to their type of practice. For example, the base rate of a specific disorder will be very different in a general practice than in a clinic specializing in a specific disorder, and there may be differences based on other demographic data (e.g., sex, geographical region, ethnicity, age ( Youngstrom and Van Meter 2016 )).
Although clinicians should consider base rates as part of EBA, there are some noted limitations. First, most studies looking at base rate neglect have been conducted in laboratory settings to find errors ( Koehler 1996 ), leading to a limited understanding of the conditions under which base rate neglect occurs in real-life settings. A lack of information about the occurrence in practical settings makes it unclear how often base rate neglect is a problem, suggesting that the problem might be overemphasized in the research ( Koehler 1996 ). Second, there are no clear guidelines or formulas that psychologists can use to apply base-rate information in their practice ( Kleinmuntz 1990 ). Third, during assessments, psychologists not only diagnose but provide information on the client’s strengths and weaknesses, functioning, and prognosis, which cannot be accounted for by base rates ( Garb and Schramke 1996 ). Further, research is needed to elucidate how to effectively incorporate base rates into practice.
6.5. Recommendations and Feedback
Building on the previous discussion of DP, psychologists should seek feedback throughout the assessment process and after the assessment is over. The brief nature of the assessment relationship requires that psychologists intentionally and effortfully seek out this feedback ( Siegert 1999 ). As noted in the work on DP in therapy, it is necessary to seek out negative feedback in order to identify areas of growth, which is necessary to improve practice because psychologists are not likely to receive this important feedback as a matter of course ( Miller et al. 2020 ).
Although not yet a common practice connected to psychoeducational assessments, there is a value in later connecting with clients to assist with the evaluation of clinical reasoning skills in relation to improved client functioning. To maximize the client’s uptake of recommendations, one should be transparent in providing clients with evidence for the effectiveness of an assessment and recommendations, so that clients can make informed decisions ( Gambrill 2012 ). Only 5% of clients think that psychologists’ recommendations are helpful ( Postal et al. 2018 ); when there are five recommendations, the clients will follow just over half of them ( Elias et al. 2020 ). Even worse, about a third of clients do not follow any of the recommendations ( Elias et al. 2020 ). Consequently, it is important to consider how psychologists can use clinical reasoning to improve the usability of recommendations. It may be helpful to work with clients to prioritize recommendations with clients and to engage in premortem planning to identify potential barriers, to ensure that they answer meaningful questions ( Heath and Heath 2013 ), asking clients to think ahead, imagining that they did not implement the recommendation, and identifying what might prevent them from implementing the intervention. Then, the practitioner should work with the client to come up with solutions for each of those barriers. Conversely, it is also possible to ask clients to think ahead and pretend that they did implement the recommendation, and to identify what helped them to implement it. Then, we should work with clients to come up with ways to maximize those supports. This process complements motivational interviewing techniques by empowering clients to identify the recommendations that are the most meaningful to them, and encourages them to take an active role in determining the implementation of recommendations ( Suarez 2011 ).
7. Conclusions
Clinical reasoning is an integral part of EBA that is currently poorly understood. As a result, there is little information on how psychologists develop clinical reasoning, how to assess the quality of clinical reasoning during an assessment, or how to gain and improve clinical reasoning skills. This has resulted in recommendations related to pieces of the assessment process, such as test administration, base rates, and report writing, without understanding the role of clinical reasoning in ensuring an EBA that supports clients. This paper outlines the current research in the area of clinical reasoning and draws from work in related fields to provide some initial suggestions on how to intentionally attend to clinical reasoning during an assessment. However, more work is needed to better understand the process of clinical reasoning in assessment, in order to determine the best ways to teach, monitor, and improve the clinical reasoning of psychologists during the assessment process.
Funding Statement
This research received no external funding.
Author Contributions
Conceptualization: G.W., M.S., M.A.D.; Writing Original Draft: G.W.; Writing Reviewing and Editing: G.W., M.S., M.A.D. All authors have read and agreed to the published version of the manuscript.
Institutional Review Board Statement
Informed consent statement, data availability statement, conflicts of interest.
The authors declare no conflict of interest.
Disclaimer/Publisher’s Note: The statements, opinions and data contained in all publications are solely those of the individual author(s) and contributor(s) and not of MDPI and/or the editor(s). MDPI and/or the editor(s) disclaim responsibility for any injury to people or property resulting from any ideas, methods, instructions or products referred to in the content.
- Ahmed Adil H., Giri Jyothsna, Kashyap Rahul, Singh Balwinder, Dong Yue, Kilickaya Oguz, Erwin Patricia J., Murad M. Hassan, Pickering Brian W. Outcome of adverse events and medical errors in the intensive care unit: A systematic review and meta-analysis. American Journal of Medical Quality. 2015; 30 :23–30. doi: 10.1177/1062860613514770. [ PubMed ] [ CrossRef ] [ Google Scholar ]
- American Psychological Association Evidence-based practice in psychology. American Psychologist. 2006; 61 :271–85. doi: 10.1037/0003-066X.61.4.271. [ PubMed ] [ CrossRef ] [ Google Scholar ]
- American Psychological Association APA Dictionary of Psychology. [(accessed on 10 June 2022)]. n.d. Available online: https://dictionary.apa.org/critical-thinking
- Arocha José F., Patel Vimla L. Novice diagnostic reasoning in medicine: Accounting for evidence. [(accessed on 10 June 2022)]; The Journal of the Learning Sciences. 1995 4 :355–84. doi: 10.1207/s15327809jls0404_1. Available online: http://www.jstor.org/stable/1466784 [ CrossRef ] [ Google Scholar ]
- Aspel Andrew D., Willis W. Grant, Faust David. School psychologists’ diagnostic decision-making processes: Objective-subjective discrepancies. Journal of School Psychology. 1998; 36 :137–49. doi: 10.1016/S0022-4405(98)00002-8. [ CrossRef ] [ Google Scholar ]
- Bornstein Robert F. Evidence-based psychological assessment. Journal of Personality Assessment. 2017; 99 :435–45. doi: 10.1080/00223891.2016.1236343. [ PubMed ] [ CrossRef ] [ Google Scholar ]
- Burns Candace W. Base rate theory and school psychology. School Psychology Review. 1990; 19 :356–66. doi: 10.1080/02796015.1990.12085474. [ CrossRef ] [ Google Scholar ]
- Canivez Gary L. Evidence-based assessment for school psychology: Research, training, and clinical practice. Contemporary School Psychology. 2019; 23 :194–200. doi: 10.1007/s40688-019-00238-z. [ CrossRef ] [ Google Scholar ]
- Chow Daryl L., Miller Scott D., Seidel Jason A., Kane Robert T., Thornton Jennifer A. The role of deliberate practice in the development of highly effective psychotherapists. Psychotherapy. 2015; 52 :337–45. doi: 10.1037/pst0000015. [ PubMed ] [ CrossRef ] [ Google Scholar ]
- Clements-Hickman Alyssa L., Reese Robert J. Improving therapists’ effectives: Can deliberate practice help? Professional Psychology: Research and Practice. 2020; 51 :606–12. doi: 10.1037/pro0000318. [ CrossRef ] [ Google Scholar ]
- Cook Jonathan R., Hausman Estee M., Jensen-Doss Amanda, Hawley Kristin M. Assessment practices of child clinicians. Assessment. 2017; 24 :210–21. doi: 10.1177/1073191115604353. [ PMC free article ] [ PubMed ] [ CrossRef ] [ Google Scholar ]
- Dailor A. Nichole, Jacob Susan. Ethically challenging situations reported by school psychologists: Implications for training. Psychology in the Schools. 2011; 48 :619–31. doi: 10.1002/pits.20574. [ CrossRef ] [ Google Scholar ]
- de Mesquita Paul D. Diagnostic problem solving of school psychologists: Scientific method or guesswork? Journal of School Psychology. 1992; 30 :269–91. doi: 10.1016/0022-4405(92)90011-S. [ CrossRef ] [ Google Scholar ]
- Del Mar Chris, Doust Jenny, Glasziou Paul P. Critical thinking: Evidence, communication, and decision-making. Blackwell Publishing Inc.; Malden: 2006. [ Google Scholar ]
- Dombrowski Stefan C., McGill Ryan J., Farmer Ryan L., Kranzler John H., Canivez Gary L. Beyond the rhetoric of evidence-based assessment: A framework for critical thinking in clinical practice. School Psychology Review. 2021:1–4. doi: 10.1080/2372966X.2021.1960126. [ CrossRef ] [ Google Scholar ]
- Duvivier Robbert J., Dalen Jan van, Muijtjens Arno M., Moulaert Véronique R. M. P., van der Vleuten Cees P. M., Scherpbier Albert J. J. A. The role of deliberate practice in the acquisition of clinical skills. BMC Medical Education. 2011; 11 :101. doi: 10.1186/1472-6920-11-101. [ PMC free article ] [ PubMed ] [ CrossRef ] [ Google Scholar ]
- Eells Tracy D., Lombart Kenneth G., Kendjelic Edward M., Turner L. Carolyn, Lucas Cynthia P. The quality of psychotherapy case formulations: A comparison of expert, experienced, and novice cognitive-behavioral and psychodynamic therapists. Journal of Consulting and Clinical Psychology. 2005; 73 :579–89. doi: 10.1037/0022-006X.73.4.579. [ PubMed ] [ CrossRef ] [ Google Scholar ]
- Elias John, Zimak Eric, Sherwood Andrea, MacDonald Beatriz, Lozano Nubia, Long Jason, Larsen A. Denise. Do parents implement pediatric neuropsychological report recommendations? The Clinical Neuropsychologist. 2020; 35 :1117–33. doi: 10.1080/13854046.2020.1720298. [ PubMed ] [ CrossRef ] [ Google Scholar ]
- Ericsson K. Anders. The influence of experience and deliberate practice on the development of superior expert performance. In: Ericsson K. Anders, Hoffman Robert R., Kozbelt Aaron, Williams A. Mark., editors. The Cambridge Handbook of Expertise and Expert Performance. University Press; Cambridge: 2006. pp. 685–705. [ Google Scholar ]
- Ericsson K. Anders. The differential influence of experience, practice, and deliberate practice on the development of superior individual performance of experts. In: Ericsson K. Anders, Hoffman Robert R., Kozbelt Aaron, Williams A. Mark., editors. The Cambridge Handbook of Expertise and Expert Performance. 2nd ed. Cambridge University Press; Cambridge: 2018. pp. 745–69. [ Google Scholar ]
- Gambrill Eileen. Critical Thinking in Clinical Practice: Improving the Quality of Judgments and Decisions. 3rd ed. John Wiley and Sons; Hoboken: 2012. [ Google Scholar ]
- Gambrill Eileen. Critical Thinking and the Process of Evidence-Based Practice. Oxford University Press; New York: 2019. [ Google Scholar ]
- Garb Howard N., Schramke Carol J. Judgment research and neuropsychological assessment: A narrative review and meta-analyses. Psychological Bulletin. 1996; 120 :140–53. doi: 10.1037/0033-2909.120.1.140. [ PubMed ] [ CrossRef ] [ Google Scholar ]
- Gordon David, Rencic Joseph J., Lang Valerie J., Thomas Aliki, Young Meredith, Durning Steven J. Advancing the assessment of clinical reasoning across the health professions: Definitional and methodological recommendations. Perspectives on Medical Education. 2022; 11 :108–14. doi: 10.1007/S40037-022-00701-3. [ PMC free article ] [ PubMed ] [ CrossRef ] [ Google Scholar ]
- Gross Thomas J., Farmer Ryan L., Ochs Sarah E. Evidence-based assessment: Best practices, customary practices, and recommendations for field-based assessment. Contemporary School Psychology. 2019; 23 :304–26. doi: 10.1007/s40688-018-0186-x. [ CrossRef ] [ Google Scholar ]
- Gruppen Larry D. Clinical reasoning: Defining it, teaching it, assessing it, studying it. The Western Journal of Emergency Medicine. 2017; 18 :4–7. doi: 10.5811/westjem.2016.11.33191. [ PMC free article ] [ PubMed ] [ CrossRef ] [ Google Scholar ]
- Harding Thomas P. Clinical decision-making: How prepared are we? Training and Education in Professional Psychology. 2007; 1 :95–104. doi: 10.1037/1931-3918.1.2.95. [ CrossRef ] [ Google Scholar ]
- Haynes R. Brian, Devereaux P. J., Guyatt Gordon H. Clinical expertise in the era of evidence-based medicine and patient choice. BMJ Evidence-Based Medicine. 2002; 7 :36–38. doi: 10.1136/ebm.7.2.36. [ PubMed ] [ CrossRef ] [ Google Scholar ]
- Heath Chip, Heath Dan. Decisive: How to Make Better Choices in Lift and Work. Random House Canada; Toronto: 2013. [ Google Scholar ]
- Kahneman Daniel, Sibony Olivier, Sunstein Cass R. Noise: A Flaw in Human Judgement. Little Brown Spark; New York: 2021. [ Google Scholar ]
- Kamphuis Jan H., Finn Stephen E. Incorporating base rate information in daily clinical decision making. In: Butcher James N., editor. Clinical Personality Assessment: Practical Approaches. Oxford University Press; New York: 2002. pp. 256–68. [ Google Scholar ]
- Kleinmuntz Benjamin. Why we still use our heads instead of formulas. Psychological Bulleti. 1990; 107 :296–310. doi: 10.1037/0033-2909.107.3.296. [ PubMed ] [ CrossRef ] [ Google Scholar ]
- Koehler Jonathan J. The base rate fallacy reconsidered: Descriptive, normative, and methodological challenges. Behavioral and Brain Sciences. 1996; 19 :1–53. doi: 10.1017/S0140525X00041157. [ CrossRef ] [ Google Scholar ]
- Krishnamurthy Radhika, Creek Leon Vande, Kaslow Nadine J., Tazeau Yvette N., Miville Marie L., Kerns Robert, Stegman Robert, Suzuki Lisa, Benton Sheryl A. Achieving competency in psychological assessment: Directions for education and training. Journal of Clinical Psychology. 2004; 60 :725–39. doi: 10.1002/jclp.20010. [ PubMed ] [ CrossRef ] [ Google Scholar ]
- Lillienfeld Scott O., Basterfield Candice. Reflective practice in clinical psychology: Reflections from basis psychological science. Clinical Psychology: Science and Practic. 2020; 27 :e12352. doi: 10.1111/cpsp.12352. [ CrossRef ] [ Google Scholar ]
- Mash Eric J., Hunsley John. Evidence-based assessment of child and adolescent disorders: Issues and challenges. Journal of Clinical Child and Adolescent Psycholog. 2005; 34 :362–39. doi: 10.1207/s15374424jccp3403_1. [ PubMed ] [ CrossRef ] [ Google Scholar ]
- McLeod Julia. How students use deliberate practice during the first stage of counsellor training. Counselling and Psychotherapy Research. 2021; 22 :1–12. doi: 10.1002/capr.12397. [ CrossRef ] [ Google Scholar ]
- Meehl Paul E. When shall we use our heads instead of the formula? Journal of Counseling Psychology. 1957; 4 :268–73. doi: 10.1037/h0047554. [ CrossRef ] [ Google Scholar ]
- Meyer Gregory J., Finn Stephen E., Eyde Lorraine D., Kay Gary G., Moreland Kevin L., Dies Robert R., Eisman Elena J., Kubiszyn Tom W., Reed Geoffrey M. Psychological testing and psychological assessment: A review of evidence and issues. American Psychologist. 2001; 56 :123–65. doi: 10.1037/0003-066X.56.2.128. [ PubMed ] [ CrossRef ] [ Google Scholar ]
- Miller Scott D., Hubble Mark A., Chow Daryl. Better Results: Using Deliberate Practice to Improve Therapeutic Effectiveness. American Psychological Association; Washington, DC: 2020. [ Google Scholar ]
- Nelson Stephanie. Advanced Report Writing [Webinar] Massachusetts Neuropsychological Society; Hopkinton: 2021. [(accessed on 9 November 2021)]. Available online: https://www.massneuropsych.org/content.aspx?page_id=22andclub_id=41215andmodule_id=448777 [ Google Scholar ]
- Oak Erika, Viezel Kathleen D., Dumont Ron, Willis John. Wechsler administration and scoring errors made my graduate students and school psychologists. Journal of Psychoeducational Assessment. 2019; 37 :679–91. doi: 10.1177/0734282918786355. [ CrossRef ] [ Google Scholar ]
- Pelco Lynn E., Ward Sandra B., Coleman Lindsay, Young Julie. Teacher ratings of three psychological report styles. Training and Education in Professional Psychology. 2009; 3 :19–27. doi: 10.1037/1931-3918.3.1.19. [ CrossRef ] [ Google Scholar ]
- Popper Karl. In Search of a Better World: Lectures and Essays from Thirty Years. Routledge; New York: 1996. [ Google Scholar ]
- Postal Karen, Chow Clifton, Jung Sharon, Erickson-Moreo Kalen, Geier Flannery, Lanca Margaret. The stakeholders’ project in neuropsychological report writing: A survey of neuropsychologists’ and referral sources’ views of neuropsychological reports. The Clinical Neuropsychologist. 2018; 32 :326–44. doi: 10.1080/13854046.2017.1373859. [ PubMed ] [ CrossRef ] [ Google Scholar ]
- Rettew David C., Lynch Alicia Doyle, Achenbach Thomas M., Dumenci Levent, Ivanova Masha Y. Meta-analyses of agreement between diagnoses made from clinical evaluations and standardized diagnostic interviews. International Journal of Methods in Psychiatric Research. 2009; 18 :169–84. doi: 10.1002/mpr.289. [ PMC free article ] [ PubMed ] [ CrossRef ] [ Google Scholar ]
- Reynolds Cecil R. Contextualized evidence and empirically based testing and assessment. Clinical Psychology: Science and Practice. 2016; 23 :410–16. doi: 10.1111/cpsp.12181. [ CrossRef ] [ Google Scholar ]
- Sanchez Carmen, Dunning David. Overconfidence among beginners: Is a little learning a dangerous thing? Journal of Personality and Social Psychology. 2018; 114 :10–28. doi: 10.1037/pspa0000102. [ PubMed ] [ CrossRef ] [ Google Scholar ]
- Siegert Richard J. Some thoughts about reasoning in clinical neuropsychology. Behavior Change. 1999; 16 :37–48. doi: 10.1375/bech.16.1.37. [ CrossRef ] [ Google Scholar ]
- Suarez Mariann. Application of motivational interviewing to neuropsychology practice: A new frontier for evaluations and rehabilitation. In: Schoenberg Mike R., Scott James G., editors. The Little Black Book of Neuropsychology: A Syndrome-Based Approach. Springer; Boston: 2011. pp. 863–71. [ Google Scholar ]
- Suhr Julie A. Psychological Assessment: A Problem-Solving Approach. Guilford; New York: 2015. [ Google Scholar ]
- Tracey Terence J. G., Wampold Bruce E., Lichtenberg James W., Goodyear Rodney K. Expertise in psychotherapy: An elusive goal? American Psychologist. 2014; 69 :218–29. doi: 10.1037/a0035099. [ PubMed ] [ CrossRef ] [ Google Scholar ]
- Victor-Chmil Joyce. Critical thinking versus clinical reasoning versus clinical judgment: Differential diagnosis. Nurse Educator. 2013; 38 :34–36. doi: 10.1097/NNE.0b013e318276dfbe. [ PubMed ] [ CrossRef ] [ Google Scholar ]
- Ward Thomas J. EBA: Good idea but is it feasible? Contemporary School Psychology. 2019; 23 :190–93. doi: 10.1007/s40688-019-00239-y. [ CrossRef ] [ Google Scholar ]
- Watkins Marley W. Errors in diagnostic decision making and clinical judgment. In: Gutkin Terry B., Reynolds Cecil R., editors. The Handbook of School Psychology. 4th ed. John Wiley and Sons Inc.; Hoboken: 2009. pp. 210–29. [ Google Scholar ]
- Wilcox Gabrielle, Schroeder Meadow. What comes before report writing? Attending to clinical reasoning and thinking errors in school psychology. Journal of Psychoeducational Assessment. 2015; 33 :652–61. doi: 10.1177/0734282914562212. [ CrossRef ] [ Google Scholar ]
- Wright A. Jordan, Pade Hadas, Gottfried Emily D., Arbisi Paul A., McCord David M., Wygant Dustin B. Evidence-based clinical psychological assessment (EBCPA): A review of the current state of the literature and best practices. Professional Psychology: Research and Practice. 2022; 53 :372–86. doi: 10.1037/pro0000447. [ CrossRef ] [ Google Scholar ]
- Wright A. Jordan. Conducting Psychological Assessment: A Guide for Practitioners. 2nd ed. Wiley; Hoboken: 2021. [ Google Scholar ]
- Young Meredith E., Thomas Aliki, Lubarsky Stuart, Gordon David, Gruppen Larry D., Rencic Joseph, Ballard Tiffany, Holmboe Eric, Da Silva Ana, Ratcliffe Temple, et al. Mapping clinical reasoning literature across the health professions: A scoping review. BMC Medical Education. 2020; 20 :107. doi: 10.1186/s12909-020-02012-9. [ PMC free article ] [ PubMed ] [ CrossRef ] [ Google Scholar ]
- Youngstrom Eric A., Van Meter Anna. Empirically supported assessment of children and adolescents. Clinical Psychology: Science and Practice. 2016; 23 :327–47. doi: 10.1111/cpsp.12172. [ CrossRef ] [ Google Scholar ]
- Youngstrom Eric A., Choukas-Bradley Sophia, Calhoun Casey D., Jensen-Doss Amanda. Clinical guide to the evidence-based assessment approach to diagnosis and treatment. Behavioral Practice. 2015; 22 :20–35. doi: 10.1016/j.cbpra.2013.12.005. [ CrossRef ] [ Google Scholar ]
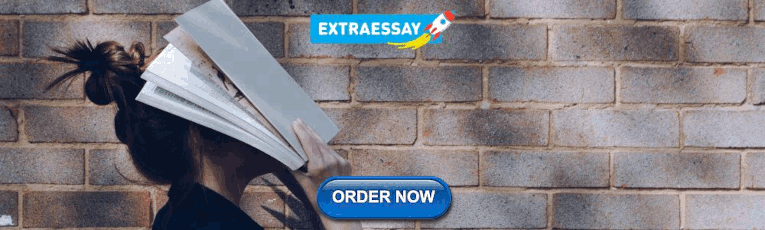
COMMENTS
The outcome of critical thinking, clinical reasoning, and decision making is known as clinical _____, the conclusion, decision, or opinion reached after analyzing information. judgment A nursing student is committed to providing thoughtful, person-centered care.
Study with Quizlet and memorize flashcards containing terms like The outcome of critical thinking or clinical reasoning is known as clinical _____, the conclusion, decision, or opinion the nurse makes., Avoiding information contrary to one's opinion is an example of _____, an approach that leads to potential errors in clinical decision making., Critical thinking is defined as "a systematic way ...
Study with Quizlet and memorize flashcards containing terms like The outcome of critical thinking or clinical reasoning is known as clinical _____, the conclusion, decision, or opinion the nurse makes., Avoiding information contrary to one's opinion is an example of _____, an approach that leads to potential errors in clinical decision making., Critical thinking is defined as "a systematic way ...
What Is Critical Thinking, Clinical Reasoning, and Clinical ...
Clinical Reasoning, Decisionmaking, and Action: Thinking ...
Clinical decision-making integrates critical thinking and clinical judgment to choose effective interventions and strategies for achieving positive patient outcomes. While critical thinking focuses on the process of reasoning and evaluating information, clinical judgment applies this thinking to clinical situations to interpret and prioritize ...
The Foundation for Critical Thinking Clinical Reasoning Based on Critical Thinking Concepts and Tools The Thinker's Guide to Client: Foundation for Critical Thinking Project Title: Clinical Reasoning Guide ©2010 (09-032) Proof 14 Proof 15 Proof 16 Proof 17 2/5/10 8:00am 1:45p 2/8/10 9am
In nursing, critical thinking is a broad term that includes reasoning about clinical issues such as teamwork, collaboration, and streamlining workflow." On the other hand, clinical reasoning is defined as a complex cognitive process that uses formal and informal thinking strategies to gather and analyze patient information, evaluate the ...
As detailed in the table, multiple themes surrounding the cognitive and meta-cognitive processes that underpin clinical reasoning have been identified. Central to these processes is the practice of critical thinking. Much like the definition of clinical reasoning, there is also diversity with regard to definitions and conceptualisation of critical thinking in the healthcare setting.
Improving clinical reasoning techniques is the right way to facilitate decision-making from prognostic, diagnostic, and therapeutic points of view. However, the process to do that is to fill knowledge gaps by studying and growing experience and knowing some cognitive aspects to raise the awareness of thinking mechanisms to avoid cognitive ...
Rather than a historical overview as in Chap. 2, this chapter provides the reader with insight into the various approaches that have been used to understand clinical reasoning. We review concepts and major scholars who have been involved in such investigations. Cognitive psychologists Newel and Simon theorized about problem-solving skills and artificial intelligence and initiated the use of ...
Study with Quizlet and memorize flashcards containing terms like The outcome of critical thinking, clinical reasoning, and decision making is known as clinical _____, the conclusion, decision, or opinion reached after analyzing information, Ignoring information contrary to one's own opinion is an example of _____, an approach that leads to potential errors in clinical decision making, Patient ...
System 1: FAST - intuitive, effortless, using information that is readily available (often visual), and usually occurring without our knowing (i.e. pattern recognition) System 2: SLOW - analytical and deliberate and relies on the active assembly of collected information (logistic and probabilities) (i.e. diagnostic schema) Diagnostic schema ...
Advanced practice: critical thinking and clinical reasoning
efore engaging in the process of clinical reasoning. This guide does not address the knowledge and skills requir. d to competently gather and interpret clinical data. Rather, the guide is intended to help clinicians take the next step, which is determining the best course of action to take based on what is known or wh.
The outcome of critical thinking, clinical reasoning, and decision making is known as clinical reasoning _____ the conclusion, decision or opinion reached after analyzing information. Judgment. patient safety and transparency of information are two principles of _____- centered care that can be used by every organization.
Summary. Clinical reasoning is at the core of all health-related professions, and it is long recognized as a critical skill for clinical practice. Yet, it is difficult to characterize it, as clinical reasoning combines different high-thinking abilities. Also, it is not content that is historically taught or learned in a particular subject.
Teaching clinical reasoning is challenging, particularly in the time-pressured and complicated environment of the ICU. Clinical reasoning is a complex process in which one identifies and prioritizes pertinent clinical data to develop a hypothesis and a plan to confirm or refute that hypothesis. Clinical reasoning is related to and dependent on critical thinking skills, which are defined as one ...
Teaching Strategies for Developing Clinical Reasoning ...
Clinical reasoning concerns how to think and make the best decision-making process associated with the clinical practice (9). The core elements of clinical reasoning (10) can be summarized in: 1. Evidence-based skills, 2. Interpretation and use of diagnostic tests, 3. Understanding cognitive biases, 4.
Background Successful deployment of clinical prediction models for clinical deterioration relates not only to predictive performance but to integration into the decision making process. Models may demonstrate good discrimination and calibration, but fail to match the needs of practising acute care clinicians who receive, interpret, and act upon model outputs or alerts. We sought to understand ...
This paper will first define the role of clinical reasoning in evidence-based assessment and the research related to this area, outlining some of the contemporary challenges in the training and research related to clinical reasoning in assessment. The second section will summarize the current, albeit limited, literature on how psychologists ...