
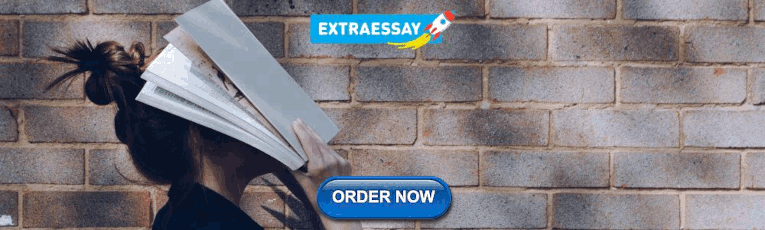
Artificial intelligence in education
Artificial Intelligence (AI) has the potential to address some of the biggest challenges in education today, innovate teaching and learning practices, and accelerate progress towards SDG 4. However, rapid technological developments inevitably bring multiple risks and challenges, which have so far outpaced policy debates and regulatory frameworks. UNESCO is committed to supporting Member States to harness the potential of AI technologies for achieving the Education 2030 Agenda, while ensuring that its application in educational contexts is guided by the core principles of inclusion and equity. UNESCO’s mandate calls inherently for a human-centred approach to AI . It aims to shift the conversation to include AI’s role in addressing current inequalities regarding access to knowledge, research and the diversity of cultural expressions and to ensure AI does not widen the technological divides within and between countries. The promise of “AI for all” must be that everyone can take advantage of the technological revolution under way and access its fruits, notably in terms of innovation and knowledge.
Furthermore, UNESCO has developed within the framework of the Beijing Consensus a publication aimed at fostering the readiness of education policy-makers in artificial intelligence. This publication, Artificial Intelligence and Education: Guidance for Policy-makers , will be of interest to practitioners and professionals in the policy-making and education communities. It aims to generate a shared understanding of the opportunities and challenges that AI offers for education, as well as its implications for the core competencies needed in the AI era

The UNESCO Courier, October-December 2023

- Plurilingual
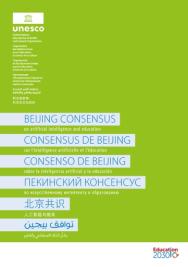
by Stefania Giannini, UNESCO Assistant Director-General for Education
International Forum on artificial intelligence and education
- More information
- Analytical report

Through its projects, UNESCO affirms that the deployment of AI technologies in education should be purposed to enhance human capacities and to protect human rights for effective human-machine collaboration in life, learning and work, and for sustainable development. Together with partners, international organizations, and the key values that UNESCO holds as pillars of their mandate, UNESCO hopes to strengthen their leading role in AI in education, as a global laboratory of ideas, standard setter, policy advisor and capacity builder. If you are interested in leveraging emerging technologies like AI to bolster the education sector, we look forward to partnering with you through financial, in-kind or technical advice contributions. 'We need to renew this commitment as we move towards an era in which artificial intelligence – a convergence of emerging technologies – is transforming every aspect of our lives (…),' said Ms Stefania Giannini, UNESCO Assistant Director-General for Education at the International Conference on Artificial Intelligence and Education held in Beijing in May 2019. 'We need to steer this revolution in the right direction, to improve livelihoods, to reduce inequalities and promote a fair and inclusive globalization.’'

Related items
- Artificial intelligence
How AI can accelerate students’ holistic development and make teaching more fulfilling
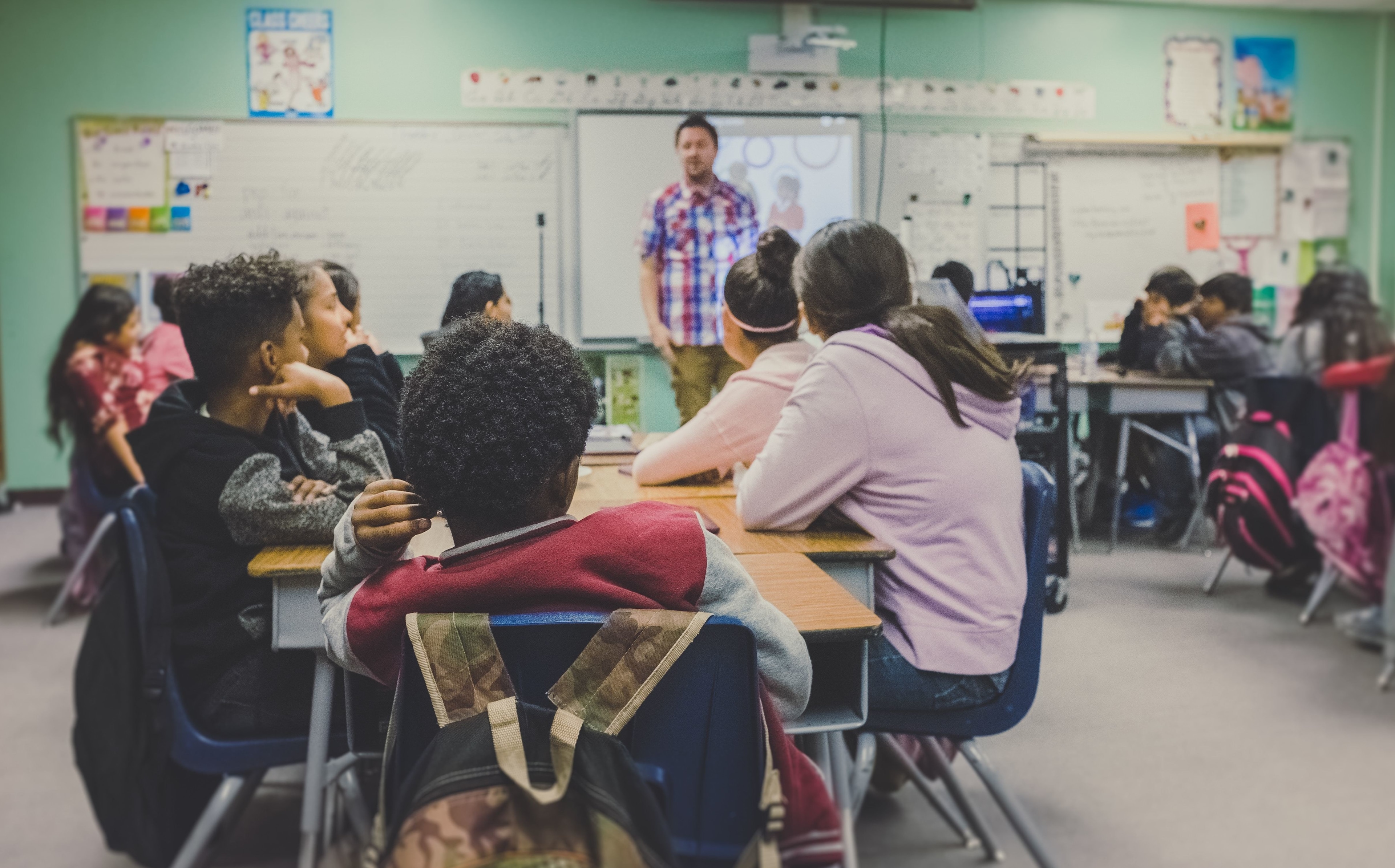
AI can free up time in the classroom. Image: Kenny Eliason on Unsplash
.chakra .wef-1c7l3mo{-webkit-transition:all 0.15s ease-out;transition:all 0.15s ease-out;cursor:pointer;-webkit-text-decoration:none;text-decoration:none;outline:none;color:inherit;}.chakra .wef-1c7l3mo:hover,.chakra .wef-1c7l3mo[data-hover]{-webkit-text-decoration:underline;text-decoration:underline;}.chakra .wef-1c7l3mo:focus,.chakra .wef-1c7l3mo[data-focus]{box-shadow:0 0 0 3px rgba(168,203,251,0.5);} Wendy Kopp
Bo stjerne thomsen.
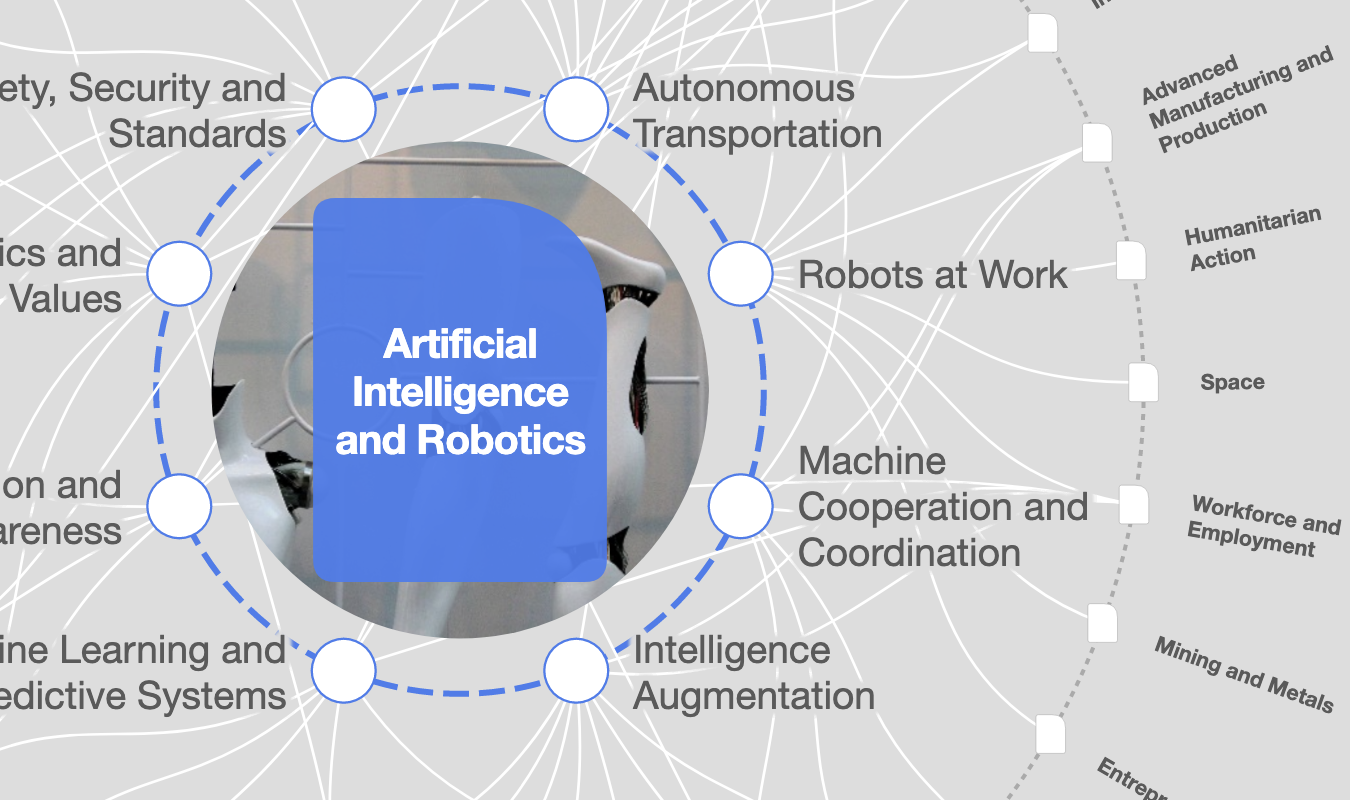
.chakra .wef-9dduvl{margin-top:16px;margin-bottom:16px;line-height:1.388;font-size:1.25rem;}@media screen and (min-width:56.5rem){.chakra .wef-9dduvl{font-size:1.125rem;}} Explore and monitor how .chakra .wef-15eoq1r{margin-top:16px;margin-bottom:16px;line-height:1.388;font-size:1.25rem;color:#F7DB5E;}@media screen and (min-width:56.5rem){.chakra .wef-15eoq1r{font-size:1.125rem;}} Artificial Intelligence is affecting economies, industries and global issues
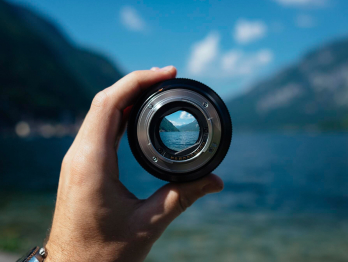
.chakra .wef-1nk5u5d{margin-top:16px;margin-bottom:16px;line-height:1.388;color:#2846F8;font-size:1.25rem;}@media screen and (min-width:56.5rem){.chakra .wef-1nk5u5d{font-size:1.125rem;}} Get involved with our crowdsourced digital platform to deliver impact at scale
Stay up to date:, society and equity.
Listen to the article
- Advances in artificial intelligence (AI) could transform education systems and make them more equitable.
- It can accelerate the long overdue transformation of education systems towards inclusive learning that will prepare young people to thrive and shape a better future.
- At the same time, teachers can use these technologies to enhance their teaching practice and professional experience.
With the rapidly accelerating integration of artificial intelligence (AI) in our work, life, and classrooms, educators all over the world are re-evaluating the purpose of education in light of these outsized implications. At Teach For All and the LEGO Foundation, we see the potential of AI to accelerate the long overdue transformation of education systems towards inclusive learning that will prepare young people to thrive and shape a better future.
At the same time, we see huge opportunities for teachers to use these technologies to enhance their own teaching practice and professional experience.
Have you read?
Our children are growing up with ai. here's what you need to know, chatgpt and cheating: 5 ways to change how students are graded, here's what americans think about generative ai like chatgpt and dall-e, the future of jobs report 2023.
Dialogue on the future of work and education has long emphasized the importance of developing skills and values that are uniquely human and less likely to be replaced by technology. The rise of ChatGPT is yet another proof point. Most students and teachers agree that ChatGPT is “just another example of why we can’t keep doing things the old way for schools in the modern world”. (Although please note that this blog was written in the "old way" as an interactive collaboration between humans, and not generated by AI.)
How AI tools can help educators and students
Our hope is that the advent of AI will spur educators, students, parents, and policy-makers to come together to consider what skills our students really need to navigate uncertainty, solve complex challenges and shape meaningful futures in a changing economy. This means embracing the challenge to provide learning that fosters agency, awareness, critical thinking and problem-solving skills, connectedness, and well-being. We already see that AI tools deployed by well-trained and well-supported teachers can be invaluable for accelerating progress towards this vision.
AI can help foster the skills students will need to navigate and shape the future. Tools like ChatGPT, as Dr. Kathy Hirsh-Pasek and Elias Blinkoff argue , can help promote students’ critical thinking when used in sophisticated ways for deeper, more engaged learning.
Vriti Saraf, CEO and founder of K20 Educators, in New York, agrees: “The less students need educators to be the main source of knowledge, the more educators can focus on developing the ability to curate, guide, critically assess learning, and help students gain skills that are so much more important than memorizing information.”
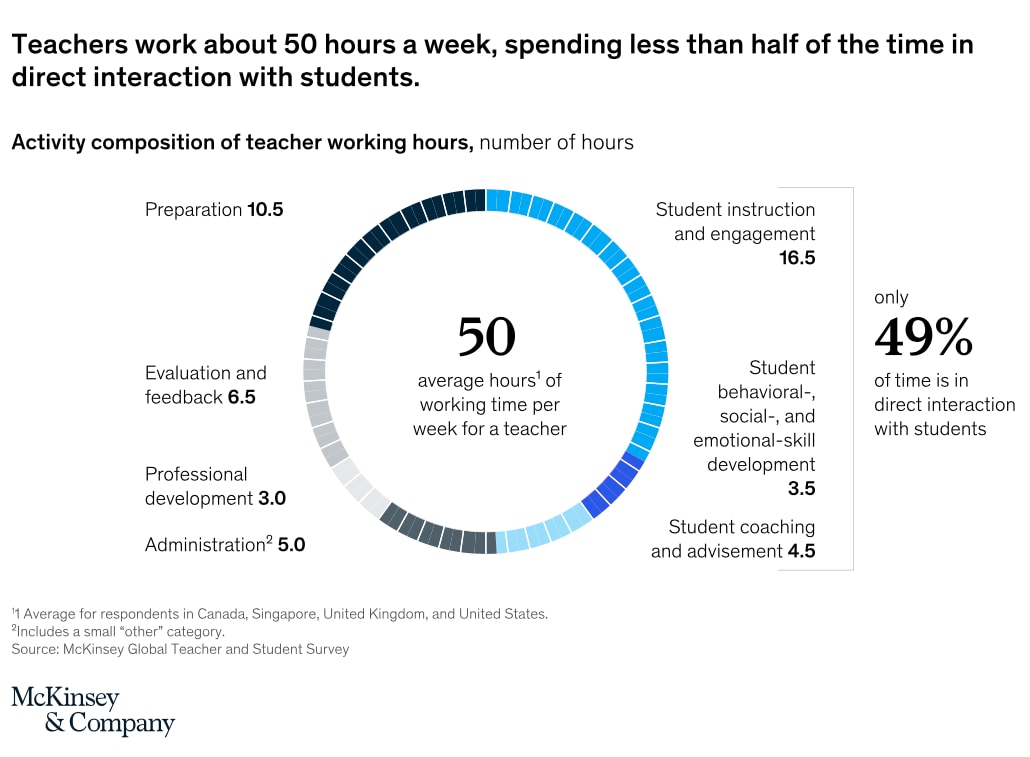
Noting the increased importance of social-emotional learning, Henry May, CEO of Co-School in Bogota, Colombia, notes that teachers have an essential role in shaping childhood experiences away from screens. So, "teachers must be trained on how to educate students on ethical principles; how to use AI tools appropriately; and how to mitigate the potential risk of AI to reduce human connection and belonging and increase loneliness."
Another potential benefit is that AI can free up time in the classroom. Teachers often cite unmanageable administrative tasks as their greatest source of exhaustion and burnout. By automating routine administrative tasks, AI could help streamline teacher workflows, giving them more time to build relationships with students and foster their learning and development.
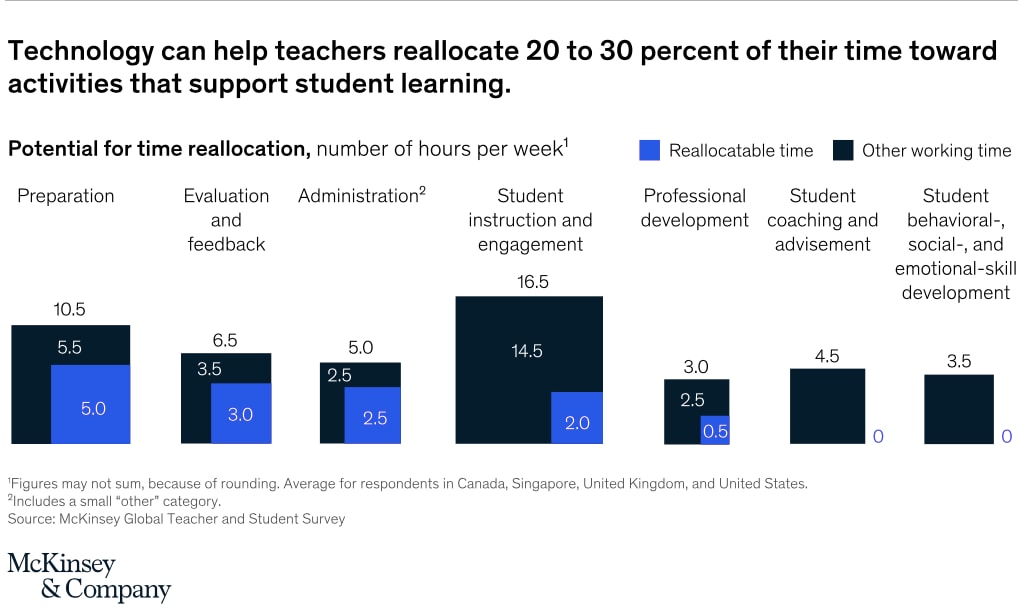
A classroom view of AI tools
Quim Sabría, a former teacher in Barcelona, Spain and co-founder of Edpuzzle, says AI could improve teacher productivity across areas like lesson planning and differentiation, grading and providing quality feedback, teacher-parent communication, and professional development.
In Lagos, Nigeria, teachers are beginning to see the efficiency and ease that AI brings to their work. Oluwaseun Kayode, who taught in Lagos and founded Schoolinka, is currently seeing an increasing number of teachers from across West Africa using AI to identify children’s literacy levels, uncover where students are struggling, and deepen personalized learning experiences.
In the US state of Illinois, a similar pattern is seen where Diego Marin , an 8th-grade math teacher, likens ChatGPT to “a personalized 1:1 tutor that is super valuable for students.”
The Top 10 Emerging Technologies of 2023 report outlined the technologies poised to positively impact society in the next few years, from health technology to AI to sustainable computing.
The World Economic Forum’s Centre for the Fourth Industrial Revolution is driving responsible technology governance, enabling industry transformation, addressing planetary health, and promoting equity and inclusion.
Learn more about our impact:
- Digital inclusion: Our EDISON Alliance is mobilizing leaders from across sectors to accelerate digital inclusion, having positively impacted the lives of 454 million people through the activation of 250 initiatives across 90 countries.
- AI in developing economies: Our Centre for the Fourth Industrial Revolution Rwanda is promoting the adoption of new technologies in the country, enabling over 4,000 daily health consultations using AI.
- Innovative healthcare: Our Medicine from the Sky initiative is using drones to deliver medicine to remote areas in India, completing over 950 successful drone flights.
- AI for agriculture: We are working with the Government of India to scale up agricultural technology in the country, helping more than 7,000 farmers monitor the health of their crops and soil using AI.
Want to know more about our centre’s impact or get involved? Contact us .
Equitable use of AI in education
We see tremendous promise for AI to spur educators around the world to reorient their energy away from routine administrative tasks towards accelerating students’ growth and learning, thus making teaching more fulfilling.
But we need to stay vigilant to ensure that AI is a force for equity and quality. We’ve all heard stories of technology being used to create shortcuts for lesson planning, and we must keep fine-tuning AI so that it does not replicate existing biases.
AI tools can be a catalyst for the transformation of our education systems but only with a commitment to a shared vision for equitable holistic education that gives all children the opportunity to thrive. To ensure AI benefits all students, including the most marginalized, we recommend being mindful of the following principles:
- Co-creation: Bring together ed-tech executives and equity-conscious educators from diverse communities to collaborate on applications of AI that reflect strong pedagogy, address local needs and cultural contexts, and overcome existing biases and inequities.
- Easy entry points: Support teachers to access and apply technologies to reduce administrative burdens and provide more personalized learning by providing open access resources and collaborative spaces to help them integrate AI into their work.
- Digital literacy: Invest in IT fundamentals and AI literacy to mitigate the growing digital divide, ensuring access to devices, bandwidth, and digital literacy development for teachers and students to overcome barriers.
- Best practice: Collect and shareinspiring examples of teachers using technologies to support student voice, curiosity, and agency for more active forms of learning to help inspire other teachers to leverage AI in these ways.
- Innovation and adaptation: Work with school leaders to support teacher professional development and foster a culture of innovation and adaptability. Recognize and reward teachers for new applications of AI and encourage peer-to-peer learning and specialized training.
Don't miss any update on this topic
Create a free account and access your personalized content collection with our latest publications and analyses.
License and Republishing
World Economic Forum articles may be republished in accordance with the Creative Commons Attribution-NonCommercial-NoDerivatives 4.0 International Public License, and in accordance with our Terms of Use.
The views expressed in this article are those of the author alone and not the World Economic Forum.
Related topics:
The agenda .chakra .wef-n7bacu{margin-top:16px;margin-bottom:16px;line-height:1.388;font-weight:400;} weekly.
A weekly update of the most important issues driving the global agenda
.chakra .wef-1dtnjt5{display:-webkit-box;display:-webkit-flex;display:-ms-flexbox;display:flex;-webkit-align-items:center;-webkit-box-align:center;-ms-flex-align:center;align-items:center;-webkit-flex-wrap:wrap;-ms-flex-wrap:wrap;flex-wrap:wrap;} More on Forum Institutional .chakra .wef-nr1rr4{display:-webkit-inline-box;display:-webkit-inline-flex;display:-ms-inline-flexbox;display:inline-flex;white-space:normal;vertical-align:middle;text-transform:uppercase;font-size:0.75rem;border-radius:0.25rem;font-weight:700;-webkit-align-items:center;-webkit-box-align:center;-ms-flex-align:center;align-items:center;line-height:1.2;-webkit-letter-spacing:1.25px;-moz-letter-spacing:1.25px;-ms-letter-spacing:1.25px;letter-spacing:1.25px;background:none;padding:0px;color:#B3B3B3;-webkit-box-decoration-break:clone;box-decoration-break:clone;-webkit-box-decoration-break:clone;}@media screen and (min-width:37.5rem){.chakra .wef-nr1rr4{font-size:0.875rem;}}@media screen and (min-width:56.5rem){.chakra .wef-nr1rr4{font-size:1rem;}} See all
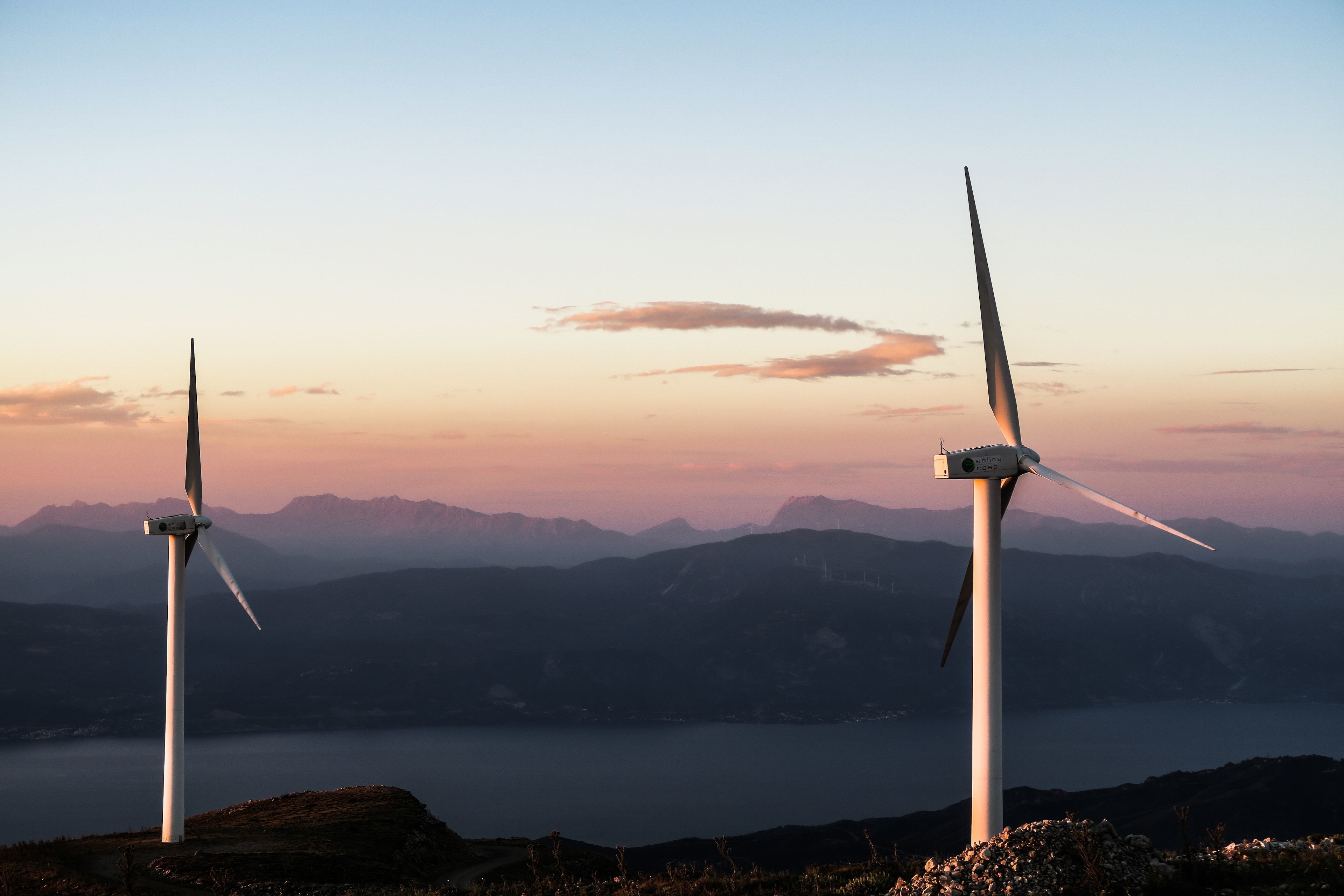
Climate finance: What are debt-for-nature swaps and how can they help countries?
Kate Whiting
April 26, 2024
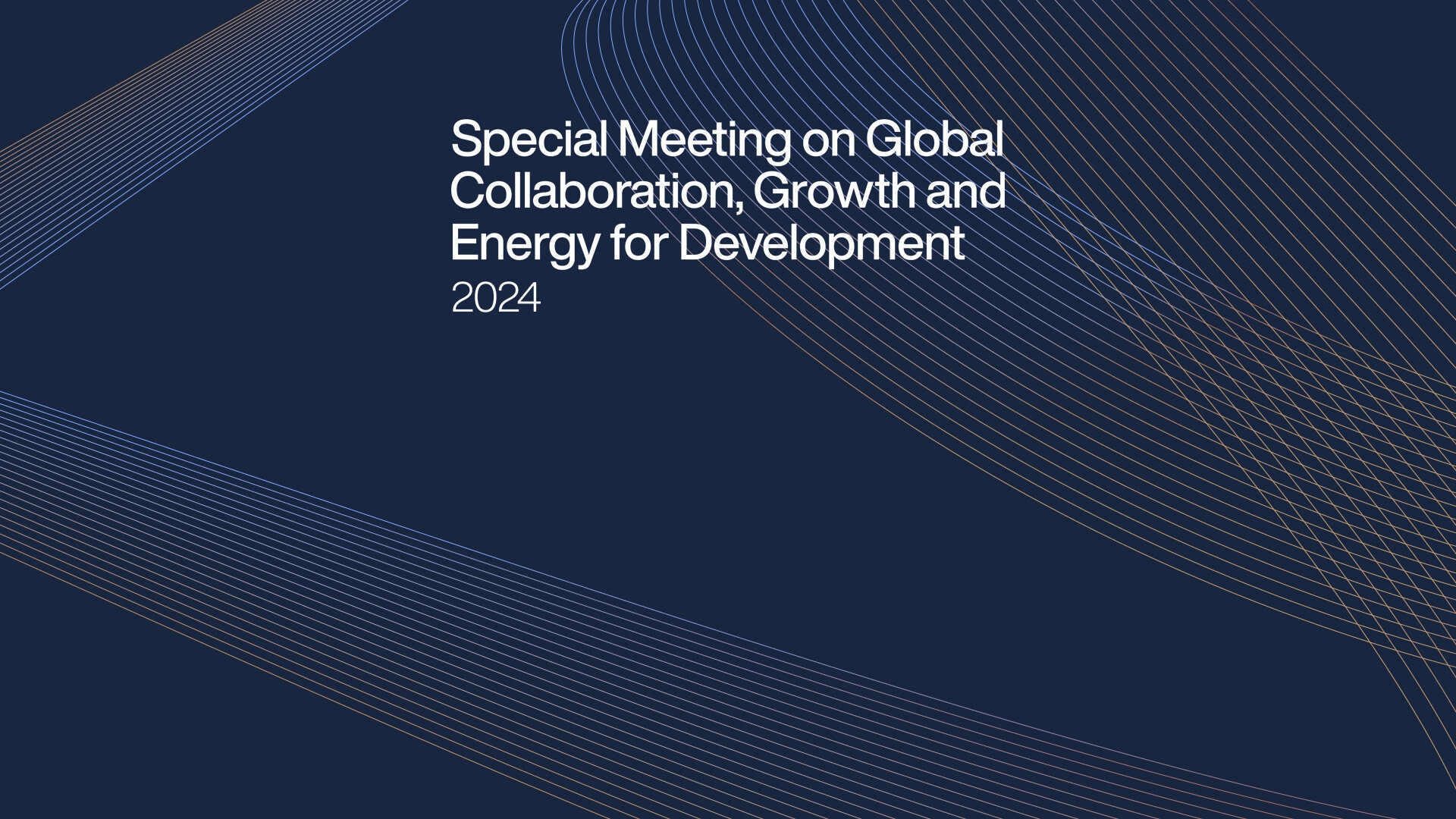
What to expect at the Special Meeting on Global Collaboration, Growth and Energy for Development
Spencer Feingold and Gayle Markovitz
April 19, 2024
From 'Quit-Tok' to proximity bias, here are 11 buzzwords from the world of hybrid work
April 17, 2024
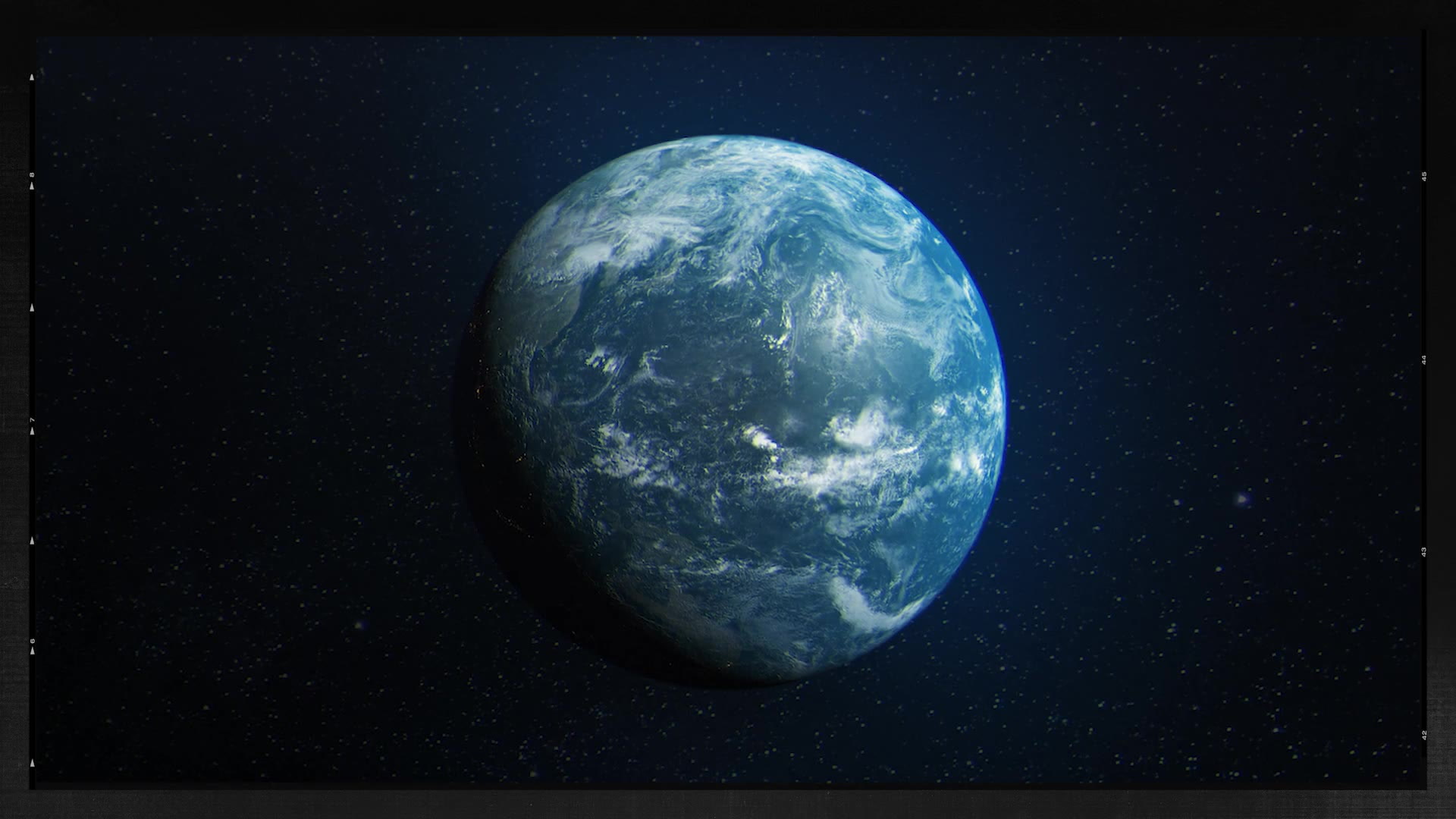
Davos 2024 Opening Film
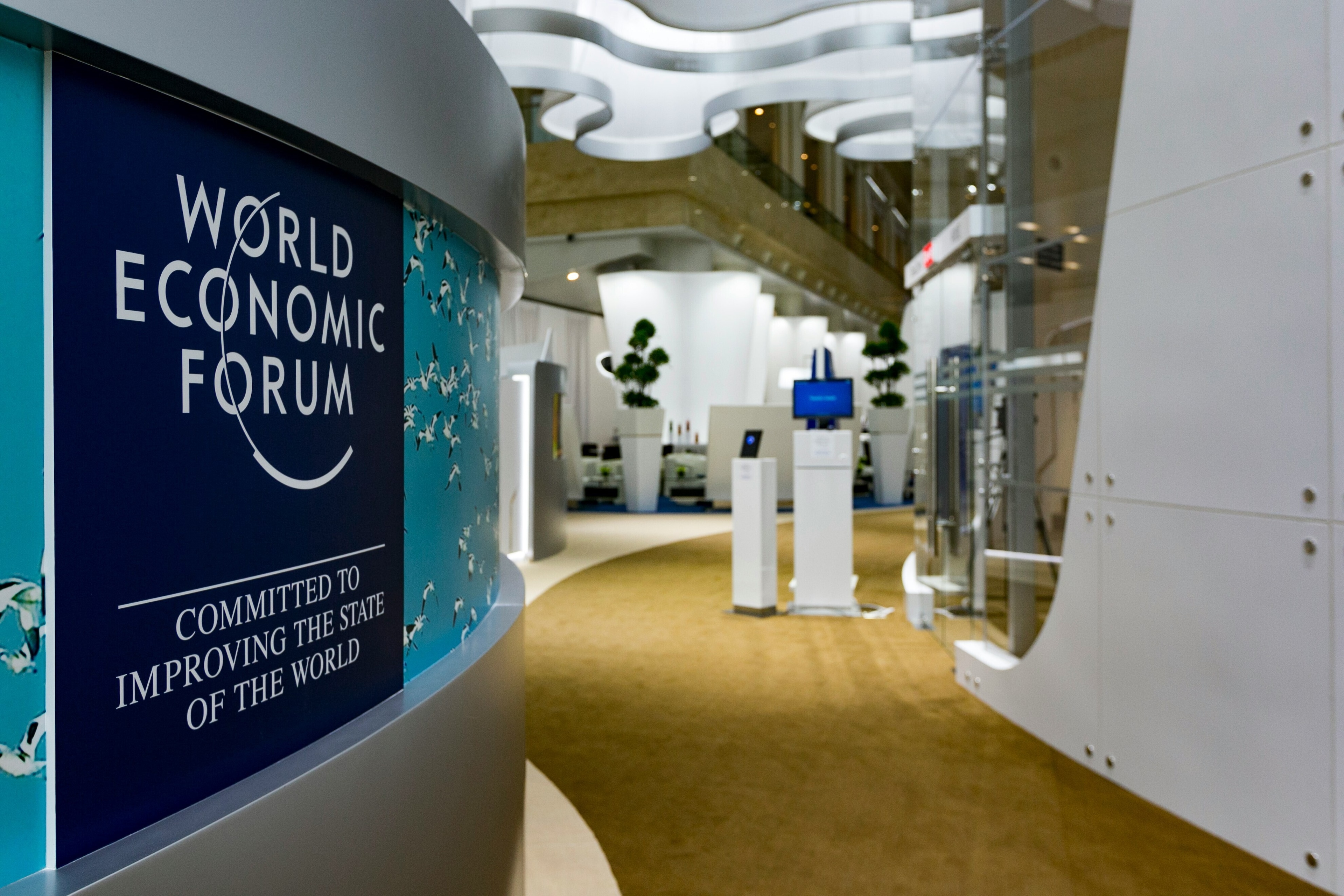
Building trust amid uncertainty – 3 risk experts on the state of the world in 2024
Andrea Willige
March 27, 2024
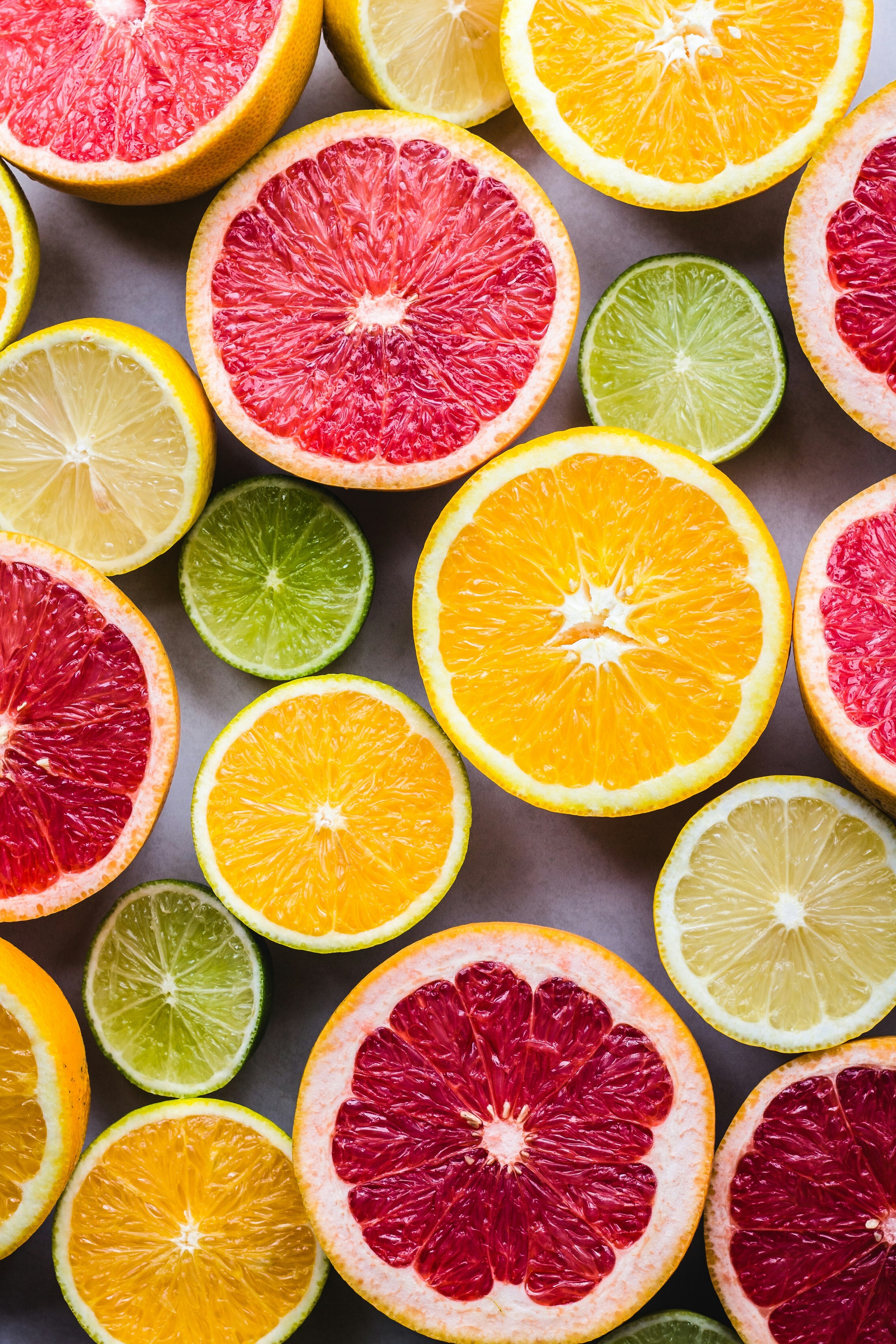
Why obesity is rising and how we can live healthy lives
Shyam Bishen
March 20, 2024

U.S. Department of Education
U.S. Department of Education Shares Insights and Recommendations for Artificial Intelligence
Today, the U.S. Department of Education's Office of Educational Technology (OET) released a new report, "Artificial Intelligence (AI) and the Future of Teaching and Learning: Insights and Recommendations" that summarizes the opportunities and risks for AI in teaching, learning, research, and assessment based on public input. This report is part of the Biden-Harris Administration's ongoing effort to advance a cohesive and comprehensive approach to AI-related opportunities and risks.
The new report addresses the clear need for sharing knowledge, engaging educators and communities, and refining technology plans and policies for AI use in education. It recognizes AI as a rapidly advancing set of technologies that can enable new forms of interaction between educators and students, help educators address variability in learning, increase feedback loops, and support educators. It also outlines risks associated with AI—including algorithmic bias—and the importance of trust, safety, and appropriate guardrails to protect educators and students. The report recommends that the Department continue working with states, institutions of higher education, school districts and other partners to collaborate on the following steps:
- Emphasize Humans-in-the-Loop
- Align AI Models to a Shared Vision for Education
- Design AI Using Modern Learning Principles
- Prioritize Strengthening Trust
- Inform and Involve Educators
- Focus R&D on Addressing Context and Enhancing Trust and Safety
- Develop Education-specific Guidelines and Guardrails
To gather information and formulate insights, OET partnered with Digital Promise , a global nonprofit that works to expand opportunities for every learner. Over 700 educational stakeholders participated in a series of four public listening sessions in summer 2022. Stakeholders described promising opportunities they see for AI in education and discussed risks–especially risks of algorithmic bias–and called for stronger educational technology guidance. The Biden-Harris Administration remains committed to addressing the increasingly urgent need to leverage technology in the education sector and promote novel and impactful ways to bring together educators, researchers, and developers to craft better policies. OET looks forward to leading further work to align AI models to a shared vision for education, inform and involve educators, and develop education-specific guidelines and guardrails.
Join the public webinar scheduled June 13, 2023, 2:30 – 3:30pm ET to learn more about the report and the Department's vision for supporting information sharing and supporting policies for AI. Read the full report here , core messages here , and look for more information about the webinar at tech.ed.gov/ai .
- Tags: Press Releases
How Do I Find...?
- Student loans, forgiveness
- Higher Education Rulemaking
- College accreditation
- Every Student Succeeds Act (ESSA)
- 1098, tax forms
Information About...
- Elevating Teaching
- Early Learning
- Engage Every Student
- Unlocking Career Success
- Cybersecurity
Search press releases
Find by month.
- February 2024
- January 2024
- December 2023
- November 2023
- October 2023
- September 2023
- August 2023
- All Press Releases
How artificial intelligence will impact K–12 teachers
The teaching profession is under siege. Working hours for teachers are increasing as student needs become more complex and administrative and paperwork burdens increase. According to a recent McKinsey survey, conducted in a research partnership with Microsoft, teachers are working an average of 50 hours a week 1 McKinsey Global Teacher and Student Survey. Average of Canada, Singapore, United Kingdom, and United States in 2017. —a number that the Organisation for Economic Co-operation and Development Teaching and Learning International Survey suggests has increased by 3 percent over the past five years. 2 TALIS 2018 Results: Teachers and School Leaders as Lifelong Learners , volume 1, Paris, France: OECD Publishing, 2019. Comparison of 2013 and 2018 total working hours for teachers in the United States.
While most teachers report enjoying their work, they do not report enjoying the late nights marking papers, preparing lesson plans, or filling out endless paperwork. Burnout and high attrition rates are testaments to the very real pressures on teachers. In the neediest schools in the United States, for example, teacher turnover tops 16 percent per annum. 3 Desiree Carver-Thomas and Linda Darling-Hammond, “Teacher turnover: Why it matters and what we can do about it,” Learning Policy Institute, August 16, 2017, learningpolicyinstitute.org. Note that the turnover in Title I schools is 16 percent, which is 50 percent greater than that in non–Title I schools. It is even higher for teachers with three or fewer years of experience, at 28 percent. In the United Kingdom, the situation is even worse, with 81 percent of teachers considering leaving teaching altogether because of their workloads. 4 Teachers and workload , National Education Union, March 2018. Further disheartening to teachers is the news that some education professors have even gone so far as to suggest that teachers can be replaced by robots, computers, and artificial intelligence (AI) . 5 “Is education obsolete? Sugata Mitra at the MIT Media Lab,” MIT Center for Civic Media, May 16, 2012, civic.mit.edu; John von Radowitz, “Intelligent machines will replace teachers within 10 years, leading public school headteacher predicts,” Independent , September 11, 2017, independent.co.uk.
Our research offers a glimmer of hope in an otherwise bleak landscape. The McKinsey Global Institute’s 2018 report on the future of work suggests that, despite the dire predictions, teachers are not going away any time soon. In fact, we estimate the school teachers will grow by 5 to 24 percent in the United States between 2016 and 2030. For countries such as China and India, the estimated growth will be more than 100 percent. 6 Parul Batra, Jacques Bughin, Michael Chui, Ryan Ko, Susan Lund, James Manyika, Saurabh Sanghvi, and Jonathan Woetzel, Jobs lost, jobs gained: What the future of work will mean for jobs, skills, and wages , McKinsey Global Institute, November 2017. Moreover, our research suggests that, rather than replacing teachers, existing and emerging technologies will help them do their jobs better and more efficiently.
Our current research suggests that 20 to 40 percent of current teacher hours are spent on activities that could be automated using existing technology. That translates into approximately 13 hours per week that teachers could redirect toward activities that lead to higher student outcomes and higher teacher satisfaction. In short, our research suggests that existing technology can help teachers reallocate 20 to 40 percent of their time to activities that support student learning.
Further advances in technology could push this number higher and result in changes to classroom structure and learning modalities, but are unlikely to displace teachers in the foreseeable future. Many of the attributes that make good teachers great are the very things that AI or other technology fails to emulate: inspiring students, building positive school and class climates, resolving conflicts, creating connection and belonging, seeing the world from the perspective of individual students, and mentoring and coaching students. These things represent the heart of a teacher’s work and cannot—and should not—be automated.
Make no mistake, the value of a good education starts early and lasts a lifetime. Research suggests that simply having an effective kindergarten teacher can affect the likelihood of a student completing college thus boosting their lifetime earnings by about $320,000. 7 Raj Chetty et al., “$320,000 kindergarten teachers,” Phi Delta Kappan , November 2010, Volume 92, Number 3, pp. 22–5, journals.sagepub.com. Technology, when used correctly, can facilitate good teaching, but it will never replace teachers. In the remainder of this article, we will outline how teachers spend their time today, how technology can help to save teacher time, and where that additional time might go. Note that we are intentionally focused on the impact of technology on teacher time. In future articles we will address its broader impact on student learning.
How teachers spend their time
To understand how teachers are spending their time today and how that might change in a more automated world, we surveyed more than 2,000 teachers in four countries with high adoption rates for education technology: Canada, Singapore, the United Kingdom, and the United States. 8 Teachers surveyed by country: 501 in Canada, 134 in Singapore, 509 in the United Kingdom, and 1,028 in the United States. We asked teachers how much time they spend on 37 core activities, from lesson planning to teaching to grading to maintaining student records.
We asked where teachers would like to spend more and less time. We asked what technologies teachers and students were currently using in the classroom to discover new content, practice skills, and provide feedback. Finally, we asked what was working well and where they faced challenges, both in the application of technology and more broadly across their role as teacher. Our findings were unequivocal: teachers, across the board, were spending less time in direct instruction and engagement than in preparation, evaluation, and administrative duties (Exhibit 1).
How technology can aid teachers
Once we understood how teachers spend their time, we evaluated automation potential across each activity, based on an evaluation of existing technology and expert interviews. We concluded that the areas with the biggest potential for automation are preparation, administration, evaluation, and feedback. Conversely, actual instruction, engagement, coaching, and advising are more immune to automation (Exhibit 2).
Where to save time with technology
The area with the biggest automation potential is one that teachers deal with before they even get to the classroom: preparation. Across the four countries we studied, teachers spend an average of 11 hours a week in preparation activities. We estimate that effective use of technology could cut the time to just six hours. Even if teachers spend the same amount of time preparing, technology could make that time more effective, helping them come up with even better lesson plans and approaches. For example, several software providers offer mathematics packages to help teachers assess the current level of their students’ understanding, group students according to learning needs, and suggest lesson plans, materials, and problem sets for each group. In other subjects, collaboration platforms enable teachers to search and find relevant materials posted by other teachers or administrators.
Technology has the least potential to save teacher time in areas where teachers are directly engaging with students: direct instruction and engagement, coaching and advisement, and behavioral-, social-, and emotional-skill development. It is worth pausing here for a moment to note that we are not denying that technology will change the student experience of learning, although we would recommend caution and measured expectations.
While controlled pilot studies have shown improvements in student learning from technology-rich, personalized blended learning , 9 John F. Pane et al., “How does personalized learning affect student achievement?,” RAND, 2017, rand.org. these improvements have not yet been realized on a large scale. The most recent Program for International Student Assessment scores suggest that, globally, students who use tablets, laptops, and e-readers in the classroom are performing worse than those who do not . Why the disconnect?
Our hypothesis is that implementing technology in the classroom at scale is hard. Just providing hardware is easy. Integrating effective software that links to student-learning goals within the curriculum—and training teachers on how to adapt to it—is difficult. This underscores why we believe that technology in the classroom is not going to save much direct instructional time. To improve student outcomes, the teacher still needs to be in the classroom, but their role will shift from instructor to facilitator and coach. For example, some teachers are using flipped learning in their classrooms. Instead of teaching a concept in the classroom and then having students go home to practice it, they assign self-paced videos as homework to give the basic instruction and then have students practice in the classroom, where the teacher can provide support and fill gaps in understanding.
How to improve student educational outcomes
Evaluation and feedback complete the teaching loop. As teachers understand what their students know and can do, they can then prepare for the next lesson. Technology has already helped here—for example, computer grading of multiple-choice questions was possible long before AI and is particularly penetrated in math instruction. More is possible. Advances in natural-language processing make it possible for computers to assess and give detailed, formative feedback across long-form answers in all subject areas. For example, writing software can look at trends in writing across multiple essays to provide targeted student feedback that teachers can review and tailor. Combined, these technologies could save three of the current six hours a week that teachers spend on evaluation and feedback.
Finally, administration is a bugbear of teachers globally. After all, who prefers filling out paperwork to interacting with children? Good news is on the horizon. Automation could reduce the amount of time teachers spend on administrative responsibilities—down from five to just three hours per week. Software can automatically fill out forms (or provide menus of potential responses); maintain inventories of materials, equipment, and products; and even automatically order replacements.
Where the time will go
What will teachers do with the additional 13 hours a week saved by the application of technology? Some of this time, hopefully, will be given back to teachers themselves—to spend time with their families and their communities—thus increasing the attractiveness of teaching as a profession.
Much of the time saved, however, can be plowed back into improving education through more personalized learning and more direct coaching and mentoring. In our survey, about a third of teachers said that they wanted to personalize learning but did not feel that they were doing so effectively at present. Their biggest barriers: time, resources, materials, and technology (Exhibit 3). Automation can help with all of these. Even when teachers believed that they were already providing tailored materials—and personalized feedback—to students, students often disagreed. While 60 percent of the teachers surveyed believed that their feedback was personalized to each student, only 44 percent of the students surveyed felt the same way.
Additional time can also help support social–emotional learning and the development of the 21st-century skills that will be necessary to thrive in an increasingly automated workplace. It will enable teachers to foster one-on-one relationships with students, encourage self-regulation and perseverance, and help students collaborate with each other. Research shows that strong relationships with teachers promote student learning and well-being, especially for students from low-income families. 10 Desiree W. Murray et al., Self-regulation and toxic stress: Foundations for understanding self-regulation from an applied developmental perspective , Office of Planning, Research & Evaluation, February 13, 2015, acf.hhs.gov. Automation within the teaching profession could thus be a catalyst in reducing educational inequalities.
Finally, teachers could spend more time collaborating with each other. More time for collaboration should translate into better outcomes for students. International comparative studies show that high-performing school systems double down on peer coaching and collaborative lesson planning. 11 Andreas Schleicher, “Teaching Excellence through Professional Learning and Policy Reform: Lessons from around the World,” Paris, France: OECD Publishing, 2016. These practices can support teachers in improving and developing their craft. 11 Andreas Schleicher, “Teaching Excellence through Professional Learning and Policy Reform: Lessons from around the World,” Paris, France: OECD Publishing, 2016. For example, the leerkRACHT Foundation in the Netherlands has introduced peer collaboration into 10 percent of Dutch schools, with 80 percent of teachers reporting improvement in student learning.
How to make it happen
All of this begs a question: How will we capture the promise of technology in our schools? The good news is that this is not about technology we have not yet invented. It will not require AI systems that pass the Turing test. To the contrary, achieving these savings in teacher time is mostly about adoption of existing education technology. Just bringing the average school to the level of the best would have a huge impact.
This, however, is no small task. It will require commitment across a broad range of stakeholders, including governments, school leaders, technology companies, and, of course, teachers and learners themselves. Four imperatives stand out as schools move to adopt technology wisely: target investment, start with easy solutions, share what is working, and build teacher and school-leader capacity to harness technology effectively.
The schools that are currently best in applying technology to save teacher time have often been able to access more funding than the average school. Democratizing these gains will entail increased investment in every school, especially those that are currently under-resourced. As investment increases, it will be critical to target it to the areas that can most effectively save teacher time and improve student outcomes (rather than to flashy but ineffective hardware).
Starting with easy solutions will provide early momentum. Proven technology that can replace simple administrative tasks or simple evaluative tools for formative testing can immediately provide teachers with respite, whetting their appetite for more holistic solutions.
Part of the problems that schools face today is the myriad of competing solutions, some of which are fantastic, but many of which promise great things but deliver little. Sharing what is working—and what is not working—is therefore critical. Neutral arbiters bringing objective and rigorous performance data, similar to the service that EdReports.org provides on curriculum, are necessary in the education-technology space. It will also be necessary to make best-practice solutions available to teachers at all types of schools and school systems.
Finally, building the capacity of teachers and school leaders to harness technology effectively will ensure maximum gains in not only saving teacher time but also improving student outcomes. Districts and schools need to balance introducing new technologies with fully integrating existing ones into the curriculum and teachers’ professional development. Districts need to use accepted, widely adopted tools for consistency. However, teachers should have the freedom to pilot alternatives, and they should have a strong voice in deciding which tools are working in the classroom and should roll out districtwide. Technology companies, too, need to be better in including the voice of the teacher when guiding product development.
If these four imperatives are met, then we are hopeful that automation will be a boon and not a bane for teachers. Ten years from now, with the support of a range of education technologies, teachers should have more time for themselves—and more time for their students. They can pour that time into improving student outcomes and preparing students for a more challenging and automated workforce.
Jake Bryant is an associate partner in McKinsey’s Washington, DC, office, Christine Heitz is a consultant in the Denver office, Saurabh Sanghvi is an associate partner in the Silicon Valley office, and Dilip Wagle is a senior partner in the Seattle office.
Explore a career with us
Related articles.

Building operational excellence in higher education

How higher-education institutions can transform themselves using advanced analytics
The past, present and future of AI in education
On UCI Podcast, Shayan Doroudi and Nia Nixon share expertise on tech evolutions in teaching and learning

ChatGPT, a popular artificial intelligence tool that can generate written materials in response to prompts, reached 100 million monthly active users in January 2023, just two months after its launch. The unprecedented escalation made OpenAI’s creation the fastest-growing consumer application in history, according to a report from UBS based on analytics from Similarweb .
Responses from the world of education to an AI tool that could write essays for students were immediate and mixed , with some teachers concerned about student cheating and plagiarism and others celebrating its potential to generate new ideas for instruction and lesson planning.
Various researchers in UC Irvine’s School of Education are studying the wide range of technological innovations available to enhance education, including assistant professors Shayan Doroudi and Nia Nixon . One facet of Doroudi’s research focuses on how different technologies improve learning. A segment of Nixon’s work centers on developing AI-based interventions to promote inclusivity in team problem-solving environments.
How are artificial intelligence tools currently affecting teaching and learning? What are some of the most promising applications that have been developed so far? How are AI tools being used to personalize learning experiences – and what are the benefits and drawbacks of that approach? What’s next? These are some of the questions Nixon and Doroudi address in this episode of the UCI Podcast.
The music for this episode, titled “Computer Bounce,” was provided by Geographer via the audio library in YouTube Studio.
To get the latest episodes of the UCI Podcast delivered automatically, subscribe at:
Apple Podcasts – Spotify
Cara Capuano / The UCI Podcast:
From the University of California, Irvine, I’m Cara Capuano. Thank you for listening to The UCI Podcast. Today’s episode focuses on artificial intelligence and education, and we have a pair of guests willing to share their wisdom on this ever-changing topic. They’re from UC Irvine’s School of Education – Shayan Doroudi and Nia Nixon – both assistant professors.
Professor Doroudi runs the Mathe lab. Mathe is a Greek word for “learn,” but it’s also an acronym for Models, Analytics, Technologies, and Histories of Education. The lab has a particular focus on the multifaceted relationship between AI and education, including the historical connections between the two fields, which spans over 50 years.
Nixon heads the Language and Learning Analytics Lab – or LaLa Lab – which explores the intersections of technology with learning and education, with a particular focus on learning analytics, AI and collaborative engagement. Thank you both for joining us today.
Thank you for having us.
Shayan Doroudi:
Yeah, thank you for having us.
Let’s start our conversation with what makes you tick. What first drew your attention to AI and education?
So, for me, I was an undergrad at the University of Memphis, and I was exploring different research labs. So, I tried cognitive psychology and clinical psychology and then I got into what was called the Affective Computing Lab. And so, in that lab we did a lot of analysis and assessment of students’ emotions while they were learning. So, we would track their pupil movements, postural shifts, language, while they were engaging with intelligent tutoring systems. It was inherently a very AI-focused lab and that sort of birthed my interest in the field and all of its possibilities.
And what about you, Professor Doroudi?
Yeah, so, I didn’t start as early in my undergraduate career, but while I was an undergraduate student, I took a class online. It was a MOOC – massive open online course. So, there was one course that was about artificial intelligence, and it drew like 160,000 students or something. I was one of those many, many students. I liked the content of the course, but I also liked how the course was being delivered and the educational experience. I think that sort of seeded my interest, in some sense, in both AI and education.
I did an internship at Udacity, which was a company that put out that course. And at some point in that internship, I said, “I think I want to do this for my Ph.D. I want to study how to improve education with tools like AI and machine learning.” And so, that sort of started my experience.
And I didn’t know about intelligent tutoring systems – which Nia referred to – but when I actually started at my Ph.D. at Carnegie Mellon University, I realized, “Oh, people have been working on this for decades.” And then I learned about intelligent student tutoring systems and started working on them for my Ph.D. as well.
It’s nice for me to hear that you had “discovery moments” with the tools because they are ever- changing and, in the grand scheme of life, they’re still fairly new. So, it’s good to hear from who I see as seasoned experts in the field that you also had that new “ah ha!” moment and came to AI through kind of a genuine experience.
How are AI tools currently impacting teaching and learning, and what are some of the most promising applications that you’ve seen?
It’s interesting. If you asked me this like two years ago, I would’ve talked about certain tools, but I think probably most listeners are aware that things have changed a lot over the past year with ChatGPT and generative artificial intelligence. Now, there are so many new tools that are popping up, so many new ways that people are trying to use it. And one hope I have is that people don’t forget that people have been actually working on this before ChatGPT.
There’s lots of things that we mentioned – intelligent tutoring systems. These are platforms to help students learn sort of in an individualized or personalized way to guide them through problem solving. So, there’s more traditional ones of those and then now, with ChatGPT, people are trying to create these chatbots that can help tutor students. And I think we’ll get to this a little bit later – there are pros and cons of the different approaches, and there’s things to watch out for. But yeah, I think there’s a lot of interesting tools being developed currently.
I completely agree with Shayan. If you walk away with anything from this conversation, it’s that this isn’t a new field. Decades of research have been put into using AI in educational context. And a lot of those sort of fall into three super broad categories of assessment – using AI to assess education in different ways. Personalization – so, intelligent tutoring systems is a great example of that. And then educational delivery, content delivery. But that’s definitely been incredibly transformed in the last two years by all of the things that he was just discussing.
One of the most promising things? That’s a huge question, and it’s really hard for me to even begin to answer because I also know that this is being recorded. So, I think what I think is the most promising thing in this moment today versus tomorrow will probably be different.
But I will say that I think the conversational aspects of these newer models – and the social aspects in the context of education – are huge. And what we can do with that – the human-like engagement that we can do – it opens the door for a host of different possibilities.
Professor Nixon, you just talked about the personalization aspect, one of the ways that AI tools are being used. How do they personalize learning experiences for students? How can they do that?
Right, great question. Historically, we’ve been able to sort of map out a student’s knowledge of a particular subject and then provide them with – or expose them to – different levels of difficulty in content as they navigate through any educational platform. So that means you – as a novice – I might unfold things in a personalized way for you to not overwhelm you and not have you disengage or become frustrated.
Another way is dealing with emotion. So, as I mentioned earlier, I started out in an affective computing lab and one of the huge things that came out of that is emotions are important for learning, which is odd that that’s a new kind of thing – relatively new – but when you’re confused or frustrated, you’re more likely to disengage than you when you’re in flow and everything disappears and everything is at the right level for you.
So, AI can be used to go, “Hey, I think you look a little confused. Let me give you a hint. Oh, it looks like you might have a misconception. Let me correct that for you.” So, you don’t slip into these unproductive states of learning – affective states of learning. So, those are two examples. There are tons more of how AI can be used to kind of personalize the learning journey for students.
What are the benefits and the potential drawbacks of that kind of personalized approach?
One of the drawbacks is our kind of over-reliance on technology. I struggle with this thought because it feels antiquated in some way because I feel like if you look in history, there was pushback on writing things down when we first started writing things down. There was pushback on the printing press. And there’s pushback here because we’re saying, “Oh, we’re over relying on technology and AI and we’re outsourcing so much of our cognitive abilities to AI.” But also, we got past all of these other obstacles and those weren’t actually very accurate. So, there’s a tension there, when I say about that being a drawback.
I think one benefit is that teachers can’t necessarily give individualized attention to every student. So, if we are able to personalize experiences as well for individual students, they might be able to get sort of a unique experience that they wouldn’t otherwise be able to get in a large classroom.
At the same time, I don’t want to overemphasize that because I think there’s a lot of hype and a lot of companies will try to sell the products as doing this perfect kind of personalization, but we still haven’t figured it out really. And a good teacher can do certain things – or a good tutor can do certain things – that I don’t think we’ve been able to replicate with technology, with AI.
You know, we can personalize in certain ways, as Nia mentioned, but I think learning is very complex and this is something I’ve realized in my own research. I’ve tried to do some of this work, and I’ve realized it’s easier said than done, right? And so, learning is just very complex. And when you bring in the emotional pieces, the social pieces, like we don’t really know how to model all of that to know what’s the right thing to do.
And the technology’s limited by what it can do, whereas a teacher can say, “Okay, if this isn’t working, you know, let’s all just go outside. Let’s do something totally different.” And a teacher can come up with that on the spot. No AI tool that I know of is doing something like that.
With modern approaches now with these language-based tutors – these chatbots – they can seem like they can personalize very well, but they actually lack some of the rich understanding that Nia talked about earlier, like modeling exactly the kinds of knowledge that we want students to learn and knowing exactly what to do.
The way it’s approaching it is totally different. It’s doing it in a way that we don’t really – can’t really – predict what it’s going to do. And so, as researchers and educators, we don’t really know what it’s going to do. So, sometimes it’ll behave really well, and sometimes it might not – a lot of times it doesn’t actually. So, that’s one of the drawbacks to really be aware of.
You alluded earlier, Professor Doroudi, to some of the ethical considerations that go into integrating AI into education. What do those look like?
Yeah, I think there’s a number of ethical considerations. One is data from students and data privacy issues. I’m not an expert on that but I think, “Where’s that data going? Who has access to it?” Sometimes these are companies that make these tools. What do they do with that data? Are they selling it to people or to other companies? And so, I think there’s lots of considerations there.
And another one that I’ve been interested in – in my own work – is this issue of equity. And AI has a lot of biases that when we fit models to data that can be biased in many different ways. And these biases sometimes, you know, it’s not that someone’s not well-intentioned. Sometimes we have the best of intentions, but now we’re sort of seeding some of our authority to this tool that we’ve developed, but we don’t really know what it’s going to do in all cases.
So, it might behave differently with different students from different backgrounds. For example, with ChatGPT or these language-based AI tools, they’re trained on data. And their data might be more representative of certain kinds of students and not others, right? And then when interacting with students who might speak different dialects or just come from a different cultural background from whatever cultural backgrounds were most representing that data, the AI might interact differently with those students in ways that we might not even expect ahead of time.
We’ve talked about some of the concerns that arise when we implement AI in the learning environment. Are there any that haven’t been mentioned yet?
When we think about what these systems could look like in a couple of years, they’re going to move from just focusing on cognitive development – or primarily cognitive development – to becoming multimodal sensing agents. And by that, I mean we can start to have rooms that can track your facial expressions when you move in and out of them and track different physical shifts as well as your language and discourse and use all of that for good, in one instance, where we’re saying, “Oh, we can track when a student is stressed out, or different social developmental things that would be helpful.”
I think another concern there is a different type of privacy that I don’t hear talked about a lot – beyond just the data privacy – but maybe we could call it like emotional privacy of students and sort of what we expect to be our internal states of being and being kind of exposed by these AI systems. And so, I think that that’s an interesting one – one I’m still percolating on. I don’t know how to best discuss it just yet, but I think that it will become, um, a topic of conversation moving forward.
Yeah, there’s a lot of concern that these tools are going to be used for surveillance, right, and for ill intentions, right? Like, it might sound like, “Oh, this is great. We’re able to track all of these things. We have all these sensors in the classroom,” and it’s like, “Well, what are you doing with it?”
And as we’ve seen with, for example, like during the pandemic, a lot of universities and high schools, they were using these proctoring software and they would be using video data to see is the student doing something – misbehaving. At the beginning, they used some facial recognition software and sometimes some software wouldn’t detect students with darker skin tones and so there’s issues like that. And then sometimes the student might be doing something – maybe they have a tic or something – and the software would flag them as cheating, right? So, it’s like surveillance that really has negative repercussions, again, due to biases that I mentioned earlier.
With this increasing reliance on AI and education, how do we ensure equitable access to these technologies for all students, regardless of their socioeconomic background or perhaps their geographical location?
I think that kind of a task for policymakers, right? Prioritizing projects that are aimed at contributing to that – I think it’s a huge one. And – to some of Shayan’s concerns as well – we need policies in place to both protect students and ensure access to these things. And that’s kind of two sides of the same coin, right? We want you to have it and we want to protect you from it as well.
Looking ahead, what do you envision as the next breakthrough for the use of AI in education?
Forefront in my mind is something that I’ve been very fascinated by for the last couple of years – and that we actually have a collaboration going on around – is this idea of… well, I also want to give a shout out to an article called “Machines as Teammates” – it’s got like 12 authors. It’s a conceptual piece all around “what does it look like when we stop using AI as a tool?”
So, like Alexa or Siri, like, “Hey, do this for me, put this on my shopping list.” And it becomes something akin to you and I speaking right now. We treat it very much like another human. We engage with it, we help it, it helps us, we navigate and solve problems together in teams.
And so, I think – to your question – I think the next kind of big breakthrough, or one of the next big breakthroughs that we are working on, is imagining, or starting to study, AI as teammates. So, AI not as a virtual agent or a virtual tutor, but AI is another peer student trying to solve the problem alongside you with all the same and different, perhaps unique emotions and cognitions and things. So, I think that that will be interesting to see.
I’m always wary to make predictions because it’s so hard, you know, I wouldn’t have predicted this sort of boom in interest in AI and education that came about when ChatGPT got released. But I think one prediction that I might make is that the future of AI in education is going to be bespoke.
By that, I mean that we’re not going to see like one killer app that everyone’s going to be using and it’s going to be used in all schools. That’s never really happened in the history of educational technology. So many people have talked about the promise of a particular application or a particular software or tool, and for a while there was a lot of interest in that, and then it sort of died out for various reasons.
But I think what we see now happening is that sort of the AI is being put in the hands of people who previously couldn’t create their own tools. Now they can sort of try to create tools with the AI, right? Through things like prompt engineering, you can prompt the AI to behave in a certain way. And as I mentioned – as we’ve discussed earlier – this has a lot of limitations. You can’t always expect it to behave in the way you expect, but now teachers can create a custom application for their classroom that was not really possible before. Or school districts can come up with custom applications with AI that, again, wasn’t really possible before – you know, a company had to develop something that many school districts would adopt.
So, I think we’re going to see a lot of these sort of custom tools being built by individual educators, by districts and various stakeholders, right? By students themselves, right? Students can create AI tools – that itself is an educational experience. So, I think we’re going to this sort of proliferation of different tools that we don’t even know, you know… as researchers, we won’t even know what’s being developed. But some of them will be working really well in some cases. Some of them might not. And then, hopefully they’ll move on and try something different.
What steps can educators and policymakers take to kind of prepare for whatever the next wave is? I mean, ChatGPT came in like a tsunami and washed over all of us and was a gigantic “wow!” And not knowing what’s next, is there anything that educators and policymakers can do to get ready for that?
Yeah, that’s a tough one. I part of getting ready for the next step is really understanding what’s going on with what AI really is, how these tools work. And I think that speaks to AI literacy. You know, we talk about this for students, often. This has been a growing area: that students need to be literate about AI tools because they’re common society. So many jobs are now requiring them, otherwise they might displace people’s jobs – you know, a lot of the rhetoric that exists out there.
But I think teachers also need to be AI literate. They have to understand what these tools are, how they work, when they don’t work. And part of that AI literacy, I think, could be the more you have of it – if a new tool comes about, you can more quickly get up to speed with that, right? Rather than going from scratch to like, “Oh, I have to understand what this tool is entirely.”
So, if we work – you know, policymakers, researchers and educators – if we work together to increase efforts in AI literacy, both for students and for teachers and administrators, all the stakeholders, then I think people will have some familiarity with what these tools are and how they work. Just like people, hopefully, teachers already have familiarity with computers, the internet, these tools. So, if new things come about, they can adapt to these things.
But with AI because it’s sort of a little bit more foreign and people don’t have a good sense of what’s happening behind the scenes, I think there needs to be more work developing that. And that’s one thing we’re doing right now in my lab with a Ph.D. student of mine: we’re actually trying to survey undergraduate students to see how much literacy they have about these new generative AI tools and what some common misconceptions might be. So that’s the first step, understanding what people already know and what they don’t know, and then working to address those barriers and challenges.
I couldn’t agree more. So, policymakers can support efforts for AI literacy. One of my classes I teach is called “21st Century Literacies.” And in that class, we cover collaboration, communication, creativity – all of these things that have become increasingly important in the 21st century – not that they weren’t important before – but as we’ve moved from an industrial, individualized sort to more collectivist working environments, collaborative working environments, I think AI literacy is just as, if not more important, than all of those. And I’ve started to integrate that into the classroom because it’s so critical for students and teachers to have some type of foundation to navigate because I feel like a lot of the flailing that you might kind of see right now in education and AI is a lack of education around AI and/or misinformation around it. And so, addressing some of those is going be great moving forward.
Is there anything that either of you wanted to share that I didn’t ask about that you thought, “This is something I want to make sure I bring to this conversation and share with the audience?”
Maybe a closing point is there’s been a lot of discussion around the pros and cons of AI and education and some people just trying to shut it down initially, or shut down the most recent wave, completely remove it from the classroom. And I don’t think that that is a realistic approach or a helpful approach. I think this ties nicely into the AI literacy where this is not a switch that we can turn off. We are here, for better or for worse. And I think doing rigorous research around a lot of the topics that we discussed today is how we move forward, combined with educating students and teachers, and learning how to use this to our benefit. Instead of being fearful of it.
One thing I’d like to add is we’ve been talking so far about AI tools, right? And AI – for practical purposes – how it’s going to be used, for better or for worse, in classrooms. But one focus of my research has been AI not only as sort of this practical tool, but as this lens to understand human intelligence and ourselves as people. And that was actually really the quest for developing AI in the early days – was really focused on developing tools that that could help us understand how the mind works from a cognitive science perspective. And so, I think that’s sort of been … I wouldn’t say completely forgotten. There are still people thinking about that, but I think it’s been largely abandoned because AI has become so powerful as a tool that people just focus on it as like, “What can we do with it?”
And the AIs that we’ve developed have looked very different from people. So, I think because of that, people have just sort of moved away from that. But I think thinking about how AI can help us understand ourselves better, and this has a lot of educational implications. A lot of those early researchers were interested in, “Well, how can we understand how people learn and then use that to improve education?” And I think there’s a lot of opportunities there. With some of the new tools, for example, a lot of people talk about how, “Oh, these tools are amazing! They seem to show aspects of intelligence, but they also have these weird behaviors that are very not human-like.” So, by reflecting on these tools – by reflecting on things like ChatGPT – we can think about, “What does that tell us about ourselves as people?”
And how can students engage in experiences with these AIs to understand what makes us distinctly human in a sense? And one project we’re trying to get started on this, I’m collaborating with UCI philosophy professor Duncan Pritchard – who was actually a previous guest on this podcast – and we’re thinking about how what AI can tell us about intellectual virtues and how children interacting, or youth interacting, with AI can learn more about the importance of intellectual virtues, which AI, I would say, does not have.
Yes, there’s a whole “Anteater Virtues” that Professor Pritchard is in charge of. Thank you both so much for joining us today to share your in-depth knowledge about AI and education.
I’m Cara Capuano. Thank you for listening to our conversation. For the latest UCI News, please visit news.uci.edu. The UCI Podcast is a production of Strategic Communications and Public Affairs at the University of California, Irvine. Please subscribe wherever you listen to podcasts.
- Research article
- Open access
- Published: 24 April 2023
Artificial intelligence in higher education: the state of the field
- Helen Crompton ORCID: orcid.org/0000-0002-1775-8219 1 , 3 &
- Diane Burke 2
International Journal of Educational Technology in Higher Education volume 20 , Article number: 22 ( 2023 ) Cite this article
77k Accesses
67 Citations
59 Altmetric
Metrics details
This systematic review provides unique findings with an up-to-date examination of artificial intelligence (AI) in higher education (HE) from 2016 to 2022. Using PRISMA principles and protocol, 138 articles were identified for a full examination. Using a priori, and grounded coding, the data from the 138 articles were extracted, analyzed, and coded. The findings of this study show that in 2021 and 2022, publications rose nearly two to three times the number of previous years. With this rapid rise in the number of AIEd HE publications, new trends have emerged. The findings show that research was conducted in six of the seven continents of the world. The trend has shifted from the US to China leading in the number of publications. Another new trend is in the researcher affiliation as prior studies showed a lack of researchers from departments of education. This has now changed to be the most dominant department. Undergraduate students were the most studied students at 72%. Similar to the findings of other studies, language learning was the most common subject domain. This included writing, reading, and vocabulary acquisition. In examination of who the AIEd was intended for 72% of the studies focused on students, 17% instructors, and 11% managers. In answering the overarching question of how AIEd was used in HE, grounded coding was used. Five usage codes emerged from the data: (1) Assessment/Evaluation, (2) Predicting, (3) AI Assistant, (4) Intelligent Tutoring System (ITS), and (5) Managing Student Learning. This systematic review revealed gaps in the literature to be used as a springboard for future researchers, including new tools, such as Chat GPT.
A systematic review examining AIEd in higher education (HE) up to the end of 2022.
Unique findings in the switch from US to China in the most studies published.
A two to threefold increase in studies published in 2021 and 2022 to prior years.
AIEd was used for: Assessment/Evaluation, Predicting, AI Assistant, Intelligent Tutoring System, and Managing Student Learning.
Introduction
The use of artificial intelligence (AI) in higher education (HE) has risen quickly in the last 5 years (Chu et al., 2022 ), with a concomitant proliferation of new AI tools available. Scholars (viz., Chen et al., 2020 ; Crompton et al., 2020 , 2021 ) report on the affordances of AI to both instructors and students in HE. These benefits include the use of AI in HE to adapt instruction to the needs of different types of learners (Verdú et al., 2017 ), in providing customized prompt feedback (Dever et al., 2020 ), in developing assessments (Baykasoğlu et al., 2018 ), and predict academic success (Çağataylı & Çelebi, 2022 ). These studies help to inform educators about how artificial intelligence in education (AIEd) can be used in higher education.
Nonetheless, a gap has been highlighted by scholars (viz., Hrastinski et al., 2019 ; Zawacki-Richter et al., 2019 ) regarding an understanding of the collective affordances provided through the use of AI in HE. Therefore, the purpose of this study is to examine extant research from 2016 to 2022 to provide an up-to-date systematic review of how AI is being used in the HE context.
Artificial intelligence has become pervasive in the lives of twenty-first century citizens and is being proclaimed as a tool that can be used to enhance and advance all sectors of our lives (Górriz et al., 2020 ). The application of AI has attracted great interest in HE which is highly influenced by the development of information and communication technologies (Alajmi et al., 2020 ). AI is a tool used across subject disciplines, including language education (Liang et al., 2021 ), engineering education (Shukla et al., 2019 ), mathematics education (Hwang & Tu, 2021 ) and medical education (Winkler-Schwartz et al., 2019 ),
Artificial intelligence
The term artificial intelligence is not new. It was coined in 1956 by McCarthy (Cristianini, 2016 ) who followed up on the work of Turing (e.g., Turing, 1937 , 1950 ). Turing described the existence of intelligent reasoning and thinking that could go into intelligent machines. The definition of AI has grown and changed since 1956, as there has been significant advancements in AI capabilities. A current definition of AI is “computing systems that are able to engage in human-like processes such as learning, adapting, synthesizing, self-correction and the use of data for complex processing tasks” (Popenici et al., 2017 , p. 2). The interdisciplinary interest from scholars from linguistics, psychology, education, and neuroscience who connect AI to nomenclature, perceptions and knowledge in their own disciplines could create a challenge when defining AI. This has created the need to create categories of AI within specific disciplinary areas. This paper focuses on the category of AI in Education (AIEd) and how AI is specifically used in higher educational contexts.
As the field of AIEd is growing and changing rapidly, there is a need to increase the academic understanding of AIEd. Scholars (viz., Hrastinski et al., 2019 ; Zawacki-Richter et al., 2019 ) have drawn attention to the need to increase the understanding of the power of AIEd in educational contexts. The following section provides a summary of the previous research regarding AIEd.
Extant systematic reviews
This growing interest in AIEd has led scholars to investigate the research on the use of artificial intelligence in education. Some scholars have conducted systematic reviews to focus on a specific subject domain. For example, Liang et. al. ( 2021 ) conducted a systematic review and bibliographic analysis the roles and research foci of AI in language education. Shukla et. al. ( 2019 ) focused their longitudinal bibliometric analysis on 30 years of using AI in Engineering. Hwang and Tu ( 2021 ) conducted a bibliometric mapping analysis on the roles and trends in the use of AI in mathematics education, and Winkler-Schwartz et. al. ( 2019 ) specifically examined the use of AI in medical education in looking for best practices in the use of machine learning to assess surgical expertise. These studies provide a specific focus on the use of AIEd in HE but do not provide an understanding of AI across HE.
On a broader view of AIEd in HE, Ouyang et. al. ( 2022 ) conducted a systematic review of AIEd in online higher education and investigated the literature regarding the use of AI from 2011 to 2020. The findings show that performance prediction, resource recommendation, automatic assessment, and improvement of learning experiences are the four main functions of AI applications in online higher education. Salas-Pilco and Yang ( 2022 ) focused on AI applications in Latin American higher education. The results revealed that the main AI applications in higher education in Latin America are: (1) predictive modeling, (2) intelligent analytics, (3) assistive technology, (4) automatic content analysis, and (5) image analytics. These studies provide valuable information for the online and Latin American context but not an overarching examination of AIEd in HE.
Studies have been conducted to examine HE. Hinojo-Lucena et. al. ( 2019 ) conducted a bibliometric study on the impact of AIEd in HE. They analyzed the scientific production of AIEd HE publications indexed in Web of Science and Scopus databases from 2007 to 2017. This study revealed that most of the published document types were proceedings papers. The United States had the highest number of publications, and the most cited articles were about implementing virtual tutoring to improve learning. Chu et. al. ( 2022 ) reviewed the top 50 most cited articles on AI in HE from 1996 to 2020, revealing that predictions of students’ learning status were most frequently discussed. AI technology was most frequently applied in engineering courses, and AI technologies most often had a role in profiling and prediction. Finally, Zawacki-Richter et. al. ( 2019 ) analyzed AIEd in HE from 2007 to 2018 to reveal four primary uses of AIEd: (1) profiling and prediction, (2) assessment and evaluation, (3) adaptive systems and personalization, and (4) intelligent tutoring systems. There do not appear to be any studies examining the last 2 years of AIEd in HE, and these authors describe the rapid speed of both AI development and the use of AIEd in HE and call for further research in this area.
Purpose of the study
The purpose of this study is in response to the appeal from scholars (viz., Chu et al., 2022 ; Hinojo-Lucena et al., 2019 ; Zawacki-Richter et al., 2019 ) to research to investigate the benefits and challenges of AIEd within HE settings. As the academic knowledge of AIEd HE finished with studies examining up to 2020, this study provides the most up-to-date analysis examining research through to the end of 2022.
The overarching question for this study is: what are the trends in HE research regarding the use of AIEd? The first two questions provide contextual information, such as where the studies occurred and the disciplines AI was used in. These contextual details are important for presenting the main findings of the third question of how AI is being used in HE.
In what geographical location was the AIEd research conducted, and how has the trend in the number of publications evolved across the years?
What departments were the first authors affiliated with, and what were the academic levels and subject domains in which AIEd research was being conducted?
Who are the intended users of the AI technologies and what are the applications of AI in higher education?
A PRISMA systematic review methodology was used to answer three questions guiding this study. PRISMA principles (Page et al., 2021 ) were used throughout the study. The PRISMA extension Preferred Reporting Items for Systematic Reviews and Meta-Analysis for Protocols (PRISMA-P; Moher et al., 2015 ) were utilized in this study to provide an a priori roadmap to conduct a rigorous systematic review. Furthermore, the Preferred Reporting Items for Systematic Reviews and Meta-Analysis (PRISMA principles; Page et al., 2021 ) were used to search, identify, and select articles to be included in the research were used for searching, identifying, and selecting articles, then in how to read, extract, and manage the secondary data gathered from those studies (Moher et al., 2015 , PRISMA Statement, 2021 ). This systematic review approach supports an unbiased synthesis of the data in an impartial way (Hemingway & Brereton, 2009 ). Within the systematic review methodology, extracted data were aggregated and presented as whole numbers and percentages. A qualitative deductive and inductive coding methodology was also used to analyze extant data and generate new theories on the use of AI in HE (Gough et al., 2017 ).
The research begins with the search for the research articles to be included in the study. Based on the research question, the study parameters are defined including the search years, quality and types of publications to be included. Next, databases and journals are selected. A Boolean search is created and used for the search of those databases and journals. Once a set of publications are located from those searches, they are then examined against an inclusion and exclusion criteria to determine which studies will be included in the final study. The relevant data to match the research questions is then extracted from the final set of studies and coded. This method section is organized to describe each of these methods with full details to ensure transparency.
Search strategy
Only peer-reviewed journal articles were selected for examination in this systematic review. This ensured a level of confidence in the quality of the studies selected (Gough et al., 2017 ). The search parameters narrowed the search focus to include studies published in 2016 to 2022. This timeframe was selected to ensure the research was up to date, which is especially important with the rapid change in technology and AIEd.
The data retrieval protocol employed an electronic and a hand search. The electronic search included educational databases within EBSCOhost. Then an additional electronic search was conducted of Wiley Online Library, JSTOR, Science Direct, and Web of Science. Within each of these databases a full text search was conducted. Aligned to the research topic and questions, the Boolean search included terms related to AI, higher education, and learning. The Boolean search is listed in Table 1 . In the initial test search, the terms “machine learning” OR “intelligent support” OR “intelligent virtual reality” OR “chatbot” OR “automated tutor” OR “intelligent agent” OR “expert system” OR “neural network” OR “natural language processing” were used. These were removed as they were subcategories of terms found in Part 1 of the search. Furthermore, inclusion of these specific AI terms resulted in a large number of computer science courses that were focused on learning about AI and not the use of AI in learning.
Part 2 of the search ensured that articles involved formal university education. The terms higher education and tertiary were both used to recognize the different terms used in different countries. The final Boolean search was “Artificial intelligence” OR AI OR “smart technologies” OR “intelligent technologies” AND “higher education” OR tertiary OR graduate OR undergraduate. Scholars (viz., Ouyang et al., 2022 ) who conducted a systematic review on AIEd in HE up to 2020 noted that they missed relevant articles from their study, and other relevant journals should intentionally be examined. Therefore, a hand search was also conducted to include an examination of other journals relevant to AIEd that may not be included in the databases. This is important as the field of AIEd is still relatively new, and journals focused on this field may not yet be indexed in databases. The hand search included: The International Journal of Learning Analytics and Artificial Intelligence in Education, the International Journal of Artificial Intelligence in Education, and Computers & Education: Artificial Intelligence.
Electronic and hand searches resulted in 371 articles for possible inclusion. The search parameters within the electronic database search narrowed the search to articles published from 2016 to 2022, per-reviewed journal articles, and duplicates. Further screening was conducted manually, as each of the 138 articles were reviewed in full by two researchers to examine a match against the inclusion and exclusion criteria found in Table 2 .
The inter-rater reliability was calculated by percentage agreement (Belur et al., 2018 ). The researchers reached a 95% agreement for the coding. Further discussion of misaligned articles resulted in a 100% agreement. This screening process against inclusion and exclusion criteria resulted in the exclusion of 237 articles. This included the duplicates and those removed as part of the inclusion and exclusion criteria, see Fig. 1 . Leaving 138 articles for inclusion in this systematic review.
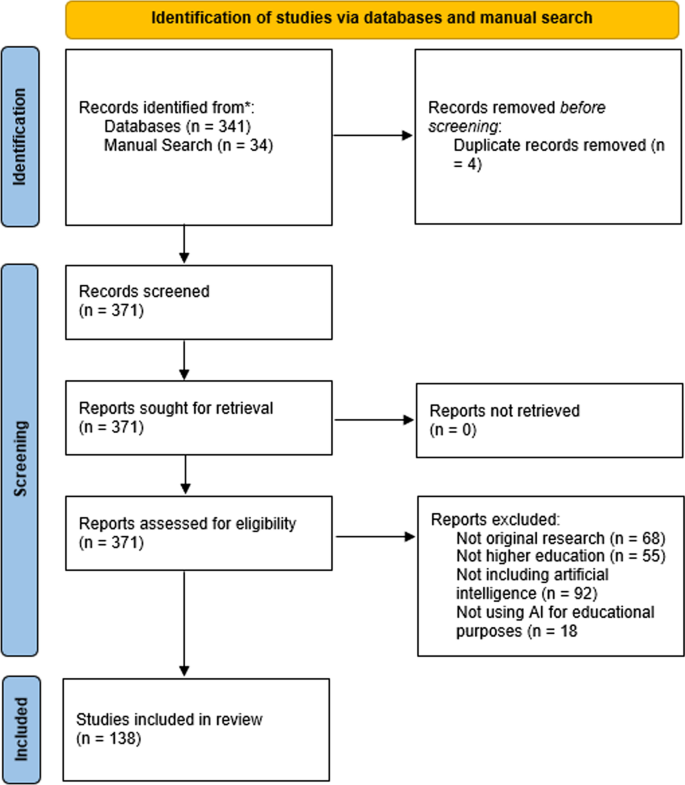
(From: Page et al., 2021 )
PRISMA flow chart of article identification and screening
The 138 articles were then coded to answer each of the research questions using deductive and inductive coding methods. Deductive coding involves examining data using a priori codes. A priori are pre-determined criteria and this process was used to code the countries, years, author affiliations, academic levels, and domains in the respective groups. Author affiliations were coded using the academic department of the first author of the study. First authors were chosen as that person is the primary researcher of the study and this follows past research practice (e.g., Zawacki-Richter et al., 2019 ). Who the AI was intended for was also coded using the a priori codes of Student, Instructor, Manager or Others. The Manager code was used for those who are involved in organizational tasks, e.g., tracking enrollment. Others was used for those not fitting the other three categories.
Inductive coding was used for the overarching question of this study in examining how the AI was being used in HE. Researchers of extant systematic reviews on AIEd in HE (viz., Chu et al., 2022 ; Zawacki-Richter et al., 2019 ) often used an a priori framework as researchers matched the use of AI to pre-existing frameworks. A grounded coding methodology (Strauss & Corbin, 1995 ) was selected for this study to allow findings of the trends on AIEd in HE to emerge from the data. This is important as it allows a direct understanding of how AI is being used rather than how researchers may think it is being used and fitting the data to pre-existing ideas.
Grounded coding process involved extracting how the AI was being used in HE from the articles. “In vivo” (Saldana, 2015 ) coding was also used alongside grounded coding. In vivo codes are when codes use language directly from the article to capture the primary authors’ language and ensure consistency with their findings. The grounded coding design used a constant comparative method. Researchers identified important text from articles related to the use of AI, and through an iterative process, initial codes led to axial codes with a constant comparison of uses of AI with uses of AI, then of uses of AI with codes, and codes with codes. Codes were deemed theoretically saturated when the majority of the data fit with one of the codes. For both the a priori and the grounded coding, two researchers coded and reached an inter-rater percentage agreement of 96%. After discussing misaligned articles, a 100% agreement was achieved.
Findings and discussion
The findings and discussion section are organized by the three questions guiding this study. The first two questions provide contextual information on the AIEd research, and the final question provides a rigorous investigation into how AI is being used in HE.
RQ1. In what geographical location was the AIEd research conducted, and how has the trend in the number of publications evolved across the years?
The 138 studies took place across 31 countries in six of seven continents of the world. Nonetheless, that distribution was not equal across continents. Asia had the largest number of AIEd studies in HE at 41%. Of the seven countries represented in Asia, 42 of the 58 studies were conducted in Taiwan and China. Europe, at 30%, was the second largest continent and had 15 countries ranging from one to eight studies a piece. North America, at 21% of the studies was the continent with the third largest number of studies, with the USA producing 21 of the 29 studies in that continent. The 21 studies from the USA places it second behind China. Only 1% of studies were conducted in South America and 2% in Africa. See Fig. 2 for a visual representation of study distribution across countries. Those continents with high numbers of studies are from high income countries and those with low numbers have a paucity of publications in low-income countries.
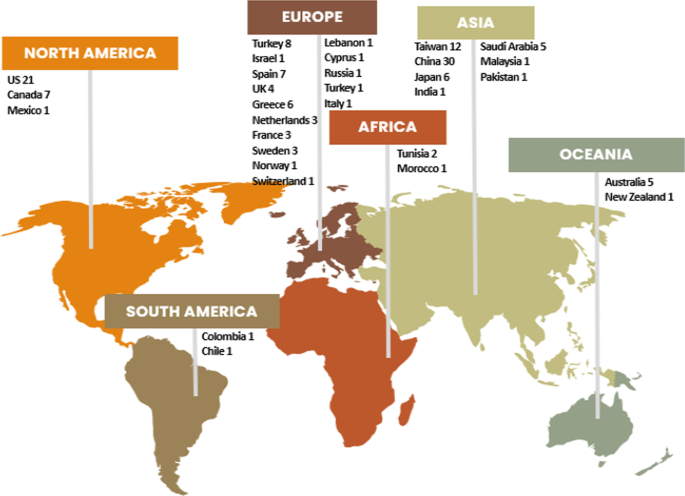
Geographical distribution of the AIEd HE studies
Data from Zawacki-Richter et. al.’s ( 2019 ) 2007–2018 systematic review examining countries found that the USA conducted the most studies across the globe at 43 out of 146, and China had the second largest at eleven of the 146 papers. Researchers have noted a rapid trend in Chinese researchers publishing more papers on AI and securing more patents than their US counterparts in a field that was originally led by the US (viz., Li et al., 2021 ). The data from this study corroborate this trend in China leading in the number of AIEd publications.
With the accelerated use of AI in society, gathering data to examine the use of AIEd in HE is useful in providing the scholarly community with specific information on that growth and if it is as prolific as anticipated by scholars (e.g., Chu et al., 2022 ). The analysis of data of the 138 studies shows that the trend towards the use of AIEd in HE has greatly increased. There is a drop in 2019, but then a great rise in 2021 and 2022; see Fig. 3 .
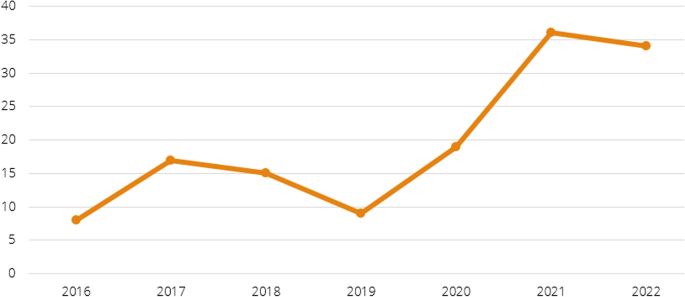
Chronological trend in AIEd in HE
Data on the rise in AIEd in HE is similar to the findings of Chu et. al. ( 2022 ) who noted an increase from 1996 to 2010 and 2011–2020. Nonetheless Chu’s parameters are across decades, and the rise is to be anticipated with a relatively new technology across a longitudinal review. Data from this study show a dramatic rise since 2020 with a 150% increase from the prior 2 years 2020–2019. The rise in 2021 and 2022 in HE could have been caused by the vast increase in HE faculty having to teach with technology during the pandemic lockdown. Faculty worldwide were using technologies, including AI, to explore how they could continue teaching and learning that was often face-to-face prior to lockdown. The disadvantage of this rapid adoption of technology is that there was little time to explore the possibilities of AI to transform learning, and AI may have been used to replicate past teaching practices, without considering new strategies previously inconceivable with the affordances of AI.
However, in a further examination of the research from 2021 to 2022, it appears that there are new strategies being considered. For example, Liu et. al.’s, 2022 study used AIEd to provide information on students’ interactions in an online environment and examine their cognitive effort. In Yao’s study in 2022, he examined the use of AI to determine student emotions while learning.
RQ2. What departments were the first authors affiliated with, and what were the academic levels and subject domains in which AIEd research was being conducted?
Department affiliations
Data from the AIEd HE studies show that of the first authors were most frequently from colleges of education (28%), followed by computer science (20%). Figure 4 presents the 15 academic affiliations of the authors found in the studies. The wide variety of affiliations demonstrate the variety of ways AI can be used in various educational disciplines, and how faculty in diverse areas, including tourism, music, and public affairs were interested in how AI can be used for educational purposes.
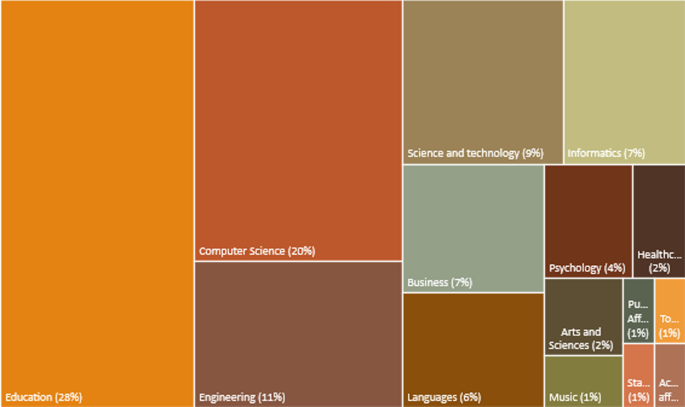
Research affiliations
In an extant AIED HE systematic review, Zawacki-Richter et. al.’s ( 2019 ) named their study Systematic review of research on artificial intelligence applications in higher education—where are the educators? In this study, the authors were keen to highlight that of the AIEd studies in HE, only six percent were written by researchers directly connected to the field of education, (i.e., from a college of education). The researchers found a great lack in pedagogical and ethical implications of implementing AI in HE and that there was a need for more educational perspectives on AI developments from educators conducting this work. It appears from our data that educators are now showing greater interest in leading these research endeavors, with the highest affiliated group belonging to education. This may again be due to the pandemic and those in the field of education needing to support faculty in other disciplines, and/or that they themselves needed to explore technologies for their own teaching during the lockdown. This may also be due to uptake in professors in education becoming familiar with AI tools also driven by a societal increased attention. As the focus of much research by education faculty is on teaching and learning, they are in an important position to be able to share their research with faculty in other disciplines regarding the potential affordances of AIEd.
Academic levels
The a priori coding of academic levels show that the majority of studies involved undergraduate students with 99 of the 138 (72%) focused on these students. This was in comparison to the 12 of 138 (9%) for graduate students. Some of the studies used AI for both academic levels: see Fig. 5
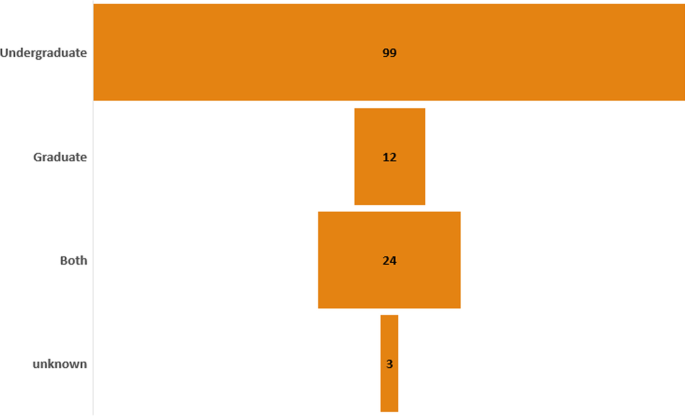
Academic level distribution by number of articles
This high percentage of studies focused on the undergraduate population was congruent with an earlier AIED HE systematic review (viz., Zawacki-Richter et al., 2019 ) who also reported student academic levels. This focus on undergraduate students may be due to the variety of affordances offered by AIEd, such as predictive analytics on dropouts and academic performance. These uses of AI may be less required for graduate students who already have a record of performance from their undergraduate years. Another reason for this demographic focus can also be convenience sampling, as researchers in HE typically has a much larger and accessible undergraduate population than graduates. This disparity between undergraduates and graduate populations is a concern, as AIEd has the potential to be valuable in both settings.
Subject domains
The studies were coded into 14 areas in HE; with 13 in a subject domain and one category of AIEd used in HE management of students; See Fig. 6 . There is not a wide difference in the percentages of top subject domains, with language learning at 17%, computer science at 16%, and engineering at 12%. The management of students category appeared third on the list at 14%. Prior studies have also found AIEd often used for language learning (viz., Crompton et al., 2021 ; Zawacki-Richter et al., 2019 ). These results are different, however, from Chu et. al.’s ( 2022 ) findings that show engineering dramatically leading with 20 of the 50 studies, with other subjects, such as language learning, appearing once or twice. This study appears to be an outlier that while the searches were conducted in similar databases, the studies only included 50 studies from 1996 to 2020.
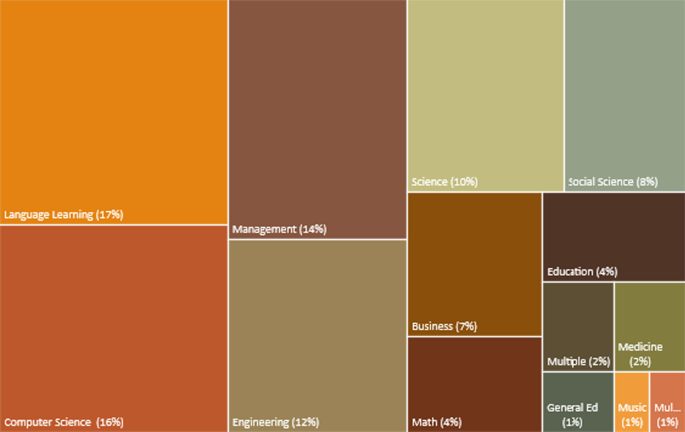
Subject domains of AIEd in HE
Previous scholars primarily focusing on language learning using AI for writing, reading, and vocabulary acquisition used the affordances of natural language processing and intelligent tutoring systems (e.g., Liang et al., 2021 ). This is similar to the findings in studies with AI used for automated feedback of writing in a foreign language (Ayse et al., 2022 ), and AI translation support (Al-Tuwayrish, 2016 ). The large use of AI for managerial activities in this systematic review focused on making predictions (12 studies) and then admissions (three studies). This is positive to see this use of AI to look across multiple databases to see trends emerging from data that may not have been anticipated and cross referenced before (Crompton et al., 2022 ). For example, to examine dropouts, researchers may consider examining class attendance, and may not examine other factors that appear unrelated. AI analysis can examine all factors and may find that dropping out is due to factors beyond class attendance.
RQ3. Who are the intended users of the AI technologies and what are the applications of AI in higher education?
Intended user of AI
Of the 138 articles, the a priori coding shows that 72% of the studies focused on Students, followed by a focus on Instructors at 17%, and Managers at 11%, see Fig. 7 . The studies provided examples of AI being used to provide support to students, such as access to learning materials for inclusive learning (Gupta & Chen, 2022 ), provide immediate answers to student questions, self-testing opportunities (Yao, 2022 ), and instant personalized feedback (Mousavi et al., 2020 ).
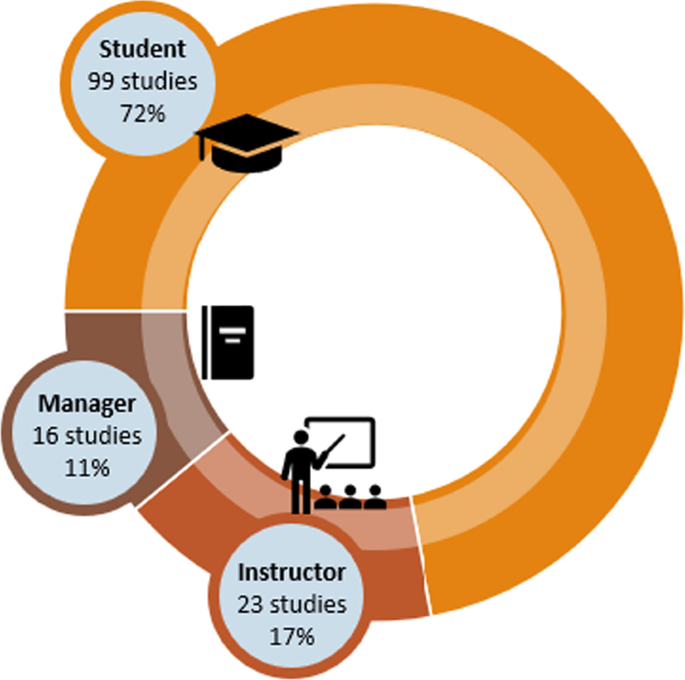
Intended user
The data revealed a large emphasis on students in the use of AIEd in HE. This user focus is different from a recent systematic review on AIEd in K-12 that found that AIEd studies in K-12 settings prioritized teachers (Crompton et al., 2022 ). This may appear that HE uses AI to focus more on students than in K-12. However, this large number of student studies in HE may be due to the student population being more easily accessibility to HE researchers who may study their own students. The ethical review process is also typically much shorter in HE than in K-12. Therefore, the data on the intended focus should be reviewed while keeping in mind these other explanations. It was interesting that Managers were the lowest focus in K-12 and also in this study in HE. AI has great potential to collect, cross reference and examine data across large datasets that can allow data to be used for actionable insight. More focus on the use of AI by managers would tap into this potential.
How is AI used in HE
Using grounded coding, the use of AIEd from each of the 138 articles was examined and six major codes emerged from the data. These codes provide insight into how AI was used in HE. The five codes are: (1) Assessment/Evaluation, (2) Predicting, (3) AI Assistant, (4) Intelligent Tutoring System (ITS), and (5) Managing Student Learning. For each of these codes there are also axial codes, which are secondary codes as subcategories from the main category. Each code is delineated below with a figure of the codes with further descriptive information and examples.
Assessment/evaluation
Assessment and Evaluation was the most common use of AIEd in HE. Within this code there were six axial codes broken down into further codes; see Fig. 8 . Automatic assessment was most common, seen in 26 of the studies. It was interesting to see that this involved assessment of academic achievement, but also other factors, such as affect.
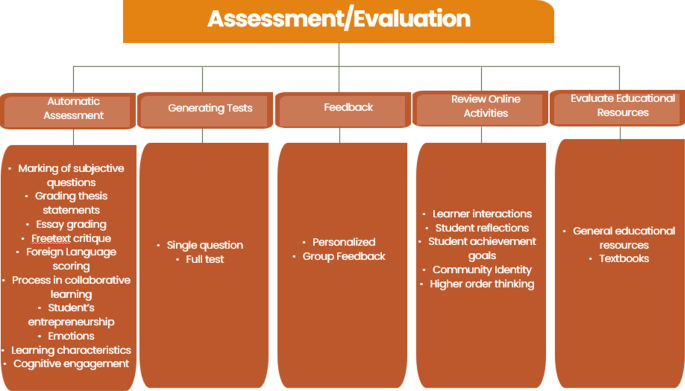
Codes and axial codes for assessment and evaluation
Automatic assessment was used to support a variety of learners in HE. As well as reducing the time it takes for instructors to grade (Rutner & Scott, 2022 ), automatic grading showed positive use for a variety of students with diverse needs. For example, Zhang and Xu ( 2022 ) used automatic assessment to improve academic writing skills of Uyghur ethnic minority students living in China. Writing has a variety of cultural nuances and in this study the students were shown to engage with the automatic assessment system behaviorally, cognitively, and affectively. This allowed the students to engage in self-regulated learning while improving their writing.
Feedback was a description often used in the studies, as students were given text and/or images as feedback as a formative evaluation. Mousavi et. al. ( 2020 ) developed a system to provide first year biology students with an automated personalized feedback system tailored to the students’ specific demographics, attributes, and academic status. With the unique feature of AIEd being able to analyze multiple data sets involving a variety of different students, AI was used to assess and provide feedback on students’ group work (viz., Ouatik et al., 2021 ).
AI also supports instructors in generating questions and creating multiple question tests (Yang et al., 2021 ). For example, (Lu et al., 2021 ) used natural language processing to create a system that automatically created tests. Following a Turing type test, researchers found that AI technologies can generate highly realistic short-answer questions. The ability for AI to develop multiple questions is a highly valuable affordance as tests can take a great deal of time to make. However, it would be important for instructors to always confirm questions provided by the AI to ensure they are correct and that they match the learning objectives for the class, especially in high value summative assessments.
The axial code within assessment and evaluation revealed that AI was used to review activities in the online space. This included evaluating student’s reflections, achievement goals, community identity, and higher order thinking (viz., Huang et al., 2021 ). Three studies used AIEd to evaluate educational materials. This included general resources and textbooks (viz., Koć‑Januchta et al., 2022 ). It is interesting to see the use of AI for the assessment of educational products, rather than educational artifacts developed by students. While this process may be very similar in nature, this shows researchers thinking beyond the traditional use of AI for assessment to provide other affordances.
Predicting was a common use of AIEd in HE with 21 studies focused specifically on the use of AI for forecasting trends in data. Ten axial codes emerged on the way AI was used to predict different topics, with nine focused on predictions regarding students and the other on predicting the future of higher education. See Fig. 9 .
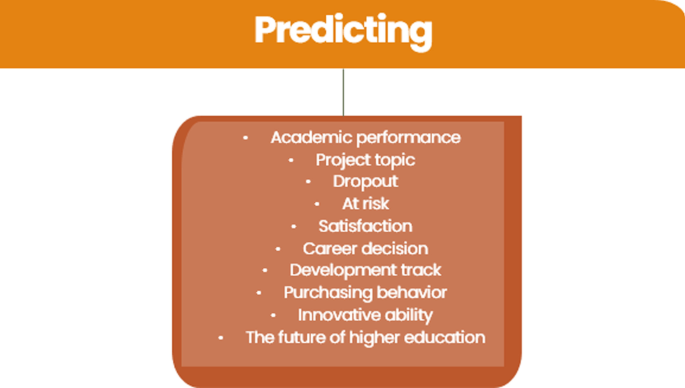
Predicting axial codes
Extant systematic reviews on HE highlighted the use of AIEd for prediction (viz., Chu et al., 2022 ; Hinojo-Lucena et al., 2019 ; Ouyang et al., 2022 ; Zawacki-Richter et al., 2019 ). Ten of the articles in this study used AI for predicting academic performance. Many of the axial codes were often overlapping, such as predicting at risk students, and predicting dropouts; however, each provided distinct affordances. An example of this is the study by Qian et. al. ( 2021 ). These researchers examined students taking a MOOC course. MOOCs can be challenging environments to determine information on individual students with the vast number of students taking the course (Krause & Lowe, 2014 ). However, Qian et al., used AIEd to predict students’ future grades by inputting 17 different learning features, including past grades, into an artificial neural network. The findings were able to predict students’ grades and highlight students at risk of dropping out of the course.
In a systematic review on AIEd within the K-12 context (viz., Crompton et al., 2022 ), prediction was less pronounced in the findings. In the K-12 setting, there was a brief mention of the use of AI in predicting student academic performance. One of the studies mentioned students at risk of dropping out, but this was immediately followed by questions about privacy concerns and describing this as “sensitive”. The use of prediction from the data in this HE systematic review cover a wide range of AI predictive affordances. students Sensitivity is still important in a HE setting, but it is positive to see the valuable insight it provides that can be used to avoid students failing in their goals.
AI assistant
The studies evaluated in this review indicated that the AI Assistant used to support learners had a variety of different names. This code included nomenclature such as, virtual assistant, virtual agent, intelligent agent, intelligent tutor, and intelligent helper. Crompton et. al. ( 2022 ), described the difference in the terms to delineate the way that the AI appeared to the user. For example, if there was an anthropomorphic presence to the AI, such as an avatar, or if the AI appeared to support via other means, such as text prompt. The findings of this systematic review align to Crompton et. al.’s ( 2022 ) descriptive differences of the AI Assistant. Furthermore, this code included studies that provide assistance to students, but may not have specifically used the word assistance. These include the use of chatbots for student outreach, answering questions, and providing other assistance. See Fig. 10 for the axial codes for AI Assistant.
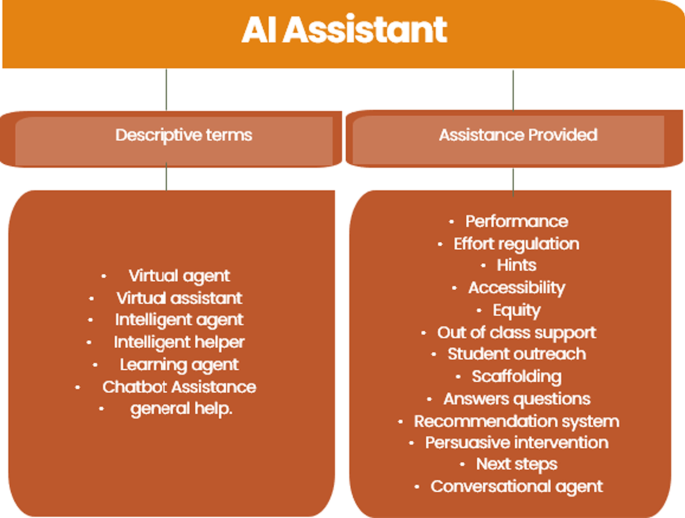
AI assistant axial codes
Many of these assistants offered multiple supports to students, such as Alex , the AI described as a virtual change agent in Kim and Bennekin’s ( 2016 ) study. Alex interacted with students in a college mathematics course by asking diagnostic questions and gave support depending on student needs. Alex’s support was organized into four stages: (1) goal initiation (“Want it”), (2) goal formation (“Plan for it”), (3) action control (“Do it”), and (4) emotion control (“Finish it”). Alex provided responses depending on which of these four areas students needed help. These messages supported students with the aim of encouraging persistence in pursuing their studies and degree programs and improving performance.
The role of AI in providing assistance connects back to the seminal work of Vygotsky ( 1978 ) and the Zone of Proximal Development (ZPD). ZPD highlights the degree to which students can rapidly develop when assisted. Vygotsky described this assistance often in the form of a person. However, with technological advancements, the use of AI assistants in these studies are providing that support for students. The affordances of AI can also ensure that the support is timely without waiting for a person to be available. Also, assistance can consider aspects on students’ academic ability, preferences, and best strategies for supporting. These features were evident in Kim and Bennekin’s ( 2016 ) study using Alex.
Intelligent tutoring system
The use of Intelligent Tutoring Systems (ITS) was revealed in the grounded coding. ITS systems are adaptive instructional systems that involve the use of AI techniques and educational methods. An ITS system customizes educational activities and strategies based on student’s characteristics and needs (Mousavinasab et al., 2021 ). While ITS may be an anticipated finding in AIED HE systematic reviews, it was interesting that extant reviews similar to this study did not always describe their use in HE. For example, Ouyang et. al. ( 2022 ), included “intelligent tutoring system” in search terms describing it as a common technique, yet ITS was not mentioned again in the paper. Zawacki-Richter et. al. ( 2019 ) on the other hand noted that ITS was in the four overarching findings of the use of AIEd in HE. Chu et. al. ( 2022 ) then used Zawacki-Richter’s four uses of AIEd for their recent systematic review.
In this systematic review, 18 studies specifically mentioned that they were using an ITS. The ITS code did not necessitate axial codes as they were performing the same type of function in HE, namely, in providing adaptive instruction to the students. For example, de Chiusole et. al. ( 2020 ) developed Stat-Knowlab, an ITS that provides the level of competence and best learning path for each student. Thus Stat-Knowlab personalizes students’ learning and provides only educational activities that the student is ready to learn. This ITS is able to monitor the evolution of the learning process as the student interacts with the system. In another study, Khalfallah and Slama ( 2018 ) built an ITS called LabTutor for engineering students. LabTutor served as an experienced instructor in enabling students to access and perform experiments on laboratory equipment while adapting to the profile of each student.
The student population in university classes can go into the hundreds and with the advent of MOOCS, class sizes can even go into the thousands. Even in small classes of 20 students, the instructor cannot physically provide immediate unique personalize questions to each student. Instructors need time to read and check answers and then take further time to provide feedback before determining what the next question should be. Working with the instructor, AIEd can provide that immediate instruction, guidance, feedback, and following questioning without delay or becoming tired. This appears to be an effective use of AIEd, especially within the HE context.
Managing student learning
Another code that emerged in the grounded coding was focused on the use of AI for managing student learning. AI is accessed to manage student learning by the administrator or instructor to provide information, organization, and data analysis. The axial codes reveal the trends in the use of AI in managing student learning; see Fig. 11 .
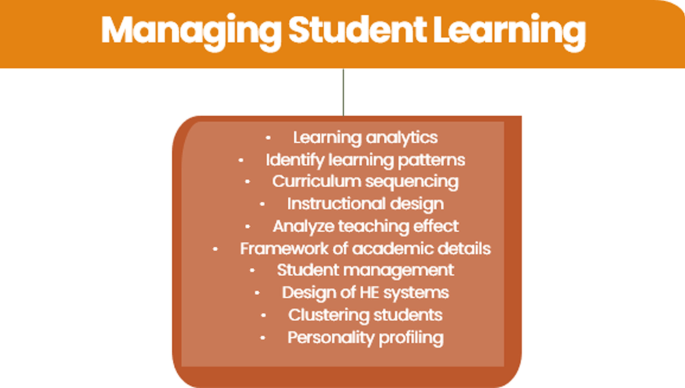
Learning analytics was an a priori term often found in studies which describes “the measurement, collection, analysis and reporting of data about learners and their contexts, for purposes of understanding and optimizing learning and the environments in which it occurs” (Long & Siemens, 2011 , p. 34). The studies investigated in this systematic review were across grades and subject areas and provided administrators and instructors different types of information to guide their work. One of those studies was conducted by Mavrikis et. al. ( 2019 ) who described learning analytics as teacher assistance tools. In their study, learning analytics were used in an exploratory learning environment with targeted visualizations supporting classroom orchestration. These visualizations, displayed as screenshots in the study, provided information such as the interactions between the students, goals achievements etc. These appear similar to infographics that are brightly colored and draw the eye quickly to pertinent information. AI is also used for other tasks, such as organizing the sequence of curriculum in pacing guides for future groups of students and also designing instruction. Zhang ( 2022 ) described how designing an AI teaching system of talent cultivation and using the digital affordances to establish a quality assurance system for practical teaching, provides new mechanisms for the design of university education systems. In developing such a system, Zhang found that the stability of the instructional design, overcame the drawbacks of traditional manual subjectivity in the instructional design.
Another trend that emerged from the studies was the use of AI to manage student big data to support learning. Ullah and Hafiz ( 2022 ) lament that using traditional methods, including non-AI digital techniques, asking the instructor to pay attention to every student’s learning progress is very difficult and that big data analysis techniques are needed. The ability to look across and within large data sets to inform instruction is a valuable affordance of AIEd in HE. While the use of AIEd to manage student learning emerged from the data, this study uncovered only 19 studies in 7 years (2016–2022) that focused on the use of AIEd to manage student data. This lack of the use was also noted in a recent study in the K-12 space (Crompton et al., 2022 ). In Chu et. al.’s ( 2022 ) study examining the top 50 most cited AIEd articles, they did not report the use of AIEd for managing student data in the top uses of AIEd HE. It would appear that more research should be conducted in this area to fully explore the possibilities of AI.
Gaps and future research
From this systematic review, six gaps emerged in the data providing opportunities for future studies to investigate and provide a fuller understanding of how AIEd can used in HE. (1) The majority of the research was conducted in high income countries revealing a paucity of research in developing countries. More research should be conducted in these developing countries to expand the level of understanding about how AI can enhance learning in under-resourced communities. (2) Almost 50% of the studies were conducted in the areas of language learning, computer science and engineering. Research conducted by members from multiple, different academic departments would help to advance the knowledge of the use of AI in more disciplines. (3) This study revealed that faculty affiliated with schools of education are taking an increasing role in researching the use of AIEd in HE. As this body of knowledge grows, faculty in Schools of Education should share their research regarding the pedagogical affordances of AI so that this knowledge can be applied by faculty across disciplines. (4) The vast majority of the research was conducted at the undergraduate level. More research needs to be done at the graduate student level, as AI provides many opportunities in this environment. (5) Little study was done regarding how AIEd can assist both instructors and managers in their roles in HE. The power of AI to assist both groups further research. (6) Finally, much of the research investigated in this systematic review revealed the use of AIEd in traditional ways that enhance or make more efficient current practices. More research needs to focus on the unexplored affordances of AIEd. As AI becomes more advanced and sophisticated, new opportunities will arise for AIEd. Researchers need to be on the forefront of these possible innovations.
In addition, empirical exploration is needed for new tools, such as ChatGPT that was available for public use at the end of 2022. With the time it takes for a peer review journal article to be published, ChatGPT did not appear in the articles for this study. What is interesting is that it could fit with a variety of the use codes found in this study, with students getting support in writing papers and instructors using Chat GPT to assess students work and with help writing emails or descriptions for students. It would be pertinent for researchers to explore Chat GPT.
Limitations
The findings of this study show a rapid increase in the number of AIEd studies published in HE. However, to ensure a level of credibility, this study only included peer review journal articles. These articles take months to publish. Therefore, conference proceedings and gray literature such as blogs and summaries may reveal further findings not explored in this study. In addition, the articles in this study were all published in English which excluded findings from research published in other languages.
In response to the call by Hinojo-Lucena et. al. ( 2019 ), Chu et. al. ( 2022 ), and Zawacki-Richter et. al. ( 2019 ), this study provides unique findings with an up-to-date examination of the use of AIEd in HE from 2016 to 2022. Past systematic reviews examined the research up to 2020. The findings of this study show that in 2021 and 2022, publications rose nearly two to three times the number of previous years. With this rapid rise in the number of AIEd HE publications, new trends have emerged.
The findings show that of the 138 studies examined, research was conducted in six of the seven continents of the world. In extant systematic reviews showed that the US led by a large margin in the number of studies published. This trend has now shifted to China. Another shift in AIEd HE is that while extant studies lamented the lack of focus on professors of education leading these studies, this systematic review found education to be the most common department affiliation with 28% and computer science coming in second at 20%. Undergraduate students were the most studied students at 72%. Similar to the findings of other studies, language learning was the most common subject domain. This included writing, reading, and vocabulary acquisition. In examination of who the AIEd was intended for, 72% of the studies focused on students, 17% instructors, and 11% managers.
Grounded coding was used to answer the overarching question of how AIEd was used in HE. Five usage codes emerged from the data: (1) Assessment/Evaluation, (2) Predicting, (3) AI Assistant, (4) Intelligent Tutoring System (ITS), and (5) Managing Student Learning. Assessment and evaluation had a wide variety of purposes, including assessing academic progress and student emotions towards learning, individual and group evaluations, and class based online community assessments. Predicting emerged as a code with ten axial codes, as AIEd predicted dropouts and at-risk students, innovative ability, and career decisions. AI Assistants were specific to supporting students in HE. These assistants included those with an anthropomorphic presence, such as virtual agents and persuasive intervention through digital programs. ITS systems were not always noted in extant systematic reviews but were specifically mentioned in 18 of the studies in this review. ITS systems in this study provided customized strategies and approaches to student’s characteristics and needs. The final code in this study highlighted the use of AI in managing student learning, including learning analytics, curriculum sequencing, instructional design, and clustering of students.
The findings of this study provide a springboard for future academics, practitioners, computer scientists, policymakers, and funders in understanding the state of the field in AIEd HE, how AI is used. It also provides actionable items to ameliorate gaps in the current understanding. As the use AIEd will only continue to grow this study can serve as a baseline for further research studies in the use of AIEd in HE.
Availability of data and materials
The datasets used and/or analysed during the current study are available from the corresponding author on reasonable request.
Alajmi, Q., Al-Sharafi, M. A., & Abuali, A. (2020). Smart learning gateways for Omani HEIs towards educational technology: Benefits, challenges and solutions. International Journal of Information Technology and Language Studies, 4 (1), 12–17.
Google Scholar
Al-Tuwayrish, R. K. (2016). An evaluative study of machine translation in the EFL scenario of Saudi Arabia. Advances in Language and Literary Studies, 7 (1), 5–10.
Ayse, T., & Nil, G. (2022). Automated feedback and teacher feedback: Writing achievement in learning English as a foreign language at a distance. The Turkish Online Journal of Distance Education, 23 (2), 120–139. https://doi.org/10.7575/aiac.alls.v.7n.1p.5
Article Google Scholar
Baykasoğlu, A., Özbel, B. K., Dudaklı, N., Subulan, K., & Şenol, M. E. (2018). Process mining based approach to performance evaluation in computer-aided examinations. Computer Applications in Engineering Education, 26 (5), 1841–1861. https://doi.org/10.1002/cae.21971
Belur, J., Tompson, L., Thornton, A., & Simon, M. (2018). Interrater reliability in systematic review methodology: Exploring variation in coder decision-making. Sociological Methods & Research, 13 (3), 004912411887999. https://doi.org/10.1177/0049124118799372
Çağataylı, M., & Çelebi, E. (2022). Estimating academic success in higher education using big five personality traits, a machine learning approach. Arab Journal Scientific Engineering, 47 , 1289–1298. https://doi.org/10.1007/s13369-021-05873-4
Chen, L., Chen, P., & Lin, Z. (2020). Artificial intelligence in education: A review. IEEE Access, 8 , 75264–75278. https://doi.org/10.1109/ACCESS.2020.2988510
Chu, H., Tu, Y., & Yang, K. (2022). Roles and research trends of artificial intelligence in higher education: A systematic review of the top 50 most-cited articles. Australasian Journal of Educational Technology, 38 (3), 22–42. https://doi.org/10.14742/ajet.7526
Cristianini, N. (2016). Intelligence reinvented. New Scientist, 232 (3097), 37–41. https://doi.org/10.1016/S0262-4079(16)31992-3
Crompton, H., Bernacki, M. L., & Greene, J. (2020). Psychological foundations of emerging technologies for teaching and learning in higher education. Current Opinion in Psychology, 36 , 101–105. https://doi.org/10.1016/j.copsyc.2020.04.011
Crompton, H., & Burke, D. (2022). Artificial intelligence in K-12 education. SN Social Sciences, 2 , 113. https://doi.org/10.1007/s43545-022-00425-5
Crompton, H., Jones, M., & Burke, D. (2022). Affordances and challenges of artificial intelligence in K-12 education: A systematic review. Journal of Research on Technology in Education . https://doi.org/10.1080/15391523.2022.2121344
Crompton, H., & Song, D. (2021). The potential of artificial intelligence in higher education. Revista Virtual Universidad Católica Del Norte, 62 , 1–4. https://doi.org/10.35575/rvuen.n62a1
de Chiusole, D., Stefanutti, L., Anselmi, P., & Robusto, E. (2020). Stat-Knowlab. Assessment and learning of statistics with competence-based knowledge space theory. International Journal of Artificial Intelligence in Education, 30 , 668–700. https://doi.org/10.1007/s40593-020-00223-1
Dever, D. A., Azevedo, R., Cloude, E. B., & Wiedbusch, M. (2020). The impact of autonomy and types of informational text presentations in game-based environments on learning: Converging multi-channel processes data and learning outcomes. International Journal of Artificial Intelligence in Education, 30 (4), 581–615. https://doi.org/10.1007/s40593-020-00215-1
Górriz, J. M., Ramírez, J., Ortíz, A., Martínez-Murcia, F. J., Segovia, F., Suckling, J., Leming, M., Zhang, Y. D., Álvarez-Sánchez, J. R., Bologna, G., Bonomini, P., Casado, F. E., Charte, D., Charte, F., Contreras, R., Cuesta-Infante, A., Duro, R. J., Fernández-Caballero, A., Fernández-Jover, E., … Ferrández, J. M. (2020). Artificial intelligence within the interplay between natural and artificial computation: Advances in data science, trends and applications. Neurocomputing, 410 , 237–270. https://doi.org/10.1016/j.neucom.2020.05.078
Gough, D., Oliver, S., & Thomas, J. (2017). An introduction to systematic reviews (2nd ed.). Sage.
Gupta, S., & Chen, Y. (2022). Supporting inclusive learning using chatbots? A chatbot-led interview study. Journal of Information Systems Education, 33 (1), 98–108.
Hemingway, P. & Brereton, N. (2009). In Hayward Medical Group (Ed.). What is a systematic review? Retrieved from http://www.medicine.ox.ac.uk/bandolier/painres/download/whatis/syst-review.pdf
Hinojo-Lucena, F., Arnaz-Diaz, I., Caceres-Reche, M., & Romero-Rodriguez, J. (2019). A bibliometric study on its impact the scientific literature. Education Science . https://doi.org/10.3390/educsci9010051
Hrastinski, S., Olofsson, A. D., Arkenback, C., Ekström, S., Ericsson, E., Fransson, G., Jaldemark, J., Ryberg, T., Öberg, L.-M., Fuentes, A., Gustafsson, U., Humble, N., Mozelius, P., Sundgren, M., & Utterberg, M. (2019). Critical imaginaries and reflections on artificial intelligence and robots in postdigital K-12 education. Postdigital Science and Education, 1 (2), 427–445. https://doi.org/10.1007/s42438-019-00046-x
Huang, C., Wu, X., Wang, X., He, T., Jiang, F., & Yu, J. (2021). Exploring the relationships between achievement goals, community identification and online collaborative reflection. Educational Technology & Society, 24 (3), 210–223.
Hwang, G. J., & Tu, Y. F. (2021). Roles and research trends of artificial intelligence in mathematics education: A bibliometric mapping analysis and systematic review. Mathematics, 9 (6), 584. https://doi.org/10.3390/math9060584
Khalfallah, J., & Slama, J. B. H. (2018). The effect of emotional analysis on the improvement of experimental e-learning systems. Computer Applications in Engineering Education, 27 (2), 303–318. https://doi.org/10.1002/cae.22075
Kim, C., & Bennekin, K. N. (2016). The effectiveness of volition support (VoS) in promoting students’ effort regulation and performance in an online mathematics course. Instructional Science, 44 , 359–377. https://doi.org/10.1007/s11251-015-9366-5
Koć-Januchta, M. M., Schönborn, K. J., Roehrig, C., Chaudhri, V. K., Tibell, L. A. E., & Heller, C. (2022). “Connecting concepts helps put main ideas together”: Cognitive load and usability in learning biology with an AI-enriched textbook. International Journal of Educational Technology in Higher Education, 19 (11), 11. https://doi.org/10.1186/s41239-021-00317-3
Krause, S. D., & Lowe, C. (2014). Invasion of the MOOCs: The promise and perils of massive open online courses . Parlor Press.
Li, D., Tong, T. W., & Xiao, Y. (2021). Is China emerging as the global leader in AI? Harvard Business Review. https://hbr.org/2021/02/is-china-emerging-as-the-global-leader-in-ai
Liang, J. C., Hwang, G. J., Chen, M. R. A., & Darmawansah, D. (2021). Roles and research foci of artificial intelligence in language education: An integrated bibliographic analysis and systematic review approach. Interactive Learning Environments . https://doi.org/10.1080/10494820.2021.1958348
Liu, S., Hu, T., Chai, H., Su, Z., & Peng, X. (2022). Learners’ interaction patterns in asynchronous online discussions: An integration of the social and cognitive interactions. British Journal of Educational Technology, 53 (1), 23–40. https://doi.org/10.1111/bjet.13147
Long, P., & Siemens, G. (2011). Penetrating the fog: Analytics in learning and education. Educause Review, 46 (5), 31–40.
Lu, O. H. T., Huang, A. Y. Q., Tsai, D. C. L., & Yang, S. J. H. (2021). Expert-authored and machine-generated short-answer questions for assessing students learning performance. Educational Technology & Society, 24 (3), 159–173.
Mavrikis, M., Geraniou, E., Santos, S. G., & Poulovassilis, A. (2019). Intelligent analysis and data visualization for teacher assistance tools: The case of exploratory learning. British Journal of Educational Technology, 50 (6), 2920–2942. https://doi.org/10.1111/bjet.12876
Moher, D., Shamseer, L., Clarke, M., Ghersi, D., Liberati, A., Petticrew, M., Shekelle, P., & Stewart, L. (2015). Preferred reporting items for systematic review and meta-analysis protocols (PRISMA-P) 2015 statement. Systematic Reviews, 4 (1), 1–9. https://doi.org/10.1186/2046-4053-4-1
Mousavi, A., Schmidt, M., Squires, V., & Wilson, K. (2020). Assessing the effectiveness of student advice recommender agent (SARA): The case of automated personalized feedback. International Journal of Artificial Intelligence in Education, 31 (2), 603–621. https://doi.org/10.1007/s40593-020-00210-6
Mousavinasab, E., Zarifsanaiey, N., Kalhori, S. R. N., Rakhshan, M., Keikha, L., & Saeedi, M. G. (2021). Intelligent tutoring systems: A systematic review of characteristics, applications, and evaluation methods. Interactive Learning Environments, 29 (1), 142–163. https://doi.org/10.1080/10494820.2018.1558257
Ouatik, F., Ouatikb, F., Fadlic, H., Elgoraria, A., Mohadabb, M. E. L., Raoufia, M., et al. (2021). E-Learning & decision making system for automate students assessment using remote laboratory and machine learning. Journal of E-Learning and Knowledge Society, 17 (1), 90–100. https://doi.org/10.20368/1971-8829/1135285
Ouyang, F., Zheng, L., & Jiao, P. (2022). Artificial intelligence in online higher education: A systematic review of empirical research from 2011–2020. Education and Information Technologies, 27 , 7893–7925. https://doi.org/10.1007/s10639-022-10925-9
Page, M. J., McKenzie, J. E., Bossuyt, P. M., Boutron, I., Hoffmann, T., Mulrow, C., Shamseer, L., Tetzlaff, J. M., Akl, E. A., Brennan, S. E., Chou, R., Glanville, J., Grimshaw, J. M., Hróbjartsson, A., Lalu, M. M., Li, T., Loder, E. W., Mayo-Wilson, E., McDonald, S., … Moher, D. (2021). The PRISMA 2020 statement: An updated guideline for reporting systematic reviews. British Medical Journal . https://doi.org/10.1136/bmj.n71
Popenici, S. A. D., & Kerr, S. (2017). Exploring the impact of artificial intelligence on teaching and learning in higher education. Research and Practice in Technology Enhanced Learning, 12 (22), 1–13. https://doi.org/10.1186/s41039-017-0062-8
PRISMA Statement. (2021). PRISMA endorsers. PRISMA statement website. http://www.prisma-statement.org/Endorsement/PRISMAEndorsers
Qian, Y., Li, C.-X., Zou, X.-G., Feng, X.-B., Xiao, M.-H., & Ding, Y.-Q. (2022). Research on predicting learning achievement in a flipped classroom based on MOOCs by big data analysis. Computer Applied Applications in Engineering Education, 30 , 222–234. https://doi.org/10.1002/cae.22452
Rutner, S. M., & Scott, R. A. (2022). Use of artificial intelligence to grade student discussion boards: An exploratory study. Information Systems Education Journal, 20 (4), 4–18.
Salas-Pilco, S., & Yang, Y. (2022). Artificial Intelligence application in Latin America higher education: A systematic review. International Journal of Educational Technology in Higher Education, 19 (21), 1–20. https://doi.org/10.1186/S41239-022-00326-w
Saldana, J. (2015). The coding manual for qualitative researchers (3rd ed.). Sage.
Shukla, A. K., Janmaijaya, M., Abraham, A., & Muhuri, P. K. (2019). Engineering applications of artificial intelligence: A bibliometric analysis of 30 years (1988–2018). Engineering Applications of Artificial Intelligence, 85 , 517–532. https://doi.org/10.1016/j.engappai.2019.06.010
Strauss, A., & Corbin, J. (1995). Grounded theory methodology: An overview. In N. K. Denzin & Y. S. Lincoln (Eds.), Handbook of qualitative research (pp. 273–285). Sage.
Turing, A. M. (1937). On computable numbers, with an application to the Entscheidungs problem. Proceedings of the London Mathematical Society, 2 (1), 230–265.
Turing, A. M. (1950). Computing machinery and intelligence. Mind, 59 , 443–460.
MathSciNet Google Scholar
Ullah, H., & Hafiz, M. A. (2022). Exploring effective classroom management strategies in secondary schools of Punjab. Journal of the Research Society of Pakistan, 59 (1), 76.
Verdú, E., Regueras, L. M., Gal, E., et al. (2017). Integration of an intelligent tutoring system in a course of computer network design. Educational Technology Research and Development, 65 , 653–677. https://doi.org/10.1007/s11423-016-9503-0
Vygotsky, L. S. (1978). Mind and society: The development of higher psychological processes . Harvard University Press.
Winkler-Schwartz, A., Bissonnette, V., Mirchi, N., Ponnudurai, N., Yilmaz, R., Ledwos, N., Siyar, S., Azarnoush, H., Karlik, B., & Del Maestro, R. F. (2019). Artificial intelligence in medical education: Best practices using machine learning to assess surgical expertise in virtual reality simulation. Journal of Surgical Education, 76 (6), 1681–1690. https://doi.org/10.1016/j.jsurg.2019.05.015
Yang, A. C. M., Chen, I. Y. L., Flanagan, B., & Ogata, H. (2021). Automatic generation of cloze items for repeated testing to improve reading comprehension. Educational Technology & Society, 24 (3), 147–158.
Yao, X. (2022). Design and research of artificial intelligence in multimedia intelligent question answering system and self-test system. Advances in Multimedia . https://doi.org/10.1155/2022/2156111
Zawacki-Richter, O., Marín, V. I., Bond, M., & Gouverneur, F. (2019). Systematic review of research on artificial intelligence applications in higher education—Where are the educators? International Journal of Educational Technology in Higher Education, 16 (1), 1–27. https://doi.org/10.1186/s41239-019-0171-0
Zhang, F. (2022). Design and application of artificial intelligence technology-driven education and teaching system in universities. Computational and Mathematical Methods in Medicine . https://doi.org/10.1155/2022/8503239
Zhang, Z., & Xu, L. (2022). Student engagement with automated feedback on academic writing: A study on Uyghur ethnic minority students in China. Journal of Multilingual and Multicultural Development . https://doi.org/10.1080/01434632.2022.2102175
Download references
Acknowledgements
The authors would like to thank Mildred Jones, Katherina Nako, Yaser Sendi, and Ricardo Randall for data gathering and organization.
Author information
Authors and affiliations.
Department of Teaching and Learning, Old Dominion University, Norfolk, USA
Helen Crompton
ODUGlobal, Norfolk, USA
Diane Burke
RIDIL, ODUGlobal, Norfolk, USA
You can also search for this author in PubMed Google Scholar
Contributions
HC: Conceptualization; Data curation; Project administration; Formal analysis; Methodology; Project administration; original draft; and review & editing. DB: Conceptualization; Data curation; Project administration; Formal analysis; Methodology; Project administration; original draft; and review & editing. Both authors read and approved this manuscript.
Corresponding author
Correspondence to Helen Crompton .
Ethics declarations
Competing interests.
The authors declare that they have no competing interests.
Additional information
Publisher's note.
Springer Nature remains neutral with regard to jurisdictional claims in published maps and institutional affiliations.
Rights and permissions
Open Access This article is licensed under a Creative Commons Attribution 4.0 International License, which permits use, sharing, adaptation, distribution and reproduction in any medium or format, as long as you give appropriate credit to the original author(s) and the source, provide a link to the Creative Commons licence, and indicate if changes were made. The images or other third party material in this article are included in the article's Creative Commons licence, unless indicated otherwise in a credit line to the material. If material is not included in the article's Creative Commons licence and your intended use is not permitted by statutory regulation or exceeds the permitted use, you will need to obtain permission directly from the copyright holder. To view a copy of this licence, visit http://creativecommons.org/licenses/by/4.0/ .
Reprints and permissions
About this article
Cite this article.
Crompton, H., Burke, D. Artificial intelligence in higher education: the state of the field. Int J Educ Technol High Educ 20 , 22 (2023). https://doi.org/10.1186/s41239-023-00392-8
Download citation
Received : 30 January 2023
Accepted : 23 March 2023
Published : 24 April 2023
DOI : https://doi.org/10.1186/s41239-023-00392-8
Share this article
Anyone you share the following link with will be able to read this content:
Sorry, a shareable link is not currently available for this article.
Provided by the Springer Nature SharedIt content-sharing initiative
- Artificial Intelligence
- Systematic review
- Higher education
Smart learning: AI resources every educator should know
April 18, 2024.
By Microsoft Education Team
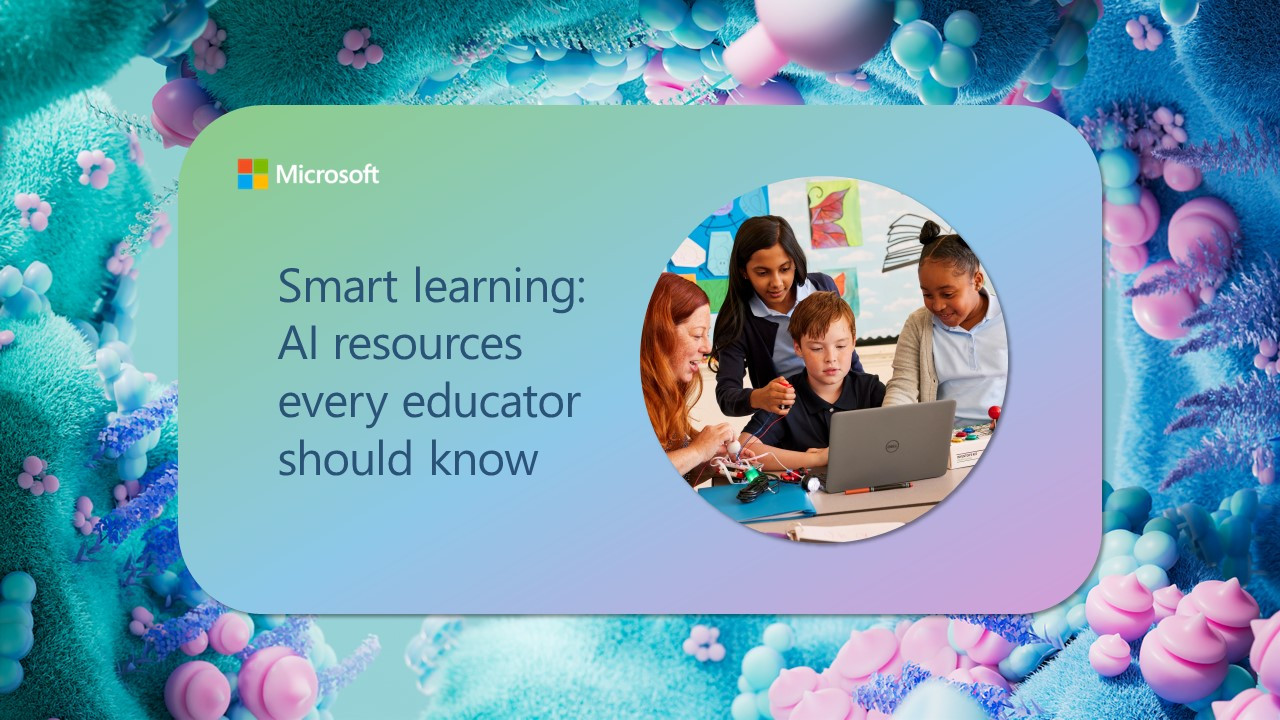
Share this article
On April 19 in the United States, we celebrate National AI Literacy Day , a nationwide initiative aimed at fostering understanding and engagement with AI. With developments in AI happening so quickly and new products and features launching all the time, it can be difficult to keep up. We want to equip you with the knowledge needed to navigate the world of AI. We’ve gathered some resources, activities, and training to help you get up to speed on all things AI, and how it can be useful in education. In addition to the items below, you’ll find a collection of AI for education resources and learning opportunities on the Microsoft Learn Educator Center .
AI literacy is crucial in today's digital age, where AI technologies are increasingly integrated into our daily lives. Our latest insights from the AI in Education: Microsoft Special Report demonstrate a disconnect—use of AI in education is outpacing the understanding of the technology. There’s an urgent need to increase AI literacy and AI integration in strategic priorities, standards, policies, and professional development.
For educators, it's about preparing your students for a future where AI will be a fundamental part of many professions. It’s also about guiding learners to use AI tools safely and responsibly, ensuring they understand the implications of AI on privacy and data security. By fostering AI literacy, we can shape a future where everyone can navigate and benefit from AI advancements confidently and responsibly. Whether you’re a teacher, parent/guardian, or curious learner, here are some valuable resources to enhance your AI literacy from Microsoft Education .
11 resources for educators to amp up your AI literacy
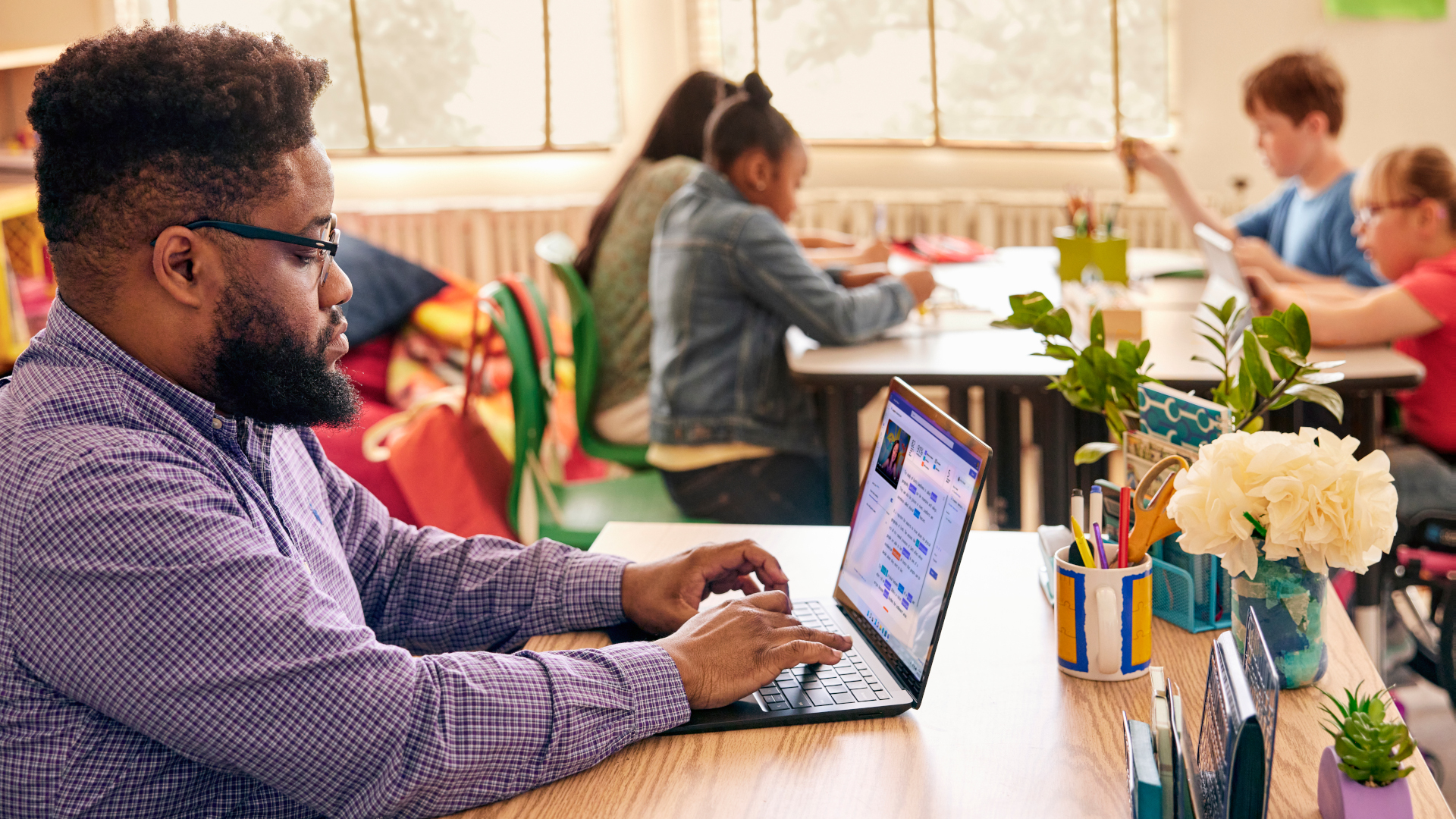
For National AI Literacy Day 2024, explore the AI in education professional development opportunities available from Microsoft.
AI in education professional development
- AI for Educators training : Spend a few hours on your professional development and learn about the history of AI, large language models (LLMs), generative AI, how to create a prompt, and uses of AI in education. The AI for Educators Learning Path on Microsoft Learn is made up of three modules: “Empower educators to explore the potential of artificial intelligence,” “Enhance teaching and learning with Microsoft Copilot,” and “Equip and support learners with AI tools from Microsoft.” From now until April 30, 2024, participate in the AI skills challenge for educators and benchmark your progress against fellow educators and friends.
- Flip AI for educators series : Flip offers free professional development training sessions that provide easy-to-follow instructions, best practices, and inspiration on various topics, including AI! You can catch up on the great AI for educators series events that have already happened, and be sure to register for upcoming professional development events as well.
- Microsoft Education AI Toolkit : The Microsoft Education AI Toolkit provides education leaders with relevant background knowledge, strategies, and recommendations for launching AI initiatives in K-20 settings. It also includes customer stories and technical profiles that showcase how institutions around the globe are already using AI for teaching, learning, and administration. What’s inside of the toolkit provides those in charge with the necessary information that they need to jumpstart their own AI journey. Learn more about the Microsoft Education AI Toolkit and how to use this resource in this article: Kickstart your school’s AI journey with the Microsoft Education AI Toolkit .
Get started using Microsoft Copilot in education
- Introduction to Microsoft Copilot : Learn all about Microsoft Copilot, your AI-powered assistant for education. Get an overview of how to use Copilot, as well as ideas and inspiration for how you can use Copilot to save time, differentiate instruction, and enhance student learning. You can save or print this quick guide to Microsoft Copilot to refer back to as needed.
- Copilot resources for education : Dive deeper into what Copilot can do with resources for education. Whether you’re an educator, IT professional, or parent/guardian, you’ll find helpful resources to get started using Copilot.
- Copilot lab : While it’s not specific to education, the Copilot lab is a great resource to help you learn more about Copilot, how to write a prompt, and ideas of how to get started using Copilot.
Improve your students’ AI literacy, too!
By fostering AI literacy, together we can shape a future where everyone can navigate and benefit from AI advancements.
- Classroom toolkit: Unlocking generative AI safely and responsibly is a creative resource that blends engaging narrative stories with instructional information to create an immersive and effective learning experience for educators and students aged 13-15 years. The toolkit is designed to assist educators in initiating important conversations about responsible AI practices in the classroom, such as the critical topics of content fabrications, privacy considerations, bias awareness, and mental wellbeing.
- Minecraft AI Prompt Lab : Embracing the ever-changing world of education calls for innovation and tech-savvy teaching methods. The Minecraft AI Prompt Lab is a new series of resources that demonstrates how to use Microsoft Copilot with Minecraft Education to design amazing learning experiences. Crafted for educators like you, this game-changing guide is here to revolutionize the way you deliver educational content with Minecraft. In Prompt Lab: Module 1 , learn how to write prompts, develop learning content and assessments, and generate creative ideas for lesson plans will help you unlock the power of game-based learning with Minecraft Education. In Prompt Lab: Module 2 , learn the basics of Code Builder, the in-game coding feature of Minecraft Education.
- Minecraft Hour of Code: Generation AI : All students deserve opportunities to explore AI technology to understand its implications, access career pathways, and be empowered to safely, confidently navigate an AI-powered world. Designed for anyone ages 7 and up, Minecraft Hour of Code: Generation AI is a fun, accessible way to explore the fundamentals of coding and responsible AI. Students will venture through time to create helpful AI-powered inventions to solve problems and make daily life easier. Learn coding basics and essential principles of computer science, all while encouraging thoughtful discussions around responsible coding and AI development. With free downloadable educator resources exploring the amazing potential of AI has never been more exiting or immersive!
Online safety and information literacy are the foundation of AI literacy
- Microsoft Family Safety Toolkit : To help young people, educators, and families navigate the digital world, Microsoft has also released an online safety resource, the Microsoft Family Safety Toolkit . This toolkit provides guidance on how to leverage Microsoft’s safety features and family safety settings to support and enhance digital parenting, plus guidance for families looking to navigate the world of generative AI together. Bonus resource for young children: PBS Kids launched an educational series on AI supported by Microsoft.
- Search Progress and Coach : Empowering learners to seek, evaluate, and use online sources responsibly is a critical step in helping them to navigate AI-generated content and the wider information ecosystem with confidence. This short course on our newest Learning Accelerators, Search Progress and Search Coach , showcases how educators can help foster information literacy skills through any research-based assignment in Microsoft Teams for Education.
Let’s celebrate knowledge, curiosity, and the transformative power of AI. Join us this National AI Literacy Day to explore these resources and take a step towards a more informed and inclusive future with AI. Whether you're an educator looking to bring AI into the classroom or a parent guiding your child in the digital world, these resources will equip you with the knowledge to embrace AI's potential responsibly. Let's celebrate the day by committing to lifelong learning and curiosity in the ever-evolving field of AI.
Related stories
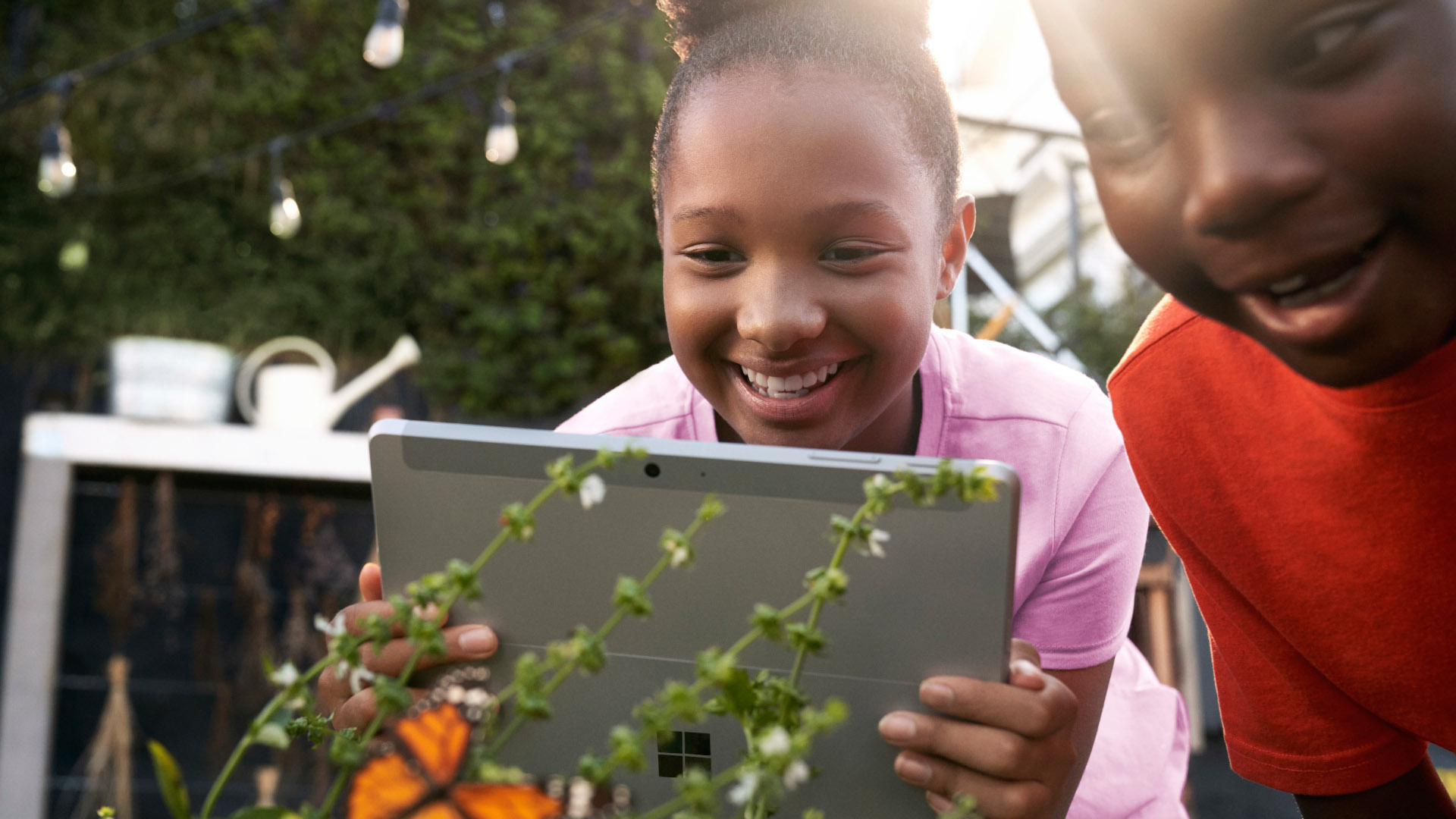
How to celebrate Earth Day 2024 with your students
Spark your students' curiosity with Earth Day activities and more from Microsoft. From video games to projects, try these fun Earth Day activities for your class.
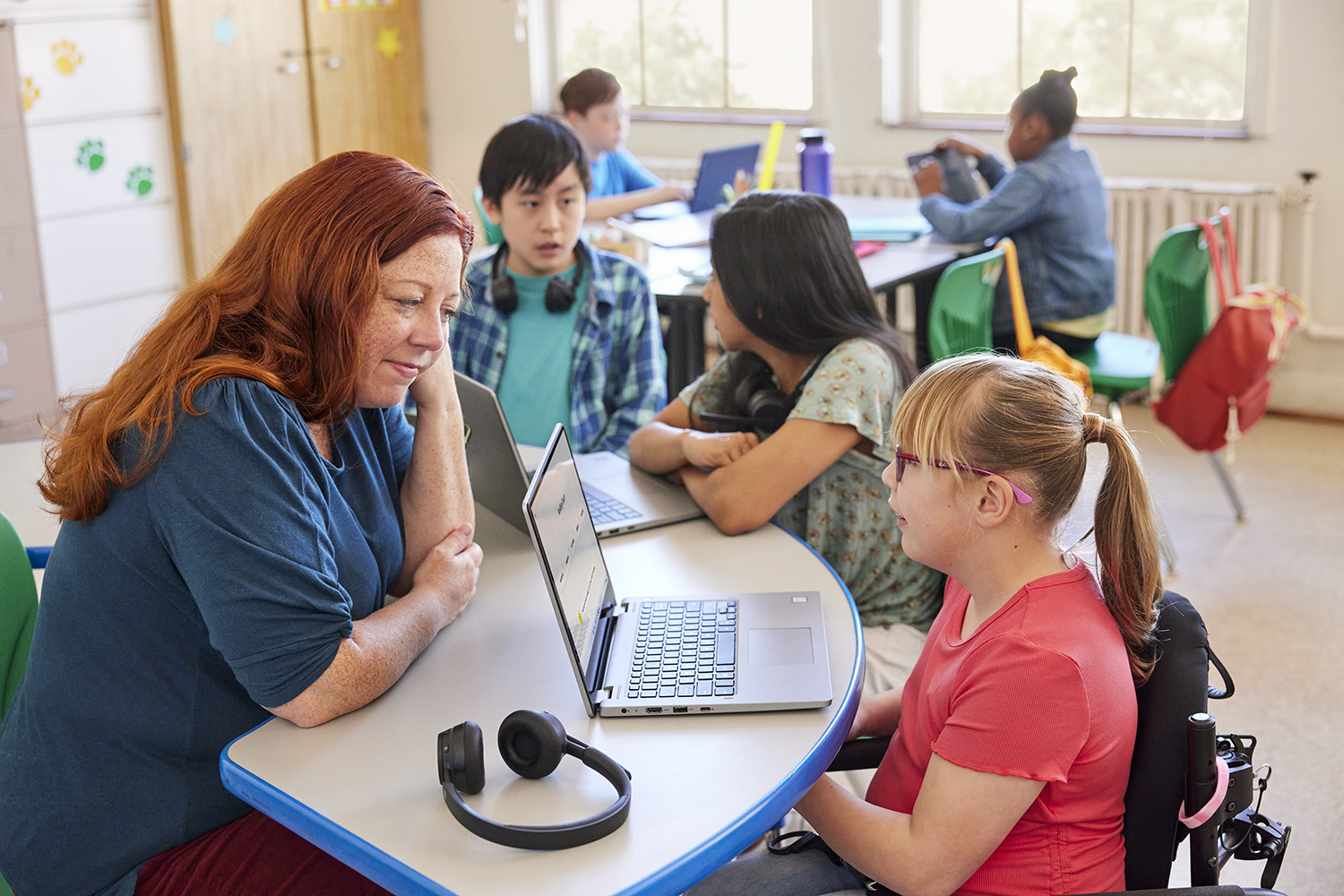
Stay ahead with 8 new updates from Microsoft Education
We recognize that teachers often look for new and effective ways to engage their students and support their learning goals. That's why we're constantly working to improve Microsoft Education solutions, features, and training, so you can have access to powerful classroom tools for teaching.
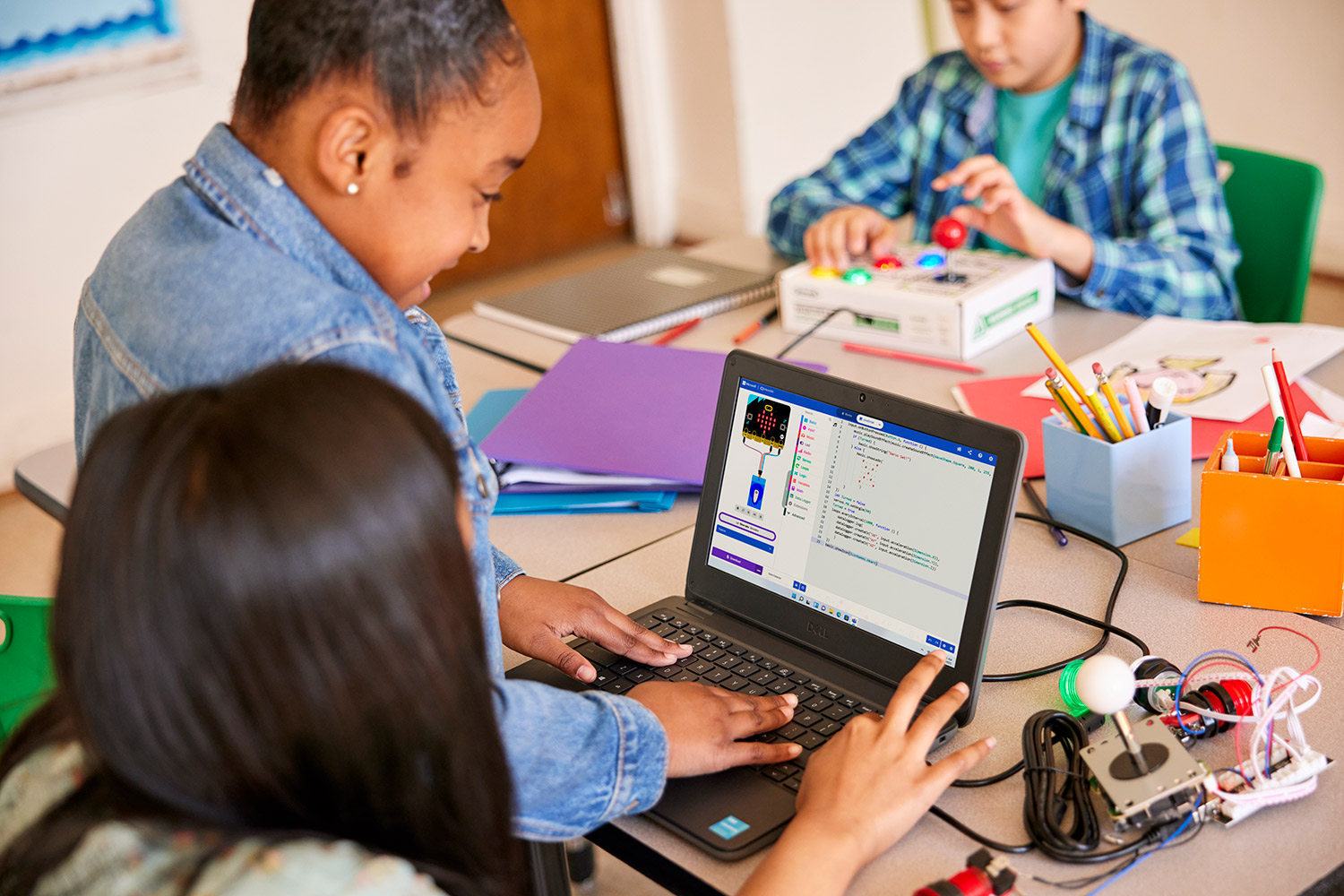
Gearing up for Computer Science Education Week
It’s the most wonderful time of the year: Computer Science Education Week! All year round, but especially December 4–10, 2023, we aim to inspire students to engage in computer science with opportunities to learn about AI, advocate for equity in digital careers, and become responsible coders.
- SCHOOL STORIES
- MICROSOFT EDUCATOR CENTER
- CONTACT SALES
- India Today
- Business Today
- Reader’s Digest
- Harper's Bazaar
- Brides Today
- Cosmopolitan
- Aaj Tak Campus
- India Today Hindi
Explained | What is the role of AI in the education sector?
Artificial intelligence provides a secure solution to ensure the integrity of online test system assessments in a cost-effective and scalable manner..
Listen to Story
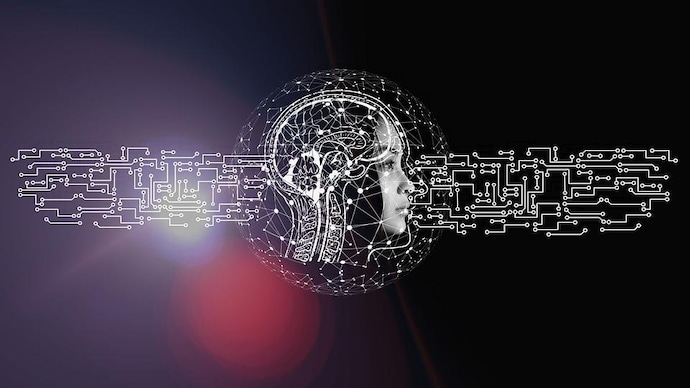
The advent of Artificial Intelligence (AI) is bringing drastic changes in technical fields, where it can be used to automate systems for better performance and efficiency, while we aren't really aware of how AI makes everyday life simpler and easier. Artificial Intelligence enhances the speed, precision, and effectiveness of human efforts.
It is essential for AI and machine learning tools to learn various types of data annotations in order to produce the proper results, as AI has now been widely implemented in a wide variety of fields, including mobile phones, social networks, and the prevention and response of active threats.
A real-time and accurate diagnosis of diseases can be accomplished by AI in an array of fields, including Automotive (self-driving cars), Virtual assistants or chatbots, Retail and E-commerce, Manufacturing, Cyber Security, Healthcare and Medical Imaging Analysis, and many others, including Education.
Recently, many AI applications have been developed for the education sector. And so many processes have become simpler and faster. Students can participate in online courses without interruption and access all learning materials via computers, laptops and smart devices, so students can easily join classes and do not need to attend physical classes.
AI IN EDUCATION:
Artificial intelligence provides a secure solution to ensure the integrity of online test system assessments in a cost-effective and scalable manner. The use of AI can reduce or even eliminate the need for physical supervisors/inspectors and can make deployment far more scalable. And with AI-supported online supervision, such incidents are automatically highlighted. Warnings are often automated as well.
This makes the system fairly reliable for conducting high-stakes exams without the hassle and risk of going to a center to take the test. One can conduct online exams for remote users securely with AI-powered remote proctoring.
A secure and cost-effective way to prevent cheating during online exams. System algorithms can help one to detect and prevent cheating during the online exam process.
1. AI-POWERED REMOTE PROCTORING
2. how does ai helps conduct fair exams.
The AI Proctored assessment uses a combination of artificial intelligence and human proctors. Since a video of the candidate taking the test is recorded through a webcam, the AI is able to flag or report any suspicious movement or activity.
An AI-assisted proctor is software that is often powered by artificial intelligence (AI), which keeps an eye on a candidate. It helps educational institutions by detecting voices, detecting another person apart from the examinee.
3. LEARNING THROUGH CHATBOTS
4. personalised learning through recommendations.
AI helps students get personalised answers to relevant questions from teachers. It also helps educate students according to the issues and questions they face in class materials and online sessions. Students now have access to a larger system for interacting with professors.
5. EDUCATION WITHOUT BOUNDARIES
AI can now help manage education systems, including exams, beyond boundaries. AI is facilitating the learning of any course across the globe and at anytime and anywhere.
Many AI applications are being used within the framework of the education system to help students get educated through online courses and online exams and to help many schools and colleges acquire the right students around the world.
Artificial Intelligence in Education
- First Online: 25 August 2022
Cite this chapter
- Venkata Rajasekhar Moturu 11 &
- Srinivas Dinakar Nethi 11
Part of the book series: Lecture Notes in Networks and Systems ((LNNS,volume 478))
740 Accesses
3 Citations
Artificial intelligence-deployed applications for learning are in use and made way into the field of education for quite some time. Therefore, its requirement forces teachers, learners, and stakeholders more than ever in the past. The aim of the current study is to provide focus on the matter that implementation of AI is not a choice but a need. As education technology evolves as a new standard, all the stakeholders involved in education must deploy AI to obtain the basic education goals, i.e., it must be individualized, effective, transformative, output based, integrative and long lasting. The current research is to portray the transformation in methods of education and put forward the current directions in incorporating artificial intelligence. The investigators contemplate on this issue and attempt to address how advancement of AI is contemporaneous with advancement in education. The pursuit to examine this circumstantial believes to add productive surface for a fruitful consideration on probing the power of artificial intelligence-based e-learning applications.
This is a preview of subscription content, log in via an institution to check access.
Access this chapter
- Available as PDF
- Read on any device
- Instant download
- Own it forever
- Available as EPUB and PDF
- Compact, lightweight edition
- Dispatched in 3 to 5 business days
- Free shipping worldwide - see info
- Durable hardcover edition
Tax calculation will be finalised at checkout
Purchases are for personal use only
Institutional subscriptions
(Online) BusinessWire: Global Ed tech Market Report 2021: Industry Trends, Market Size, and Forecasts 2019–2027—ResearchAndMarkets.com Available at: “ https://www.businesswire.com/news/home/20211118005651/en/Global-EdTech-Market-Report-2021-Industry-Analysis-Trends-Market-Size-and-Forecasts-2019-2027---ResearchAndMarkets.com
(Online) IBEF (2022) Available at: “ https://www.ibef.org/blogs/india-to-become-the-edtech-capital-of-the-world
Evans HK, Cordova V (2015) Lecture videos in online courses: a follow-up. J Political Sci Educ 11:472–482
Google Scholar
Scagnoli NI, Choo J, Tian J (2019) Students’ insights on the use of video lectures in online classes. British J Educ Technol 50(1):399–414
Agre PE (1999) Information technology in higher education: the global academic village and intellectual standardization. On the Horizon 7(5):8–11
Hawkins BL (1999) Distributed learning and institutional restructuring. Educom Rev 34(4):12–15, 42–44
Feenberg A (2002) In: Transforming technology: a critical theory revisited. Oxford University Press
Noble D (1998) F: Digital diploma mills: the automation of higher education. Science as Culture 7(3):355–368
Article Google Scholar
Kenneth KL, Dedrick J, Sharma P (2009) One laptop per child: vision versus reality. Communications of the ACM 52(6):66–73
Arroyo I, Royer JM, Woolf BP (2011) Using an intelligent tutor and math fluency training to improve math performance. Int J Artif Intell Educ 21(2):135–152
Woolf BP, Lane HC, Chaudhri VK, Kolodner J (2013) L: AI grand challenges for education. AI Mag 34(4):66
Hao K (2019) China has started a grand experiment in AI education. it could reshape how the world learns. MIT Technology Review
Liyanagunawardena TR, Williams S, Adams AA (2014) The impact and reach of MOOCs: a developing countries’ perspective. eLearning Papers 38–46
Tomlinson CA (2000) Reconcilable differences? standards based teaching and differentiation. Educational Leadership: J Department of Supervision and Curriculum Development, N.E.A. 581:6–13
Van Lehn K (2011) The relative effectiveness of human tutoring, intelligent tutoring systems, and other tutoring systems. Educational Psychol 46(4):197–221
Collins A, Halverson R (2010) The second educational revolution: rethinking education in the age of technology. J Comput Assisted Learn 26(1):18–27
Heffernan NT, Heffernan C (2014) L: The assistments ecosystem: building a platform that brings scientists and teachers together for minimally invasive research on human learning and teaching. Int J Artif Intell Educ 24(4):470–497
Article MathSciNet Google Scholar
Dillenbourg P (2013) Design for classroom orchestration. Comput Educ 69(1):485–492
Morrison CD (2014) From ‘sage on the stage’ to ‘guide on the side’: a good start. Int J Sch Teach Learn 8(1)
Toner P (2011) Workforce skills and innovation: An overview of major themes in the literature. OECD Education Working Paper, OECD Publications No. 55
Banfield J, Wilkerson B (2014) Increasing student intrinsic motivation and self-efficacy through gamification pedagogy. Contemporary Issues in Educ Res 7(4):291
Koedinger KR, Corbett AT (2006) Cognitive tutors: technology bringing learning science to the classroom. In: Sawyer K (ed) The Cambridge handbook of the learning sciences. Cambridge University Press, pp 61–78
Baker RSJD, Corbett AT, Koedinger KR, Evenson SE, Roll I, Wagner AZ, Naim M, Raspat J, Baker DJ, Beck J (2006) Adapting to when students game an intelli- gent tutoring system. In: Proceedings of the 8th international conference on intelligent tutoring systems
Roll I, Wylie R (2016) Evolution and revolution in artificial intelligence in education. Int J Artif Intell Educ 26(2):582–599
Bingimlas K (2009) Barriers to the successful integration of ICT in teaching and learning environments: a review of the literature. Eurasia J Mathem Sci Technol Educ 5(3):235–245
Fishman B, Penuel WR, Allen A, Cheng BH, Sabelli N (2013) Design-based implementation research: an emerging model for transforming the relationship of research and practice. In Fishman AJ, Penuel WR, Allen A-R, Cheng BH (eds) Design-based implementation research: theories, methods, and exemplars. National Society for the Study of Education Yearbook, vol 112(2), pp 136–156
Rosenberger R (2017) The ICT educator’s fallacy. Found Sci 22:395–399
Feenberg A (2017) The online education controversy. Found Sci 22(2):363–371
Winner L (1980) Do artifacts have politics? In: Kaplan DM (ed), Readings in the philosophy of technology (pp 289–203). Albany: Rowman & Littlefield
Dreyfus HL (2002) Anonynmity versus commitment: the dangers of education on the internet. Educ Philos Theory 34(4):369–378
Boulay B (2011) Towards a motivationally intelligent pedagogy: How should an intelligent tutor respond to the unmotivated or the demotivated? In: Clavo R, D’Mello S (eds) New perspectives on affect and learning technologies. Explorations in the learning sciences, instructional systems and performance technologies, vol 3
Christensen G, Steinmetz A, Alcorn B, Bennett A, Woods D, Emanuel E (2013) The MOOC phenomenon: who takes massive open online courses and why?. Available at SSRN 2350964
Simonite T (2013) Search under way for gold in online education data trove. MIT Technology Review
Christensen G, Steinmetz A, Alcorn B, Bennett A, Woods D, Emanuel EJ (2013) The MOOC phenomenon: who takes massive open online courses and why?
Francesco I (2019) “Do Artifacts Have Politics?” by Langdon Winner. A reflection” Available at: “ https://francescoimola.medium.com/do-artifacts-have-politics-by-langdon-winner-a-reflection-bde891f9f546 ” online (2019)
Bingimlas K (2010) Evaluating the quality of science teachers’ practices in ICT-supported learning and teaching environments in Saudi primary schools (Doctoral dissertation, RMIT University)
Download references
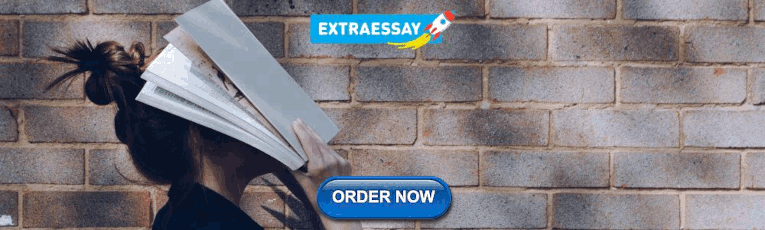
Author information
Authors and affiliations.
Assistant (Academics and Research), Indian Institute of Management Visakhapatnam, Visakhapatnam, Andhra Pradesh, India
Venkata Rajasekhar Moturu & Srinivas Dinakar Nethi
You can also search for this author in PubMed Google Scholar
Corresponding author
Correspondence to Venkata Rajasekhar Moturu .
Editor information
Editors and affiliations.
Muffakham Jah College Engineering and Technology, Hyderabad, India
Mousmi Ajay Chaurasia
National Chung Hsing University, Taichung, Taiwan
Chia-Feng Juang
Rights and permissions
Reprints and permissions
Copyright information
© 2023 The Author(s), under exclusive license to Springer Nature Singapore Pte Ltd.
About this chapter
Moturu, V.R., Nethi, S.D. (2023). Artificial Intelligence in Education. In: Chaurasia, M.A., Juang, CF. (eds) Emerging IT/ICT and AI Technologies Affecting Society. Lecture Notes in Networks and Systems, vol 478. Springer, Singapore. https://doi.org/10.1007/978-981-19-2940-3_16
Download citation
DOI : https://doi.org/10.1007/978-981-19-2940-3_16
Published : 25 August 2022
Publisher Name : Springer, Singapore
Print ISBN : 978-981-19-2939-7
Online ISBN : 978-981-19-2940-3
eBook Packages : Engineering Engineering (R0)
Share this chapter
Anyone you share the following link with will be able to read this content:
Sorry, a shareable link is not currently available for this article.
Provided by the Springer Nature SharedIt content-sharing initiative
- Publish with us
Policies and ethics
- Find a journal
- Track your research
- Join our email list
- Post an article
How can we help you?
5 main roles of artificial intelligence in education.
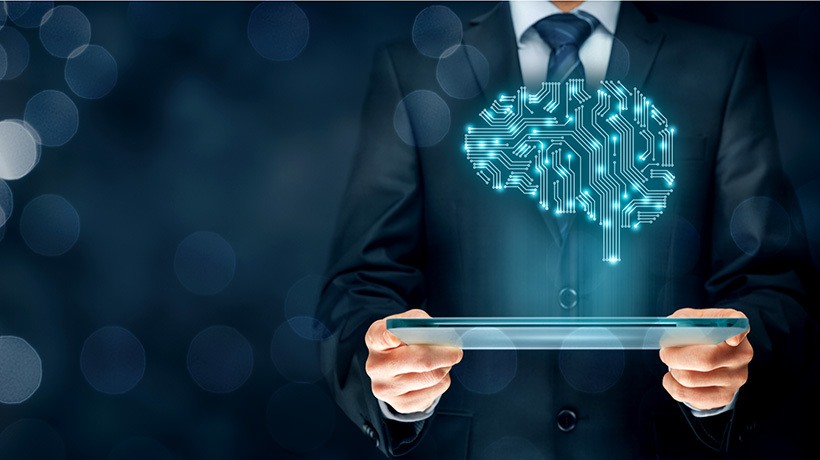
How AI Transforms The Learning Experience
"Our intelligence is what makes us human, and AI is an extension of that quality. " – Yann LeCun Professor, New York University
Artificial Intelligence is a branch of science producing and studying the machines aimed at the stimulation of human intelligence processes. The main objective of AI is to optimize the routine processes, improving their speed and efficiency (provided it has been implemented and supported properly). As a result, the number of companies adopting AI continues to grow worldwide.
According to Research and Markets, “The analysts forecast the Artificial Intelligence Market in the US Education Sector to grow at a CAGR of 47.77% during the period 2018-2022.”
AI tools mostly comply with 3 basic principles:
- Learning: Acquiring and processing the new experience, creating new behavior models
- Self-correction: Refining the algorithms to ensure the most accurate results
- Reasoning: Picking up the specific algorithms to resolve a specific task
And are presented in 4 basic forms:
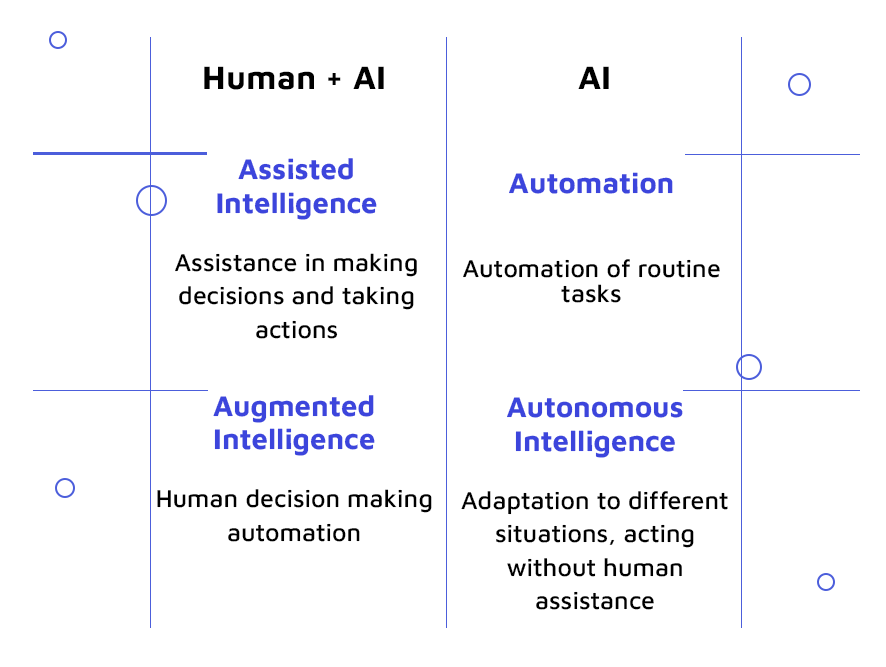
The forms of AI in the first row are incapable of learning from their experience.
Regarding the big opportunities, AI tools open for every sector including the educational one. The adoption of technology seems to be one of the most promising ways to transform organizations.
Roles Of AI In Education
Global adoption of technology in education is transforming the way we teach and learn. Artificial Intelligence is one of the disruptive techniques to customize the experience of different learning groups, teachers, and tutors.
This is how Artificial Intelligence tools may be applied to improve study processes:
1. Personalize Education
Artificial Intelligence helps find out what a student does and does not know, building a personalized study schedule for each learner considering the knowledge gaps. In such a way, AI tailors studies according to student’s specific needs, increasing their efficiency.
To do it, many companies train their AIs, armed by the Knowledge Space Theory , to define and represent the knowledge gaps, taking into account the complexity of scientific concepts relations between each other (one can stimulate the learning of another or become a basis for filling in the gap).
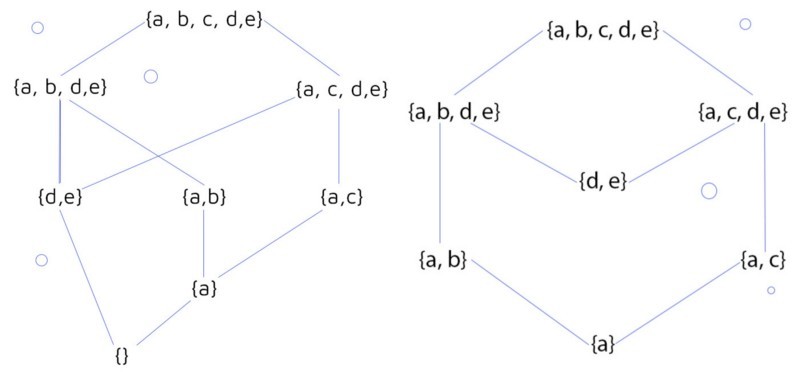
2. Produce Smart Content
- Digital lessons Digital learning interfaces with customization options, digital textbooks, study guides, bite-sized lessons, and much more can be generated with the help of AI.
- Information visualization New ways of perceiving information, such as visualization, simulation, web-based study environments, can be powered by AI.
- Learning content updates Besides, AI helps generate and update the content of the lessons, keeping the information up to date and customizing it for different learning curves.
3. Contribute To Task Automation
Administrative tasks simplification: grading, assessing, and replying to students is a time-consuming activity that could be optimized by the teacher using AI.
Do you remember the hints Gmail provides in the messages you compose based on the overview of your current and past messages plus the business vocabulary essentials? It would be great to have such an option on any Learning Management System or learning platform envisaging the feedback.
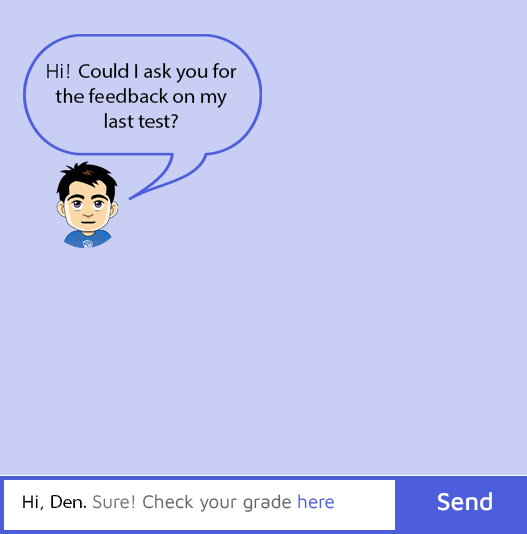
(AI-powered grading tool could be trained to display the information on the learning progress of each student)
Entrusting a set of routine tasks to AI helps teachers make room for something more important: concentrating on grading the assignments impossible to delegate to Artificial Intelligence, self-education, upgrading the quality of the lessons.
4. Do Tutoring
Continuously evolving personal study programs take into account student’s gaps to fill during individual lessons. Personal tutoring and support for the students outside of the classroom help learners keep up with the course and keep their parents from struggling to explain algebra to their kids. AI tutors are great time-savers for the teachers, as they do not need to spend extra time explaining challenging topics to students. With AI-powered chatbots or AI virtual personal assistants, students can avoid being embarrassed by asking for additional help in front of their friends.
5. Ensure Access To Education For Students With Special Needs
The adoption of innovative AI technologies opens up new ways of interacting for students with learning disabilities. AI grants access to education for students with special needs : deaf and hard of hearing, visually impaired, people with ASD…
Artificial Intelligence tools can be successfully trained to help any group of students with special needs.
Benefits Of AI For Students
24/7 access to learning.
With AI helpers based online, students always have access to learning. They are free to plan their day without being linked to a specific place. They can study on the go, at any place and time they want. They can build their schedule based on their most productive hours.
Better Engagement
Individualized schedules, custom tasks, interaction with digital technologies, and personal recommendations are part of the personal approach each student gets using AI. Besides, a personal approach helps students feel special, increasing their engagement and raising interest in studies in such a way.
Less Pressure
Lessons tailored to the needs of different learning groups allow students to stop comparing them to each other. Earlier, a student should have asked a teacher for help in front of the class. Now, it’s enough to type a query using a personal virtual assistant and get an instant explanation.
These opportunities offered by AI tools make personal progress come to the fore, reducing the pressure in the classroom. Less pressure means less stress and more enthusiasm to study.
How To Start Implementing AI
If you’re considering AI as an option to customize the learning experience, these steps will help you to plan your project.
- Identify your needs and AI technologies The starting point of implementing any technology is the identification of the pain points this technology can address and resolve. Find the system bottlenecks and research the ways AI offers to optimize these processes.
- Determine the strategic objectives of AI transformation in your organization Determine your appetite: Do you want to be an early adopter or the follower? Which technologies will fit your company best? Are you aware of the AI drawbacks and how are you going to address them? The completion of which business objectives should AI technology contribute to? Based on responses to these questions, you should develop a cost-benefit analysis for AI automation and augmentation.
- Make the right culture, talent, and technology meet To make the most of the AI tools, you should not only choose the right team to adopt the technology but also create the right environment driven by analytical insights and focused on actionable decisions on all organizational levels.
- Smart ways to control the outcome of AI transformation Creating an environment for both human beings and AI to work side-by-side, it’s important to ensure the processes' transparency and keep pace with the key considerations and metrics of AI adoption. Based on the custom characteristics of your organization and type of AI implemented, decide on the performance indicators to track, security concerns to keep under control, and technical ecosystems to support.
Personalized Learning Made Possible With AI
If you’re keeping up with the global trends, you know: personalization is everywhere . The main advantage of AI is the possibility to train it to perform a long list of tasks, offering in such a way a personalized approach to education. It’s a universal solution to get a set of tools tailored to the specific needs of learners and educators to optimize their routine, increase efficiency, improve accessibility, and scale the processes.
Originally published at jellyfish.tech .
- Incorporating Artificial Intelligence Into The Classroom: An Examination Of Benefits, Challenges, And Best Practices
- The Role Of Artificial Intelligence In eLearning: Integrating AI Tech Into Education
- AI Tutors: How Artificial Intelligence Is Shaping Educational Support
- The Pros And Cons Of Using AI In Learning: Is ChatGPT Helping Or Hindering Learning Outcomes?
- How AI Is Responsible For The Transformation Of The Education Industry

An official website of the United States government
The .gov means it’s official. Federal government websites often end in .gov or .mil. Before sharing sensitive information, make sure you’re on a federal government site.
The site is secure. The https:// ensures that you are connecting to the official website and that any information you provide is encrypted and transmitted securely.
- Publications
- Account settings
Preview improvements coming to the PMC website in October 2024. Learn More or Try it out now .
- Advanced Search
- Journal List
- Springer Nature - PMC COVID-19 Collection

Artificial intelligence in education: Addressing ethical challenges in K-12 settings
Selin akgun.
Michigan State University, East Lansing, MI USA
Christine Greenhow
Associated data.
Not applicable.
Artificial intelligence (AI) is a field of study that combines the applications of machine learning, algorithm productions, and natural language processing. Applications of AI transform the tools of education. AI has a variety of educational applications, such as personalized learning platforms to promote students’ learning, automated assessment systems to aid teachers, and facial recognition systems to generate insights about learners’ behaviors. Despite the potential benefits of AI to support students’ learning experiences and teachers’ practices, the ethical and societal drawbacks of these systems are rarely fully considered in K-12 educational contexts. The ethical challenges of AI in education must be identified and introduced to teachers and students. To address these issues, this paper (1) briefly defines AI through the concepts of machine learning and algorithms; (2) introduces applications of AI in educational settings and benefits of AI systems to support students’ learning processes; (3) describes ethical challenges and dilemmas of using AI in education; and (4) addresses the teaching and understanding of AI by providing recommended instructional resources from two providers—i.e., the Massachusetts Institute of Technology’s (MIT) Media Lab and Code.org. The article aims to help practitioners reap the benefits and navigate ethical challenges of integrating AI in K-12 classrooms, while also introducing instructional resources that teachers can use to advance K-12 students’ understanding of AI and ethics.
Introduction
“Success in creating AI would be the biggest event in human history. Unfortunately, it might also be the last, unless we learn how to avoid the risks.” — Stephen Hawking.
We may not think about artificial intelligence (AI) on a daily basis, but it is all around us, and we have been using it for years. When we are doing a Google search, reading our emails, getting a doctor’s appointment, asking for driving directions, or getting movie and music recommendations, we are constantly using the applications of AI and its assistance in our lives. This need for assistance and our dependence on AI systems has become even more apparent during the COVID-19 pandemic. The growing impact and dominance of AI systems reveals itself in healthcare, education, communications, transportation, agriculture, and more. It is almost impossible to live in a modern society without encountering applications powered by AI [ 10 , 32 ].
Artificial intelligence (AI) can be defined briefly as the branch of computer science that deals with the simulation of intelligent behavior in computers and their capacity to mimic, and ideally improve, human behavior [ 43 ]. AI dominates the fields of science, engineering, and technology, but also is present in education through machine-learning systems and algorithm productions [ 43 ]. For instance, AI has a variety of algorithmic applications in education, such as personalized learning systems to promote students’ learning, automated assessment systems to support teachers in evaluating what students know, and facial recognition systems to provide insights about learners’ behaviors [ 49 ]. Besides these platforms, algorithm systems are prominent in education through different social media outlets, such as social network sites, microblogging systems, and mobile applications. Social media are increasingly integrated into K-12 education [ 7 ] and subordinate learners’ activities to intelligent algorithm systems [ 17 ]. Here, we use the American term “K–12 education” to refer to students’ education in kindergarten (K) (ages 5–6) through 12th grade (ages 17–18) in the United States, which is similar to primary and secondary education or pre-college level schooling in other countries. These AI systems can increase the capacity of K-12 educational systems and support the social and cognitive development of students and teachers [ 55 , 8 ]. More specifically, applications of AI can support instruction in mixed-ability classrooms; while personalized learning systems provide students with detailed and timely feedback about their writing products, automated assessment systems support teachers by freeing them from excessive workloads [ 26 , 42 ].
Despite the benefits of AI applications for education, they pose societal and ethical drawbacks. As the famous scientist, Stephen Hawking, pointed out that weighing these risks is vital for the future of humanity. Therefore, it is critical to take action toward addressing them. The biggest risks of integrating these algorithms in K-12 contexts are: (a) perpetuating existing systemic bias and discrimination, (b) perpetuating unfairness for students from mostly disadvantaged and marginalized groups, and (c) amplifying racism, sexism, xenophobia, and other forms of injustice and inequity [ 40 ]. These algorithms do not occur in a vacuum; rather, they shape and are shaped by ever-evolving cultural, social, institutional and political forces and structures [ 33 , 34 ]. As academics, scientists, and citizens, we have a responsibility to educate teachers and students to recognize the ethical challenges and implications of algorithm use. To create a future generation where an inclusive and diverse citizenry can participate in the development of the future of AI, we need to develop opportunities for K-12 students and teachers to learn about AI via AI- and ethics-based curricula and professional development [ 2 , 58 ]
Toward this end, the existing literature provides little guidance and contains a limited number of studies that focus on supporting K-12 students and teachers’ understanding of social, cultural, and ethical implications of AI [ 2 ]. Most studies reflect university students’ engagement with ethical ideas about algorithmic bias, but few addresses how to promote students’ understanding of AI and ethics in K-12 settings. Therefore, this article: (a) synthesizes ethical issues surrounding AI in education as identified in the educational literature, (b) reflects on different approaches and curriculum materials available for teaching students about AI and ethics (i.e., featuring materials from the MIT Media Lab and Code.org), and (c) articulates future directions for research and recommendations for practitioners seeking to navigate AI and ethics in K-12 settings.
Next, we briefly define the notion of artificial intelligence (AI) and its applications through machine-learning and algorithm systems. As educational and educational technology scholars working in the United States, and at the risk of oversimplifying, we provide only a brief definition of AI below, and recognize that definitions of AI are complex, multidimensional, and contested in the literature [ 9 , 16 , 38 ]; an in-depth discussion of these complexities, however, is beyond the scope of this paper. Second, we describe in more detail five applications of AI in education, outlining their potential benefits for educators and students. Third, we describe the ethical challenges they raise by posing the question: “how and in what ways do algorithms manipulate us?” Fourth, we explain how to support students’ learning about AI and ethics through different curriculum materials and teaching practices in K-12 settings. Our goal here is to provide strategies for practitioners to reap the benefits while navigating the ethical challenges. We acknowledge that in centering this work within U.S. education, we highlight certain ethical issues that educators in other parts of the world may see as less prominent. For example, the European Union (EU) has highlighted ethical concerns and implications of AI, emphasized privacy protection, surveillance, and non-discrimination as primary areas of interest, and provided guidelines on how trustworthy AI should be [ 3 , 15 , 23 ]. Finally, we reflect on future directions for educational and other research that could support K-12 teachers and students in reaping the benefits while mitigating the drawbacks of AI in education.
Definition and applications of artificial intelligence
The pursuit of creating intelligent machines that replicate human behavior has accelerated with the realization of artificial intelligence. With the latest advancements in computer science, a proliferation of definitions and explanations of what counts as AI systems has emerged. For instance, AI has been defined as “the ability of a digital computer or computer-controlled robot to perform tasks commonly associated with intelligent beings” [ 49 ]. This particular definition highlights the mimicry of human behavior and consciousness. Furthermore, AI has been defined as “the combination of cognitive automation, machine learning, reasoning, hypothesis generation and analysis, natural language processing, and intentional algorithm mutation producing insights and analytics at or above human capability” [ 31 ]. This definition incorporates the different sub-fields of AI together and underlines their function while reaching at or above human capability.
Combining these definitions, artificial intelligence can be described as the technology that builds systems to think and act like humans with the ability of achieving goals . AI is mainly known through different applications and advanced computer programs, such as recommender systems (e.g., YouTube, Netflix), personal assistants (e.g., Apple’s Siri), facial recognition systems (e.g., Facebook’s face detection in photographs), and learning apps (e.g., Duolingo) [ 32 ]. To build on these programs, different sub-fields of AI have been used in a diverse range of applications. Evolutionary algorithms and machine learning are most relevant to AI in K-12 education.
Algorithms are the core elements of AI. The history of AI is closely connected to the development of sophisticated and evolutionary algorithms. An algorithm is a set of rules or instructions that is to be followed by computers in problem-solving operations to achieve an intended end goal. In essence, all computer programs are algorithms. They involve thousands of lines of codes which represent mathematical instructions that the computer follows to solve the intended problems (e.g., as computing numerical calculation, processing an image, and grammar-checking in an essay). AI algorithms are applied to fields that we might think of as essentially human behavior—such as speech and face recognition, visual perception, learning, and decision-making and learning. In that way, algorithms can provide instructions for almost any AI system and application we can conceive [ 27 ].
Machine learning
Machine learning is derived from statistical learning methods and uses data and algorithms to perform tasks which are typically performed by humans [ 43 ]. Machine learning is about making computers act or perform without being given any line-by-line step [ 29 ]. The working mechanism of machine learning is the learning model’s exposure to ample amounts of quality data [ 41 ]. Machine-learning algorithms first analyze the data to determine patterns and to build a model and then predict future values through these models. In other words, machine learning can be considered a three-step process. First, it analyzes and gathers the data, and then, it builds a model to excel for different tasks, and finally, it undertakes the action and produces the desired results successfully without human intervention [ 29 , 56 ]. The widely known AI applications such as recommender or facial recognition systems have all been made possible through the working principles of machine learning.
Benefits of AI applications in education
Personalized learning systems, automated assessments, facial recognition systems, chatbots (social media sites), and predictive analytics tools are being deployed increasingly in K-12 educational settings; they are powered by machine-learning systems and algorithms [ 29 ]. These applications of AI have shown promise to support teachers and students in various ways: (a) providing instruction in mixed-ability classrooms, (b) providing students with detailed and timely feedback on their writing products, (c) freeing teachers from the burden of possessing all knowledge and giving them more room to support their students while they are observing, discussing, and gathering information in their collaborative knowledge-building processes [ 26 , 50 ]. Below, we outline benefits of each of these educational applications in the K-12 setting before turning to a synthesis of their ethical challenges and drawbacks.
Personalized learning systems
Personalized learning systems, also known as adaptive learning platforms or intelligent tutoring systems, are one of the most common and valuable applications of AI to support students and teachers. They provide students access to different learning materials based on their individual learning needs and subjects [ 55 ]. For example, rather than practicing chemistry on a worksheet or reading a textbook, students may use an adaptive and interactive multimedia version of the course content [ 39 ]. Comparing students’ scores on researcher-developed or standardized tests, research shows that the instruction based on personalized learning systems resulted in higher test scores than traditional teacher-led instruction [ 36 ]. Microsoft’s recent report (2018) of over 2000 students and teachers from Singapore, the U.S., the UK, and Canada shows that AI supports students’ learning progressions. These platforms promise to identify gaps in students’ prior knowledge by accommodating learning tools and materials to support students’ growth. These systems generate models of learners using their knowledge and cognition; however, the existing platforms do not yet provide models for learners’ social, emotional, and motivational states [ 28 ]. Considering the shift to remote K-12 education during the COVID-19 pandemic, personalized learning systems offer a promising form of distance learning that could reshape K-12 instruction for the future [ 35 ].
Automated assessment systems
Automated assessment systems are becoming one of the most prominent and promising applications of machine learning in K-12 education [ 42 ]. These scoring algorithm systems are being developed to meet the need for scoring students’ writing, exams and assignments, and tasks usually performed by the teacher. Assessment algorithms can provide course support and management tools to lessen teachers’ workload, as well as extend their capacity and productivity. Ideally, these systems can provide levels of support to students, as their essays can be graded quickly [ 55 ]. Providers of the biggest open online courses such as Coursera and EdX have integrated automated scoring engines into their learning platforms to assess the writings of hundreds of students [ 42 ]. On the other hand, a tool called “Gradescope” has been used by over 500 universities to develop and streamline scoring and assessment [ 12 ]. By flagging the wrong answers and marking the correct ones, the tool supports instructors by eliminating their manual grading time and effort. Thus, automated assessment systems deal very differently with marking and giving feedback to essays compared to numeric assessments which analyze right or wrong answers on the test. Overall, these scoring systems have the potential to deal with the complexities of the teaching context and support students’ learning process by providing them with feedback and guidance to improve and revise their writing.
Facial recognition systems and predictive analytics
Facial recognition software is used to capture and monitor students’ facial expressions. These systems provide insights about students’ behaviors during learning processes and allow teachers to take action or intervene, which, in turn, helps teachers develop learner-centered practices and increase student’s engagement [ 55 ]. Predictive analytics algorithm systems are mainly used to identify and detect patterns about learners based on statistical analysis. For example, these analytics can be used to detect university students who are at risk of failing or not completing a course. Through these identifications, instructors can intervene and get students the help they need [ 55 ].
Social networking sites and chatbots
Social networking sites (SNSs) connect students and teachers through social media outlets. Researchers have emphasized the importance of using SNSs (such as Facebook) to expand learning opportunities beyond the classroom, monitor students’ well-being, and deepen student–teacher relations [ 5 ]. Different scholars have examined the role of social media in education, describing its impact on student and teacher learning and scholarly communication [ 6 ]. They point out that the integration of social media can foster students’ active learning, collaboration skills, and connections with communities beyond the classroom [ 6 ]. Chatbots also take place in social media outlets through different AI systems [ 21 ]. They are also known as dialogue systems or conversational agents [ 26 , 52 ]. Chatbots are helpful in terms of their ability to respond naturally with a conversational tone. For instance, a text-based chatbot system called “Pounce” was used at Georgia State University to help students through the registration and admission process, as well as financial aid and other administrative tasks [ 7 ].
In summary, applications of AI can positively impact students’ and teachers’ educational experiences and help them address instructional challenges and concerns. On the other hand, AI cannot be a substitute for human interaction [ 22 , 47 ]. Students have a wide range of learning styles and needs. Although AI can be a time-saving and cognitive aide for teachers, it is but one tool in the teachers’ toolkit. Therefore, it is critical for teachers and students to understand the limits, potential risks, and ethical drawbacks of AI applications in education if they are to reap the benefits of AI and minimize the costs [ 11 ].
Ethical concerns and potential risks of AI applications in education
The ethical challenges and risks posed by AI systems seemingly run counter to marketing efforts that present algorithms to the public as if they are objective and value-neutral tools. In essence, algorithms reflect the values of their builders who hold positions of power [ 26 ]. Whenever people create algorithms, they also create a set of data that represent society’s historical and systemic biases, which ultimately transform into algorithmic bias. Even though the bias is embedded into the algorithmic model with no explicit intention, we can see various gender and racial biases in different AI-based platforms [ 54 ].
Considering the different forms of bias and ethical challenges of AI applications in K-12 settings, we will focus on problems of privacy, surveillance, autonomy, bias, and discrimination (see Fig. 1 ). However, it is important to acknowledge that educators will have different ethical concerns and challenges depending on their students’ grade and age of development. Where strategies and resources are recommended, we indicate the age and/or grade level of student(s) they are targeting (Fig. (Fig.2 2 ).

Potential ethical and societal risks of AI applications in education

Student work from the activity of “Youtube Redesign” (MIT Media Lab, AI and Ethics Curriculum, p.1, [ 45 ])
One of the biggest ethical issues surrounding the use of AI in K-12 education relates to the privacy concerns of students and teachers [ 47 , 49 , 54 ]. Privacy violations mainly occur as people expose an excessive amount of personal information in online platforms. Although existing legislation and standards exist to protect sensitive personal data, AI-based tech companies’ violations with respect to data access and security increase people’s privacy concerns [ 42 , 54 ]. To address these concerns, AI systems ask for users’ consent to access their personal data. Although consent requests are designed to be protective measures and to help alleviate privacy concerns, many individuals give their consent without knowing or considering the extent of the information (metadata) they are sharing, such as the language spoken, racial identity, biographical data, and location [ 49 ]. Such uninformed sharing in effect undermines human agency and privacy. In other words, people’s agency diminishes as AI systems reduce introspective and independent thought [ 55 ]. Relatedly, scholars have raised the ethical issue of forcing students and parents to use these algorithms as part of their education even if they explicitly agree to give up privacy [ 14 , 48 ]. They really have no choice if these systems are required by public schools.
Another ethical concern surrounding the use of AI in K-12 education is surveillance or tracking systems which gather detailed information about the actions and preferences of students and teachers. Through algorithms and machine-learning models, AI tracking systems not only necessitate monitoring of activities but also determine the future preferences and actions of their users [ 47 ]. Surveillance mechanisms can be embedded into AI’s predictive systems to foresee students’ learning performances, strengths, weaknesses, and learning patterns . For instance, research suggests that teachers who use social networking sites (SNSs) for pedagogical purposes encounter a number of problems, such as concerns in relation to boundaries of privacy, friendship authority, as well as responsibility and availability [ 5 ]. While monitoring and patrolling students’ actions might be considered part of a teacher’s responsibility and a pedagogical tool to intervene in dangerous online cases (such as cyber-bullying or exposure to sexual content), such actions can also be seen as surveillance systems which are problematic in terms of threatening students’ privacy. Monitoring and tracking students’ online conversations and actions also may limit their participation in the learning event and make them feel unsafe to take ownership for their ideas. How can students feel secure and safe, if they know that AI systems are used for surveilling and policing their thoughts and actions? [ 49 ].
Problems also emerge when surveillance systems trigger issues related to autonomy, more specifically, the person’s ability to act on her or his own interest and values. Predictive systems which are powered by algorithms jeopardize students and teachers’ autonomy and their ability to govern their own life [ 46 , 47 ]. Use of algorithms to make predictions about individuals’ actions based on their information raise questions about fairness and self-freedom [ 19 ]. Therefore, the risks of predictive analysis also include the perpetuation of existing bias and prejudices of social discrimination and stratification [ 42 ].
Finally, bias and discrimination are critical concerns in debates of AI ethics in K-12 education [ 6 ]. In AI platforms, the existing power structures and biases are embedded into machine-learning models [ 6 ]. Gender bias is one of the most apparent forms of this problem, as the bias is revealed when students in language learning courses use AI to translate between a gender-specific language and one that is less-so. For example, while Google Translate translated the Turkish equivalent of “S he/he is a nurse ” into the feminine form, it also translated the Turkish equivalent of “ She/he is a doctor ” into the masculine form [ 33 ]. This shows how AI models in language translation carry the societal biases and gender-specific stereotypes in the data [ 40 ]. Similarly, a number of problematic cases of racial bias are also associated with AI’s facial recognition systems. Research shows that facial recognition software has improperly misidentified a number of African American and Latino American people as convicted felons [ 42 ].
Additionally, biased decision-making algorithms reveal themselves throughout AI applications in K-12 education: personalized learning, automated assessment, SNSs, and predictive systems in education. Although the main promise of machine-learning models is increased accuracy and objectivity, current incidents have revealed the contrary. For instance, England’s A-level and GCSE secondary level examinations were cancelled due to the pandemic in the summer of 2020 [ 1 , 57 ]. An alternative assessment method was implemented to determine the qualification grades of students. The grade standardization algorithm was produced by the regulator Ofqual. With the assessment of Ofqual’s algorithm based on schools' previous examination results, thousands of students were shocked to receive unexpectedly low grades. Although a full discussion of the incident is beyond the scope of this article [ 51 ] it revealed how the score distribution favored students who attended private or independent schools, while students from underrepresented groups were hit hardest. Unfortunately, automated assessment algorithms have the potential to reconstruct unfair and inconsistent results by disrupting student’s final scores and future careers [ 53 ].
Teaching and understanding AI and ethics in educational settings
These ethical concerns suggest an urgent need to introduce students and teachers to the ethical challenges surrounding AI applications in K-12 education and how to navigate them. To meet this need, different research groups and nonprofit organizations offer a number of open-access resources based on AI and ethics. They provide instructional materials for students and teachers, such as lesson plans and hands-on activities, and professional learning materials for educators, such as open virtual learning sessions. Below, we describe and evaluate three resources: “AI and Ethics” curriculum and “AI and Data Privacy” workshop from the Massachusetts Institute of Technology (MIT) Media Lab as well as Code.org’s “AI and Oceans” activity. For readers who seek to investigate additional approaches and resources for K-12 level AI and ethics interaction, see: (a) The Chinese University of Hong Kong (CUHK)’s AI for the Future Project (AI4Future) [ 18 ]; (b) IBM’s Educator’s AI Classroom Kit [ 30 ], Google’s Teachable Machine [ 25 ], UK-based nonprofit organization Apps for Good [ 4 ], and Machine Learning for Kids [ 37 ].
"AI and Ethics Curriulum" for middle school students by MIT Media Lab
The MIT Media Lab team offers an open-access curriculum on AI and ethics for middle school students and teachers. Through a series of lesson plans and hand-on activities, teachers are guided to support students’ learning of the technical terminology of AI systems as well as the ethical and societal implications of AI [ 2 ]. The curriculum includes various lessons tied to learning objectives. One of the main learning goals is to introduce students to basic components of AI through algorithms, datasets, and supervised machine-learning systems all while underlining the problem of algorithmic bias [ 45 ]. For instance, in the activity “ AI Bingo” , students are given bingo cards with various AI systems, such as online search engine, customer service bot, and weather app. Students work with their partners collaboratively on these AI systems. In their AI Bingo chart, students try to identify what prediction the selected AI system makes and what dataset it uses. In that way, they become more familiar with the notions of dataset and prediction in the context of AI systems [ 45 ].
In the second investigation, “Algorithms as Opinions” , students think about algorithms as recipes, which are created by set of instructions that modify an input to produce an output [ 45 ]. Initially, students are asked to write an algorithm to make the “ best” jelly sandwich and peanut butter. They explore what it means to be “ best” and see how their opinions of best in their recipes are reflected in their algorithms. In this way, students are able to figure out that algorithms can have various motives and goals. Following this activity, students work on the “Ethical Matrix” , building on the idea of the algorithms as opinions [ 45 ]. During this investigation, students first refer back to their developed algorithms through their “best” jelly sandwich and peanut butter. They discuss what counts as the “best” sandwich for themselves (most healthy, practical, delicious, etc.). Then, through their ethical matrix (chart), students identify different stakeholders (such as their parents, teacher, or doctor) who care about their peanut butter and jelly sandwich algorithm. In this way, the values and opinions of those stakeholders also are embedded in the algorithm. Students fill out an ethical matrix and look for where those values conflict or overlap with each other. This matrix is a great tool for students to recognize different stakeholders in a system or society and how they are able to build and utilize the values of the algorithms in an ethical matrix.
The final investigation which teaches about the biased nature of algorithms is “Learning and Algorithmic Bias” [ 45 ]. During the investigation, students think further about the concept of classification. Using Google’s Teachable Machine tool [ 2 ], students explore the supervised machine-learning systems. Students train a cat–dog classifier using two different datasets. While the first dataset reflects the cats as the over-represented group, the second dataset indicates the equal and diverse representation between dogs and cats [ 2 ]. Using these datasets, students compare the accuracy between the classifiers and then discuss which dataset and outcome are fairer. This activity leads students into a discussion about the occurrence of bias in facial recognition algorithms and systems [ 2 ].
In the rest of the curriculum, similar to the AI Bingo investigation, students work with their partners to determine the various forms of AI systems in the YouTube platform (such as its recommender algorithm and advertisement matching algorithm). Through the investigation of “ YouTube Redesign”, students redesign YouTube’s recommender system. They first identify stakeholders and their values in the system, and then use an ethical matrix to reflect on the goals of their YouTube’s recommendation algorithm [ 45 ]. Finally, through the activity of “YouTube Socratic Seminar” , students read an abridged version of Wall Street Journal article by participating in a Socratic seminar. The article was edited to shorten the text and to provide more accessible language for middle school students. They discuss which stakeholders were most influential or significant in proposing changes in the YouTube Kids app and whether or not technologies like auto play should ever exist. During their discussion, students engage with the questions of: “Which stakeholder is making the most change or has the most power?”, “Have you ever seen an inappropriate piece of content on YouTube? What did you do?” [ 45 ].
Overall, the MIT Media Lab’s AI and Ethics curriculum is a high quality, open-access resource with which teachers can introduce middle school students to the risks and ethical implications of AI systems. The investigations described above involve students in collaborative, critical thinking activities that force them to wrestle with issues of bias and discrimination in AI, as well as surveillance and autonomy through the predictive systems and algorithmic bias.
“AI and Data Privacy” workshop series for K-9 students by MIT Media Lab
Another quality resource from the MIT Media Lab’s Personal Robots Group is a workshop series designed to teach students (between the ages 7 and 14) about data privacy and introduce them to designing and prototyping data privacy features. The group has made the content, materials, worksheets, and activities of the workshop series into an open-access online document, freely available to teachers [ 44 ].
The first workshop in the series is “ Mystery YouTube Viewer: A lesson on Data Privacy” . During the workshop, students engage with the question of what privacy and data mean [ 44 ]. They observe YouTube’s home page from the perspective of a mystery user. Using the clues from the videos, students make predictions about what the characters in the videos might look like or where they might live. In a way, students imitate YouTube algorithms’ prediction mode about the characters. Engaging with these questions and observations, students think further about why privacy and boundaries are important and how each algorithm will interpret us differently based on who creates the algorithm itself.
The second workshop in the series is “ Designing ads with transparency: A creative workshop” . Through this workshop, students are able to think further about the meaning, aim, and impact of advertising and the role of advertisements in our lives [ 44 ]. Students collaboratively create an advertisement using an everyday object. The objective is to make the advertisement as “transparent” as possible. To do that, students learn about notions of malware and adware, as well as the components of YouTube advertisements (such as sponsored labels, logos, news sections, etc.). By the end of the workshop, students design their ads as a poster, and they share with their peers.
The final workshop in MIT’s AI and data privacy series is “ Designing Privacy in Social Media Platforms”. This workshop is designed to teach students about YouTube, design, civics, and data privacy [ 44 ]. During the workshop, students create their own designs to solve one of the biggest challenges of the digital era: problems associated with online consent. The workshop allows students to learn more about the privacy laws and how they impact youth in terms of media consumption. Students consider YouTube within the lenses of the Children’s Online Privacy Protections Rule (COPPA). In this way, students reflect on one of the components of the legislation: how might students get parental permission (or verifiable consent)?
Such workshop resources seem promising in helping educate students and teachers about the ethical challenges of AI in education. Specifically, social media such as YouTube are widely used as a teaching and learning tool within K-12 classrooms and beyond them, in students’ everyday lives. These workshop resources may facilitate teachers’ and students’ knowledge of data privacy issues and support them in thinking further about how to protect privacy online. Moreover, educators seeking to implement such resources should consider engaging students in the larger question: who should own one’s data? Teaching students the underlying reasons for laws and facilitating debate on the extent to which they are just or not could help get at this question.
Investigation of “AI for Oceans” by Code.org
A third recommended resource for K-12 educators trying to navigate the ethical challenges of AI with their students comes from Code.org, a nonprofit organization focused on expanding students’ participation in computer science. Sponsored by Microsoft, Facebook, Amazon, Google, and other tech companies, Code.org aims to provide opportunities for K-12 students to learn about AI and machine-learning systems [ 20 ]. To support students (grades 3–12) in learning about AI, algorithms, machine learning, and bias, the organization offers an activity called “ AI for Oceans ”, where students are able to train their machine-learning models.
The activity is provided as an open-access tutorial for teachers to help their students explore how to train, model and classify data , as well as to understand how human bias plays a role in machine-learning systems. During the activity, students first classify the objects as either “fish” or “not fish” in an attempt to remove trash from the ocean. Then, they expand their training data set by including other sea creatures that belong underwater. Throughout the activity, students are also able to watch and interact with a number of visuals and video tutorials. With the support of their teachers, they discuss machine learning, steps and influences of training data, as well as the formation and risks of biased data [ 20 ].
Future directions for research and teaching on AI and ethics
In this paper, we provided an overview of the possibilities and potential ethical and societal risks of AI integration in education. To help address these risks, we highlighted several instructional strategies and resources for practitioners seeking to integrate AI applications in K-12 education and/or instruct students about the ethical issues they pose. These instructional materials have the potential to help students and teachers reap the powerful benefits of AI while navigating ethical challenges especially related to privacy concerns and bias. Existing research on AI in education provides insight on supporting students’ understanding and use of AI [ 2 , 13 ]; however, research on how to develop K-12 teachers’ instructional practices regarding AI and ethics is still in its infancy.
Moreover, current resources, as demonstrated above, mainly address privacy and bias-related ethical and societal concerns of AI. Conducting more exploratory and critical research on teachers’ and students’ surveillance and autonomy concerns will be important to designing future resources. In addition, curriculum developers and workshop designers might consider centering culturally relevant and responsive pedagogies (by focusing on students’ funds of knowledge, family background, and cultural experiences) while creating instructional materials that address surveillance, privacy, autonomy, and bias. In such student-centered learning environments, students voice their own cultural and contextual experiences while trying to critique and disrupt existing power structures and cultivate their social awareness [ 24 , 36 ].
Finally, as scholars in teacher education and educational technology, we believe that educating future generations of diverse citizens to participate in the ethical use and development of AI will require more professional development for K-12 teachers (both pre-service and in-service). For instance, through sustained professional learning sessions, teachers could engage with suggested curriculum resources and teaching strategies as well as build a community of practice where they can share and critically reflect on their experiences with other teachers. Further research on such reflective teaching practices and students’ sense-making processes in relation to AI and ethics lessons will be essential to developing curriculum materials and pedagogies relevant to a broad base of educators and students.
This work was supported by the Graduate School at Michigan State University, College of Education Summer Research Fellowship.
Data availability
Code availability, declarations.
The authors declare that they have no conflict of interest.
Publisher's Note
Springer Nature remains neutral with regard to jurisdictional claims in published maps and institutional affiliations.
REPORT on artificial intelligence in education, culture and the audiovisual sector
19.4.2021 - ( 2020/2017(INI) )
Committee on Culture and Education Rapporteur: Sabine Verheyen Rapporteur for the opinion (*): Ondřej Kovařík, Committee on Civil Liberties, Justice and Home Affairs (*) Associated committee – Rule 57 of the Rules of Procedure
MOTION FOR A EUROPEAN PARLIAMENT RESOLUTION
Explanatory statement.
- OPINION OF THE COMMITTEE ON CIVIL LIBERTIES, JUSTICE AND HOME AFFAIRS
- OPINION OF THE COMMITTEE ON THE INTERNAL MARKET AND CONSUMER PROTECTION
- OPINION OF THE COMMITTEE ON LEGAL AFFAIRS
- OPINION OF THE COMMITTEE ON WOMEN'S RIGHTS AND GENDER EQUALITY
INFORMATION ON ADOPTION IN COMMITTEE RESPONSIBLE
Final vote by roll call in committee responsible.
on artificial intelligence in education, culture and the audiovisual sector
( 2020/2017(INI) )
The European Parliament ,
– having regard to the Charter of Fundamental Rights of the European Union,
– having regard to Articles 165, 166 and 167 of the Treaty on the Functioning of the European Union,
– having regard to the Council conclusions of 9 June 2020 on shaping Europe’s digital future [1] ,
– having regard to the opinion of the European Economic and Social Committee of 19 September 2018 on the digital gender gap [2] ,
– having regard to the Commission proposal for a regulation of the European Parliament and of the Council of 6 June 2018 establishing the Digital Europe Programme for the period 2021-2027 ( COM(2018)0434 ),
– having regard to the Commission communication of 30 September 2020 on the Digital Education Action Plan 2021-2027: Resetting education and training for the digital age ( COM(2020)0624 ),
– having regard to the Commission communication of 30 September 2020 on achieving the European Education Area by 2025 ( COM(2020)0625 ),
– having regard to the Commission report of 19 February 2020 on the safety and liability implications of artificial intelligence, the Internet of Things and robotics ( COM(2020)0064 ),
– having regard to the Commission white paper of 19 February 2020 entitled ‘Artificial Intelligence – A European approach to excellence and trust’ ( COM(2020)0065 ),
– having regard to the Commission communication of 19 February 2020 on a European strategy for data ( COM(2020)0066 ),
– having regard to the Commission communication of 25 April 2018 entitled ‘Artificial Intelligence for Europe’ ( COM(2018)0237 ),
– having regard to the Commission communication of 17 January 2018 on the Digital Education Action Plan ( COM(2018)0022 ),
– having regard to the report of the Commission High-Level Expert Group on Artificial Intelligence of 8 April 2019 entitled ‘Ethics Guidelines for Trustworthy AI’,
– having regard to its resolution of 12 February 2019 on a comprehensive European industrial policy on artificial intelligence and robotics [3] ,
– having regard to its resolution of 11 September 2018 on language equality in the digital age [4] ,
– having regard to its resolution of 12 June 2018 on modernisation of education in the EU [5] ,
– having regard to its resolution of 16 February 2017 with recommendations to the Commission on Civil Law Rules on Robotics [6] ,
– having regard to its resolution of 1 June 2017 on digitising European industry [7] ,
– having regard to the briefing of its Policy Department for Structural and Cohesion Policies of May 2020 on the use of artificial intelligence in the cultural and creative sectors,
– having regard to the in-depth analysis of its Policy Department for Structural and Cohesion Policies of May 2020 on the use of artificial intelligence in the audiovisual sector,
– having regard to the study of its Policy Department for Citizens’ Rights and Constitutional Affairs of April 2020 on the education and employment of women in science, technology and the digital economy, including AI and its influence on gender equality,
– having regard to Rule 54 of its Rules of Procedure,
– having regard to the opinions of the Committee on Civil Liberties, Justice and Home Affairs, the Committee on the Internal Market and Consumer Protection, the Committee on Legal Affairs and the Committee on Women’s Rights and Gender Equality,
– having regard to the report of the Committee on Culture and Education (A9-0127/2021),
A. whereas artificial intelligence (AI) technologies, which may have a direct impact on our societies, are being developed at a fast pace and are increasingly being used in almost all areas of our lives, including education, culture and the audiovisual sector; whereas ethical AI is likely to help improve labour productivity and help accelerate economic growth;
B. whereas the development, deployment and use of AI, including the software, algorithms and data used and produced by it, should be guided by the ethical principles of transparency, explainability, fairness, accountability and responsibility;
C. whereas public investment in AI in the Union has been vastly lagging behind other major economies; whereas underinvestment in AI will be likely to have an impact on the Union’s competitiveness across all sectors;
D. whereas an integrated approach to AI and the availability, collection and interpretation of high-quality, trustworthy, fair, transparent, reliable, secure and compatible data are essential for the development of ethical AI;
E. whereas Article 21 of the EU Charter of Fundamental Rights prohibits discrimination on a wide range of grounds; whereas multiple forms of discrimination should not be replicated in the development, deployment and use of AI systems;
F. whereas gender equality is a core principle of the Union enshrined in the Treaties and should be reflected in all Union policies, including in education, culture and the audiovisual sector, as well as in the development of technologies such as AI;
G. whereas past experiences, especially in technical fields, have shown that developments and innovations are often based mainly on male data and that women’s needs are not fully reflected; whereas addressing these biases requires greater vigilance, technical solutions and the development of clear requirements of fairness, accountability and transparency;
H. whereas incomplete and inaccurate data sets, the lack of gender-disaggregated data and incorrect algorithms can distort the processing of an AI system and jeopardise the achievement of gender equality in society; whereas data on disadvantaged groups and intersectional forms of discrimination tends to be incomplete or even absent;
I. whereas gender inequalities, stereotypes and discrimination can also be created and replicated through the language and images disseminated by the media and AI-powered applications; whereas education, cultural programmes and audiovisual content have considerable influence in shaping people’s beliefs and values and are a fundamental tool for combatting gender stereotypes, decreasing the digital gender gap and establishing strong role models; whereas an ethical and regulatory framework must be in place ahead of implementing automatised solutions for these key areas in society;
J. whereas science and innovation can bring life-changing benefits, especially for those who are furthest behind, such as women and girls living in remote areas; whereas scientific education is important for obtaining skills, decent work and jobs of the future, as well as for breaking with gender stereotypes that regard these as stereotypically masculine fields; whereas science and scientific thinking are key to democratic culture, which in turn is fundamental for advancing gender equality;
K. whereas one woman in ten in the Union has already suffered some form of cyber-violence since the age of 15 and whereas cyber-harassment remains a concern in the development of AI, including in education; whereas cyber-violence is often directed at women in public life, such as activists, women politicians and other public figures; whereas AI and other emerging technologies can play an important role in preventing cyber-violence against women and girls and educating people;
L. whereas the Union and its Member States have a particular responsibility to harness, promote and enhance the added value of AI technologies and to make sure that these technologies are safe and serve the well-being and general interest of Europeans; whereas these technologies can make a huge contribution to achieving our common goal of improving the lives of our citizens and fostering prosperity in the Union by helping to develop better strategies and innovation in a number of areas, namely in education, culture and the audiovisual sector;
M. whereas most AI is based on open-source software, which means that source codes can be inspected, modified and enhanced;
N. whereas certain adjustments to specific existing EU legislative instruments may be necessary to reflect the digital transformation and address new challenges posed by the use of AI technologies in education, culture and the audiovisual sector, such as the protection of personal data and privacy, combatting discrimination, promoting gender equality, and respecting intellectual property rights (IPR), environmental protection and consumers’ rights;
O. whereas it is important to provide the audiovisual sector with access to data from the global platforms and major players in order to ensure a level playing field;
P. whereas AI and future applications or inventions made with the help of AI can have a dual nature, much like with any other technology; whereas AI and related technologies raise many concerns regarding the ethics and transparency of their development, deployment and use, notably on data collection, use and dissemination; whereas the benefits and risks of AI technologies in education, culture and the audiovisual sector must be carefully assessed and their effects on all aspects of society thoroughly and continuously analysed, without undermining their potential;
Q. whereas education aims to achieve human potential, creativity and authentic social change, while using data-driven AI systems incorrectly may hinder human and social development;
R. whereas education and educational opportunities are a fundamental right; whereas the development, deployment and use of AI technologies in the education sector should be classified as high risk and subject to stricter requirements on safety, transparency, fairness and accountability;
S. whereas high-quality, fast and secure pervasive connectivity, broadband, high-capacity networks, IT expertise, digital skills, digital equipment and infrastructure, as well as societal acceptance and a targeted and accommodating policy framework, are some of the preconditions for the broad and successful deployment of AI in the Union; whereas it is essential that such infrastructure and equipment be deployed equally across the Union in order to tackle the persistent digital gap between its regions and citizens;
T. whereas addressing the gender gap in science, technology, engineering, arts and maths (STEAM) subjects is an absolute necessity to ensure that the whole of society is equally and fairly represented when developing, deploying and using AI technologies, including the software, algorithms and data used and produced by them;
U. whereas it is essential to ensure that all people in the Union acquire the necessary skills from an early age in order to better understand the capabilities and limitations of AI, to prepare themselves for the increasing presence of AI and related technologies in all aspects of human activity, and to be able to fully embrace the opportunities that they offer; whereas the widespread acquisition of digital skills across all parts of society in the Union is a precondition for achieving a fair digital transformation beneficial to all;
V. whereas, with that aim in view, the Member States must invest in digital education and media training, equipping schools with the proper infrastructure and the necessary end devices, and place greater emphasis on the teaching of digital skills and capabilities as part of school curricula;
W. whereas AI and related technologies can be used to improve learning and teaching methods, notably by helping education systems to use fair data to improve educational equity and quality, while promoting tailor-made curricula and better access to education and improving and automating certain administrative tasks; whereas equal and fair access to digital technologies and high-speed connectivity are required in order to make the use of AI beneficial to the whole of society; whereas it is of the utmost importance to ensure that digital education is accessible to all, including those from disadvantaged backgrounds and people with disabilities; whereas learning outcomes do not depend on technology per se, but on how teachers can use technology in pedagogically meaningful ways;
X. whereas AI has particular potential to offer solutions for the day-to-day challenges of the education sector, such as the personalisation of learning, monitoring learning difficulties, the automation of subject-specific content/knowledge, providing better professional training and supporting the transition to a digital society;
Y. whereas AI could have practical applications in terms of reducing the administrative work of educators and educational institutions, freeing up time for their core teaching and learning activities;
Z. whereas new AI-based applications in education are facilitating progress in a variety of disciplines, such as language learning and maths;
AA. whereas AI-enabled personalised learning experiences can not only help to increase students’ motivation and enable them to reach their full potential, but also reduce drop-out rates;
AB. whereas AI can increasingly help make teachers more effective by giving them a better understanding of students’ learning methods and styles and helping them to identify learning difficulties and better assess individual progress;
AC. whereas the Union’s digital labour market is lacking almost half a million experts in big data sciences and data analysis, who are intrinsic to the development and use of quality and trustworthy AI;
AD. whereas the application of AI in education raises concerns around the ethical use of data, learners’ rights, data access and protection of personal data, and therefore entails risks to fundamental rights such as the creation of stereotyped models of learners’ profiles and behaviour that could lead to discrimination or risks of doing harm by the scaling-up of bad pedagogical practices;
AE. whereas culture plays a central role in the use of AI technologies at scale and is emerging as a key discipline for cultural heritage thanks to the development of innovative technologies and tools and their effective application to respond to the needs of the sector;
AF. whereas AI technologies can be used to promote and protect cultural heritage, including by using digital tools to preserve historical sites and finding innovative ways to make the datasets of cultural artefacts held by cultural institutions across the Union more widely and easily accessible, while allowing users to navigate the vast amount of cultural and creative content; whereas the promotion of interoperability standards and frameworks is key in this regard;
AG. whereas the use of AI technologies for cultural and creative content, notably media content and tailored content recommendations, raises issues around data protection, discrimination and cultural and linguistic diversity, risks producing discriminatory output based on biased entry data, and could restrict diversity of opinion and media pluralism;
AH. whereas AI-based personalised content recommendations can often better target individuals’ specific needs, including cultural and linguistic preferences; whereas AI can help to promote linguistic diversity in the Union and contribute to the wider dissemination of European audiovisual works, in particular through automatic subtitling and dubbing of audiovisual content in other languages; whereas making media content across languages is therefore fundamental to support cultural and linguistic diversity;
AI. whereas AI drives innovation in newsrooms by automating a variety of mundane tasks, interpreting data and even generating news such as weather forecasts and sports results;
AJ. whereas Europe’s linguistic diversity means that promoting computational linguistics for rights-based AI offers specific potential for innovations which can be used to make global cultural and information exchanges in the digital age democratic and non-discriminatory;
AK. whereas AI technologies may have the potential to benefit special needs education, as well as the accessibility of cultural and creative content for people with disabilities; whereas AI enables solutions such as speech recognition, virtual assistants and digital representations of physical objects; whereas digital creations are already playing their part in making such content available to people with disabilities;
AL. whereas AI applications are omnipresent in the audiovisual sector, in particular on audiovisual content platforms;
AM. whereas AI technologies therefore contribute to the creation, planning, management, production, distribution, localisation and consumption of audiovisual media products;
AN. whereas while AI can be used to generate fake content, such as ‘deepfakes’, which are growing exponentially and constitute an imminent threat to democracy, it can also be used as an invaluable tool for identifying and immediately combatting such malicious activity, for example through real-time fact checking or labelling of content; whereas most deepfake material is easy to spot; whereas at the same time, AI-powered detection tools are generally successful in flagging and filtering out such content; whereas there is a lack of a legal framework on this issue;
General observations
1. Underlines the strategic importance of AI and related technologies for the Union; stresses that the approach to AI and its related technologies must be human-centred and anchored in human rights and ethics, so that AI genuinely becomes an instrument that serves people, the common good and the general interest of citizens;
2. Underlines that the development, deployment and use of AI in education, culture and the audiovisual sector must fully respect fundamental rights, freedoms and values, including human dignity, privacy, the protection of personal data, non-discrimination and freedom of expression and information, as well as cultural diversity and intellectual property rights, as enshrined in the Union Treaties and the Charter of Fundamental Rights;
3. Asserts that education, culture and the audiovisual sector are sensitive areas as far as the use of AI and related technologies is concerned, as they have the potential to impact on the cornerstones of the fundamental rights and values of our society; stresses, therefore, that ethical principles should be observed in the development, deployment and use of AI and related technologies in these sectors, including the software, algorithms and data used and produced by them;
4. Recalls that algorithms and AI should be ‘ethical by design’, with no built-in bias, in a way that guarantees maximum protection of fundamental rights;
5. Reiterates the importance of developing quality, compatible and inclusive AI and related technologies for use in deep learning which respect and defend the values of the Union, notably gender equality, multilingualism and the conditions necessary for intercultural dialogue, as the use of low-quality, outdated, incomplete or incorrect data may lead to poor predictions and in turn discrimination and bias; highlights that it is essential to develop capabilities at both national and Union level to improve data collection, safety, systematisation and transferability, without harming privacy; takes note of the Commission’s proposal to create a single European data space;
6. Recalls that AI may give rise to biases and thus to various forms of discrimination based on sex, race, colour, ethnic or social origin, genetic features, language, religion or belief, political or any other opinion, membership of a national minority, property, birth, disability, age or sexual orientation; recalls, in this regard, that the rights of all people must be ensured and that AI and related technologies must not be discriminatory in any form;
7. Emphasises that such bias and discrimination can arise from already biased datasets that reflect existing discrimination in society; recalls, in this context, that it is essential to involve the relevant stakeholders, including civil society, to prevent gender, social and cultural biases from being inadvertently included in AI algorithms, systems and applications; stresses the need to work on the most efficient way of reducing bias in AI systems in line with ethical and non-discrimination standards; underlines that the datasets used to train AI should be as broad as possible in order to represent society in the best and most relevant way, that the outputs should be reviewed to avoid all forms of stereotypes, discrimination and bias and that, where appropriate, AI should be used to identify and rectify human bias wherever it exists; calls on the Commission to encourage and facilitate the sharing of de-biasing strategies for data;
8. Calls on the Commission and the Member States to take into account ethical aspects, including from a gender perspective, when developing AI policy and legislation, and, if necessary, to adapt the current legislation, including Union programmes and ethical guidelines for the use of AI;
9. Calls on the Commission and the Member States to devise measures that fully incorporate the gender dimension, such as awareness-raising campaigns, training and curricula, which should provide information to citizens on how algorithms operate and their impact on their daily lives; further calls on them to nurture gender-equal mindsets and working conditions that lead to the development of more inclusive technology products and work environments; urges the Commission and the Member States to ensure the inclusion of digital skills and AI training in school curricula and to make them accessible to all, as a way to close the digital gender divide;
10. Stresses the need for training for workers and educators dealing with AI to promote the ability to identify and correct gender-discriminatory practices in the workplace and in education, and for workers developing AI systems and applications to identify and remedy gender-based discrimination in the AI systems and applications they develop; calls for the establishment of clear responsibilities in companies and educational institutions to ensure that there is no gender-based discrimination in the workplace or educational context; highlights that genderless images of AI and robots should be used for educational and cultural purposes, unless gender is a key factor for some reason;
11. Highlights the importance of the development and deployment of AI applications in education, culture and the audiovisual sector in collecting gender-disaggregated and other equality data, and of applying modern machine learning de-biasing techniques, if needed, to correct gender stereotypes and gender biases which may have negative impacts;
12. Calls on the Commission to include education in the regulatory framework for high-risk AI applications, given the importance of ensuring that education continues to contribute to the public good, as well as the high sensitivity of data on pupils, students and other learners; emphasises that in the education sector, this deployment should involve educators, learners and the wider society and should take into account the needs of all and the expected benefits in order to ensure that AI is used purposefully and ethically;
13. Calls on the Commission to encourage the use of Union programmes such as Horizon Europe, Digital Europe and Erasmus+ to promote multidisciplinary research, pilot projects, experiments and the development of tools including training, for the identification of gender biases in AI, as well as awareness-raising campaigns for the general public;
14. Stresses the need to create diverse teams of developers and engineers to work alongside the main actors in education, culture and the audiovisual sector in order to prevent gender or social bias from being inadvertently included in AI algorithms, systems and applications; stresses the need to consider the variety of different theories through which AI has been developed to date and could be further advanced in the future;
15. Points out that taking due care to eliminate bias and discrimination against particular groups, including gender stereotypes, should not halt technological progress;
16. Reiterates the importance of fundamental rights and the overarching supremacy of the legislation of data and privacy protection, which is imperative when dealing with such technologies; recalls that data protection and privacy can be particularly affected by AI, in particular children’s data; underlines that the principles established in the General Data Protection Regulation (GDPR) [8] are binding for the deployment of AI in that regard; recalls, moreover, that all AI applications must fully respect Union data protection law, namely the GDPR and the ePrivacy Directive [9] ; stresses the right to obtain human intervention when AI and related technologies are being used;
17. Calls on the Commission and the Member States to implement an obligation of transparency and explainability of AI-based automated individual decisions taken within the framework of prerogatives of public power, and to implement penalties to enforce this; calls for the implementation of systems which use human verification and intervention by default, and for due process, including the right of appeal and redress as well as access to remedies;
18. Notes the potentially negative impact of personalised advertising, in particular micro-targeted and behavioural advertising, and of the assessment of individuals, especially minors, without their consent, by interfering in the private life of individuals, asking questions as to the collection and use of the data used to personalise advertising, and offering products or services or setting prices; calls on the Commission, therefore, to introduce strict limitations on targeted advertising based on the collection of personal data, starting with a ban on cross-platform behavioural advertising, without harming small and medium-sized enterprises (SMEs); recalls that the ePrivacy Directive currently only allows targeted advertising subject to opt-in consent, otherwise making it illegal; calls on the Commission to prohibit the use of discriminatory practices for the provision of services or products;
19. Stresses the need for media organisations to be informed about the main parameters of algorithm-based AI systems that determine ranking and search results on third-party platforms, and for users to be informed about the use of AI in decision-making services and empowered to set their privacy parameters via transparent and understandable measures;
20. Stresses that AI can support content creation in education, culture and the audiovisual sector, alongside information and educational platforms, including listings of different kinds of cultural objects and a multitude of data sources; notes the risks of IPR infringement when blending AI and different technologies with a multiplicity of sources (documents, photos, films) to improve how that data is displayed, researched and visualised; calls for AI to be used to ensure a high level of IPR protection within the current legislative framework, such as by alerting individuals and businesses if they are in danger of inadvertently infringing the rules, or by assisting IPR rights holders if the rules are actually infringed; emphasises, therefore, the importance of having an appropriate legal framework at Union level for the protection of IPR in connection with the use of AI;
21. Stresses the need to strike a balance between, on the one hand, the development of AI systems and their use in education, culture and the audiovisual sector and, on the other, measures to safeguard competition and market competitiveness for AI companies in these sectors; emphasises, in this regard, the need to encourage companies to invest in the innovation of AI systems used in these sectors, while also ensuring that those providing such applications do not obtain a market monopoly; underlines the need for AI to be made widely available to the cultural and creative sectors and industries (CCSI) across Europe in order to maintain a level playing field and fair competition for all stakeholders and actors in Europe; calls on the Commission and the Member States, when taking decisions on competition policy, including mergers, to take greater account of the role played by data and algorithms in the concentration of market power;
22. Stresses the need to systematically address the social, ethical and legal issues raised by the development, deployment and use of AI such as the transparency and accountability of algorithms, non-discrimination, equal opportunities, freedom and diversity of opinion, media pluralism and the ownership, collection, use and dissemination of data and content; recommends that common European guidelines and standards to protect privacy be devised while making effective use of the data available; calls for transparency in the development and accountability in the use of algorithms;
23. Calls on the Commission to put forward a comprehensive set of provisions designed to regulate AI applications on a horizontal basis and to supplement them with sector-specific rules, for example for audiovisual media services;
24. Stresses the need for investment in research and innovation on the development, deployment and use of AI and its applications in education, culture and the audiovisual sector; highlights the importance of public investment in these services and the complementary added value provided by public-private partnerships in order to achieve this objective and deploy the full potential of AI in these sectors, in particular education, in view of the substantial amount of private investment made in recent years; calls on the Commission to find additional funding to promote research and innovation into AI applications in these sectors;
25. Underlines that algorithmic systems can be an enabler for reducing the digital divide in an accelerated way, but unequal deployment risks creating new divides or accelerating the deepening of existing ones; expresses its concern that knowledge and infrastructure are not developed in a consistent way across the Union, which limits the accessibility of products and services that rely on AI, in particular in sparsely populated and socio‑economically vulnerable areas; calls on the Commission to ensure cohesion in the sharing of the benefits of AI and related technologies;
26. Calls on the Commission to establish requirements for the procurement and deployment of AI and related technologies by Union public sector bodies in order to ensure compliance with Union law and fundamental rights; highlights the added value of using instruments such as public consultations and impact assessments prior to the procurement or deployment of AI systems, as recommended in the report of the Special Rapporteur to the UN General Assembly on AI and its impact on freedom of opinion and expression [10] ; encourages public authorities to incentivise the development and deployment of AI by public funding and public procurement; stresses the need to strengthen the market by providing SMEs with the opportunity to participate in the procurement of AI applications in order to ensure the involvement of technology companies of all sizes and thus guarantee resilience and competition;
27. Calls for independent audits to be conducted regularly to examine whether the AI applications being used and the related checks and balances are in accordance with specified criteria, and for those audits to be supervised by independent and sufficient overseeing authorities; calls for specific stress tests to assist and enforce compliance;
28. Notes the benefits and risks of AI in terms of cybersecurity and its potential in combatting cybercrime, and emphasises the need for any AI solutions to be resilient to cyberattacks while respecting Union fundamental rights, especially the protection of personal data and privacy; stresses the importance of monitoring the safe use of AI and the need for close collaboration between the public and private sectors to counter user vulnerabilities and the dangers arising in this connection; calls on the Commission to evaluate the need for better prevention in terms of cybersecurity and mitigation measures thereof;
29. Highlights that the COVID-19 pandemic crisis can be considered as a probation period for the development, deployment and use of digital and AI-related technologies in education and culture, as exemplified by the many online schooling platforms and online tools for cultural promotion employed across the Member States; calls on the Commission, therefore, to take stock of those examples when considering a common Union approach to the increased use of such technological solutions;
30. Recalls the importance of strengthening digital skills and achieving a high standard of media, digital and information literacy at Union level as a prerequisite for the use of AI in education; underlines the need, in this regard, to ensure Union-wide digital and AI literacy, in particular through the development of training opportunities for teachers; insists that the use of AI technologies in schools should help to narrow the social and regional digital gap; welcomes the Commission’s updated Digital Education Action Plan, which addresses the use of AI in education; calls on the Commission, in that regard, to make digital capabilities, media literacy and training and AI-related skills the priorities of this plan, while raising awareness about the potential misuses and malfunctioning of AI; calls on the Commission, in that connection, to place special emphasis on children and young people in precarious situations, as they need particular support in the area of digital education; urges the Commission to duly address AI and robotics initiatives in education in its forthcoming AI legislative proposals; urges the Member States to invest in digital equipment in schools, using Union funds to this end;
31. Highlights that the use of AI in education systems brings a wide range of possibilities, opportunities and tools for making it more innovative, inclusive, efficient and increasingly effective by introducing new high-quality learning methods that are quick, personalised and student-centric; stresses, however, that as it will impact education and social inclusion, the availability of such tools must be ensured for all social groups by establishing equal access to education and learning and leaving no one behind, especially people with disabilities;
32. Underlines that in order to engage with AI both critically and effectively, citizens need at least a basic understanding of this technology; calls on the Member States to integrate awareness-raising campaigns about AI in their actions on digital literacy; calls on the Commission and the Member States to promote digital literacy plans and forums for discussion to involve citizens, parents and students in a democratic dialogue with public authorities and stakeholders concerning the development, deployment and use of AI technologies in education systems; stresses the importance of providing educators, trainers and others with the right tools and know-how with regard to AI and related technologies in terms of what they are, how they are used and how to use them properly and in accordance with the law, in order to avoid IPR infringements; highlights, in particular, the importance of digital literacy for people working in the education and training sectors and of improving digital training for the elderly, bearing in mind that the younger generations already have a basic notion of these technologies, having grown up with them;
33. Stresses that the real objective of AI in education systems should be to make education as individualised as possible, offering students personalised academic paths in line with their strengths and weaknesses and didactic material tailored to their characteristics, while maintaining educational quality and the integrating principle of our education systems;
34. Recalls the fundamental and multifaceted role that teachers play in education and in making it inclusive, especially in early childhood, where skills are acquired that will enable students to progress throughout their lives, such as in personal relations, study skills, empathy and cooperative work; stresses, therefore, that AI technologies cannot be used to the detriment or at the expense of in-person education, as teachers must not be replaced by any AI or AI-related technologies;
35. Stresses that the learning benefits of using AI in education will depend not only on AI itself, but on how teachers use AI in the digital learning environment to meet the needs of pupils, students and teachers; points out, therefore, the need for AI programmers to involve teaching communities in the development, deployment and use of AI technologies where possible, creating a nexus environment to form connections and cooperation between AI programmers, developers, companies, schools, teachers and other public and private stakeholders in order to create AI technologies that are suitable for real-life educational environments, reflect the age and developmental readiness of each learner and meet the highest ethical standards; highlights that educational institutions should only deploy trustworthy, ethical, human-centred technologies which are auditable at every stage of their lifecycle by public authorities and civil society; emphasises the advantages of free and open-source solutions in this regard; calls for schools and other educational establishments to be provided with the financial and logistical support as well as the expertise required to introduce solutions for the learning of the future;
36. Highlights, moreover, the need to continuously train teachers so they can adapt to the realities of AI-powered education and acquire the necessary knowledge and skills to use AI technologies in a pedagogical and meaningful way, enabling them to fully embrace the possibilities offered by AI and to understand its limitations; calls for digital teaching to be part of every teacher’s training in the future and calls for teachers and people working in education and training to be given the opportunity to continue their training in digital teaching throughout their lives; calls, therefore, for the development of training programmes in AI for teachers in all fields and across Europe; highlights, furthermore, the importance of reforming teaching programmes for new generations of teachers allowing them to adapt to the realities of AI-powered education, as well as the importance of drawing up and updating handbooks and guidelines on AI for teachers;
37. Is concerned about the lack of specific higher education programmes for AI and the lack of public funding for AI across the Member States; believes that this is putting Europe’s future digital ambitions at risk;
38. Is worried about the fact that few AI researchers are pursuing an academic career as tech firms can offer better pay and less bureaucracy for research; believes that part of the solution would be to direct more public money towards AI research at universities;
39. Underlines the importance of equipping people with general digital skills from childhood onwards in order to close the qualifications gap and better integrate certain population groups into the digital labour market and digital society; points out that it will become more and more important to train highly skilled professionals from all backgrounds in the field of AI, ensure the mutual recognition of such qualifications throughout the Union, and upskill the existing and future workforce to enable it to cope with the future realities of the labour market; encourages the Member States, therefore, to assess their educational offer and to upgrade it with AI-related skills, where necessary, and to put in place specific curricula for AI developers, while also including AI in traditional curricula; highlights the need to ensure mutual recognition of professional qualifications in AI skills across the Union, as several Member States are upgrading their educational offer with AI-related skills and putting in place specific curricula for AI developers; welcomes the Commission’s efforts to include digital skills as one of the qualifications requirements for certain professions harmonised at Union level under the Professional Qualifications Directive [11] ; stresses the need for these to be in line with the assessment list of the ethical guidelines for trustworthy AI, and welcomes the Commission’s proposal to transform this list into an indicative curriculum for AI developers; recalls the special needs of vocational education and training (VET) with regard to AI and calls for a collaborative approach across Europe to enhance the potential offered by AI in VET; underlines the importance of training highly skilled professionals in this area, including ethical aspects in curricula, and of supporting underrepresented groups in this field, as well as of creating incentives for those professionals to seek work within the Union; recalls that women are underrepresented in AI and that this may create significant gender imbalances in the future labour market;
40. Stresses the need for governments and educational institutions to rethink, rework and adapt their educational curricula to the needs of the 21st century by devising educational programmes that place greater emphasis on STEAM subjects in order to prepare learners and consumers for the increasing prevalence of AI and facilitate the acquisition of cognitive skills; underlines, in this regard, the importance of diversifying this sector and of encouraging students, especially women and girls, to enrol in STEAM courses, in particular in robotics and AI-related subjects; calls for more financial and scientific resources to motivate skilled people to stay in the Union while attracting those with skills from third countries; notes, furthermore, the considerable number of start-ups working with AI and developing AI technologies; stresses that SMEs will require additional support and AI-related training to comply with digital and AI-related regulation;
41. Notes that automation and the development of AI may drastically and irreversibly change employment; emphasises that priority should be given to tailoring skills to the needs of the future job market, in particular in education and the CCSI; underlines, in this context, the need to upskill the future workforce; stresses, furthermore, the importance of deploying AI to reskill and upskill the European labour market in the CCSI, in particular in the audiovisual sector, which has already been severely impacted by the COVID-19 crisis;
42. Calls on the Commission to assess the level of risk of AI deployment in the education sector in order to ascertain whether AI applications in education should be included in the regulatory framework for high risk and subject to stricter requirements on safety, transparency, fairness and accountability, in view of the importance of ensuring that education continues to contribute to the public good and the acute sensitivity of data on pupils, students and other learners; underlines that datasets used to train AI should be reviewed in order to avoid reinforcing certain stereotypes and other kinds of bias;
43. Calls on the Commission to propose a futureproof legal framework for AI so as to provide legally binding ethical measures and standards to ensure fundamental rights and freedoms and the development of trustworthy, ethical and technically robust AI applications, including integrated digital tools, services and products such as robotics and machine learning, with particular regard to education; calls for the data used and produced by AI applications in education to be accessible, interoperable and of high quality, and to be shared with the relevant public authorities in an accessible way and with respect for copyright and trade secrets legislation; recalls that children constitute a vulnerable group who deserve particular attention and protection; stresses that while AI can benefit education, it is necessary to take into account its technological, regulatory and social aspects, with adequate safeguards and a human-centred approach that ultimately ensures that human beings are always able to control and correct the system’s decisions; points out, in this regard, that teachers must control and supervise any deployment and use of AI technologies in schools and universities when interacting with pupils and students; recalls that AI systems must not take any final decision that could affect educational opportunities, such as students’ final evaluation, without full human supervision; recalls that automated decisions about natural persons based on profiling, where they have legal or similar effects, must be strictly limited and always require the right to human intervention and the right to an explanation under the GDPR; underlines that this should be strictly adhered to, especially in the education system, where decisions about future chances and opportunities are taken;
44. Expresses serious concern that schools and other education providers are becoming increasingly dependent on educational technology (edtech) services, including AI applications, provided by a few private companies that enjoy a dominant market position; believes that this should be scrutinised through Union competition rules; stresses the importance, in this regard, of supporting the uptake of AI by SMEs in education, culture and the audiovisual sector through the appropriate incentives that create a level playing field; calls, in this context, for investment in European IT companies in order to develop the necessary technologies within the Union, given that the major companies that currently provide AI are based outside the Union; strongly recalls that the data of minors is strictly protected by the GDPR and can only be processed if completely anonymised or consent has been given or authorised by the holder of parental responsibility, in strict compliance with the principles of data minimisation and purpose limitation; calls for more robust protection and safeguards in the education sector where children’s data is concerned and calls on the Commission to take more effective steps in that regard; calls for clear information to be provided to children and their parents about the possible use and processing of children’s data, including through awareness-raising and information campaigns;
45. Underlines the specific risks in the use of AI automated recognition applications, which are developing at pace; recalls that children are a particularly sensitive group; recommends that the Commission and the Member States ban automated biometric identification, such as facial recognition for educational and cultural purposes, on educational and cultural premises, unless its use is allowed by law;
46. Stresses the need to increase customer choice to stimulate competition and broaden the range of services offered by AI technologies for educational purposes; encourages public authorities, in this regard, to incentivise the development and deployment of AI technologies through public funding and public procurement; considers that technologies used by public education providers or purchased with public funding should be based on open-source technologies;
47. Notes that innovation in education is overdue, as highlighted by the COVID-19 pandemic and the ensuing switch to online and distance learning; stresses that AI-driven educational tools such as those for assessing and identifying learning difficulties can improve the quality and effectiveness of online learning;
48. Stresses that next-generation digital infrastructure and internet coverage are of strategic significance for providing AI-powered education to European citizens; in light of the COVID-19 crisis, calls on the Commission to elaborate a strategy for a European 5G that ensures Europe’s strategic resilience and is not dependent on technologies from states which do not share our values;
49. Calls for the creation of a pan-European university and research network focused on AI in education, which should bring together institutions and experts from all fields to examine the impact of AI on learning and identify solutions to enhance its potential;
Cultural heritage
50. Reiterates the importance of access to culture for every citizen throughout the Union; highlights, in this context, the importance of the exchange of best practices among Member States, educational facilities and cultural institutions and similar stakeholders; further considers it of vital importance that the resources available at both Union and national level are used to the maximum of their potential in order to further improve access to culture; stresses that there are a multitude of options to access culture and that all varieties should be explored in order to determine the most appropriate option; highlights the importance of consistency with the Marrakech Treaty;
51. Stresses that AI technologies can play a significant role in preserving, restoring, documenting, analysing, promoting and managing tangible and intangible cultural heritage, including by monitoring and analysing changes to cultural heritage sites caused by threats such as climate change, natural disasters and armed conflicts;
52. Stresses that AI technologies can increase the visibility of Europe’s cultural diversity; points out that these technologies provide new opportunities for cultural institutions, such as museums, to produce innovative tools for cataloguing artefacts as well as documenting and making cultural heritage sites more accessible, including through 3D modelling and augmented virtual reality; stresses that AI will also enable museums and art galleries to introduce interactive and personalised services for visitors by providing them with a list of suggested items based on their interests, expressed in person and online;
53. Stresses that the use of AI will herald new innovative approaches, tools and methodologies allowing cultural workers and researchers to create uniform databases with suitable classification schemes as well as multimedia metadata, enabling them to make connections between different cultural heritage objects and thus increase knowledge and provide a better understanding of cultural heritage;
54. Stresses that good practices in AI technologies for the protection and accessibility of cultural heritage, in particular for people with disabilities, should be identified and shared between cultural networks across the Union, while encouraging research on the various uses of AI to promote the value, accessibility and preservation of cultural heritage; calls on the Commission and the Member States to promote the opportunities offered by the use of AI in the CCSI;
55. Stresses that AI technologies can also be used to monitor the illicit trafficking of cultural objects and the destruction of cultural property, while supporting data collection for recovery and reconstruction efforts of both tangible and intangible cultural heritage; notes, in particular, that the development, deployment and use of AI in customs screening procedures may support efforts to prevent the illicit trafficking of cultural heritage, in particular to supplement systems which allow customs authorities to target their efforts and resources on items that pose the greatest risk;
56. Notes that AI could benefit the research sector, for example through the role that predictive analytics can play in fine-tuning data analysis, for example on the acquisition and movement of cultural objects; stresses that the Union must step up investment and foster partnerships between industry and academia in order to enhance research excellence at European level;
57. Recalls that AI can be a revolutionary tool for promoting cultural tourism and highlights its considerable potential in predicting tourism flows, which could help cities struggling with over-tourism;
Cultural and creative sectors and industries (CCSI)
58. Regrets the fact that culture is not among the priorities outlined in policy options and recommendations on AI at Union level, notably the Commission’s white paper of 19 February 2020 on AI; calls for these recommendations to be revised in order to make culture an AI policy priority at Union level; calls on the Commission and the Member States to address the potential impact of the development, deployment and use of AI technologies on the CCSI and to make the most of the Next Generation EU recovery plan to digitise these sectors to respond to new forms of consumption in the 21st century;
59. Points out that AI has now reached the CCSI, as exemplified by the automatic production of texts, videos and pieces of music; emphasises that creative artists and cultural workers must have the digital skills and training required to use AI and other digital technologies; calls on the Commission and the Member States to promote the opportunities offered by the use of AI in the CCSI, by making more funding available from science and research budgets, and to establish digital creativity centres in which creative artists and cultural workers develop AI applications, learn how to use these and other technologies and test them;
60. Acknowledges that AI technologies have the potential to boost a growing number of jobs in the CCSI facilitated by greater access to these technologies; emphasises, therefore, the importance of boosting digital literacy in the CCSI to make these technologies more inclusive, usable, learnable, and interactive for these sectors;
61. Emphasises that the interaction between AI and the CCSI is complex and requires an in‑depth assessment; welcomes the Commission’s report of November 2020 entitled ‘Trends and Developments in Artificial Intelligence – Challenges to the IPRS Framework’ and the study on copyright and new technologies: copyright data management and artificial intelligence; underlines the importance of clarifying the conditions of use of copyright‑protected content as data input (images, music, films, databases, etc.) and in the production of cultural and audiovisual outputs, whether created by humans with the assistance of AI or autonomously generated by AI technologies; invites the Commission to study the impact of AI on the European creative industries; reiterates the importance of European data and welcomes the statements made by the Commission in this regard, as well as the placing of artificial intelligence and related technologies high on the agenda;
62. Stresses the need to set up a coherent vision of AI technologies in the CCSI at Union level; calls on the Member States to strengthen the focus on culture in their AI national strategies to ensure that the CCSI embrace innovation and remain competitive and that cultural diversity is safeguarded and promoted at Union level in the new digital context;
63. Stresses the importance of creating a Union-wide heterogeneous milieu for AI technologies to encourage cultural diversity and support minorities and linguistic diversity, while also strengthening the CCSI through online platforms, allowing Union citizens to be included and to participate;
64. Calls on the Commission and the Member States to support a democratic debate on AI technologies and to provide a regular forum for discussion with civil society, researchers, academia and stakeholders to raise awareness on the benefits and the challenges of its use in the CCSI; emphasises, in that connection, the role which art and culture can play in familiarising people with AI and fostering public debate about it, as they can provide vivid, tangible examples of machine learning, for example in the area of music;
65. Calls on the Commission and the Member States to address the issue of AI-generated content and its challenges to authorship and copyright infringement; asks the Commission, in that regard, to assess the impact of AI and related technologies on the audiovisual sector and the CCSI , with a view to promoting cultural and linguistic diversity, while respecting authors’ and performers’ rights;
66. Stresses that the European Institute of Innovation and Technology (EIT), in particular its future Knowledge and Innovation Community (KIC) dedicated to cultural and creative industries (CCI), should play a leading role in developing a European strategy on AI in education, culture and the audiovisual sector and can help accelerate and harvest AI applications to these sectors;
67. Notes that AI has already entered the creative value chain at the level of creation, production, dissemination and consumption and is therefore having an immense impact on the CCSI, including music, the film industry, art and literature, through new tools, software and AI-assisted production for easier production, while providing inspiration and enabling the broader public to create content;
68. Calls on the Commission to carry out studies and consider policy options to tackle the detrimental impact of AI-based control of online streaming services designed to limit diversity and/or maximise profits by including or prioritising certain content in the consumer offer, as well as how this impacts cultural diversity and creators’ earnings;
69. Believes that AI is becoming increasingly useful for the CCSI in creation and production activities;
70. Emphasises the role of an author’s personality for the expression of free and creative choices that constitute the originality of works [12] ; underlines the importance of limitations and exceptions to copyright when using content as data input, notably in education, academia and research, and in the production of cultural and creative output, such as audiovisual output and user-generated content;
71. Takes the view that consideration should be given to protecting AI-generated technical and artistic creations in order to encourage this form of creativity;
72. Stresses that in the data economy context, better copyright data management is achievable, for the purpose of better remunerating authors and performers, notably in enabling the swift identification of the authorship and right ownership of content, thus contributing to lowering the number of orphan works; further highlights that AI technological solutions should be used to improve copyright data infrastructure and the interconnection of metadata in works, but also to facilitate the transparency obligation provided in Article 19 of Directive (EU) 2019/790 on copyright and related rights in the Digital Single Market [13] for up‑to‑date, relevant and comprehensive information on the exploitation of authors’ and performers’ works and performances, particularly in the presence of a plurality of rights holders and of complex licensing schemes;
73. Calls for the intellectual property action plan announced by the Commission to address the question of AI and its impact on the creative sectors, taking account of the need to strike a balance between protecting IPR and encouraging creativity in the areas of education, culture and research; considers that the Union can be a leader in the creation of AI technologies if it adopts an operational regulatory framework and implements proactive public policies, particularly as regards training programmes and financial support for research; asks the Commission to assess the impact of IPR on the research and development of AI and related technologies, as well as on the CCSI, including the audiovisual sector, with particular regard to authorship, fair remuneration of authors and related questions;
74. Calls on the Commission to consider the legal aspects of the output produced using AI technologies, as well as cultural content generated with the use of AI and related technologies; considers it important to support the production of cultural content; reiterates, however, the importance of safeguarding the Union’s unique IPR framework and that any changes should be made with the necessary due care, in order not to disrupt the delicate balance; calls on the Commission to produce an in-depth assessment with regard to the possible legal personality of AI-produced content, as well as the application of IPR to AI-generated content and to content created with the use of AI tools;
75. Calls on the Commission, in addition, to consider developing, in very close cooperation with Member States and the relevant stakeholders, verification mechanisms or systems for publishers, authors and creators in order to assist them in verifying what content they may use and to more easily determine what is protected under IPR legislation;
76. Calls on the Commission to lay down rules designed to guarantee effective data interoperability in order to make content purchased on a platform accessible via any digital tool irrespective of brand;
Audiovisual sector
77. Notes that AI is often used to enable automated decision-making algorithms to disseminate and order the cultural and creative content displayed to users; stresses that these algorithms are a ‘black box’ for users; stresses that the algorithms used by media service providers, video sharing platforms (VSPs) and music streaming services should be designed in such a way that they do not privilege specific works by limiting their ‘personalised’ suggestions to the most popular works, for targeted advertising, commercial purposes or to maximise profit; calls for recommendation algorithms and personalised marketing to be explainable and transparent where possible, in order to give consumers an accurate and comprehensive insight into these processes and content and to ensure that personalised services are not discriminatory and in line with the recently adopted Platform to Business Regulation [14] and New Deal for Consumers Omnibus Directive [15] ; calls on the Commission to address the ways in which content moderation algorithms are optimised to engage users, and to propose recommendations to increase user control over the content they see, by guaranteeing and properly implementing the right of users to opt out of recommended and personalised services; underlines, moreover, that consumers must be informed when they are interacting with an automated decision process and that their choices and performance must not be limited; stresses that the use of AI mechanisms for the commercial surveillance of consumers must be countered, even if it concerns ‘free services’, by ensuring that it is strictly in line with fundamental rights and the GDPR; stresses that all regulatory changes must take into consideration the impact on vulnerable consumers;
78. Underlines that what is illegal offline shall be illegal online; notes that AI tools have the potential and are already used to fight illegal content online, but strongly recalls ahead of the forthcoming Digital Services Act that such tools must always respect fundamental rights, especially freedom of expression and information, and should not lead to a general monitoring obligation for the internet, or to the removal of legal material disseminated for education, journalistic, artistic or research purposes; stresses that algorithms should be used only as a flagging mechanism in content moderation, subject to human intervention, as AI is unable to reliably distinguish between legal, illegal and harmful content; notes that terms and conditions should always include community guidelines as well as an appeal procedure;
79. Recalls, furthermore, that there should be no general monitoring, as stipulated in Article 15 of the e-Commerce Directive [16] , and that specific content monitoring for audiovisual media services should be in accordance with the exceptions laid down in Union legislation; recalls that AI applications must adhere to internal and external safety protocols, which should be technically accurate and robust in nature; considers that this should extend to operation in normal, unknown and unpredictable situations alike;
80. Stresses, moreover, that the use of AI in algorithm-based content recommendations on media service providers, such as video on demand services and VSPs, may have a serious impact on cultural and linguistic diversity, notably regarding the obligation to ensure the prominence of European works under Article 13 of the Audiovisual Media Services Directive (Directive (EU) 2018/1808 [17] ); notes that the same concerns are equally relevant for the music streaming services and calls for the development of indicators to assess cultural diversity and the promotion of European works on such services;
81. Calls on the Commission and the Member States to step up their financial support for the development, deployment and use of AI in the area of the automatic subtitling and dubbing of European audiovisual works, in order to foster cultural and language diversity in the Union and enhance the dissemination of and access to European audiovisual content;
82. Calls on the Commission to establish a clear ethical framework for the use of AI technologies in media in order to prevent all forms of discrimination and ensure access to culturally and linguistically diverse content at Union level, based on accountable, transparent and inclusive algorithms, while respecting individuals’ choices and preferences;
83. Points out that AI can play a major role in the rapid spread of disinformation; stresses, in that regard, that the framework should address the misuse of AI to disseminate fake news and online misinformation and disinformation, while avoiding censorship; calls on the Commission, therefore, to assess the risks of AI assisting the spread of disinformation in the digital environment as well as solutions on how AI could be used to help counter disinformation;
84. Calls on the Commission to take regulatory measures to ensure that media service providers have access to the data generated by the provision and dissemination of their content on other providers’ platforms; emphasises that full data transfer from platform operators to media service providers is vital if the latter are to understand their audience better and thus improve the services they offer in keeping with people’s wishes;
85. Stresses the importance of increasing funding for Digital Europe, Creative Europe and Horizon Europe in order to reinforce support for the European audiovisual sector, namely by collaborative research projects and experimental pilot initiatives on the development, deployment and use of ethical AI technologies;
86. Calls for close collaboration between Member States in developing training programmes aimed at reskilling or upskilling workers to make them better prepared for the social transition that the use of AI technologies in the audiovisual sector will entail;
87. Considers that AI has enormous potential to help drive innovation in the news media sector; believes that the widespread integration of AI, such as for content generation and distribution, the monitoring of comments sections, the use of data analytics, and identifying doctored photos and videos, is key for saving on costs in newsrooms in the light of diminishing advertising revenues and for devoting more resources to reporting on the ground and thus increase the quality and variety of content;
Online disinformation: deepfakes
88. Stresses the importance of ensuring online and offline media pluralism to guarantee the quality, diversity and reliability of the information available;
89. Recalls that accuracy, independence, fairness, confidentiality, humanity, accountability and transparency, as driving forces behind the principles of freedom of expression and access to information in online and offline media, are decisive in the fight against disinformation and misinformation;
90. Notes the important role which independent media play in culture and the daily life of citizens; stresses that disinformation represents a fundamental problem, as copyright and IPR generally are being constantly infringed; calls on the Commission, in cooperation with the Member States, to continue its work on raising awareness of this problem, countering the effects of disinformation as well as the source problems; considers it important, furthermore, to develop educational strategies to specifically improve digital literacy in this regard;
91. Recalls that with new techniques rapidly emerging, detecting false and manipulated content such as deepfakes may become increasingly challenging due to the ability of malicious producers to generate sophisticated algorithms that can be successfully trained to evade detection, thus seriously undermining our basic democratic values; asks the Commission to assess the impact of AI in the creation of deepfakes, to establish appropriate legal frameworks to govern their creation, production or distribution for malicious purposes, and to propose recommendations for, among other initiatives, action against any AI-powered threats to free and fair elections and democracy;
92. Welcomes recent initiatives and projects to create more efficient deepfake detection tools and transparency requirements; stresses, in this regard, the need to explore and invest in methods for tackling deepfakes as a crucial step in combatting misinformation and harmful content; considers that AI-enabled solutions can be helpful in this regard; asks the Commission, therefore, to impose an obligation for all deepfake material or any other realistically made synthetic videos to state that the material is not original and a strict limitation when used for electoral purposes;
93. Is concerned that AI is having an ever greater influence on the way information is found and consumed online; points out that so-called filter bubbles and echo chambers are restricting diversity of opinion and undermining open debate in society; urges, therefore, that the way platform operators use algorithms to process information must be transparent and that users must be given greater freedom to decide whether and what information they want to receive;
94. Points out that AI technologies are already being used in journalism, for example to produce texts or, in the context of investigative research, to analyse large data sets; emphasises that in the context of producing information of significance to society as a whole, it is important that automated journalism should draw on correct and comprehensive data, in order to prevent the dissemination of fake news; emphasises that the basic principles of quality journalism, such as editorial supervision, must also apply to journalistic content produced using AI technologies; calls for AI-generated texts to be clearly identified as such, in order to safeguard trust in journalism;
95. Highlights the potential of AI to facilitate and encourage multilingualism by developing language-related technologies and enabling online European content to be discovered;
96. Instructs its President to forward this resolution to the Council and the Commission.
“I believe that at the end of the century the use of words and general educated opinion will have altered so much that one will be able to speak of machines thinking without expecting to be contradicted ”
Alan Turing, 1947
The last decade has been transformative for AI, arousing both fear and excitement for humanity. Seen as ‘the new electricity’, AI has advanced to the point that it will have such a systemic impact that it could substantially change all aspects of society for the next century.
Whilst it is easy to understand the potential effects of AI on sectors such telecommunications, transportation, traffic management, health care, evaluating its long-term effects on education, culture and the audiovisual sector is considerably more challenging. Although there is a consensus that AI and automation is likely to create more wealth and to simplify a vast array of processes, the use of AI has also raised serious concerns that it may result in an increase in inequality, discrimination and unemployment.
The potential impact of AI on education, culture and the audiovisual sector is however rarely discussed and is mostly unknown. Yet this question is of utmost importance because AI is already being used to teach curricula, as well as to produce movies, songs, stories and paintings.
The purpose of this report is therefore to understand concretely how AI currently impacts these sectors and how future technological advances in AI will impact them further over the next decade. In particular, the Rapporteur reflects on how AI may transform these sectors and which particular regulatory challenges the Union may have to face in that regard.
(i) AI is reshaping education
AI is transforming learning, teaching, and education radically. The whirlwind speed of technological development accelerates the radical transformation of educational practices, institutions and policies. In this field, AI has many applications, such as customisable approaches to learning, AI-based tutors, textbooks and course material with customised content, smart algorithms to determine best teaching methods, AI game engines, and adaptive user models in personalised learning environments (PLE) which can allow early identification of difficulties, such as dyslexia or risks of early school leaving.
Personalised learning experience is the cornerstone of the use of AI in education. It would allow students to enjoy an educational approach that is fully tailored to their individual abilities, needs and difficulties, whilst enabling teachers to closely monitor students’ progress. However in order to make personalised education a reality, large amounts of personal data need to be collected, used and analysed.
The Rapporteur stresses in that regard that the current lack of access to personal data on students is likely to prevent the successful implementation of AI in education. It is thus essential to ensure the safety and transparency of personal data collection, use, management and dissemination, whilst safeguarding confidentiality and privacy of learners’ personal data. Moreover, addressing the risks of AI potential bias, as well as tackling the issue of data storage should be a priority in any initiatives for the wide deployment of AI in the education system at Union level.
Although there is little chance that teachers will be replaced by machines in the near future, the increasing use of AI in education implies the need to rethink education overall, as well as to reflect on the redefinition of teaching, the role of the teachers, and, as a result, the subsequent retraining required to adapt to a AI-based educational system.
Considering that less than 40% of teachers in the Union have received courses on ICT inclusion in the classroom throughout their Initial Teacher Education (ITE), the Rapporteur would like to stress the crucial importance of training teachers so they acquire digital skills as a prerequisite to becoming familiar with AI. They could then take advantage of AI technologies, but also make them aware of the potential dangers of AI.
This issue can also be seen more widely, with 42% of the Union population still lacking basic digital skills. There are also serious regional discrepancies in access to digital infrastructure and in digital skills attainment across the Union.
Emerging technology trends related to digital transformation, such as AI, have profound implications in terms of the skills required for the evolving digital economy. In particular, the notion of lifelong learning in AI has emerged as one of the key strategies for job security and employment in the digital era.
The Rapporteur suggests that citizens are trained to acquire the necessary digital skills, whilst carefully assessing what AI-related skills are needed today and in the future, and that the necessary measures are taken to address existing and emerging skill gaps.
It also crucial to ensure that the prerequisites for the deployment and the relevant use of AI, in terms of internet access, connectivity, networks and infrastructures, are met.
(ii) AI can be used to safeguard and promote cultural heritage
In recent years, AI has been of increasing relevance to cultural heritage, notably in response to potential modern threats, such as climate change or conflicts. AI can have various applications in that regard: it can be used to enhance users’ experience by enabling visitors of cultural institutions and museums to create personal narrative trails or to enjoy virtual tour guides. Conversational bots could communicate in an interactive way about cultural heritage on any topics and in any language. They would also make the access to information easier whilst providing a vivid cultural experience to users.
AI could also facilitate the understanding of the history of the Union, such as how the ‘Time Machine Project’ aims to create advanced AI technologies to make sense of vast amounts of information from complex historical data sets stored in archives and museums. This enables the transformation of fragmented data into useable knowledge by mapping Union’s entire social, cultural and geographical evolution. This may facilitate the exploration of the cultural, economic, and historical development of European cities, and improve understanding thereof.
(iii) AI changes the way the cultural and creative industries work, in particular the audiovisual sector
AI use is rapidly expanding in media with many applications:
- Data-driven marketing and advertising, by training machine learning algorithms to develop promotional movie trailers and design advertisements,
- Personalisation of users’ experience, by using machine learning to recommend personalised content based on data from user activity and behaviour,
- Search optimisation, by using AI to improve the speed and efficiency of the media production process and the ability to organise visual assets,
- Content creation, by generating video clips from automatic video segments ready for broadcast and special effects, such as re-creating a younger version of an actor digitally or creating new content with a deceased actor,
- Script writing such as simple factual text creation (sports and news reports produced by robots), but also for writing fictional stories, such as the experimental short movie ‘Sunspring’,
- Viewer interaction on complex story lines, such as the last episode of the British series ‘Black Mirror’, ‘Bandersnatch’,
- Automated captioning and subtitling, such audio-to-text processes, for viewers with disabilities
- Automated content moderation on audiovisual content.
Whilst AI offers a wide range of opportunities in producing high quality cultural and creative content, the centralised distribution and access to such content raises a number of ethical and legal issues, notably on data protection, freedom of expression and cultural diversity.
Cultural and creative works, notably audiovisual works, are mainly distributed through
large centralised platforms, which conditions media consumption to the proprietary algorithms developed by these platforms.
The Rapporteur points out that algorithm-based personalised recommendations are potentially detrimental to cultural and linguistic diversity, preventing under-represented cultural and creative content from appearing in suggestions provided by these systems. On the largest platforms, the criteria used to select or recommend a work are neither transparent nor auditable, and are likely to be decided on the basis of economic factors that solely benefit these platforms.
The question of cultural and linguistic diversity in recommendation systems is therefore crucial and must be addressed. The Rapporteur stresses the need to set up a clear legal framework for transparent, accountable and inclusive algorithms, in order to safeguard and promote cultural and linguistic diversity .
Regulatory challenges triggered by AI applications within the audiovisual sector are also linked to existing legal acts, such as the AVMSD. Thus a more in-depth assessment might be needed as to the urgency and/or political momentum for future adaptions of these files to AI.
Whilst AI can help empower many creators, making CCS more prosperous and driving cultural diversity, the large majority of artists and entrepreneurs may not still be familiar with AI tools.
There is a lack of technical knowledge among creators precluding them from experimenting with machine learning and reaping the benefits they can bring. Therefore it is essential to assess which skills would be needed in the near future, whilst at the same time improving training systems, including upskilling and reskilling, guaranteeing lifelong learning throughout the whole working life and beyond.
In that context, the Rapporteur suggests setting up an AI observatory with an objective of harmonising and facilitating evidence-based scrutiny of new developments in AI in order to tackle the question of auditability and accountability of AI applications in CCS .
(v) Countering fake news
AI-technologies are increasingly used to disseminate fake news, notably through the use of ‘deepfakes’.
Deepfakes are synthetic images or videos generated by AI using ‘deep learning machines’ and generative adversial networks (GAN). Humans cannot distinguish deepfakes from authentic content. Deepfakes can be used for all kinds of trickery, most commonly used for ‘face swaps’, from harmless satires and film tweaks to malicious hoaxes, targeted harassment, deepfake porn or financial fraud, The danger of deepfakes is to make people believe something is real when it is not, and thus may be used as a particularly powerful and potent weapon for online disinformation, spreading virally on platforms and social media, where they can influence public opinion, voting processes and election results.
Whilst AI is frequently singled out for its role in spreading fake news, it could also play a significant role in countering and combating fake news and disinformation, as evidenced by projects such as “Fake News Challenge”. AI systems can reverse-engineer AI generated fake news, and help spot manipulated content. However algorithms generating deepfakes are getting more and more sophisticated, and detecting them as a result is getting increasingly difficult.
The Rapporteur therefore stresses the need to tackle the misuse of AI in disseminating fake news and online misinformation, notably by exploring ways to efficiently detect deepfakes.
OPINION OF THE COMMITTEE ON CIVIL LIBERTIES, JUSTICE AND HOME AFFAIRS ( 16.7.2020 )
for the Committee on Culture and Education
Rapporteur for opinion (*): Ondřej Kovařík
(*) Associated committee – Rule 57 of the Rules of Procedure
SUGGESTIONS
The Committee on Civil Liberties, Justice and Home Affairs calls on the Committee on Culture and Education, as the committee responsible, to incorporate the following suggestions into its motion for a resolution:
1. Underlines that the use of AI in the education, culture and audiovisual sectors must fully respect fundamental rights, freedoms and values, including privacy, the protection of personal data, non-discrimination and freedom of expression and information, as enshrined in the EU Treaties and the Charter of Fundamental Rights of the European Union; welcomes the Commission’s White Paper on Artificial Intelligence in this regard, and invites the Commission to include the educational sector, limited to areas posing significant risks, in the future regulatory framework for high-risk AI applications;
2. Recalls that AI may give rise to biases and thus to various forms of discrimination based on sex, race, colour, ethnic or social origin, genetic features, language, religion or belief, political or any other opinion, membership of a national minority, property, birth, disability, age or sexual orientation; in this regard, recalls that everyone’s rights must be ensured and that AI initiatives must not be discriminatory in any form;
3. Emphasises that such bias and discrimination can arise from already biased sets of data, reflecting existing discrimination in society; stresses that AI must avoid bias leading to prohibited discrimination and must not reproduce discrimination processes; underlines the need to take these risks into account when designing AI technologies, as well as, the importance of working with AI technology providers to address persistent loopholes facilitating discrimination, and recommends that AI designing and developing teams should reflect the diversity of society;
4. Notes that the use of AI in education brings a wide range of possibilities and opportunities, for instance to facilitate access to information, to improve research methods or to understand how pupils learn and to offer them customisation, while at the same time posing risks regarding equal access to education and learning equalities at an increasingly younger age and for vulnerable and historically disadvantaged groups; calls for a sufficient data-sharing infrastructure between AI applications and public research entities; points out that equity and inclusion are core values that should be duly taken into account when designing policies for AI in education; calls for the non-discriminatory use of AI in the education sector; recalls the risks and discrimination that may arise from recently developed AI tools used for purposes of school admissions, and calls for them to be rectified as soon as possible; underlines the need for a proper assessment of the AI tools used in the education sector to identify their impact on the rights of the child;
5. Acknowledges that using digital and AI technologies can help develop increasingly effective educational tools and lead to a more inclusive society, by countering traditional forms of discrimination including lack of access to services, by bringing education to disadvantaged communities, persons with disabilities in line with the EU Accessibility Act, and other categories of European citizens lacking proper access to education, and by providing access to adequate learning opportunities;
6. Underlines that the benefits of AI should be shared with all parts of society, leaving no one behind; stresses the need to fully take into consideration the specific needs of the most vulnerable groups, such as children, persons with disabilities, elderly people and other groups at risk of exclusion; expresses its concerns about limited accessibility of the internet in some regions across the EU, and calls on the Commission and the Member States to deploy sustained efforts to ameliorate telecommunications infrastructures;
7. Recognises the possibilities of AI in the culture sector in terms of developing music, art and other cultural expressions; emphasises that freedom of expression is an important freedom and value and that a pluriform cultural landscape is of great value to society; calls on the Commission to keep these values in mind when drafting its proposals on AI;
8. Welcomes the Commission’s plan to update the Digital Education Action Plan so as to make it more ambitious and integrated with a view to making educational systems fit for the digital age, notably through making better use of data and AI-based technologies; calls on all stakeholders, both public and private, to closely cooperate in implementing these educational reforms;
9. Stresses the need to ensure more general public awareness of AI at all levels, as a key element to enable the public to make informed decisions and help strengthen the resilience of our societies; underlines that this must also include public awareness of the risks in terms of privacy and biases related to AI; invites the Commission and the Member States to include the above in educational programmes and programmes which support the arts;
10. Underlines the urgent need to educate the public at every level in the use of AI and to equip all European citizens, including vulnerable groups, with basic digital skills enabling equal social and economic opportunities, as well as the need to have high-quality ICT programmes in education systems, at all levels; calls for the digital gender gap not to be underestimated and for measures to be taken to remedy it; welcomes the upcoming update of the Skills Agenda aimed at allowing everyone to benefit from EU digital transformation; emphasises the importance of training teachers and educators in the use of AI, especially those responsible for underage students; notes that significant skills shortages still exist in the digital and technology sectors; underlines the importance of diversifying this sector and to encourage students, in particular women and girls, to enrol in Science, Technology, Engineering and Mathematics (STEM) courses, in particular in robotics and AI-related subjects, in addition to those related to their career aspirations; calls for more financial and scientific resources to motivate skilled people to stay in the EU and to attract those with skills from abroad; furthermore, notes that there are a considerable number of start-ups working with AI and developing AI technologies; stresses that small and medium-sized enterprises (SMEs) will require additional support and AI-related training to comply with digital and AI-related regulation;
11. Recalls that data protection and privacy can be particularly affected by AI; underlines the principles established in Regulation (EU) 2016/679 of the European Parliament and of the Council (the General Data Protection Regulation (GDPR)) [18] as binding principles for AI deployment; recalls that all AI applications need to fully respect Union data protection law, namely the GDPR and Directive (EC) 2002/58 of the European Parliament and of the Council (the ePrivacy Directive) [19] (currently under revision);
12. Recalls that children constitute a vulnerable public who deserve particular attention and protection; recalls that automated decisions about natural persons based on profiling, where they have legal or similar effects, must be strictly limited and always require the right to human intervention and to explicability under the GDPR; underlines that this should be strictly adhered to, especially in the education system, where decisions about future chances and opportunities are taken; observes that a few private companies are dominating the educational technology (edtech) sector in some Member States, and believes this should be scrutinised through EU competition rules; strongly recalls that data of minors is strictly protected by the GDPR, and that children’s data can only be processed if completely anonymised or where consent has been given or authorised by the holder of parental responsibility over the child; therefore calls for stronger protection and safeguards in the education sector where children’s data are concerned; calls for clear information to be provided to children and their parents, including via awareness and information campaigns, about the possible use and processing of children’s data;
13. Underlines the specific risks existing in the use of AI automated recognition applications, which are currently developing rapidly; recalls that children are a particularly sensitive public; recommends that the Commission and the Member States ban automated biometric identification, such as facial recognition for educational and cultural purposes, on educational and cultural premises, unless its use is allowed in law;
14. Calls on the Commission and the Member States to implement an obligation of transparency and explainability of AI-based automated individual decisions taken within the framework of prerogatives of public power, and to implement penalties to enforce such obligations; calls for the implementation of systems which use human verification and intervention by default, and for due process, including the right of appeal, and access to remedies; recalls that automated decisions about natural persons based on profiling, where they have legal or similar effects, must be strictly limited and always require the right to human intervention and to explainability under the GDPR;
15. Calls for independent audits to be conducted regularly to examine whether AI applications being used and the related checks and balances are in accordance with specified criteria, and for those audits to be supervised by independent and sufficient overseeing authorities; calls for specific stress tests to assist and enforce compliance;
16. Points out that AI can play a major role in the rapid spread of disinformation; therefore calls on the Commission to assess the risks of AI assisting the spread of disinformation in the digital environment, and to propose recommendations, among others, for action against any AI-powered threats to free and fair elections and democracy; observes that deep fakes can also be used to manipulate elections, to disseminate disinformation and for other undesirable actions; notes furthermore that the immersive experiences facilitated by AI can be exploited by malicious actors; asks the Commission to propose recommendations, including possible restrictions in this regard, in order to adequately safeguard against the use of these technologies for illegal purposes; also calls for an assessment of how AI could be used to help counter disinformation; calls on the Commission to ensure that any future regulatory framework does not lead to censorship of legal individual content uploaded by users; recalls that critical thinking and the ability to interact with skill and confidence in the online environment are needed more than ever;
17. Notes that AI is often used to enable automated decision-making algorithms to disseminate and order the content displayed to users; stresses that these algorithms are a ‘black box’ for users; calls on the Commission to address the ways in which content moderation algorithms are optimised towards engagement of their users; also calls on the Commission to propose recommendations to increase user control over the content they see, and to ask AI applications and internet platforms to give users the possibility to choose to have content displayed in a neutral order, in order to give them more control on the way content is ranked to them, including options for ranking outside their ordinary content consumption habits and for opting out completely from any content curation;
18. Notes the potential negative impact of personalised advertising, in particular micro-targeted and behavioural advertising, and of assessment of individuals, especially minors, without their consent, by interfering in the private life of individuals, asking questions as to the collection and use of the data used to personalise advertising, and offering products or services or setting prices; calls, therefore, on the Commission to introduce strict limitations on targeted advertising based on the collection of personal data, starting by introducing a prohibition on cross-platform behavioural advertising, while not hurting SMEs; recalls that currently the ePrivacy Directive only allows targeted advertising subject to opt-in consent, otherwise making it illegal; calls on the Commission to prohibit the use of discriminatory practices for the provision of services or products;
19. Underlines that what is illegal offline shall be illegal online; notes that AI tools have the potential and are already used to fight illegal content online, but strongly recalls ahead of the Digital Services Act expected for the end of this year that such tools must always respect fundamental rights, especially freedom of expression and information, and should not lead to a general monitoring obligation for the internet, or to the removal of legal material disseminated for education, journalistic, artistic or research purposes; stresses that algorithms should be used only as a flagging mechanism in content moderation, subject to human intervention, as AI is unable to reliably distinguish between legal, illegal and harmful content; notes that terms and conditions should always include community guidelines as well as an appeal procedure;
20. Notes the benefits and risks of AI in terms of cybersecurity and its potential in combating cybercrime, and emphasises the need for any AI solutions to be resilient to cyberattacks while respecting EU fundamental rights, especially the protection of personal data and privacy; stresses the importance of monitoring the safe use of AI and the need for close collaboration between the public and private sectors to counter user vulnerabilities and the dangers arising in this connection; calls on the Commission to evaluate the need for better prevention in terms of cybersecurity and mitigation measures thereof;
21. Stresses that next-generation digital infrastructure and internet coverage are of strategic significance for providing AI-powered education to European citizens; in light of the COVID-19 crisis, calls on the Commission to elaborate a strategy for a European 5G that ensures Europe’s strategic resilience and is not dependent on technology from states who do not share our values;
22. Calls on the Commission and the Member States to support the use of AI in the area of digitalised cultural heritage.
INFORMATION ON ADOPTION IN COMMITTEE ASKED FOR OPINION
FINAL VOTE BY ROLL CALL IN COMMITTEE ASKED FOR OPINION
Key to symbols:
+ : in favour
- : against
0 : abstention
OPINION OF THE COMMITTEE ON THE INTERNAL MARKET AND CONSUMER PROTECTION ( 6.7.2020 )
Rapporteur for opinion: Kim Van Sparrentak
The Committee on the Internal Market and Consumer Protection calls on the Committee on Culture and Education, as the committee responsible, to incorporate the following suggestions into its motion for a resolution:
A. whereas artificial intelligence (AI) has the potential to offer solutions for day-to-day challenges of the education sector such as the personalisation of learning, monitoring learning difficulties, automation of subject-specific content/knowledge, providing better professional training, and supporting the transition to a digital society;
B. whereas AI could have practical applications in terms of reducing the administrative work of educators and educational institutions, freeing up time for their core teaching and learning activities;
C. whereas the application of AI in education raises concerns around the ethical use of data, learners’ rights, data access and protection of personal data, and therefore entails risks to fundamental rights such as the creation of stereotyped models of learners’ profiles and behaviour that could lead to discrimination or risks of doing harm by the scaling-up of bad pedagogical practices;
D. whereas AI applications are omnipresent in the audiovisual sector, in particular on audiovisual content platforms;
1. Notes that the Commission has proposed to support public procurement in intelligent digital services, in order to encourage public authorities to rapidly deploy products and services that rely on AI in areas of public interest and the public sector; highlights the importance of public investment in these services and the complementary added value provided by public-private partnerships in order to secure this objective and deploy the full potential of AI in the education, culture and audiovisual sectors; emphasises that in the education sector, the development and deployment of AI should involve all those participating in the educational process and wider society and take into account their needs and the expected benefits, especially for the most vulnerable and disadvantaged, in order to ensure that AI is used purposefully and ethically and delivers real improvements for those concerned; considers that products and services developed with public funding should be published under open-source licences with full respect for the applicable legislation, including Directive (EU) 2019/790 of the European Parliament and of the Council of 17 April 2019 on copyright and related rights in the Digital Single Market; stresses the importance of this deployment for reskilling and upskilling the European labour market, and particularly in the culture and audiovisual sectors, which will be severely impacted by the COVID-19 crisis;
2. Recognises that children are an especially vulnerable group in terms of influencing their behaviour; stresses that while AI can be a tool that can benefit their education, it is necessary to take into account the technological, regulatory and social aspects of the introduction of AI in education, with adequate safeguards and a human-centric approach that ensures that human beings are, ultimately, always able to control and correct the system’s decisions; points to the need for a review and updating of the relevant sectoral rules; underlines in this regard that the legal framework governing AI in the education sector should, in particular, provide for legally binding measures and standards to prevent practices that would undermine fundamental rights and freedoms, and ensure the development of trustworthy, ethical and technically robust AI applications, including integrated digital tools, services and products such as robotics and machine learning;
3. Notes the potential of AI-based products in education, especially in making high-quality education available to all pupils in the EU; stresses the need for governments and educational institutions to rethink and rework educational programmes with a stronger emphasis on STEAM subjects, in order to prepare learners and consumers for the increasing presence of AI and to facilitate the acquisition of cognitive skills; underlines the need to improve the digital skills of those participating in the educational process and wider society, while having regard to the objectives of ‘A Europe fit for the digital age’;
4. Underlines that algorithmic systems can be an enabler for reducing the digital divide in an accelerated way, but unequal deployment risks creating new divides or accelerating the deepening of the existing ones; expresses its concern that knowledge and infrastructure are not developed in a consistent way across the EU, which limits the accessibility of products and services that rely on AI, in particular in sparsely populated and socio-economically vulnerable areas; calls on the Commission to ensure cohesion in the sharing of the benefits of AI and related technologies;
5. Calls on the Commission to consider education as a sector where significant risks can be expected to occur from certain uses of AI applications, which may potentially undermine fundamental rights and result in high costs in both human and social terms, and to take this consideration into account when assessing what types or uses of AI applications would be covered by a regulatory framework for high-risk AI applications, given the importance of ensuring that education continues to contribute to the public good and given the high sensitivity of data on pupils, students and other learners; calls on the Commission to include certain AI applications in the education sector, such as those that are subject to certification schemes or include sensitive personal data, in the regulatory framework for high-risk AI applications; underlines that data sets used to train AI and the outputs should be reviewed in order to avoid all forms of stereotypes, discrimination and biases, and where appropriate, make use of AI to identify and correct human biases where they might exist; points out, accordingly, that appropriate conformity assessments are needed in order to verify and ensure that all the provisions concerning high-risk applications are complied with, including testing, inspection and certification requirements; stresses the importance of securing the integrity and the quality of the data;
6. Welcomes the efforts of the Commission to include digital skills as part of the qualification requirements for certain professions harmonised at EU level under the Professional Qualifications Directive; highlights the need to ensure mutual recognition of professional qualifications in AI skills across the EU, as several Member States are upgrading their educational offer with AI-related skills and putting in place specific curricula for AI developers; stresses the need for these to be in line with the assessment list of the Ethical Guidelines for Trustworthy AI, and welcomes the Commission’s proposal to transform this list into an indicative curriculum for AI developers; underlines the importance of training highly skilled professionals in this area, including ethical aspects in their curricula, and supporting underrepresented groups in the field, as well as creating incentives for those professionals to seek work within the EU;
7. Takes note that schools and other public education providers are increasingly using educational technology services, including AI applications; expresses its concern that these technologies are currently provided by just a few technology companies; stresses that this may lead to unequal access to data and limit competition by market dominance and restricting consumer choice; encourages public authorities to take an innovative approach towards public procurement, so as to broaden the range of offers that are made to public education providers across Europe; stresses in this regard the importance of supporting the uptake of AI by SMEs in the education, culture and audiovisual sector through the appropriate incentives that create a level playing field; calls, in this context, for investment in European IT companies in order to develop the necessary technologies within the EU; considers that technologies used by public education providers or purchased with public money should be based on open-source technology where possible, while having full respect for the applicable legislation, including Directive (EU) 2019/790 of the European Parliament and of the Council of 17 April 2019 on copyright and related rights in the Digital Single Market;
8. Calls for the data used by AI applications in the education sector to be accessible, interoperable and of high quality, and to be shared with the relevant public authorities in a standardised way and with respect for copyright and trade secrets legislation, so that the data can be used, in accordance with the European data protection and privacy rules and ethical, democratic and transparency standards, in the development of curricula and pedagogical practices (in particular when these services are purchased with public money or offered to public education providers for free, considering that education is a common good); calls on the Commission to ensure fair access to data for all companies, and in particular SMEs and cultural and creative companies, which play an essential role in sustaining social cohesion and cultural diversity in Europe, as well as democratic values;
9. Stresses the importance of developing guidelines for the public procurement of such services and applications for the public sector, including for education providers, in order to ensure the relevant educational objectives, consumer choice, a level and fair playing field for AI solution providers and respect for fundamental rights; stresses the need for public buyers to take into account specific criteria linked to the relevant educational objectives, such as non-discrimination, fundamental rights, diversity, the highest standards of privacy and data protection, accessibility for learners with special needs, environmental sustainability and, specifically when purchasing services for public education providers, the involvement of all those participating in the educational process; stresses the need to strengthen the market by providing SMEs with the opportunity to participate in the procurement of AI applications in order to ensure the involvement of technology companies of all sizes in the sector and thus guarantee resilience and competition;
10. Underlines the unreliability of the current automated means of removing illegal content from online platforms on which audiovisual content is shared, which may lead to inadvertent removal of legitimate content; notes that neither the E-Commerce Directive nor the revised Audiovisual Media Services Directive on video sharing platforms imposes a general monitoring obligation; recalls, to that end, that there should be no general monitoring, as stipulated in Article 15 of the E-Commerce Directive, and that specific content monitoring for audiovisual services should be in accordance with the exceptions laid down in the European legislation; recalls the key requirements for AI applications, such as accountability, including review structures within business processes, and reporting of negative impacts; emphasises that transparency should also include traceability and explainability of the relevant systems; recalls that AI applications must adhere to internal and external safety protocols, which should be technically accurate and robust in nature; considers that this should extend to operation in normal, unknown and unpredictable situations alike;
11. Calls for recommendation algorithms and personalised marketing on audiovisual platforms, including video streaming platforms, news platforms and platforms disseminating cultural and creative content, to be explainable, to the extent technically possible, in order to give consumers an accurate and comprehensive insight into these processes and content and ensure that personalised services are not discriminatory and are in line with the recently adopted Platform to Business Regulation and New Deal for Consumers Omnibus Directive; stresses the need to guarantee and properly implement the right of users to opt out from recommended and personalised services; points out in this regard that a description should be provided to users that allows for a general and adequate understanding of the functions concerned, notably on the data used, the purpose of the algorithm, and personalisation and its outcomes, following the principles of explainability and fairness; calls for the development of mechanisms providing monitoring of the consumer’s rights of informed consent and freedom of choice when submitting data;
12. Notes that the deployment of AI in customs screening procedures may support efforts to prevent the illicit trafficking of cultural heritage, in particular to supplement systems which allow customs authorities to target their efforts and resources on those items presenting the highest risk;
13. Underlines that consumers must be informed when they are interacting with an automated decision process and that their choices and performance must not be limited; stresses that the use of AI mechanisms for commercial surveillance of consumers must be countered, even if it concerns ‘free services’, by ensuring that it is strictly in line with fundamental rights and the GDPR; stresses that all regulatory changes must take in consideration the impact on vulnerable consumers;
14. Points out that the deployment, development and implementation of AI must make it easier for consumers and learners with some form of disability to use tools to access audiovisual content;
15. Underlines the need for upskilling of the future workforce; recognises the benefits of forecasting which jobs will be disrupted by digital technology such as automation, digitalisation and AI;
16. Points out that the AI systems that are developed, implemented and used in the European Union, in any of the three sectors referred to in this report, must reflect the EU’s cultural diversity and multilingualism.
OPINION OF THE COMMITTEE ON LEGAL AFFAIRS ( 22.9.2020 )
Rapporteur for opinion: Angel Dzhambazki
The Committee on Legal Affairs calls on the Committee on Culture and Education, as the committee responsible, to incorporate the following suggestions into its motion for a resolution:
1. Underlines the strategic importance of using artificial intelligence (AI) and related technologies, and stresses that the European approach in this regard must be human-centred so that AI genuinely becomes an instrument in the service of people and the common good, contributing to the general interest of citizens, including in the audiovisual, cultural and educational sectors; points out that AI can support content creation in those sectors; stresses that AI can support content creation in the education, culture and audiovisual sectors, alongside information and educational platforms, including listings of different kinds of cultural objects and a multitude of data sources; notes the risks of infringement of intellectual property rights (IPRs) when blending AI and different technologies with a multiplicity of sources (documents, photos, films) to improve the way those data are displayed, researched and visualised; calls for the use of AI to ensure a high level of IPR protection within the current legislative framework, for example by alerting individuals and businesses if they are in danger of inadvertently infringing the rules or assisting IPR rightholders if the rules are actually infringed; emphasises, therefore, the importance of having an appropriate European legal framework for the protection of IPRs in connection with the use of AI;
2. Highlights that the consistent integration of AI in the education sector has the potential to meet some of the biggest challenges of education, to come up with innovative teaching and learning practices, and finally, to accelerate progress towards achieving the Sustainable Development Goals in order to meet the targets of the 2030 Agenda for Education;
3. Reiterates the importance of access to culture for every citizen throughout the Union; highlights in this context the importance of the exchange of best practices among Member States, educational facilities and cultural institutions and similar stakeholders; further considers it of vital importance that the resources available at both EU and national level are used to the maximum of their potential in order to further improve access to culture; stresses that there are a multitude of options to access culture and that all varieties should be explored in order to determine the most appropriate option; highlights the importance of consistency with the Marrakech Treaty;
4. Calls on the Commission to realise the full potential of artificial intelligence (AI) for the purposes of improving communication with citizens, through cultural and audiovisual online platforms, for example by keeping citizens informed of what is happening at decision-making level, narrowing the gap between the EU and the grassroots, and promoting social cohesion between EU citizens;
5. Highlights that education, culture and the audiovisual sector are sensitive areas for the use of AI and related technologies since they have the potential to impact our societies and the fundamental rights they uphold; contends, therefore, that legally binding ethical principles should be observed in their deployment, development and use;
6. Notes how artificial intelligence and related technologies may be used in developing or applying new methods of education in areas including language learning, academia generally, specialised learning, etc; highlights the importance not only of using such technologies for educational purposes but also of digital literacy and public awareness of the former; stresses the importance of providing educators, trainers and others with the right tools and know-how with regard to AI and related technologies in terms of what they are, how they are used and how to use them as tools properly and according to the law, so as to avoid IPR infringements; highlights in particular the importance of digital literacy for staff working in education, as well as of improving digital training for the elderly, considering that newer generations already have a basic notion of these technologies, having grown up with them;
7. Emphasises that European artificial intelligence should safeguard and promote core values of our Union such as democracy, independent and free media and information sources, quality education, environmental sustainability, gender balance and cultural and linguistic diversity;
8. Calls on the Commission, the Member States and the business community to actively and fully exploit the potential of AI in providing the facts and combating fake news, disinformation, xenophobia and racism on cultural and audiovisual online platforms, while at the same time avoiding censorship;
9. Notes that the independence of the creative process raises issues related to ownership of IPRs; considers, in this connection, that it would not be appropriate to seek to impart legal personality to AI technologies;
10. Notes that AI can play an important role in promoting and protecting our European and national cultural diversity, especially when used by audiovisual online platforms in promoting content to customers;
11. Notes that AI could benefit the research sector, for example through the role that predictive analytics can play in fine-tuning data analysis, for example on the acquisition and movement of cultural objects; stresses that the EU must step up investment and foster partnerships between industry and academia in order to enhance research excellence at European level;
12. Notes the important role which independent media play in culture and the daily life of citizens; stresses that fake media represent a fundamental problem, as copyright and IPRs generally are being constantly infringed; calls on the Commission, in cooperation with the Member States, to continue its work on raising awareness of this problem, countering the effects of fake media as well as the source problems; considers it of importance, furthermore, to develop educational strategies to improve digital literacy specifically in this regard;
13. Notes that AI-based software, such as image recognition software, could vastly enhance the ability of educational facilities and teachers to provide and develop modern, innovative and high-quality schooling methods, improve the digital literacy and e-skills of the entire population, and enable education to be more accessible; considers that such schooling methods should nevertheless be assessed as to their reliability and accuracy and should ensure fairness in education, non-discrimination, and the safety of children and minors both within educational facilities and when connected remotely within an educational context; highlights the importance of privacy and data protection legislation in order to ensure adequate protection of personal data, in particular children’s data, through transparent and reliable data sources respectful of IPRs; considers it vital that these technologies are only integrated into the existing systems if the protection of fundamental rights and privacy is an absolute given; stresses, however, that recognition software must be used only for educational purposes and not under any circumstances to monitor access to establishments; highlights in this regard the dependence on external data and a few market-dominating software providers; recalls that technologies procured with public money should be developed as open source software to enable the sharing and reuse of resources, making them available throughout the EU and thus increasing benefits and reducing public spending, while ensuring full respect for the applicable legislation, including Directive (EU) 2019/790 of the European Parliament and of the Council of 17 April 2019 on copyright and related rights in the Digital Single Market [20] ;
14. Notes that if the use of AI is to benefit the education and research sector, the EU must encourage training in the skills of the future, and in particular an ethical and responsible approach to AI technologies; adds, with that aim in view, that this training must not be reserved for pupils focusing on scientific and technical subjects, who are already more familiar with these tools, but must instead target as many people as possible, in particular in the younger generations;
15. Stresses that the need for investment in research and innovation regarding the use and development of AI and its cultural, educational and audiovisual applications is a key consideration in this respect; calls on the Commission to find additional funding to promote research and innovation regarding AI applications in these sectors;
16. Expresses serious concern that schools and other education providers are becoming increasingly dependent on educational technology services, including AI applications, provided by companies with a dominant market position, most of which are based outside the EU;
17. Underlines the need to ensure EU-wide digital and AI literacy, especially through the development of training opportunities for teachers; insists that the use of AI technologies in schools should contribute to narrowing the social and regional digital gap;
18. Highlights that the COVID-19 pandemic crisis can be considered as a probation period for the development and use of digital and AI-related technologies in the educational and cultural sectors, as exemplified by the many online schooling platforms and online tools for cultural promotion employed across the Member States; calls on the Commission, therefore, to take stock of those examples when considering a common EU approach to the increased use of such technological solutions;
19. Points out that data protection and privacy can be particularly seriously affected by AI; advocates compliance with the principles laid down in the General Data Protection Regulation (GDPR);
20. Calls on the Commission to take more effective steps to protect the personal data of pupils and teachers in the education sphere;
21. Emphasises that the interaction between AI and the creative industries is complex and requires an in‑depth assessment; welcomes the ongoing study ‘Trends and Developments in Artificial Intelligence - Challenges to the IPRS Framework’ and the study on ‘Copyright and new technologies: copyright data management and Artificial Intelligence’; underlines the importance of clarifying the conditions of use of copyright‑protected content as data input (images, music, films, databases, etc) and in the production of cultural and audiovisual outputs, whether created by humans with the assistance of AI or autonomously generated by AI technologies; invites the Commission to study the impact of AI on the European creative industries; reiterates the importance of European data and welcomes the statements made by the Commission in this regard, as well as the placing of artificial intelligence and related technologies high on the agenda;
22. Emphasises the role of an author’s personality for the expression of free and creative choices that constitute the originality of works [21] ; underlines the importance of limitations and exceptions to copyright when using content as data input, notably in education, academia and research, and in the production of cultural and audiovisual outputs, including user-generated content;
23. Emphasises that the interaction between AI and the creative industries is complex and requires an in-depth assessment; takes the view that consideration should be given to protecting AI-generated technical and artistic creations, in order to encourage this form of creativity;
24. Stresses that in the data economy context, better copyright data management is achievable, for the purpose of better remunerating authors and performers, notably in enabling the swift identification of the authorship and right ownership of content, thus contributing to lowering the number of orphan works; further highlights that AI technological solutions should be used to improve copyright data infrastructure and the interconnection of metadata in works, but also to facilitate the transparency obligation provided in Article 19 of the Directive on Copyright and related rights in the Digital Single Market for up‑to‑date, relevant and comprehensive information on the exploitation of authors’ and performers’ works and performances, particularly in the presence of a plurality of rightholders and of complex licensing schemes;
25. Stresses the need to work on the most efficient way of reducing bias in AI systems, in line with ethical and non-discrimination standards; underlines that data sets used to train AI should be as broad as possible in order to represent society in the best relevant way, that the outputs should be reviewed to avoid all forms of stereotypes, discrimination and biases, and, when appropriate, AI should be made use of to identify and correct human biases where they could exist; calls on the Commission to encourage and facilitate the sharing of de-biasing strategies for data;
26. Asks the Commission to assess the impact of AI and AI-related technologies on the audiovisual and creative sector, in particular with regard to authorship and related questions;
27. Calls for the intellectual property action plan announced by the Commission to address the question of AI and its impact on the creative sectors, taking account of the need to strike a balance between protecting IPRs and encouraging creativity in the areas of education, culture and research; considers that the EU can be a leader in the creation of AI technologies if it adopts an operational regulatory framework and implements proactive public policies, particularly as regards training programmes and financial support for research; asks the Commission to assess the impact of IPRs on the research and development of AI and related technologies, as well as on the audiovisual and creative sectors, in particular with regard to authorship, fair remuneration of authors and related questions;
28. Highlights the future role that the inclusion of AI-based technological tools should have in terms of conservation, disclosure and heritage control, as also in the associated research projects;
29. Stresses the need to strike a balance between, on the one hand, the development of AI systems and their use in the educational, cultural and audiovisual sectors and, on the other, measures to safeguard competition and market competitiveness for AI companies in these sectors; emphasises in this regard the need to encourage companies to invest in the innovation of AI systems used in these sectors, while at the same time ensuring that those providing such applications do not obtain a market monopoly;
30. Stresses that in no scenario could the use of AI and related technologies become a reality without human oversight; reiterates the importance of fundamental rights and the overarching supremacy of data and privacy protection legislation, which is imperative when dealing with such technologies;
31. Asks the Commission to assess the impact of AI and AI-related technologies in creating new audiovisual works such as deep fakes, and to establish appropriate legal consequences to be attached to their creation, production or distribution for malicious purposes;
32. Notes that automation and the development of AI could pose a threat to employment, and emphasises once again that priority must be given to safeguarding jobs, in particular in the education, culture and creative sectors;
33. Calls on the Commission to launch an EU-level education plan on digital and AI literacy, in coordination with the Member States, with a particular focus on school students and youth;
34. Calls on the Commission to consider the legal aspects of the outputs produced using AI technologies, as well as cultural content generated with the use of AI and related technologies; considers it important to support the production of cultural content; reiterates, however, the importance of safeguarding the Union’s unique IPR framework and that any changes should be made with the necessary due care, in order not to disrupt the delicate balance; calls on the Commission to produce an in-depth assessment with regard to the possible legal personality of AI-produced content, as well as the application of IPRs to AI-generated content and to content created with the use of AI tools;
35. Calls on the Commission to establish requirements for the procurement and deployment of artificial intelligence and related technologies by EU public sector bodies, to ensure compliance with Union law and fundamental rights; highlights the added value of instruments such as public consultations and impact assessments, to be run prior to the procurement or deployment of artificial intelligence systems, as recommended in the report of the Special Rapporteur to the UN General Assembly on AI and its impact on freedom of opinion and expression [22] ;
36. Calls on the Commission to lay down rules designed to guarantee effective data interoperability, in order to make content purchased on a platform accessible via any digital tool irrespective of brand;
37. Emphasises that the challenges brought by the use of artificial intelligence and related technologies can only be overcome by establishing data quality obligations and transparency and oversight requirements, in order to enable the public and authorities to assess compliance with Union law and fundamental rights; awaits the Commission’s proposals following its communication on a European strategy for data [23] as regards the sharing and pooling of datasets;
38. Calls on the Commission, in addition, to consider developing, in very close cooperation with Member States and the relevant stakeholders, verification mechanisms or systems for publishers, authors, creators, etc, in order to assist them in verifying what content they may use and to more easily determine what is protected under IPR legislation.
OPINION OF THE COMMITTEE ON WOMEN'S RIGHTS AND GENDER EQUALITY ( 14.9.2020 )
Rapporteur for opinion: Maria da Graça Carvalho
The Committee on Women’s Rights and Gender Equality calls on the Committee on Culture and Education, as the committee responsible, to incorporate the following suggestions into its motion for a resolution:
A. whereas gender equality is a core principle of the European Union enshrined in the Treaties, and should be reflected in all EU policies, including in education, culture, and the audiovisual sector, as well as in the development of technologies such as Artificial Intelligence (AI), these being key channels for changing attitudes and challenging stereotypes and gender biases in existing social norms; whereas the development of digitalisation and technologies like AI are fundamentally transforming our reality and their regulation today will highly influence our future societies; whereas there is a need to advocate for a human-centred approach anchored in human rights and ethics for the development and use of AI;
B. whereas Article 21 of the EU Charter of Fundamental Rights prohibits discrimination on a wide range of grounds and should be a guiding principle; whereas multiple forms of discrimination should not be reproduced in the design, input, development and use of AI systems based on gender-biased algorithms, or in the social contexts in which such algorithms are used;
C. whereas past experiences, especially in technical fields, have shown us that developments and innovations are often based mainly on male data and that women’s needs are not fully reflected; whereas addressing these biases requires greater vigilance, technical solutions and the development of clear requirements of fairness, accountability and transparency;
D. whereas incomplete and inaccurate data sets, the lack of gender-disaggregated data and incorrect algorithms can distort the processing of an AI system and jeopardise the achievement of gender equality in society; whereas data on disadvantaged groups and intersectional forms of discrimination tend to be incomplete and even absent;
E. whereas gender inequalities, stereotypes and discrimination can also be created and replicated through the language and images disseminated by the media and AI-powered applications; whereas education, cultural programmes and audiovisual content have considerable influence in shaping people’s beliefs and values and are a fundamental tool for combatting gender stereotypes, decreasing the digital gender gap, and establishing strong role models; whereas an ethical and regulatory framework must be in place ahead of implementing automatised solutions for these key areas in society;
F. whereas science and innovation can bring life-changing benefits, especially for those who are furthest behind, such as women and girls living in remote areas; whereas scientific education is important for obtaining skills, decent work, and jobs of the future, as well as for breaking with gender stereotypes that regard these as stereotypically masculine fields; whereas science and scientific thinking are key to democratic culture, which in turn is fundamental for advancing gender equality;
G. whereas women are significantly under-represented in the AI sector, whether as creators, developers or consumers; whereas the full potential of women’s skills, knowledge and qualifications in the digital and AI fields as well as that of information, communication and technology (ICT), along with their reskilling, can contribute to boosting the European economy; whereas globally only 22 % of AI professionals are female; whereas the lack of women in AI development not only increases the risk of bias, but also deprives the EU of diversity, talent, vision and resources, and is therefore an obstacle to innovation; whereas gender diversity enhances female attitudes in teams and team performance and favours the potential for innovation in both public and private sectors;
H. whereas in the EU one woman in ten has already suffered some form of cyberviolence since the age of 15 and cyberharassment remains a concern in the development of AI, including in education; whereas cyberviolence is often directed at women in public life, such as activists, women politicians and other public figures; whereas AI and other emerging technologies can play an important role in preventing cyberviolence against women and girls and educating people;
I. whereas the EU is facing an unparalleled shortage of women in Science, Technology, Engineering and Mathematics (STEM) careers and education, given that women account for 52 % of the European population, yet only for one in three of STEM graduates;
J. whereas despite the positive trend in the involvement and interest of women in STEM education, the percentages remain insufficient, especially considering the importance of STEM-related careers in an increasingly digitalised world;
1. Considers that AI has great potential to promote gender equality provided that already existing conscious and unconscious bias are eliminated; stresses the need for further regulatory efforts to ensure that AI respects the principles and values of gender equality and non-discrimination as enshrined in Article 21 of the Charter of Fundamental Rights; stresses, further, the importance of accountability, of a differentiated and transparent risk-based approach, and of continuous monitoring of existing and new algorithms and of their results;
2. Stresses the need for media organisations to be informed about the main parameters of algorithm-based AI systems that determine ranking and search results on third-party platforms, and for users to be informed about the use of AI in decision-making services and empowered to set their privacy parameters via transparent and understandable measures;
3. Recalls that algorithms and AI should be ‘ethical by design’, with no built-in bias, in a way that guarantees maximum protection of fundamental rights;
4. Calls for policies targeted at increasing the participation of women in the fields related to STEM, AI and the research and innovation sector, and for the adoption of a multi-level approach to address the gender gap at all levels of education, with particular emphasis on primary education, as well as employment in the digital sector, highlighting the importance of upskilling and reskilling;
5. Recognises that gender stereotyping, cultural discouragement and the lack of awareness and promotion of female role models hinders and negatively affects girls’ and women’s opportunities in ICT, STEM and AI and leads to discrimination and fewer opportunities for women in the labour market; stresses the importance of increasing the number of women in these sectors, which will contribute to women’s participation and economic empowerment, as well as to reducing the risks associated with the creation of so-called ‘biased algorithms’;
6. Encourages the Commission and the Member States to purchase educational, cultural and audiovisual services from providers that apply gender balance in their workplace, promote public procurement policies and guidelines that stimulate companies to hire more women for STEM jobs, and facilitate the distribution of funds to companies in the educational, cultural and audiovisual sectors that take account of gender balance criteria;
7. Emphasises the cross-sectoral nature of gender-based discrimination rooted in conscious or unconscious gender bias and manifested in the education sector, the portrayal of women in the media and advertising on-screen and off-screen, and the responsibility of both public and private sectors in terms of proactively recruiting, developing and retaining female talent and instilling an inclusive business culture;
8. Calls on the Commission and the Member States to take into account ethical aspects, including from a gender perspective, when developing AI policy and legislation, and, if necessary, to adapt the current legislation, also including EU programmes and ethical guidelines for the use of AI;
9. Encourages the Member States to enact a strategy to promote women’s participation in STEM, ICT and AI-related studies and careers in relevant existing national strategies to achieve gender equality, defining a target for the participation of women researchers in STEM and AI projects; urges the Commission to address the gender gap in STEM, ICT and AI-related careers and education, and to set this as a priority of the Digital Skills Package in order to promote the presence of women at all levels of education, as well as in the upskilling and reskilling of the labour force;
10. Recognises that producers of AI solutions must make a greater effort to test products thoroughly in order to anticipate potential errors impacting vulnerable groups; calls for work to be stepped up on a tool to teach algorithms to recognise disturbing human behaviour, which would identify those elements that most frequently contribute to discriminatory mechanisms in the automated decision-making processes of algorithms;
11. Underlines the importance of ensuring that the interests of women experiencing multiple forms of discrimination and who belong to marginalised and vulnerable groups are adequately taken into account and represented in any future regulatory framework; notes with concern that marginalised groups risk suffering new technological, economic and social divides with the development of AI;
12. Calls for specific measures and legislation to combat cyberviolence; stresses that the Commission and the Member States should provide appropriate funding for the development of AI solutions that prevent and fight cyberviolence and online sexual harassment and exploitation directed against women and girls and help educate young people; calls for the development and implementation of effective measures tackling old and new forms of online harassment for victims in the workplace;
13. Notes that for the purpose of analysing the impacts of algorithmic systems on citizens, access to data should be extended to appropriate parties, notably independent researchers, media and civil society organisations, while fully respecting Union data protection and privacy law; points out that users must always be informed when an algorithm has been used to make a decision concerning them, particularly where the decision relates to access to benefits or to a product;
14. Calls on the Commission and the Member States to devise measures that fully incorporate the gender dimension, such as awareness-raising campaigns, training and curricula, which should provide information to citizens on how algorithms operate and their impact on their daily lives; further calls on them to nurture gender-equal mindsets and working conditions that lead to the development of more inclusive technology products and work environments; urges the Commission and the Member States to ensure the inclusion of digital skills and AI training in school curricula and to make them accessible to all, as a way to close the digital gender divide;
15. Stresses the need for training for workers and educators dealing with AI to promote the ability to identify and correct gender-discriminatory practices in the workplace and in education, and for workers developing AI systems and applications to identify and remedy gender-based discrimination in the AI systems and applications they develop; calls for the establishment of clear responsibilities in companies and educational institutions to ensure that there is no gender-based discrimination in the workplace or educational context; highlights that genderless images of AI and robots should be used for educational and cultural purposes, unless gender is a key factor for some reason;
16. Highlights the importance of the development and deployment of AI applications in the educational, cultural and audiovisual sectors in collecting gender-disaggregated and other equality data, and of applying modern machine learning de-biasing techniques, if needed, to correct gender stereotypes and gender biases which may have negative impacts;
17. Urges the Commission and the Member States to collect gender-disaggregated data in order to feed datasets in a way that promotes equality; also calls on them to measure the impact of the public policies put in place to incorporate the gender dimension by analysing the data collected; stresses the importance of using complete, reliable, timely, unbiased, non-discriminatory and gender-sensitive data in the development of AI;
18. Calls on the Commission to include education in the regulatory framework for high-risk AI applications, given the importance of ensuring that education continues to contribute to the public good, as well as the high sensitivity of data on pupils, students and other learners; emphasises that in the education sector, this deployment should involve educators, learners and the wider society and should take into account the needs of all and the expected benefits in order to ensure that AI is used purposefully and ethically;
19. Calls on the Commission to encourage the use of EU programmes such as Horizon Europe, Digital Europe and Erasmus+ to promote multidisciplinary research, pilot projects, experiments and the development of tools including training, for the identification of gender biases in AI, as well as awareness-raising campaigns for the general public;
20. Stresses the need to create diverse teams of developers and engineers to work alongside the main actors in the educational, cultural and audiovisual sectors in order to prevent gender or social bias being inadvertently included in AI algorithms, systems and applications; stresses the need to consider the variety of different theories through which AI has been developed to date and could be further advanced in the future;
21. Points out that the fact of taking due care to eliminate bias and discrimination against particular groups, including gender stereotypes, should not halt technological progress.
- [1] OJ C 202 I, 16.6.2020, p. 1.
- [2] OJ C 440, 6.12.2018, p. 37.
- [3] OJ C 449, 23.12.2020, p. 37.
- [4] OJ C 433, 23.12.2019, p. 42.
- [5] OJ C 28, 27.1.2020, p. 8.
- [6] OJ C 252, 18.7.2018, p. 239.
- [7] OJ C 307, 30.8.2018, p. 163.
- [8] Regulation (EU) 2016/679 of the European Parliament and of the Council of 27 April 2016 on the protection of natural persons with regard to the processing of personal data and on the free movement of such data, and repealing Directive 95/46/EC (OJ L 119, 4.5.2016, p. 1).
- [9] Directive 2002/58/EC of the European Parliament and of the Council of 12 July 2002 concerning the processing of personal data and the protection of privacy in the electronic communications sector (Directive on privacy and electronic communications) (OJ L 201, 31.7.2002, p. 37).
- [10] Report of the UN Special Rapporteur on the promotion and protection of the right to freedom of opinion and expression, 29 August 2018.
- [11] Directive 2005/36/EC of the European Parliament and of the Council of 7 September 2005 on the recognition of professional qualifications (OJ L 255, 30.9.2005, p. 22).
- [12] Court of Justice of the European Union, Case C-833/18, SI and Brompton Bicycle Ltd v Chedech / Get2Get.
- [13] Directive (EU) 2019/790 of the European Parliament and of the Council of 17 April 2019 on copyright and related rights in the Digital Single Market and amending Directives 96/9/EC and 2001/29/EC (OJ L 130, 17.5.2019, p. 92).
- [14] Regulation (EU) 2019/1150 of the European Parliament and of the Council of 20 June 2019 on promoting fairness and transparency for business users of online intermediation services (OJ L 186, 11.7.2019, p. 57).
- [15] Directive (EU) 2019/2161 of the European Parliament and of the Council of 27 November 2019 amending Council Directive 93/13/EEC and Directives 98/6/EC, 2005/29/EC and 2011/83/EU of the European Parliament and of the Council as regards the better enforcement and modernisation of Union consumer protection rules (OJ L 328, 18.12.2019, p. 7).
- [16] Directive 2000/31/EC of the European Parliament and of the Council of 8 June 2000 on certain legal aspects of information society services, in particular electronic commerce, in the Internal Market (OJ L 178, 17.7.2000, p. 1).
- [17] Directive (EU) 2018/1808 of the European Parliament and of the Council of 14 November 2018 amending Directive 2010/13/EU on the coordination of certain provisions laid down by law, regulation or administrative action in Member States concerning the provision of audiovisual media services in view of changing market realities (OJ L 303, 28.11.2018, p. 69).
- [18] Regulation (EU) 2016/679 of the European Parliament and of the Council of 27 April 2016 on the protection of natural persons with regard to the processing of personal data and on the free movement of such data, and repealing Directive 95/46/EC (General Data Protection Regulation) (OJ L 119, 4.5.2016, p. 1).
- [19] Directive 2002/58/EC of the European Parliament and of the Council of 12 July 2002 concerning the processing of personal data and the protection of privacy in the electronic communications sector (Directive on privacy and electronic communications) (OJ L 201, 31.7.2002, p. 37).
- [20] OJ L 130, 17.5.2019, p. 92.
- [21] Court of Justice of the European Union, SI and Brompton Bicycle Ltd v Chedech Get2Get, Case C-833/18.
- [22] Report of the Special Rapporteur on the promotion and protection of the right to freedom of opinion and expression, https://undocs.org/A/73/348.
- [23] COM(2020) 66 final, https://ec.europa.eu/info/sites/info/files/communication-european-strategy-data-19feb2020_en.pdf.
Cookies on GOV.UK
We use some essential cookies to make this website work.
We’d like to set additional cookies to understand how you use GOV.UK, remember your settings and improve government services.
We also use cookies set by other sites to help us deliver content from their services.
You have accepted additional cookies. You can change your cookie settings at any time.
You have rejected additional cookies. You can change your cookie settings at any time.

- Business and industry
- Science and innovation
- Artificial intelligence
Ofqual’s approach to regulating the use of artificial intelligence in the qualifications sector
Published in response to a request from the Secretary of State for Education and the Secretary of State for Science, Innovation and Technology.
Applies to England
Ofqual’s approach to regulating the use of artificial intelligence in the qualifications sector.
Ref: Ofqual/24/7130
The Office of Qualifications and Examinations Regulation (Ofqual) is the independent regulator of qualifications and assessments for England.
The emergence of widely accessible generative artificial intelligence (AI) in 2023 raised several new considerations for the regulation of qualifications and assessments. These include new opportunities for AI to support the design, development and delivery of high-quality assessment, alongside new risks around AI’s use in non-exam assessments. Ofqual has established 5 key objectives that have shaped our AI regulatory work:
- Ensuring fairness for students
- Maintaining validity of qualifications
- Protecting security
- Maintaining public confidence
- Enabling innovation
This position statement is published in response to a request from the Secretary of State for Education and the Secretary of State for Science, Innovation and Technology . It sets out Ofqual’s approach to regulating AI, and how our objectives connect with the overarching principles set out in the government’s A pro-innovation approach to AI regulation White Paper.
Is this page useful?
- Yes this page is useful
- No this page is not useful
AWS Public Sector Blog
The transformative power of generative artificial intelligence for the public sector.
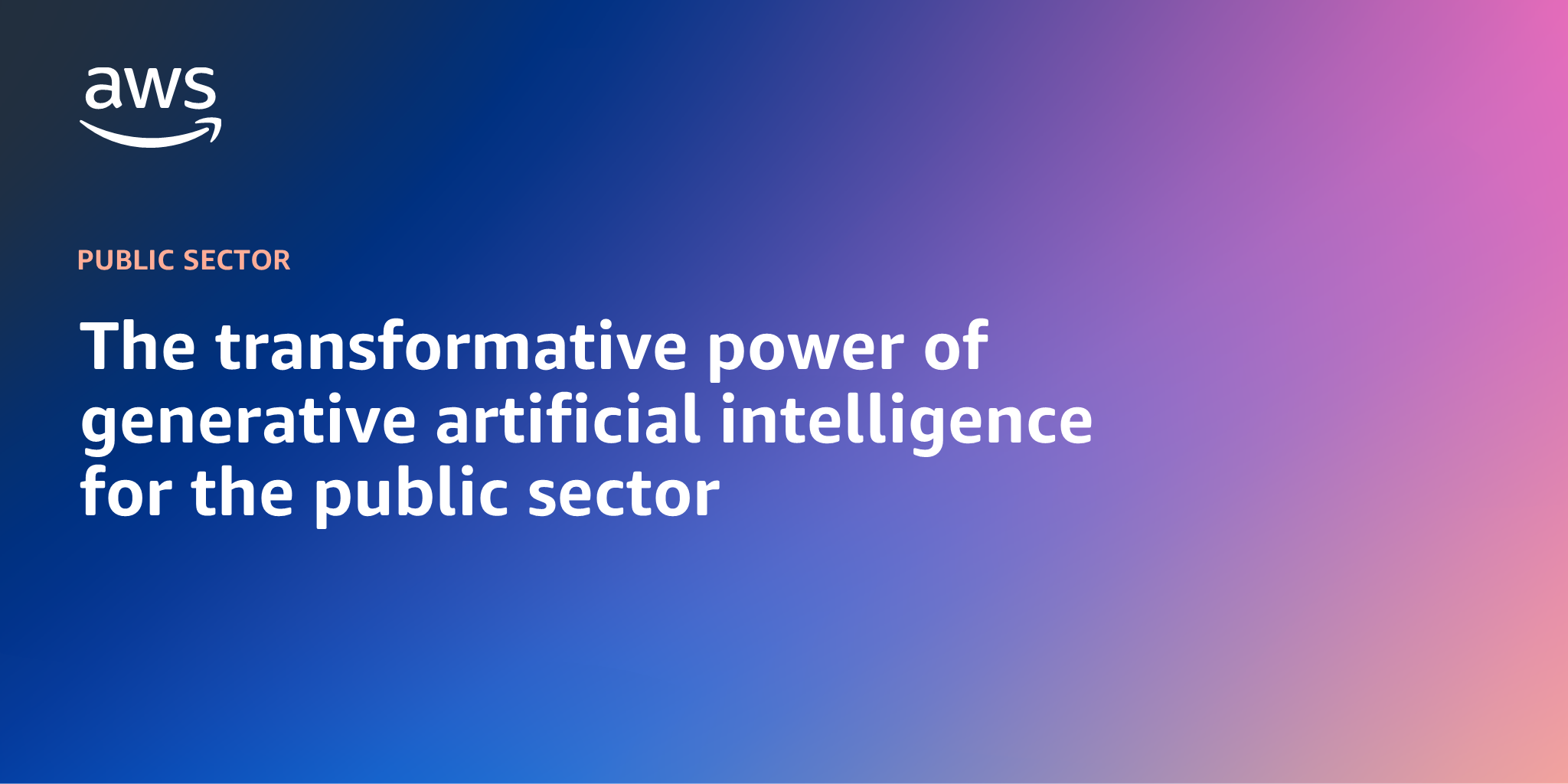
Generative artificial intelligence (AI) has recently captured widespread attention, and for good reason. This emerging technology has the potential to transform customer experiences, create novel applications, help people reach new levels of productivity, and much more. In fact, according to research from Goldman Sachs , generative AI could drive a 7 percent—or almost $7 trillion—increase in global GDP and lift worker productivity by 1.5 percentage points over a 10-year period.
Applications and user experiences are poised to be reinvented with generative AI, and the public sector is no exception. Governments, education institutions, nonprofits, and health systems must constantly adapt and innovate to meet the changing needs of their constituents, students, beneficiaries, and patients. If used responsibly, this powerful technology can open doors to endless possibilities to increase creativity, productivity, and progress. Amazon Web Services (AWS) is striving to make it possible for developers of all skill levels and for organizations of all sizes to innovate using generative AI.
The power of AI on AWS
New, simple-to-use generative AI tools such as Amazon Bedrock and Amazon SageMaker are democratizing the use of generative AI, making it accessible to anyone. Amazon Bedrock provides organizations the flexibility to choose from a wide range of foundation models (FMs), including open source and proprietary models built by leading AI startups, as well as Amazon, to identify the appropriate base model and build differentiated applications using their own data. The customer controls how their data is shared and used while remaining protected, secure, and private during the process. None of the customer’s data is used to train the original base models, and since all data is encrypted and does not leave a customer’s virtual private cloud (VPC), customers can trust that their data will remain private and confidential. Amazon SageMaker helps complete each step of the data preparation workflow, including data selection, cleansing, exploration, bias detection, and visualization from a single visual interface in minutes to easily deploy and customize FMs.
Responsible AI is an integral part of the entire AI lifecycle at AWS including design and development, deployment, and ongoing use. The relevance of responsible AI will only grow and that is why we have dedicated AWS experts wholly committed to stay on the cutting-edge of research, provide best practices, and develop rigorous methodology to build our AWS AI and machine learning (ML) services in a responsible way. We help customers transform responsible AI from theory into practice—by giving them the tools, guidance, and resources they need to get started with purpose-built services and features, such as Amazon SageMaker Clarify , Amazon SageMaker Model Monitor , and ML Governance from Amazon SageMaker .
To continue supporting best practices in the responsible use of AI, Amazon Titan FMs available within Amazon Bedrock are built to detect and remove harmful content in the data, reject inappropriate content in the user input, and filter the models’ outputs that contain inappropriate content (such as hate speech, profanity, and violence). Additionally, with Amazon Bedrock’s serverless experience, customers can get started quickly without having to manage any infrastructure. Whatever task customers are trying to accomplish with FMs—running them, building them, customizing them—they need the highest-performing, most cost-effective infrastructure that is purpose-built for ML: the AWS Cloud.
AI in the public sector
In today’s digital world, citizens expect the same world-class technology experience from government that they expect when they log in to their favorite streaming service or shop online. Generative AI is helping organizations to meet these expectations by tailoring services to individual citizens’ needs across a breadth of platforms.
Large language models (LLMs) and FMs can process and analyze vast amounts of data, oftentimes much faster and more efficiently than a human could. AWS supports government, education, nonprofit, aerospace and satellite, and healthcare organizations using generative AI to help query and analyze large amounts of information—such as public health data, economic indicators, and crime statistics—to identify patterns, trends, and correlations. This kind of analysis provides organizations with a comprehensive view of a particular topic, which in turn allows them to be more proactive in carrying out their mission. For example, the AWS Cloud Innovation Center (CIC) at California Polytechnic State University enabled students and staff to build a secure, cloud-based tool that makes cybersecurity recommendations for public sector organizations. Working with the San Diego Cyber Center of Excellence and Regional Cyber Lab , the My eCISO generative AI-enabled chatbot was developed to provide cybersecurity recommendations after a user answers a series of open-ended questions. Inputs and chat responses are securely stored and accessible to only those who have been granted access.
Generative AI is transforming how researchers work, helping them find information faster and freeing them up to pursue new experimental designs. It is helping researchers accelerate their work by creating highly complex levels of analysis that would traditionally take days, months, or even years. UK Biobank , for example, is a large-scale biomedical database and research resource, containing in-depth genetic and health information from 500,000 UK participants. It currently holds 17 million samples totaling 32 petabytes of data. Thousands of new samples are added daily as well as new datasets, such as whole genome sequencing, and UK Biobank is looking toward generative AI and cloud-based tools to help in analyzing the vast quantities of different types of data. The database is regularly augmented with additional data and is globally accessible to approved researchers undertaking vital research into the most common and life-threatening diseases.
The future of AI
According to an AWS funded study, AI is already transforming the workplace —from how businesses operate to how work gets done. What’s more, the pace of change is startling, with more than 90 percent of surveyed employers predicting that they will use AI-related solutions in their organizations by 2028. Generative AI simplifies routine tasks, allowing humans to concentrate on more important mission goals. Automating some processes and decreasing reliance on manual labor not only frees up human effort for crucial undertakings but also results in substantial cost savings over time.
While still in the early days, we believe that generative AI will greatly impact how public sector organizations operate, implement, and manage services and serve citizens and end users. As public sector organizations develop solutions powered by generative AI, it is important we understand how we should build responsible AI products and services. It’s crucial to adopt a people-centric perspective, which goes beyond just technology and incorporates people, processes, and culture. AWS is integrating responsible AI throughout the development cycle for our engineering and development teams. We equip our customers with the necessary resources, guidance, and tools to convert responsible AI concepts into actual practices. We continuously innovate and refine our responsible AI strategy to enhance and broaden our knowledge of the field as generative AI evolves. Although it’s challenging work, we’re constantly learning and growing in the realm of responsible AI.
I encourage you to gain a better understanding of generative AI and how public sector organizations are using these technologies. A couple of ways you can do this is by taking a masterclass on the best uses of AI for governments and checking out this Get started with generative AI on AWS eBook specifically for public sector.
- AWS in the Public Sector
- AWS for Government
- AWS for Education
- AWS for Nonprofits
- AWS for Public Sector Health
- AWS for Aerospace and Satellite Solutions
- Case Studies
- Fix This Podcast
- Additional Resources
- AWS for Government Twitter
- AWS Education Twitter
- AWS Nonprofits Twitter
- Newsletter Subscription
- Australia edition
- International edition
- Europe edition
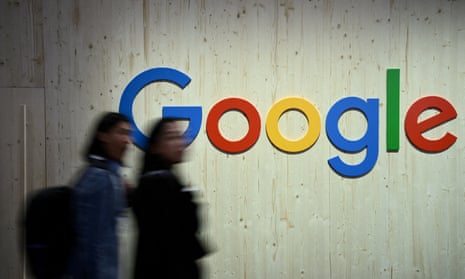
Google parent Alphabet hits $2tn valuation as it announces first dividend
Tech company’s shares rise as it plans to reward investors after strong quarterly results
Google’s parent company has hit a stock market value of $2tn (£1.6tn) as investors reacted to a declaration of its first ever dividend alongside strong results on Thursday.
Shares in Alphabet rose 10% in early Wall Street trading on Friday to give the tech group a stock market capitalisation – a measure of a corporation’s value – of more than $2tn. Alphabet last hit that level in intraday trading in 2021, but has yet to close above that benchmark after a day’s trading.
Alphabet’s shares rose after it posted results on Thursday that exceeded analyst’s expectations. Microsoft also reported strong figures on Thursday , amid heavy investment in artificial intelligence, and investors pushed the company past the $3tn mark, a level it has already crossed this year.
after newsletter promotion
Alphabet’s quarterly figures included better than expected results from its core Google search business as well as its YouTube platform, and strong figures from its cloud business, which has been boosted by the training and operation of artificial intelligence models. The company also announced its first ever dividend.
Russ Mould, the investment director at AJ Bell, an investment platform, said Alphabet joining the ranks of dividend-paying tech company was a “sign of the times”.
“Big tech firms have enjoyed stellar growth over the past decade and while most remain highly innovative, their cashflows have become so strong that there’s oodles of money left over post-reinvestment in the business to reward shareholders,” he added.
Alphabet joins a trio of US-listed companies with valuations of more than $2tn: Microsoft at more than $3tn; Apple at $2.6tn; and Nvidia, the leading chip supplier for AI products, at just more than $2tn. Apple also passed the $3tn mark last year.
- Technology sector
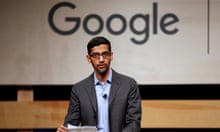
Alphabet hails ‘once-in-a-generation’ AI opportunity as revenue rises
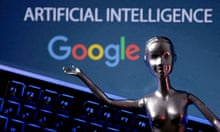
If costs force Google to charge for AI, competitors will cheer
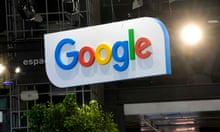
Google fined €250m in France for breaching intellectual property deal
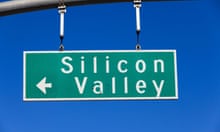
Joe Biden has just dealt a big defeat to big tech
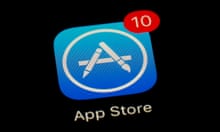
Apple reportedly faces €500m fine from EU over music streaming access
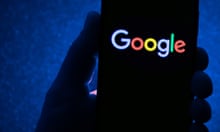
Google stops notifying publishers of ‘right to be forgotten’ removals from search results
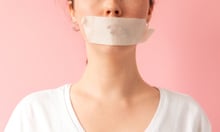
The TikTok mouth-taping trend may not be as beneficial as you’re told
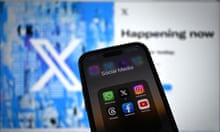
Social media firms ‘not ready to tackle misinformation’ during global elections
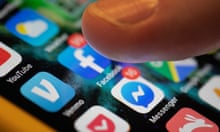
EU unveils ‘revolutionary’ laws to curb big tech firms’ power
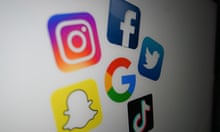
How the EU Digital Services Act affects Facebook, Google and others
Most viewed.
We couldn’t find any results matching your search.
Please try using other words for your search or explore other sections of the website for relevant information.
We’re sorry, we are currently experiencing some issues, please try again later.
Our team is working diligently to resolve the issue. Thank you for your patience and understanding.
News & Insights

Why Microsoft, Amazon, Alphabet, and Other "Magnificent Seven" Stocks Crashed on Thursday
April 25, 2024 — 02:10 pm EDT
Written by Danny Vena for The Motley Fool ->
The biggest driver of headlines across the technology sector over the past year or so has been the proliferation of artificial intelligence (AI). Many of the world's biggest companies see a vast opportunity resulting from AI and are investing accordingly to stake their claim in the AI revolution. What some investors weren't prepared for, however, is the level of spending necessary to reap the windfall that AI is expected to unleash over the next few years.
With that as a backdrop, Microsoft (NASDAQ: MSFT) slumped 3.4%, Amazon (NASDAQ: AMZN) was off 2.5%, and Alphabet (NASDAQ: GOOGL) (NASDAQ: GOOGL) fell 2.2%, as of 1:05 p.m. ET on Thursday.
To be clear, there was very little company-specific news driving these so-called " Magnificent Seven " stocks lower today (more on that in a bit). This supports the conclusion that investors are reacting to the quarterly results reported by Meta Platforms (NASDAQ: META) and what it means for other big players in the AI space.
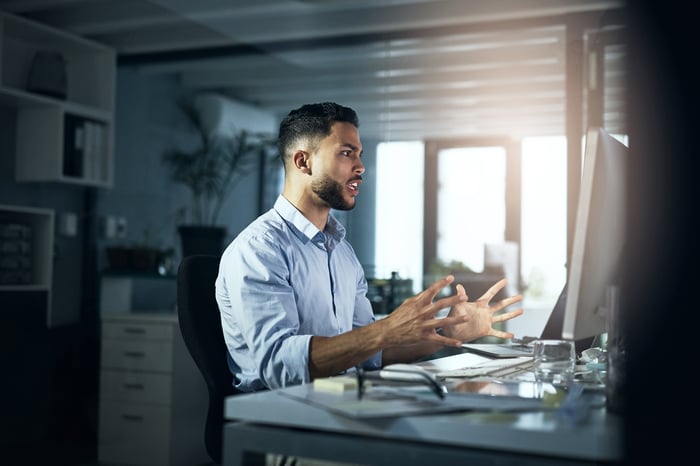
Image source: Getty Images.
You have to spend money to make money
Meta Platforms released its first-quarter report after the market closed on Wednesday, and while the results were better than expected, there was one revelation that caught investors off guard.
The company reported revenue of $36.4 billion, up 27% year over year. Expenses advanced at a more modest pace, growing just 6%. This benefited operating margins, which surged to 38%, up from 25% in the year-ago quarter. This also helped expand Meta's profits, as earnings per share (EPS) of $4.71 soared 114%.
The results easily surpassed expectations, as analysts' consensus estimates forecast revenue of $36.14 billion and EPS of $4.32. However, it was an update to the company's full-year spending plans that sent the stock reeling.
Like its Magnificent Seven counterparts, Meta has been at the forefront of generative AI , eager to earn its part of the trillions of dollars the technology is expected to generate over the coming decade or so. However, "there ain't no such thing as a free lunch," as the saying goes, and these systems are costly to develop. Not only is there the cost of the AI models themselves to consider, but the data center infrastructure necessary to maintain them.
Meta has already created its large language model Meta AI (Llama) AI system, which is recognized as one of the top AI systems in the world -- but this is just the beginning. As CEO Mark Zuckerberg noted, "We're investing and scaling a new product, but aren't yet monetizing it."
As a result, Meta increased its full-year forecast for capital expenditures to between $35 billion and $40 billion, up from its previous expectations of $30 billion to $37 billion. Meta said, "We continue to accelerate our infrastructure investments to support our AI roadmap." Meta also expects capital spending to be even higher in 2025 as it works to capture the AI opportunity.
United in the pursuit of AI
So what does this have to do with Microsoft, Amazon, and Alphabet? The common thread that weaves these tech stalwarts together is their AI aspirations, and each of the three has also been spending heavily in order to reap the rewards of AI. Fair-weather investors took Meta's AI announcement as an indication that big tech's AI-induced spending spree will negatively impact results -- but we don't yet have evidence to suggest that's the case.
Microsoft and Alphabet are scheduled to report their quarterly results after market close today, and Amazon is up next week, so some investors were selling preemptively in the event these stocks might sell off as well. Investors with a long-term outlook, however, will recognize that this is just the beginning of a multiyear profit opportunity, and volatility is the cost of admission.
Company-specific news for our trio of stocks was decidedly mixed:
- UBS analyst Stephen Ju maintained a buy rating on Amazon stock, but raised his price target to $215, which suggests 22% upside compared to Wednesday's closing price. The analyst sees potential for improvement in a broad cross-section of Amazon's business interests this year. This follows two price target increases by other analysts yesterday.
- Chinese hackers accessed U.S. government emails last year, something Microsoft could have prevented, according to a report by the Cyber Safety Review Board. The company has lost face and is working to rebuild trust in its systems, according to numerous reports.
- The potential for a TikTok ban, which was signed into law today by President Biden, could benefit Meta Platforms, Amazon, and Alphabet, according to a report in The Washington Post .
Investors would do well to step back and look at the big picture when it comes to AI. Analysts at Goldman Sachs Research suggest that as AI makes its way into business systems and the rest of society, it could generate as much as $7 trillion over the next 10 years. That's quite a windfall for the companies positioned to participate, which include Microsoft, Amazon, Alphabet, and Meta Platforms.
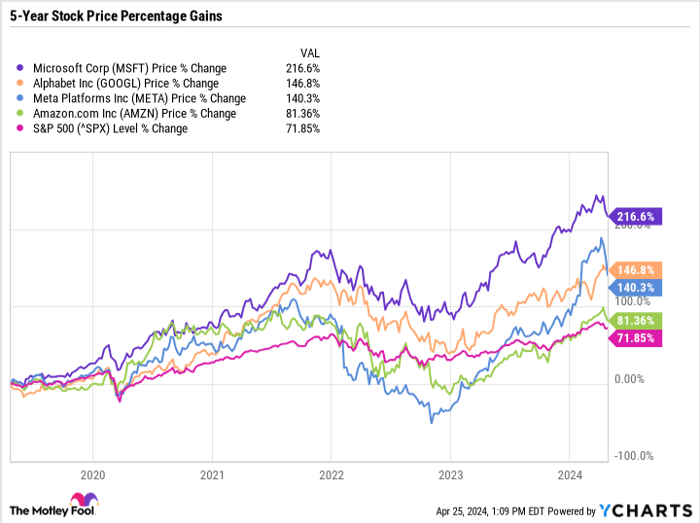
Data by YCharts
When viewed in the context of this significant opportunity, their valuations are compelling. Microsoft, Alphabet, and Meta Platforms are selling for 33 times, 23 times, and 22 times forward earnings, respectively, while Amazon is trading for roughly 2 times forward sales. Furthermore, all four stocks have easily outpaced the performance of the S&P 500 over the past five years.
Microsoft, Alphabet, Amazon, and Meta Platforms are all industry leaders on the cutting edge of AI. Investors should ignore the short-term volatility and buy the dip.
Where to invest $1,000 right now
When our analyst team has a stock tip, it can pay to listen. After all, the newsletter they have run for two decades, Motley Fool Stock Advisor , has more than tripled the market.*
They just revealed what they believe are the 10 best stocks for investors to buy right now… and Microsoft made the list -- but there are 9 other stocks you may be overlooking.
See the 10 stocks
*Stock Advisor returns as of April 22, 2024
Randi Zuckerberg, a former director of market development and spokeswoman for Facebook and sister to Meta Platforms CEO Mark Zuckerberg, is a member of The Motley Fool's board of directors. Suzanne Frey, an executive at Alphabet, is a member of The Motley Fool’s board of directors. John Mackey, former CEO of Whole Foods Market, an Amazon subsidiary, is a member of The Motley Fool’s board of directors. Danny Vena has positions in Alphabet, Amazon, Meta Platforms, and Microsoft. The Motley Fool has positions in and recommends Alphabet, Amazon, Goldman Sachs Group, Meta Platforms, and Microsoft. The Motley Fool recommends the following options: long January 2026 $395 calls on Microsoft and short January 2026 $405 calls on Microsoft. The Motley Fool has a disclosure policy .
The views and opinions expressed herein are the views and opinions of the author and do not necessarily reflect those of Nasdaq, Inc.

Stocks mentioned
More related articles.
This data feed is not available at this time.
Sign up for the TradeTalks newsletter to receive your weekly dose of trading news, trends and education. Delivered Wednesdays.
To add symbols:
- Type a symbol or company name. When the symbol you want to add appears, add it to My Quotes by selecting it and pressing Enter/Return.
- Copy and paste multiple symbols separated by spaces.
These symbols will be available throughout the site during your session.
Your symbols have been updated
Edit watchlist.
- Type a symbol or company name. When the symbol you want to add appears, add it to Watchlist by selecting it and pressing Enter/Return.
Opt in to Smart Portfolio
Smart Portfolio is supported by our partner TipRanks. By connecting my portfolio to TipRanks Smart Portfolio I agree to their Terms of Use .
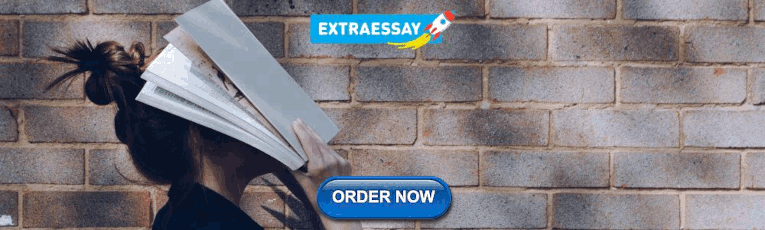
COMMENTS
Artificial Intelligence (AI) has the potential to address some of the biggest challenges in education today, innovate teaching and learning practices, and accelerate progress towards SDG 4. However, rapid technological developments inevitably bring multiple risks and challenges, which have so far outpaced policy debates and regulatory frameworks.
Advances in artificial intelligence (AI) could transform education systems and make them more equitable. It can accelerate the long overdue transformation of education systems towards inclusive learning that will prepare young people to thrive and shape a better future.; At the same time, teachers can use these technologies to enhance their teaching practice and professional experience.
The 2023 AI Index Report from the Stanford Institute for Human-Centered AI has documented notable acceleration of investment in AI as well as an increase of research on ethics, including issues of fairness and transparency.2 Of course, research on topics like ethics is increasing because problems are observed.
From unique educational perspectives, this article reports a comprehensive review of selected empirical studies on artificial intelligence in education (AIEd) published in 1993-2020, as collected in the Web of Sciences database and selected AIEd-specialized journals. ... The AI market in US Education Sector is expected to grow by 48% in 2018 ...
Today, the U.S. Department of Education's Office of Educational Technology (OET) released a new report, "Artificial Intelligence (AI) and the Future of Teaching and Learning: Insights and Recommendations" that summarizes the opportunities and risks for AI in teaching, learning, research, and assessment based on public input. This report is part of the Biden-Harris Administration's ongoing ...
The McKinsey Global Institute's 2018 report on the future of work suggests that, despite the dire predictions, teachers are not going away any time soon. In fact, we estimate the school teachers will grow by 5 to 24 percent in the United States between 2016 and 2030. For countries such as China and India, the estimated growth will be more ...
ChatGPT, a popular artificial intelligence tool that can generate written materials in response to prompts, reached 100 million monthly active users in January 2023, just two months after its launch. The unprecedented escalation made OpenAI's creation the fastest-growing consumer application in history, according to a report from UBS based on analytics from Similarweb.
emerging fundamental changes in education due to the use of technologies and their impact on education and other spheres of human life. The first issue in the series is the policy brief on Artificial Intelligence (AI), which promises enormous benefit to students, teachers, school leaders, parents and education administrators.
This systematic review provides unique findings with an up-to-date examination of artificial intelligence (AI) in higher education (HE) from 2016 to 2022. Using PRISMA principles and protocol, 138 articles were identified for a full examination. Using a priori, and grounded coding, the data from the 138 articles were extracted, analyzed, and coded. The findings of this study show that in 2021 ...
The first international workshop on multimodal artificial intelligence in education is being organized at AIEd conference to promote the importance of multimodal data in AIEd. ... The ethical implications of AI in education are dependent on the kind of disruption AI is doing in the ed-tech sector. On the one hand, this can be at an individual ...
Although artificial intelligence presents novel concerns for the education sector, most teachers we surveyed reported a positive outlook on the future. Teachers Want More Education To Understand ...
Five pros of AI in education. Assistance. Teachers who've tried AI have found that it can help make their jobs easier, from coming up with lesson plans to generating student project ideas to creating quizzes. With assistance from artificial intelligence, teachers can gain more time to spend with their students.3. Speed.
Abstract: Building intelligent computers that can carry out tasks that traditionally require human intelligence is the goal of artificial intelligence (AI), a broad field of computer science. While there are many different approaches to AI, it is an interdisciplinary discipline, and recent developments in machine learning and deep learning in particular are causing a paradigm change in almost ...
For National AI Literacy Day 2024, explore the AI in education professional development opportunities available from Microsoft. ... "Empower educators to explore the potential of artificial intelligence," "Enhance teaching and learning with Microsoft Copilot," and "Equip and support learners with AI tools from Microsoft."
Recently, many AI applications have been developed for the education sector. And so many processes have become simpler and faster. ... AI IN EDUCATION: Artificial intelligence provides a secure solution to ensure the integrity of online test system assessments in a cost-effective and scalable manner. The use of AI can reduce or even eliminate ...
In general, intelligence refers to the artificial fabrication of the human mind that can learn, plan, perceive, or understand natural language. Computer system theory and development are typically capable of executing human intelligence skills such as visual perception, voice recognition, choice, and language translation.
This work describes how Artificial Intelligence can be used and is being used in Educational sector. According to the 21 st International Conference on Artificial Intelligence in Education held in ...
Every sector is benefiting from artificial intelligence, so is education sector. Significant advancements have developed in the education field, and with the combination of artificial intelligence approaches in teaching styles, educational institutions have modified their methods of offering education from the past times.
Machine Learning (ML) and Artificial Intelligence (AI) are key drivers of growth and innovation across all industries, and the education sector is no different. According to eLearning Industry, upwards of 47% of learning management tools will be enabled with AI capabilities in the next three years.
According to Research and Markets, "The analysts forecast the Artificial Intelligence Market in the US Education Sector to grow at a CAGR of 47.77% during the period 2018-2022." AI tools mostly comply with 3 basic principles: Learning: Acquiring and processing the new experience, creating new behavior models
Abstract. Artificial intelligence (AI) is a field of study that combines the applications of machine learning, algorithm productions, and natural language processing. Applications of AI transform the tools of education. AI has a variety of educational applications, such as personalized learning platforms to promote students' learning ...
Calls on the Commission to assess the level of risk of AI deployment in the education sector in order to ascertain whether AI applications in education should be included in the regulatory framework for high risk and subject to stricter requirements on safety, ... on artificial intelligence in education, culture and the audiovisual sector
Ofqual's approach to regulating the use of artificial intelligence in the qualifications sector ... of State for Education and the Secretary of State for Science, Innovation and Technology ...
Applications and user experiences are poised to be reinvented with generative artificial intelligence (AI), and the public sector is no exception. Governments, education institutions, nonprofits, and health systems must constantly adapt and innovate to meet the changing needs of their constituents, students, beneficiaries, and patients. If used responsibly, this powerful technology can open ...
Microsoft also reported strong figures on Thursday, amid heavy investment in artificial intelligence, and investors pushed the company past the $3tn mark, a level it has already crossed this year.
The biggest driver of headlines across the technology sector over the past year or so has been the proliferation of artificial intelligence (AI). Many of the world's biggest companies see a vast ...