MYCIN: The Pioneering Expert System That Laid the Foundation for Medical AI
- by history tools
- November 12, 2023
In the early days of artificial intelligence research, scientists were determined to create computer systems that could replicate human expertise. One of the first successful "expert systems" was MYCIN, developed in the 1970s at Stanford University. Though never used in real-world medicine, MYCIN demonstrated the potential of AI in healthcare – paving the way for today‘s medical AI applications.
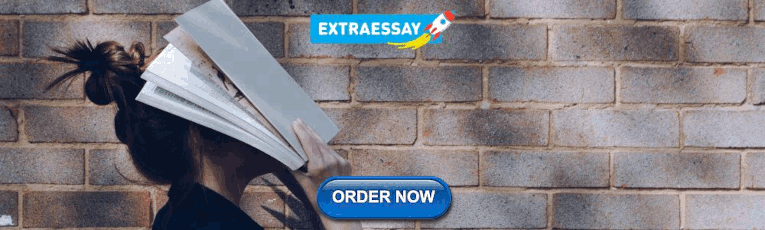
Seeking to Codify the Knowledge of Human Experts
In the 1970s, AI researchers became excited by the concept of expert systems – programs aimed at representing and automating the skills of human experts within specialized domains. A pioneering system was DENDRAL, created in the 1960s to analyze chemical compounds for novel discoveries. MYCIN built upon DENDRAL‘s techniques, aiming to capture the expertise of infectious disease specialists.
The goal was to encode the heuristic reasoning used by clinicians in an AI system. This included the ability to make diagnoses based on symptoms, handle uncertainty, and explain the logic behind conclusions. Researchers believed MYCIN could show AI‘s promise in augmenting medical experts.
Architecture: Rules and Backward Chaining Search
MYCIN‘s knowledge base consisted of around 600 rules acquired from extensive interviews with infectious disease doctors. These rules represented empirical associations and patterns that clinicians used instinctually in diagnosing difficult infections.
For example, rules connected patient symptoms and test results to the likelihood of certain bacterial organisms being responsible for an infection. Other rules linked bacteria types to optimal antibiotic therapies.
MYCIN‘s inference engine used a technique called backward chaining search. This involved starting with a hypothesis of infection, then working backwards to gather evidence to prove or disprove the hypothesis and determine the bacterial culprit.
The system would pose a series of yes/no questions to collect findings about a patient‘s infection. Each piece of evidence supported or detracted from potential bacteria according to the rules. MYCIN used a scoring system to handle the uncertainty involved.
Diagnosis and Treatment Recommendations
MYCIN focused on diagnosing serious infections like meningitis, bacteremia, and bacteria-caused clotting disorders. For a patient case, it would determine the likelihood of various bacterial organisms being responsible based on provided symptoms and test results.
The system could recommend appropriate antimicrobial drugs according to the diagnosed infection. It would also suggest the proper dosage customized to factors like patient age and kidney function.
Unlike a black-box AI, MYCIN could explain its reasoning at each step. This increased physician confidence in its diagnostic and therapeutic recommendations.
Pioneering Performance, But Reluctance to Adopt
In thorough evaluations, MYCIN demonstrated expertise and accuracy comparable to infectious disease specialists in diagnosing certain difficult infections like meningitis. It outperformed general practitioners in selecting proper antibiotics and dosages.
However, MYCIN never progressed beyond testing to real-world usage. Doctors were still reluctant to fully trust AI reasoning. Liability concerns also hampered adoption of the non-human expert system.
Influence: Enabling a New Generation of AI in Medicine
Though not adopted itself, MYCIN proved the potential for AI systems to replicate specialized medical knowledge. It showed AI could reason about uncertainty and explain conclusions – overcoming two major challenges.
MYCIN directly inspired many later medical AI systems, including:
- CADUCEUS – expert system for internal medicine
- QMR – diagnoses related to internal medicine
- Internist-I – large medical diagnosis system
The EMYCIN system provided a reusable framework based on MYCIN‘s architecture. This enabled easier development of diagnostic expert systems.
Despite limitations like brittleness and lack of common sense, MYCIN paved the way for the modern use of AI to enhance healthcare. Powerful medical AI today delivers improved diagnosis, treatment, and outcomes across specialties – with MYCIN laying the foundation decades ago.
Related posts:
- Abacus Explained — Everything You Need To Know
- The Complete History of the First ATM/Cash Machine
- Hello Friend! Here‘s Everything You Could Want to Know About the History and Impact of Compact Discs
- Pascaline Calculator Explained — Everything You Need To Know
- Cash Register: The Complete History
- Mechanical Calculators
- The Complete History of the Modem
- Sector Explained – A Revolution in Calculation
Join the conversation Cancel reply
Your email address will not be published. Required fields are marked *
Save my name, email, and website in this browser for the next time I comment.
Knowledge-Is-Power Hypothesis: Problem soving performance is a function of knowledge; the more you know, the better you perform.
IF the infection is pimary-bacteremia AND the site of the culture is one of the sterile sites AND the suspected portal of entry is the gastrointestinal tract THEN there is suggestive evidence (0.7) that infection is bacteroid.
- INDIAai Portal
Results for " "
INDIAai Recommends
Follow IndiaAI

Share on Twitter
Share on Facebook
Share on LinkedIn
AI Insights - Exploring MYCIN - an early backward chaining expert system
MYCIN was an early backward chaining expert system that used AI to identify microorganisms causing severe diseases like bacteremia and meningitis and propose antibiotics based on patient weight.

MYCIN was first developed at Stanford University in California in 1972. MYCIN would make an effort to make a diagnosis based on patient reports of symptoms and the outcomes of diagnostic procedures.
MYCIN worked with a knowledge base of about 600 rules and a simple inference engine. It would ask the doctor running the program a long list of easy yes/no or text-based questions. MYCIN ranked possible culprit bacteria from high to low based on likelihood, confidence, reasoning, and drug treatment.
Certainty factors
MYCIN started discussing how to use its "certainty factors," which are ad hoc but based on good ideas. The people who made MYCIN did studies that showed how little changes in the uncertainty metrics of individual rules affected its performance. It suggests that the system's power came from how it represented and exploited knowledge rather than its numerical uncertainty model. Some people thought that standard Bayesian statistics should have been able to be used. The people who made MYCIN said this would require either making unrealistic assumptions about independent probabilities or asking experts to give figures for many conditional probabilities.
Flexible design
Studies done in the future showed that the certainty factor model could be understood probabilistically and pointed out problems with such a model's claims. The system's flexible design would work very well and lead to the creation of graphical models like Bayesian networks.
MYCIN was never used in the real world. It wasn't because it needed to work better. Some observers raised moral and legal questions about using computers in medicine, like who would be responsible if the system made a wrong judgment. However, the state of system integration tools, especially when MYCIN was made, was the biggest problem and the main reason it was used sparingly. MYCIN was a stand-alone system that asked the user to type in answers to questions about a patient. Before personal computers were made, the program ran on an extensive time-shared system that could be reached through the early Internet (ARPANet).
In research done at Stanford Medical School, eight independent specialists gave the MYCIN treatment plan an acceptability rating of 65%, about the same as the 42.5% to 62.5% rate provided by five faculty members. People often point to this study to show that even experts can disagree about how to treat someone when there is no "gold standard" for the proper treatment.
The most crucial thing MYCIN did was show how powerful its method of representation and reasoning was. Since MYCIN introduced the technique, rule-based systems were built in many fields other than medicine. In the 1980s, "shells" for expert systems were created, such as E-MYCIN, which was based on MYCIN and was later replaced by Knowledge Engineering Environment (KEE). These "shells" helped build expert systems for various uses.
Furthermore, one problem during the development of MYCIN and other complex expert systems was getting human experts in the relevant areas to put their knowledge into the rule base so that the inference engine could use it.
Sources of Article
Image source: Unsplash
Want to publish your content?
Publish an article and share your insights to the world.
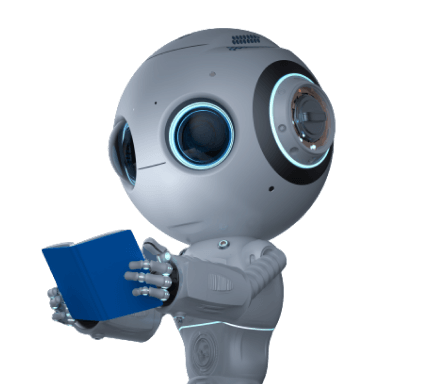
ALSO EXPLORE
The information provided on this page has been procured through secondary sources. In case you would like to suggest any update, please write to us at [email protected]

Exploring MYCIN - an early backward chaining expert system
indiaai.gov.in
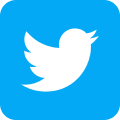
Suggestions or feedback?
MIT News | Massachusetts Institute of Technology
- Machine learning
- Social justice
- Black holes
- Classes and programs
Departments
- Aeronautics and Astronautics
- Brain and Cognitive Sciences
- Architecture
- Political Science
- Mechanical Engineering
Centers, Labs, & Programs
- Abdul Latif Jameel Poverty Action Lab (J-PAL)
- Picower Institute for Learning and Memory
- Lincoln Laboratory
- School of Architecture + Planning
- School of Engineering
- School of Humanities, Arts, and Social Sciences
- Sloan School of Management
- School of Science
- MIT Schwarzman College of Computing
When an antibiotic fails: MIT scientists are using AI to target “sleeper” bacteria
Press contact :.
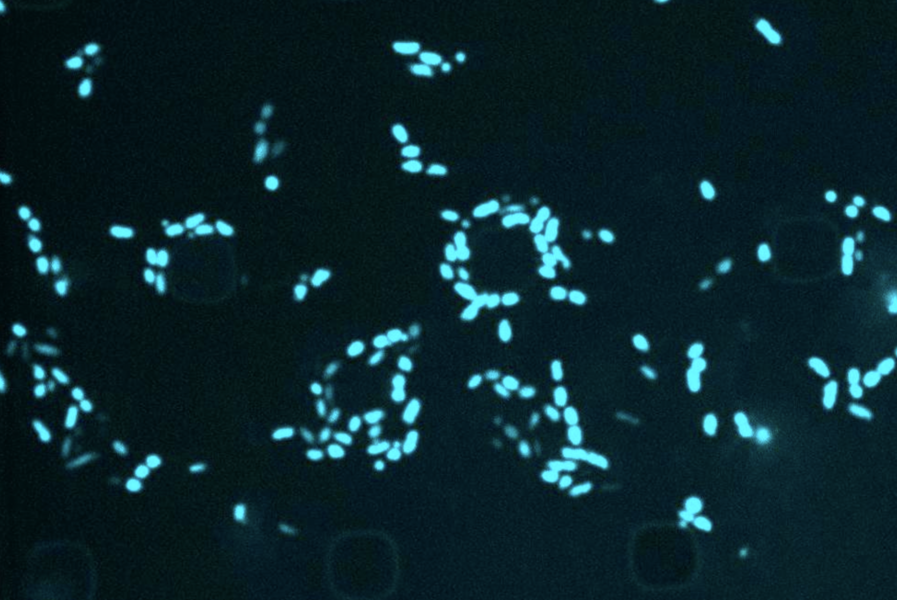
Previous image Next image
Since the 1970s, modern antibiotic discovery has been experiencing a lull. Now the World Health Organization has declared the antimicrobial resistance crisis as one of the top 10 global public health threats.
When an infection is treated repeatedly, clinicians run the risk of bacteria becoming resistant to the antibiotics. But why would an infection return after proper antibiotic treatment? One well-documented possibility is that the bacteria are becoming metabolically inert, escaping detection of traditional antibiotics that only respond to metabolic activity. When the danger has passed, the bacteria return to life and the infection reappears.
“Resistance is happening more over time, and recurring infections are due to this dormancy,” says Jackie Valeri, a former MIT-Takeda Fellow (centered within the MIT Abdul Latif Jameel Clinic for Machine Learning in Health) who recently earned her PhD in biological engineering from the Collins Lab. Valeri is the first author of a new paper published in this month’s print issue of Cell Chemical Biology that demonstrates how machine learning could help screen compounds that are lethal to dormant bacteria.
Tales of bacterial “sleeper-like” resilience are hardly news to the scientific community — ancient bacterial strains dating back to 100 million years ago have been discovered in recent years alive in an energy-saving state on the seafloor of the Pacific Ocean.
MIT Jameel Clinic's Life Sciences faculty lead James J. Collins, a Termeer Professor of Medical Engineering and Science in MIT’s Institute for Medical Engineering and Science and Department of Biological Engineering, recently made headlines for using AI to discover a new class of antibiotics, which is part of the group’s larger mission to use AI to dramatically expand the existing antibiotics available.
According to a paper published by The Lancet , in 2019, 1.27 million deaths could have been prevented had the infections been susceptible to drugs, and one of many challenges researchers are up against is finding antibiotics that are able to target metabolically dormant bacteria.
In this case, researchers in the Collins Lab employed AI to speed up the process of finding antibiotic properties in known drug compounds. With millions of molecules, the process can take years, but researchers were able to identify a compound called semapimod over a weekend, thanks to AI's ability to perform high-throughput screening.
An anti-inflammatory drug typically used for Crohn’s disease, researchers discovered that semapimod was also effective against stationary-phase Escherichia coli and Acinetobacter baumannii .
Another revelation was semapimod's ability to disrupt the membranes of so-called “Gram-negative” bacteria, which are known for their high intrinsic resistance to antibiotics due to their thicker, less-penetrable outer membrane.
Examples of Gram-negative bacteria include E. coli , A. baumannii , Salmonella , and Pseudomonis , all of which are challenging to find new antibiotics for.
“One of the ways we figured out the mechanism of sema [sic] was that its structure was really big, and it reminded us of other things that target the outer membrane,” Valeri explains. “When you start working with a lot of small molecules ... to our eyes, it’s a pretty unique structure.”
By disrupting a component of the outer membrane, semapimod sensitizes Gram-negative bacteria to drugs that are typically only active against Gram-positive bacteria.
Valeri recalls a quote from a 2013 paper published in Trends Biotechnology : “For Gram-positive infections, we need better drugs, but for Gram-negative infections we need any drugs.”
Share this news article on:
Related links.
- Collins Lab
- Abdul Latif Jameel Clinic for Machine Learning in Health
- Institute for Medical Engineering and Science
- Department of Biological Engineering
Related Topics
- Biological engineering
- Institute for Medical Engineering and Science (IMES)
- Jameel Clinic
- Artificial intelligence
- Health sciences and technology
- Drug discovery
- Antibiotics
Related Articles
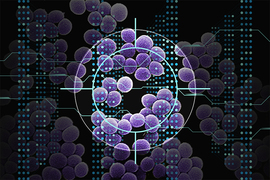
Using AI, MIT researchers identify a new class of antibiotic candidates
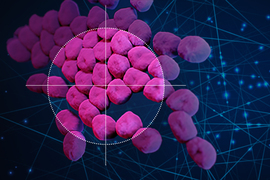
Using AI, scientists find a drug that could combat drug-resistant infections
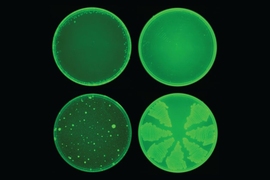
Artificial intelligence yields new antibiotic
Previous item Next item
More MIT News
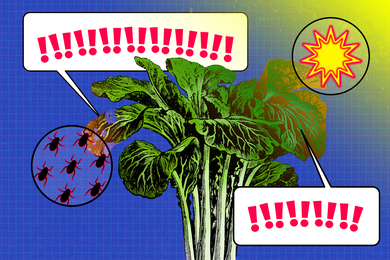
Plant sensors could act as an early warning system for farmers
Read full story →
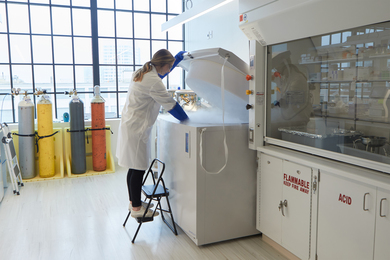
A home where world-changing innovations take flight
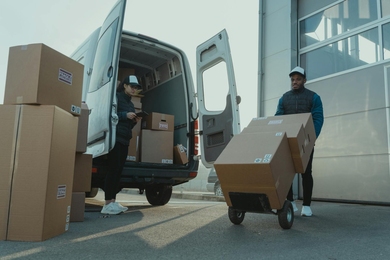
3 Questions: Enhancing last-mile logistics with machine learning
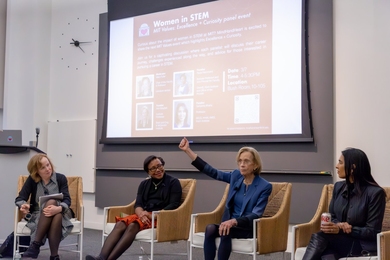
Women in STEM — A celebration of excellence and curiosity
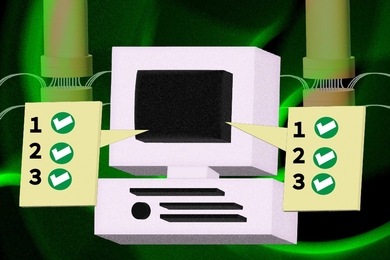
A blueprint for making quantum computers easier to program
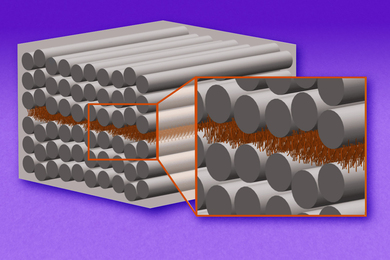
“Nanostitches” enable lighter and tougher composite materials
- More news on MIT News homepage →
Massachusetts Institute of Technology 77 Massachusetts Avenue, Cambridge, MA, USA
- Map (opens in new window)
- Events (opens in new window)
- People (opens in new window)
- Careers (opens in new window)
- Accessibility
- Social Media Hub
- MIT on Facebook
- MIT on YouTube
- MIT on Instagram
- See us on facebook
- See us on twitter
- See us on youtube
- See us on linkedin
- See us on instagram
AI improves accuracy of skin cancer diagnoses in Stanford Medicine-led study
Artificial intelligence algorithms powered by deep learning improve skin cancer diagnostic accuracy for doctors, nurse practitioners and medical students in a study led by the Stanford Center for Digital Health.
April 11, 2024 - By Krista Conger
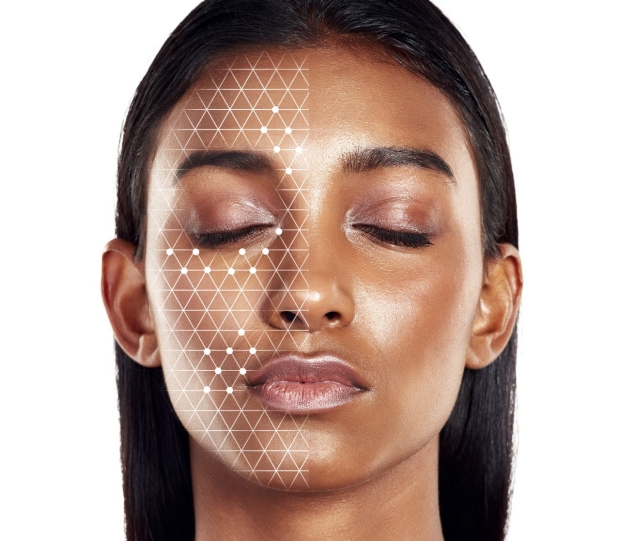
Artificial intelligence helped clinicians diagnose skin cancer more accurately, a Stanford Medicine-led study found. Chanelle Malambo/peopleimages.com - stock.adobe.com
A new study led by researchers at Stanford Medicine finds that computer algorithms powered by artificial intelligence based on deep learning can help health care practitioners to diagnose skin cancers more accurately. Even dermatologists benefit from AI guidance, although their improvement is less than that seen for non-dermatologists.
“This is a clear demonstration of how AI can be used in collaboration with a physician to improve patient care,” said professor of dermatology and of epidemiology Eleni Linos , MD. Linos leads the Stanford Center for Digital Health , which was launched to tackle some of the most pressing research questions at the intersection of technology and health by promoting collaboration between engineering, computer science, medicine and the humanities.
Linos, associate dean of research and the Ben Davenport and Lucy Zhang Professor in Medicine, is the senior author of the study , which was published on April 9 in npj Digital Medicine . Postdoctoral scholar Jiyeong Kim , PhD, and visiting researcher Isabelle Krakowski, MD, are the lead authors of the research.
“Previous studies have focused on how AI performs when compared with physicians,” Kim said. “Our study compared physicians working without AI assistance with physicians using AI when diagnosing skin cancers.”
AI algorithms are increasingly used in clinical settings, including dermatology. They are created by feeding a computer hundreds of thousands or even millions of images of skin conditions labeled with information such as diagnosis and patient outcome. Through a process called deep learning, the computer eventually learns to recognize telltale patterns in the images that correlate with specific skin diseases including cancers. Once trained, an algorithm written by the computer can be used to suggest possible diagnoses based on an image of a patient’s skin that it has not been exposed to.
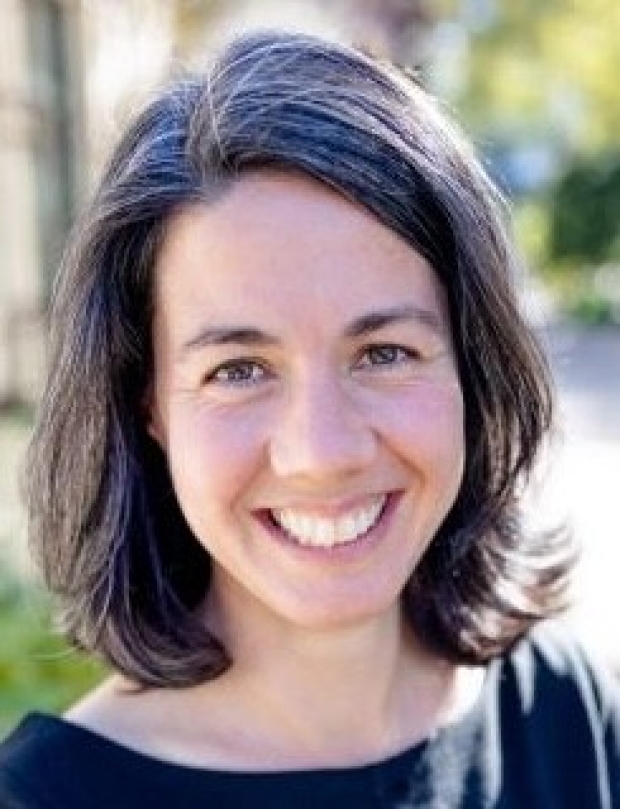
Eleni Linos
These diagnostic algorithms aren’t used alone, however. They are overseen by clinicians who also assess the patient, come to their own conclusions about a patient’s diagnosis and choose whether to accept the algorithm’s suggestion.
An accuracy boost
Kim and Linos’ team reviewed 12 studies detailing more than 67,000 evaluations of potential skin cancers by a variety of practitioners with and without AI assistance. They found that, overall, health care practitioners working without aid from artificial intelligence were able to accurately diagnose about 75% of people with skin cancer — a statistical measurement known as sensitivity. Conversely, the workers correctly diagnosed about 81.5% of people with cancer-like skin conditions but who did not have cancer — a companion measurement known as specificity.
Health care practitiones who used AI to guide their diagnoses did better. Their diagnoses were about 81.1% sensitive and 86.1% specific. The improvement may seem small, but the differences are critical for people told they don’t have cancer, but do, or for those who do have cancer but are told they are healthy.
When the researchers split the health care practitioners by specialty or level of training, they saw that medical students, nurse practitioners and primary care doctors benefited the most from AI guidance — improving on average about 13 points in sensitivity and 11 points in specificity. Dermatologists and dermatology residents performed better overall, but the sensitivity and specificity of their diagnoses also improved with AI.
“I was surprised to see everyone’s accuracy improve with AI assistance, regardless of their level of training,” Linos said. “This makes me very optimistic about the use of AI in clinical care. Soon our patients will not just be accepting, but expecting, that we use AI assistance to provide them with the best possible care.”
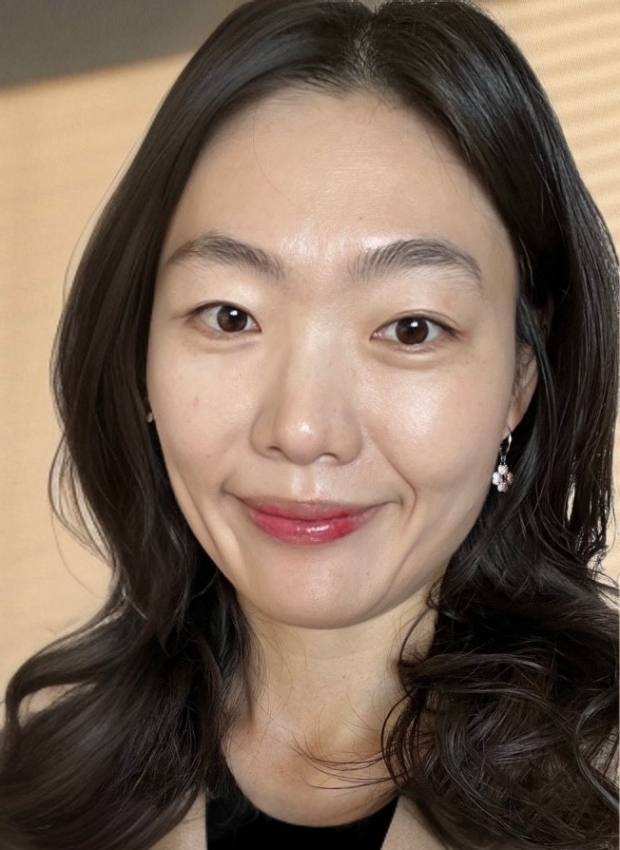
Jiyeong Kim
Researchers at the Stanford Center for Digital Health, including Kim, are interested in learning more about the promise of and barriers to integrating AI-based tools into health care. In particular, they are planning to investigate how the perceptions and attitudes of physicians and patients to AI will influence its implementation.
“We want to better understand how humans interact with and use AI to make clinical decisions,” Kim said.
Previous studies have indicated that a clinician’s degree of confidence in their own clinical decision, the degree of confidence of the AI, and whether the clinician and the AI agree on the diagnosis all influence whether the clinician incorporates the algorithm’s advice when making clinical decisions for a patient.
Medical specialties like dermatology and radiology, which rely heavily on images — visual inspection, pictures, X-rays, MRIs and CT scans, among others — for diagnoses are low-hanging fruit for computers that can pick out levels of detail beyond what a human eye (or brain) can reasonably process. But even other more symptom-based specialties, or prediction modeling, are likely to benefit from AI intervention, Linos and Kim feel. And it’s not just patients who stand to benefit.
“If this technology can simultaneously improve a doctor’s diagnostic accuracy and save them time, it’s really a win-win. In addition to helping patients, it could help reduce physician burnout and improve the human interpersonal relationships between doctors and their patients,” Linos said. “I have no doubt that AI assistance will eventually be used in all medical specialties. The key question is how we make sure it is used in a way that helps all patients regardless of their background and simultaneously supports physician well-being.”
Researchers from the Karolinska Institute, the Karolinska University Hospital and the University of Nicosia contributed to the research.
The study was funded by the National Institutes of Health (grants K24AR075060 and R01AR082109), Radiumhemmet Research, the Swedish Cancer Society and the Swedish Research Council.
For more news about responsible AI in health and medicine, sign up for the RAISE Health newsletter.
Register for the RAISE Health Symposium on May 14.
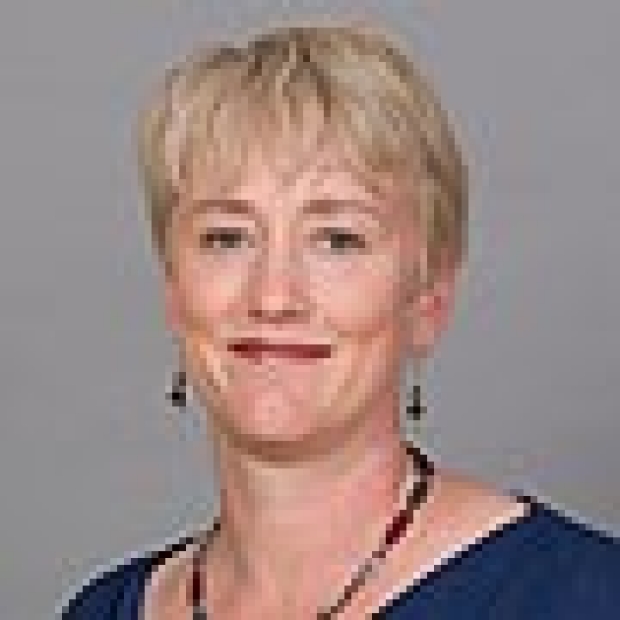
About Stanford Medicine
Stanford Medicine is an integrated academic health system comprising the Stanford School of Medicine and adult and pediatric health care delivery systems. Together, they harness the full potential of biomedicine through collaborative research, education and clinical care for patients. For more information, please visit med.stanford.edu .
Artificial intelligence
Exploring ways AI is applied to health care
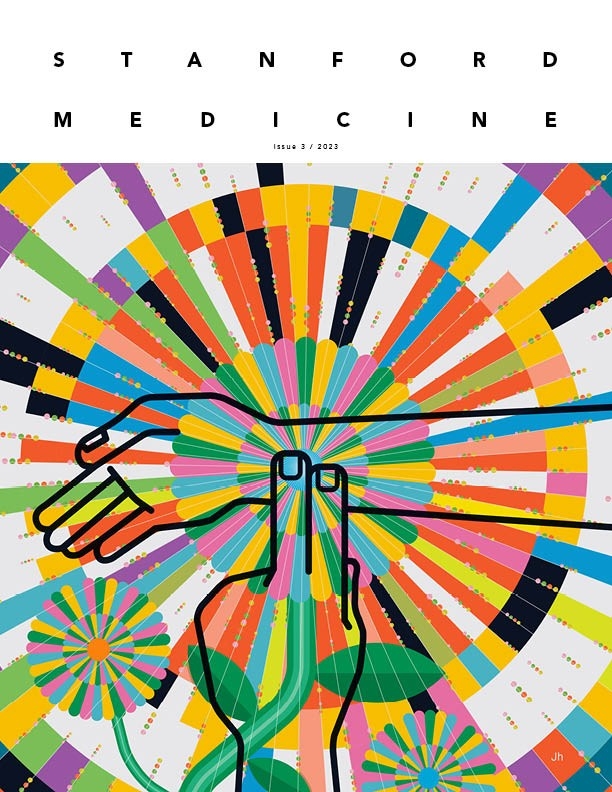
- Digital Marketing
- Facebook Marketing
- Instagram Marketing
- Ecommerce Marketing
- Content Marketing
- Data Science Certification
- Machine Learning
- Artificial Intelligence
- Data Analytics
- Graphic Design
- Adobe Illustrator
- Web Designing
- UX UI Design
- Interior Design
- Front End Development
- Back End Development Courses
- Business Analytics
- Entrepreneurship
- Supply Chain
- Financial Modeling
- Corporate Finance
- Project Finance
- Harvard University
- Stanford University
- Yale University
- Princeton University
- Duke University
- UC Berkeley
- Harvard University Executive Programs
- MIT Executive Programs
- Stanford University Executive Programs
- Oxford University Executive Programs
- Cambridge University Executive Programs
- Yale University Executive Programs
- Kellog Executive Programs
- CMU Executive Programs
- 45000+ Free Courses
- Free Certification Courses
- Free DigitalDefynd Certificate
- Free Harvard University Courses
- Free MIT Courses
- Free Excel Courses
- Free Google Courses
- Free Finance Courses
- Free Coding Courses
- Free Digital Marketing Courses
20 Detailed Artificial Intelligence Case Studies [2024]
In this dynamic era of technological advancements, Artificial Intelligence (AI) emerges as a pivotal force, reshaping the way industries operate and charting new courses for business innovation. This article presents an in-depth exploration of 20 diverse and compelling AI case studies from across the globe. Each case study offers a deep dive into the challenges faced by companies, the AI-driven solutions implemented, their substantial impacts, and the valuable lessons learned. From healthcare and finance to transportation and retail, these stories highlight AI’s transformative power in solving complex problems, optimizing processes, and driving growth, offering insightful glimpses into the potential and versatility of AI in shaping our world.
Related: How to Become an AI Thought Leader?
1. IBM Watson Health: Revolutionizing Patient Care with AI
Task/Conflict: The healthcare industry faces challenges in handling vast amounts of patient data, accurately diagnosing diseases, and creating effective treatment plans. IBM Watson Health aimed to address these issues by harnessing AI to process and analyze complex medical information, thus improving the accuracy and efficiency of patient care.
Solution: Utilizing the cognitive computing capabilities of IBM Watson, this solution involves analyzing large volumes of medical records, research papers, and clinical trial data. The system uses natural language processing to understand and process medical jargon, making sense of unstructured data to aid medical professionals in diagnosing and treating patients.
Overall Impact:
- Enhanced accuracy in patient diagnosis and treatment recommendations.
- Significant improvement in personalized healthcare services.
Key Learnings:
- AI can complement medical professionals’ expertise, leading to better healthcare outcomes.
- The integration of AI in healthcare can lead to significant advancements in personalized medicine.
2. Google DeepMind’s AlphaFold: Unraveling the Mysteries of Protein Folding
Task/Conflict: The scientific community has long grappled with the protein folding problem – understanding how a protein’s amino acid sequence determines its 3D structure. Solving this problem is crucial for drug discovery and understanding diseases at a molecular level, yet it remained a formidable challenge due to the complexity of biological structures.
Solution: AlphaFold, developed by Google DeepMind, is an AI model trained on vast datasets of known protein structures. It assesses the distances and angles between amino acids to predict how a protein folds, outperforming existing methods in terms of speed and accuracy. This breakthrough represents a major advancement in computational biology.
- Significant acceleration in drug discovery and disease understanding.
- Set a new benchmark for computational methods in biology.
- AI’s predictive power can solve complex biological problems.
- The application of AI in scientific research can lead to groundbreaking discoveries.
3. Amazon: Transforming Supply Chain Management through AI
Task/Conflict: Managing a global supply chain involves complex challenges like predicting product demand, optimizing inventory levels, and streamlining logistics. Amazon faced the task of efficiently managing its massive inventory while minimizing costs and meeting customer demands promptly.
Solution: Amazon employs sophisticated AI algorithms for predictive inventory management, which forecast product demand based on various factors like buying trends, seasonality, and market changes. This system allows for real-time adjustments, adapting swiftly to changing market dynamics.
- Reduced operational costs through efficient inventory management.
- Improved customer satisfaction with timely deliveries and availability.
- AI can significantly enhance supply chain efficiency and responsiveness.
- Predictive analytics in inventory management leads to reduced waste and cost savings.
4. Tesla’s Autonomous Vehicles: Driving the Future of Transportation
Task/Conflict: The development of autonomous vehicles represents a major technological and safety challenge. Tesla aimed to create self-driving cars that are not only reliable and safe but also capable of navigating complex traffic conditions without human intervention.
Solution: Tesla’s solution involves advanced AI and machine learning algorithms that process data from various sensors and cameras to understand and navigate the driving environment. Continuous learning from real-world driving data allows the system to improve over time, making autonomous driving safer and more efficient.
- Leadership in the autonomous vehicle sector, enhancing road safety.
- Continuous improvements in self-driving technology through AI-driven data analysis.
- Continuous data analysis is key to advancing autonomous driving technologies.
- AI can significantly improve road safety and driving efficiency.
Related: High-Paying AI Career Options
5. Zara: Fashioning the Future with AI in Retail
Task/Conflict: In the fast-paced fashion industry, predicting trends and managing inventory efficiently are critical for success. Zara faced the challenge of quickly adapting to changing fashion trends while avoiding overstock and meeting consumer demand.
Solution: Zara employs AI algorithms to analyze fashion trends, customer preferences, and sales data. The AI system also assists in managing inventory, ensuring that popular items are restocked promptly and that stores are not overburdened with unsold products. This approach optimizes both production and distribution.
- Increased sales and profitability through optimized inventory.
- Enhanced customer satisfaction by aligning products with current trends.
- AI can accurately predict consumer behavior and trends.
- Effective inventory management through AI can significantly impact business success.
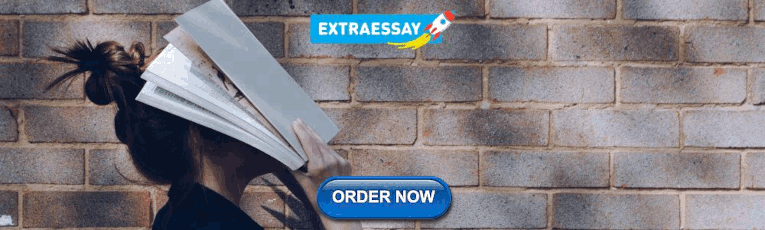
6. Netflix: Personalizing Entertainment with AI
Task/Conflict: In the competitive streaming industry, providing a personalized user experience is key to retaining subscribers. Netflix needed to recommend relevant content to each user from its vast library, ensuring that users remained engaged and satisfied.
Solution: Netflix developed an advanced AI-driven recommendation engine that analyzes individual viewing habits, ratings, and preferences. This personalized approach keeps users engaged, as they are more likely to find content that interests them, enhancing their overall viewing experience.
- Increased viewer engagement and longer watch times.
- Higher subscription retention rates due to personalized content.
- Personalized recommendations significantly enhance user experience.
- AI-driven content curation is essential for success in digital entertainment.
7. Airbus: Elevating Aircraft Maintenance with AI
Task/Conflict: Aircraft maintenance is crucial for ensuring flight safety and operational efficiency. Airbus faced the challenge of predicting maintenance needs to prevent equipment failures and reduce downtime, which is critical in the aviation industry.
Solution: Airbus implemented AI algorithms for predictive maintenance, analyzing data from aircraft sensors to identify potential issues before they lead to failures. This system assesses the condition of various components, predicting when maintenance is needed. The solution not only enhances safety but also optimizes maintenance schedules, reducing unnecessary inspections and downtime.
- Decreased maintenance costs and reduced aircraft downtime.
- Improved safety with proactive maintenance measures.
- AI can predict and prevent potential equipment failures.
- Predictive maintenance is essential for operational efficiency and safety in aviation.
8. American Express: Securing Transactions with AI
Task/Conflict: Credit card fraud is a significant issue in the financial sector, leading to substantial losses and undermining customer trust. American Express needed an efficient way to detect and prevent fraudulent transactions in real-time.
Solution: American Express utilizes machine learning models to analyze transaction data. These models identify unusual patterns and behaviors indicative of fraud. By constant learning from refined data, the system becomes increasingly accurate in detecting fraudulent activities, providing real-time alerts and preventing unauthorized transactions.
- Minimized financial losses due to reduced fraudulent activities.
- Enhanced customer trust and security in financial transactions.
- Machine learning is highly effective in fraud detection.
- Real-time data analysis is crucial for preventing financial fraud.
Related: Is AI a Good Career Option for Women?
9. Stitch Fix: Tailoring the Future of Fashion Retail
Task/Conflict: In the competitive fashion retail industry, providing a personalized shopping experience is key to customer satisfaction and business growth. Stitch Fix aimed to offer customized clothing selections to each customer, based on their unique preferences and style.
Solution: Stitch Fix uses AI and algorithms analyze customer feedback, style preferences, and purchase history to recommend clothing items. This personalized approach is complemented by human stylists, ensuring that each customer receives a tailored selection that aligns with their individual style.
- Increased customer satisfaction through personalized styling services.
- Business growth driven by a unique, AI-enhanced shopping experience.
- AI combined with human judgment can create highly effective personalization.
- Tailoring customer experiences using AI leads to increased loyalty and business success.
10. Baidu: Breaking Language Barriers with Voice Recognition
Task/Conflict: Voice recognition technology faces the challenge of accurately understanding and processing speech in various languages and accents. Baidu aimed to enhance its voice recognition capabilities to provide more accurate and user-friendly interactions in multiple languages.
Solution: Baidu employs deep learning algorithms for voice and speech recognition, training its system on a diverse range of languages and dialects. This approach allows for more accurate recognition of speech patterns, enabling the technology to understand and respond to voice commands more effectively. The system continuously improves as it processes more voice data, making technology more accessible to users worldwide.
- Enhanced user interaction with technology in multiple languages.
- Reduced language barriers in voice-activated services and devices.
- AI can effectively bridge language gaps in technology.
- Continuous learning from diverse data sets is key to improving voice recognition.
11. JP Morgan: Revolutionizing Legal Document Analysis with AI
Task/Conflict: Analyzing legal documents, such as contracts, is a time-consuming and error-prone process. JP Morgan sought to streamline this process, reducing the time and effort required while increasing accuracy.
Solution: JP Morgan implemented an AI-powered tool, COIN (Contract Intelligence), to analyze legal documents quickly and accurately. COIN uses NLP to interpret and extract relevant information from contracts, significantly reducing the time required for document review.
- Dramatic reduction in time required for legal document analysis.
- Increased accuracy and reduced human error in contract interpretation.
- AI can efficiently handle large volumes of data, offering speed and accuracy.
- Automation in legal processes can significantly enhance operational efficiency.
12. Microsoft: AI for Accessibility
Task/Conflict: People with disabilities often face challenges in accessing technology. Microsoft aimed to create AI-driven tools to enhance accessibility, especially for individuals with visual, hearing, or cognitive impairments.
Solution: Microsoft developed a range of AI-powered tools including applications for voice recognition, visual assistance, and cognitive support, making technology more accessible and user-friendly. For instance, Seeing AI, an app developed by Microsoft, helps visually impaired users to understand their surroundings by describing people, texts, and objects.
- Improved accessibility and independence for people with disabilities.
- Creation of more inclusive technology solutions.
- AI can significantly contribute to making technology accessible for all.
- Developing inclusive technology is essential for societal progress.
Related: How to get an Internship in AI?
13. Alibaba’s City Brain: Revolutionizing Urban Traffic Management
Task/Conflict: Urban traffic congestion is a major challenge in many cities, leading to inefficiencies and environmental concerns. Alibaba’s City Brain project aimed to address this issue by using AI to optimize traffic flow and improve public transportation in urban areas.
Solution: City Brain uses AI to analyze real-time data from traffic cameras, sensors, and GPS systems. It processes this information to predict traffic patterns and optimize traffic light timing, reducing congestion. The system also provides data-driven insights for urban planning and emergency response coordination, enhancing overall city management.
- Significant reduction in traffic congestion and improved urban transportation.
- Enhanced efficiency in city management and emergency response.
- AI can effectively manage complex urban systems.
- Data-driven solutions are key to improving urban living conditions.
14. Deep 6 AI: Accelerating Clinical Trials with Artificial Intelligence
Task/Conflict: Recruiting suitable patients for clinical trials is often a slow and cumbersome process, hindering medical research. Deep 6 AI sought to accelerate this process by quickly identifying eligible participants from a vast pool of patient data.
Solution: Deep 6 AI employs AI to sift through extensive medical records, identifying potential trial participants based on specific criteria. The system analyzes structured and unstructured data, including doctor’s notes and diagnostic reports, to find matches for clinical trials. This approach significantly speeds up the recruitment process, enabling faster trial completions and advancements in medical research.
- Quicker recruitment for clinical trials, leading to faster research progress.
- Enhanced efficiency in medical research and development.
- AI can streamline the patient selection process for clinical trials.
- Efficient recruitment is crucial for the advancement of medical research.
15. NVIDIA: Revolutionizing Gaming Graphics with AI
Task/Conflict: Enhancing the realism and performance of gaming graphics is a continuous challenge in the gaming industry. NVIDIA aimed to revolutionize gaming visuals by leveraging AI to create more realistic and immersive gaming experiences.
Solution: NVIDIA’s AI-driven graphic processing technologies, such as ray tracing and deep learning super sampling (DLSS), provide highly realistic and detailed graphics. These technologies use AI to render images more efficiently, improving game performance without compromising on visual quality. This innovation sets new standards in gaming graphics, making games more lifelike and engaging.
- Elevated gaming experiences with state-of-the-art graphics.
- Set new industry standards for graphic realism and performance.
- AI can significantly enhance creative industries, like gaming.
- Balancing performance and visual quality is key to gaming innovation.
16. Palantir: Mastering Data Integration and Analysis with AI
Task/Conflict: Integrating and analyzing large-scale, diverse datasets is a complex task, essential for informed decision-making in various sectors. Palantir Technologies faced the challenge of making sense of vast amounts of data to provide actionable insights for businesses and governments.
Solution: Palantir developed AI-powered platforms that integrate data from multiple sources, providing a comprehensive view of complex systems. These platforms use machine learning to analyze data, uncover patterns, and predict outcomes, assisting in strategic decision-making. This solution enables users to make informed decisions in real-time, based on a holistic understanding of their data.
- Enhanced decision-making capabilities in complex environments.
- Greater insights and efficiency in data analysis across sectors.
- Effective data integration is crucial for comprehensive analysis.
- AI-driven insights are essential for strategic decision-making.
Related: Surprising AI Facts & Statistics
17. Blue River Technology: Sowing the Seeds of AI in Agriculture
Task/Conflict: The agriculture industry faces challenges in increasing efficiency and sustainability while minimizing environmental impact. Blue River Technology aimed to enhance agricultural practices by using AI to make farming more precise and efficient.
Solution: Blue River Technology developed AI-driven agricultural robots that perform tasks like precise planting and weed control. These robots use ML to identify plants and make real-time decisions, such as applying herbicides only to weeds. This targeted approach reduces chemical usage and promotes sustainable farming practices, leading to better crop yields and environmental conservation.
- Significant reduction in chemical usage in farming.
- Increased crop yields through precision agriculture.
- AI can contribute significantly to sustainable agricultural practices.
- Precision farming is key to balancing productivity and environmental conservation.
18. Salesforce: Enhancing Customer Relationship Management with AI
Task/Conflict: In the realm of customer relationship management (CRM), personalizing interactions and gaining insights into customer behavior are crucial for business success. Salesforce aimed to enhance CRM capabilities by integrating AI to provide personalized customer experiences and actionable insights.
Solution: Salesforce incorporates AI-powered tools into its CRM platform, enabling businesses to personalize customer interactions, automate responses, and predict customer needs. These tools analyze customer data, providing insights that help businesses tailor their strategies and communications. The AI integration not only improves customer engagement but also streamlines sales and marketing efforts.
- Improved customer engagement and satisfaction.
- Increased business growth through tailored marketing and sales strategies.
- AI-driven personalization is key to successful customer relationship management.
- Leveraging AI for data insights can significantly impact business growth.
19. OpenAI: Transforming Natural Language Processing
Task/Conflict: OpenAI aimed to advance NLP by developing models capable of generating coherent and contextually relevant text, opening new possibilities in AI-human interaction.
Solution: OpenAI developed the Generative Pre-trained Transformer (GPT) models, which use deep learning to generate text that closely mimics human language. These models are trained on vast datasets, enabling them to understand context and generate responses in a conversational and coherent manner.
- Pioneered advancements in natural language understanding and generation.
- Expanded the possibilities for AI applications in communication.
- AI’s ability to mimic human language has vast potential applications.
- Advancements in NLP are crucial for improving AI-human interactions.
20. Siemens: Pioneering Industrial Automation with AI
Task/Conflict: Industrial automation seeks to improve productivity and efficiency in manufacturing processes. Siemens faced the challenge of optimizing these processes using AI to reduce downtime and enhance output quality.
Solution: Siemens employs AI-driven solutions for predictive maintenance and process optimization to reduce downtime in industrial settings. Additionally, AI optimizes manufacturing processes, ensuring quality and efficiency.
- Increased productivity and reduced downtime in industrial operations.
- Enhanced quality and efficiency in manufacturing processes.
- AI is a key driver in the advancement of industrial automation.
- Predictive analytics are crucial for maintaining efficiency in manufacturing.
Related: Top Books for Learning AI
Closing Thoughts
These 20 case studies illustrate the transformative power of AI across various industries. By addressing specific challenges and leveraging AI solutions, companies have achieved remarkable outcomes, from enhancing customer experiences to solving complex scientific problems. The key learnings from these cases underscore AI’s potential to revolutionize industries, improve efficiencies, and open up new possibilities for innovation and growth.
- 6 Ways to Pay for an Online Course [2024]
- How to Choose an Online Course? [An Ultimate Checklist] [2024]
Team DigitalDefynd
We help you find the best courses, certifications, and tutorials online. Hundreds of experts come together to handpick these recommendations based on decades of collective experience. So far we have served 4 Million+ satisfied learners and counting.
Impact of Artificial Intelligence on Supply Chain Management [2024]

How to negotiate a high AI Engineer salary? [2024]

Impact of AI in Pharmaceutical Industry [2024]

Can AI replace CMOs? How to save yourself? [2024]

10 Ways AI Is Helping Out In Farming [2024]

How to Become an AI Thought Leader [2024]
- Open access
- Published: 08 April 2024
AI-based support for optical coherence tomography in age-related macular degeneration
- Virginia Mares 1 , 2 ,
- Marcio B. Nehemy 2 ,
- Hrvoje Bogunovic 1 ,
- Sophie Frank 1 ,
- Gregor S. Reiter 1 &
- Ursula Schmidt-Erfurth 1
International Journal of Retina and Vitreous volume 10 , Article number: 31 ( 2024 ) Cite this article
250 Accesses
Metrics details
Artificial intelligence (AI) has emerged as a transformative technology across various fields, and its applications in the medical domain, particularly in ophthalmology, has gained significant attention. The vast amount of high-resolution image data, such as optical coherence tomography (OCT) images, has been a driving force behind AI growth in this field. Age-related macular degeneration (AMD) is one of the leading causes for blindness in the world, affecting approximately 196 million people worldwide in 2020. Multimodal imaging has been for a long time the gold standard for diagnosing patients with AMD, however, currently treatment and follow-up in routine disease management are mainly driven by OCT imaging. AI-based algorithms have by their precision, reproducibility and speed, the potential to reliably quantify biomarkers, predict disease progression and assist treatment decisions in clinical routine as well as academic studies. This review paper aims to provide a summary of the current state of AI in AMD, focusing on its applications, challenges, and prospects.
Introduction
Artificial intelligence (AI) spans a wide range of techniques within computer science, executing tasks that were traditionally performed by humans [ 1 ]. Machine learning (ML) is a branch of AI firstly described by Arthur Samuel in 1959 as the combination of computational science and mathematical concepts used to perform specific tasks without being explicitly programmed [ 2 ]. Deep learning (DL) is a class of machine learning techniques dedicated towards developing artificial neural networks with multiple levels of abstraction in which task-specific features are not prespecified by human engineers, but they are learned directly from data using a general-purpose learning procedure [ 3 ]. The integration of AI into the field of medicine is not a recent phenomenon. The MYCIN system was developed at Stanford University in the 1970s to assist in diagnosing and treating bacterial infections [ 4 ]. From there, the amount of automated algorithms multiplied and more advanced AI-based systems were developed including risk prediction scales that are currently used in clinical practice [ 5 , 6 , 7 ]. AI has emerged as a transformative technology across various fields, and its applications in ophthalmology have gained significant attention due to the availability of large digital datasets from retinal imaging. The exponential growth of interest from the scientific community can be easily identified when analysing the raising numbers of publications in recent years. Using “artificial intelligence” and “ophthalmology” as keywords in PubMed there are 3187 publications from 1900 to 2023, 2820 (88%) of them were published in the last 5 years.
Due to the large potential of multi-modal imaging utilized for diagnostics and monitoring of vision-threatening conditions in clinical routine, the retina emerged as the most promising field for application of AI. Age-related macular degeneration (AMD) is one of the leading causes of severe visual loss worldwide and is described as a multifactorial interaction of metabolic, functional, genetic, and environmental factors [ 8 , 9 ]. Late-stage AMD is characterized by vision-impairing lesions in the macula such as geographic atrophy (GA) in non-exudative AMD or macular neovascularization (MNV) in neovascular AMD (nAMD) [ 10 ]. Over the past 20 years, there were significant advances in diagnostic tools, ultimately spectral-domain (SD) optical coherence tomography (OCT) has become the gold standard imaging modality in AMD [ 11 , 12 ]. 10–15% of all AMD cases advance to nAMD and often suffer from fast progressing devastating visual impairment. Thus, the introduction of intravitreal vascular endothelial growth factor (VEGF) inhibition in 2006 for treating nAMD was a landmark event in disease management [ 11 , 12 ]. However, the half-lives of these biological drugs are short and in the context of chronic treatment over lifetime, may represent an important burden to the patient and to the health care system [ 13 , 14 ]. Clinical trials such as PULSAR, TENAYA and LUCERNE analyzed treatment efficacy of more extended regimens in order to address this problem [ 15 , 16 ]. Furthermore, the first intravitreal complement system inhibitors were approved by the US Food and Drug Administration (FDA) in 2023 to treat patients with GA, [ 17 ] representing an additional burden on already stressed health care systems.
Fortunately, efficient AI algorithms relying on high resolution imaging have the potential to reduce time effort and improve quality standards in evaluating disease activity in clinical practice. OCT images are characterized by their high-resolution depiction of retinal structures, containing many millions of pixels in each volume, providing the most critical parameters for guiding treatment decisions in AMD [ 18 ]. This review paper aims to provide an overview of the current state of AI in OCT in AMD focusing on its applications, challenges, and prospects.
AI in retinal imaging
Before the advent of AI-based tools, management of retinal pathologies relied mostly on dichotomous parameters, meaning subjective assessment of presence or absence of specific biomarkers. A binary approach often underestimates biomarker dynamics and the intricate nature of all retinal conditions, including AMD. The multi-modal approaches lead to an enormous amount of information for each patient at each visit. However, traditional methods struggle to capture the subtle variations in pathology, such as atrophy progression, fluid volume and fluctuation. Moreover, novel biomarkers of relevance are subclinical in nature such as photoreceptor layer loss consistent with the ellipsoid layer attenuation. Hence, advanced AI detection tools are needed to reliably inform the clinician about the state of the disease in GA. Nevertheless, the ageing population causes a continuous growth in the prevalence of AMD worldwide, demanding novel strategies capable of screening as well as precisely following disease progression in a faster and reliable manner. AI enables a prompt analysis by quantifying various parameters, that are usually challenging to assess comprehensively by humans in a busy clinical practice [ 19 , 20 ].
Early detection is paramount in managing retinal diseases as it allows for timely intervention in case of conversion to sight-threatening stages of the disease. For example, the presence of large confluent drusen, subretinal drusenoid deposits, refractile deposits, large and central pigment epithelial detachment (PED) and vitelliform material on intermediate AMD were described as phenotype precursors for GA development [ 21 , 22 ]. Multimodal imaging is a praised gold standard to identify retinal biomarkers. Historically, color fundus photography (CFP) played an important role in screening due to its non-invasive and fast acquisition and broader availability of devices, including the recent emergence of portable handheld devices or applications on smartphones. Due to lower complexity of this imaging modality potentially less intricate algorithms are able to automatically analyze the images [ 23 ]. AI-based technology applied on CFP enables the detection of biomarkers such as drusen, haemorrhages and pigment abnormalities and may classify the eye following a binary (referable or non-referable) or multi-class scale (no AMD, early, intermediate, or advanced AMD) [ 24 , 25 ]. A previous study showed sensitivity and specificity of AI-based screening for intermediate and advanced AMD using CFP achieving 93.2% and 88.7%, respectively [ 26 ]. However, limitations including real-world applicability and generalizability as well as demonstrating the long-term benefits on functional outcomes still need further prospective studies. So far, CFP has not been able to provide other than descriptive epiphenomena of AMD.
Fundus autofluorescence (FAF) emerged as a non-invasive imaging modality able to detect light emission from fluorophores such as lipofuscin, supposedly present within the outer segment of photoreceptors and the RPE. This property allowed FAF to further distinguish retinal lesions such as pseudo-drusen and atrophic regions [ 27 ]. Usually, blue-light FAF (488 nm excitation wavelength) is most commonly used for imaging AMD, however longer wavelengths, such as green or near infrared, have shown advantages in detecting subtle changes. Recently, AI-based algorithms applied on FAF imaging were developed with the potential of automated segment macular lesions in GA [ 28 , 29 ]. However, FAF devices are not readily available in clinical practices. Furthermore, the perilesional patterns such as diffusely or focal granular, branching or reticular lipofuscin deposits can be subjectively interpreted and do not reliably correlate with GA lesion progression as neurosensory structures such as photoreceptors are not depicted by FAF.
The amount of information increases by millions due to the pixel-wise extraction when using AI on OCT images [ 30 , 31 ]. OCT is a non-invasive real-time high-resolution imaging tool of the retina, which, combined with robust algorithms, has the potential to diagnose retinal disease and predict advanced stages, treatment response and visual outcomes in AMD [ 32 ].
De Faw et al. from the Google group presented the use of automated algorithms in the triage of retinal diseases at Moorfields Eye hospital, determining therapeutic referral need of a patient’s condition. One of the algorithms presented referral recommendations reaching or exceeding the performance of eye care professionals for a range of sight-threatening retinal diseases [ 33 ]. The use of this technology on screening and referral pathways may open a cost-effective solution and could increase accessibility in areas of imbalance between caregivers and patients.
Since safety is a key issue in the AI field, the performance of human specialists and algorithms are compared to test and validate automated algorithms. For detection of retinal fluid in AMD patients, an AI-based algorithm showed higher accuracy than eye care professionals, [ 34 ] reinforcing the power of these automated measurement tools.
Post hoc analyses offer a good opportunity to validate and refine AI algorithms. However, the utilization of large-scale datasets, such as the Intelligent Research in Sight (IRIS) Registry, may be instrumental in prospectively evaluating AI systems in real-world scenarios [ 35 ]. The integration of AI with big real-world datasets not only facilitates the validation of AI technologies, but also contributes to the evolution of precision medicine by tailoring interventions based on the complexities observed in diverse patient populations. Nevertheless, in IRIS, image collection has not yet been integrated into AI and the variability of multiple devices and scan patterns used will present an important obstacle in performing a uniform image analysis.
AI techniques in OCT analysis
AI-based OCT analysis typically rely on different DL methods including convolutional neural networks (CNNs) and generative adversarial networks (GANs). CNNs in particular have been at the forefront of AI-driven image analysis in ophthalmology and in retina [ 36 ]. They exploit the fact that adjacent pixel values in the image are correlated and they excel at extracting hierarchical features from images, making them particularly effective for the vast amount of high-resolution image data present on OCT. In the context of AMD, CNNs can automatically learn and detect relevant retinal features to perform diverse tasks, such as layer segmentation and fluid quantification (Fig. 1 ) [ 37 ]. For example, Mishra et al. used CNNs to develop a shortest-path algorithm of 11 retinal layers, drusen and subretinal drusenoid deposits in SD-OCT based on probability maps (Fig. 2 ) [ 38 ]. Transfer learning, which involves fine-tuning pre-trained CNN models on OCT data, has proven beneficial for small datasets by leveraging knowledge gained from larger related datasets.
Layer segmentation is a critical step in extracting anatomical structures from OCT images. AI-powered segmentation algorithms employ techniques like U-Net, a convolutional network architecture tailored for segmentation tasks. Indeed, a precise segmentation aids in identifying disease-specific features such as photoreceptor integrity, defined as continued segmentation of the area between the top of the ellipsoid zone and the outer boundary of the interdigitation zone, and RPE layer disruption, enabling more accurate diagnosis and disease progression assessment [ 39 , 40 , 41 ].
Furthermore, GAN is a deep learning method that consist of two neural networks, a generator, and a discriminator, automatically working in tandem to produce high-quality synthetic data. In OCT analysis for AMD, GANs can aid in data augmentation for addressing the challenge of imbalanced datasets and for training models to detect abnormalities on OCT without the need for manual labelling [ 42 ]. GAN-generated images can also be applied for super-resolution and denoising in OCT images [ 43 ].
The foundation model’s ability to leverage vast datasets for nuanced pattern recognition can play an important role in the early detection of retinal diseases [ 44 ]. A self-supervised learning-based foundation model was recently described as capable of training on unlabelled retinal images and showing satisfactory performance in detecting ocular disease and predicting systemic disease based on retinal imaging. Furthermore, it holds promise for democratizing access to medical AI and advancing clinical implementation by providing a publicly available resource for further research and applications [ 44 ].
AI in OCT in intermediate AMD
Patients with early and intermediate AMD frequently show few to no symptoms. The speed of progression to advanced stages varies widely, hence it is of great importance to accurately identify key biomarkers and to predict the patient’s conversion, as vision loss occurs only in advanced stages with foveal destruction. Since early interventions with intravitreal therapies are more beneficial in preserving visual function of patients, there is a strong drive towards early detection of converters [ 45 ]. Multiple prognostic markers have been identified preceding conversion to advanced AMD including demographics, genotype and structural features. For example, the location, volume and size of drusen indicated the progression to nAMD, whereas outer retinal thinning led to GA [ 46 ]. However, the features are confluent suggesting a common mechanism among the different types of AMD.
Subretinal drusenoid deposits (SDD) have been shown to be a significant biomarker, associated with a higher risk for developing type 3 MNV, outer retinal atrophy and GA [ 47 , 48 ]. Therefore, it is desirable to automatically quantify them and implement in predictive progression models. However, segmentation of SDD can be challenging. A previous study showed an overall substantial inter-reader agreement regarding the presence of SDD, but a slight and moderate inter-reader agreement for presence of type 1 and type 2 SDD on selected OCT B-scans [ 49 ]. Furthermore, a larger difference between human graders and AI was reported for SDD when compared to drusen [ 38 ]. With targeted efforts in this field, automated SDD detection is advancing.
The ongoing PINNACLE trial consisting of multimodal imaging including over 400.000 OCT images from AMD patients with a follow-up of up to 3 years is conducted to characterize and validate biomarkers for conversion and, as secondary outcome, develop predictive risk models [ 50 ]. Recently, a DL classifier was developed using data from the PINNACLE study identifying normal eyes and the onset of early and intermediate AMD, GA and nAMD automatically. It consists of a two-stage CNN categorizing disease stages with an AUC of 0.94 in a real-world test set [ 51 ]. Based on multiple risk factors for conversion identified, ML algorithms were developed to predict nAMD or GA conversion in fellow eyes of nAMD patients [ 52 , 53 ]. Using the HARBOR trial dataset, an algorithm showed that converters to nAMD present different patterns than GA converters such as thickening of the RPE drusen complex, increased drusen area, HRF and outer nuclear layer (ONL) thickening in areas with hyperreflective foci. GA-converters on the other hand show global ONL thinning, RPE and inner segment/outer segment junction (IS/OS) thinning and hyperreflective foci in the ONL. This predictive model achieved an AUC of 0.68 with 0.46 specificity and 0.80 sensitivity for nAMD and a higher AUC of 0.80 with 0.69 specificity and 0.80 sensitivity for GA prediction [ 53 ].
AI in OCT in nAMD
Automated tools for guiding anti-VEGF therapy in nAMD is not new. Central subfield thickness (CST) is an automated measurement tool, widely used as endpoint and guide for treatment decisions in many clinical trials [ 14 , 54 ]. However, this parameter does not provide any detailed information about location and extension of disease-specific activity and is often not adequately aligned in diseased macula. Furthermore, it does not distinguish between neurosensorial layers, retinal fluid compartment and pigment epithelial detachments. Previous studies have shown a weak correlation between CST and visual acuity as well as between CST and retinal fluid volumes [ 55 , 56 ]. Moreover, in recent clinical studies comparing durability of the novel substances CRT values were used in various and irreproducible combinations making an objective comparison of the novel therapeutics impossible.
The most recent AI-based algorithms developed for OCT involve biomarkers such as fluid volume quantification in each compartment (intra- and subretinal), fibrovascular PED, subretinal hyperreflective material (SRHM) and hyperreflective foci [ 10 , 19 ]. Despite the importance of all high-order biomarkers on disease classification and progression, [ 57 , 58 ] post hoc analysis from TREND study and from the Fight Retinal Blindness! dataset using DL and ML showed that retinal fluid is still the most important anatomical biomarker for predicting disease activity, treatment demand and visual outcomes in nAMD [ 59 , 60 ]. Indeed, recent analysis showed that not only the location of fluid is important for disease progression, however the dynamic fluctuation of each retinal fluid has a high impact on the outomes [ 61 ]. Volumes and changes in volumes of retinal fluid have a substantial impact on vision outcome. Higher IRF and PED were associated with worse visual outcomes, despite IRF being the fluid type with faster response to anti-VEGF therapy. SRF showed slower resolution, intuitively leading to an increased number of injections during the first year in a pro-re-nata regimen, however no significant correlation with worse functional outcomes was found presumably due to the predominant location of SRF outside of the central 1 mm of the fovea [ 59 , 60 , 62 ]. This highlights the notion that traditional treatment patterns have to undergo a reality check by a rigorous structure/function correlation. In long-term follow up, retinal fluid volumes and visual acuity are not generally, but individually correlated, indicating a concomitant neurodegenerative process [ 63 ]. Furthermore, despite “regular” treatment, most of nAMD patients are prone to develop subretinal fibrosis and macular atrophy with time [ 64 , 65 ]. Higher amounts of fluid, particularly volume fluctuations, the presence of subretinal hyperreflective material and MNV type revealed to be correlated with atrophy or fibrosis development [ 61 , 66 , 67 , 68 ]. Photoreceptor loss and RPE loss were strongly correlated with development of macular atrophy [ 69 ]. Thus, to better understand late anatomical outcomes, early changes on the photoreceptor layers in association with fluid behaviour may be further investigated through precise in vivo measurments [ 70 ]. Furthermore, there is still an open question if SRF, overall or dependent on its dynamics, might be protective against macula atrophy development in patients with nAMD [ 71 ].
OCT angiography (OCTA) represents a powerful non-invasive technology able to analyse choroidal and retinal vessels, including MNV characteristics in nAMD. A previous study analysing MNV characteristics using OCTA found a correlation between higher vessel tortuosity within the MNV area and worse visual outcomes as well as stronger trend to atrophic changes, despite lower exudation at baseline [ 72 ]. In the other hand, Sulzbacher et al. reported no significant correlation between OCTA patterns such as vessels density of the neovascular lesion and BCVA [ 73 ]. An automated and precise tool applied to choroidal flow, vessel density and other MNV characteristics may open new perspectives on vascular biomarkers.
Home monitoring OCT
Home monitoring OCT has emerged as an innovative technological paradigm aimed at optimizing the surveillance of individuals affected by chronic sight-threatening pathologies, notably those requiring recurrent monitoring and fast intervention, such as nAMD [ 74 ]. Home screening tests for nAMD are not a novelty. Amsler grid and preferential hyperacuity perimetry has been previously proposed to detect metamorphopsia as an early sign of choroidal neovascularization in AMD patients [ 74 , 75 ]. The current development of an AI-based fluid monitoring algorithm implemented on a home OCT device (The Notal Vision Home OCT system) showed promising results with feasible self-scan rates. This technology is not yet available on the market, however it showed feasibility in early detection of biomarkers and advanced stages of AMD such as neovascularization [ 76 ]. Home monitoring OCT may bring advantages including a decreased number of visits to the eye hospital and close monitoring of disease progression. However, there are challenges such as quality of data acquisition, safety of data transfer and integration of the acquired data into a local healthcare system. A previous economic evaluation based on a simulation showed that a home visual-field monitoring system for early CNV detection was cost-effective compared with scheduled examinations alone on patients at risk of developing nAMD [ 77 ]. Further cost-effective simulations should be conducted for new devices. Additionally, the concomitant escalation in data volume stemming from increased imaging monitoring frequencies is a major issue. Also, compliance has always prevented reliable and long-term self-monitoring in chronic disease. Shared-care as already implemented in Great Britain with community-based monitoring “around the corner” by fully equipped opticians may be a more realistic model, particularly if supported by standardized AI-based detection tools.
AI in geographic atrophy
GA is characterized by degeneration of the photoreceptors and RPE, accompanied by degeneration of the subjacent choriocapillaris, leading to irreversible vision loss [ 78 , 79 ]. The literature mentions that GA affects around 5 million people worldwide, [ 80 ] however this number might be underestimating the total number of patients, as it primarily accounts for fovea-centered lesions. Consequently, it overlooks a significant spectrum of the disease in earlier stages all arising from the perifoveal area and being non-symptomatic. The diagnosis of GA was initially based on fundus photography [ 81 ]. Blue-light fundus autofluorescence (FAF) emerged as a valuable tool in diagnosing GA, since the degeneration of the RPE results in a clearly demarcated area of hypo-autofluorescence. Near-infrared reflectance (NIR) imaging, which has a longer wavelength, has also provided a benefit for visualization of GA lesions with lower interference caused by the macular luteal pigments [ 28 , 82 ]. Therefore, the number, location and size of GA lesions as well as other disease-specific biomarkers could be measured easier with FAF [ 83 ]. Therefore, FDA and European Medicines Agencies accepted FAF-based measurements of changes in GA area as anatomical endpoint in the early clinical trials [ 84 , 85 ]. Availability of high-resolution three-dimensional OCT imaging together with AI tools detecting the pathognomonic neurosensory, yet subclinical features not accessible to human specialists by retinal images alone, but accurately visualized on OCT-based AI analysis represent the novel horizon of precision medicine [ 28 , 29 , 86 ].
In clinical practice, OCT devices are widely available and are the gold standard in monitoring AMD. SD-OCT provides a more detailed information of the condition of the outer retinal layers, including the degeneration of photoreceptors in the lesions’ active junctional zone [ 47 , 87 ]. Therefore, OCT might provide more insights into progression patterns due to the detailed visualization of photoreceptor alteration. In a post-hoc analysis of the FILLY phase 2 clinical trial data set, findings from OCT imaging were consistent with FAF results measuring the RPE defect. Both imaging modalities could prove the superiority of treated patients regarding RPE loss, but only three-dimensional assessment in OCT was able to reveal early superior maintenance of photoreceptor integrity with complement inhibition [ 88 ]. To precisely measure these parameters, a DL algorithm segmenting A-scan regions on SD-OCT was clinically validated and tested using study datasets as well as real world images (Fig. 3 ) [ 88 ]. The GA monitor computes topographic maps and measurements of RPE and photoreceptor integrity loss based on 3D imaging and is accessible by a simple upload of a standard OCT image to the Heidelberg engineering Spectralis AppWay [ 41 ]. In the consecutive phase 3 studies, Oaks and Derby, high statistical significance with p < 0.0001 was provided demonstrating that disease activity, i.e. GA growth correlated with the ratio between photoreceptor integrity loss and RPE loss, i.e. the PR/RPE loss ratio. The PR/RPE ratio also strongly determined the level of therapeutic benefit in the study results qualifying as a most reliable clinical parameter for treatment indications in GA (Fig. 4 ) [ 89 , 90 ].
As the question remains unclear which patients should be treated, clinicians search for predictors of disease activity. For this purpose, a validated algorithm was used to predict topographic progression of GA by analysing RPE loss, photoreceptor integrity and hyperreflective foci (HRF) [ 53 ]. Higher progression rates of GA were associated with atrophies closer to the fovea, HRF at the junctional zone and thinner photoreceptor layers. These tools could help in identifying progression patterns, which would support clinicians to better identify patients, who would benefit from a potentially life-long treatment.
AI-based OCT tools in clinical trials
Despite the previously mentioned potential of using AI in clinical practice, another evident application pertains to the recruitment processes in clinical trials for AMD. Developing a new drug presents a financial challenge, attributable to regulatory requirements, extensive data collection and usually prolonged timelines associated with clinical trials. The use of ML and DL models exhibit substantial promise in accelerating the enrolment of participants who are more likely to present faster progression to advanced stages of the disease, may present stronger response to specific novel therapies, and demonstrate a reduced likelihood of premature withdrawal from a trial [ 91 ]. An efficient selection process can increase sample size and consequently the detection of statistically significant differences between groups on a trial [ 53 ]. On the other hand, selecting patients with higher disease activity and possibly stronger response to a specific therapy could improve patient enrolment in clinical trials. Additionally, automated algorithms can assess morphological endpoints in a precise and reliable manner, most importantly in real-time for all clinical sites providing highest image quality as the images immediately undergo analysis control. Especially in GA, functional endpoints such as best-corrected visual acuity are frequently insufficient to describe all aspects of visual impairment [ 83 ]. Structural biomarkers are less influenced by patient compliance than functional endpoints. Clinical trials have been performed to study how disease activity assessment is influenced and supported by AI-based enrichment of OCT images. RAZORBILL (NCT04662944), Notal Vision Home OCT study (NCT04642183), and a prospective study using fluid monitoring (NCT05093374) are examples of ongoing trials using automated segmentation of retinal fluid volumes. A clinical trial using a DL algorithm on OCTA (NCT05969418) to analyze neovascular membrane vessel characteristics has started and as previously mentioned in this review the PINNACLE clinical trial cohort is being conducted for AI-based segmentation of early atrophy and fluid-related biomarkers on OCT (NCT04269304). Less intricate algorithms based on CFP segmentation are also under investigation for screening purposes such as iPredict (NCT04863391) and VeriSee AMD (NCT05593913).
The limitations of AI-based algorithms
The implementation of AI-based algorithms in AMD diagnosis and treatment comes with the known limitations of innovative approaches. The demand of large datasets for training AI models may introduce biases, as certain demographics or variations in disease characteristics may be underrepresented. The ethical considerations surrounding patient privacy, data security, and the interpretability of AI decisions also raise concerns and must comply with different regulations in each nation. Another aspect to consider are adequate reimbursement models. Furthermore, the diversity of devices, launch of new imaging machines, and variations in imaging protocols across different clinical settings can hinder the development of universally applicable algorithms. While AI in retinal images holds promise, addressing these limitations is crucial to realizing its full potential in enhancing diagnostic accuracy and ensure its effective and ethical integration into clinical practice. The overwhelming introduction of OCT imaging hand-in-hand with anti-VEGF therapy using OCT hardware as a “fluid meter” clearly serves as a potent role model for the introduction of OCT-based AI analysis in times of GA management, an even bigger responsibility, 20 years later.
AI-based models can strongly benefit clinical practice and research in AMD. Multimodal imaging, the conventional standard for diagnosing patients with AMD, is being replaced by OCT imaging. Furthermore, DL and ML algorithms showed the potential of reliably quantifying biomarkers, predicting disease progression and assisting treatment decisions. The next steps have to be taken for bringing AI from clinical research to its clearly needed application in everyday clinical practice, however, ongoing advances in the field are steadily narrowing this gap.
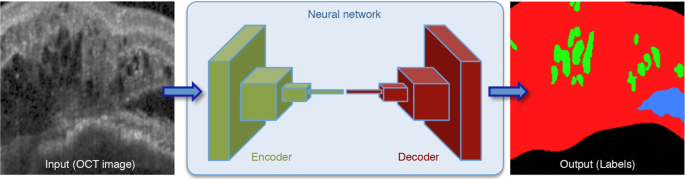
Convolutional neural network with an encoder - decoder architecture to identify intraretinal fluid (green) and subretinal fluid (blue). The retinal tissue is marked in red. Reproduced with permission from Schlegl et al., 2022 [ 37 ]. (For interpretation of the references to color in this figure legend, the reader is referred to the Web version of this article)
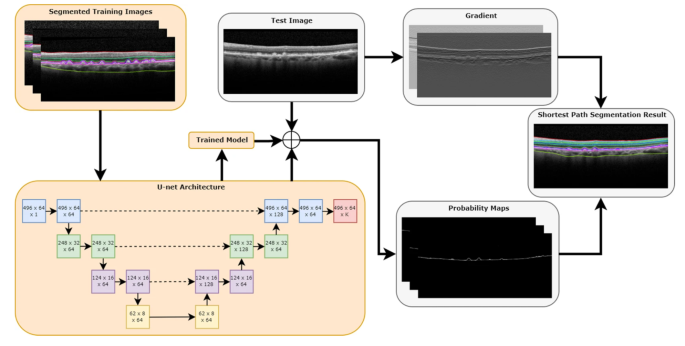
Deep learning utilizing a short-path approach for segmenting retinal layers. Orange boxes represent training steps and gray boxes represent evaluation steps. Reproduced with permission from Mishra et al., 2020 [ 38 ]
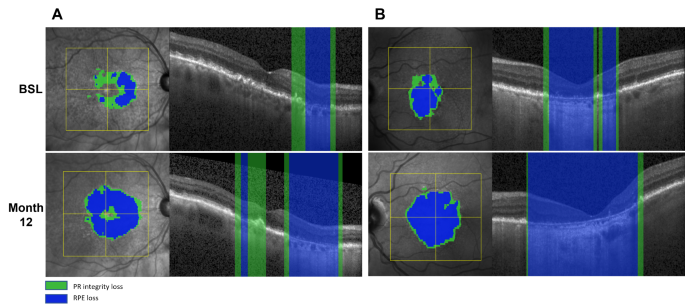
Geographic atrophy (GA) lesion from two patients at baseline (BSL) (upper row) and at month 12 (lower row) from OAKS clinical trial dataset automated segmented with the AI-based GA monitor. Retinal pigment epithelium (RPE) loss (blue) and photoreceptor integrity loss (green) are shown as en face visualizations (left) and example B-scans (right). RPE loss extends into regions of preexisting PR loss
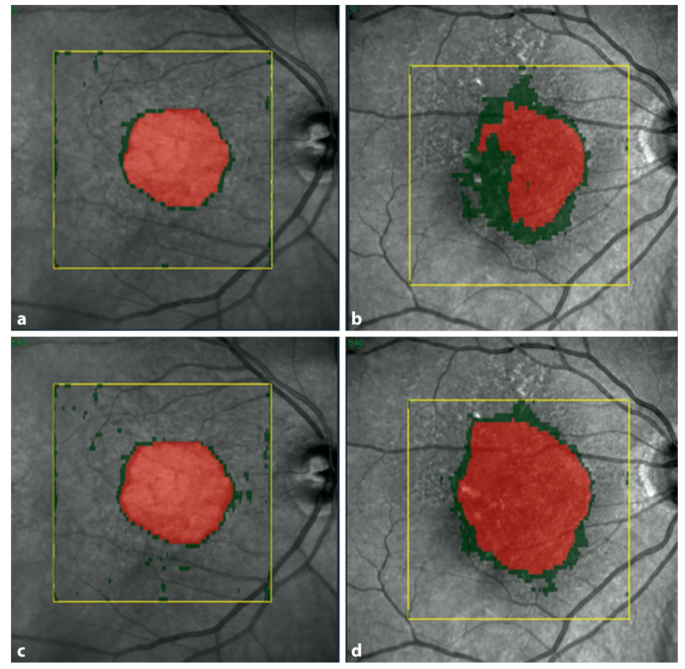
Example of 2 lesions with geographic atrophy and different PR/RPE loss ratios. PR loss is marked in green and RPE loss in red. ( a ) shows a lesion with a small PR/RPE loss ratio and ( b ) a lesion with a large PR/RPE loss ratio at baseline. Letters ( c ) and ( d ) show the respective lesions at month 12 with significantly faster growth in the lesion with the higher ratio ( d ). Reproduced with permission from Schmidt-Erfurth et al., 2023 [ 90 ]
Feigenbaum MJ, Mermin ND. Artificial Intelligence A Modern Approach, 4th Ed. Prentice Hall . Vol 56.; 1988. https://doi.org/10.1119/1.15422 .
Samuel AL. Some studies in machine learning. IBM J Res Dev. 1959;3(3):210–29. https://ieeexplore.ieee.org/stamp/stamp.jsp?tp=&arnumber=5392560 .
Article Google Scholar
Lecun Y, Bengio Y, Hinton G. Deep learning. Nature. 2015;521(7553):436–44. https://doi.org/10.1038/nature14539 .
Article CAS PubMed Google Scholar
Shortliffe EH. Mycin: a Knowledge-Based Computer Program Applied To Infectious Diseases. 1977;(May):66–69.
Ghanem-Zoubi NO, Vardi M, Laor A, Weber G, Bitterman H. Assessment of disease-severity scoring systems for patients with sepsis in general internal medicine departments. Crit Care. 2011;15(2). https://doi.org/10.1186/cc10102 .
Henry KE, Hager DN, Pronovost PJ, Saria S. A targeted real-time early warning score (TREWScore) for septic shock. Sci Transl Med. 2015;7(299). https://doi.org/10.1126/scitranslmed.aab3719 .
Nitski O, Azhie A, Qazi-Arisar FA, et al. Long-term mortality risk stratification of liver transplant recipients: real-time application of deep learning algorithms on longitudinal data. Lancet Digit Heal. 2021;3(5):e295–305. https://doi.org/10.1016/S2589-7500(21)00040-6 .
Article CAS Google Scholar
Fleckenstein M, Keenan TDL, Guymer RH, et al. Age-related macular degeneration. Nat Rev Dis Prim. 2021;7(1). https://doi.org/10.1038/s41572-021-00265-2 .
Lim LS, Mitchell P, Seddon JM, Holz FG, Wong TY. Age-related macular degeneration. Lancet. 2012;379(9827):1728–38. https://doi.org/10.1016/S0140-6736(12)60282-7 .
Article PubMed Google Scholar
Schmidt-Erfurth U, Waldstein SM. A paradigm shift in imaging biomarkers in neovascular age-related macular degeneration. Prog Retin Eye Res. 2016;50:1–24. https://doi.org/10.1016/J.PRETEYERES.2015.07.007 .
Martin DF, Maguire MG, Fine SL, et al. Ranibizumab and Bevacizumab for treatment of neovascular age-related macular degeneration: two-year results. Ophthalmology. 2012;119(7):1388–98. https://doi.org/10.1016/j.ophtha.2012.03.053 .
Brown DM, Kaiser PK, Michels M, et al. Ranibizumab versus Verteporfin for Neovascular Age-Related Macular Degeneration. N Engl J Med. 2006;355(14):1432–44. https://doi.org/10.1056/nejmoa062655 .
Kaiser SM, Arepalli S, Ehlers JP. Current and future anti-VEGF agents for neovascular age-related macular degeneration. J Exp Pharmacol. 2021;13:905–12. https://doi.org/10.2147/JEP.S259298 .
Article PubMed PubMed Central Google Scholar
Bakri SJ, Thorne JE, Ho AC, et al. Safety and efficacy of anti-vascular endothelial growth factor therapies for Neovascular Age-Related Macular Degeneration: a report by the American Academy of Ophthalmology. Ophthalmology. 2019;126(1):55–63. https://doi.org/10.1016/j.ophtha.2018.07.028 .
Heier JS, Khanani AM, Quezada Ruiz C, et al. Efficacy, durability, and safety of intravitreal faricimab up to every 16 weeks for neovascular age-related macular degeneration (TENAYA and LUCERNE): two randomised, double-masked, phase 3, non-inferiority trials. Lancet. 2022;399(10326):729–40. https://doi.org/10.1016/S0140-6736(22)00010-1 .
Study of the Effects of High Dose Aflibercept Injected Into the Eye of Patients With an Age-related Disorder That Causes Loss of Vision Due to Growth of Abnormal Blood Vessels at the Back of the Eye (PULSAR), NCT04423718. Accessed January 16. 2024. https://clinicaltrials.gov/study/NCT04423718 .
Majid Khan OE, Akinyemi M, Masud MJ, Thomas, Nicholas Martin AMK. Targeting the complement system in geographic atrophy: mechanisms and therapeutics. Expert Rev Ophthalmol. 2024;19(1):47–65. https://doi.org/10.1080/17469899.2023.2286607 .
Oganov AC, Seddon I, Jabbehdari S, et al. Artificial intelligence in retinal image analysis: development, advances, and challenges. Surv Ophthalmol. 2023;68(5):905–19. https://doi.org/10.1016/j.survophthal.2023.04.001 .
Moraes G, Fu DJ, Wilson M, et al. Quantitative Analysis of OCT for Neovascular Age-Related Macular Degeneration using deep learning. Ophthalmology. 2021;128(5):693–705. https://doi.org/10.1016/j.ophtha.2020.09.025 .
Schmidt-Erfurth U, Sadeghipour A, Gerendas BS, Waldstein SM, Bogunović H. Artificial intelligence in retina. Prog Retin Eye Res. 2018;67. https://doi.org/10.1016/j.preteyeres.2018.07.004 .
Thiele S, Nadal J, Pfau M, et al. Prognostic value of intermediate age-related macular degeneration phenotypes for geographic atrophy progression. Br J Ophthalmol. 2021;105(2):239–45. https://doi.org/10.1136/bjophthalmol-2020-316004 .
Hollaus M, Iby J, Brugger J, et al. The influence of drusenoid pigment epithelial detachments on the progression of age-related macular degeneration and visual acuity. Can J Ophthalmol Can D’ophtalmologie. 2024;0008-41821–7. https://doi.org/10.1016/j.jcjo.2023.12.007 .
Das S, Kuht HJ, De Silva I, et al. Feasibility and clinical utility of handheld fundus cameras for retinal imaging. Eye. 2023;37(2):274–9. https://doi.org/10.1038/s41433-021-01926-y .
Burlina PM, Joshi N, Pekala M, Pacheco KD, Freund DE, Bressler NM. Automated grading of age-related macular degeneration from color fundus images using deep convolutional neural networks. JAMA Ophthalmol. 2017;135(11):1170–6. https://doi.org/10.1001/jamaophthalmol.2017.3782 .
Yellapragada B, Hornauer S, Snyder K, Yu S, Yiu G. Self-supervised feature learning and phenotyping for assessing age-related Macular Degeneration using Retinal Fundus images. Ophthalmol Retin. 2022;6(2):116–29. https://doi.org/10.1016/j.oret.2021.06.010 .
Ting DSW, Cheung CYL, Lim G, et al. Development and validation of a deep learning system for diabetic retinopathy and related eye diseases using retinal images from multiethnic populations with diabetes. JAMA - J Am Med Assoc. 2017;318(22):2211–23. https://doi.org/10.1001/jama.2017.18152 .
Ly A, Nivison-Smith L, Assaad NKM. Fundus Autofluorescence in Age-related Macular Degeneration. Age-related Macular Degeneration. Volume 94. CRC; 2007. pp. 209–20. https://doi.org/10.1097/OPX.0000000000000997 .
Spaide T, Jiang J, Patil J, et al. Geographic Atrophy Segmentation using Multimodal Deep Learning. Transl Vis Sci Technol. 2023;12(7):1–13. https://doi.org/10.1167/tvst.12.7.10 .
Arslan J, Samarasinghe G, Sowmya A, et al. Deep learning applied to automated segmentation of geographic atrophy in fundus autofluorescence images. Transl Vis Sci Technol. 2021;10(8):1–17. https://doi.org/10.1167/tvst.10.8.2 .
Bogunović H, Montuoro A, Baratsits M, et al. Machine learning of the progression of Intermediate Age-Related Macular Degeneration based on OCT imaging. Investig Ophthalmol Vis Sci. 2017;58(6):BIO141–50. https://doi.org/10.1167/iovs.17-21789 .
Lee CS, Baughman DM, Lee AY. Deep learning is effective for classifying normal versus age-related Macular Degeneration OCT images. Ophthalmol Retin. 2017;1(4):322–7. https://doi.org/10.1016/j.oret.2016.12.009 .
Romond K, Alam M, Kravets S, et al. Imaging and artificial intelligence for progression of age-related macular degeneration. Exp Biol Med. 2021;246(20):2159–69. https://doi.org/10.1177/15353702211031547 .
De Fauw J, Ledsam JR, Romera-Paredes B, et al. Clinically applicable deep learning for diagnosis and referral in retinal disease. Nat Med. 2018;24(9):1342–50. https://doi.org/10.1038/s41591-018-0107-6 .
Keenan TDL, Clemons TE, Domalpally A, et al. Retinal specialist versus Artificial Intelligence Detection of Retinal Fluid from OCT: Age-Related Eye Disease Study 2: 10-Year Follow-On study. Ophthalmology. 2021;128(1):100–9. https://doi.org/10.1016/J.OPHTHA.2020.06.038 .
Knapp AN, Leng T, Rahimy E. Ophthalmology at the forefront of Big Data Integration in Medicine: insights from the IRIS Registry Database. Yale J Biol Med. 2023;96(3):421–6. https://doi.org/10.59249/VUPM2510 .
Wang D, Zhang M. Artificial Intelligence in Optical Communications: from machine learning to Deep Learning. Front Commun Networks. 2021;2(March):1–9. https://doi.org/10.3389/frcmn.2021.656786 .
Schlegl T, Waldstein SM, Bogunovic H, et al. Fully Automated Detection and Quantification of Macular Fluid in OCT using deep learning. Ophthalmology. 2018;125(4):549–58. https://doi.org/10.1016/j.ophtha.2017.10.031 .
Mishra Z, Ganegoda A, Selicha J, Wang Z, Sadda SVR, Hu Z. Automated retinal layer segmentation using graph-based Algorithm Incorporating Deep-learning-derived information. Sci Rep. 2020;10(1):1–8. https://doi.org/10.1038/s41598-020-66355-5 .
Fazekas B, Lachinov D, Aresta G, Mai J, Schmidt-Erfurth U, Bogunović H. Segmentation of Bruch’s membrane in retinal OCT with AMD using anatomical priors and uncertainty quantification. IEEE J Biomed Heal Inf. 2023;27(1):41–52. https://doi.org/10.1109/JBHI.2022.3217962 .
Orlando JI, Gerendas BS, Riedl S, et al. Automated quantification of photoreceptor alteration in macular disease using Optical Coherence Tomography and Deep Learning. Sci Rep. 2020;10(1):1–12. https://doi.org/10.1038/s41598-020-62329-9 .
Mai J, Lachinov D, Riedl S, et al. Clinical validation for automated geographic atrophy monitoring on OCT under complement inhibitory treatment. Sci Rep. 2023;13(1):1–11. https://doi.org/10.1038/s41598-023-34139-2 .
Schlegl T, Seeböck P, Waldstein SM, Langs G, Schmidt-Erfurth U. f-AnoGAN: fast unsupervised anomaly detection with generative adversarial networks. Med Image Anal. 2019;54:30–44. https://doi.org/10.1016/j.media.2019.01.010 .
Kugelman J, Alonso-Caneiro D, Read SA, Collins MJ. A review of generative adversarial network applications in optical coherence tomography image analysis. J Optom. 2022;15:S1–11. https://doi.org/10.1016/j.optom.2022.09.004 .
Zhou Y, Chia MA, Wagner SK, et al. A foundation model for generalizable disease detection from retinal images. Nature. 2023;622(7981):156–63. https://doi.org/10.1038/s41586-023-06555-x .
Article CAS PubMed PubMed Central Google Scholar
Muntean GA, Marginean A, Groza A, et al. The predictive capabilities of Artificial Intelligence-based OCT analysis for age-related Macular Degeneration Progression—A systematic review. Diagnostics. 2023;13(14):1–35. https://doi.org/10.3390/diagnostics13142464 .
Hagag AM, Kaye R, Hoang V, et al. Systematic review of prognostic factors associated with progression to late age-related macular degeneration: Pinnacle study report 2. Surv Ophthalmol. 2023. https://doi.org/10.1016/j.survophthal.2023.10.010 .
Reiter GS, Told R, Schranz M, et al. Subretinal drusenoid deposits and photoreceptor loss detecting global and local progression of geographic atrophy by SD-OCT imaging. Investig Ophthalmol Vis Sci. 2020;61(6). https://doi.org/10.1167/IOVS.61.6.11 .
Sleiman K, Veerappan M, Winter KP, et al. Optical coherence tomography predictors of risk for progression to non-neovascular atrophic age-related Macular Degeneration. Ophthalmology. 2017;124(12):1764–77. https://doi.org/10.1016/j.ophtha.2017.06.032 .
Wu Z, Schmitz-Valckenberg S, Blodi BA, et al. Reticular pseudodrusen: interreader agreement of evaluation on OCT imaging in Age-Related Macular Degeneration. Ophthalmol Sci. 2023;3(4):100325. https://doi.org/10.1016/j.xops.2023.100325 .
Sutton J, Menten MJ, Riedl S, et al. Developing and validating a multivariable prediction model which predicts progression of intermediate to late age-related macular degeneration—the PINNACLE trial protocol. Eye. 2023;37(6):1275–83. https://doi.org/10.1038/s41433-022-02097-0 .
Leingang O, Riedl S, Mai J, et al. Automated deep learning-based AMD detection and staging in real-world OCT datasets (PINNACLE study report 5). Sci Rep. 2023;13(1):1–13. https://doi.org/10.1038/s41598-023-46626-7 .
Yim J, Chopra R, Spitz T, et al. Predicting conversion to wet age-related macular degeneration using deep learning. Nat Med. 2020;26(6):892–9. https://doi.org/10.1038/s41591-020-0867-7 .
Schmidt-Erfurth U, Waldstein SM, Klimscha S, et al. Prediction of individual disease conversion in early AMD using artificial intelligence. Investig Ophthalmol Vis Sci. 2018;59(8):3199–208. https://doi.org/10.1167/iovs.18-24106 .
Fung AE, Lalwani GA, Rosenfeld PJ, et al. An optical coherence Tomography-Guided, variable dosing regimen with Intravitreal Ranibizumab (Lucentis) for Neovascular Age-related Macular Degeneration. Am J Ophthalmol. 2007;143(4). https://doi.org/10.1016/j.ajo.2007.01.028 .
Pawloff M, Bogunovic H, Gruber A, Michl M, Riedl S, Schmidt-Erfurth U. SYSTEMATIC CORRELATION of CENTRAL SUBFIELD THICKNESS with RETINAL FLUID VOLUMES QUANTIFIED by DEEP LEARNING in the MAJOR EXUDATIVE MACULAR DISEASES. Retina. 2022;42(5):831–41. https://doi.org/10.1097/IAE.0000000000003385 .
NANEGRUNGSUNK O, GU SZ, BRESSLER SB, et al. Correlation of change in Central Subfield Thickness and Change in Visual Acuity in Neovascular AMD: Post Hoc Analysis of VIEW 1 and 2. Am J Ophthalmol. 2022;238:97–102. https://doi.org/10.1016/j.ajo.2021.11.020 .
Ehlers JP, Zahid R, Kaiser PK, et al. Longitudinal Assessment of Ellipsoid Zone Integrity, Subretinal Hyperreflective Material, and Subretinal Pigment Epithelium Disease in Neovascular Age-Related Macular Degeneration. Ophthalmol Retin. 2021;5(12):1204–13. https://doi.org/10.1016/j.oret.2021.02.012 .
Hosoda Y, Miyake M, Yamashiro K, et al. Deep phenotype unsupervised machine learning revealed the significance of pachychoroid features in etiology and visual prognosis of age-related macular degeneration. Sci Rep. 2020;10(1):1–13. https://doi.org/10.1038/s41598-020-75451-5 .
Bogunović H, Mares V, Reiter GS, Schmidt-Erfurth U. Predicting treat-and-extend outcomes and treatment intervals in neovascular age-related macular degeneration from retinal optical coherence tomography using artificial intelligence. Front Med. 2022;9(August):1–12. https://doi.org/10.3389/fmed.2022.958469 .
Mares V, Schmidt UM, Leingang O, et al. Approved AI- based fluid monitoring to identify morphological and functional treatment outcomes in related macular degeneration in real- world routine (FRB!). Published Online. 2023;1–7. https://doi.org/10.1136/bjo-2022-323014 .
Chakravarthy U, Havilio M, Syntosi A, et al. Impact of macular fluid volume fluctuations on visual acuity during anti-VEGF therapy in eyes with nAMD. Eye. 2021;35(11):2983–90. https://doi.org/10.1038/s41433-020-01354-4 .
Schmidt-Erfurth U, Vogl WD, Jampol LM, Bogunović H. Application of automated quantification of Fluid volumes to Anti–VEGF therapy of Neovascular Age-Related Macular Degeneration. Ophthalmology. 2020;127(9):1211–9. https://doi.org/10.1016/j.ophtha.2020.03.010 .
Schmidt-Erfurth U, Reiter GS, Riedl S, et al. AI-based monitoring of retinal fluid in disease activity and under therapy. Prog Retin Eye Res. 2022;86. https://doi.org/10.1016/j.preteyeres.2021.100972 .
Gillies MC, Hunyor AP, Arnold JJ, et al. Macular Atrophy in Neovascular Age-Related Macular Degeneration: a randomized clinical trial comparing Ranibizumab and Aflibercept (RIVAL Study). Ophthalmology. 2020;127(2):198–210. https://doi.org/10.1016/j.ophtha.2019.08.023 .
Teo KYC, Zhao J, Ibrahim FI et al. Features associated with vision in eyes with sub foveal fibrosis from neovascular AMD. Am J Ophthalmol. Published online 2023. https://doi.org/10.1016/j.ajo.2023.12.011 .
Reiter GS, Mares V, Leingang O, et al. Long-term effect of fluid volumes during the maintenance phase in neovascular age-related macular degeneration in the real world: results from Fight Retinal blindness! Can J Ophthalmol Published Online. 2023. https://doi.org/10.1016/j.jcjo.2023.10.017 .
Mathis T, Holz FG, Sivaprasad S, et al. Characterisation of macular neovascularisation subtypes in age-related macular degeneration to optimise treatment outcomes. Eye. 2023;37(9):1758–65. https://doi.org/10.1038/s41433-022-02231-y .
Llorente-González S, Hernandez M, González-Zamora J, et al. The role of retinal fluid location in atrophy and fibrosis evolution of patients with neovascular age-related macular degeneration long-term treated in real world. Acta Ophthalmol. 2022;100(2):e521–31. https://doi.org/10.1111/aos.14905 .
Finn AP, Pistilli M, Tai V, et al. Localized Optical Coherence Tomography precursors of Macular Atrophy and Fibrotic Scar in the comparison of age-related Macular Degeneration treatments trials. Am J Ophthalmol. 2021;223:338–47. https://doi.org/10.1016/j.ajo.2020.11.002 .
Riedl S, Cooney L, Grechenig C, et al. Topographic analysis of photoreceptor loss correlated with disease morphology in neovascular age-related macular degeneration. Retina. 2020;40(11):2148–57. https://doi.org/10.1097/IAE.0000000000002717 .
Siedlecki J, Fischer C, Schworm B, et al. Impact of Sub-retinal Fluid on the long-term incidence of Macular Atrophy in Neovascular Age-related Macular Degeneration under treat & extend anti-vascular endothelial growth factor inhibitors. Sci Rep. 2020;10(1):1–8. https://doi.org/10.1038/s41598-020-64901-9 .
Arrigo A, Aragona E, Bordato A, et al. Morphological and functional relationship between OCTA and FA/ICGA quantitative features in AMD-Related Macular Neovascularization. Front Med. 2021;8(October):1–9. https://doi.org/10.3389/fmed.2021.758668 .
Sulzbacher F, Pollreisz A, Kaider A, Kickinger S, Sacu S, Schmidt-Erfurth U. Identification and clinical role of choroidal neovascularization characteristics based on optical coherence tomography angiography. Acta Ophthalmol. 2017;95(4):414–20. https://doi.org/10.1111/aos.13364 .
Keenan TDL, Loewenstein A. Artificial intelligence for home monitoring devices. Curr Opin Ophthalmol. 2023;34(5):441–8. https://doi.org/10.1097/ICU.0000000000000981 .
Bjerager J, Schneider M, Potapenko I, et al. Diagnostic accuracy of the Amsler Grid Test for detecting Neovascular Age-Related Macular Degeneration: a systematic review and Meta-analysis. JAMA Ophthalmol. 2023;141(4):315–23. https://doi.org/10.1001/jamaophthalmol.2022.6396 .
Liu Y, Holekamp NM, Heier JS. Prospective, longitudinal study: Daily Self-Imaging with Home OCT for Neovascular Age-Related Macular Degeneration. Ophthalmol Retin. 2022;6(7):575–85. https://doi.org/10.1016/j.oret.2022.02.011 .
Wittenborn JS, Clemons T, Regillo C, Rayess N, Liffmann Kruger D, Rein D. Economic evaluation of a home-based age-related macular degeneration monitoring system. JAMA Ophthalmol. 2017;135(5):452–9. https://doi.org/10.1001/jamaophthalmol.2017.0255 .
Li M, Huisingh C, Messinger J, et al. Histology of geographic atrophy secondary to age-related macular degeneration a multilayer approach. Retina. 2018;38(10):1937–53. https://doi.org/10.1097/IAE.0000000000002182 .
Sadda SR, Guymer R, Holz FG, et al. Consensus Definition for Atrophy Associated with Age-Related Macular Degeneration on OCT: classification of Atrophy Report 3. Ophthalmology. 2018;125(4):537–48. https://doi.org/10.1016/j.ophtha.2017.09.028 .
Boyer DS, Schmidt-Erfurth U, Van Lookeren Campagne M, Henry EC, Brittain C. The pathophysiology of geographic atrophy secondary to age-related macular degeneration and the complement pathway as a therapeutic target. Retina. 2017;37(5):819–35. https://doi.org/10.1097/IAE.0000000000001392 .
Lindblad AS, Lloyd PC, Clemons TE, et al. Change in area of geographic atrophy in the age-related eye disease study: AREDS report number 26. Arch Ophthalmol. 2009;127(9):1168–74. https://doi.org/10.1001/archophthalmol.2009.198 .
Abdelfattah NS, Sadda J, Wang Z, Hu Z, Sadda S. Near-Infrared Reflectance Imaging for Quantification of Atrophy Associated with Age-Related Macular Degeneration. Am J Ophthalmol. 2020;212:169–74. https://doi.org/10.1016/j.ajo.2020.01.005 .
Sadda S, Usha C, Birch DG, Staurenghi G, Henry EC, Brittain C. Review CLINICAL ENDPOINTS FOR THE STUDY OF AGE-RELATED MACULAR DEGENERATION. Retina. 2016;36:1806–22.
Jaffe GJ, Westby K, Csaky KG, et al. C5 inhibitor Avacincaptad Pegol for Geographic Atrophy due to age-related Macular Degeneration: a Randomized Pivotal Phase 2/3 Trial. Ophthalmology. 2021;128(4):576–86. https://doi.org/10.1016/j.ophtha.2020.08.027 .
Steinle NC, Pearce I, Monés J, et al. Impact of baseline characteristics on Geographic Atrophy Progression in the FILLY Trial evaluating the complement C3 inhibitor Pegcetacoplan. Am J Ophthalmol. 2021;227:116–24. https://doi.org/10.1016/j.ajo.2021.02.031 .
Anegondi N, Gao SS, Steffen V, et al. Deep learning to Predict Geographic Atrophy Area and Growth Rate from Multimodal Imaging. Ophthalmol Retin. 2023;7(3):243–52. https://doi.org/10.1016/j.oret.2022.08.018 .
Fleckenstein M, Schmitz-Valckenberg S, Adrion C, et al. Tracking progression with spectral-domain optical coherence tomography in geographic atrophy caused by age-related macular degeneration. Investig Ophthalmol Vis Sci. 2010;51(8):3846–52. https://doi.org/10.1167/iovs.09-4533 .
Mai J, Riedl S, Reiter GS, et al. Comparison of Fundus Autofluorescence Versus Optical Coherence Tomography–based evaluation of the therapeutic response to Pegcetacoplan in Geographic Atrophy. Am J Ophthalmol. 2022;244:175–82. https://doi.org/10.1016/j.ajo.2022.06.023 .
Riedl S, Vogl WD, Mai J, et al. The Effect of Pegcetacoplan Treatment on Photoreceptor Maintenance in Geographic Atrophy monitored by Artificial intelligence–based OCT analysis. Ophthalmol Retin. 2022;6(11):1009–18. https://doi.org/10.1016/j.oret.2022.05.030 .
Schmidt-Erfurth U, Mai J, Reiter GS, et al. Monitoring of the progression of geographic atrophy with optical coherence tomography. Ophthalmologie. 2023;120(9):965–9. https://doi.org/10.1007/s00347-023-01891-9 .
Yaghy A, Lee AY, Keane PA, et al. Artificial intelligence-based strategies to identify patient populations and advance analysis in age-related macular degeneration clinical trials. Exp Eye Res. 2022;220(April):109092. https://doi.org/10.1016/j.exer.2022.109092 .
Download references
HB received research funds from Heidelberg Engineering and Apellis. GSR received research funds from RetInSight and is a scientific consultant for Bayer. US-E is a scientific consultant for Abbvie, Annexon, Apellis, Aviceda, Complement Therapeutix, Genentech, Heidelberg Engineering, Kodiak, RetInSight, Novartis, Roche, Topcon. Others report nothing to declare.
Author information
Authors and affiliations.
Laboratory for Ophthalmic Image Analysis, Department of Ophthalmology and Optometry, Medical University of Vienna, Währinger Gürtel 18-20, 1090, Vienna, Austria
Virginia Mares, Hrvoje Bogunovic, Sophie Frank, Gregor S. Reiter & Ursula Schmidt-Erfurth
Department of Ophthalmology, Federal University of Minas Gerais, Belo Horizonte, Brazil
Virginia Mares & Marcio B. Nehemy
You can also search for this author in PubMed Google Scholar
Contributions
VM: Major contributor in writing the manuscript. MBN: Writing/reviewing the manuscript. HB: Writing/reviewing the manuscript. SF: Writing the manuscript. GSR: Writing/reviewing the manuscript. US-E: Writing/reviewing the manuscript and supervisor of the project. All authors read and approved the final manuscript.
Corresponding author
Correspondence to Ursula Schmidt-Erfurth .
Ethics declarations
Conflict of interest.
No conflicting relationship exists for any author.
Competing interests
The author(s) declare(s) that they have no competing interests related with this publication.
Additional information
Publisher’s note.
Springer Nature remains neutral with regard to jurisdictional claims in published maps and institutional affiliations.
Rights and permissions
Open Access This article is licensed under a Creative Commons Attribution 4.0 International License, which permits use, sharing, adaptation, distribution and reproduction in any medium or format, as long as you give appropriate credit to the original author(s) and the source, provide a link to the Creative Commons licence, and indicate if changes were made. The images or other third party material in this article are included in the article’s Creative Commons licence, unless indicated otherwise in a credit line to the material. If material is not included in the article’s Creative Commons licence and your intended use is not permitted by statutory regulation or exceeds the permitted use, you will need to obtain permission directly from the copyright holder. To view a copy of this licence, visit http://creativecommons.org/licenses/by/4.0/ . The Creative Commons Public Domain Dedication waiver ( http://creativecommons.org/publicdomain/zero/1.0/ ) applies to the data made available in this article, unless otherwise stated in a credit line to the data.
Reprints and permissions
About this article
Cite this article.
Mares, V., Nehemy, M.B., Bogunovic, H. et al. AI-based support for optical coherence tomography in age-related macular degeneration. Int J Retin Vitr 10 , 31 (2024). https://doi.org/10.1186/s40942-024-00549-1
Download citation
Received : 14 February 2024
Accepted : 16 March 2024
Published : 08 April 2024
DOI : https://doi.org/10.1186/s40942-024-00549-1
Share this article
Anyone you share the following link with will be able to read this content:
Sorry, a shareable link is not currently available for this article.
Provided by the Springer Nature SharedIt content-sharing initiative
- Age-related macular degeneration
- Artificial intelligence
- Choroidal neovascularization
- Deep learning
- Geographic atrophy
- Optical coherence tomography
International Journal of Retina and Vitreous
ISSN: 2056-9920
- Submission enquiries: Access here and click Contact Us
- General enquiries: [email protected]
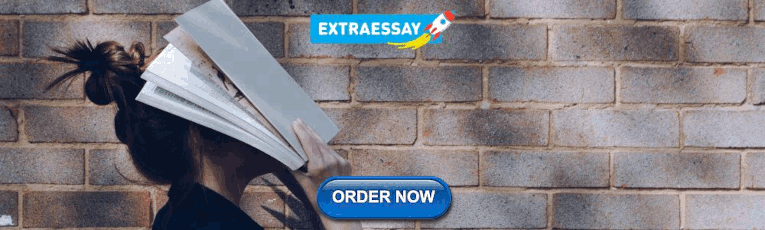
COMMENTS
MYCIN Expert System was an expert system using backward chaining, a form of artificial intelligence, to treat meningitis." ... For a patient case, it would determine the likelihood of various bacterial organisms being responsible based on provided symptoms and test results.
MYCIN was an early backward chaining expert system that used artificial intelligence to identify bacteria causing severe infections, such as bacteremia and meningitis, and to recommend antibiotics, with the dosage adjusted for patient's body weight — the name derived from the antibiotics themselves, as many antibiotics have the suffix "-mycin".The Mycin system was also used for the diagnosis ...
MYCIN, an early expert system, or artificial intelligence (AI) program, for treating blood infections. In 1972 work began on MYCIN at Stanford University in California. MYCIN would attempt to diagnose patients based on reported symptoms and medical test results. The program could request further information concerning the patient, as well as suggest additional laboratory tests, to arrive at a ...
Share to Linkedin. MYCIN was an AI program developed at Stanford University in the early 1970s, designed to assist physicians by recommending treatments for certain infectious diseases. AI pioneer ...
Control Conditions in Mycin: A Case Study. Mycin, developed at Stanford University by E.H.Shortliffe (Buchanan and Shortliffe, 1984) in the mid 1970s, was the first expert system to demonstrate impressive levels of performance in a medical domain. ... Exper imental Methods for Artificial Intelligence, Paul R. Cohen, 1995 Mon Jul 15 17:05:56 MDT ...
The Context of the MYCIN Experiments Artificial Intelligence (AI) is that branch of computer science dealing with symbolic, nonalgorithmic methods of problem solving. Several aspects of ... The whole expert system is used to perform a task, in MYCIN's case to provide diagnostic and therapeutic advice about a patient with an in- fection as ...
This suggests the need for general tools to aid in the construction of knowledge-based systems. This chapter describes an effective domain-independent framework for constructing one class of expert programs: rule-based consultants. The system, called EMYCIN, is based on the domain-independent core of the MYCIN program.
Mycin was an expert system developed at Stanford in the 1970s. Its job was to diagnose and recommend treatment for certain blood infections. To do the diagnosis ``properly'' involves growing cultures of the infecting organism. Unfortunately this takes around 48 hours, and if doctors waited until this was complete their patient might be dead!
The last seven years have seen the field of artificial intelligence (AI) trans-formed. This transformation is not simple, nor has it yet run its course. ... MYCIN provides a pure case of the original pun. It is expert in an ... AI is case-study science with a vengeance. But if that were not enough of a problem, the payoff structure of AI ...
Slide 13 of 20
Artificial Intelligence 61 (1993) 209-261 Elsevier ARTINT 959 209 ... Lindsay, R.K., B.G. Buchanan, E.A. Feigenbaum and J. Lederberg, DENDRAL: a case study of the first expert system for scientific hypothesis formation, Artificial Intelligence 61 ... Allen Newell identifies MYCIN as "the granddaddy of expert
The present paper provides summary of rule application concepts of MYCIN expert system, an AI program designed to provide expert level solutions to complex problems, to be understandable, and to be flexible enough to accommodate new knowledge easily. MYCIN was the one of the initial expert systems to perform with the level of expertise of a human expert and to provide users with complete ...
A rule-based expert system is described which uses artificial intelligence techniques, and a model of the interaction between physicians and human consultants, to attempt to satisfy the demands of ...
MYCIN was an early expert system that used artificial intelligence to identify severe infections, such as bacteremia and meningitis, and to recommend antibiotics, with the dosage adjusted for ...
Despite deep learning's wide adoption in dental artificial intelligence (AI) research, researchers from other dental fields and, more so, dental professionals may find it challenging to understand and interpret deep learning studies, their employed methods, and outcomes. The objective of this primer is to explain the basic concept of deep learning.
MYCIN was an early backward chaining expert system that used AI to identify microorganisms causing severe diseases like bacteremia and meningitis and propose antibiotics based on patient weight. MYCIN was first developed at Stanford University in California in 1972. MYCIN would make an effort to make a diagnosis based on patient reports of ...
MYCIN is an interactive computer program, relying to a large extent upon artificial intelligence (AI) techniques, which uses decision rules acquired from experts to advise physicians who request ...
In this case, researchers in the Collins Lab employed AI to speed up the process of finding antibiotic properties in known drug compounds. With millions of molecules, the process can take years, but researchers were able to identify a compound called semapimod over a weekend, thanks to AI's ability to perform high-throughput screening.
Artificial intelligence, or AI, is largely an experimental science--at least as much progress has been made by building and analyzing programs as by examining theoretical questions. MYCIN is one of several well-known pro-grams that embody some intelligence and provide data on the extent to which intelligent behavior can be programmed.
MYCIN was produced more than five or six years in the mid-1970s at Stanford University. It was composed in Lispas the doctoral paper of Edward Shortliffe under the heading of Bruce G. Buchanan, Stanley N. Cohen and others. It emerged in the lab that had made the before Dendral master framework. Fig. 1. Major parts of an expert system.
The first Expert System 'MYCIN' was developed at Stanford . . . ... EXPERT SYSTEM CASE STUDY cs 375 Artificial Intelligence Dr. J. Wunderlich . Dr. J. Wunderlich VhT . 1 #2) Develq) an expert system to help a custc*TEr deci& on a toy for a chi Id. (VP-EXPERT was used) ASSUMPTIONS: 1) is not relevant for chi Idren than the age of one. 2) Chi ...
A new study led by researchers at Stanford Medicine finds that computer algorithms powered by artificial intelligence based on deep learning can help health care practitioners to diagnose skin cancers more accurately. Even dermatologists benefit from AI guidance, although their improvement is less than that seen for non-dermatologists.
In this dynamic era of technological advancements, Artificial Intelligence (AI) emerges as a pivotal force, reshaping the way industries operate and charting new courses for business innovation. This article presents an in-depth exploration of 20 diverse and compelling AI case studies from across the globe.
Artificial intelligence in the medical has given rise to expert systems that can replace the role of experts (doctors). Tools to detect someone affected by COVID-19 have not been widely applied in ...
As artificial intelligence, or AI, takes off in the public sphere, what about medicine? The health care industry has been using some form of AI for decades, yet very recent advancements are upping the ante. This episode of Safety Net presents excerpts from a recent talk to malpractice attorneys by health care AI expert, Dr. Steven Horng, MD, MMSC, of Beth Israel Deaconess Medical Center and ...
Study uses artificial intelligence to show how personality influences the expression of our genes. ScienceDaily. Retrieved April 12, 2024 from www.sciencedaily.com / releases / 2024 / 04 ...
Artificial Intelligence 61 (1993) 209-261 209 Elsevier ARTINT 959 DENDRAL: a case study of the first expert system for scientific hypothesis formation* Robert K. Lindsay University of Michigan, 205 Zina Pitcher Place, Ann Arbor, MI 48109, USA Bruce G. Buchanan Computer Science Department, University of Pittsburgh, Pittsburgh, PA 15260, USA Edward A. Feigenbaum Knowledge Systems Laboratory ...
WASHINGTON, D.C.— Today, in a bipartisan letter to the Senate artificial intelligence (AI) working group leaders, U.S. Senators Angus King (I-ME), Co-Chair of the Cyberspace Solarium Commission, Mitt Romney (R-UT), Jack Reed (D-RI), Jerry Moran (R-KS) unveiled the first congressional framework to deal exclusively with the extreme risks posed by future developments in advanced AI models.
Artificial intelligence (AI) has emerged as a transformative technology across various fields, and its applications in the medical domain, particularly in ophthalmology, has gained significant attention. The vast amount of high-resolution image data, such as optical coherence tomography (OCT) images, has been a driving force behind AI growth in this field. Age-related macular degeneration (AMD ...