- En español – ExME
- Em português – EME
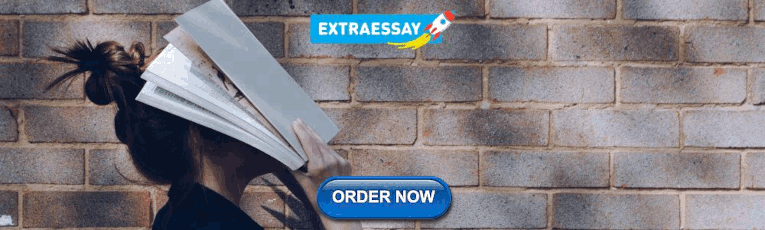
What are sampling methods and how do you choose the best one?
Posted on 18th November 2020 by Mohamed Khalifa
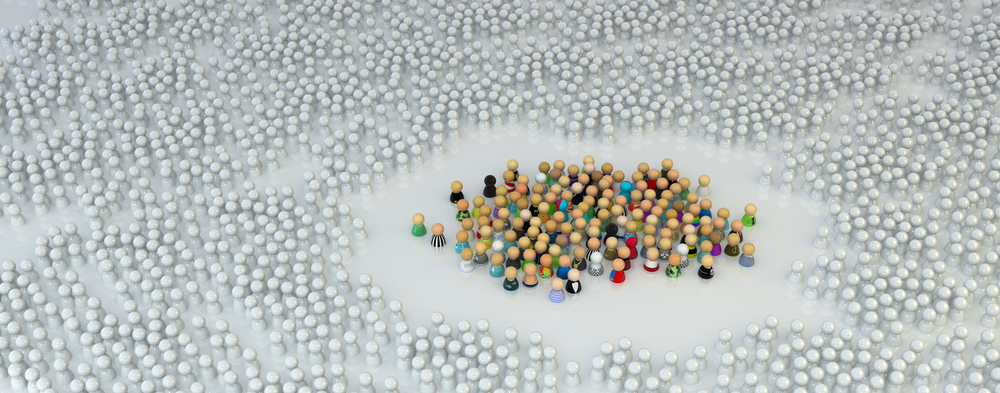
This tutorial will introduce sampling methods and potential sampling errors to avoid when conducting medical research.
Introduction to sampling methods
Examples of different sampling methods, choosing the best sampling method.
It is important to understand why we sample the population; for example, studies are built to investigate the relationships between risk factors and disease. In other words, we want to find out if this is a true association, while still aiming for the minimum risk for errors such as: chance, bias or confounding .
However, it would not be feasible to experiment on the whole population, we would need to take a good sample and aim to reduce the risk of having errors by proper sampling technique.
What is a sampling frame?
A sampling frame is a record of the target population containing all participants of interest. In other words, it is a list from which we can extract a sample.
What makes a good sample?
A good sample should be a representative subset of the population we are interested in studying, therefore, with each participant having equal chance of being randomly selected into the study.
We could choose a sampling method based on whether we want to account for sampling bias; a random sampling method is often preferred over a non-random method for this reason. Random sampling examples include: simple, systematic, stratified, and cluster sampling. Non-random sampling methods are liable to bias, and common examples include: convenience, purposive, snowballing, and quota sampling. For the purposes of this blog we will be focusing on random sampling methods .
Example: We want to conduct an experimental trial in a small population such as: employees in a company, or students in a college. We include everyone in a list and use a random number generator to select the participants
Advantages: Generalisable results possible, random sampling, the sampling frame is the whole population, every participant has an equal probability of being selected
Disadvantages: Less precise than stratified method, less representative than the systematic method
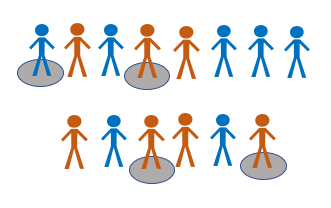
Example: Every nth patient entering the out-patient clinic is selected and included in our sample
Advantages: More feasible than simple or stratified methods, sampling frame is not always required
Disadvantages: Generalisability may decrease if baseline characteristics repeat across every nth participant
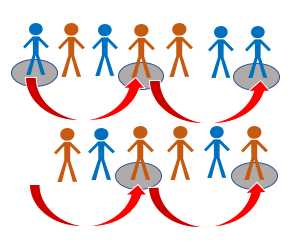
Example: We have a big population (a city) and we want to ensure representativeness of all groups with a pre-determined characteristic such as: age groups, ethnic origin, and gender
Advantages: Inclusive of strata (subgroups), reliable and generalisable results
Disadvantages: Does not work well with multiple variables
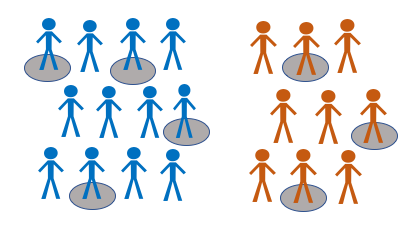
Example: 10 schools have the same number of students across the county. We can randomly select 3 out of 10 schools as our clusters
Advantages: Readily doable with most budgets, does not require a sampling frame
Disadvantages: Results may not be reliable nor generalisable
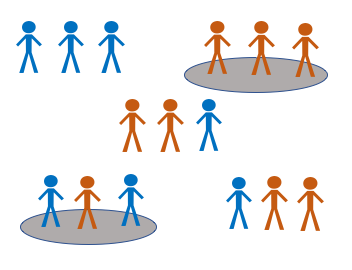
How can you identify sampling errors?
Non-random selection increases the probability of sampling (selection) bias if the sample does not represent the population we want to study. We could avoid this by random sampling and ensuring representativeness of our sample with regards to sample size.
An inadequate sample size decreases the confidence in our results as we may think there is no significant difference when actually there is. This type two error results from having a small sample size, or from participants dropping out of the sample.
In medical research of disease, if we select people with certain diseases while strictly excluding participants with other co-morbidities, we run the risk of diagnostic purity bias where important sub-groups of the population are not represented.
Furthermore, measurement bias may occur during re-collection of risk factors by participants (recall bias) or assessment of outcome where people who live longer are associated with treatment success, when in fact people who died were not included in the sample or data analysis (survivors bias).
By following the steps below we could choose the best sampling method for our study in an orderly fashion.
Research objectiveness
Firstly, a refined research question and goal would help us define our population of interest. If our calculated sample size is small then it would be easier to get a random sample. If, however, the sample size is large, then we should check if our budget and resources can handle a random sampling method.
Sampling frame availability
Secondly, we need to check for availability of a sampling frame (Simple), if not, could we make a list of our own (Stratified). If neither option is possible, we could still use other random sampling methods, for instance, systematic or cluster sampling.
Study design
Moreover, we could consider the prevalence of the topic (exposure or outcome) in the population, and what would be the suitable study design. In addition, checking if our target population is widely varied in its baseline characteristics. For example, a population with large ethnic subgroups could best be studied using a stratified sampling method.
Random sampling
Finally, the best sampling method is always the one that could best answer our research question while also allowing for others to make use of our results (generalisability of results). When we cannot afford a random sampling method, we can always choose from the non-random sampling methods.
To sum up, we now understand that choosing between random or non-random sampling methods is multifactorial. We might often be tempted to choose a convenience sample from the start, but that would not only decrease precision of our results, and would make us miss out on producing research that is more robust and reliable.
References (pdf)
Mohamed Khalifa
Leave a reply cancel reply.
Your email address will not be published. Required fields are marked *
Save my name, email, and website in this browser for the next time I comment.
No Comments on What are sampling methods and how do you choose the best one?
Thank you for this overview. A concise approach for research.
really helps! am an ecology student preparing to write my lab report for sampling.
I learned a lot to the given presentation.. It’s very comprehensive… Thanks for sharing…
Very informative and useful for my study. Thank you
Oversimplified info on sampling methods. Probabilistic of the sampling and sampling of samples by chance does rest solely on the random methods. Factors such as the random visits or presentation of the potential participants at clinics or sites could be sufficiently random in nature and should be used for the sake of efficiency and feasibility. Nevertheless, this approach has to be taken only after careful thoughts. Representativeness of the study samples have to be checked at the end or during reporting by comparing it to the published larger studies or register of some kind in/from the local population.
Thank you so much Mr.mohamed very useful and informative article
Subscribe to our newsletter
You will receive our monthly newsletter and free access to Trip Premium.
Related Articles
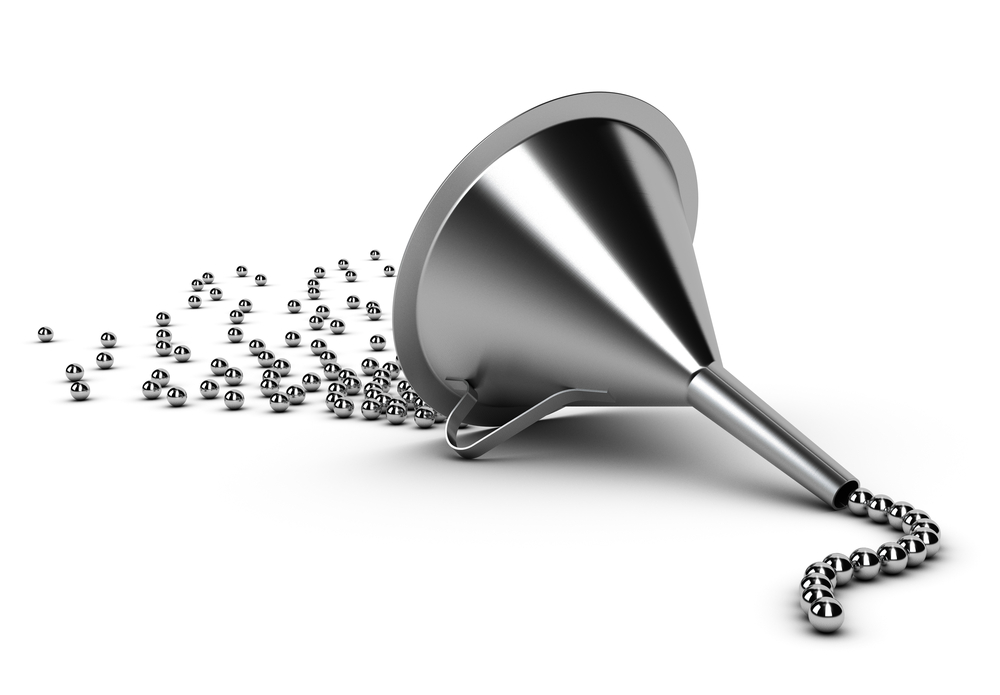
How to read a funnel plot
This blog introduces you to funnel plots, guiding you through how to read them and what may cause them to look asymmetrical.
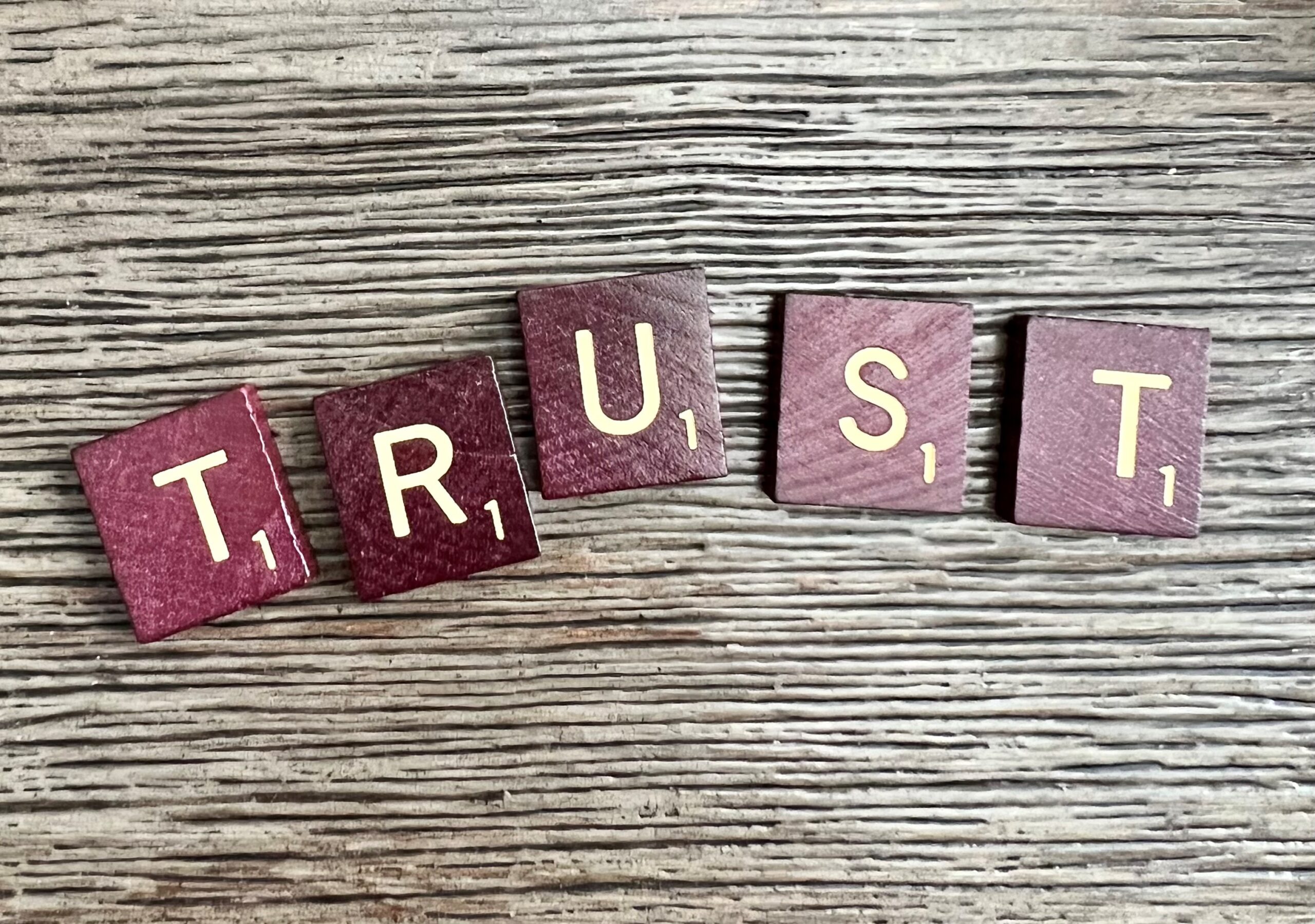
Internal and external validity: what are they and how do they differ?
Is this study valid? Can I trust this study’s methods and design? Can I apply the results of this study to other contexts? Learn more about internal and external validity in research to help you answer these questions when you next look at a paper.
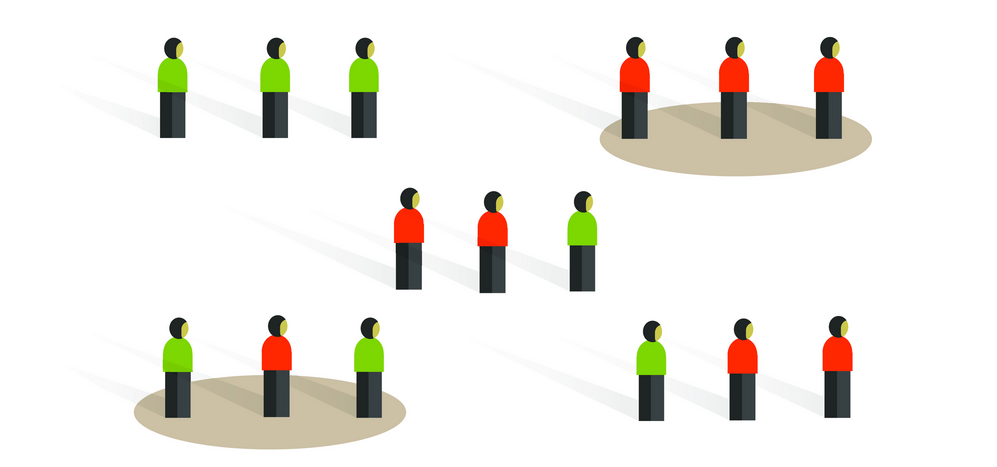
Cluster Randomized Trials: Concepts
This blog summarizes the concepts of cluster randomization, and the logistical and statistical considerations while designing a cluster randomized controlled trial.
- Privacy Policy

Home » Sampling Methods – Types, Techniques and Examples
Sampling Methods – Types, Techniques and Examples
Table of Contents

Sampling refers to the process of selecting a subset of data from a larger population or dataset in order to analyze or make inferences about the whole population.
In other words, sampling involves taking a representative sample of data from a larger group or dataset in order to gain insights or draw conclusions about the entire group.
Sampling Methods
Sampling methods refer to the techniques used to select a subset of individuals or units from a larger population for the purpose of conducting statistical analysis or research.
Sampling is an essential part of the Research because it allows researchers to draw conclusions about a population without having to collect data from every member of that population, which can be time-consuming, expensive, or even impossible.
Types of Sampling Methods
Sampling can be broadly categorized into two main categories:
Probability Sampling
This type of sampling is based on the principles of random selection, and it involves selecting samples in a way that every member of the population has an equal chance of being included in the sample.. Probability sampling is commonly used in scientific research and statistical analysis, as it provides a representative sample that can be generalized to the larger population.
Type of Probability Sampling :
- Simple Random Sampling: In this method, every member of the population has an equal chance of being selected for the sample. This can be done using a random number generator or by drawing names out of a hat, for example.
- Systematic Sampling: In this method, the population is first divided into a list or sequence, and then every nth member is selected for the sample. For example, if every 10th person is selected from a list of 100 people, the sample would include 10 people.
- Stratified Sampling: In this method, the population is divided into subgroups or strata based on certain characteristics, and then a random sample is taken from each stratum. This is often used to ensure that the sample is representative of the population as a whole.
- Cluster Sampling: In this method, the population is divided into clusters or groups, and then a random sample of clusters is selected. Then, all members of the selected clusters are included in the sample.
- Multi-Stage Sampling : This method combines two or more sampling techniques. For example, a researcher may use stratified sampling to select clusters, and then use simple random sampling to select members within each cluster.
Non-probability Sampling
This type of sampling does not rely on random selection, and it involves selecting samples in a way that does not give every member of the population an equal chance of being included in the sample. Non-probability sampling is often used in qualitative research, where the aim is not to generalize findings to a larger population, but to gain an in-depth understanding of a particular phenomenon or group. Non-probability sampling methods can be quicker and more cost-effective than probability sampling methods, but they may also be subject to bias and may not be representative of the larger population.
Types of Non-probability Sampling :
- Convenience Sampling: In this method, participants are chosen based on their availability or willingness to participate. This method is easy and convenient but may not be representative of the population.
- Purposive Sampling: In this method, participants are selected based on specific criteria, such as their expertise or knowledge on a particular topic. This method is often used in qualitative research, but may not be representative of the population.
- Snowball Sampling: In this method, participants are recruited through referrals from other participants. This method is often used when the population is hard to reach, but may not be representative of the population.
- Quota Sampling: In this method, a predetermined number of participants are selected based on specific criteria, such as age or gender. This method is often used in market research, but may not be representative of the population.
- Volunteer Sampling: In this method, participants volunteer to participate in the study. This method is often used in research where participants are motivated by personal interest or altruism, but may not be representative of the population.
Applications of Sampling Methods
Applications of Sampling Methods from different fields:
- Psychology : Sampling methods are used in psychology research to study various aspects of human behavior and mental processes. For example, researchers may use stratified sampling to select a sample of participants that is representative of the population based on factors such as age, gender, and ethnicity. Random sampling may also be used to select participants for experimental studies.
- Sociology : Sampling methods are commonly used in sociological research to study social phenomena and relationships between individuals and groups. For example, researchers may use cluster sampling to select a sample of neighborhoods to study the effects of economic inequality on health outcomes. Stratified sampling may also be used to select a sample of participants that is representative of the population based on factors such as income, education, and occupation.
- Social sciences: Sampling methods are commonly used in social sciences to study human behavior and attitudes. For example, researchers may use stratified sampling to select a sample of participants that is representative of the population based on factors such as age, gender, and income.
- Marketing : Sampling methods are used in marketing research to collect data on consumer preferences, behavior, and attitudes. For example, researchers may use random sampling to select a sample of consumers to participate in a survey about a new product.
- Healthcare : Sampling methods are used in healthcare research to study the prevalence of diseases and risk factors, and to evaluate interventions. For example, researchers may use cluster sampling to select a sample of health clinics to participate in a study of the effectiveness of a new treatment.
- Environmental science: Sampling methods are used in environmental science to collect data on environmental variables such as water quality, air pollution, and soil composition. For example, researchers may use systematic sampling to collect soil samples at regular intervals across a field.
- Education : Sampling methods are used in education research to study student learning and achievement. For example, researchers may use stratified sampling to select a sample of schools that is representative of the population based on factors such as demographics and academic performance.
Examples of Sampling Methods
Probability Sampling Methods Examples:
- Simple random sampling Example : A researcher randomly selects participants from the population using a random number generator or drawing names from a hat.
- Stratified random sampling Example : A researcher divides the population into subgroups (strata) based on a characteristic of interest (e.g. age or income) and then randomly selects participants from each subgroup.
- Systematic sampling Example : A researcher selects participants at regular intervals from a list of the population.
Non-probability Sampling Methods Examples:
- Convenience sampling Example: A researcher selects participants who are conveniently available, such as students in a particular class or visitors to a shopping mall.
- Purposive sampling Example : A researcher selects participants who meet specific criteria, such as individuals who have been diagnosed with a particular medical condition.
- Snowball sampling Example : A researcher selects participants who are referred to them by other participants, such as friends or acquaintances.
How to Conduct Sampling Methods
some general steps to conduct sampling methods:
- Define the population: Identify the population of interest and clearly define its boundaries.
- Choose the sampling method: Select an appropriate sampling method based on the research question, characteristics of the population, and available resources.
- Determine the sample size: Determine the desired sample size based on statistical considerations such as margin of error, confidence level, or power analysis.
- Create a sampling frame: Develop a list of all individuals or elements in the population from which the sample will be drawn. The sampling frame should be comprehensive, accurate, and up-to-date.
- Select the sample: Use the chosen sampling method to select the sample from the sampling frame. The sample should be selected randomly, or if using a non-random method, every effort should be made to minimize bias and ensure that the sample is representative of the population.
- Collect data: Once the sample has been selected, collect data from each member of the sample using appropriate research methods (e.g., surveys, interviews, observations).
- Analyze the data: Analyze the data collected from the sample to draw conclusions about the population of interest.
When to use Sampling Methods
Sampling methods are used in research when it is not feasible or practical to study the entire population of interest. Sampling allows researchers to study a smaller group of individuals, known as a sample, and use the findings from the sample to make inferences about the larger population.
Sampling methods are particularly useful when:
- The population of interest is too large to study in its entirety.
- The cost and time required to study the entire population are prohibitive.
- The population is geographically dispersed or difficult to access.
- The research question requires specialized or hard-to-find individuals.
- The data collected is quantitative and statistical analyses are used to draw conclusions.
Purpose of Sampling Methods
The main purpose of sampling methods in research is to obtain a representative sample of individuals or elements from a larger population of interest, in order to make inferences about the population as a whole. By studying a smaller group of individuals, known as a sample, researchers can gather information about the population that would be difficult or impossible to obtain from studying the entire population.
Sampling methods allow researchers to:
- Study a smaller, more manageable group of individuals, which is typically less time-consuming and less expensive than studying the entire population.
- Reduce the potential for data collection errors and improve the accuracy of the results by minimizing sampling bias.
- Make inferences about the larger population with a certain degree of confidence, using statistical analyses of the data collected from the sample.
- Improve the generalizability and external validity of the findings by ensuring that the sample is representative of the population of interest.
Characteristics of Sampling Methods
Here are some characteristics of sampling methods:
- Randomness : Probability sampling methods are based on random selection, meaning that every member of the population has an equal chance of being selected. This helps to minimize bias and ensure that the sample is representative of the population.
- Representativeness : The goal of sampling is to obtain a sample that is representative of the larger population of interest. This means that the sample should reflect the characteristics of the population in terms of key demographic, behavioral, or other relevant variables.
- Size : The size of the sample should be large enough to provide sufficient statistical power for the research question at hand. The sample size should also be appropriate for the chosen sampling method and the level of precision desired.
- Efficiency : Sampling methods should be efficient in terms of time, cost, and resources required. The method chosen should be feasible given the available resources and time constraints.
- Bias : Sampling methods should aim to minimize bias and ensure that the sample is representative of the population of interest. Bias can be introduced through non-random selection or non-response, and can affect the validity and generalizability of the findings.
- Precision : Sampling methods should be precise in terms of providing estimates of the population parameters of interest. Precision is influenced by sample size, sampling method, and level of variability in the population.
- Validity : The validity of the sampling method is important for ensuring that the results obtained from the sample are accurate and can be generalized to the population of interest. Validity can be affected by sampling method, sample size, and the representativeness of the sample.
Advantages of Sampling Methods
Sampling methods have several advantages, including:
- Cost-Effective : Sampling methods are often much cheaper and less time-consuming than studying an entire population. By studying only a small subset of the population, researchers can gather valuable data without incurring the costs associated with studying the entire population.
- Convenience : Sampling methods are often more convenient than studying an entire population. For example, if a researcher wants to study the eating habits of people in a city, it would be very difficult and time-consuming to study every single person in the city. By using sampling methods, the researcher can obtain data from a smaller subset of people, making the study more feasible.
- Accuracy: When done correctly, sampling methods can be very accurate. By using appropriate sampling techniques, researchers can obtain a sample that is representative of the entire population. This allows them to make accurate generalizations about the population as a whole based on the data collected from the sample.
- Time-Saving: Sampling methods can save a lot of time compared to studying the entire population. By studying a smaller sample, researchers can collect data much more quickly than they could if they studied every single person in the population.
- Less Bias : Sampling methods can reduce bias in a study. If a researcher were to study the entire population, it would be very difficult to eliminate all sources of bias. However, by using appropriate sampling techniques, researchers can reduce bias and obtain a sample that is more representative of the entire population.
Limitations of Sampling Methods
- Sampling Error : Sampling error is the difference between the sample statistic and the population parameter. It is the result of selecting a sample rather than the entire population. The larger the sample, the lower the sampling error. However, no matter how large the sample size, there will always be some degree of sampling error.
- Selection Bias: Selection bias occurs when the sample is not representative of the population. This can happen if the sample is not selected randomly or if some groups are underrepresented in the sample. Selection bias can lead to inaccurate conclusions about the population.
- Non-response Bias : Non-response bias occurs when some members of the sample do not respond to the survey or study. This can result in a biased sample if the non-respondents differ from the respondents in important ways.
- Time and Cost : While sampling can be cost-effective, it can still be expensive and time-consuming to select a sample that is representative of the population. Depending on the sampling method used, it may take a long time to obtain a sample that is large enough and representative enough to be useful.
- Limited Information : Sampling can only provide information about the variables that are measured. It may not provide information about other variables that are relevant to the research question but were not measured.
- Generalization : The extent to which the findings from a sample can be generalized to the population depends on the representativeness of the sample. If the sample is not representative of the population, it may not be possible to generalize the findings to the population as a whole.
About the author
Muhammad Hassan
Researcher, Academic Writer, Web developer
You may also like

Stratified Random Sampling – Definition, Method...

Non-probability Sampling – Types, Methods and...

Probability Sampling – Methods, Types and...

Cluster Sampling – Types, Method and Examples

Snowball Sampling – Method, Types and Examples

Purposive Sampling – Methods, Types and Examples
Educational resources and simple solutions for your research journey
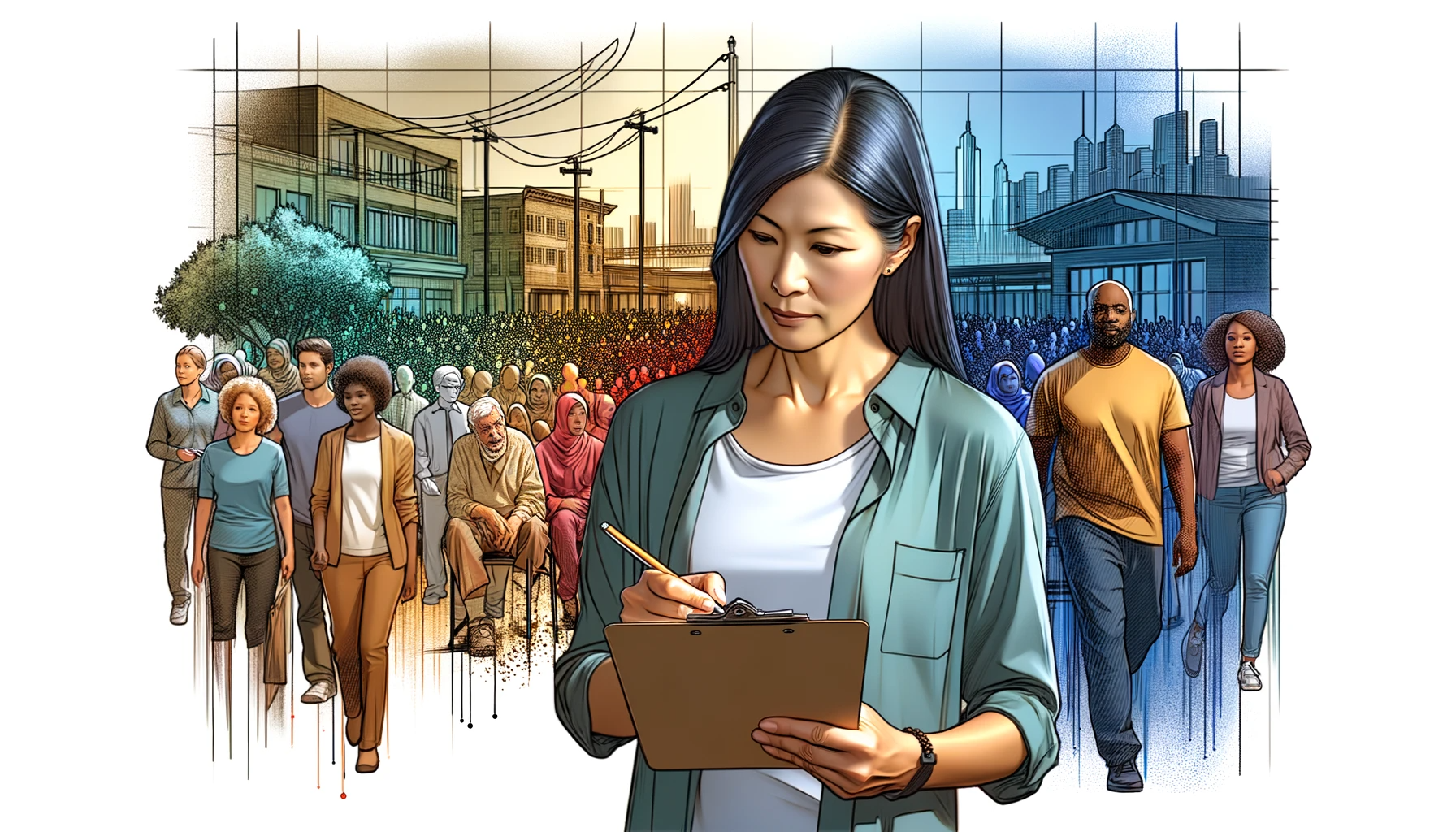
What are Sampling Methods? Techniques, Types, and Examples
Every type of research includes samples from which inferences are drawn. The sample could be biological specimens or a subset of a specific group or population selected for analysis. The goal is often to conclude the entire population based on the characteristics observed in the sample. Now, the question comes to mind: how does one collect the samples? Answer: Using sampling methods. Various sampling strategies are available to researchers to define and collect samples that will form the basis of their research study.
In a study focusing on individuals experiencing anxiety, gathering data from the entire population is practically impossible due to the widespread prevalence of anxiety. Consequently, a sample is carefully selected—a subset of individuals meant to represent (or not in some cases accurately) the demographics of those experiencing anxiety. The study’s outcomes hinge significantly on the chosen sample, emphasizing the critical importance of a thoughtful and precise selection process. The conclusions drawn about the broader population rely heavily on the selected sample’s characteristics and diversity.
Table of Contents
What is sampling?
Sampling involves the strategic selection of individuals or a subset from a population, aiming to derive statistical inferences and predict the characteristics of the entire population. It offers a pragmatic and practical approach to examining the features of the whole population, which would otherwise be difficult to achieve because studying the total population is expensive, time-consuming, and often impossible. Market researchers use various sampling methods to collect samples from a large population to acquire relevant insights. The best sampling strategy for research is determined by criteria such as the purpose of the study, available resources (time and money), and research hypothesis.
For example, if a pet food manufacturer wants to investigate the positive impact of a new cat food on feline growth, studying all the cats in the country is impractical. In such cases, employing an appropriate sampling technique from the extensive dataset allows the researcher to focus on a manageable subset. This enables the researcher to study the growth-promoting effects of the new pet food. This article will delve into the standard sampling methods and explore the situations in which each is most appropriately applied.
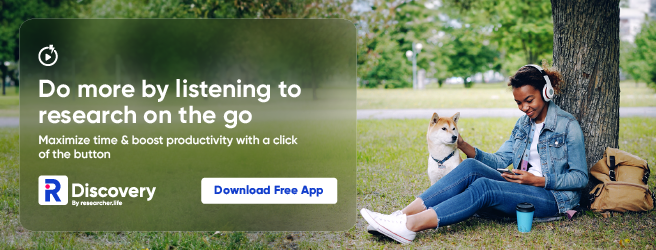
What are sampling methods or sampling techniques?
Sampling methods or sampling techniques in research are statistical methods for selecting a sample representative of the whole population to study the population’s characteristics. Sampling methods serve as invaluable tools for researchers, enabling the collection of meaningful data and facilitating analysis to identify distinctive features of the people. Different sampling strategies can be used based on the characteristics of the population, the study purpose, and the available resources. Now that we understand why sampling methods are essential in research, we review the various sample methods in the following sections.
Types of sampling methods
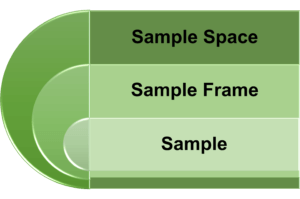
Before we go into the specifics of each sampling method, it’s vital to understand terms like sample, sample frame, and sample space. In probability theory, the sample space comprises all possible outcomes of a random experiment, while the sample frame is the list or source guiding sample selection in statistical research. The sample represents the group of individuals participating in the study, forming the basis for the research findings. Selecting the correct sample is critical to ensuring the validity and reliability of any research; the sample should be representative of the population.
There are two most common sampling methods:
- Probability sampling: A sampling method in which each unit or element in the population has an equal chance of being selected in the final sample. This is called random sampling, emphasizing the random and non-zero probability nature of selecting samples. Such a sampling technique ensures a more representative and unbiased sample, enabling robust inferences about the entire population.
- Non-probability sampling: Another sampling method is non-probability sampling, which involves collecting data conveniently through a non-random selection based on predefined criteria. This offers a straightforward way to gather data, although the resulting sample may or may not accurately represent the entire population.
Irrespective of the research method you opt for, it is essential to explicitly state the chosen sampling technique in the methodology section of your research article. Now, we will explore the different characteristics of both sampling methods, along with various subtypes falling under these categories.
What is probability sampling?
The probability sampling method is based on the probability theory, which means that the sample selection criteria involve some random selection. The probability sampling method provides an equal opportunity for all elements or units within the entire sample space to be chosen. While it can be labor-intensive and expensive, the advantage lies in its ability to offer a more accurate representation of the population, thereby enhancing confidence in the inferences drawn in the research.
Types of probability sampling
Various probability sampling methods exist, such as simple random sampling, systematic sampling, stratified sampling, and clustered sampling. Here, we provide detailed discussions and illustrative examples for each of these sampling methods:
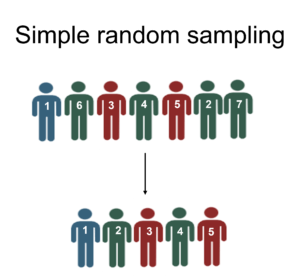
- Simple random sampling: In simple random sampling, each individual has an equal probability of being chosen, and each selection is independent of the others. Because the choice is entirely based on chance, this is also known as the method of chance selection. In the simple random sampling method, the sample frame comprises the entire population.
For example, A fitness sports brand is launching a new protein drink and aims to select 20 individuals from a 200-person fitness center to try it. Employing a simple random sampling approach, each of the 200 people is assigned a unique identifier. Of these, 20 individuals are then chosen by generating random numbers between 1 and 200, either manually or through a computer program. Matching these numbers with the individuals creates a randomly selected group of 20 people. This method minimizes sampling bias and ensures a representative subset of the entire population under study.
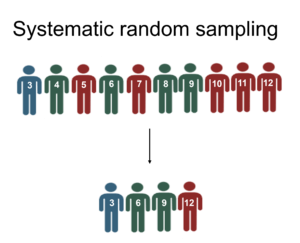
- Systematic sampling: The systematic sampling approach involves selecting units or elements at regular intervals from an ordered list of the population. Because the starting point of this sampling method is chosen at random, it is more convenient than essential random sampling. For a better understanding, consider the following example.
For example, considering the previous model, individuals at the fitness facility are arranged alphabetically. The manufacturer then initiates the process by randomly selecting a starting point from the first ten positions, let’s say 8. Starting from the 8th position, every tenth person on the list is then chosen (e.g., 8, 18, 28, 38, and so forth) until a sample of 20 individuals is obtained.
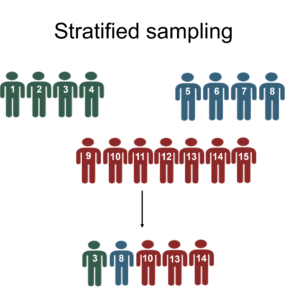
- Stratified sampling: Stratified sampling divides the population into subgroups (strata), and random samples are drawn from each stratum in proportion to its size in the population. Stratified sampling provides improved representation because each subgroup that differs in significant ways is included in the final sample.
For example, Expanding on the previous simple random sampling example, suppose the manufacturer aims for a more comprehensive representation of genders in a sample of 200 people, consisting of 90 males, 80 females, and 30 others. The manufacturer categorizes the population into three gender strata (Male, Female, and Others). Within each group, random sampling is employed to select nine males, eight females, and three individuals from the others category, resulting in a well-rounded and representative sample of 200 individuals.
- Clustered sampling: In this sampling method, the population is divided into clusters, and then a random sample of clusters is included in the final sample. Clustered sampling, distinct from stratified sampling, involves subgroups (clusters) that exhibit characteristics similar to the whole sample. In the case of small clusters, all members can be included in the final sample, whereas for larger clusters, individuals within each cluster may be sampled using the sampling above methods. This approach is referred to as multistage sampling. This sampling method is well-suited for large and widely distributed populations; however, there is a potential risk of sample error because ensuring that the sampled clusters truly represent the entire population can be challenging.
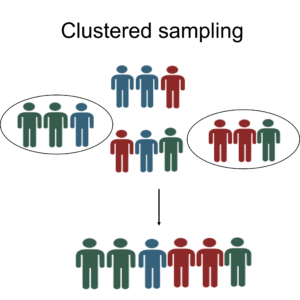
For example, Researchers conducting a nationwide health study can select specific geographic clusters, like cities or regions, instead of trying to survey the entire population individually. Within each chosen cluster, they sample individuals, providing a representative subset without the logistical challenges of attempting a nationwide survey.
Use s of probability sampling
Probability sampling methods find widespread use across diverse research disciplines because of their ability to yield representative and unbiased samples. The advantages of employing probability sampling include the following:
- Representativeness
Probability sampling assures that every element in the population has a non-zero chance of being included in the sample, ensuring representativeness of the entire population and decreasing research bias to minimal to non-existent levels. The researcher can acquire higher-quality data via probability sampling, increasing confidence in the conclusions.
- Statistical inference
Statistical methods, like confidence intervals and hypothesis testing, depend on probability sampling to generalize findings from a sample to the broader population. Probability sampling methods ensure unbiased representation, allowing inferences about the population based on the characteristics of the sample.
- Precision and reliability
The use of probability sampling improves the precision and reliability of study results. Because the probability of selecting any single element/individual is known, the chance variations that may occur in non-probability sampling methods are reduced, resulting in more dependable and precise estimations.
- Generalizability
Probability sampling enables the researcher to generalize study findings to the entire population from which they were derived. The results produced through probability sampling methods are more likely to be applicable to the larger population, laying the foundation for making broad predictions or recommendations.
- Minimization of Selection Bias
By ensuring that each member of the population has an equal chance of being selected in the sample, probability sampling lowers the possibility of selection bias. This reduces the impact of systematic errors that may occur in non-probability sampling methods, where data may be skewed toward a specific demographic due to inadequate representation of each segment of the population.
What is non-probability sampling?
Non-probability sampling methods involve selecting individuals based on non-random criteria, often relying on the researcher’s judgment or predefined criteria. While it is easier and more economical, it tends to introduce sampling bias, resulting in weaker inferences compared to probability sampling techniques in research.
Types of Non-probability Sampling
Non-probability sampling methods are further classified as convenience sampling, consecutive sampling, quota sampling, purposive or judgmental sampling, and snowball sampling. Let’s explore these types of sampling methods in detail.
- Convenience sampling: In convenience sampling, individuals are recruited directly from the population based on the accessibility and proximity to the researcher. It is a simple, inexpensive, and practical method of sample selection, yet convenience sampling suffers from both sampling and selection bias due to a lack of appropriate population representation.
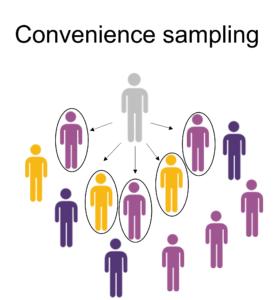
For example, imagine you’re a researcher investigating smartphone usage patterns in your city. The most convenient way to select participants is by approaching people in a shopping mall on a weekday afternoon. However, this convenience sampling method may not be an accurate representation of the city’s overall smartphone usage patterns as the sample is limited to individuals present at the mall during weekdays, excluding those who visit on other days or never visit the mall.
- Consecutive sampling: Participants in consecutive sampling (or sequential sampling) are chosen based on their availability and desire to participate in the study as they become available. This strategy entails sequentially recruiting individuals who fulfill the researcher’s requirements.
For example, In researching the prevalence of stroke in a hospital, instead of randomly selecting patients from the entire population, the researcher can opt to include all eligible patients admitted over three months. Participants are then consecutively recruited upon admission during that timeframe, forming the study sample.
- Quota sampling: The selection of individuals in quota sampling is based on non-random selection criteria in which only participants with certain traits or proportions that are representative of the population are included. Quota sampling involves setting predetermined quotas for specific subgroups based on key demographics or other relevant characteristics. This sampling method employs dividing the population into mutually exclusive subgroups and then selecting sample units until the set quota is reached.
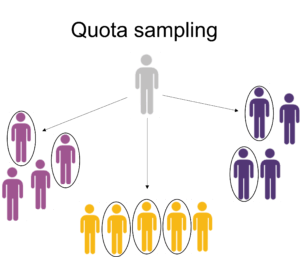
For example, In a survey on a college campus to assess student interest in a new policy, the researcher should establish quotas aligned with the distribution of student majors, ensuring representation from various academic disciplines. If the campus has 20% biology majors, 30% engineering majors, 20% business majors, and 30% liberal arts majors, participants should be recruited to mirror these proportions.
- Purposive or judgmental sampling: In purposive sampling, the researcher leverages expertise to select a sample relevant to the study’s specific questions. This sampling method is commonly applied in qualitative research, mainly when aiming to understand a particular phenomenon, and is suitable for smaller population sizes.
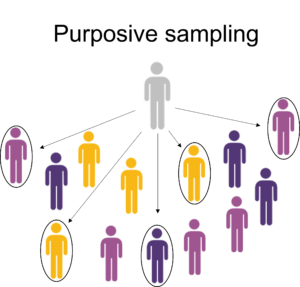
For example, imagine a researcher who wants to study public policy issues for a focus group. The researcher might purposely select participants with expertise in economics, law, and public administration to take advantage of their knowledge and ensure a depth of understanding.
- Snowball sampling: This sampling method is used when accessing the population is challenging. It involves collecting the sample through a chain-referral process, where each recruited candidate aids in finding others. These candidates share common traits, representing the targeted population. This method is often used in qualitative research, particularly when studying phenomena related to stigmatized or hidden populations.

For example, In a study focusing on understanding the experiences and challenges of individuals in hidden or stigmatized communities (e.g., LGBTQ+ individuals in specific cultural contexts), the snowball sampling technique can be employed. The researcher initiates contact with one community member, who then assists in identifying additional candidates until the desired sample size is achieved.
Uses of non-probability sampling
Non-probability sampling approaches are employed in qualitative or exploratory research where the goal is to investigate underlying population traits rather than generalizability. Non-probability sampling methods are also helpful for the following purposes:
- Generating a hypothesis
In the initial stages of exploratory research, non-probability methods such as purposive or convenience allow researchers to quickly gather information and generate hypothesis that helps build a future research plan.
- Qualitative research
Qualitative research is usually focused on understanding the depth and complexity of human experiences, behaviors, and perspectives. Non-probability methods like purposive or snowball sampling are commonly used to select participants with specific traits that are relevant to the research question.
- Convenience and pragmatism
Non-probability sampling methods are valuable when resource and time are limited or when preliminary data is required to test the pilot study. For example, conducting a survey at a local shopping mall to gather opinions on a consumer product due to the ease of access to potential participants.
Probability vs Non-probability Sampling Methods
Selection of participants | Random selection of participants from the population using randomization methods | Non-random selection of participants from the population based on convenience or criteria |
Representativeness | Likely to yield a representative sample of the whole population allowing for generalizations | May not yield a representative sample of the whole population; poor generalizability |
Precision and accuracy | Provides more precise and accurate estimates of population characteristics | May have less precision and accuracy due to non-random selection |
Bias | Minimizes selection bias | May introduce selection bias if criteria are subjective and not well-defined |
Statistical inference | Suited for statistical inference and hypothesis testing and for making generalization to the population | Less suited for statistical inference and hypothesis testing on the population |
Application | Useful for quantitative research where generalizability is crucial | Commonly used in qualitative and exploratory research where in-depth insights are the goal |
Frequently asked questions
- What is multistage sampling ? Multistage sampling is a form of probability sampling approach that involves the progressive selection of samples in stages, going from larger clusters to a small number of participants, making it suited for large-scale research with enormous population lists.
- What are the methods of probability sampling? Probability sampling methods are simple random sampling, stratified random sampling, systematic sampling, cluster sampling, and multistage sampling.
- How to decide which type of sampling method to use? Choose a sampling method based on the goals, population, and resources. Probability for statistics and non-probability for efficiency or qualitative insights can be considered . Also, consider the population characteristics, size, and alignment with study objectives.
- What are the methods of non-probability sampling? Non-probability sampling methods are convenience sampling, consecutive sampling, purposive sampling, snowball sampling, and quota sampling.
- Why are sampling methods used in research? Sampling methods in research are employed to efficiently gather representative data from a subset of a larger population, enabling valid conclusions and generalizations while minimizing costs and time.
R Discovery is a literature search and research reading platform that accelerates your research discovery journey by keeping you updated on the latest, most relevant scholarly content. With 250M+ research articles sourced from trusted aggregators like CrossRef, Unpaywall, PubMed, PubMed Central, Open Alex and top publishing houses like Springer Nature, JAMA, IOP, Taylor & Francis, NEJM, BMJ, Karger, SAGE, Emerald Publishing and more, R Discovery puts a world of research at your fingertips.
Try R Discovery Prime FREE for 1 week or upgrade at just US$72 a year to access premium features that let you listen to research on the go, read in your language, collaborate with peers, auto sync with reference managers, and much more. Choose a simpler, smarter way to find and read research – Download the app and start your free 7-day trial today !
Related Posts

How Publishers Can Enhance Reader Engagement with R Discovery’s Article Recommendation System

What is Research? Definition, Types, Methods, and Examples

Sampling Methods & Strategies 101
Everything you need to know (including examples)
By: Derek Jansen (MBA) | Expert Reviewed By: Kerryn Warren (PhD) | January 2023
If you’re new to research, sooner or later you’re bound to wander into the intimidating world of sampling methods and strategies. If you find yourself on this page, chances are you’re feeling a little overwhelmed or confused. Fear not – in this post we’ll unpack sampling in straightforward language , along with loads of examples .
Overview: Sampling Methods & Strategies
- What is sampling in a research context?
- The two overarching approaches
Simple random sampling
Stratified random sampling, cluster sampling, systematic sampling, purposive sampling, convenience sampling, snowball sampling.
- How to choose the right sampling method
What (exactly) is sampling?
At the simplest level, sampling (within a research context) is the process of selecting a subset of participants from a larger group . For example, if your research involved assessing US consumers’ perceptions about a particular brand of laundry detergent, you wouldn’t be able to collect data from every single person that uses laundry detergent (good luck with that!) – but you could potentially collect data from a smaller subset of this group.
In technical terms, the larger group is referred to as the population , and the subset (the group you’ll actually engage with in your research) is called the sample . Put another way, you can look at the population as a full cake and the sample as a single slice of that cake. In an ideal world, you’d want your sample to be perfectly representative of the population, as that would allow you to generalise your findings to the entire population. In other words, you’d want to cut a perfect cross-sectional slice of cake, such that the slice reflects every layer of the cake in perfect proportion.
Achieving a truly representative sample is, unfortunately, a little trickier than slicing a cake, as there are many practical challenges and obstacles to achieving this in a real-world setting. Thankfully though, you don’t always need to have a perfectly representative sample – it all depends on the specific research aims of each study – so don’t stress yourself out about that just yet!
With the concept of sampling broadly defined, let’s look at the different approaches to sampling to get a better understanding of what it all looks like in practice.
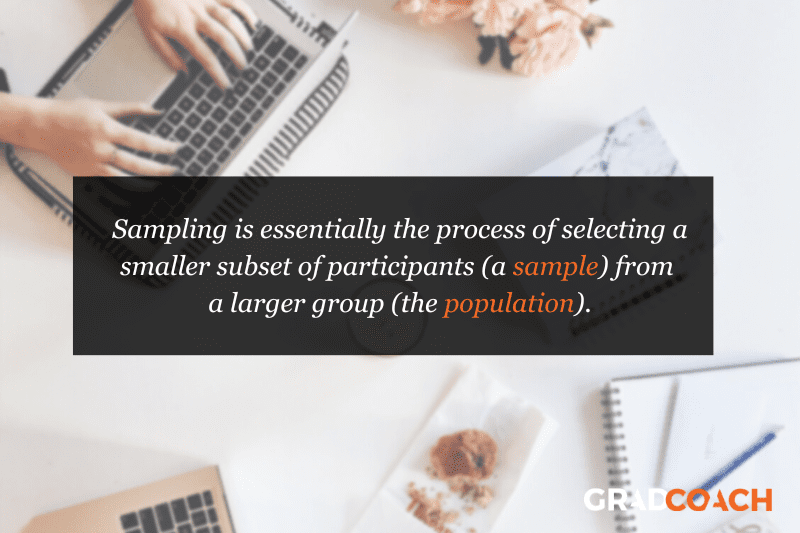
The two overarching sampling approaches
At the highest level, there are two approaches to sampling: probability sampling and non-probability sampling . Within each of these, there are a variety of sampling methods , which we’ll explore a little later.
Probability sampling involves selecting participants (or any unit of interest) on a statistically random basis , which is why it’s also called “random sampling”. In other words, the selection of each individual participant is based on a pre-determined process (not the discretion of the researcher). As a result, this approach achieves a random sample.
Probability-based sampling methods are most commonly used in quantitative research , especially when it’s important to achieve a representative sample that allows the researcher to generalise their findings.
Non-probability sampling , on the other hand, refers to sampling methods in which the selection of participants is not statistically random . In other words, the selection of individual participants is based on the discretion and judgment of the researcher, rather than on a pre-determined process.
Non-probability sampling methods are commonly used in qualitative research , where the richness and depth of the data are more important than the generalisability of the findings.
If that all sounds a little too conceptual and fluffy, don’t worry. Let’s take a look at some actual sampling methods to make it more tangible.
Need a helping hand?
Probability-based sampling methods
First, we’ll look at four common probability-based (random) sampling methods:
Importantly, this is not a comprehensive list of all the probability sampling methods – these are just four of the most common ones. So, if you’re interested in adopting a probability-based sampling approach, be sure to explore all the options.
Simple random sampling involves selecting participants in a completely random fashion , where each participant has an equal chance of being selected. Basically, this sampling method is the equivalent of pulling names out of a hat , except that you can do it digitally. For example, if you had a list of 500 people, you could use a random number generator to draw a list of 50 numbers (each number, reflecting a participant) and then use that dataset as your sample.
Thanks to its simplicity, simple random sampling is easy to implement , and as a consequence, is typically quite cheap and efficient . Given that the selection process is completely random, the results can be generalised fairly reliably. However, this also means it can hide the impact of large subgroups within the data, which can result in minority subgroups having little representation in the results – if any at all. To address this, one needs to take a slightly different approach, which we’ll look at next.
Stratified random sampling is similar to simple random sampling, but it kicks things up a notch. As the name suggests, stratified sampling involves selecting participants randomly , but from within certain pre-defined subgroups (i.e., strata) that share a common trait . For example, you might divide the population into strata based on gender, ethnicity, age range or level of education, and then select randomly from each group.
The benefit of this sampling method is that it gives you more control over the impact of large subgroups (strata) within the population. For example, if a population comprises 80% males and 20% females, you may want to “balance” this skew out by selecting a random sample from an equal number of males and females. This would, of course, reduce the representativeness of the sample, but it would allow you to identify differences between subgroups. So, depending on your research aims, the stratified approach could work well.
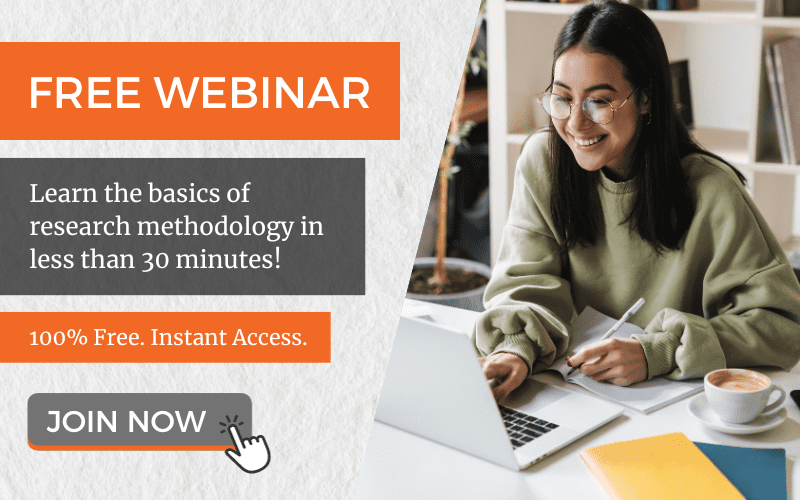
Next on the list is cluster sampling. As the name suggests, this sampling method involves sampling from naturally occurring, mutually exclusive clusters within a population – for example, area codes within a city or cities within a country. Once the clusters are defined, a set of clusters are randomly selected and then a set of participants are randomly selected from each cluster.
Now, you’re probably wondering, “how is cluster sampling different from stratified random sampling?”. Well, let’s look at the previous example where each cluster reflects an area code in a given city.
With cluster sampling, you would collect data from clusters of participants in a handful of area codes (let’s say 5 neighbourhoods). Conversely, with stratified random sampling, you would need to collect data from all over the city (i.e., many more neighbourhoods). You’d still achieve the same sample size either way (let’s say 200 people, for example), but with stratified sampling, you’d need to do a lot more running around, as participants would be scattered across a vast geographic area. As a result, cluster sampling is often the more practical and economical option.
If that all sounds a little mind-bending, you can use the following general rule of thumb. If a population is relatively homogeneous , cluster sampling will often be adequate. Conversely, if a population is quite heterogeneous (i.e., diverse), stratified sampling will generally be more appropriate.
The last probability sampling method we’ll look at is systematic sampling. This method simply involves selecting participants at a set interval , starting from a random point .
For example, if you have a list of students that reflects the population of a university, you could systematically sample that population by selecting participants at an interval of 8 . In other words, you would randomly select a starting point – let’s say student number 40 – followed by student 48, 56, 64, etc.
What’s important with systematic sampling is that the population list you select from needs to be randomly ordered . If there are underlying patterns in the list (for example, if the list is ordered by gender, IQ, age, etc.), this will result in a non-random sample, which would defeat the purpose of adopting this sampling method. Of course, you could safeguard against this by “shuffling” your population list using a random number generator or similar tool.
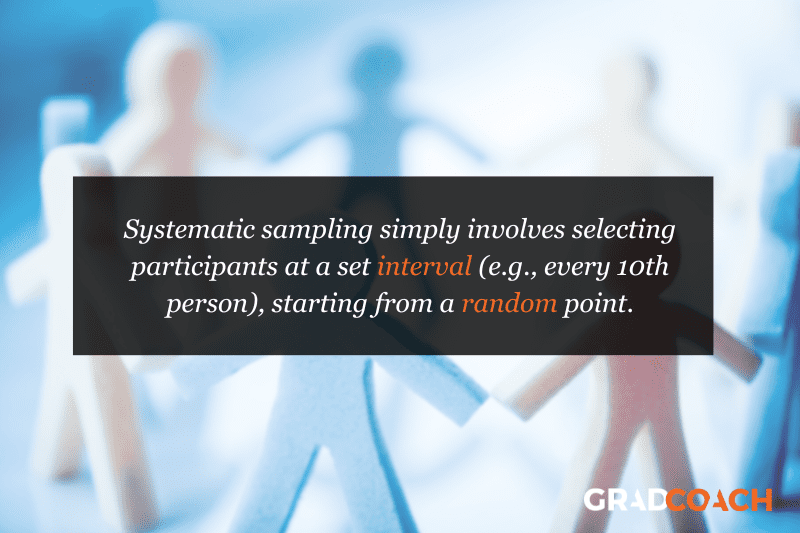
Non-probability-based sampling methods
Right, now that we’ve looked at a few probability-based sampling methods, let’s look at three non-probability methods :
Again, this is not an exhaustive list of all possible sampling methods, so be sure to explore further if you’re interested in adopting a non-probability sampling approach.
First up, we’ve got purposive sampling – also known as judgment , selective or subjective sampling. Again, the name provides some clues, as this method involves the researcher selecting participants using his or her own judgement , based on the purpose of the study (i.e., the research aims).
For example, suppose your research aims were to understand the perceptions of hyper-loyal customers of a particular retail store. In that case, you could use your judgement to engage with frequent shoppers, as well as rare or occasional shoppers, to understand what judgements drive the two behavioural extremes .
Purposive sampling is often used in studies where the aim is to gather information from a small population (especially rare or hard-to-find populations), as it allows the researcher to target specific individuals who have unique knowledge or experience . Naturally, this sampling method is quite prone to researcher bias and judgement error, and it’s unlikely to produce generalisable results, so it’s best suited to studies where the aim is to go deep rather than broad .
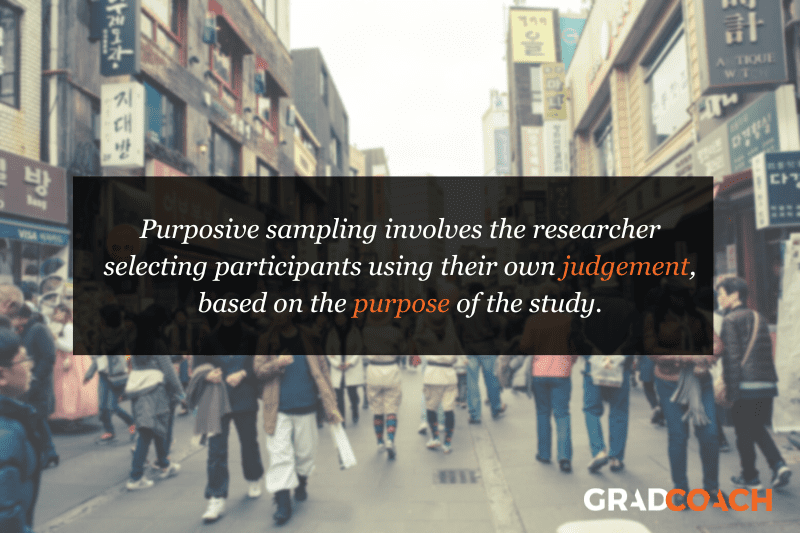
Next up, we have convenience sampling. As the name suggests, with this method, participants are selected based on their availability or accessibility . In other words, the sample is selected based on how convenient it is for the researcher to access it, as opposed to using a defined and objective process.
Naturally, convenience sampling provides a quick and easy way to gather data, as the sample is selected based on the individuals who are readily available or willing to participate. This makes it an attractive option if you’re particularly tight on resources and/or time. However, as you’d expect, this sampling method is unlikely to produce a representative sample and will of course be vulnerable to researcher bias , so it’s important to approach it with caution.
Last but not least, we have the snowball sampling method. This method relies on referrals from initial participants to recruit additional participants. In other words, the initial subjects form the first (small) snowball and each additional subject recruited through referral is added to the snowball, making it larger as it rolls along .
Snowball sampling is often used in research contexts where it’s difficult to identify and access a particular population. For example, people with a rare medical condition or members of an exclusive group. It can also be useful in cases where the research topic is sensitive or taboo and people are unlikely to open up unless they’re referred by someone they trust.
Simply put, snowball sampling is ideal for research that involves reaching hard-to-access populations . But, keep in mind that, once again, it’s a sampling method that’s highly prone to researcher bias and is unlikely to produce a representative sample. So, make sure that it aligns with your research aims and questions before adopting this method.
How to choose a sampling method
Now that we’ve looked at a few popular sampling methods (both probability and non-probability based), the obvious question is, “ how do I choose the right sampling method for my study?”. When selecting a sampling method for your research project, you’ll need to consider two important factors: your research aims and your resources .
As with all research design and methodology choices, your sampling approach needs to be guided by and aligned with your research aims, objectives and research questions – in other words, your golden thread. Specifically, you need to consider whether your research aims are primarily concerned with producing generalisable findings (in which case, you’ll likely opt for a probability-based sampling method) or with achieving rich , deep insights (in which case, a non-probability-based approach could be more practical). Typically, quantitative studies lean toward the former, while qualitative studies aim for the latter, so be sure to consider your broader methodology as well.
The second factor you need to consider is your resources and, more generally, the practical constraints at play. If, for example, you have easy, free access to a large sample at your workplace or university and a healthy budget to help you attract participants, that will open up multiple options in terms of sampling methods. Conversely, if you’re cash-strapped, short on time and don’t have unfettered access to your population of interest, you may be restricted to convenience or referral-based methods.
In short, be ready for trade-offs – you won’t always be able to utilise the “perfect” sampling method for your study, and that’s okay. Much like all the other methodological choices you’ll make as part of your study, you’ll often need to compromise and accept practical trade-offs when it comes to sampling. Don’t let this get you down though – as long as your sampling choice is well explained and justified, and the limitations of your approach are clearly articulated, you’ll be on the right track.
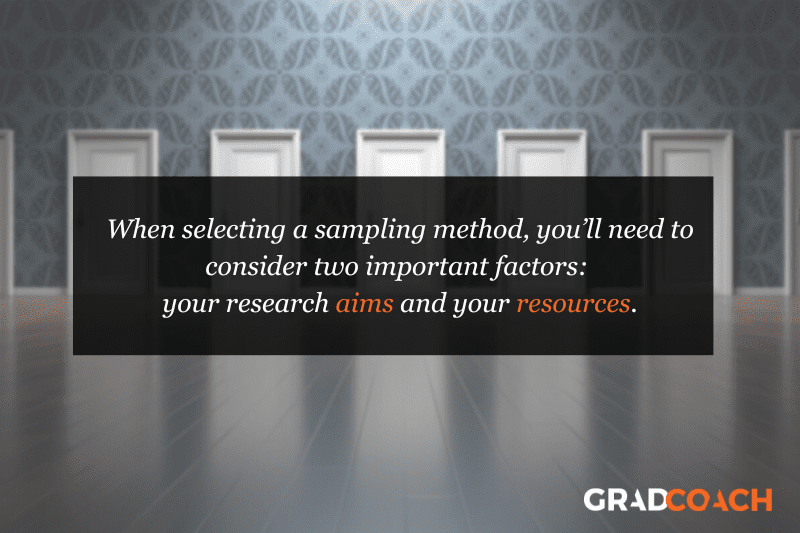
Let’s recap…
In this post, we’ve covered the basics of sampling within the context of a typical research project.
- Sampling refers to the process of defining a subgroup (sample) from the larger group of interest (population).
- The two overarching approaches to sampling are probability sampling (random) and non-probability sampling .
- Common probability-based sampling methods include simple random sampling, stratified random sampling, cluster sampling and systematic sampling.
- Common non-probability-based sampling methods include purposive sampling, convenience sampling and snowball sampling.
- When choosing a sampling method, you need to consider your research aims , objectives and questions, as well as your resources and other practical constraints .
If you’d like to see an example of a sampling strategy in action, be sure to check out our research methodology chapter sample .
Last but not least, if you need hands-on help with your sampling (or any other aspect of your research), take a look at our 1-on-1 coaching service , where we guide you through each step of the research process, at your own pace.
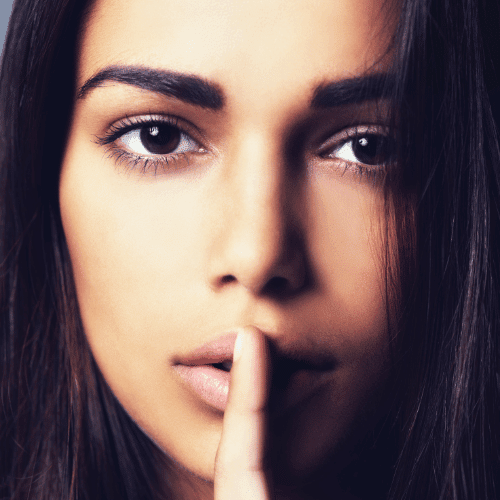
Psst... there’s more!
This post was based on one of our popular Research Bootcamps . If you're working on a research project, you'll definitely want to check this out ...
You Might Also Like:
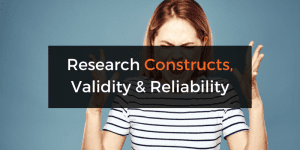
Excellent and helpful. Best site to get a full understanding of Research methodology. I’m nolonger as “clueless “..😉
Excellent and helpful for junior researcher!
Grad Coach tutorials are excellent – I recommend them to everyone doing research. I will be working with a sample of imprisoned women and now have a much clearer idea concerning sampling. Thank you to all at Grad Coach for generously sharing your expertise with students.
Submit a Comment Cancel reply
Your email address will not be published. Required fields are marked *
Save my name, email, and website in this browser for the next time I comment.
- Print Friendly
Have a language expert improve your writing
Run a free plagiarism check in 10 minutes, automatically generate references for free.
- Knowledge Base
- Methodology
- Sampling Methods | Types, Techniques, & Examples
Sampling Methods | Types, Techniques, & Examples
Published on 3 May 2022 by Shona McCombes . Revised on 10 October 2022.
When you conduct research about a group of people, it’s rarely possible to collect data from every person in that group. Instead, you select a sample. The sample is the group of individuals who will actually participate in the research.
To draw valid conclusions from your results, you have to carefully decide how you will select a sample that is representative of the group as a whole. There are two types of sampling methods:
- Probability sampling involves random selection, allowing you to make strong statistical inferences about the whole group. It minimises the risk of selection bias .
- Non-probability sampling involves non-random selection based on convenience or other criteria, allowing you to easily collect data.
You should clearly explain how you selected your sample in the methodology section of your paper or thesis.
Table of contents
Population vs sample, probability sampling methods, non-probability sampling methods, frequently asked questions about sampling.
First, you need to understand the difference between a population and a sample , and identify the target population of your research.
- The population is the entire group that you want to draw conclusions about.
- The sample is the specific group of individuals that you will collect data from.
The population can be defined in terms of geographical location, age, income, and many other characteristics.
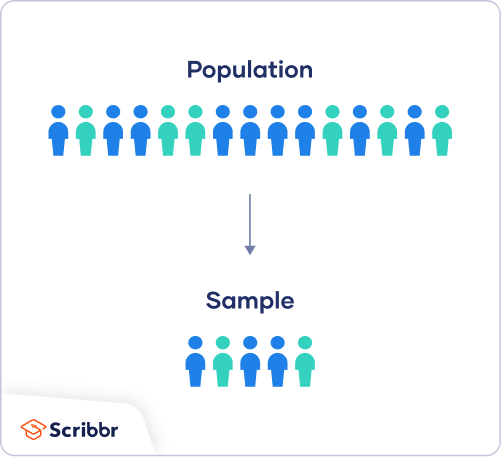
It is important to carefully define your target population according to the purpose and practicalities of your project.
If the population is very large, demographically mixed, and geographically dispersed, it might be difficult to gain access to a representative sample.
Sampling frame
The sampling frame is the actual list of individuals that the sample will be drawn from. Ideally, it should include the entire target population (and nobody who is not part of that population).
You are doing research on working conditions at Company X. Your population is all 1,000 employees of the company. Your sampling frame is the company’s HR database, which lists the names and contact details of every employee.
Sample size
The number of individuals you should include in your sample depends on various factors, including the size and variability of the population and your research design. There are different sample size calculators and formulas depending on what you want to achieve with statistical analysis .
Prevent plagiarism, run a free check.
Probability sampling means that every member of the population has a chance of being selected. It is mainly used in quantitative research . If you want to produce results that are representative of the whole population, probability sampling techniques are the most valid choice.
There are four main types of probability sample.
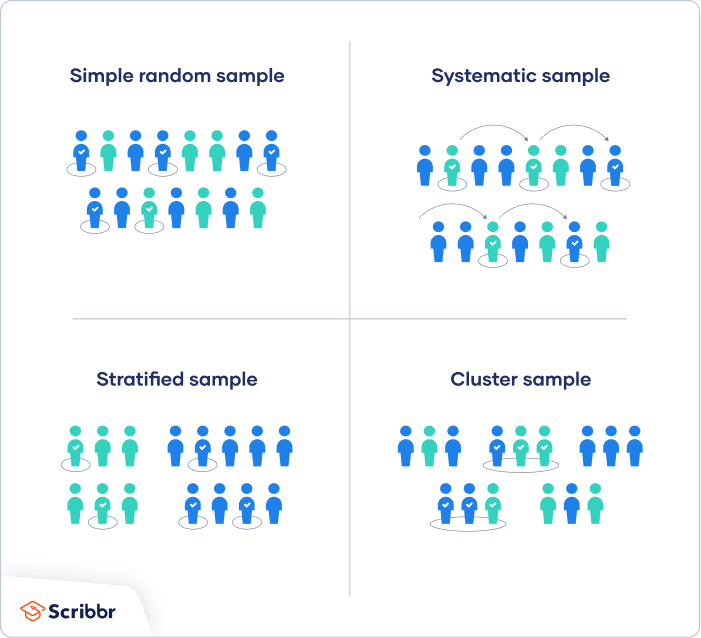
1. Simple random sampling
In a simple random sample , every member of the population has an equal chance of being selected. Your sampling frame should include the whole population.
To conduct this type of sampling, you can use tools like random number generators or other techniques that are based entirely on chance.
You want to select a simple random sample of 100 employees of Company X. You assign a number to every employee in the company database from 1 to 1000, and use a random number generator to select 100 numbers.
2. Systematic sampling
Systematic sampling is similar to simple random sampling, but it is usually slightly easier to conduct. Every member of the population is listed with a number, but instead of randomly generating numbers, individuals are chosen at regular intervals.
All employees of the company are listed in alphabetical order. From the first 10 numbers, you randomly select a starting point: number 6. From number 6 onwards, every 10th person on the list is selected (6, 16, 26, 36, and so on), and you end up with a sample of 100 people.
If you use this technique, it is important to make sure that there is no hidden pattern in the list that might skew the sample. For example, if the HR database groups employees by team, and team members are listed in order of seniority, there is a risk that your interval might skip over people in junior roles, resulting in a sample that is skewed towards senior employees.
3. Stratified sampling
Stratified sampling involves dividing the population into subpopulations that may differ in important ways. It allows you draw more precise conclusions by ensuring that every subgroup is properly represented in the sample.
To use this sampling method, you divide the population into subgroups (called strata) based on the relevant characteristic (e.g., gender, age range, income bracket, job role).
Based on the overall proportions of the population, you calculate how many people should be sampled from each subgroup. Then you use random or systematic sampling to select a sample from each subgroup.
The company has 800 female employees and 200 male employees. You want to ensure that the sample reflects the gender balance of the company, so you sort the population into two strata based on gender. Then you use random sampling on each group, selecting 80 women and 20 men, which gives you a representative sample of 100 people.
4. Cluster sampling
Cluster sampling also involves dividing the population into subgroups, but each subgroup should have similar characteristics to the whole sample. Instead of sampling individuals from each subgroup, you randomly select entire subgroups.
If it is practically possible, you might include every individual from each sampled cluster. If the clusters themselves are large, you can also sample individuals from within each cluster using one of the techniques above. This is called multistage sampling .
This method is good for dealing with large and dispersed populations, but there is more risk of error in the sample, as there could be substantial differences between clusters. It’s difficult to guarantee that the sampled clusters are really representative of the whole population.
The company has offices in 10 cities across the country (all with roughly the same number of employees in similar roles). You don’t have the capacity to travel to every office to collect your data, so you use random sampling to select 3 offices – these are your clusters.
In a non-probability sample , individuals are selected based on non-random criteria, and not every individual has a chance of being included.
This type of sample is easier and cheaper to access, but it has a higher risk of sampling bias . That means the inferences you can make about the population are weaker than with probability samples, and your conclusions may be more limited. If you use a non-probability sample, you should still aim to make it as representative of the population as possible.
Non-probability sampling techniques are often used in exploratory and qualitative research . In these types of research, the aim is not to test a hypothesis about a broad population, but to develop an initial understanding of a small or under-researched population.
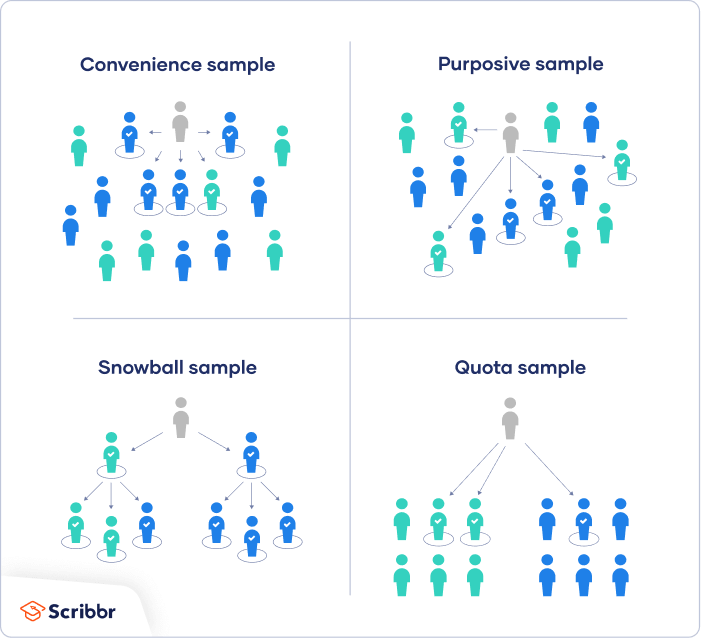
1. Convenience sampling
A convenience sample simply includes the individuals who happen to be most accessible to the researcher.
This is an easy and inexpensive way to gather initial data, but there is no way to tell if the sample is representative of the population, so it can’t produce generalisable results.
You are researching opinions about student support services in your university, so after each of your classes, you ask your fellow students to complete a survey on the topic. This is a convenient way to gather data, but as you only surveyed students taking the same classes as you at the same level, the sample is not representative of all the students at your university.
2. Voluntary response sampling
Similar to a convenience sample, a voluntary response sample is mainly based on ease of access. Instead of the researcher choosing participants and directly contacting them, people volunteer themselves (e.g., by responding to a public online survey).
Voluntary response samples are always at least somewhat biased, as some people will inherently be more likely to volunteer than others.
You send out the survey to all students at your university and many students decide to complete it. This can certainly give you some insight into the topic, but the people who responded are more likely to be those who have strong opinions about the student support services, so you can’t be sure that their opinions are representative of all students.
3. Purposive sampling
Purposive sampling , also known as judgement sampling, involves the researcher using their expertise to select a sample that is most useful to the purposes of the research.
It is often used in qualitative research , where the researcher wants to gain detailed knowledge about a specific phenomenon rather than make statistical inferences, or where the population is very small and specific. An effective purposive sample must have clear criteria and rationale for inclusion.
You want to know more about the opinions and experiences of students with a disability at your university, so you purposely select a number of students with different support needs in order to gather a varied range of data on their experiences with student services.
4. Snowball sampling
If the population is hard to access, snowball sampling can be used to recruit participants via other participants. The number of people you have access to ‘snowballs’ as you get in contact with more people.
You are researching experiences of homelessness in your city. Since there is no list of all homeless people in the city, probability sampling isn’t possible. You meet one person who agrees to participate in the research, and she puts you in contact with other homeless people she knows in the area.
A sample is a subset of individuals from a larger population. Sampling means selecting the group that you will actually collect data from in your research.
For example, if you are researching the opinions of students in your university, you could survey a sample of 100 students.
Statistical sampling allows you to test a hypothesis about the characteristics of a population. There are various sampling methods you can use to ensure that your sample is representative of the population as a whole.
Samples are used to make inferences about populations . Samples are easier to collect data from because they are practical, cost-effective, convenient, and manageable.
Probability sampling means that every member of the target population has a known chance of being included in the sample.
Probability sampling methods include simple random sampling , systematic sampling , stratified sampling , and cluster sampling .
In non-probability sampling , the sample is selected based on non-random criteria, and not every member of the population has a chance of being included.
Common non-probability sampling methods include convenience sampling , voluntary response sampling, purposive sampling , snowball sampling , and quota sampling .
Sampling bias occurs when some members of a population are systematically more likely to be selected in a sample than others.
Cite this Scribbr article
If you want to cite this source, you can copy and paste the citation or click the ‘Cite this Scribbr article’ button to automatically add the citation to our free Reference Generator.
McCombes, S. (2022, October 10). Sampling Methods | Types, Techniques, & Examples. Scribbr. Retrieved 18 June 2024, from https://www.scribbr.co.uk/research-methods/sampling/
Is this article helpful?
Shona McCombes
Other students also liked, what is quantitative research | definition & methods, a quick guide to experimental design | 5 steps & examples, controlled experiments | methods & examples of control.
An overview of sampling methods
Last updated
27 February 2023
Reviewed by
Cathy Heath
When researching perceptions or attributes of a product, service, or people, you have two options:
Survey every person in your chosen group (the target market, or population), collate your responses, and reach your conclusions.
Select a smaller group from within your target market and use their answers to represent everyone. This option is sampling .
Sampling saves you time and money. When you use the sampling method, the whole population being studied is called the sampling frame .
The sample you choose should represent your target market, or the sampling frame, well enough to do one of the following:
Generalize your findings across the sampling frame and use them as though you had surveyed everyone
Use the findings to decide on your next step, which might involve more in-depth sampling
Make research less tedious
Dovetail streamlines research to help you uncover and share actionable insights
How was sampling developed?
Valery Glivenko and Francesco Cantelli, two mathematicians studying probability theory in the early 1900s, devised the sampling method. Their research showed that a properly chosen sample of people would reflect the larger group’s status, opinions, decisions, and decision-making steps.
They proved you don't need to survey the entire target market, thereby saving the rest of us a lot of time and money.
- Why is sampling important?
We’ve already touched on the fact that sampling saves you time and money. When you get reliable results quickly, you can act on them sooner. And the money you save can pay for something else.
It’s often easier to survey a sample than a whole population. Sample inferences can be more reliable than those you get from a very large group because you can choose your samples carefully and scientifically.
Sampling is also useful because it is often impossible to survey the entire population. You probably have no choice but to collect only a sample in the first place.
Because you’re working with fewer people, you can collect richer data, which makes your research more accurate. You can:
Ask more questions
Go into more detail
Seek opinions instead of just collecting facts
Observe user behaviors
Double-check your findings if you need to
In short, sampling works! Let's take a look at the most common sampling methods.
- Types of sampling methods
There are two main sampling methods: probability sampling and non-probability sampling. These can be further refined, which we'll cover shortly. You can then decide which approach best suits your research project.
Probability sampling method
Probability sampling is used in quantitative research , so it provides data on the survey topic in terms of numbers. Probability relates to mathematics, hence the name ‘quantitative research’. Subjects are asked questions like:
How many boxes of candy do you buy at one time?
How often do you shop for candy?
How much would you pay for a box of candy?
This method is also called random sampling because everyone in the target market has an equal chance of being chosen for the survey. It is designed to reduce sampling error for the most important variables. You should, therefore, get results that fairly reflect the larger population.
Non-probability sampling method
In this method, not everyone has an equal chance of being part of the sample. It's usually easier (and cheaper) to select people for the sample group. You choose people who are more likely to be involved in or know more about the topic you’re researching.
Non-probability sampling is used for qualitative research. Qualitative data is generated by questions like:
Where do you usually shop for candy (supermarket, gas station, etc.?)
Which candy brand do you usually buy?
Why do you like that brand?
- Probability sampling methods
Here are five ways of doing probability sampling:
Simple random sampling (basic probability sampling)
Systematic sampling
Stratified sampling.
Cluster sampling
Multi-stage sampling
Simple random sampling.
There are three basic steps to simple random sampling:
Choose your sampling frame.
Decide on your sample size. Make sure it is large enough to give you reliable data.
Randomly choose your sample participants.
You could put all their names in a hat, shake the hat to mix the names, and pull out however many names you want in your sample (without looking!)
You could be more scientific by giving each participant a number and then using a random number generator program to choose the numbers.
Instead of choosing names or numbers, you decide beforehand on a selection method. For example, collect all the names in your sampling frame and start at, for example, the fifth person on the list, then choose every fourth name or every tenth name. Alternatively, you could choose everyone whose last name begins with randomly-selected initials, such as A, G, or W.
Choose your system of selecting names, and away you go.
This is a more sophisticated way to choose your sample. You break the sampling frame down into important subgroups or strata . Then, decide how many you want in your sample, and choose an equal number (or a proportionate number) from each subgroup.
For example, you want to survey how many people in a geographic area buy candy, so you compile a list of everyone in that area. You then break that list down into, for example, males and females, then into pre-teens, teenagers, young adults, senior citizens, etc. who are male or female.
So, if there are 1,000 young male adults and 2,000 young female adults in the whole sampling frame, you may want to choose 100 males and 200 females to keep the proportions balanced. You then choose the individual survey participants through the systematic sampling method.
Clustered sampling
This method is used when you want to subdivide a sample into smaller groups or clusters that are geographically or organizationally related.
Let’s say you’re doing quantitative research into candy sales. You could choose your sample participants from urban, suburban, or rural populations. This would give you three geographic clusters from which to select your participants.
This is a more refined way of doing cluster sampling. Let’s say you have your urban cluster, which is your primary sampling unit. You can subdivide this into a secondary sampling unit, say, participants who typically buy their candy in supermarkets. You could then further subdivide this group into your ultimate sampling unit. Finally, you select the actual survey participants from this unit.
- Uses of probability sampling
Probability sampling has three main advantages:
It helps minimizes the likelihood of sampling bias. How you choose your sample determines the quality of your results. Probability sampling gives you an unbiased, randomly selected sample of your target market.
It allows you to create representative samples and subgroups within a sample out of a large or diverse target market.
It lets you use sophisticated statistical methods to select as close to perfect samples as possible.
- Non-probability sampling methods
To recap, with non-probability sampling, you choose people for your sample in a non-random way, so not everyone in your sampling frame has an equal chance of being chosen. Your research findings, therefore, may not be as representative overall as probability sampling, but you may not want them to be.
Sampling bias is not a concern if all potential survey participants share similar traits. For example, you may want to specifically focus on young male adults who spend more than others on candy. In addition, it is usually a cheaper and quicker method because you don't have to work out a complex selection system that represents the entire population in that community.
Researchers do need to be mindful of carefully considering the strengths and limitations of each method before selecting a sampling technique.
Non-probability sampling is best for exploratory research , such as at the beginning of a research project.
There are five main types of non-probability sampling methods:
Convenience sampling
Purposive sampling, voluntary response sampling, snowball sampling, quota sampling.
The strategy of convenience sampling is to choose your sample quickly and efficiently, using the least effort, usually to save money.
Let's say you want to survey the opinions of 100 millennials about a particular topic. You could send out a questionnaire over the social media platforms millennials use. Ask respondents to confirm their birth year at the top of their response sheet and, when you have your 100 responses, begin your analysis. Or you could visit restaurants and bars where millennials spend their evenings and sign people up.
A drawback of convenience sampling is that it may not yield results that apply to a broader population.
This method relies on your judgment to choose the most likely sample to deliver the most useful results. You must know enough about the survey goals and the sampling frame to choose the most appropriate sample respondents.
Your knowledge and experience save you time because you know your ideal sample candidates, so you should get high-quality results.
This method is similar to convenience sampling, but it is based on potential sample members volunteering rather than you looking for people.
You make it known you want to do a survey on a particular topic for a particular reason and wait until enough people volunteer. Then you give them the questionnaire or arrange interviews to ask your questions directly.
Snowball sampling involves asking selected participants to refer others who may qualify for the survey. This method is best used when there is no sampling frame available. It is also useful when the researcher doesn’t know much about the target population.
Let's say you want to research a niche topic that involves people who may be difficult to locate. For our candy example, this could be young males who buy a lot of candy, go rock climbing during the day, and watch adventure movies at night. You ask each participant to name others they know who do the same things, so you can contact them. As you make contact with more people, your sample 'snowballs' until you have all the names you need.
This sampling method involves collecting the specific number of units (quotas) from your predetermined subpopulations. Quota sampling is a way of ensuring that your sample accurately represents the sampling frame.
- Uses of non-probability sampling
You can use non-probability sampling when you:
Want to do a quick test to see if a more detailed and sophisticated survey may be worthwhile
Want to explore an idea to see if it 'has legs'
Launch a pilot study
Do some initial qualitative research
Have little time or money available (half a loaf is better than no bread at all)
Want to see if the initial results will help you justify a longer, more detailed, and more expensive research project
- The main types of sampling bias, and how to avoid them
Sampling bias can fog or limit your research results. This will have an impact when you generalize your results across the whole target market. The two main causes of sampling bias are faulty research design and poor data collection or recording. They can affect probability and non-probability sampling.
Faulty research
If a surveyor chooses participants inappropriately, the results will not reflect the population as a whole.
A famous example is the 1948 presidential race. A telephone survey was conducted to see which candidate had more support. The problem with the research design was that, in 1948, most people with telephones were wealthy, and their opinions were very different from voters as a whole. The research implied Dewey would win, but it was Truman who became president.
Poor data collection or recording
This problem speaks for itself. The survey may be well structured, the sample groups appropriate, the questions clear and easy to understand, and the cluster sizes appropriate. But if surveyors check the wrong boxes when they get an answer or if the entire subgroup results are lost, the survey results will be biased.
How do you minimize bias in sampling?
To get results you can rely on, you must:
Know enough about your target market
Choose one or more sample surveys to cover the whole target market properly
Choose enough people in each sample so your results mirror your target market
Have content validity . This means the content of your questions must be direct and efficiently worded. If it isn’t, the viability of your survey could be questioned. That would also be a waste of time and money, so make the wording of your questions your top focus.
If using probability sampling, make sure your sampling frame includes everyone it should and that your random sampling selection process includes the right proportion of the subgroups
If using non-probability sampling, focus on fairness, equality, and completeness in identifying your samples and subgroups. Then balance those criteria against simple convenience or other relevant factors.
What are the five types of sampling bias?
Self-selection bias. If you mass-mail questionnaires to everyone in the sample, you’re more likely to get results from people with extrovert or activist personalities and not from introverts or pragmatists. So if your convenience sampling focuses on getting your quota responses quickly, it may be skewed.
Non-response bias. Unhappy customers, stressed-out employees, or other sub-groups may not want to cooperate or they may pull out early.
Undercoverage bias. If your survey is done, say, via email or social media platforms, it will miss people without internet access, such as those living in rural areas, the elderly, or lower-income groups.
Survivorship bias. Unsuccessful people are less likely to take part. Another example may be a researcher excluding results that don’t support the overall goal. If the CEO wants to tell the shareholders about a successful product or project at the AGM, some less positive survey results may go “missing” (to take an extreme example.) The result is that your data will reflect an overly optimistic representation of the truth.
Pre-screening bias. If the researcher, whose experience and knowledge are being used to pre-select respondents in a judgmental sampling, focuses more on convenience than judgment, the results may be compromised.
How do you minimize sampling bias?
Focus on the bullet points in the next section and:
Make survey questionnaires as direct, easy, short, and available as possible, so participants are more likely to complete them accurately and send them back
Follow up with the people who have been selected but have not returned their responses
Ignore any pressure that may produce bias
- How do you decide on the type of sampling to use?
Use the ideas you've gleaned from this article to give yourself a platform, then choose the best method to meet your goals while staying within your time and cost limits.
If it isn't obvious which method you should choose, use this strategy:
Clarify your research goals
Clarify how accurate your research results must be to reach your goals
Evaluate your goals against time and budget
List the two or three most obvious sampling methods that will work for you
Confirm the availability of your resources (researchers, computer time, etc.)
Compare each of the possible methods with your goals, accuracy, precision, resource, time, and cost constraints
Make your decision
- The takeaway
Effective market research is the basis of successful marketing, advertising, and future productivity. By selecting the most appropriate sampling methods, you will collect the most useful market data and make the most effective decisions.
Should you be using a customer insights hub?
Do you want to discover previous research faster?
Do you share your research findings with others?
Do you analyze research data?
Start for free today, add your research, and get to key insights faster
Editor’s picks
Last updated: 18 April 2023
Last updated: 27 February 2023
Last updated: 6 February 2023
Last updated: 15 January 2024
Last updated: 6 October 2023
Last updated: 5 February 2023
Last updated: 16 April 2023
Last updated: 7 March 2023
Last updated: 9 March 2023
Last updated: 12 December 2023
Last updated: 11 March 2024
Last updated: 13 May 2024
Latest articles
Related topics, .css-je19u9{-webkit-align-items:flex-end;-webkit-box-align:flex-end;-ms-flex-align:flex-end;align-items:flex-end;display:-webkit-box;display:-webkit-flex;display:-ms-flexbox;display:flex;-webkit-flex-direction:row;-ms-flex-direction:row;flex-direction:row;-webkit-box-flex-wrap:wrap;-webkit-flex-wrap:wrap;-ms-flex-wrap:wrap;flex-wrap:wrap;-webkit-box-pack:center;-ms-flex-pack:center;-webkit-justify-content:center;justify-content:center;row-gap:0;text-align:center;max-width:671px;}@media (max-width: 1079px){.css-je19u9{max-width:400px;}.css-je19u9>span{white-space:pre;}}@media (max-width: 799px){.css-je19u9{max-width:400px;}.css-je19u9>span{white-space:pre;}} decide what to .css-1kiodld{max-height:56px;display:-webkit-box;display:-webkit-flex;display:-ms-flexbox;display:flex;-webkit-align-items:center;-webkit-box-align:center;-ms-flex-align:center;align-items:center;}@media (max-width: 1079px){.css-1kiodld{display:none;}} build next, decide what to build next.

Users report unexpectedly high data usage, especially during streaming sessions.

Users find it hard to navigate from the home page to relevant playlists in the app.

It would be great to have a sleep timer feature, especially for bedtime listening.

I need better filters to find the songs or artists I’m looking for.
Log in or sign up
Get started for free

An official website of the United States government
The .gov means it’s official. Federal government websites often end in .gov or .mil. Before sharing sensitive information, make sure you’re on a federal government site.
The site is secure. The https:// ensures that you are connecting to the official website and that any information you provide is encrypted and transmitted securely.
- Publications
- Account settings
- My Bibliography
- Collections
- Citation manager
Save citation to file
Email citation, add to collections.
- Create a new collection
- Add to an existing collection
Add to My Bibliography
Your saved search, create a file for external citation management software, your rss feed.
- Search in PubMed
- Search in NLM Catalog
- Add to Search
Sampling Methods: A guide for researchers
Affiliation.
- 1 Arizona School of Dentistry & Oral Health A.T. Still University, Mesa, AZ, USA [email protected].
- PMID: 37553279
Sampling is a critical element of research design. Different methods can be used for sample selection to ensure that members of the study population reflect both the source and target populations, including probability and non-probability sampling. Power and sample size are used to determine the number of subjects needed to answer the research question. Characteristics of individuals included in the sample population should be clearly defined to determine eligibility for study participation and improve power. Sample selection methods differ based on study design. The purpose of this short report is to review common sampling considerations and related errors.
Keywords: research design; sample size; sampling.
Copyright © 2023 The American Dental Hygienists’ Association.
PubMed Disclaimer
Similar articles
- Common Sampling Errors in Research Studies. Spolarich AE. Spolarich AE. J Dent Hyg. 2023 Dec;97(6):50-53. J Dent Hyg. 2023. PMID: 38061808
- Folic acid supplementation and malaria susceptibility and severity among people taking antifolate antimalarial drugs in endemic areas. Crider K, Williams J, Qi YP, Gutman J, Yeung L, Mai C, Finkelstain J, Mehta S, Pons-Duran C, Menéndez C, Moraleda C, Rogers L, Daniels K, Green P. Crider K, et al. Cochrane Database Syst Rev. 2022 Feb 1;2(2022):CD014217. doi: 10.1002/14651858.CD014217. Cochrane Database Syst Rev. 2022. PMID: 36321557 Free PMC article.
- [A comparison of convenience sampling and purposive sampling]. Suen LJ, Huang HM, Lee HH. Suen LJ, et al. Hu Li Za Zhi. 2014 Jun;61(3):105-11. doi: 10.6224/JN.61.3.105. Hu Li Za Zhi. 2014. PMID: 24899564 Chinese.
- Concepts in sample size determination. Rao UK. Rao UK. Indian J Dent Res. 2012 Sep-Oct;23(5):660-4. doi: 10.4103/0970-9290.107385. Indian J Dent Res. 2012. PMID: 23422614 Review.
- Power and sample size. Case LD, Ambrosius WT. Case LD, et al. Methods Mol Biol. 2007;404:377-408. doi: 10.1007/978-1-59745-530-5_19. Methods Mol Biol. 2007. PMID: 18450060 Review.
- Search in MeSH
Related information
- Citation Manager
NCBI Literature Resources
MeSH PMC Bookshelf Disclaimer
The PubMed wordmark and PubMed logo are registered trademarks of the U.S. Department of Health and Human Services (HHS). Unauthorized use of these marks is strictly prohibited.

In order to continue enjoying our site, we ask that you confirm your identity as a human. Thank you very much for your cooperation.
- Search Search Please fill out this field.
What Is Sampling?
- How It Works
The Bottom Line
- Corporate Finance
- Financial Ratios
Sampling: What It Is, Different Types, and How Auditors and Marketers Use It
:max_bytes(150000):strip_icc():format(webp)/NatalyaYashina234619_smallerfile-ca61e07c6ec9470c9a0e5a074bf5b359.jpg)
Ariel Courage is an experienced editor, researcher, and former fact-checker. She has performed editing and fact-checking work for several leading finance publications, including The Motley Fool and Passport to Wall Street.
:max_bytes(150000):strip_icc():format(webp)/ArielCourage-50e270c152b046738d83fb7355117d67.jpg)
Sampling is a process in statistical analysis where researchers take a predetermined number of observations from a larger population. Sampling allows researchers to conduct studies about a large group by using a small portion of the population. The method of sampling depends on the type of analysis being performed, but it may include simple random sampling or systematic sampling . Sampling is commonly done in statistics, psychology, and the financial industry.
Key Takeaways
- Sampling allows researchers to use a small group from a larger population to make observations and determinations.
- Types of sampling include random sampling, block sampling, judgment sampling, and systematic sampling.
- Researchers should be aware of sampling errors, which may be the result of random sampling or bias.
- Companies use sampling as a marketing tool to identify the needs and wants of their target market.
- Certified public accountants use sampling during audits to determine the accuracy and completeness of account balances.
Investopedia / Crea Taylor
How Sampling Works
It can be difficult for researchers to conduct accurate studies on large populations . In some cases, it can be impossible to study every individual in the group. That's why they often choose a small portion to represent the entire group. This is called a sample. Samples allow researchers to use characteristics of the small group to make estimates of the larger population.
The chosen sample should be a fair representation of the entire population. When taking a sample from a larger population, it is important to consider how the sample is chosen. To get a representative sample , it must be drawn randomly and encompass the whole population. For example, a lottery system could be used to determine the average age of students in a university by sampling 10% of the student body.
Sampling is commonly used when studying large portions of the population for economic purposes. For instance, the monthly employment report involves the use of sampling, the U.S. Bureau of Labor Statistics (BLS) reports:
- The Current Employment Statistics by using 122,000 businesses and government agencies
- The Current Population Survey with a sample of 60,000 different households across the country
Researchers should be aware of sampling errors . This occurs when the sample that is selected doesn't represent the entire population. This means that the results taken from the sample deviate from the larger population. Sampling error may occur randomly or because there is some form of bias. For instance, some members of the sample group may choose not to participate, or they differ in some way from other participants.
Sampling isn't an exact science, so the results should be taken as generalizations. As such, don't make conclusions about the broader population based on the sample group.
Types of Audit Sampling
As noted above, there are several different types of sampling that researchers can use. These include random, judgment, block, and systemic sampling. These are discussed in more detail below.
Random Sampling
With random sampling , every item within a population has an equal probability of being chosen. It is the furthest removed from any potential bias because there is no human judgement involved in selecting the sample.
For example, a random sample may include choosing the names of 25 employees out of a hat in a company of 250 employees. The population is all 250 employees, and the sample is random because each employee has an equal chance of being chosen.
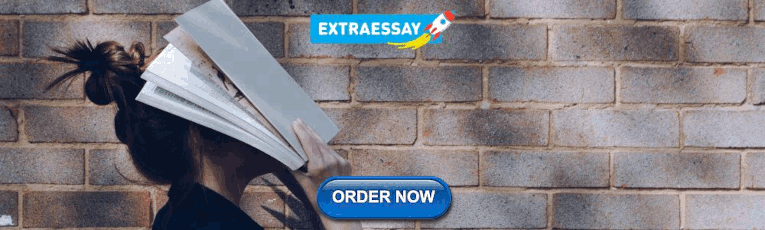
Judgment Sampling
Auditor judgment may be used to select the sample from the full population. An auditor may only be concerned about transactions of a material nature. For example, assume the auditor sets the threshold for materiality for accounts payable transactions at $10,000. If the client provides a complete list of 15 transactions over $10,000, the auditor may just choose to review all transactions due to the small population size.
The auditor may alternatively identify all general ledger accounts with a variance greater than 10% from the prior period. In this case, the auditor is limiting the population from which the sample selection is being derived. Unfortunately, human judgment used in sampling always comes with the potential for bias, whether explicit or implicit.
Block Sampling
Block sampling takes a consecutive series of items within the population to use as the sample. For example, a list of all sales transactions in an accounting period could be sorted in various ways, including by date or by dollar amount.
An auditor may request that the company's accountant provide the list in one format or the other in order to select a sample from a specific segment of the list. This method requires very little modification on the auditor's part, but it is likely that a block of transactions will not be representative of the full population.
Systematic Sampling
Systematic sampling begins at a random starting point within the population and uses a fixed, periodic interval to select items for a sample. The sampling interval is calculated as the population size divided by the sample size. Despite the sample population being selected in advance, systematic sampling is still considered random if the periodic interval is determined beforehand and the starting point is random.
Assume that an auditor reviews the internal controls related to a company's cash account and wants to test the company policy that stipulates that checks exceeding $10,000 must be signed by two people. The population consists of every company check exceeding $10,000 during the fiscal year, which, in this example, was 300. The auditor uses probability statistics and determines that the sample size should be 20% of the population or 60 checks. The sampling interval is 5 or 300 checks ÷ 60 sample checks.
Therefore, the auditor selects every fifth check for testing. Assuming no errors are found in the sampling test work, the statistical analysis gives the auditor a 95% confidence rate that the check procedure was performed correctly. The auditor tests the sample of 60 checks and finds no errors, so he concludes that the internal control over cash is working properly.
Example of Sampling
Market sampling.
Businesses aim to sell their products and/or services to target markets . Before presenting products to the market, companies generally identify the needs and wants of their target audience. To do so, they may employ sampling of the target market population to gain a better understanding of those needs to later create a product and/or service that meets those needs. In this case, gathering the opinions of the sample helps to identify the needs of the whole.
Audit Sampling
During a financial audit , a certified public accountant (CPA) may use sampling to determine the accuracy and completeness of account balances in their client's financial statements. This is called audit sampling. Audit sampling is necessary when the population (the account transaction information) is large.
What Is Sampling Error?
Sampling error is what happens when the sample collected for review doesn't represent the entire population being studied. This jeopardizes the accuracy and validity of the study being conducted. For instance, sampling error occurs if researchers include professors in the sample when they're trying to determine how students feel about the university experience. Sampling error may be random or the result of some type of bias.
What Is Cluster Sampling?
Cluster sampling is a form of probability sampling. When researchers conduct cluster sampling, they divide the population into smaller groups. They then select individuals randomly from these groups to form their samples and conduct their studies. This kind of sampling is used when both the overall population and sample size is too large to handle.
What's the Difference Between Probability and Non-Probability Sampling?
Probability sampling gives researchers the chance to come to stronger conclusions about the entire population that is being studied. It involves the use of random sampling, which means that all of the participants in the group are equally likely to get a chance to be chosen as a representative sample of the entire population. The result is often unbiased.
Non-probability sampling, on the other hand, allows researchers to easily collect information. This type of sampling is generally biased as it is unknown which participants will be chosen as a sample.
Statisticians often resort to sampling in order to conduct research when they're dealing with large populations. Sampling is a technique that involves taking a small number of participants from a much bigger group. This is often found when data needs to be collected about the population, including statistical analysis, population surveys, and economic studies.
U.S. Bureau of Labor Statistics. " Monthly Employment Situation Report: Quick Guide to Methods and Measurement Issues ."
American Institute of Certified Public Accountants. " Section 350 Audit Sampling ," Page 2067.
:max_bytes(150000):strip_icc():format(webp)/systematicsampling-767f6f7962b6455f8ae1435a4f9aa515.jpg)
- Terms of Service
- Editorial Policy
- Privacy Policy
Find Study Materials for
- Explanations
- Business Studies
- Combined Science
- Engineering
- English Literature
- Environmental Science
- Human Geography
- Macroeconomics
- Microeconomics
- Social Studies
- Browse all subjects
- Read our Magazine
Create Study Materials
- Flashcards Create and find the best flashcards.
- Notes Create notes faster than ever before.
- Study Sets Everything you need for your studies in one place.
- Study Plans Stop procrastinating with our smart planner features.
- Sampling Plan
Do you like free samples? I do too! Unfortunately, this is not an explanation of free samples, but it's an article about something that sounds quite similar - a sampling plan.
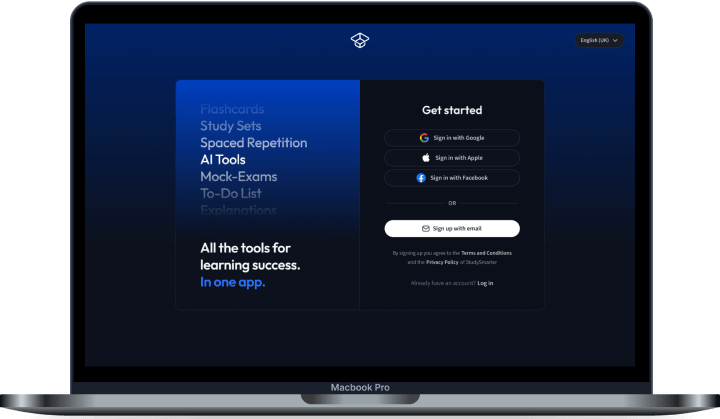
Create learning materials about Sampling Plan with our free learning app!
- Instand access to millions of learning materials
- Flashcards, notes, mock-exams and more
- Everything you need to ace your exams
- Customer Driven Marketing Strategy
- Digital Marketing
- Integrated Marketing Communications
- International Marketing
- Introduction to Marketing
- Marketing Campaign Examples
- Marketing Information Management
- Behavioral Targeting
- Customer Relationship Management
- Ethics in Marketing
- Experimental Research
- Focus Groups
- Interview in Research
- Market Calculations
- Market Mapping
- Market Research
- Marketing Analytics
- Marketing Information System
- Marketing KPIs
- Methods of Market Research
- Multi level Marketing
- Neuromarketing
- Observational Research
- Online Focus Groups
- PED and YED
- Primary Market Research
- Research Instrument
- Secondary Market Research
- Survey Research
- Understanding Markets and Customers
- Marketing Management
- Strategic Marketing Planning
This might not be a term you are very familiar with, but it is a significant part of marketing. We know how important research is for marketing. We need to know the target audience to plan a successful marketing campaign, and a sampling plan is essential to make it successful. Wondering how? Keep reading to find out!
Sampling Plan Definition
Knowing the target audience is vital to understanding their needs and wants. Researchers need to study the population to draw conclusions. These conclusions will serve as a basis for constructing a suitable marketing campaign. But observing every person in the selected location is impractical and, at times, impossible. Therefore, researchers select a group of individuals representative of the population. A sampling plan is an outline based on which research is conducted.
A sampling plan outlines the individuals chosen to represent the target population under consideration for research purposes.
It is crucial to verify that the sampling plan is representative of all kinds of people to draw accurate conclusions.
Sampling Plan Research
The sampling plan is an essential part of the implementation phase in market research - it is the first step of implementing market research.
Check out our explanation of market research to find out more.
Researchers decide the sampling unit, size, and procedure when creating a sampling plan.
Deciding the sampling unit involves defining the target population. The area of interest for the research may contain people that may be out of the scope of the research. Therefore, the researcher must first identify the type of people within the research's parameters.
The sample size will specify how many people from the sampling unit will be surveyed or studied. Usually, in realistic cases, the target population is colossal. Analyzing every single individual is an arduous task. Therefore, the researcher must decide which individuals should be considered and how many people to survey.
The sampling procedure decides how the sample size is chosen. Researchers can do this based on both probability sampling methods and non-probability sampling methods. We will talk about this in more detail in the following sections.
Sampling Plan Types
The sampling plan mainly consists of two different types of methods - one based on probability methods and the other based on non-probability methods .
In the probability sampling method, the researcher lists a few criteria and then chooses people randomly from the population. In this method, all people of the population have an equal chance to be selected. The probability methods are further classified into:
1. Simple Random Sampling - as the name suggests, this type of sampling picks individuals randomly from the selection.
2. Cluster Sampling - the whole population gets divided into groups or clusters. Researchers then survey people from the selected clusters.
3. Systematic Sampling - researchers select individuals at a regular interval; for example, the researcher will select every 15th person on the list for interviews.
4. Stratified Sampling - researchers divide the group into smaller subgroups called strata based on their characteristics. Researchers then pick individuals at random from the strata.
Difference between cluster sampling and stratified sampling
In cluster sampling, all individuals are put into different groups, and all people in the selected groups are studied.
In stratified sampling, all the individuals are put into different groups, and some people from all groups are surveyed.
A non-probability method involves choosing people at random without any defined criteria. This means that not everybody has an equal chance of being selected for the survey. N on-probability techniques can be further classified into:
1. Convenience Sampling - this depends on the ease of accessing a person of interest.
2. Judgemental Sampling - also known as purposive sampling, includes selecting people with a particular characteristic that supports the scope of the research.
3. Snowball Sampling - used when trying to find people with traits that are difficult to trace. In such cases, the researcher would find one or two people with the traits and then ask them to refer to people with similar characteristics.
4. Quota Sampling - this involves collecting information from a homogenous group.
Steps of a Sample Plan
A sampling plan helps researchers collect data and get results quicker, as only a group of individuals is selected to be studied instead of the whole population. But how is a sampling plan conducted? What are the steps of a sample plan?
A sampling plan study consists of 5 main steps:
1. Sample Definition - this step involves identifying the research goals or what the research is trying to achieve. Defining the sample will help the researcher identify what they have to look for in the sample.
2. Sample Selection - after the sample definition, researchers now have to obtain a sample frame. The sample frame will give the researchers a list of the population from which the researcher chooses people to sample.
3. Sample Size Determination - the sample size is the number of individuals that will be considered while determining the sampling plan. This step defines the number of individuals that the researcher will survey.
4. Sample Design - in this step, the samples are picked from the population. Researchers can select individuals based on probability or non-probability methods.
5. Sample Assessment - this step ensures that the samples chosen are representative enough of the population and ensures quality data collection.
After these processes are finalized, researchers carry forward with the rest of the research, such as drawing conclusions that form a basis for the marketing campaign.
Probability sampling methods are more complex, costly, and time-consuming than non-probability methods.
Sampling Plans Example
Different methods of sampling plans help to yield different types of data. The sampling plan will depend on the company's research goals and limitations. Given below are a few examples of companies that use different types of sampling plans:
1. Simple Random Sampling - A district manager wants to evaluate employee satisfaction at a store. Now, he would go to the store, pick a few employees randomly, and ask them about their satisfaction. Every employee has an equal chance of being selected by the district manager for the survey.
2. Cluster Sampling - A reputed private school is planning to launch in a different city. To gain a better insight into the city, they divided the population based on families with school-aged kids and people with high incomes. These insights will help them decide if starting a branch in that particular city would be worth it or not.
3. Systematic Sampling - A supermarket with many branches decides to reallocate its staff to improve efficiency. The manager decides that every third person, chosen per their employee number, would be transferred to a different location.
4. Stratified Sampling - A research startup is trying to understand people's sleep patterns based on different age groups. Therefore, the whole sampling unit gets divided into different age groups (or strata), such as 0-3 months, 4-12 months, 1-2 years, 3-5 years, 6-12 years, and so on. Some people from all the groups are studied.
5. Convenience Sampling - An NGO is trying to get people to sign up for a "street-clean" program as part of the Earth Day campaign. They have stationed themselves on the sidewalks of a busy shopping street, and are approaching people who pass them by to try and pursue them to join the program.
6. Judgemental Sampling - A real estate company is trying to determine how the rental price hike affects people. To find the answer to this question, they would only have to consider people that live in rented houses, meaning that people who own a home would be excluded from this survey.
7. Snowball Sampling - A pharmaceutical company is trying to get a list of patients with leukemia. As the company cannot go to hospitals to ask for patients' information, they would first find a couple of patients with the illness and then ask them to refer patients with the same illness.
8. Quota Sampling - Recruiters that want to hire employees with a degree from a particular school will group them into a separate subgroup. This type of selection is called quota selection.
Sampling plan - Key takeaways
- During a sampling plan in research, the sampling unit, the sampling size, and the sampling procedure are determined.
- The sample size will specify how many people from the sampling unit will be surveyed or studied.
- The sampling procedure decides how researchers will select the sample size.
- The methods of probability sampling include simple random, cluster, systematic, and stratified sampling.
- The non-probability sampling plan methods include convenience, judgemental, snowball, and quota sampling.
- Sample definition, sample selection, sample size determination, sample design, and sample assessment are the steps of a sample plan.
Flashcards in Sampling Plan 18
Define sampling plan.
A sampling plan outlines the individuals chosen to represent the target population under consideration for research purposes.
The sampling plan is a part of the _________ phase.
During a sampling plan in research, _____________, ___________, and the sampling procedure are decided.
During a sampling plan in research, the sampling unit , the sampling size , and the sampling procedure are decided.
The ___________ involves deciding the target population.
sampling unit
The sample size
will specify how many people from the sampling unit will be surveyed or studied.
What are the two types of sampling plans?
Probability and non-probability sampling .
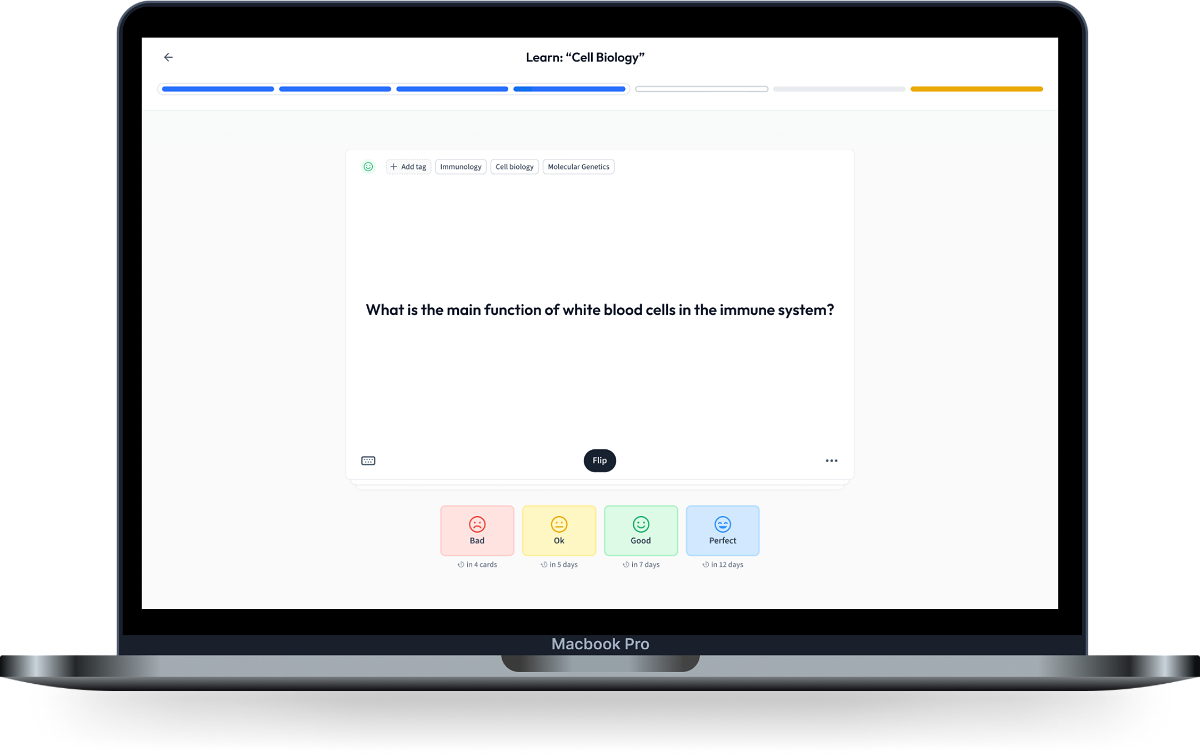
Learn with 18 Sampling Plan flashcards in the free StudySmarter app
We have 14,000 flashcards about Dynamic Landscapes.
Already have an account? Log in
Frequently Asked Questions about Sampling Plan
What is a sample plan in marketing?
Researchers need to study the population to draw conclusions. But observing every person in the selected location is impractical and, at times, impossible. Therefore, researchers select a group of individuals representative of the population. A sampling plan outlines the individuals chosen to represent the target population under consideration for research purposes.
What is a sampling plan and its types?
The sampling plan mainly consists of two different types of methods - one based on probability methods and the other based on non-probability methods. Probability sampling methods include simple random, cluster, systematic, and stratified sampling. The non-probability sampling methods include convenience, judgemental, snowball, and quota sampling.
Why is the sampling plan important?
The sampling plan is an essential part of the implementation phase in market research - it is the first step of implementing market research. Observing every person in the selected location is impractical. Therefore, researchers select a group of individuals representative of the population called the sampling unit. This is outlined in the sampling plan.
What should a marketing plan include?
A good marketing plan should include the target market, the unique selling proposition, SWOT analysis, marketing strategies, the budget, and the duration of the research.
What are the components of a sampling plan?
The sample definition, sample selection, sample size determination, sample design, and sample assessment are the components of a sampling plan.
Test your knowledge with multiple choice flashcards
The ___________ involves deciding the target population.
The sample size
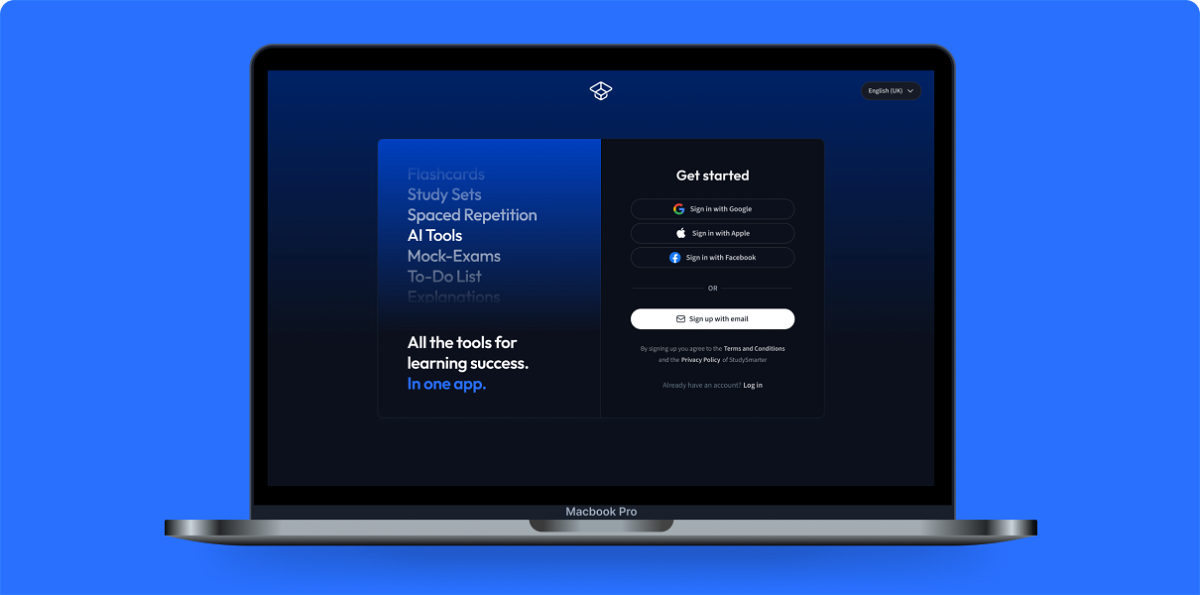
Join the StudySmarter App and learn efficiently with millions of flashcards and more!
Keep learning, you are doing great.
Discover learning materials with the free StudySmarter app
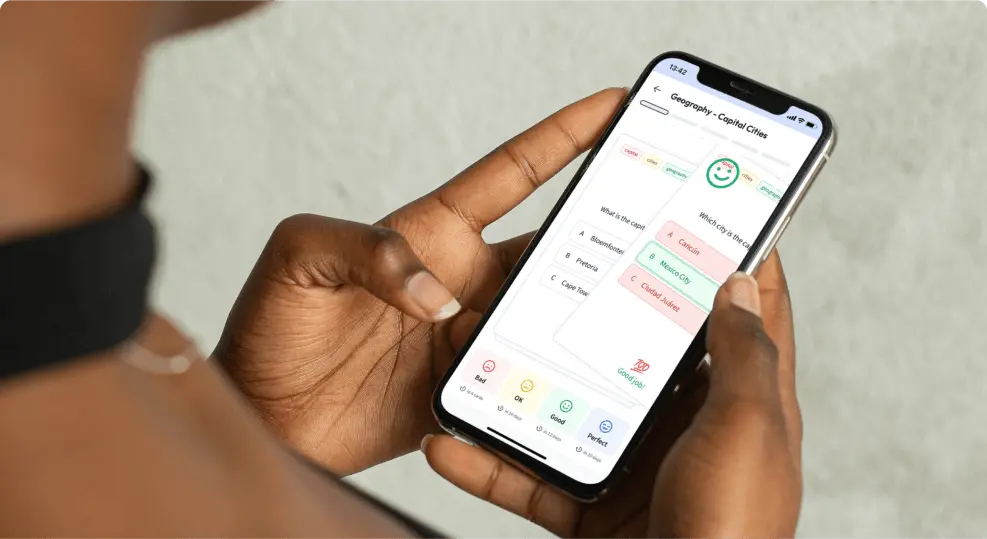
About StudySmarter
StudySmarter is a globally recognized educational technology company, offering a holistic learning platform designed for students of all ages and educational levels. Our platform provides learning support for a wide range of subjects, including STEM, Social Sciences, and Languages and also helps students to successfully master various tests and exams worldwide, such as GCSE, A Level, SAT, ACT, Abitur, and more. We offer an extensive library of learning materials, including interactive flashcards, comprehensive textbook solutions, and detailed explanations. The cutting-edge technology and tools we provide help students create their own learning materials. StudySmarter’s content is not only expert-verified but also regularly updated to ensure accuracy and relevance.
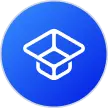
StudySmarter Editorial Team
Team Marketing Teachers
- 9 minutes reading time
- Checked by StudySmarter Editorial Team
Study anywhere. Anytime.Across all devices.
Create a free account to save this explanation..
Save explanations to your personalised space and access them anytime, anywhere!
By signing up, you agree to the Terms and Conditions and the Privacy Policy of StudySmarter.
Sign up to highlight and take notes. It’s 100% free.
Join over 22 million students in learning with our StudySmarter App
The first learning app that truly has everything you need to ace your exams in one place
- Flashcards & Quizzes
- AI Study Assistant
- Study Planner
- Smart Note-Taking
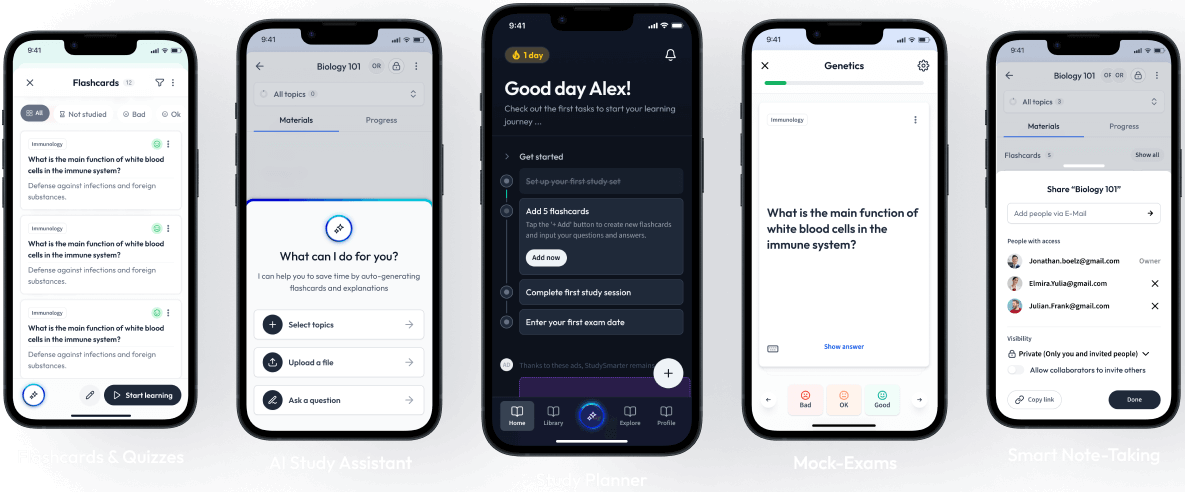
Get unlimited access with a free StudySmarter account.
- Instant access to millions of learning materials.
- Flashcards, notes, mock-exams, AI tools and more.
- Everything you need to ace your exams.
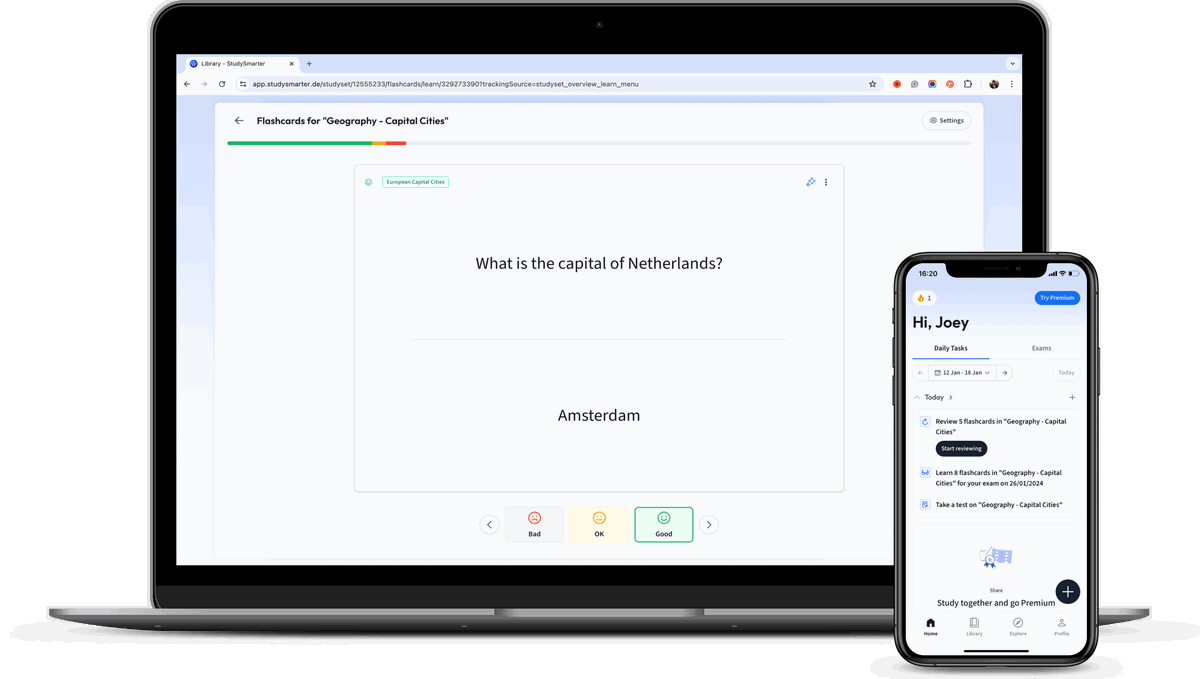
- Skip to main content
- Skip to primary sidebar
- Skip to footer
- QuestionPro

- Solutions Industries Gaming Automotive Sports and events Education Government Travel & Hospitality Financial Services Healthcare Cannabis Technology Use Case NPS+ Communities Audience Contactless surveys Mobile LivePolls Member Experience GDPR Positive People Science 360 Feedback Surveys
- Resources Blog eBooks Survey Templates Case Studies Training Help center

Home Market Research
Sampling Methods: Guide To All Types with Examples
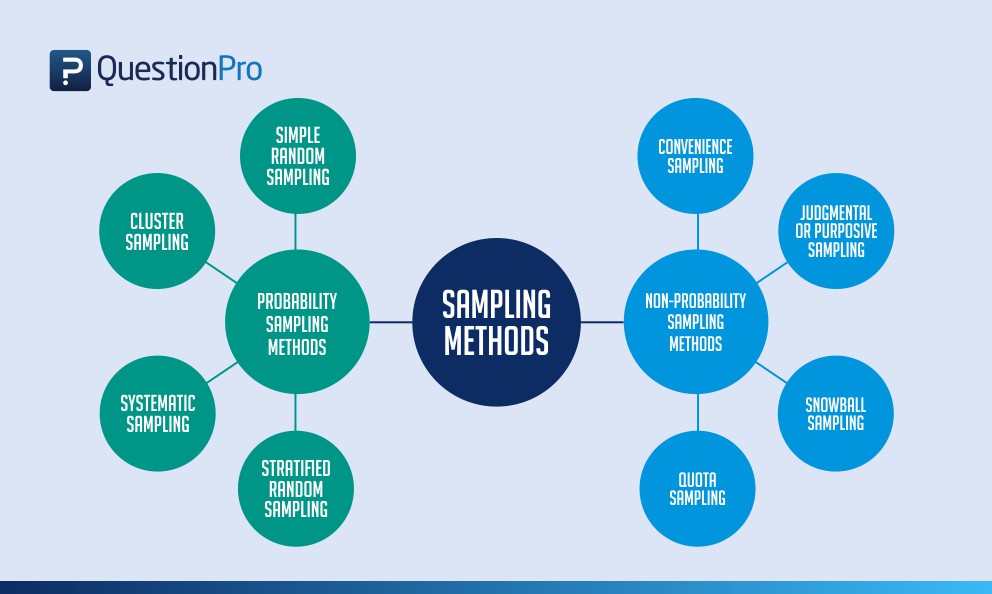
Sampling is an essential part of any research project. The right sampling method can make or break the validity of your research, and it’s essential to choose the right method for your specific question. In this article, we’ll take a closer look at some of the most popular sampling methods and provide real-world examples of how they can be used to gather accurate and reliable data.
LEARN ABOUT: Research Process Steps
From simple random sampling to complex stratified sampling , we’ll explore each method’s pros, cons, and best practices. So, whether you’re a seasoned researcher or just starting your journey, this article is a must-read for anyone looking to master sampling methods. Let’s get started!
Content Index
What is sampling?
Types of sampling: sampling methods, types of probability sampling with examples:, uses of probability sampling, types of non-probability sampling with examples, uses of non-probability sampling, how do you decide on the type of sampling to use, difference between probability sampling and non-probability sampling methods.
Sampling is a technique of selecting individual members or a subset of the population to make statistical inferences from them and estimate the characteristics of the whole population. Different sampling methods are widely used by researchers in market research so that they do not need to research the entire population to collect actionable insights.
It is also a time-convenient and cost-effective method and hence forms the basis of any research design . Sampling techniques can be used in research survey software for optimum derivation.
For example, suppose a drug manufacturer would like to research the adverse side effects of a drug on the country’s population. In that case, it is almost impossible to conduct a research study that involves everyone. In this case, the researcher decides on a sample of people from each demographic and then researches them, giving him/her indicative feedback on the drug’s behavior.
Learn more about Audience by QuestionPro
Sampling in market action research is of two types – probability sampling and non-probability sampling. Let’s take a closer look at these two methods of sampling.
- Probability sampling: Probability sampling is a sampling technique where a researcher selects a few criteria and chooses members of a population randomly. All the members have an equal opportunity to participate in the sample with this selection parameter.
- Non-probability sampling: In non-probability sampling, the researcher randomly chooses members for research. This sampling method is not a fixed or predefined selection process. This makes it difficult for all population elements to have equal opportunities to be included in a sample.
This blog discusses the various probability and non-probability sampling methods you can implement in any market research study.
LEARN ABOUT: Survey Sampling
Probability sampling is a technique in which researchers choose samples from a larger population based on the theory of probability. This sampling method considers every member of the population and forms samples based on a fixed process.
For example, in a population of 1000 members, every member will have a 1/1000 chance of being selected to be a part of a sample. Probability sampling eliminates sampling bias in the population and allows all members to be included in the sample.
There are four types of probability sampling techniques:
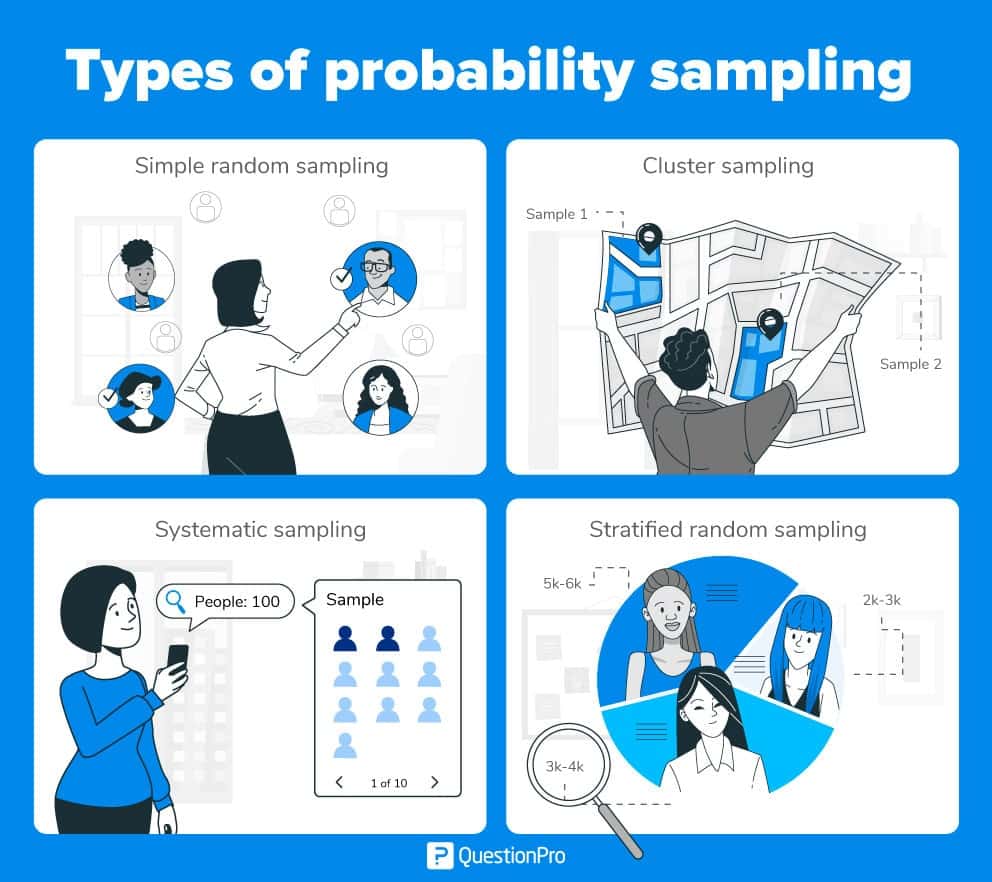
- Simple random sampling: One of the best probability sampling techniques that helps in saving time and resources is the Simple Random Sampling method. It is a reliable method of obtaining information where every single member of a population is chosen randomly, merely by chance. Each individual has the same probability of being chosen to be a part of a sample. For example, in an organization of 500 employees, if the HR team decides on conducting team-building activities, they would likely prefer picking chits out of a bowl. In this case, each of the 500 employees has an equal opportunity of being selected.
- Cluster sampling: Cluster sampling is a method where the researchers divide the entire population into sections or clusters representing a population. Clusters are identified and included in a sample based on demographic parameters like age, sex, location, etc. This makes it very simple for a survey creator to derive effective inferences from the feedback. For example, suppose the United States government wishes to evaluate the number of immigrants living in the Mainland US. In that case, they can divide it into clusters based on states such as California, Texas, Florida, Massachusetts, Colorado, Hawaii, etc. This way of conducting a survey will be more effective as the results will be organized into states and provide insightful immigration data.
- Systematic sampling: Researchers use the systematic sampling method to choose the sample members of a population at regular intervals. It requires selecting a starting point for the sample and sample size determination that can be repeated at regular intervals. This type of sampling method has a predefined range; hence, this sampling technique is the least time-consuming. For example, a researcher intends to collect a systematic sample of 500 people in a population of 5000. He/she numbers each element of the population from 1-5000 and will choose every 10th individual to be a part of the sample (Total population/ Sample Size = 5000/500 = 10).
- Stratified random sampling: Stratified random sampling is a method in which the researcher divides the population into smaller groups that don’t overlap but represent the entire population. While sampling, these groups can be organized, and then draw a sample from each group separately. For example, a researcher looking to analyze the characteristics of people belonging to different annual income divisions will create strata (groups) according to the annual family income. Eg – less than $20,000, $21,000 – $30,000, $31,000 to $40,000, $41,000 to $50,000, etc. By doing this, the researcher concludes the characteristics of people belonging to different income groups. Marketers can analyze which income groups to target and which ones to eliminate to create a roadmap that would bear fruitful results.
LEARN ABOUT: Purposive Sampling
There are multiple uses of probability sampling:
- Reduce Sample Bias: Using the probability sampling method, the research bias in the sample derived from a population is negligible to non-existent. The sample selection mainly depicts the researcher’s understanding and inference. Probability sampling leads to higher-quality data collection as the sample appropriately represents the population.
- Diverse Population: When the population is vast and diverse, it is essential to have adequate representation so that the data is not skewed toward one demographic . For example, suppose Square would like to understand the people that could make their point-of-sale devices. In that case, a survey conducted from a sample of people across the US from different industries and socio-economic backgrounds helps.
- Create an Accurate Sample: Probability sampling helps the researchers plan and create an accurate sample. This helps to obtain well-defined data.
The non-probability method is a sampling method that involves a collection of feedback based on a researcher or statistician’s sample selection capabilities and not on a fixed selection process. In most situations, the output of a survey conducted with a non-probable sample leads to skewed results, which may not represent the desired target population. But, there are situations, such as the preliminary stages of research or cost constraints for conducting research, where non-probability sampling will be much more useful than the other type.
Four types of non-probability sampling explain the purpose of this sampling method in a better manner:
- Convenience sampling: This method depends on the ease of access to subjects such as surveying customers at a mall or passers-by on a busy street. It is usually termed as convenience sampling because of the researcher’s ease of carrying it out and getting in touch with the subjects. Researchers have nearly no authority to select the sample elements, and it’s purely done based on proximity and not representativeness. This non-probability sampling method is used when there are time and cost limitations in collecting feedback. In situations with resource limitations, such as the initial stages of research, convenience sampling is used. For example, startups and NGOs usually conduct convenience sampling at a mall to distribute leaflets of upcoming events or promotion of a cause – they do that by standing at the mall entrance and giving out pamphlets randomly.
- Judgmental or purposive sampling: Judgmental or purposive samples are formed at the researcher’s discretion. Researchers purely consider the purpose of the study, along with the understanding of the target audience. For instance, when researchers want to understand the thought process of people interested in studying for their master’s degree. The selection criteria will be: “Are you interested in doing your masters in …?” and those who respond with a “No” are excluded from the sample.
- Snowball sampling: Snowball sampling is a sampling method that researchers apply when the subjects are difficult to trace. For example, surveying shelterless people or illegal immigrants will be extremely challenging. In such cases, using the snowball theory, researchers can track a few categories to interview and derive results. Researchers also implement this sampling method when the topic is highly sensitive and not openly discussed—for example, surveys to gather information about HIV Aids. Not many victims will readily respond to the questions. Still, researchers can contact people they might know or volunteers associated with the cause to get in touch with the victims and collect information.
- Quota sampling: In Quota sampling , members in this sampling technique selection happens based on a pre-set standard. In this case, as a sample is formed based on specific attributes, the created sample will have the same qualities found in the total population. It is a rapid method of collecting samples.
Non-probability sampling is used for the following:
- Create a hypothesis: Researchers use the non-probability sampling method to create an assumption when limited to no prior information is available. This method helps with the immediate return of data and builds a base for further research.
- Exploratory research: Researchers use this sampling technique widely when conducting qualitative research, pilot studies, or exploratory research .
- Budget and time constraints: The non-probability method when there are budget and time constraints, and some preliminary data must be collected. Since the survey design is not rigid, it is easier to pick respondents randomly and have them take the survey or questionnaire .
For any research, it is essential to choose a sampling method accurately to meet the goals of your study. The effectiveness of your sampling relies on various factors. Here are some steps expert researchers follow to decide the best sampling method.
- Jot down the research goals. Generally, it must be a combination of cost, precision, or accuracy.
- Identify the effective sampling techniques that might potentially achieve the research goals.
- Test each of these methods and examine whether they help achieve your goal.
- Select the method that works best for the research.
Unlock the power of accurate sampling!
We have looked at the different types of sampling methods above and their subtypes. To encapsulate the whole discussion, though, the significant differences between probability sampling methods and non-probability sampling methods are as below:
Probability Sampling is a sampling technique in which samples from a larger population are chosen using a method based on the theory of probability. | Non-probability sampling is a sampling technique in which the researcher selects samples based on the researcher’s subjective judgment rather than random selection. | |
Random sampling method. | Non-random sampling method | |
The population is selected randomly. | The population is selected arbitrarily. | |
The research is conclusive. | The research is exploratory. | |
Since there is a method for deciding the sample, the population demographics are conclusively represented. | Since the sampling method is arbitrary, the population demographics representation is almost always skewed. | |
Takes longer to conduct since the research design defines the selection parameters before the market research study begins. | This type of sampling method is quick since neither the sample nor the selection criteria of the sample are undefined. | |
This type of sampling is entirely unbiased; hence, the results are also conclusive. | This type of sampling is entirely biased, and hence the results are biased, too, rendering the research speculative. | |
In probability sampling, there is an underlying hypothesis before the study begins, and this method aims to prove the hypothesis. | In non-probability sampling, the hypothesis is derived after conducting the research study. |
Now that we have learned how different sampling methods work and are widely used by researchers in market research so that they don’t need to research the entire population to collect actionable insights, let’s go over a tool that can help you manage these insights.
LEARN ABOUT: 12 Best Tools for Researchers
QuestionPro understands the need for an accurate, timely, and cost-effective method to select the proper sample; that’s why we bring QuestionPro Software, a set of tools that allow you to efficiently select your target audience , manage your insights in an organized, customizable repository and community management for post-survey feedback.
Don’t miss the chance to elevate the value of research.
FREE TRIAL LEARN MORE
MORE LIKE THIS
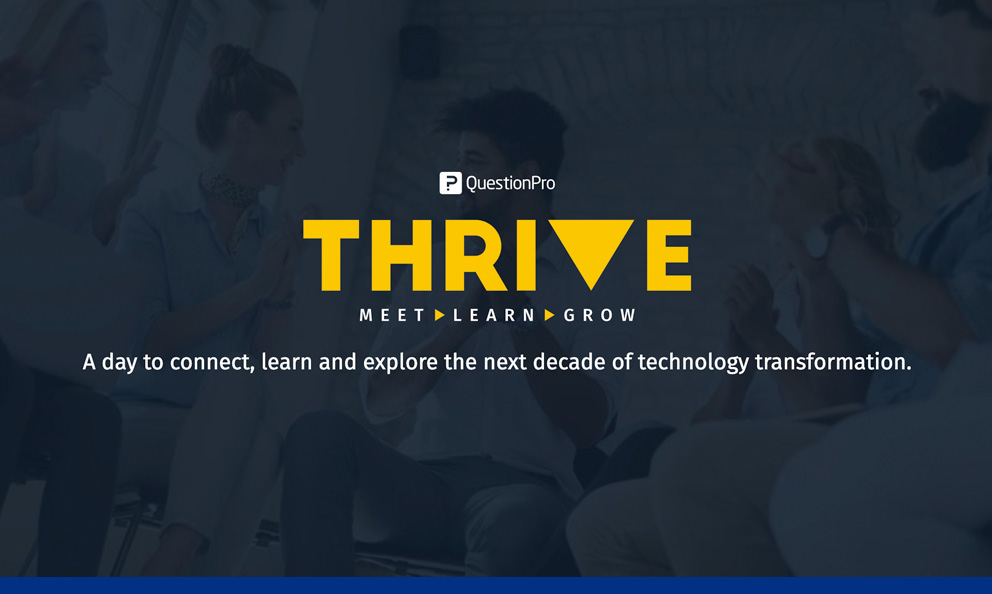
QuestionPro Thrive: A Space to Visualize & Share the Future of Technology
Jun 18, 2024
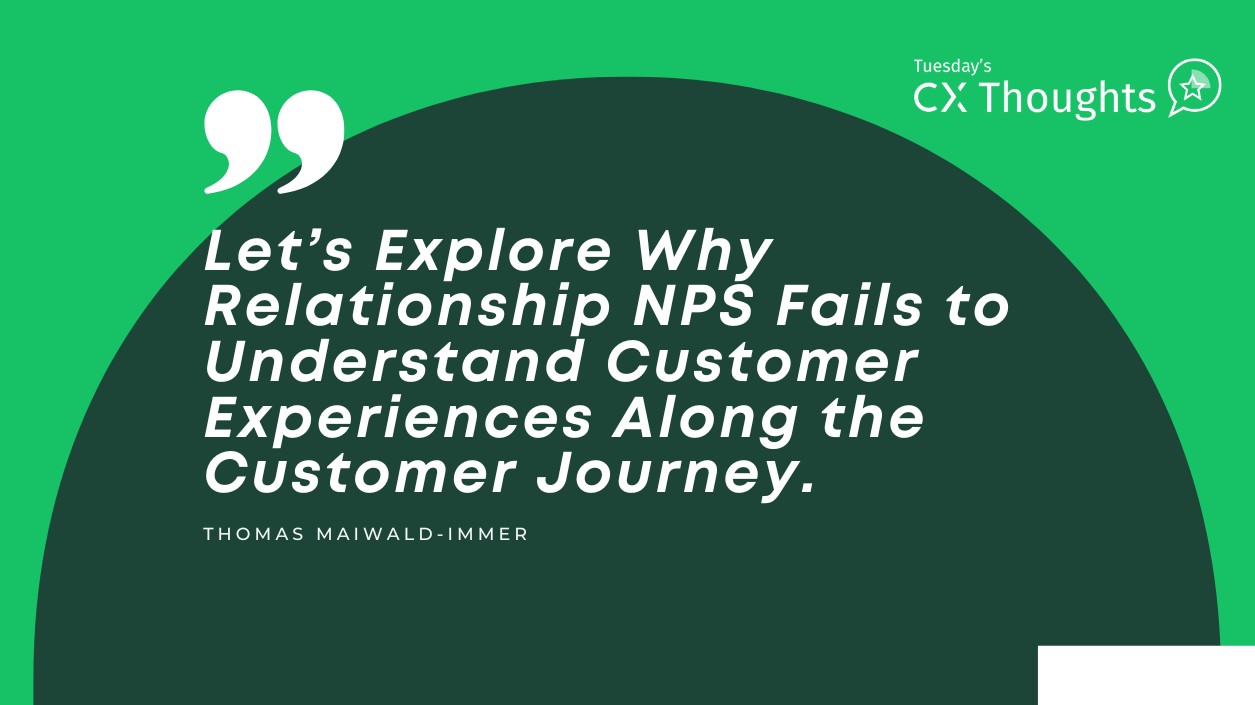
Relationship NPS Fails to Understand Customer Experiences — Tuesday CX
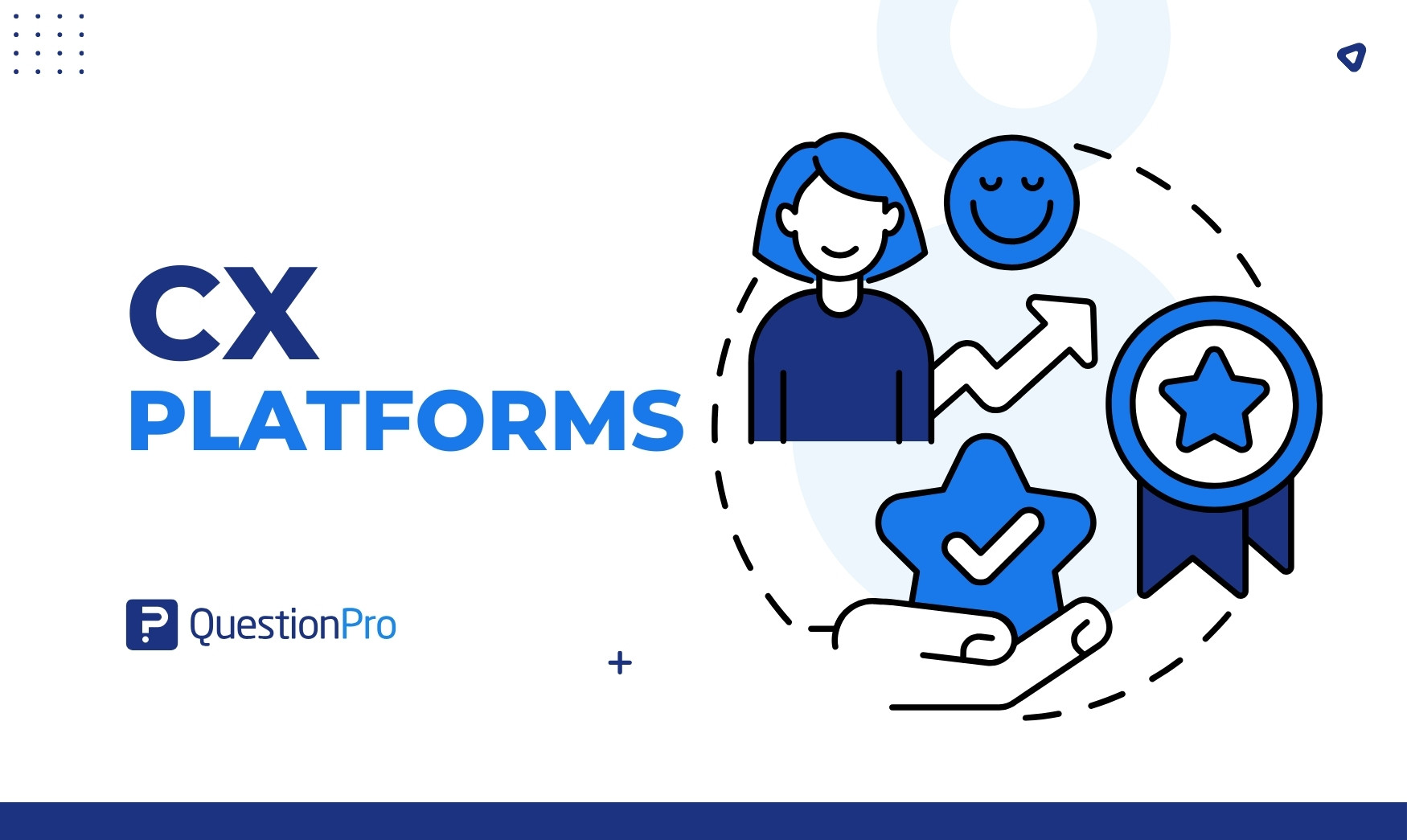
CX Platform: Top 13 CX Platforms to Drive Customer Success
Jun 17, 2024
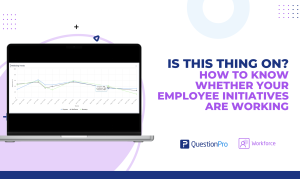
How to Know Whether Your Employee Initiatives are Working
Other categories.
- Academic Research
- Artificial Intelligence
- Assessments
- Brand Awareness
- Case Studies
- Communities
- Consumer Insights
- Customer effort score
- Customer Engagement
- Customer Experience
- Customer Loyalty
- Customer Research
- Customer Satisfaction
- Employee Benefits
- Employee Engagement
- Employee Retention
- Friday Five
- General Data Protection Regulation
- Insights Hub
- Life@QuestionPro
- Market Research
- Mobile diaries
- Mobile Surveys
- New Features
- Online Communities
- Question Types
- Questionnaire
- QuestionPro Products
- Release Notes
- Research Tools and Apps
- Revenue at Risk
- Survey Templates
- Training Tips
- Tuesday CX Thoughts (TCXT)
- Uncategorized
- Video Learning Series
- What’s Coming Up
- Workforce Intelligence
Root out friction in every digital experience, super-charge conversion rates, and optimise digital self-service
Uncover insights from any interaction, deliver AI-powered agent coaching, and reduce cost to serve
Increase revenue and loyalty with real-time insights and recommendations delivered straight to teams on the ground
Know how your people feel and empower managers to improve employee engagement, productivity, and retention
Take action in the moments that matter most along the employee journey and drive bottom line growth
Whatever they’re are saying, wherever they’re saying it, know exactly what’s going on with your people
Get faster, richer insights with qual and quant tools that make powerful market research available to everyone
Run concept tests, pricing studies, prototyping + more with fast, powerful studies designed by UX research experts
Track your brand performance 24/7 and act quickly to respond to opportunities and challenges in your market
Meet the operating system for experience management
- Free Account
- Product Demos
- For Digital
- For Customer Care
- For Human Resources
- For Researchers
- Financial Services
- All Industries
Popular Use Cases
- Customer Experience
- Employee Experience
- Employee Exit Interviews
- Net Promoter Score
- Voice of Customer
- Customer Success Hub
- Product Documentation
- Training & Certification
- XM Institute
- Popular Resources
- Customer Stories
- Artificial Intelligence
- Market Research
- Partnerships
- Marketplace
The annual gathering of the experience leaders at the world’s iconic brands building breakthrough business results.
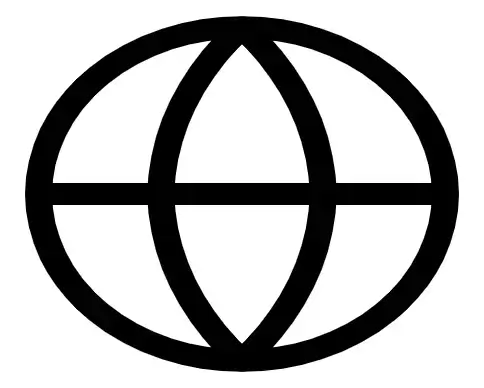
- English/AU & NZ
- Español/Europa
- Español/América Latina
- Português Brasileiro
- REQUEST DEMO
- Experience Management
- Sampling Methods
Try Qualtrics for free
Sampling methods, types & techniques.
15 min read Your comprehensive guide to the different sampling methods available to researchers – and how to know which is right for your research.
What is sampling?
In survey research, sampling is the process of using a subset of a population to represent the whole population. To help illustrate this further, let’s look at data sampling methods with examples below.
Let’s say you wanted to do some research on everyone in North America. To ask every person would be almost impossible. Even if everyone said “yes”, carrying out a survey across different states, in different languages and timezones, and then collecting and processing all the results , would take a long time and be very costly.
Sampling allows large-scale research to be carried out with a more realistic cost and time-frame because it uses a smaller number of individuals in the population with representative characteristics to stand in for the whole.
However, when you decide to sample, you take on a new task. You have to decide who is part of your sample list and how to choose the people who will best represent the whole population. How you go about that is what the practice of sampling is all about.
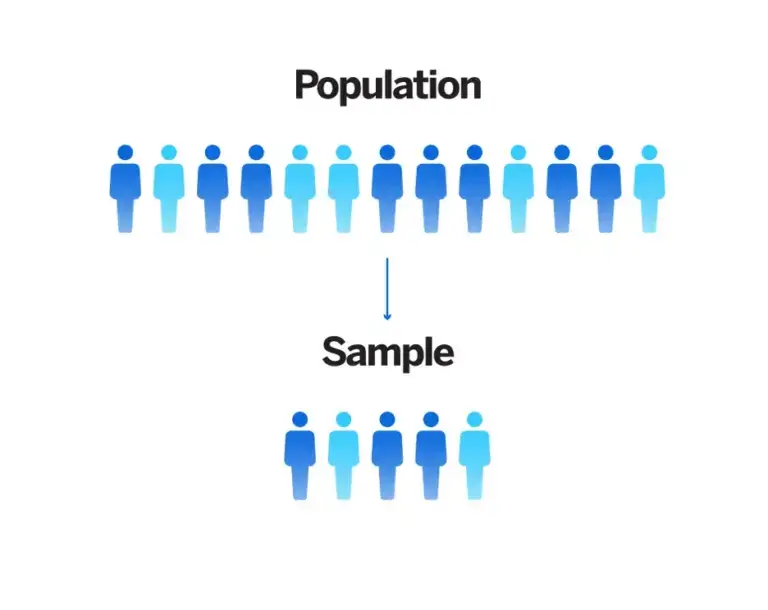
Sampling definitions
- Population: The total number of people or things you are interested in
- Sample: A smaller number within your population that will represent the whole
- Sampling: The process and method of selecting your sample
Free eBook: 2024 Market Research Trends
Why is sampling important?
Although the idea of sampling is easiest to understand when you think about a very large population, it makes sense to use sampling methods in research studies of all types and sizes. After all, if you can reduce the effort and cost of doing a study, why wouldn’t you? And because sampling allows you to research larger target populations using the same resources as you would smaller ones, it dramatically opens up the possibilities for research.
Sampling is a little like having gears on a car or bicycle. Instead of always turning a set of wheels of a specific size and being constrained by their physical properties, it allows you to translate your effort to the wheels via the different gears, so you’re effectively choosing bigger or smaller wheels depending on the terrain you’re on and how much work you’re able to do.
Sampling allows you to “gear” your research so you’re less limited by the constraints of cost, time, and complexity that come with different population sizes.
It allows us to do things like carrying out exit polls during elections, map the spread and effects rates of epidemics across geographical areas, and carry out nationwide census research that provides a snapshot of society and culture.
Types of sampling
Sampling strategies in research vary widely across different disciplines and research areas, and from study to study.
There are two major types of sampling methods: probability and non-probability sampling.
- Probability sampling , also known as random sampling , is a kind of sample selection where randomisation is used instead of deliberate choice. Each member of the population has a known, non-zero chance of being selected.
- Non-probability sampling techniques are where the researcher deliberately picks items or individuals for the sample based on non-random factors such as convenience, geographic availability, or costs.
As we delve into these categories, it’s essential to understand the nuances and applications of each method to ensure that the chosen sampling strategy aligns with the research goals.
Probability sampling methods
There’s a wide range of probability sampling methods to explore and consider. Here are some of the best-known options.
1. Simple random sampling
With simple random sampling , every element in the population has an equal chance of being selected as part of the sample. It’s something like picking a name out of a hat. Simple random sampling can be done by anonymising the population – e.g. by assigning each item or person in the population a number and then picking numbers at random.
Pros: Simple random sampling is easy to do and cheap. Designed to ensure that every member of the population has an equal chance of being selected, it reduces the risk of bias compared to non-random sampling.
Cons: It offers no control for the researcher and may lead to unrepresentative groupings being picked by chance.
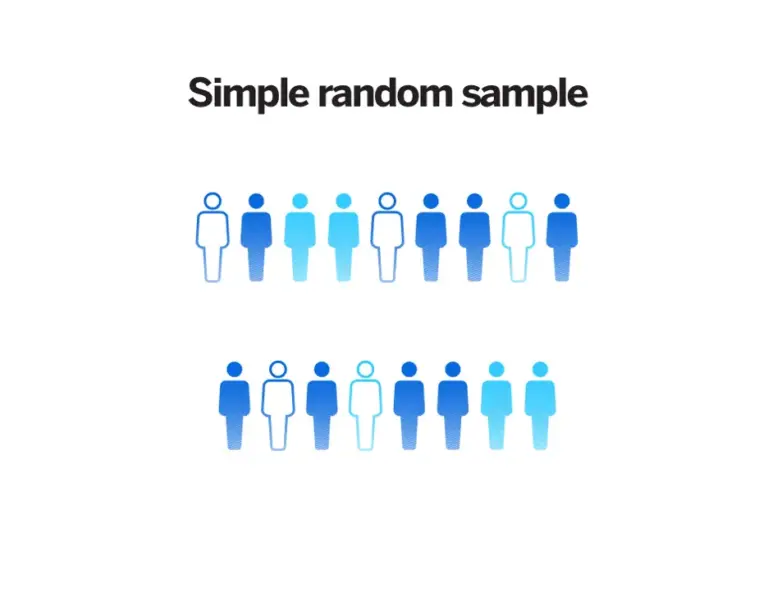
2. Systematic sampling
With systematic sampling the random selection only applies to the first item chosen. A rule then applies so that every nth item or person after that is picked.
Best practice is to sort your list in a random way to ensure that selections won’t be accidentally clustered together. This is commonly achieved using a random number generator. If that’s not available you might order your list alphabetically by first name and then pick every fifth name to eliminate bias, for example.
Next, you need to decide your sampling interval – for example, if your sample will be 10% of your full list, your sampling interval is one in 10 – and pick a random start between one and 10 – for example three. This means you would start with person number three on your list and pick every tenth person.
Pros: Systematic sampling is efficient and straightforward, especially when dealing with populations that have a clear order. It ensures a uniform selection across the population.
Cons: There’s a potential risk of introducing bias if there’s an unrecognised pattern in the population that aligns with the sampling interval.
3. Stratified sampling
Stratified sampling involves random selection within predefined groups. It’s a useful method for researchers wanting to determine what aspects of a sample are highly correlated with what’s being measured. They can then decide how to subdivide (stratify) it in a way that makes sense for the research.
For example, you want to measure the height of students at a college where 80% of students are female and 20% are male. We know that gender is highly correlated with height, and if we took a simple random sample of 200 students (out of the 2,000 who attend the college), we could by chance get 200 females and not one male. This would bias our results and we would underestimate the height of students overall. Instead, we could stratify by gender and make sure that 20% of our sample (40 students) are male and 80% (160 students) are female.
Pros: Stratified sampling enhances the representation of all identified subgroups within a population, leading to more accurate results in heterogeneous populations.
Cons: This method requires accurate knowledge about the population’s stratification, and its design and execution can be more intricate than other methods.
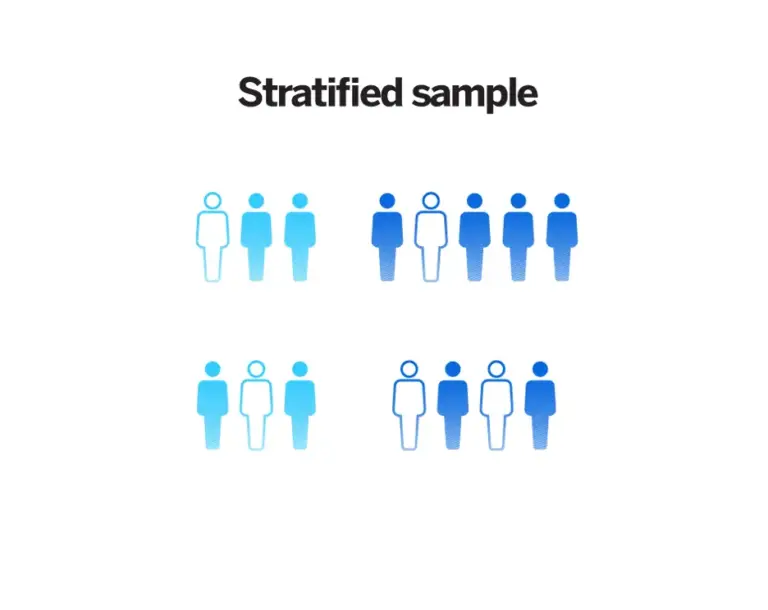
4. Cluster sampling
With cluster sampling, groups rather than individual units of the target population are selected at random for the sample. These might be pre-existing groups, such as people in certain zip codes or students belonging to an academic year.
Cluster sampling can be done by selecting the entire cluster, or in the case of two-stage cluster sampling, by randomly selecting the cluster itself, then selecting at random again within the cluster.
Pros: Cluster sampling is economically beneficial and logistically easier when dealing with vast and geographically dispersed populations.
Cons: Due to potential similarities within clusters, this method can introduce a greater sampling error compared to other methods.
Non-probability sampling methods
The non-probability sampling methodology doesn’t offer the same bias-removal benefits as probability sampling, but there are times when these types of sampling are chosen for expediency or simplicity. Here are some forms of non-probability sampling and how they work.
1. Convenience sampling
People or elements in a sample are selected on the basis of their accessibility and availability. If you are doing a research survey and you work at a university, for example, a convenience sample might consist of students or co-workers who happen to be on campus with open schedules who are willing to take your questionnaire .
This kind of sample can have value, especially if it’s done as an early or preliminary step, but significant bias will be introduced.
Pros: Convenience sampling is the most straightforward method, requiring minimal planning, making it quick to implement.
Cons: Due to its non-random nature, the method is highly susceptible to biases, and the results are often lacking in their application to the real world.
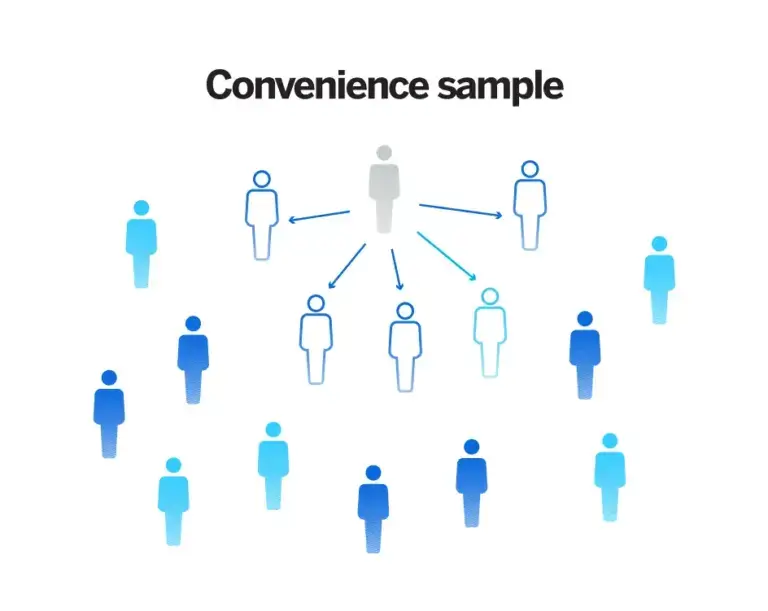
2. Quota sampling
Like the probability-based stratified sampling method, this approach aims to achieve a spread across the target population by specifying who should be recruited for a survey according to certain groups or criteria.
For example, your quota might include a certain number of males and a certain number of females. Alternatively, you might want your samples to be at a specific income level or in certain age brackets or ethnic groups.
Pros: Quota sampling ensures certain subgroups are adequately represented, making it great for when random sampling isn’t feasible but representation is necessary.
Cons: The selection within each quota is non-random and researchers’ discretion can influence the representation, which both strongly increase the risk of bias.
3. Purposive sampling
Participants for the sample are chosen consciously by researchers based on their knowledge and understanding of the research question at hand or their goals.
Also known as judgment sampling, this technique is unlikely to result in a representative sample , but it is a quick and fairly easy way to get a range of results or responses.
Pros: Purposive sampling targets specific criteria or characteristics, making it ideal for studies that require specialised participants or specific conditions.
Cons: It’s highly subjective and based on researchers’ judgment, which can introduce biases and limit the study’s real-world application.
4. Snowball or referral sampling
With this approach, people recruited to be part of a sample are asked to invite those they know to take part, who are then asked to invite their friends and family and so on. The participation radiates through a community of connected individuals like a snowball rolling downhill.
Pros: Especially useful for hard-to-reach or secretive populations, snowball sampling is effective for certain niche studies.
Cons: The method can introduce bias due to the reliance on participant referrals, and the choice of initial seeds can significantly influence the final sample.
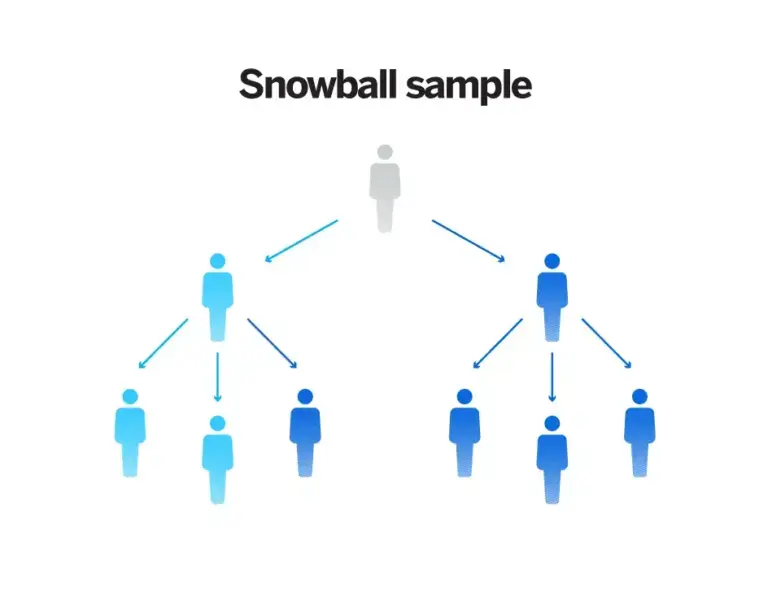
What type of sampling should I use?
Choosing the right sampling method is a pivotal aspect of any research process, but it can be a stumbling block for many.
Here’s a structured approach to guide your decision.
1) Define your research goals
If you aim to get a general sense of a larger group, simple random or stratified sampling could be your best bet. For focused insights or studying unique communities, snowball or purposive sampling might be more suitable.
2) Assess the nature of your population
The nature of the group you’re studying can guide your method. For a diverse group with different categories, stratified sampling can ensure all segments are covered. If they’re widely spread geographically , cluster sampling becomes useful. If they’re arranged in a certain sequence or order, systematic sampling might be effective.
3) Consider your constraints
Your available time, budget and ease of accessing participants matter. Convenience or quota sampling can be practical for quicker studies, but they come with some trade-offs. If reaching everyone in your desired group is challenging, snowball or purposive sampling can be more feasible.
4) Determine the reach of your findings
Decide if you want your findings to represent a much broader group. For a wider representation, methods that include everyone fairly (like probability sampling ) are a good option. For specialised insights into specific groups, non-probability sampling methods can be more suitable.
5) Get feedback
Before fully committing, discuss your chosen method with others in your field and consider a test run.
Avoid or reduce sampling errors and bias
Using a sample is a kind of short-cut. If you could ask every single person in a population to take part in your study and have each of them reply, you’d have a highly accurate (and very labor-intensive) project on your hands.
But since that’s not realistic, sampling offers a “good-enough” solution that sacrifices some accuracy for the sake of practicality and ease. How much accuracy you lose out on depends on how well you control for sampling error, non-sampling error, and bias in your survey design . Our blog post helps you to steer clear of some of these issues.
How to choose the correct sample size
Finding the best sample size for your target population is something you’ll need to do again and again, as it’s different for every study.
To make life easier, we’ve provided a sample size calculator . To use it, you need to know your:
- Population size
- Confidence level
- Margin of error (confidence interval)
If any of those terms are unfamiliar, have a look at our blog post on determining sample size for details of what they mean and how to find them.
Unlock the insights of yesterday to shape tomorrow
In the ever-evolving business landscape, relying on the most recent market research is paramount. Reflecting on 2022, brands and businesses can harness crucial insights to outmaneuver challenges and seize opportunities.
Equip yourself with this knowledge by exploring Qualtrics’ ‘2022 Market Research Global Trends’ report.
Delve into this comprehensive study to unearth:
- How businesses made sense of tricky situations in 2022
- Tips that really helped improve research results
- Steps to take your findings and put them into action
Find out how Qualtrics XM can help you conduct world-class research
Related resources
Sampling and non-sampling errors 10 min read, how to determine sample size 16 min read, convenience sampling 15 min read, non-probability sampling 17 min read, probability sampling 8 min read, stratified random sampling 13 min read, simple random sampling 10 min read, request demo.
Ready to learn more about Qualtrics?

An official website of the United States government
The .gov means it’s official. Federal government websites often end in .gov or .mil. Before sharing sensitive information, make sure you’re on a federal government site.
The site is secure. The https:// ensures that you are connecting to the official website and that any information you provide is encrypted and transmitted securely.
- Publications
- Account settings
Preview improvements coming to the PMC website in October 2024. Learn More or Try it out now .
- Advanced Search
- Journal List
- An Bras Dermatol
- v.91(3); May-Jun 2016
Sampling: how to select participants in my research study? *
Jeovany martínez-mesa.
1 Faculdade Meridional (IMED) - Passo Fundo (RS), Brazil.
David Alejandro González-Chica
2 University of Adelaide - Adelaide, Australia.
Rodrigo Pereira Duquia
3 Universidade Federal de Ciências da Saúde de Porto Alegre (UFCSPA) - Porto Alegre (RS), Brazil.
Renan Rangel Bonamigo
João luiz bastos.
4 Universidade Federal de Santa Catarina (UFSC) - Florianópolis (RS), Brazil.
In this paper, the basic elements related to the selection of participants for a health research are discussed. Sample representativeness, sample frame, types of sampling, as well as the impact that non-respondents may have on results of a study are described. The whole discussion is supported by practical examples to facilitate the reader's understanding.
To introduce readers to issues related to sampling.
INTRODUCTION
The essential topics related to the selection of participants for a health research are: 1) whether to work with samples or include the whole reference population in the study (census); 2) the sample basis; 3) the sampling process and 4) the potential effects nonrespondents might have on study results. We will refer to each of these aspects with theoretical and practical examples for better understanding in the sections that follow.
TO SAMPLE OR NOT TO SAMPLE
In a previous paper, we discussed the necessary parameters on which to estimate the sample size. 1 We define sample as a finite part or subset of participants drawn from the target population. In turn, the target population corresponds to the entire set of subjects whose characteristics are of interest to the research team. Based on results obtained from a sample, researchers may draw their conclusions about the target population with a certain level of confidence, following a process called statistical inference. When the sample contains fewer individuals than the minimum necessary, but the representativeness is preserved, statistical inference may be compromised in terms of precision (prevalence studies) and/or statistical power to detect the associations of interest. 1 On the other hand, samples without representativeness may not be a reliable source to draw conclusions about the reference population (i.e., statistical inference is not deemed possible), even if the sample size reaches the required number of participants. Lack of representativeness can occur as a result of flawed selection procedures (sampling bias) or when the probability of refusal/non-participation in the study is related to the object of research (nonresponse bias). 1 , 2
Although most studies are performed using samples, whether or not they represent any target population, census-based estimates should be preferred whenever possible. 3 , 4 For instance, if all cases of melanoma are available on a national or regional database, and information on the potential risk factors are also available, it would be preferable to conduct a census instead of investigating a sample.
However, there are several theoretical and practical reasons that prevent us from carrying out census-based surveys, including:
- Ethical issues: it is unethical to include a greater number of individuals than that effectively required;
- Budgetary limitations: the high costs of a census survey often limits its use as a strategy to select participants for a study;
- Logistics: censuses often impose great challenges in terms of required staff, equipment, etc. to conduct the study;
- Time restrictions: the amount of time needed to plan and conduct a census-based survey may be excessive; and,
- Unknown target population size: if the study objective is to investigate the presence of premalignant skin lesions in illicit drugs users, lack of information on all existing users makes it impossible to conduct a census-based study.
All these reasons explain why samples are more frequently used. However, researchers must be aware that sample results can be affected by the random error (or sampling error). 3 To exemplify this concept, we will consider a research study aiming to estimate the prevalence of premalignant skin lesions (outcome) among individuals >18 years residing in a specific city (target population). The city has a total population of 4,000 adults, but the investigator decided to collect data on a representative sample of 400 participants, detecting an 8% prevalence of premalignant skin lesions. A week later, the researcher selects another sample of 400 participants from the same target population to confirm the results, but this time observes a 12% prevalence of premalignant skin lesions. Based on these findings, is it possible to assume that the prevalence of lesions increased from the first to the second week? The answer is probably not. Each time we select a new sample, it is very likely to obtain a different result. These fluctuations are attributed to the "random error." They occur because individuals composing different samples are not the same, even though they were selected from the same target population. Therefore, the parameters of interest may vary randomly from one sample to another. Despite this fluctuation, if it were possible to obtain 100 different samples of the same population, approximately 95 of them would provide prevalence estimates very close to the real estimate in the target population - the value that we would observe if we investigated all the 4,000 adults residing in the city. Thus, during the sample size estimation the investigator must specify in advance the highest or maximum acceptable random error value in the study. Most population-based studies use a random error ranging from 2 to 5 percentage points. Nevertheless, the researcher should be aware that the smaller the random error considered in the study, the larger the required sample size. 1
SAMPLE FRAME
The sample frame is the group of individuals that can be selected from the target population given the sampling process used in the study. For example, to identify cases of cutaneous melanoma the researcher may consider to utilize as sample frame the national cancer registry system or the anatomopathological records of skin biopsies. Given that the sample may represent only a portion of the target population, the researcher needs to examine carefully whether the selected sample frame fits the study objectives or hypotheses, and especially if there are strategies to overcome the sample frame limitations (see Chart 1 for examples and possible limitations).
Examples of sample frames and potential limitations as regards representativeness
Sample frames | Limitations |
---|---|
Population census | • If the census was not conducted in recent years, areas with high migration might be outdated |
• Homeless or itinerant people cannot be represented | |
Hospital or Health Services records | • Usually include only data of affected people (this is a limitation, depending on the study objectives) |
• Depending on the service, data may be incomplete and/or outdated | |
• If the lists are from public units, results may differ from those who seek private services | |
School lists | • School lists are currently available only in the public sector |
• Children/ teenagers not attending school will not be represented | |
• Lists are quickly outdated | |
• There will be problems in areas with high percentage of school absenteeism | |
List of phone numbers | • Several population groups are not represented: individuals with no phone line at home (low-income families, young people who use only cell phones), those who spend less time at home, etc. |
Mailing lists | • Individuals with multiple email addresses, which increase the chance of selection compared to individuals with only one address |
• Individuals without an email address may be different from those who have it, according to age, education, etc. |
Sampling can be defined as the process through which individuals or sampling units are selected from the sample frame. The sampling strategy needs to be specified in advance, given that the sampling method may affect the sample size estimation. 1 , 5 Without a rigorous sampling plan the estimates derived from the study may be biased (selection bias). 3
TYPES OF SAMPLING
In figure 1 , we depict a summary of the main sampling types. There are two major sampling types: probabilistic and nonprobabilistic.

Sampling types used in scientific studies
NONPROBABILISTIC SAMPLING
In the context of nonprobabilistic sampling, the likelihood of selecting some individuals from the target population is null. This type of sampling does not render a representative sample; therefore, the observed results are usually not generalizable to the target population. Still, unrepresentative samples may be useful for some specific research objectives, and may help answer particular research questions, as well as contribute to the generation of new hypotheses. 4 The different types of nonprobabilistic sampling are detailed below.
Convenience sampling : the participants are consecutively selected in order of apperance according to their convenient accessibility (also known as consecutive sampling). The sampling process comes to an end when the total amount of participants (sample saturation) and/or the time limit (time saturation) are reached. Randomized clinical trials are usually based on convenience sampling. After sampling, participants are usually randomly allocated to the intervention or control group (randomization). 3 Although randomization is a probabilistic process to obtain two comparable groups (treatment and control), the samples used in these studies are generally not representative of the target population.
Purposive sampling: this is used when a diverse sample is necessary or the opinion of experts in a particular field is the topic of interest. This technique was used in the study by Roubille et al, in which recommendations for the treatment of comorbidities in patients with rheumatoid arthritis, psoriasis, and psoriatic arthritis were made based on the opinion of a group of experts. 6
Quota sampling: according to this sampling technique, the population is first classified by characteristics such as gender, age, etc. Subsequently, sampling units are selected to complete each quota. For example, in the study by Larkin et al., the combination of vemurafenib and cobimetinib versus placebo was tested in patients with locally-advanced melanoma, stage IIIC or IV, with BRAF mutation. 7 The study recruited 495 patients from 135 health centers located in several countries. In this type of study, each center has a "quota" of patients.
"Snowball" sampling : in this case, the researcher selects an initial group of individuals. Then, these participants indicate other potential members with similar characteristics to take part in the study. This is frequently used in studies investigating special populations, for example, those including illicit drugs users, as was the case of the study by Gonçalves et al, which assessed 27 users of cocaine and crack in combination with marijuana. 8
PROBABILISTIC SAMPLING
In the context of probabilistic sampling, all units of the target population have a nonzero probability to take part in the study. If all participants are equally likely to be selected in the study, equiprobabilistic sampling is being used, and the odds of being selected by the research team may be expressed by the formula: P=1/N, where P equals the probability of taking part in the study and N corresponds to the size of the target population. The main types of probabilistic sampling are described below.
Simple random sampling: in this case, we have a full list of sample units or participants (sample basis), and we randomly select individuals using a table of random numbers. An example is the study by Pimenta et al, in which the authors obtained a listing from the Health Department of all elderly enrolled in the Family Health Strategy and, by simple random sampling, selected a sample of 449 participants. 9
Systematic random sampling: in this case, participants are selected from fixed intervals previously defined from a ranked list of participants. For example, in the study of Kelbore et al, children who were assisted at the Pediatric Dermatology Service were selected to evaluate factors associated with atopic dermatitis, selecting always the second child by consulting order. 10
Stratified sampling: in this type of sampling, the target population is first divided into separate strata. Then, samples are selected within each stratum, either through simple or systematic sampling. The total number of individuals to be selected in each stratum can be fixed or proportional to the size of each stratum. Each individual may be equally likely to be selected to participate in the study. However, the fixed method usually involves the use of sampling weights in the statistical analysis (inverse of the probability of selection or 1/P). An example is the study conducted in South Australia to investigate factors associated with vitamin D deficiency in preschool children. Using the national census as the sample frame, households were randomly selected in each stratum and all children in the age group of interest identified in the selected houses were investigated. 11
Cluster sampling: in this type of probabilistic sampling, groups such as health facilities, schools, etc., are sampled. In the above-mentioned study, the selection of households is an example of cluster sampling. 11
Complex or multi-stage sampling: This probabilistic sampling method combines different strategies in the selection of the sample units. An example is the study of Duquia et al. to assess the prevalence and factors associated with the use of sunscreen in adults. The sampling process included two stages. 12 Using the 2000 Brazilian demographic census as sampling frame, all 404 census tracts from Pelotas (Southern Brazil) were listed in ascending order of family income. A sample of 120 tracts were systematically selected (first sampling stage units). In the second stage, 12 households in each of these census tract (second sampling stage units) were systematically drawn. All adult residents in these households were included in the study (third sampling stage units). All these stages have to be considered in the statistical analysis to provide correct estimates.
NONRESPONDENTS
Frequently, sample sizes are increased by 10% to compensate for potential nonresponses (refusals/losses). 1 Let us imagine that in a study to assess the prevalence of premalignant skin lesions there is a higher percentage of nonrespondents among men (10%) than among women (1%). If the highest percentage of nonresponse occurs because these men are not at home during the scheduled visits, and these participants are more likely to be exposed to the sun, the number of skin lesions will be underestimated. For this reason, it is strongly recommended to collect and describe some basic characteristics of nonrespondents (sex, age, etc.) so they can be compared to the respondents to evaluate whether the results may have been affected by this systematic error.
Often, in study protocols, refusal to participate or sign the informed consent is considered an "exclusion criteria". However, this is not correct, as these individuals are eligible for the study and need to be reported as "nonrespondents".
SAMPLING METHOD ACCORDING TO THE TYPE OF STUDY
In general, clinical trials aim to obtain a homogeneous sample which is not necessarily representative of any target population. Clinical trials often recruit those participants who are most likely to benefit from the intervention. 3 Thus, the more strict criteria for inclusion and exclusion of subjects in clinical trials often make it difficult to locate participants: after verification of the eligibility criteria, just one out of ten possible candidates will enter the study. Therefore, clinical trials usually show limitations to generalize the results to the entire population of patients with the disease, but only to those with similar characteristics to the sample included in the study. These peculiarities in clinical trials justify the necessity of conducting a multicenter and/or global studiesto accelerate the recruitment rate and to reach, in a shorter time, the number of patients required for the study. 13
In turn, in observational studies to build a solid sampling plan is important because of the great heterogeneity usually observed in the target population. Therefore, this heterogeneity has to be also reflected in the sample. A cross-sectional population-based study aiming to assess disease estimates or identify risk factors often uses complex probabilistic sampling, because the sample representativeness is crucial. However, in a case-control study, we face the challenge of selecting two different samples for the same study. One sample is formed by the cases, which are identified based on the diagnosis of the disease of interest. The other consists of controls, which need to be representative of the population that originated the cases. Improper selection of control individuals may introduce selection bias in the results. Thus, the concern with representativeness in this type of study is established based on the relationship between cases and controls (comparability).
In cohort studies, individuals are recruited based on the exposure (exposed and unexposed subjects), and they are followed over time to evaluate the occurrence of the outcome of interest. At baseline, the sample can be selected from a representative sample (population-based cohort studies) or a non-representative sample. However, in the successive follow-ups of the cohort member, study participants must be a representative sample of those included in the baseline. 14 , 15 In this type of study, losses over time may cause follow-up bias.
Researchers need to decide during the planning stage of the study if they will work with the entire target population or a sample. Working with a sample involves different steps, including sample size estimation, identification of the sample frame, and selection of the sampling method to be adopted.
Financial Support: None.
* Study performed at Faculdade Meridional - Escola de Medicina (IMED) - Passo Fundo (RS), Brazil.
Have a language expert improve your writing
Run a free plagiarism check in 10 minutes, generate accurate citations for free.
- Knowledge Base
Methodology
- What Is a Research Design | Types, Guide & Examples
What Is a Research Design | Types, Guide & Examples
Published on June 7, 2021 by Shona McCombes . Revised on November 20, 2023 by Pritha Bhandari.
A research design is a strategy for answering your research question using empirical data. Creating a research design means making decisions about:
- Your overall research objectives and approach
- Whether you’ll rely on primary research or secondary research
- Your sampling methods or criteria for selecting subjects
- Your data collection methods
- The procedures you’ll follow to collect data
- Your data analysis methods
A well-planned research design helps ensure that your methods match your research objectives and that you use the right kind of analysis for your data.
Table of contents
Step 1: consider your aims and approach, step 2: choose a type of research design, step 3: identify your population and sampling method, step 4: choose your data collection methods, step 5: plan your data collection procedures, step 6: decide on your data analysis strategies, other interesting articles, frequently asked questions about research design.
- Introduction
Before you can start designing your research, you should already have a clear idea of the research question you want to investigate.
There are many different ways you could go about answering this question. Your research design choices should be driven by your aims and priorities—start by thinking carefully about what you want to achieve.
The first choice you need to make is whether you’ll take a qualitative or quantitative approach.
Qualitative approach | Quantitative approach |
---|---|
and describe frequencies, averages, and correlations about relationships between variables |
Qualitative research designs tend to be more flexible and inductive , allowing you to adjust your approach based on what you find throughout the research process.
Quantitative research designs tend to be more fixed and deductive , with variables and hypotheses clearly defined in advance of data collection.
It’s also possible to use a mixed-methods design that integrates aspects of both approaches. By combining qualitative and quantitative insights, you can gain a more complete picture of the problem you’re studying and strengthen the credibility of your conclusions.
Practical and ethical considerations when designing research
As well as scientific considerations, you need to think practically when designing your research. If your research involves people or animals, you also need to consider research ethics .
- How much time do you have to collect data and write up the research?
- Will you be able to gain access to the data you need (e.g., by travelling to a specific location or contacting specific people)?
- Do you have the necessary research skills (e.g., statistical analysis or interview techniques)?
- Will you need ethical approval ?
At each stage of the research design process, make sure that your choices are practically feasible.
Here's why students love Scribbr's proofreading services
Discover proofreading & editing
Within both qualitative and quantitative approaches, there are several types of research design to choose from. Each type provides a framework for the overall shape of your research.
Types of quantitative research designs
Quantitative designs can be split into four main types.
- Experimental and quasi-experimental designs allow you to test cause-and-effect relationships
- Descriptive and correlational designs allow you to measure variables and describe relationships between them.
Type of design | Purpose and characteristics |
---|---|
Experimental | relationships effect on a |
Quasi-experimental | ) |
Correlational | |
Descriptive |
With descriptive and correlational designs, you can get a clear picture of characteristics, trends and relationships as they exist in the real world. However, you can’t draw conclusions about cause and effect (because correlation doesn’t imply causation ).
Experiments are the strongest way to test cause-and-effect relationships without the risk of other variables influencing the results. However, their controlled conditions may not always reflect how things work in the real world. They’re often also more difficult and expensive to implement.
Types of qualitative research designs
Qualitative designs are less strictly defined. This approach is about gaining a rich, detailed understanding of a specific context or phenomenon, and you can often be more creative and flexible in designing your research.
The table below shows some common types of qualitative design. They often have similar approaches in terms of data collection, but focus on different aspects when analyzing the data.
Type of design | Purpose and characteristics |
---|---|
Grounded theory | |
Phenomenology |
Your research design should clearly define who or what your research will focus on, and how you’ll go about choosing your participants or subjects.
In research, a population is the entire group that you want to draw conclusions about, while a sample is the smaller group of individuals you’ll actually collect data from.
Defining the population
A population can be made up of anything you want to study—plants, animals, organizations, texts, countries, etc. In the social sciences, it most often refers to a group of people.
For example, will you focus on people from a specific demographic, region or background? Are you interested in people with a certain job or medical condition, or users of a particular product?
The more precisely you define your population, the easier it will be to gather a representative sample.
- Sampling methods
Even with a narrowly defined population, it’s rarely possible to collect data from every individual. Instead, you’ll collect data from a sample.
To select a sample, there are two main approaches: probability sampling and non-probability sampling . The sampling method you use affects how confidently you can generalize your results to the population as a whole.
Probability sampling | Non-probability sampling |
---|---|
Probability sampling is the most statistically valid option, but it’s often difficult to achieve unless you’re dealing with a very small and accessible population.
For practical reasons, many studies use non-probability sampling, but it’s important to be aware of the limitations and carefully consider potential biases. You should always make an effort to gather a sample that’s as representative as possible of the population.
Case selection in qualitative research
In some types of qualitative designs, sampling may not be relevant.
For example, in an ethnography or a case study , your aim is to deeply understand a specific context, not to generalize to a population. Instead of sampling, you may simply aim to collect as much data as possible about the context you are studying.
In these types of design, you still have to carefully consider your choice of case or community. You should have a clear rationale for why this particular case is suitable for answering your research question .
For example, you might choose a case study that reveals an unusual or neglected aspect of your research problem, or you might choose several very similar or very different cases in order to compare them.
Data collection methods are ways of directly measuring variables and gathering information. They allow you to gain first-hand knowledge and original insights into your research problem.
You can choose just one data collection method, or use several methods in the same study.
Survey methods
Surveys allow you to collect data about opinions, behaviors, experiences, and characteristics by asking people directly. There are two main survey methods to choose from: questionnaires and interviews .
Questionnaires | Interviews |
---|---|
) |
Observation methods
Observational studies allow you to collect data unobtrusively, observing characteristics, behaviors or social interactions without relying on self-reporting.
Observations may be conducted in real time, taking notes as you observe, or you might make audiovisual recordings for later analysis. They can be qualitative or quantitative.
Quantitative observation | |
---|---|
Other methods of data collection
There are many other ways you might collect data depending on your field and topic.
Field | Examples of data collection methods |
---|---|
Media & communication | Collecting a sample of texts (e.g., speeches, articles, or social media posts) for data on cultural norms and narratives |
Psychology | Using technologies like neuroimaging, eye-tracking, or computer-based tasks to collect data on things like attention, emotional response, or reaction time |
Education | Using tests or assignments to collect data on knowledge and skills |
Physical sciences | Using scientific instruments to collect data on things like weight, blood pressure, or chemical composition |
If you’re not sure which methods will work best for your research design, try reading some papers in your field to see what kinds of data collection methods they used.
Secondary data
If you don’t have the time or resources to collect data from the population you’re interested in, you can also choose to use secondary data that other researchers already collected—for example, datasets from government surveys or previous studies on your topic.
With this raw data, you can do your own analysis to answer new research questions that weren’t addressed by the original study.
Using secondary data can expand the scope of your research, as you may be able to access much larger and more varied samples than you could collect yourself.
However, it also means you don’t have any control over which variables to measure or how to measure them, so the conclusions you can draw may be limited.
Prevent plagiarism. Run a free check.
As well as deciding on your methods, you need to plan exactly how you’ll use these methods to collect data that’s consistent, accurate, and unbiased.
Planning systematic procedures is especially important in quantitative research, where you need to precisely define your variables and ensure your measurements are high in reliability and validity.
Operationalization
Some variables, like height or age, are easily measured. But often you’ll be dealing with more abstract concepts, like satisfaction, anxiety, or competence. Operationalization means turning these fuzzy ideas into measurable indicators.
If you’re using observations , which events or actions will you count?
If you’re using surveys , which questions will you ask and what range of responses will be offered?
You may also choose to use or adapt existing materials designed to measure the concept you’re interested in—for example, questionnaires or inventories whose reliability and validity has already been established.
Reliability and validity
Reliability means your results can be consistently reproduced, while validity means that you’re actually measuring the concept you’re interested in.
Reliability | Validity |
---|---|
) ) |
For valid and reliable results, your measurement materials should be thoroughly researched and carefully designed. Plan your procedures to make sure you carry out the same steps in the same way for each participant.
If you’re developing a new questionnaire or other instrument to measure a specific concept, running a pilot study allows you to check its validity and reliability in advance.
Sampling procedures
As well as choosing an appropriate sampling method , you need a concrete plan for how you’ll actually contact and recruit your selected sample.
That means making decisions about things like:
- How many participants do you need for an adequate sample size?
- What inclusion and exclusion criteria will you use to identify eligible participants?
- How will you contact your sample—by mail, online, by phone, or in person?
If you’re using a probability sampling method , it’s important that everyone who is randomly selected actually participates in the study. How will you ensure a high response rate?
If you’re using a non-probability method , how will you avoid research bias and ensure a representative sample?
Data management
It’s also important to create a data management plan for organizing and storing your data.
Will you need to transcribe interviews or perform data entry for observations? You should anonymize and safeguard any sensitive data, and make sure it’s backed up regularly.
Keeping your data well-organized will save time when it comes to analyzing it. It can also help other researchers validate and add to your findings (high replicability ).
On its own, raw data can’t answer your research question. The last step of designing your research is planning how you’ll analyze the data.
Quantitative data analysis
In quantitative research, you’ll most likely use some form of statistical analysis . With statistics, you can summarize your sample data, make estimates, and test hypotheses.
Using descriptive statistics , you can summarize your sample data in terms of:
- The distribution of the data (e.g., the frequency of each score on a test)
- The central tendency of the data (e.g., the mean to describe the average score)
- The variability of the data (e.g., the standard deviation to describe how spread out the scores are)
The specific calculations you can do depend on the level of measurement of your variables.
Using inferential statistics , you can:
- Make estimates about the population based on your sample data.
- Test hypotheses about a relationship between variables.
Regression and correlation tests look for associations between two or more variables, while comparison tests (such as t tests and ANOVAs ) look for differences in the outcomes of different groups.
Your choice of statistical test depends on various aspects of your research design, including the types of variables you’re dealing with and the distribution of your data.
Qualitative data analysis
In qualitative research, your data will usually be very dense with information and ideas. Instead of summing it up in numbers, you’ll need to comb through the data in detail, interpret its meanings, identify patterns, and extract the parts that are most relevant to your research question.
Two of the most common approaches to doing this are thematic analysis and discourse analysis .
Approach | Characteristics |
---|---|
Thematic analysis | |
Discourse analysis |
There are many other ways of analyzing qualitative data depending on the aims of your research. To get a sense of potential approaches, try reading some qualitative research papers in your field.
If you want to know more about the research process , methodology , research bias , or statistics , make sure to check out some of our other articles with explanations and examples.
- Simple random sampling
- Stratified sampling
- Cluster sampling
- Likert scales
- Reproducibility
Statistics
- Null hypothesis
- Statistical power
- Probability distribution
- Effect size
- Poisson distribution
Research bias
- Optimism bias
- Cognitive bias
- Implicit bias
- Hawthorne effect
- Anchoring bias
- Explicit bias
A research design is a strategy for answering your research question . It defines your overall approach and determines how you will collect and analyze data.
A well-planned research design helps ensure that your methods match your research aims, that you collect high-quality data, and that you use the right kind of analysis to answer your questions, utilizing credible sources . This allows you to draw valid , trustworthy conclusions.
Quantitative research designs can be divided into two main categories:
- Correlational and descriptive designs are used to investigate characteristics, averages, trends, and associations between variables.
- Experimental and quasi-experimental designs are used to test causal relationships .
Qualitative research designs tend to be more flexible. Common types of qualitative design include case study , ethnography , and grounded theory designs.
The priorities of a research design can vary depending on the field, but you usually have to specify:
- Your research questions and/or hypotheses
- Your overall approach (e.g., qualitative or quantitative )
- The type of design you’re using (e.g., a survey , experiment , or case study )
- Your data collection methods (e.g., questionnaires , observations)
- Your data collection procedures (e.g., operationalization , timing and data management)
- Your data analysis methods (e.g., statistical tests or thematic analysis )
A sample is a subset of individuals from a larger population . Sampling means selecting the group that you will actually collect data from in your research. For example, if you are researching the opinions of students in your university, you could survey a sample of 100 students.
In statistics, sampling allows you to test a hypothesis about the characteristics of a population.
Operationalization means turning abstract conceptual ideas into measurable observations.
For example, the concept of social anxiety isn’t directly observable, but it can be operationally defined in terms of self-rating scores, behavioral avoidance of crowded places, or physical anxiety symptoms in social situations.
Before collecting data , it’s important to consider how you will operationalize the variables that you want to measure.
A research project is an academic, scientific, or professional undertaking to answer a research question . Research projects can take many forms, such as qualitative or quantitative , descriptive , longitudinal , experimental , or correlational . What kind of research approach you choose will depend on your topic.
Cite this Scribbr article
If you want to cite this source, you can copy and paste the citation or click the “Cite this Scribbr article” button to automatically add the citation to our free Citation Generator.
McCombes, S. (2023, November 20). What Is a Research Design | Types, Guide & Examples. Scribbr. Retrieved June 18, 2024, from https://www.scribbr.com/methodology/research-design/
Is this article helpful?
Shona McCombes
Other students also liked, guide to experimental design | overview, steps, & examples, how to write a research proposal | examples & templates, ethical considerations in research | types & examples, get unlimited documents corrected.
✔ Free APA citation check included ✔ Unlimited document corrections ✔ Specialized in correcting academic texts
Marketing91
What is Sampling plan and its application in Market research?
June 12, 2023 | By Hitesh Bhasin | Filed Under: Marketing
Once you are ready with your market research plan, then comes the implementation part. And the first step of implementation is determining your sampling plan.
A sampling plan basically comprises of different sample units or sample population whom you are going to contact to collect market research data . This sampling unit is a representative of the total population, though it might be a fraction of the total population.
In simple language, if you have 1 lakh customers, you cannot conduct an interview of 1 lakh customers. Instead, you take a sample population of 1000 customers (1 % of your total population). This sample gives you primary data and this is assumed to suit 99% of your customers. Naturally, the 1% whom you have interviewed need to be important to your company . And hence the need of a sampling plan.
There are four steps to making a Sampling plan for Market research .
Table of Contents
1) Define the sample population
More commonly known as the Sample unit, it comprises of the type of customers / people that you want to contact for your market research study. To determine the sample population, first you need to decide what the ideal customer for the firm looks like.
If yours is a restaurant, you will like your sample population to comprise of people who have visited at least 5 times to your restaurant. They will be a fair judge of things you can improve on. Or on the other hand, you can interview people who are just walking in, if you want to improve the ambiance of the restaurant and note down their ideas .
Overall, you need to understand that you cannot interview 100% of your customers. Hence the Sample population will be a small population which will be extrapolated later on. So this step is important and you need to choose your customers with care. They should be a strong representative of the type of business you want to become.
2) Define the size of the population
In the above example of a restaurant, the market research can have 1 of two objectives
- To find out what makes old customers happy
- To improve the ambiance of the restaurant which can be suggested by new people entering the restaurant.
In the above example, in Case A, you need to approach a hand picked customer base who have visited your restaurant time and time again and you need to implement what they say. This customer base can be anywhere between 50 to 200 of your most valuable customers.
In case B, where you want to improve the ambiance of the restaurant, every new comer who is new to the restaurant will have a different suggestion. And the research will be endless, so you can put a questionnaire right at the table for things the customer will like to improve.
Thus as you can see, the sampling size and the size of the population in the sampling plan changes as per the objective of the market research . If the objective is an ongoing objective to make something better all the time, then the population will be large. If it is something you need to determine within a given time period, then the population will be less but it should be important.
Once your Sampling size is decided you can decide on the contact method for your sampling plan.
3) What type of contact options are you using
There are many options in Market research which can be used time and time again to carry out primary data collection . These options include questionnaires, mailers, telephonic interviews and whatnot.
Your contact options depend on your sampling size and your sampling units. If your customers are the busy types and there are a handful of them, then personal interviews with an appointment will serve you perfectly. However, if your customers keep coming and there is a large population of them, then it is manually not possible to touch each and every one personally.
So your sampling plan and its contact method will depend on the size of the population you are going to contact. Mind you, large companies still prefer contacting their customers personally to get personal feedback from them. But smaller companies have various options such as telephonic interview or Forms and questionnaires or mailers to get the work done smoothly .
4) Form a sampling frame
So once you have the sampling units, the sample size and the population you are going to contact, you decide on the contact plan. You need to put it on paper whom you are going to contact when. A market research study might take a single day (in which case you dont need a sampling frame) or it may take months (in which case you definitely need a sampling frame).
Think of a sampling frame as an organizer. If you are too busy, then you are better off with an organizer in your sampling plan. However, if the work is going to get done quickly, then you dont need the sampling frame at all. If you expect facing any problems or questions when the process starts, then you can prepare the sampling frame to answer such questions. It can be the FAQ of your market research plan.
How are you going to analyse the results? – In the sampling plan, you need to decide on the analysis part as well. There are two ways to analyse the results of a Market research study
a) Probabilistic sampling – Most likely to be used for quantitative research, it can pin point sampling errors and therefore gives a correct data. However, it is heavy on time consumed for analysis. It uses mainly an objective questionnaire with proper “Yes / No” type questions and statistical answers.
b) Non probability sampling – It is used mainly for qualitative research wherein you can marketing and customer insights as it is not data based but more based on the quality of the answers. This is more of a subjective questionnaire then objective.
The type of analysis you are going to carry out (probability or non probability) has to be determined in the sampling plan because you need to contact the customers accordingly. Do note, that this has to be incorporated in your market research study so you need to decide your population accordingly as well.
Overall, once you are done with the 5 steps of sampling plan given above, then you are done with the following process
- Who are the customers you are going to contact
- How many customers you are going to contact
- How will you contact the customers
- What is the time frame or the contact frame for getting in touch with customers
- What is the analysis method you are going to use later on.
Once you do the above, you are ready with your sampling plan.
Here is a video by Marketing91 on Sampling.
Liked this post? Check out the complete series on Market research
Related posts:
- Intensive distribution and its application in business
- Usage based segmentation and its application in Marketing
- Geographical pricing and its application in Marketing
- Odd Even Pricing and its application in Marketing
- Cold Canvassing and its Application in Marketing
- What is Survey Research? Objectives, Sampling Process, Types and Advantages
- What is Product Sampling? Types, Methods & Tips
- Quota Sampling – Definition, Meaning, Advantages, Disadvantages
- Convenience Sampling | How to analyze a convenience sample?
- Sampling and Sample Design – Types and Steps Involved
About Hitesh Bhasin
Hitesh Bhasin is the CEO of Marketing91 and has over a decade of experience in the marketing field. He is an accomplished author of thousands of insightful articles, including in-depth analyses of brands and companies. Holding an MBA in Marketing, Hitesh manages several offline ventures, where he applies all the concepts of Marketing that he writes about.
All Knowledge Banks (Hub Pages)
- Marketing Hub
- Management Hub
- Marketing Strategy
- Advertising Hub
- Branding Hub
- Market Research
- Small Business Marketing
- Sales and Selling
- Marketing Careers
- Internet Marketing
- Business Model of Brands
- Marketing Mix of Brands
- Brand Competitors
- Strategy of Brands
- SWOT of Brands
- Customer Management
- Top 10 Lists
Leave a Reply Cancel reply
Your email address will not be published. Required fields are marked *
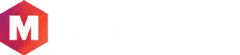
- About Marketing91
- Marketing91 Team
- Privacy Policy
- Cookie Policy
- Terms of Use
- Editorial Policy
WE WRITE ON
- Digital Marketing
- Human Resources
- Operations Management
- Marketing News
- Marketing mix's
- Competitors

An official website of the United States government
Here’s how you know
Official websites use .gov A .gov website belongs to an official government organization in the United States.
Secure .gov websites use HTTPS A lock ( Lock A locked padlock ) or https:// means you’ve safely connected to the .gov website. Share sensitive information only on official, secure websites.
https://www.nist.gov/chips
CHIPS for America
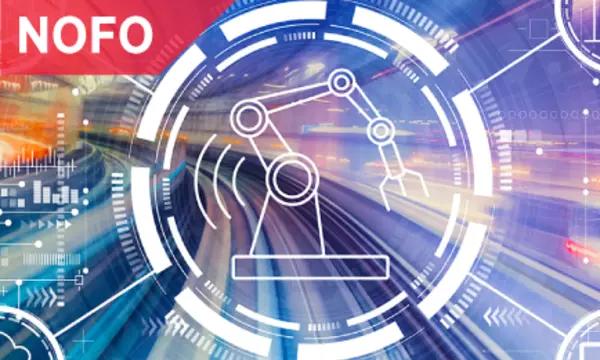
About CHIPS for America
Semiconductors, or chips, are tiny electronic devices that are integral to America’s economic and national security. These devices power tools as simple as a light switch and as complex as a fighter jet or a smartphone. Semiconductors power our consumer electronics, automobiles, data centers, critical infrastructure, and virtually all military systems. They are also essential building blocks of the technologies that will shape our future, including artificial intelligence, biotechnology, and clean energy.
While the United States remains a global leader in semiconductor design and research and development, it has fallen behind in manufacturing and now accounts for only about 10 percent of global commercial production. Today, none of the most advanced logic and memory chips—the chips that power PCs, smartphones, and supercomputers—are manufactured at commercial scale in the United States. In addition, many elements of the semiconductor supply chain are geographically concentrated, leaving them vulnerable to disruption and endangering the global economy and U.S. national security.
That’s why President Biden signed the bipartisan CHIPS and Science Act of 2022 into law. The law provides the Department of Commerce with $50 billion for a suite of programs to strengthen and revitalize the U.S. position in semiconductor research, development, and manufacturing—while also investing in American workers. CHIPS for America encompasses two offices responsible for implementing the law: The CHIPS Research and Development Office is investing $11 billion into developing a robust domestic R&D ecosystem, while the CHIPS Program Office is dedicating $39 billion to provide incentives for investment in facilities and equipment in the United States. Learn more about CHIPS for America from this video message from the Secretary of Commerce .
News and Press Releases
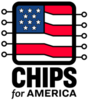
U.S. Department of Commerce Announces Two New Voluntary Company Commitments to the CHIPS Women in Construction Framework
Biden-harris administration announces preliminary terms with rocket lab to expand production of compound semiconductors that power spacecrafts and satellites, biden-harris administration announces preliminary terms with absolics to support development of glass substrate technology for semiconductor advanced packaging, biden-harris administration announces preliminary terms with polar semiconductor to establish an independent american foundry.
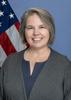
Marla Dowell Recognized as a Distinguished Executive with 2023 Presidential Rank Award
For general inquiries about CHIPS for America, contact askchips [at] chips.gov (askchips[at]chips[dot]gov) .
For inquiries about the CHIPS Incentives Program, contact apply [at] chips.gov .
For Congressional inquiries about CHIPS for America, contact legislativeaffairs [at] chips.gov (legislativeaffairs[at]chips[dot]gov) .
To request a meeting with a CHIPS staff member or an appearance at an event, visit https://askchips.chips.gov .
The CHIPS Incentives Program Portal can be found at https://applications.chips.gov .
Information
- Author Services
Initiatives
You are accessing a machine-readable page. In order to be human-readable, please install an RSS reader.
All articles published by MDPI are made immediately available worldwide under an open access license. No special permission is required to reuse all or part of the article published by MDPI, including figures and tables. For articles published under an open access Creative Common CC BY license, any part of the article may be reused without permission provided that the original article is clearly cited. For more information, please refer to https://www.mdpi.com/openaccess .
Feature papers represent the most advanced research with significant potential for high impact in the field. A Feature Paper should be a substantial original Article that involves several techniques or approaches, provides an outlook for future research directions and describes possible research applications.
Feature papers are submitted upon individual invitation or recommendation by the scientific editors and must receive positive feedback from the reviewers.
Editor’s Choice articles are based on recommendations by the scientific editors of MDPI journals from around the world. Editors select a small number of articles recently published in the journal that they believe will be particularly interesting to readers, or important in the respective research area. The aim is to provide a snapshot of some of the most exciting work published in the various research areas of the journal.
Original Submission Date Received: .
- Active Journals
- Find a Journal
- Proceedings Series
- For Authors
- For Reviewers
- For Editors
- For Librarians
- For Publishers
- For Societies
- For Conference Organizers
- Open Access Policy
- Institutional Open Access Program
- Special Issues Guidelines
- Editorial Process
- Research and Publication Ethics
- Article Processing Charges
- Testimonials
- Preprints.org
- SciProfiles
- Encyclopedia
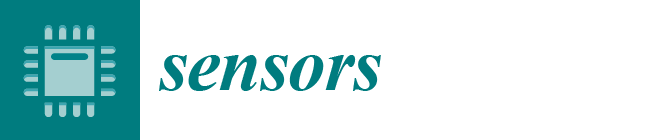
Article Menu
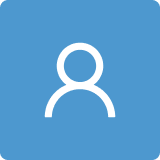
- Subscribe SciFeed
- Recommended Articles
- Google Scholar
- on Google Scholar
- Table of Contents
Find support for a specific problem in the support section of our website.
Please let us know what you think of our products and services.
Visit our dedicated information section to learn more about MDPI.
JSmol Viewer
Research on autonomous vehicle path planning algorithm based on improved rrt* algorithm and artificial potential field method.
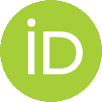
1. Introduction
2. traditional algorithm, 2.1. rrt* algorithm, 2.2. traditional artificial potential field method, 3. improved rrt* algorithm, 3.1. introducing the concept of artificial potential fields, 3.1.1. introducing the gravitational component, 3.1.2. introduction of repulsive force component, 3.2. probabilistic sampling optimization, 3.3. adaptive step size strategy, 3.4. global path post-processing, 3.4.1. node pruning strategy, 3.4.2. node optimization, 4. improved artificial potential field method, 4.1. obstacle avoidance constraint, 4.2. road repulsion potential field, 4.3. increase distance factor, 4.4. virtual target point, 4.5. elliptical groove treatment, 5. fusion algorithm, 5.1. analysis of the limitation of the algorithm, 5.2. algorithm fusion strategy, 5.3. path smoothing strategy, 6. simulation analysis, 6.1. simulation analysis of improved rrt* algorithm, 6.1.1. experiment 1, 6.1.2. experiment 2, 6.2. simulation analysis of fusion algorithm, 7. conclusions, author contributions, institutional review board statement, informed consent statement, data availability statement, conflicts of interest.
- Achiri, A.T.; Mbue, I.N.; Merlin, A.; Gerard, A. Automobile Crash Investigation Based on Vehicle System Related Causes: Systematic Literature Review. World J. Eng. Technol. 2022 , 10 , 139–157. [ Google Scholar ] [ CrossRef ]
- Gaba, S.; Pawar, S.; Chinta, P.P.; Sweta, S. A computational model of deep learning in self-driving car. AIP Conf. Proc. 2023 , 2755 , 020019. [ Google Scholar ] [ CrossRef ]
- Lin, S.-L.; Li, X.-Q.; Wu, J.-Y.; Lin, B.-C. Research on overtaking path planning of autonomous vehicles. In Proceedings of the 2021 IEEE International Future Energy Electronics Conference (IFEEC), Taipei, Taiwan, 16–19 November 2021; pp. 1–4. [ Google Scholar ] [ CrossRef ]
- Reda, M.; Onsy, A.; Haikal, A.Y.; Ghanbari, A. Path planning algorithms in the autonomous driving system: A comprehensive review. Robot. Auton. Syst. 2024 , 174 , 104630. [ Google Scholar ] [ CrossRef ]
- Li, X. Path planning of intelligent mobile robot based on Dijkstra algorithm. J. Phys. Conf. Ser. 2021 , 2083 , 042034. [ Google Scholar ] [ CrossRef ]
- He, Z.; Liu, C.; Chu, X.; Negenborn, R.R.; Wu, Q. Dynamic anti-collision A-star algorithm for multi-ship encounter situations. Appl. Ocean. Res. 2022 , 118 , 102995. [ Google Scholar ] [ CrossRef ]
- Li, Q.; Xu, Y.; Bu, S.; Yang, J. Smart Vehicle Path Planning Based on Modified PRM Algorithm. Sensors 2022 , 22 , 6581. [ Google Scholar ] [ CrossRef ] [ PubMed ]
- Huang, G.; Ma, Q. Research on Path Planning Algorithm of Autonomous Vehicles Based on Improved RRT Algorithm. Int. J. Intell. Transp. Syst. Res. 2021 , 20 , 170–180. [ Google Scholar ] [ CrossRef ]
- Karaman, S.; Frazzoli, E. Sampling-based algorithms for optimal motion planning. Int. J. Robot. Res. 2011 , 30 , 846–894. [ Google Scholar ] [ CrossRef ]
- Cong, J.; Hu, J.; Wang, Y.; He, Z.; Han, L.; Su, M. FF-RRT*: A sampling-improved path planning algorithm for mobile robots against concave cavity obstacle. Complex Intell. Syst. 2023 , 9 , 7249–7267. [ Google Scholar ] [ CrossRef ]
- Lin, Y.; Zhang, L. An improved Quick Informed-RRT* algorithm based on hybrid bidirectional search and adaptive adjustment strategies. Intell. Serv. Robot. 2024 . [ Google Scholar ] [ CrossRef ]
- Gu, X.; Han, M.; Zhang, W.; Xue, G.; Zhang, G.; Han, Y. Intelligent vehicle path planning based on improved artificial potential field algorithm. In Proceedings of the 2019 International Conference on High Performance Big Data and Intelligent Systems (HPBD&IS), Shenzhen, China, 9–11 May 2019; pp. 104–109. [ Google Scholar ] [ CrossRef ]
- Li, Y.; Yang, W.; Zhang, X.; Kang, X.; Li, M. Research on Automatic Driving Trajectory Planning and Tracking Control Based on Improvement of the Artificial Potential Field Method. Sustainability 2022 , 14 , 12131. [ Google Scholar ] [ CrossRef ]
- Xu, X.; Hu, Y.; Zhai, J.M.; Li, L.Z.; Guo, P.S. A novel non-collision trajectory planning algorithm based on velocity potential field for robotic manipulator. Int. J. Adv. Robot. Syst. 2018 , 15 , 1729881418787075. [ Google Scholar ] [ CrossRef ]
- Zhai, S.; Pei, Y. The Dynamic Path Planning of Autonomous Vehicles on Icy and Snowy Roads Based on an Improved Artificial Potential Field. Sustainability 2023 , 15 , 15377. [ Google Scholar ] [ CrossRef ]
- Huang, S. Path planning based on mixed algorithm of RRT and artificial potential field method. In Proceedings of the 2021 4th International Conference on Intelligent Robotics and Control Engineering (IRCE), Lanzhou, China, 18–20 September 2021; pp. 149–155. [ Google Scholar ] [ CrossRef ]
- Zhang, W.; Wang, N.; Wu, W. A hybrid path planning algorithm considering AUV dynamic constraints based on improved A* algorithm and APF algorithm. Ocean. Eng. 2023 , 285 , 115333. [ Google Scholar ] [ CrossRef ]
- Wu, H.; Zhang, Y.; Huang, L.; Zhang, J.; Luan, Z.; Zhao, W.; Chen, F. Research on vehicle obstacle avoidance path planning based on APF-PSO. Proc. Inst. Mech. Eng. Part D J. Automob. Eng. 2022 , 237 , 1391–1405. [ Google Scholar ] [ CrossRef ]
- Dasiah, N.B.J.a.p.a. Modified RRT* for Path Planning in Autonomous Driving. arXiv 2024 , arXiv:arXiv:2402.12129. [ Google Scholar ] [ CrossRef ]
- Nguyen, K.; Dang, V.T.; Pham, D.D.; Dao, P.N. Formation control scheme with reinforcement learning strategy for a group of multiple surface vehicles. Int. J. Robust Nonlinear Control. 2023 , 34 , 2252–2279. [ Google Scholar ] [ CrossRef ]
- Shi, Y.; Dong, X.; Hua, Y.; Yu, J.; Ren, Z. Distributed output formation tracking control of heterogeneous multi-agent systems using reinforcement learning. ISA Trans. 2023 , 138 , 318–328. [ Google Scholar ] [ CrossRef ] [ PubMed ]
- Wei, K.; Ren, B. A Method on Dynamic Path Planning for Robotic Manipulator Autonomous Obstacle Avoidance Based on an Improved RRT Algorithm. Sensors 2018 , 18 , 571. [ Google Scholar ] [ CrossRef ]
- Xin, P.; Wang, X.; Liu, X.; Wang, Y.; Zhai, Z.; Ma, X. Improved Bidirectional RRT* Algorithm for Robot Path Planning. Sensors 2023 , 23 , 1041. [ Google Scholar ] [ CrossRef ]
- Dong, M.; Chen, T.; Yang, J. Simulation Research on path Planning of Unmanned vehicle based on improved RRT algorithm. Comput. Simul. 2019 , 36 , 96–100. [ Google Scholar ]
- Zhuge, C.; Wang, Q.; Liu, J.; Yao, L. An Improved Q-RRT* Algorithm Based on Virtual Light. Comput. Syst. Sci. Eng. 2021 , 39 , 107–119. [ Google Scholar ] [ CrossRef ]
- Li, W.; Jin, J. Optimal path Convergence method based on artificial potential Field method and heuristic sampling. Comput. Appl. 2021 , 41 , 2912. [ Google Scholar ] [ CrossRef ]
- Liu, X.; Cao, L. Research on path Planning of manipulator based on improved RRT* algorithm. J. Sichuan Univ. Sci. Eng. (Nat. Sci. Ed.) 2024 , 37 , 61–70. [ Google Scholar ] [ CrossRef ]
- Zhang, Y.; Gao, F.; Zhao, F. Research on Path Planning and Tracking Control of Autonomous Vehicles Based on Improved RRT* and PSO-LQR. Processes 2023 , 11 , 1841. [ Google Scholar ] [ CrossRef ]
- Wang, J.; Li, B.; Meng, M.Q.H. Kinematic Constrained Bi-directional RRT with Efficient Branch Pruning for robot path planning. Expert Syst. Appl. 2021 , 170 , 114541. [ Google Scholar ] [ CrossRef ]
- Guo, Y.; Liu, H.; Fan, X.; Lyu, W.; Chen, H. Research Progress of Path Planning Methods for Autonomous Underwater Vehicle. Math. Probl. Eng. 2021 , 2021 , 8847863. [ Google Scholar ] [ CrossRef ]
- Liu, H. Research on Path Planning of RRT Algorithm Based on APF. Master’s Thesis, Jiangsu University, Zhenjiang, China, 2022. [ Google Scholar ] [ CrossRef ]
- Wang, W.; Li, G.; Liu, S.; Yang, Q. Trajectory Planning of a Semi-Trailer Train Based on Constrained Iterative LQR. Appl. Sci. 2023 , 13 , 10614. [ Google Scholar ] [ CrossRef ]
- Hongyu, H.; Chi, Z.; Yuhuan, S.; Bin, Z.; Fei, G.J.I.-P. An improved artificial potential field model considering vehicle velocity for autonomous driving. IFAC-PapersOnLine 2018 , 51 , 863–867. [ Google Scholar ] [ CrossRef ]
- Ruan, S. Research on Path Planning of Mobile Robot Based on Improved RRT* and Artificial Potential Field Method ; Hangzhou University of Electronic Science and Technology: Hangzhou, China, 2023. [ Google Scholar ] [ CrossRef ]
- Xie, C.; Tao, T.; Li, J. Research on path Planning based on improved artificial potential Field method. J. Jilin Univ. (Inf. Sci. Ed.) 2023 , 41 , 998–1006. [ Google Scholar ] [ CrossRef ]
- Li, Y.; Jin, R.; Xu, X.; Qian, Y.; Wang, H.; Xu, S.; Wang, Z. A Mobile Robot Path Planning Algorithm Based on Improved A* Algorithm and Dynamic Window Approach. IEEE Access 2022 , 10 , 57736–57747. [ Google Scholar ] [ CrossRef ]
- Lai, R.; Wu, Z.; Liu, X.; Zeng, N. Fusion Algorithm of the Improved A* Algorithm and Segmented Bézier Curves for the Path Planning of Mobile Robots. Sustainability 2023 , 15 , 2483. [ Google Scholar ] [ CrossRef ]
- Li, X.; Zheng, H.; Wang, J.; Xia, Y.; Song, H. A Global Path Planning Method for Unmanned Ground Vehicles in Off-Road Scenarios Based on Terrain Data. Soc. Sci. Res. Netw. 2024 . [ Google Scholar ] [ CrossRef ]
Click here to enlarge figure
Candidate Point | Trails | Path Distance |
---|---|---|
5 | 0-5-9 | 0-5-9 = 8 |
6 | 0-4-6-9 | 0-4-6-9 = 16 |
8 | 0-5-8-9 | 0-5-8-9 = 9 |
Neighboring Node | Original Consideration | New Pathway | New Price |
---|---|---|---|
4 | 10 | 0-5-9-4 | 0-5-9-4 = 12 |
6 | 10 + 5 = 15 | 0-5-9-6 | 0-5-9-6 = 9 |
8 | 3 + 5 + 1 = 6 | 0-5-9-8 | 0-5-9-8 = 11 |
Algorithm | Average Path Length | Average Number of Nodes | Average Simulation Time (s) | Average Iterations |
---|---|---|---|---|
RRT algorithm | 37.92 | 81 | 29.61 | 6150 |
RRT* algorithm | 34.05 | 42 | 20.09 | 5590 |
Traditional improved RRT algorithm | 33.89 | 47 | 2.24 | 575 |
This paper improves the algorithm. | 32.47 | 12 | 1.21 | 279 |
Algorithm | Average Path Length | Average Number of Nodes | Average Simulation Time (s) | Average Iterations |
---|---|---|---|---|
Dijkstra algorithm | 31.49 | 7 | 1.42 | 313 |
A* algorithm | 31.65 | 6 | 1.37 | 175 |
Ant colony algorithm | 32.93 | 16 | 3.47 | - |
Improved A* algorithm | 31.78 | 4 | 1.15 | 116 |
This paper improves the algorithm. | 32.47 | 12 | 1.21 | 279 |
Algorithm | Average Path Length | Average Number of Nodes |
---|---|---|
This paper improves the algorithm. | 32.47 | 12 |
An improved algorithm after node optimization | 26.52 | 9 |
The statements, opinions and data contained in all publications are solely those of the individual author(s) and contributor(s) and not of MDPI and/or the editor(s). MDPI and/or the editor(s) disclaim responsibility for any injury to people or property resulting from any ideas, methods, instructions or products referred to in the content. |
Share and Cite
Li, X.; Li, G.; Bian, Z. Research on Autonomous Vehicle Path Planning Algorithm Based on Improved RRT* Algorithm and Artificial Potential Field Method. Sensors 2024 , 24 , 3899. https://doi.org/10.3390/s24123899
Li X, Li G, Bian Z. Research on Autonomous Vehicle Path Planning Algorithm Based on Improved RRT* Algorithm and Artificial Potential Field Method. Sensors . 2024; 24(12):3899. https://doi.org/10.3390/s24123899
Li, Xiang, Gang Li, and Zijian Bian. 2024. "Research on Autonomous Vehicle Path Planning Algorithm Based on Improved RRT* Algorithm and Artificial Potential Field Method" Sensors 24, no. 12: 3899. https://doi.org/10.3390/s24123899
Article Metrics
Article access statistics, further information, mdpi initiatives, follow mdpi.
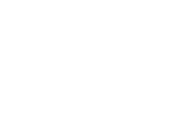
Subscribe to receive issue release notifications and newsletters from MDPI journals
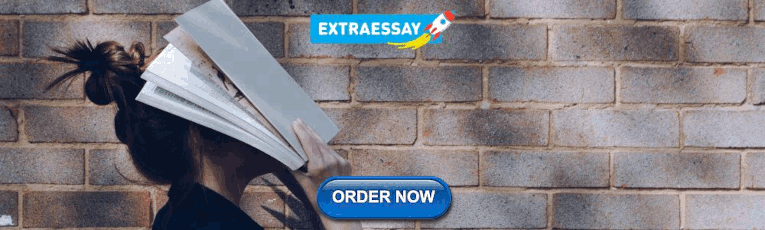
COMMENTS
Sampling methods are crucial for conducting reliable research. In this article, you will learn about the types, techniques and examples of sampling methods, and how to choose the best one for your study. Scribbr also offers free tools and guides for other aspects of academic writing, such as citation, bibliography, and fallacy.
We could choose a sampling method based on whether we want to account for sampling bias; a random sampling method is often preferred over a non-random method for this reason. Random sampling examples include: simple, systematic, stratified, and cluster sampling. Non-random sampling methods are liable to bias, and common examples include ...
A sampling plan is a formal plan specifying a sampling method, a sample size, and procedure for recruiting participants (Box 1) . A qualitative sampling plan describes how many observations, interviews, focus-group discussions or cases are needed to ensure that the findings will contribute rich data.
Sampling methods refer to the techniques used to select a subset of individuals or units from a larger population for the purpose of conducting statistical analysis or research. Sampling is an essential part of the Research because it allows researchers to draw conclusions about a population without having to collect data from every member of ...
Understand sampling methods in research, from simple random sampling to stratified, systematic, and cluster sampling. Learn how these sampling techniques boost data accuracy and representation, ensuring robust, reliable results. Check this article to learn about the different sampling method techniques, types and examples.
Simple random sampling. Simple random sampling involves selecting participants in a completely random fashion, where each participant has an equal chance of being selected.Basically, this sampling method is the equivalent of pulling names out of a hat, except that you can do it digitally.For example, if you had a list of 500 people, you could use a random number generator to draw a list of 50 ...
1. Simple random sampling. In a simple random sample, every member of the population has an equal chance of being selected. Your sampling frame should include the whole population. To conduct this type of sampling, you can use tools like random number generators or other techniques that are based entirely on chance.
Evaluate your goals against time and budget. List the two or three most obvious sampling methods that will work for you. Confirm the availability of your resources (researchers, computer time, etc.) Compare each of the possible methods with your goals, accuracy, precision, resource, time, and cost constraints.
Abstract. Knowledge of sampling methods is essential to design quality research. Critical questions are provided to help researchers choose a sampling method. This article reviews probability and non-probability sampling methods, lists and defines specific sampling techniques, and provides pros and cons for consideration.
The sampling method will depend on the research question. For instance, the researcher may want to understand an issue in greater detail for one particular population rather than worry about the ' generalizability' of these results. ... We plan to conduct the study in the outpatient department of our hospital. This is a common scenario for ...
A sampling plan is a detailed outline of which measurements will be taken at what times, on which material, in what manner, and by whom. A statistical sampling plan follows the laws of probability, allowing you to make valid infer ences about a population from the statistics of the samples taken from it.
Systematic sampling is a probability sampling method in which researchers select members of the population at a regular interval (or k) determined in advance. If the population order is random or random-like (e.g., alphabetical), then this method will give you a representative sample that can be used to draw conclusions about your population of ...
Purposive sampling was the approach utilized to gather the sample data on this research. To be more precise, purposive sampling was used to choose the firms that are pertinent to the research [32 ...
Sampling is a critical element of research design. Different methods can be used for sample selection to ensure that members of the study population reflect both the source and target populations, including probability and non-probability sampling. Power and sample size are used to determine the number of subjects needed to answer the research ...
The main purpose of sampling in research is to make the research process doable. The research sample helps to reduce bias, accurately present the population and is cost-effective.
Sampling types. There are two major categories of sampling methods ( figure 1 ): 1; probability sampling methods where all subjects in the target population have equal chances to be selected in the sample [ 1, 2] and 2; non-probability sampling methods where the sample population is selected in a non-systematic process that does not guarantee ...
Sampling is a process used in statistical analysis in which a predetermined number of observations are taken from a larger population. The methodology used to sample from a larger population ...
A sampling plan outlines the individuals chosen to represent the target population under consideration for research purposes. During a sampling plan in research, the sampling unit, the sampling size, and the sampling procedure are determined. The sample size will specify how many people from the sampling unit will be surveyed or studied.
Sampling in market action research is of two types - probability sampling and non-probability sampling. Let's take a closer look at these two methods of sampling. Probability sampling:Probability sampling is a sampling technique where a researcher selects a few criteria and chooses members of a population randomly.
What is sampling? In survey research, sampling is the process of using a subset of a population to represent the whole population. To help illustrate this further, let's look at data sampling methods with examples below. Let's say you wanted to do some research on everyone in North America. To ask every person would be almost impossible.
Sampling can be defined as the process through which individuals or sampling units are selected from the sample frame. The sampling strategy needs to be specified in advance, given that the sampling method may affect the sample size estimation. 1,5 Without a rigorous sampling plan the estimates derived from the study may be biased (selection ...
A research design is a strategy for answering your research question using empirical data. Creating a research design means making decisions about: Your overall research objectives and approach. Whether you'll rely on primary research or secondary research. Your sampling methods or criteria for selecting subjects. Your data collection methods.
A sampling plan basically comprises of different sample units or sample population whom you are going to contact to collect market research data. This sampling unit is a representative of the total population, though it might be a fraction of the total population. In simple language, if you have 1 lakh customers, you cannot conduct an interview ...
Sampling design and plan. The study relies on a sample of Class A clients from Egypt's luxury sector. Given the population's size and diversity, a careful selection guide is employed to identify and authenticate these clients. ... Research methodology methods and techniques. New Age International (P) Ltd., Publishers, p. 91. Google Scholar ...
A sampling plan is a formal plan specifying a sampling method, a sample size, and procedure for recruiting participants (Box 1) [Citation 3]. A qualitative sampling plan describes how many observations, interviews, focus-group discussions or cases are needed to ensure that the findings will contribute rich data.
CHIPS for America encompasses two offices responsible for implementing the law: The CHIPS Research and Development Office is investing $11 billion into developing a robust domestic R&D ecosystem, while the CHIPS Program Office is dedicating $39 billion to provide incentives for investment in facilities and equipment in the United States.
For the RRT* algorithm, there are problems such as greater randomness, longer time consumption, more redundant nodes, and inability to perform local obstacle avoidance when encountering unknown obstacles in the path planning process of autonomous vehicles. And the artificial potential field method (APF) applied to autonomous vehicles is prone to problems such as local optimality, unreachable ...