A composite variable is a combination of multiple variables. It is used to measure multidimensional aspects that are difficult to observe. | - Entertainment
- Online education
- Database management, storage, and retrieval
Frequently Asked QuestionsWhat are the 10 types of variables in research. The 10 types of variables in research are: - Independent
- Confounding
- Categorical
- Extraneous.
What is an independent variable?An independent variable, often termed the predictor or explanatory variable, is the variable manipulated or categorized in an experiment to observe its effect on another variable, called the dependent variable. It’s the presumed cause in a cause-and-effect relationship, determining if changes in it produce changes in the observed outcome. What is a variable?In research, a variable is any attribute, quantity, or characteristic that can be measured or counted. It can take on various values, making it “variable.” Variables can be classified as independent (manipulated), dependent (observed outcome), or control (kept constant). They form the foundation for hypotheses, observations, and data analysis in studies. What is a dependent variable?A dependent variable is the outcome or response being studied in an experiment or investigation. It’s what researchers measure to determine the effect of changes in the independent variable. In a cause-and-effect relationship, the dependent variable is presumed to be influenced or caused by the independent variable. What is a variable in programming?In programming, a variable is a symbolic name for a storage location that holds data or values. It allows data storage and retrieval for computational operations. Variables have types, like integer or string, determining the nature of data they can hold. They’re fundamental in manipulating and processing information in software. What is a control variable?A control variable in research is a factor that’s kept constant to ensure that it doesn’t influence the outcome. By controlling these variables, researchers can isolate the effects of the independent variable on the dependent variable, ensuring that other factors don’t skew the results or introduce bias into the experiment. What is a controlled variable in science?In science, a controlled variable is a factor that remains constant throughout an experiment. It ensures that any observed changes in the dependent variable are solely due to the independent variable, not other factors. By keeping controlled variables consistent, researchers can maintain experiment validity and accurately assess cause-and-effect relationships. How many independent variables should an investigation have?Ideally, an investigation should have one independent variable to clearly establish cause-and-effect relationships. Manipulating multiple independent variables simultaneously can complicate data interpretation. However, in advanced research, experiments with multiple independent variables (factorial designs) are used, but they require careful planning to understand interactions between variables. You May Also LikeAction research for my dissertation?, A brief overview of action research as a responsive, action-oriented, participative and reflective research technique. Thematic analysis is commonly used for qualitative data. Researchers give preference to thematic analysis when analysing audio or video transcripts. A survey includes questions relevant to the research topic. The participants are selected, and the questionnaire is distributed to collect the data. USEFUL LINKS LEARNING RESOURCES 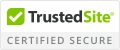 COMPANY DETAILS 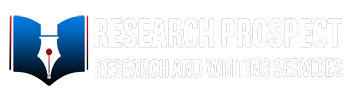  An official website of the United States government The .gov means itâs official. Federal government websites often end in .gov or .mil. Before sharing sensitive information, make sure youâre on a federal government site. The site is secure. The https:// ensures that you are connecting to the official website and that any information you provide is encrypted and transmitted securely. - Publications
- Account settings
Preview improvements coming to the PMC website in October 2024. Learn More or Try it out now . - Advanced Search
- Journal List
- Indian Dermatol Online J
- v.10(1); Jan-Feb 2019
Types of Variables, Descriptive Statistics, and Sample SizeFeroze kaliyadan. Department of Dermatology, King Faisal University, Al Hofuf, Saudi Arabia Vinay Kulkarni1 Department of Dermatology, Prayas Amrita Clinic, Pune, Maharashtra, India This short “snippet” covers three important aspects related to statistics – the concept of variables , the importance, and practical aspects related to descriptive statistics and issues related to sampling – types of sampling and sample size estimation. What is a variable?[ 1 , 2 ] To put it in very simple terms, a variable is an entity whose value varies. A variable is an essential component of any statistical data. It is a feature of a member of a given sample or population, which is unique, and can differ in quantity or quantity from another member of the same sample or population. Variables either are the primary quantities of interest or act as practical substitutes for the same. The importance of variables is that they help in operationalization of concepts for data collection. For example, if you want to do an experiment based on the severity of urticaria, one option would be to measure the severity using a scale to grade severity of itching. This becomes an operational variable. For a variable to be “good,” it needs to have some properties such as good reliability and validity, low bias, feasibility/practicality, low cost, objectivity, clarity, and acceptance. Variables can be classified into various ways as discussed below. Quantitative vs qualitativeA variable can collect either qualitative or quantitative data. A variable differing in quantity is called a quantitative variable (e.g., weight of a group of patients), whereas a variable differing in quality is called a qualitative variable (e.g., the Fitzpatrick skin type) A simple test which can be used to differentiate between qualitative and quantitative variables is the subtraction test. If you can subtract the value of one variable from the other to get a meaningful result, then you are dealing with a quantitative variable (this of course will not apply to rating scales/ranks). Quantitative variables can be either discrete or continuousDiscrete variables are variables in which no values may be assumed between the two given values (e.g., number of lesions in each patient in a sample of patients with urticaria). Continuous variables, on the other hand, can take any value in between the two given values (e.g., duration for which the weals last in the same sample of patients with urticaria). One way of differentiating between continuous and discrete variables is to use the “mid-way” test. If, for every pair of values of a variable, a value exactly mid-way between them is meaningful, the variable is continuous. For example, two values for the time taken for a weal to subside can be 10 and 13 min. The mid-way value would be 11.5 min which makes sense. However, for a number of weals, suppose you have a pair of values – 5 and 8 – the midway value would be 6.5 weals, which does not make sense. Under the umbrella of qualitative variables, you can have nominal/categorical variables and ordinal variablesNominal/categorical variables are, as the name suggests, variables which can be slotted into different categories (e.g., gender or type of psoriasis). Ordinal variables or ranked variables are similar to categorical, but can be put into an order (e.g., a scale for severity of itching). Dependent and independent variablesIn the context of an experimental study, the dependent variable (also called outcome variable) is directly linked to the primary outcome of the study. For example, in a clinical trial on psoriasis, the PASI (psoriasis area severity index) would possibly be one dependent variable. The independent variable (sometime also called explanatory variable) is something which is not affected by the experiment itself but which can be manipulated to affect the dependent variable. Other terms sometimes used synonymously include blocking variable, covariate, or predictor variable. Confounding variables are extra variables, which can have an effect on the experiment. They are linked with dependent and independent variables and can cause spurious association. For example, in a clinical trial for a topical treatment in psoriasis, the concomitant use of moisturizers might be a confounding variable. A control variable is a variable that must be kept constant during the course of an experiment. Descriptive StatisticsStatistics can be broadly divided into descriptive statistics and inferential statistics.[ 3 , 4 ] Descriptive statistics give a summary about the sample being studied without drawing any inferences based on probability theory. Even if the primary aim of a study involves inferential statistics, descriptive statistics are still used to give a general summary. When we describe the population using tools such as frequency distribution tables, percentages, and other measures of central tendency like the mean, for example, we are talking about descriptive statistics. When we use a specific statistical test (e.g., Mann–Whitney U-test) to compare the mean scores and express it in terms of statistical significance, we are talking about inferential statistics. Descriptive statistics can help in summarizing data in the form of simple quantitative measures such as percentages or means or in the form of visual summaries such as histograms and box plots. Descriptive statistics can be used to describe a single variable (univariate analysis) or more than one variable (bivariate/multivariate analysis). In the case of more than one variable, descriptive statistics can help summarize relationships between variables using tools such as scatter plots. Descriptive statistics can be broadly put under two categories: - Sorting/grouping and illustration/visual displays
- Summary statistics.
Sorting and groupingSorting and grouping is most commonly done using frequency distribution tables. For continuous variables, it is generally better to use groups in the frequency table. Ideally, group sizes should be equal (except in extreme ends where open groups are used; e.g., age “greater than” or “less than”). Another form of presenting frequency distributions is the “stem and leaf” diagram, which is considered to be a more accurate form of description. Suppose the weight in kilograms of a group of 10 patients is as follows: 56, 34, 48, 43, 87, 78, 54, 62, 61, 59 The “stem” records the value of the “ten's” place (or higher) and the “leaf” records the value in the “one's” place [ Table 1 ]. Stem and leaf plot | |
---|
0 | - | 1 | - | 2 | - | 3 | 4 | 4 | 3 8 | 5 | 4 6 9 | 6 | 1 2 | 7 | 8 | 8 | 7 | 9 | - |
Illustration/visual display of dataThe most common tools used for visual display include frequency diagrams, bar charts (for noncontinuous variables) and histograms (for continuous variables). Composite bar charts can be used to compare variables. For example, the frequency distribution in a sample population of males and females can be illustrated as given in Figure 1 .  Composite bar chart A pie chart helps show how a total quantity is divided among its constituent variables. Scatter diagrams can be used to illustrate the relationship between two variables. For example, global scores given for improvement in a condition like acne by the patient and the doctor [ Figure 2 ].  Scatter diagram Summary statisticsThe main tools used for summary statistics are broadly grouped into measures of central tendency (such as mean, median, and mode) and measures of dispersion or variation (such as range, standard deviation, and variance). Imagine that the data below represent the weights of a sample of 15 pediatric patients arranged in ascending order: 30, 35, 37, 38, 38, 38, 42, 42, 44, 46, 47, 48, 51, 53, 86 Just having the raw data does not mean much to us, so we try to express it in terms of some values, which give a summary of the data. The mean is basically the sum of all the values divided by the total number. In this case, we get a value of 45. The problem is that some extreme values (outliers), like “'86,” in this case can skew the value of the mean. In this case, we consider other values like the median, which is the point that divides the distribution into two equal halves. It is also referred to as the 50 th percentile (50% of the values are above it and 50% are below it). In our previous example, since we have already arranged the values in ascending order we find that the point which divides it into two equal halves is the 8 th value – 42. In case of a total number of values being even, we choose the two middle points and take an average to reach the median. The mode is the most common data point. In our example, this would be 38. The mode as in our case may not necessarily be in the center of the distribution. The median is the best measure of central tendency from among the mean, median, and mode. In a “symmetric” distribution, all three are the same, whereas in skewed data the median and mean are not the same; lie more toward the skew, with the mean lying further to the skew compared with the median. For example, in Figure 3 , a right skewed distribution is seen (direction of skew is based on the tail); data values' distribution is longer on the right-hand (positive) side than on the left-hand side. The mean is typically greater than the median in such cases.  Location of mode, median, and mean Measures of dispersionThe range gives the spread between the lowest and highest values. In our previous example, this will be 86-30 = 56. A more valuable measure is the interquartile range. A quartile is one of the values which break the distribution into four equal parts. The 25 th percentile is the data point which divides the group between the first one-fourth and the last three-fourth of the data. The first one-fourth will form the first quartile. The 75 th percentile is the data point which divides the distribution into a first three-fourth and last one-fourth (the last one-fourth being the fourth quartile). The range between the 25 th percentile and 75 th percentile is called the interquartile range. Variance is also a measure of dispersion. The larger the variance, the further the individual units are from the mean. Let us consider the same example we used for calculating the mean. The mean was 45. For the first value (30), the deviation from the mean will be 15; for the last value (86), the deviation will be 41. Similarly we can calculate the deviations for all values in a sample. Adding these deviations and averaging will give a clue to the total dispersion, but the problem is that since the deviations are a mix of negative and positive values, the final total becomes zero. To calculate the variance, this problem is overcome by adding squares of the deviations. So variance would be the sum of squares of the variation divided by the total number in the population (for a sample we use “n − 1”). To get a more realistic value of the average dispersion, we take the square root of the variance, which is called the “standard deviation.” The box plotThe box plot is a composite representation that portrays the mean, median, range, and the outliers [ Figure 4 ].  The concept of skewness and kurtosisSkewness is a measure of the symmetry of distribution. Basically if the distribution curve is symmetric, it looks the same on either side of the central point. When this is not the case, it is said to be skewed. Kurtosis is a representation of outliers. Distributions with high kurtosis tend to have “heavy tails” indicating a larger number of outliers, whereas distributions with low kurtosis have light tails, indicating lesser outliers. There are formulas to calculate both skewness and kurtosis [Figures [Figures5 5 – 8 ].  Positive skew  High kurtosis (positive kurtosis – also called leptokurtic)  Negative skew  Low kurtosis (negative kurtosis – also called “Platykurtic”) Sample SizeIn an ideal study, we should be able to include all units of a particular population under study, something that is referred to as a census.[ 5 , 6 ] This would remove the chances of sampling error (difference between the outcome characteristics in a random sample when compared with the true population values – something that is virtually unavoidable when you take a random sample). However, it is obvious that this would not be feasible in most situations. Hence, we have to study a subset of the population to reach to our conclusions. This representative subset is a sample and we need to have sufficient numbers in this sample to make meaningful and accurate conclusions and reduce the effect of sampling error. We also need to know that broadly sampling can be divided into two types – probability sampling and nonprobability sampling. Examples of probability sampling include methods such as simple random sampling (each member in a population has an equal chance of being selected), stratified random sampling (in nonhomogeneous populations, the population is divided into subgroups – followed be random sampling in each subgroup), systematic (sampling is based on a systematic technique – e.g., every third person is selected for a survey), and cluster sampling (similar to stratified sampling except that the clusters here are preexisting clusters unlike stratified sampling where the researcher decides on the stratification criteria), whereas nonprobability sampling, where every unit in the population does not have an equal chance of inclusion into the sample, includes methods such as convenience sampling (e.g., sample selected based on ease of access) and purposive sampling (where only people who meet specific criteria are included in the sample). An accurate calculation of sample size is an essential aspect of good study design. It is important to calculate the sample size much in advance, rather than have to go for post hoc analysis. A sample size that is too less may make the study underpowered, whereas a sample size which is more than necessary might lead to a wastage of resources. We will first go through the sample size calculation for a hypothesis-based design (like a randomized control trial). The important factors to consider for sample size calculation include study design, type of statistical test, level of significance, power and effect size, variance (standard deviation for quantitative data), and expected proportions in the case of qualitative data. This is based on previous data, either based on previous studies or based on the clinicians' experience. In case the study is something being conducted for the first time, a pilot study might be conducted which helps generate these data for further studies based on a larger sample size). It is also important to know whether the data follow a normal distribution or not. Two essential aspects we must understand are the concept of Type I and Type II errors. In a study that compares two groups, a null hypothesis assumes that there is no significant difference between the two groups, and any observed difference being due to sampling or experimental error. When we reject a null hypothesis, when it is true, we label it as a Type I error (also denoted as “alpha,” correlating with significance levels). In a Type II error (also denoted as “beta”), we fail to reject a null hypothesis, when the alternate hypothesis is actually true. Type II errors are usually expressed as “1- β,” correlating with the power of the test. While there are no absolute rules, the minimal levels accepted are 0.05 for α (corresponding to a significance level of 5%) and 0.20 for β (corresponding to a minimum recommended power of “1 − 0.20,” or 80%). Effect size and minimal clinically relevant differenceFor a clinical trial, the investigator will have to decide in advance what clinically detectable change is significant (for numerical data, this is could be the anticipated outcome means in the two groups, whereas for categorical data, it could correlate with the proportions of successful outcomes in two groups.). While we will not go into details of the formula for sample size calculation, some important points are as follows: In the context where effect size is involved, the sample size is inversely proportional to the square of the effect size. What this means in effect is that reducing the effect size will lead to an increase in the required sample size. Reducing the level of significance (alpha) or increasing power (1-β) will lead to an increase in the calculated sample size. An increase in variance of the outcome leads to an increase in the calculated sample size. A note is that for estimation type of studies/surveys, sample size calculation needs to consider some other factors too. This includes an idea about total population size (this generally does not make a major difference when population size is above 20,000, so in situations where population size is not known we can assume a population of 20,000 or more). The other factor is the “margin of error” – the amount of deviation which the investigators find acceptable in terms of percentages. Regarding confidence levels, ideally, a 95% confidence level is the minimum recommended for surveys too. Finally, we need an idea of the expected/crude prevalence – either based on previous studies or based on estimates. Sample size calculation also needs to add corrections for patient drop-outs/lost-to-follow-up patients and missing records. An important point is that in some studies dealing with rare diseases, it may be difficult to achieve desired sample size. In these cases, the investigators might have to rework outcomes or maybe pool data from multiple centers. Although post hoc power can be analyzed, a better approach suggested is to calculate 95% confidence intervals for the outcome and interpret the study results based on this. Financial support and sponsorshipConflicts of interest. There are no conflicts of interest. - Translators
- Graphic Designers
 Please enter the email address you used for your account. Your sign in information will be sent to your email address after it has been verified. Understanding Independent vs. Dependent Variables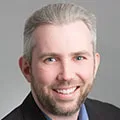 In the realm of scientific research, variables serve as the backbone to any form of inquiry. A variable, by definition, refers to any characteristic, number, or quantity that can be measured or counted. They provide a systematic way to describe, explain, and predict how and why certain phenomena change. The entire process of scientific researchâfrom formulating a hypothesis, designing the experiment, collecting and analyzing data, to drawing conclusionsâhinges on the accurate identification and management of variables. Without them, research lacks structure and reproducibility, two fundamental elements in the scientific world. Grasping the differences between independent and dependent variables is a fundamental aspect of conducting research. The relationship between these two types of variables forms the foundation of scientific experiments, dictating the cause-and-effect paradigm that allows researchers to draw meaningful conclusions. Misunderstanding or incorrectly identifying these variables can distort research outcomes, leading to invalid results and incorrect conclusions. Thus, an accurate understanding is not just crucialâit's indispensable. At a glance, an independent variable is what the researcher manipulates or changes in a study to observe the effect on the outcome. It's the cause in a cause-and-effect relationship. Conversely, a dependent variable is what's being tested and measuredâthe effect or outcome. It's the variable that researchers anticipate will change in response to the manipulation of the independent variable. This distinction is key to the design, execution, and interpretation of any research experiment, which we'll delve into in the subsequent sections of this post. What is a variable?In research, a variable refers to a characteristic, number, or attribute that can be measured or counted. It's a crucial concept that takes on different values and helps researchers to understand patterns, correlations, and causal relationships. For instance, in a study exploring the relationship between physical activity and mental health, both physical activity (measured in hours per week) and mental health (measured via a psychological well-being scale) can be considered variables. Variables are pivotal in research methodology as they help to provide structure to experiments, surveys, observations, and other research designs . They form the basis of hypotheses and are used to operationalize concepts, allowing for the empirical and quantitative study of phenomena. Without variables, there would be no metrics to analyze or patterns to interpret, making it impossible to answer research questions or test hypotheses. Types of variables other than independent and dependentWhile independent and dependent variables hold significant roles in research, other types of variables also exist and are worth mentioning: - Control Variables: These are variables that researchers keep constant during an experiment to ensure that any changes observed in the dependent variable are solely due to the manipulation of the independent variable. For example, in a study examining the effects of sunlight on plant growth, the type of plant might be a control variable to ensure that any differences in growth are due to sunlight and not different plant species.
- Confounding Variables: These are outside influences that change the effect of a dependent and an independent variable. This change often gives a false impression of a correlation between the dependent and independent variables. For example, in a study investigating the relationship between physical activity and weight loss, diet could act as a confounding variable if not controlled.
- Moderator Variables: These influence the strength of a relationship between two other variables. For instance, in a study exploring the impact of stress on academic performance, coping skills may act as a moderator variable, as it could affect how stress influences academic performance.
- Mediator Variables: These explain the relationship between the dependent and independent variables. They're the intermediary variables that describe how or why a certain effect or relationship occurs.
Understanding these variables and their roles is crucial for robust and well-designed research. They help researchers not only in the study design but also in making valid and accurate conclusions. Understanding independent variablesIndependent variables, in the context of scientific research, are variables that researchers deliberately manipulate or change to observe the effect on the dependent variable. They are the "inputs" or causes that determine the conditions of the experiment and are chosen based on the research question or hypothesis . The role of independent variables is central to research as they facilitate the exploration of cause-and-effect relationships. By manipulating the independent variable and observing changes in the dependent variable, researchers can begin to understand how one variable affects another. This helps to establish causality, a fundamental aspect of scientific inquiry that enables researchers to predict outcomes, inform theory, and develop interventions. Examples of independent variables in different research contextsIndependent variables can span a wide range of forms, varying from research context to research context. Here are a few examples: - In a medical study investigating the effect of a drug on patients' symptoms, the independent variable could be the dosage of the drug administered.
- In a social science study examining the impact of educational attainment on income level, the independent variable would be the level of education obtained.
- In a psychological study exploring the effects of sleep deprivation on cognitive performance, the independent variable might be the amount of sleep a participant gets.
Challenges and considerations when selecting independent variablesSelecting the right independent variable requires careful consideration. Misidentifying the independent variable can lead to invalid results and inaccurate conclusions. Here are some challenges and considerations: - Ensuring Causality: One of the main challenges is ensuring that the independent variable is indeed the cause of changes observed in the dependent variable. Establishing a clear causal relationship requires careful experimental design.
- Controlling for Confounding Variables: It's important to control for other variables that might influence the dependent variable, as they could confound the relationship between the independent and dependent variables.
- Operationalizing Variables: The independent variable must be clearly defined and measurable. Researchers need to decide how to best represent or manipulate the independent variable for their specific study.
- Ethical Considerations: The selection and manipulation of independent variables must also be ethical. In some cases, manipulating a variable for the sake of an experiment may pose ethical concerns , which must be carefully managed.
Understanding independent variables and their crucial role in research is the first step in the journey of scientific discovery, driving our ability to answer complex questions about the world around us. Understanding dependent variablesDependent variables are the "outputs" or effects in a research study. They are the variables researchers are interested in observing and measuring to see how they respond to changes in the independent variable. Essentially, the dependent variable is what the researcher hopes to predict or explain through the study. The dependent variable is central to the purpose of most research. The goal of a study typically revolves around understanding how or why the dependent variable changes, providing insights into phenomena of interest. Dependent variables allow researchers to assess the effects of manipulating the independent variable, which aids in the development of theories, informs policy and practice, and contributes to scientific knowledge. Examples of dependent variables in different research contextsLike independent variables, dependent variables can be diverse and context-dependent. Here are a few examples: - In a medical study examining the effect of a drug on patients' symptoms, the dependent variable could be the severity of symptoms.
- In a social science study investigating the impact of educational attainment on income level, the dependent variable would be the income level.
- In a psychological study looking at the effects of sleep deprivation on cognitive performance, the dependent variable might be the score on a cognitive test.
Challenges and considerations when selecting dependent variablesSelection of dependent variables is a crucial step in the research process and can pose some challenges: - Measurability: A dependent variable should be quantifiable. It can be a challenge to quantify some types of outcomes, such as feelings, attitudes, or beliefs.
- Sensitivity: The dependent variable should be sensitive enough to detect changes or differences when the independent variable is manipulated.
- Relevance: The dependent variable should be relevant and meaningful for the research question or hypothesis.
- Reliability and Validity: The measures used to quantify the dependent variable should be reliable (consistent in their results over time) and valid (truly measuring what they are intended to measure) .
Understanding the dependent variable and its role in research is pivotal for elucidating the effects or impacts of various factors, thereby helping to shape our understanding of the world. 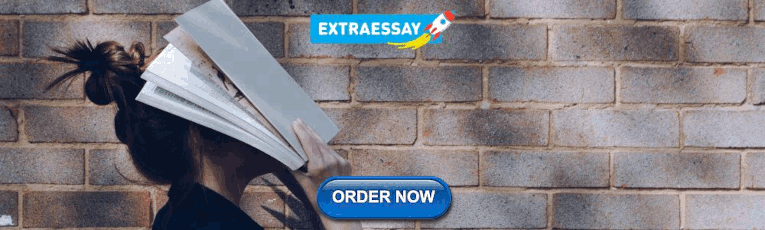 Key differences between independent and dependent variablesThe primary difference between independent and dependent variables lies in their role within a study. An independent variable is manipulated or changed by the researcher to examine its impact, while a dependent variable is what is being tested or measured - the outcome of the research. In other words, the independent variable is the cause, and the dependent variable is the effect. The independent variable precedes the dependent variable in time, while the reverse is not true. In research, understanding the relationship between independent and dependent variables is crucial as it forms the basis for the cause-and-effect reasoning. The independent variable, as the cause, directly influences the outcome, i.e., the dependent variable. If the independent variable is changed, then an effect is seen in the dependent variable. For example, in a study examining the effect of temperature on plant growth, temperature is the independent variable (cause), and plant growth is the dependent variable (effect). Mistakes to avoid in determining independent and dependent variablesIdentifying independent and dependent variables is a fundamental step in any research project, and mistakes in this stage can lead to flawed results. Here are some common mistakes to avoid: - Confusing the Independent and Dependent Variables: Ensure that the variable being manipulated (independent variable) and the variable being measured (dependent variable) are not switched.
- Ignoring Confounding Variables: Failing to control for confounding variables can lead to misleading results, as it may appear that the independent variable is causing an effect on the dependent variable when the effect is actually due to the confounding variable.
- Choosing an Irrelevant Dependent Variable: The dependent variable should directly measure the outcome that the research is interested in. Choosing a variable that does not directly measure the outcome can lead to irrelevant or meaningless results.
- Overlooking the Need for Operational Definitions: Both the independent and dependent variables need to be clearly defined so that they can be reliably measured or manipulated. Overlooking this step can lead to ambiguity and inconsistent results.
By understanding these differences and the relationship between independent and dependent variables, researchers can formulate clear and effective research designs, ensuring the validity and reliability of their findings. Practical applicationRole of independent and dependent variables in experimental design. The roles of independent and dependent variables are fundamental to experimental design. The independent variable is what the researcher manipulates or changes during the experiment. It forms the basis for experimental groups (those exposed to the manipulation) and control groups (those not exposed). The dependent variable, on the other hand, is what the researcher measures in each group to determine the effects of manipulating the independent variable. For example, in a study examining the impact of a new teaching method on students' test scores, the teaching method would be the independent variable. Students would be assigned either to an experimental group (receiving the new teaching method) or a control group (receiving the standard teaching method). The dependent variableâstudents' test scoresâwould be measured in both groups to see if the new teaching method has a significant effect. How independent and dependent variables are used in data analysisIndependent and dependent variables form the basis for data analysis in research. Statistical tests are chosen based on the type and number of independent variables and the type of dependent variable. For instance, a simple linear regression might be used to predict a dependent variable (like sales) based on one independent variable (like advertising spend). In more complex cases, multiple regression might be used to predict a dependent variable based on multiple independent variables (like advertising spend, market trends, and product price). Practical examples illustrating the correct use of independent and dependent variablesHere are a few examples illustrating the correct use of independent and dependent variables: - In a clinical trial to test a new drug, the independent variable could be the administration of the drug, with two levels: the experimental group receiving the drug and the control group receiving a placebo. The dependent variable could be the improvement in symptoms, measured on a standardized scale.
- In a study exploring the effect of temperature on ice cream sales, the independent variable is the temperature, and the dependent variable is the quantity of ice cream sold.
- In an experiment studying the effect of light exposure on sleep quality, the independent variable might be the amount of light exposure in the evening (with two levels: exposure to blue light and exposure to red light), and the dependent variable would be sleep quality, perhaps measured through a sleep quality index or actigraphy.
In each of these examples, the independent variable is manipulated to observe its impact on the dependent variable, illustrating the cause-and-effect relationship inherent in experimental research. By correctly identifying and using independent and dependent variables, researchers can create solid research designs that yield valid and reliable results. Understanding the difference between independent and dependent variables is fundamental to conducting rigorous and impactful research. These two types of variables form the bedrock of scientific inquiry, allowing us to explore cause-and-effect relationships, test hypotheses, and ultimately, expand our knowledge across a range of fields. The independent variable, which is manipulated or changed, plays a pivotal role in driving the experimental design and defining the cause. The dependent variable, on the other hand, is the effect or outcome measured in response to changes in the independent variable. Together, they offer a structured approach to investigate and understand the intricacies of various phenomena. However, the correct identification and utilization of these variables demand a clear and precise understanding to avoid common mistakes and ensure valid results. This understanding becomes even more crucial when dealing with complex studies where confounding, moderating, or mediating variables might come into play. In a practical sense, the use of independent and dependent variables permeates all facets of research, from experimental design to data analysis. Their correct application forms the core of reliable and valid scientific investigation, enhancing the credibility of the findings, and contributing to the broader knowledge landscape. Whether you're examining the effect of a new drug, exploring the relationship between education and income, or studying the impact of climate change, the key to a well-structured study lies in the thoughtful application of independent and dependent variables. Header image by Karolina Grabowska . - Academic Writing Advice
- All Blog Posts
- Writing Advice
- Admissions Writing Advice
- Book Writing Advice
- Short Story Advice
- Employment Writing Advice
- Business Writing Advice
- Web Content Advice
- Article Writing Advice
- Magazine Writing Advice
- Grammar Advice
- Dialect Advice
- Editing Advice
- Freelance Advice
- Legal Writing Advice
- Poetry Advice
- Graphic Design Advice
- Logo Design Advice
- Translation Advice
- Blog Reviews
- Short Story Award Winners
- Scholarship Winners
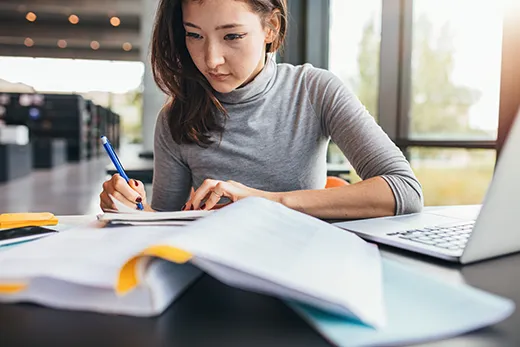 Need an academic editor before submitting your work?- Bipolar Disorder
- Therapy Center
- When To See a Therapist
- Types of Therapy
- Best Online Therapy
- Best Couples Therapy
- Best Family Therapy
- Managing Stress
- Sleep and Dreaming
- Understanding Emotions
- Self-Improvement
- Healthy Relationships
- Student Resources
- Personality Types
- Guided Meditations
- Verywell Mind Insights
- 2024 Verywell Mind 25
- Mental Health in the Classroom
- Editorial Process
- Meet Our Review Board
- Crisis Support
Types of Variables in Psychology ResearchExamples of Independent and Dependent Variables Dependent and Independent Variables- Intervening Variables
- Extraneous Variables
- Controlled Variables
- Confounding Variables
- Operationalizing Variables
Frequently Asked QuestionsVariables in psychology are things that can be changed or altered, such as a characteristic or value. Variables are generally used in psychology experiments to determine if changes to one thing result in changes to another. Variables in psychology play a critical role in the research process. By systematically changing some variables in an experiment and measuring what happens as a result, researchers are able to learn more about cause-and-effect relationships. The two main types of variables in psychology are the independent variable and the dependent variable. Both variables are important in the process of collecting data about psychological phenomena. This article discusses different types of variables that are used in psychology research. It also covers how to operationalize these variables when conducting experiments. Students often report problems with identifying the independent and dependent variables in an experiment. While this task can become more difficult as the complexity of an experiment increases, in a psychology experiment: - The independent variable is the variable that is manipulated by the experimenter. An example of an independent variable in psychology: In an experiment on the impact of sleep deprivation on test performance, sleep deprivation would be the independent variable. The experimenters would have some of the study participants be sleep-deprived while others would be fully rested.
- The dependent variable is the variable that is measured by the experimenter. In the previous example, the scores on the test performance measure would be the dependent variable.
So how do you differentiate between the independent and dependent variables? Start by asking yourself what the experimenter is manipulating. The things that change, either naturally or through direct manipulation from the experimenter, are generally the independent variables. What is being measured? The dependent variable is the one that the experimenter is measuring. Intervening Variables in PsychologyIntervening variables, also sometimes called intermediate or mediator variables, are factors that play a role in the relationship between two other variables. In the previous example, sleep problems in university students are often influenced by factors such as stress. As a result, stress might be an intervening variable that plays a role in how much sleep people get, which may then influence how well they perform on exams. Extraneous Variables in PsychologyIndependent and dependent variables are not the only variables present in many experiments. In some cases, extraneous variables may also play a role. This type of variable is one that may have an impact on the relationship between the independent and dependent variables. For example, in our previous example of an experiment on the effects of sleep deprivation on test performance, other factors such as age, gender, and academic background may have an impact on the results. In such cases, the experimenter will note the values of these extraneous variables so any impact can be controlled for. There are two basic types of extraneous variables: - Participant variables : These extraneous variables are related to the individual characteristics of each study participant that may impact how they respond. These factors can include background differences, mood, anxiety, intelligence, awareness, and other characteristics that are unique to each person.
- Situational variables : These extraneous variables are related to things in the environment that may impact how each participant responds. For example, if a participant is taking a test in a chilly room, the temperature would be considered an extraneous variable. Some participants may not be affected by the cold, but others might be distracted or annoyed by the temperature of the room.
Other extraneous variables include the following: - Demand characteristics : Clues in the environment that suggest how a participant should behave
- Experimenter effects : When a researcher unintentionally suggests clues for how a participant should behave
Controlled Variables in PsychologyIn many cases, extraneous variables are controlled for by the experimenter. A controlled variable is one that is held constant throughout an experiment. In the case of participant variables, the experiment might select participants that are the same in background and temperament to ensure that these factors don't interfere with the results. Holding these variables constant is important for an experiment because it allows researchers to be sure that all other variables remain the same across all conditions.  Using controlled variables means that when changes occur, the researchers can be sure that these changes are due to the manipulation of the independent variable and not caused by changes in other variables. It is important to also note that a controlled variable is not the same thing as a control group . The control group in a study is the group of participants who do not receive the treatment or change in the independent variable. All other variables between the control group and experimental group are held constant (i.e., they are controlled). The dependent variable being measured is then compared between the control group and experimental group to see what changes occurred because of the treatment. Confounding Variables in PsychologyIf a variable cannot be controlled for, it becomes what is known as a confounding variabl e. This type of variable can have an impact on the dependent variable, which can make it difficult to determine if the results are due to the influence of the independent variable, the confounding variable, or an interaction of the two. Operationalizing Variables in PsychologyAn operational definition describes how the variables are measured and defined in the study. Before conducting a psychology experiment , it is essential to create firm operational definitions for both the independent variable and dependent variables. For example, in our imaginary experiment on the effects of sleep deprivation on test performance, we would need to create very specific operational definitions for our two variables. If our hypothesis is "Students who are sleep deprived will score significantly lower on a test," then we would have a few different concepts to define: - Students : First, what do we mean by "students?" In our example, letâs define students as participants enrolled in an introductory university-level psychology course.
- Sleep deprivation : Next, we need to operationally define the "sleep deprivation" variable. In our example, letâs say that sleep deprivation refers to those participants who have had less than five hours of sleep the night before the test.
- Test variable : Finally, we need to create an operational definition for the test variable. For this example, the test variable will be defined as a studentâs score on a chapter exam in the introductory psychology course.
Once all the variables are operationalized, we're ready to conduct the experiment. Variables play an important part in psychology research. Manipulating an independent variable and measuring the dependent variable allows researchers to determine if there is a cause-and-effect relationship between them. A Word From VerywellUnderstanding the different types of variables used in psychology research is important if you want to conduct your own psychology experiments. It is also helpful for people who want to better understand what the results of psychology research really mean and become more informed consumers of psychology information . Independent and dependent variables are used in experimental research. Unlike some other types of research (such as correlational studies ), experiments allow researchers to evaluate cause-and-effect relationships between two variables. Researchers can use statistical analyses to determine the strength of a relationship between two variables in an experiment. Two of the most common ways to do this are to calculate a p-value or a correlation. The p-value indicates if the results are statistically significant while the correlation can indicate the strength of the relationship. In an experiment on how sugar affects short-term memory, sugar intake would be the independent variable and scores on a short-term memory task would be the independent variable. In an experiment looking at how caffeine intake affects test anxiety, the amount of caffeine consumed before a test would be the independent variable and scores on a test anxiety assessment would be the dependent variable. Just as with other types of research, the independent variable in a cognitive psychology study would be the variable that the researchers manipulate. The specific independent variable would vary depending on the specific study, but it might be focused on some aspect of thinking, memory, attention, language, or decision-making. American Psychological Association. Operational definition . APA Dictionary of Psychology. American Psychological Association. Mediator . APA Dictionary of Psychology. Altun I, Cınar N, Dede C. The contributing factors to poor sleep experiences in according to the university students: A cross-sectional study . J Res Med Sci . 2012;17(6):557-561. PMID:23626634 Skelly AC, Dettori JR, Brodt ED. Assessing bias: The importance of considering confounding . Evid Based Spine Care J . 2012;3(1):9-12. doi:10.1055/s-0031-1298595 - Evans, AN & Rooney, BJ. Methods in Psychological Research. Thousand Oaks, CA: SAGE Publications; 2014.
- Kantowitz, BH, Roediger, HL, & Elmes, DG. Experimental Psychology. Stamfort, CT: Cengage Learning; 2015.
By Kendra Cherry, MSEd Kendra Cherry, MS, is a psychosocial rehabilitation specialist, psychology educator, and author of the "Everything Psychology Book." Independent and Dependent VariablesThis guide discusses how to identify independent and dependent variables effectively and incorporate their description within the body of a research paper. A variable can be anything you might aim to measure in your study, whether in the form of numerical data or reflecting complex phenomena such as feelings or reactions. Dependent variables change due to the other factors measured, especially if a study employs an experimental or semi-experimental design. Independent variables are stable: they are both presumed causes and conditions in the environment or milieu being manipulated. Identifying Independent and Dependent VariablesEven though the definitions of the terms independent and dependent variables may appear to be clear, in the process of analyzing data resulting from actual research, identifying the variables properly might be challenging. Here is a simple rule that you can apply at all times: the independent variable is what a researcher changes, whereas the dependent variable is affected by these changes. To illustrate the difference, a number of examples are provided below. - The purpose of Study 1 is to measure the impact of different plant fertilizers on how many fruits apple trees bear. Independent variable : plant fertilizers (chosen by researchers) Dependent variable : fruits that the trees bear (affected by choice of fertilizers)
- The purpose of Study 2 is to find an association between living in close vicinity to hydraulic fracturing sites and respiratory diseases. Independent variable: proximity to hydraulic fracturing sites (a presumed cause and a condition of the environment) Dependent variable: the percentage/ likelihood of suffering from respiratory diseases
Confusion is possible in identifying independent and dependent variables in the social sciences. When considering psychological phenomena and human behavior, it can be difficult to distinguish between cause and effect. For example, the purpose of Study 3 is to establish how tactics for coping with stress are linked to the level of stress-resilience in college students. Even though it is feasible to speculate that these variables are interdependent, the following factors should be taken into account in order to clearly define which variable is dependent and which is interdependent. - The dependent variable is usually the objective of the research. In the study under examination, the levels of stress resilience are being investigated.
- The independent variable precedes the dependent variable. The chosen stress-related coping techniques help to build resilience; thus, they occur earlier.
Writing Style and StructureUsually, the variables are first described in the introduction of a research paper and then in the method section. No strict guidelines for approaching the subject exist; however, academic writing demands that the researcher make clear and concise statements. It is only reasonable not to leave readers guessing which of the variables is dependent and which is independent. The description should reflect the literature review, where both types of variables are identified in the context of the previous research. For instance, in the case of Study 3, a researcher would have to provide an explanation as to the meaning of stress resilience and coping tactics. In properly organizing a research paper, it is essential to outline and operationalize the appropriate independent and dependent variables. Moreover, the paper should differentiate clearly between independent and dependent variables. Finding the dependent variable is typically the objective of a study, whereas independent variables reflect influencing factors that can be manipulated. Distinguishing between the two types of variables in social sciences may be somewhat challenging as it can be easy to confuse cause with effect. Academic format calls for the author to mention the variables in the introduction and then provide a detailed description in the method section. Unfortunately, your browser is too old to work on this site. For full functionality of this site it is necessary to enable JavaScript. Our websites may use cookies to personalize and enhance your experience. By continuing without changing your cookie settings, you agree to this collection. For more information, please see our University Websites Privacy Notice . Neag School of Education Educational Research Basics by Del SiegleEach person/thing we collect data on is called an OBSERVATION (in our work these are usually people/subjects. Currently, the term participant rather than subject is used when describing the people from whom we collect data). OBSERVATIONS (participants) possess a variety of CHARACTERISTICS . If a CHARACTERISTIC of an OBSERVATION (participant) is the same for every member of the group (doesn’t vary) it is called a CONSTANT . If a CHARACTERISTIC of an OBSERVATION (participant) differs for group members it is called a VARIABLE . In research we don’t get excited about CONSTANTS (since everyone is the same on that characteristic); we’re more interested in VARIABLES. Variables can be classified as QUANTITATIVE or QUALITATIVE (also known as CATEGORICAL). QUANTITATIVE variables are ones that exist along a continuum that runs from low to high. Ordinal, interval, and ratio variables are quantitative. QUANTITATIVE variables are sometimes called CONTINUOUS VARIABLES because they have a variety (continuum) of characteristics. Height in inches and scores on a test would be examples of quantitative variables. QUALITATIVE variables do not express differences in amount, only differences. They are sometimes referred to as CATEGORICAL variables because they classify by categories. Nominal variables such as gender, religion, or eye color are CATEGORICAL variables. Generally speaking, categorical variables Categorical variables are groups…such as gender or type of degree sought. Quantitative variables are numbers that have a range…like weight in pounds or baskets made during a ball game. When we analyze data we do turn the categorical variables into numbers but only for identification purposes…e.g. 1 = male and 2 = female. Just because 2 = female does not mean that females are better than males who are only 1. With quantitative data having a higher number means you have more of something. So higher values have meaning. | A special case of a CATEGORICAL variable is a DICHOTOMOUS VARIABLE. DICHOTOMOUS variables have only two CHARACTERISTICS (male or female). When naming QUALITATIVE variables, it is important to name the category rather than the levels (i.e., gender is the variable name, not male and female). Variables have different purposes or roles… Independent (Experimental, Manipulated, Treatment, Grouping) Variable- That factor which is measured, manipulated, or selected by the experimenter to determine its relationship to an observed phenomenon. “In a research study, independent variables are antecedent conditions that are presumed to affect a dependent variable. They are either manipulated by the researcher or are observed by the researcher so that their values can be related to that of the dependent variable. For example, in a research study on the relationship between mosquitoes and mosquito bites, the number of mosquitoes per acre of ground would be an independent variable” (Jaeger, 1990, p. 373) While the independent variable is often manipulated by the researcher, it can also be a classification where subjects are assigned to groups. In a study where one variable causes the other, the independent variable is the cause. In a study where groups are being compared, the independent variable is the group classification. Dependent (Outcome) Variable- That factor which is observed and measured to determine the effect of the independent variable, i.e., that factor that appears, disappears, or varies as the experimenter introduces, removes, or varies the independent variable. “In a research study, the independent variable defines a principal focus of research interest. It is the consequent variable that is presumably affected by one or more independent variables that are either manipulated by the researcher or observed by the researcher and regarded as antecedent conditions that determine the value of the dependent variable. For example, in a study of the relationship between mosquitoes and mosquito bites, the number of mosquito bites per hour would be the dependent variable” (Jaeger, 1990, p. 370). The dependent variable is the participant’s response. The dependent variable is the outcome. In an experiment, it may be what was caused or what changed as a result of the study. In a comparison of groups, it is what they differ on. Moderator Variable- That factor which is measured, manipulated, or selected by the experimenter to discover whether it modifies the relationship of the independent variable to an observed phenomenon. It is a special type of independent variable. The independent variable’s relationship with the dependent variable may change under different conditions. That condition is the moderator variable. In a study of two methods of teaching reading, one of the methods of teaching reading may work better with boys than girls. Method of teaching reading is the independent variable and reading achievement is the dependent variable. Gender is the moderator variable because it moderates or changes the relationship between the independent variable (teaching method) and the dependent variable (reading achievement). Suppose we do a study of reading achievement where we compare whole language with phonics, and we also include studentsâ social economic status (SES) as a variable. The students are randomly assigned to either whole language instruction or phonics instruction. There are students of high and low SES in each group. Letâs assume that we found that whole language instruction worked better than phonics instruction with the high SES students, but phonics instruction worked better than whole language instruction with the low SES students. Later you will learn in statistics that this is an interaction effect. In this study, language instruction was the independent variable (with two levels: phonics and whole language). SES was the moderator variable (with two levels: high and low). Reading achievement was the dependent variable (measured on a continuous scale so there arenât levels). With a moderator variable, we find the type of instruction did make a difference, but it worked differently for the two groups on the moderator variable. We select this moderator variable because we think it is a variable that will moderate the effect of the independent on the dependent. We make this decision before we start the study. If the moderator had not been in the study above, we would have said that there was no difference in reading achievement between the two types of reading instruction. This would have happened because the average of the high and low scores of each SES group within a reading instruction group would cancel each other an produce what appears to be average reading achievement in each instruction group (i.e., Phonics: Lowâ6 and Highâ2; Whole Language:  Lowâ2 and Highâ6; Phonics has an average of 4 and Whole Language has an average of 4. If we just look at the averages (without regard to the moderator), it appears that the instruction types produced similar results). Extraneous Variable- Those factors which cannot be controlled. Extraneous variables are independent variables that have not been controlled. They may or may not influence the results. One way to control an extraneous variable which might influence the results is to make it a constant (keep everyone in the study alike on that characteristic). If SES were thought to influence achievement, then restricting the study to one SES level would eliminate SES as an extraneous variable. Here are some examples similar to your homework: Null Hypothesis: Students who receive pizza coupons as a reward do not read more books than students who do not receive pizza coupon rewards. Independent Variable: Reward Status Dependent Variable: Number of Books Read High achieving students do not perform better than low achieving student when writing stories regardless of whether they use paper and pencil or a word processor. Independent Variable: Instrument Used for Writing Moderator Variable: Ability Level of the Students Dependent Variable: Quality of Stories Written When we are comparing two groups, the groups are the independent variable. When we are testing whether something influences something else, the influence (cause) is the independent variable. The independent variable is also the one we manipulate. For example, consider the hypothesis “Teachers given higher pay will have more positive attitudes toward children than teachers given lower pay.” One approach is to ask ourselves “Are there two or more groups being compared?” The answer is “Yes.” “What are the groups?” Teachers who are given higher pay and teachers who are given lower pay. Therefore, the independent variable is teacher pay (it has two levels– high pay and low pay). The dependent variable (what the groups differ on) is attitude towards school. We could also approach this another way. “Is something causing something else?” The answer is “Yes.” “What is causing what?” Teacher pay is causing attitude towards school. Therefore, teacher pay is the independent variable (cause) and attitude towards school is the dependent variable (outcome). Research Questions and Hypotheses The research question drives the study. It should specifically state what is being investigated. Statisticians often convert their research questions to null and alternative hypotheses. The null hypothesis states that no relationship (correlation study) or difference (experimental study) exists. Converting research questions to hypotheses is a simple task. Take the questions and make it a positive statement that says a relationship exists (correlation studies) or a difference exists (experiment study) between the groups and we have the alternative hypothesis. Write a statement that a relationship does not exist or a difference does not exist and we have the null hypothesis. Format for sample research questions and accompanying hypotheses: Research Question for Relationships: Is there a relationship between height and weight? Null Hypothesis: There is no relationship between height and weight. Alternative Hypothesis:  There is a relationship between height and weight. When a researcher states a nondirectional hypothesis in a study that compares the performance of two groups, she doesnât state which group she believes will perform better. If the word âmoreâ or âlessâ appears in the hypothesis, there is a good chance that we are reading a directional hypothesis. A directional hypothesis is one where the researcher states which group she believes will perform better. Most researchers use nondirectional hypotheses. We usually write the alternative hypothesis (what we believe might happen) before we write the null hypothesis (saying it wonât happen). Directional Research Question for Differences: Do boys like reading more than girls? Null Hypothesis:  Boys do not like reading more than girls. Alternative Hypothesis:  Boys do like reading more than girls. Nondirectional Research Question for Differences: Is there a difference between boys’ and girls’ attitude towards reading? –or– Do boys’ and girls’ attitude towards reading differ? Null Hypothesis:  There is no difference between boys’ and girls’ attitude towards reading. –or– Boys’ and girls’ attitude towards reading do not differ. Alternative Hypothesis:  There is a difference between boys’ and girls’ attitude towards reading. –or– Boys’ and girls’ attitude towards reading differ. Del Siegle, Ph.D. Neag School of Education – University of Connecticut [email protected] www.delsiegle.com Kraemer Family LibraryHow to recognize nurs study methodology. - How to use this guide
- Primary | Secondary
- Observational | Experimental
- Cross Sectional | Longitudinal
- Prospective | Retrospective
- Independent | Dependent Variables
Independent v Dependent Variables Variables are any characteristics in the study that can take on different values. The main difference between independent and dependent variables is cause and effect. The independent variable is not expected to be impacted by the study (it's independent), but to cause the difference in the dependent variable. The dependent variable is the effect. The dependent variable expected to change because of the independent variable (it depends on the other factors involved). Independent Variables - What to look forIs this a variable that the researchers deliberately introduced or that would have occurred regardless of the study? The independent variable is the cause, not the effect. So if researchers introduce something in the experiment, like an intervention, that's the independent variable. For observational studies, the independent variable is what was already present in the patients before the outcome that's being measured. An observational study wants to know if patients who worked high stress jobs had more strokes. Having a high stress job is the independent variable. It's not really the variable that's being measured. It's the variable that may or may not cause strokes. An experimental study wants to know if training soccer players on knee stability exercises reduces the number of injuries in a season. The knee stability training is the independent variable. Here, the researchers deliberately introduced training on knee stability exercises. It's not what they want to measure; they want to measure injuries. But this variable that they've introduced is what may or may not cause a reduction in injuries. Dependent Variables - What to look forIs this the variable that is being studied/measured? The easiest way to know what is the dependent variable is to look at what the study is trying to measure. That's the dependent variable, it's what the researchers expect will be impacted by other factors in the study, it's the factor that they're wanting to measure. If this is an experimental study, is this the variable that would be impacted by the intervention? The dependent variable depends on the other variables. It is the thing that will be affected by the other variables in the study. An observational study wants to know if patients who worked high stress jobs had more strokes. Having or not having a stroke is the dependent variable. An experimental study wants to know if training soccer players on knee stability exercises reduces the number of injuries in a season. The number of injuries in the season is the dependent variable. - << Previous: Prospective | Retrospective
- Last Updated: Jul 12, 2024 4:37 PM
- URL: https://libguides.uccs.edu/nurs-methods
Need Research Assistance? Get Help Variables in Quantitative Research: A Beginner's Guide (COUN)Quantitative variables. Because quantitative methodology requires measurement, the concepts being investigated need to be defined in a way that can be measured. Organizational change, reading comprehension, emergency response, or depression are concepts, but they cannot be measured as such. Frequency of organizational change, reading comprehension scores, emergency response time, or types of depression can be measured. They are variables (concepts that can vary). - Independent variables (IV).
- Dependent variables (DV).
- Sample variables.
- Extraneous variables.
Independent Variables (IV)Independent variables (IV) are those that are suspected of being the cause in a causal relationship. If you are asking a cause and effect question, your IV will be the variable (or variables) that you suspect causes the effect. There are two main sorts of IV—active independent variables and attribute independent variables: - Active IV are interventions or conditions that are being applied to the participants. A special tutorial for the third graders, a new therapy for clients, or a new training program being tested on employees would be active IVs.
- Attribute IV are intrinsic characteristics of the participants that are suspected of causing a result. For example, if you are examining whether gender which is intrinsic to the participants results in higher or lower scores on some skill, gender is an attribute IV.
- Both types of IV can have what are called levels. For example:
- In the example above, the active IV special tutorial , receiving the tutorial is one level, and tutorial withheld (control) is a second level.
- In the same example, being a third grader would be an attribute IV. It could be defined as only one level—being in third grade or you might wish to define it with more than one level, such as first half of third grade and second half of third grade. Indeed, that attribute IV could take many more, for example, if you wished to look at each month of third grade.
Independent variables are frequently called different things depending on the nature of the research question. In predictive questions, where a variable is thought to predict another but it is not yet appropriate to ask whether it causes the other, the IV is usually called a predictor or criterion variable rather than an independent variable. Dependent Variables (DV)- Dependent variables are variables that depend on or are influenced by the independent variables.
- They are outcomes or results of the influence of the independent variable.
- Dependent variables answer the question, "What do I observe happening when I apply the intervention?"
- The dependent variable receives the intervention.
In questions where full clausation is not assumed, such as a predictive question or a question about differences between groups but no manipulation of an IV, the dependent variables are usually called outcome variable s, and the independent variables are usually called the predictor or criterion variables. Sample VariablesIn some studies, some characteristic of the participants must be measured for some reason, but that characteristic is not the IV or the DV. In this case, these are called sample variables. For example, suppose you are investigating whether amount of sleep affects level of concentration in depressed people. In order to obtain a sample of depressed people, a standard test of depression will be given. So the presence or absence of depression will be a sample variable. That score is not used as an IV or a DV, but simply to get the appropriate people into the sample. When there is no measure of a characteristic of the participants, the characteristic is called a sample characteristic . When the characteristic must be measured, it is called a sample variable . Extraneous VariablesExtraneous variables are not of interest to the study, but may influence the dependent variable. For this reason, most quantitative studies attempt to control extraneous variables. The literature should inform you what extraneous variables to account for. For example, in the study of third graders' reading scores, variables such as noise levels in the testing room, the size or lighting or temperature of the room, and whether the children had had a good breakfast might be extraneous variables. There is a special class of extraneous variables called confounding variables. These are variables that can cause the effect we are looking for if they are not controlled for, resulting in a false finding that the IV is effective when it is not. In a study of changes in skill levels in a group of caseworkers after a training program, if the follow-up measure is taken relatively late after the training, the simple effect of practicing the skills might explain improved scores, and the training might be mistakenly thought to be successful when it was not. There are many details about variables not covered in this handout. Please consult any text on research methods for a more comprehensive review. Doc. reference: phd_t2_coun_u02s2_h02_quantvar.html  Research MisconductLearn what research misconduct is, how allegations are handled, and find resources to address misconduct. NIH aims to enable scientific discovery while assuring honesty, transparency, integrity, fair merit-based competition, and protection of intellectual capital and proprietary information. As the largest public funder of biomedical research, NIH sets the standard for innovation and scientific discovery. We promote the highest levels of scientific integrity and public accountability in the conduct of science. The scientific research enterprise is built on a deep foundation of trust and shared values. As the National Academies wrote in their 2019 On Being a Scientist report, "this trust will endure only if the scientific community devotes itself to exemplifying and transmitting the values associated with ethical scientific conduct" Falsifying, fabricating, or plagiarizing data—all forms of research misconduct—are contrary to these values and ethical conduct. What is Research Integrity?Failing to uphold research integrity undermines the public’s confidence and trust in the outcomes of NIH supported research. Research integrity includes: - the use of honest and verifiable methods in proposing, performing, and evaluating research
- reporting research results with particular attention to adherence to rules, regulations, and guidelines
- following commonly accepted professional codes or norms
Shared values in scientific research- Honesty: convey information truthfully and honoring commitments
- Accuracy: report findings precisely and take care to avoid errors
- Efficiency: use resources wisely and avoid waste
- Objectivity: let the facts speak for themselves and avoid bias
How does research integrity affect you?- Researchers rely on trustworthy results of other researchers to make scientific progress.
- Researchers rely on public support, whether through public investments or their voluntary participation in research, to further science.
- The public relies on scientific progress to better the lives of everyone.
- The public could actually be harmed by researchers who are dishonest and act without regards to integrity
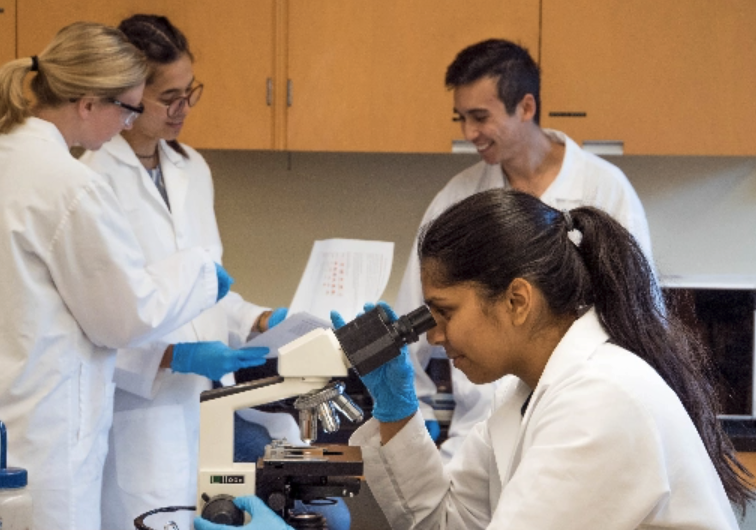 About Research Misconduct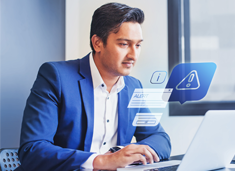 Reporting a Concern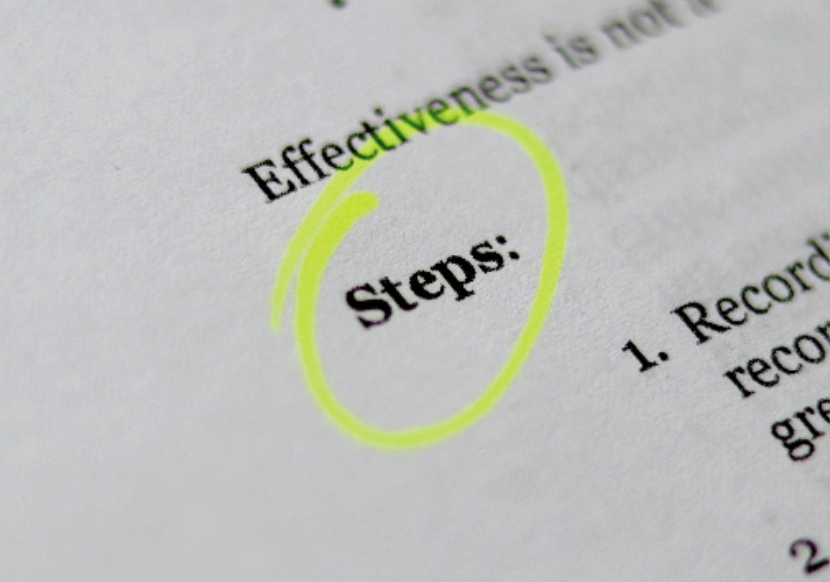 Process for Handling Allegations of Research Misconduct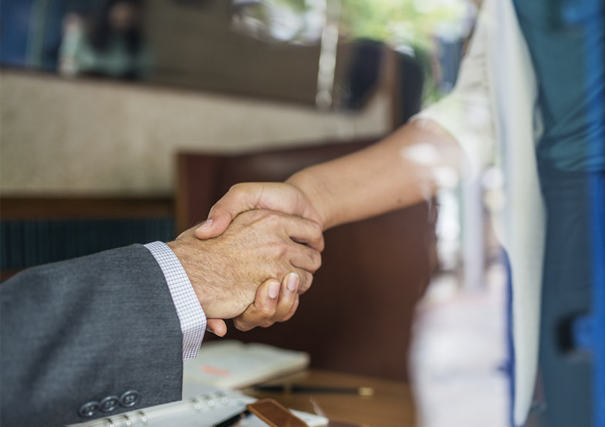 NIH Expectations, Policies, and Requirements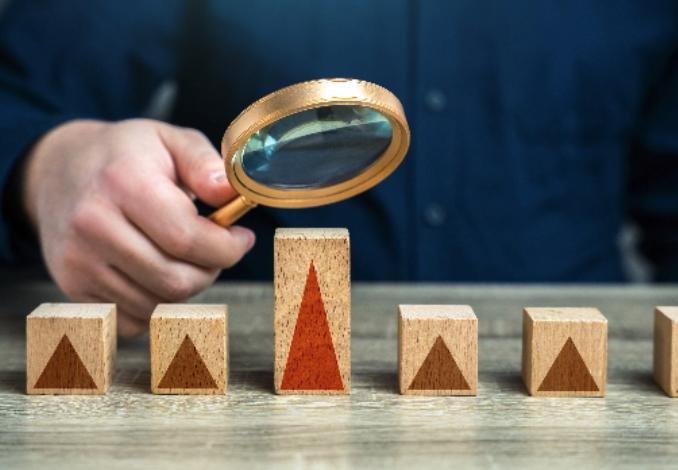 NIH Actions and Oversight after a Finding of Research Misconduct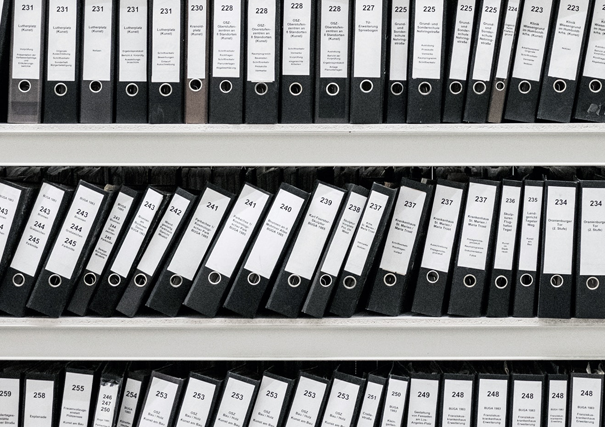 Notices, Statements and Reports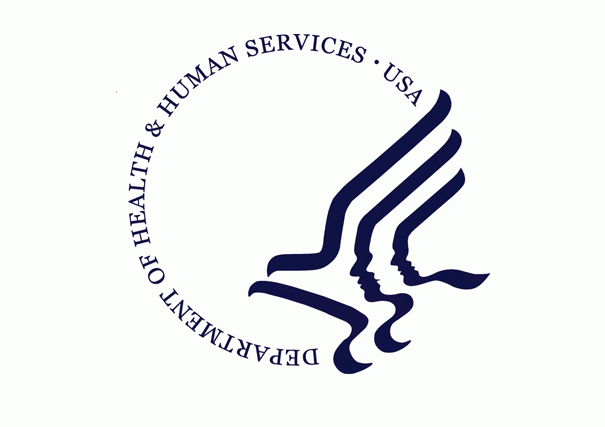 Responsible Conduct of Research (RCR)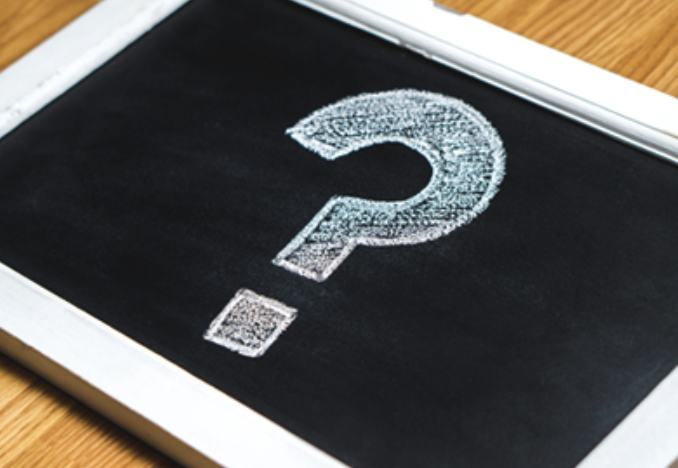 Resources for NIH StaffThis page last updated on: July 15, 2024 - Bookmark & Share
- E-mail Updates
- Privacy Notice
- Accessibility
- National Institutes of Health (NIH), 9000 Rockville Pike, Bethesda, Maryland 20892
- NIH... Turning Discovery Into Health
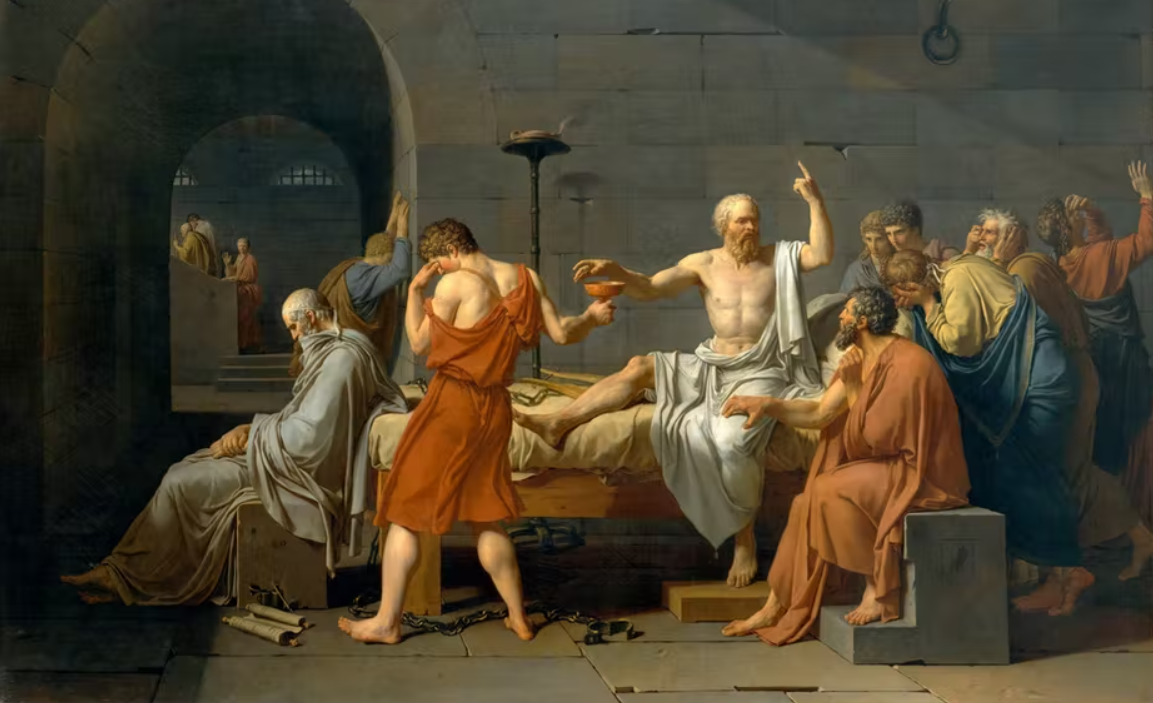 PHILO-notes Free Online Learning Materials What are Variables and Why are They Important in Research?In research, variables are crucial components that help to define and measure the concepts and phenomena under investigation. Variables are defined as any characteristic or attribute that can vary or change in some way. They can be measured, manipulated, or controlled to investigate the relationship between different factors and their impact on the research outcomes. In this essay, I will discuss the importance of variables in research, highlighting their role in defining research questions, designing studies, analyzing data, and drawing conclusions. Defining Research Questions Variables play a critical role in defining research questions. Research questions are formulated based on the variables that are under investigation. These questions guide the entire research process, including the selection of research methods, data collection procedures, and data analysis techniques. Variables help researchers to identify the key concepts and phenomena that they wish to investigate, and to formulate research questions that are specific, measurable, and relevant to the research objectives. For example, in a study on the relationship between exercise and stress, the variables would be exercise and stress. The research question might be: “What is the relationship between the frequency of exercise and the level of perceived stress among young adults?” Designing Studies Variables also play a crucial role in the design of research studies. The selection of variables determines the type of research design that will be used, as well as the methods and procedures for collecting and analyzing data. Variables can be independent, dependent, or moderator variables, depending on their role in the research design. Independent variables are the variables that are manipulated or controlled by the researcher. They are used to determine the effect of a particular factor on the dependent variable. Dependent variables are the variables that are measured or observed to determine the impact of the independent variable. Moderator variables are the variables that influence the relationship between the independent and dependent variables. For example, in a study on the effect of caffeine on athletic performance, the independent variable would be caffeine, and the dependent variable would be athletic performance. The moderator variables could include factors such as age, gender, and fitness level. Analyzing Data Variables are also essential in the analysis of research data. Statistical methods are used to analyze the data and determine the relationships between the variables. The type of statistical analysis that is used depends on the nature of the variables, their level of measurement, and the research design. For example, if the variables are categorical or nominal, chi-square tests or contingency tables can be used to determine the relationships between them. If the variables are continuous, correlation analysis or regression analysis can be used to determine the strength and direction of the relationship between them. Drawing Conclusions Finally, variables are crucial in drawing conclusions from research studies. The results of the study are based on the relationship between the variables and the conclusions drawn depend on the validity and reliability of the research methods and the accuracy of the statistical analysis. Variables help to establish the cause-and-effect relationships between different factors and to make predictions about the outcomes of future events. For example, in a study on the effect of smoking on lung cancer, the independent variable would be smoking, and the dependent variable would be lung cancer. The conclusion would be that smoking is a risk factor for lung cancer, based on the strength and direction of the relationship between the variables. In conclusion, variables play a crucial role in research across different fields and disciplines. They help to define research questions, design studies, analyze data, and draw conclusions. By understanding the importance of variables in research, researchers can design studies that are relevant, accurate, and reliable, and can provide valuable insights into the phenomena under investigation. Therefore, it is essential to consider variables carefully when designing, conducting, and interpreting research studies. - Research Note
- Open access
- Published: 17 July 2024
Thermographic analysis of perforations in polyurethane blocks performed with experimental conical drill bit in comparison to conventional orthopedic drill bit: a preliminary study- InĂĄcio Bernhardt Rovaris 1 ,
- Anderson Luiz de Carvalho 2 ,
- Gabriel Aardewijn Silva 3 ,
- Daniel GuimarĂŁes Gerardi 1 &
- Marcelo Meller Alievi 1 Â
BMC Research Notes volume  17 , Article number: 197 ( 2024 ) Cite this article 52 Accesses Metrics details Conical orthopedic drill bits may have the potential to improve the stabilization of orthopedic screws. During perforations, heat energy is released, and elevated temperatures could be related to thermal osteonecrosis. This study was designed to evaluate the thermal behavior of an experimental conical drill bit, when compared to the conventional cylindrical drill, using polyurethane blocks perforations. The sample was divided into two groups, according to the method of drilling, including 25 polyurethane blocks in each: In Group 1, perforations were performed with a conventional orthopedic cylindrical drill; while in Group 2, an experimental conical drill was used. No statistically significant difference was observed in relation to the maximum temperature (MT) during the entire drilling in the groups, however the perforation time (PT) was slightly longer in Group 2. Each drill bit perforated five times and number of perforations was not correlated with a temperature increase, when evaluated universally or isolated by groups. The PT had no correlation with an increase in temperature when evaluating the perforations universally ( n â=â50) and in Group 1 alone; however, Group 2 showed an inversely proportional correlation for these variables, indicating that, for the conical drill bit, drillings with longer PT had lower MT. Peer Review reports IntroductionBone perforation with orthopedic drill bits is present in most orthopedic, orthodontic and neurosurgery procedures [ 1 , 2 , 3 ]. During the drill rotations in the creation of bone holes for the introduction of implants, friction is generated at the interface of the drill and bone, releasing energy in the form of heat [ 4 ]. Several factors influence the temperature of bone drilling, including tool design, cutting depth, rotational speed, axial loading, irrigation technique, and bone density [ 4 , 5 ]. Shu et al. evaluated the cellular damage of osteoblasts in the face of elevated temperatures and observed that increasing temperature and its exposure time is directly related to cell death [ 5 ]. This type of injury is denominated thermal necrosis. Thermal necrosis may be related to cell apoptosis and reabsorption, infection, and early loosening of implants, resulting in direct or indirect loss of stability of the osteosynthesis fixation systems [ 6 , 7 , 8 ]. Infrared cameras have been used in several studies for conducting thermal tests on bone drilling [ 4 , 5 , 6 , 9 , 10 , 11 , 12 , 13 ]. The development of conical orthopedic devices is related to the need to increase implant resistance and provide greater stability for fixation, reducing complication rates [ 14 , 15 , 16 ]. The aim of this work is to evaluate the thermal behavior of an experimental conical drill, which presents a possible important mechanical potential, when compared to the conventional orthopedic cylindrical drill. Our hypothesis is that the experimental conical drill does not produce more heat compared to the conventional orthopedic cylindrical drill, when drilling in polyurethane blocks, which would enable it, from a thermal perspective, to be evaluated in future biomechanics assays. Materials and methodsPolyurethane blocks and groups. Bicortical polyurethane blocks (PB) (Nacional Ossos Âź , SĂŁo Paulo, Brazil) were used, with a density of 40 pounds per cubic foot (PCF)/(0.96 g/cm 3 ) in the two cortical (2 mm thickness); and 20 PCF/(0.16 g/cm 3 ) in its central part (30 mm thickness), which represents the medullary portion. The PB had total dimensions of 47.5 mm x 45 mm x 34 mm (Fig. 1 A), respecting the Brazilian Technical Standards Association (ABNT) NBR15678 and NBR15675-4 regulations [ 17 , 18 ], referring to the use of rigid polyurethane foam for testing implants and the test method for determination of axial pullout resistance, respectively. 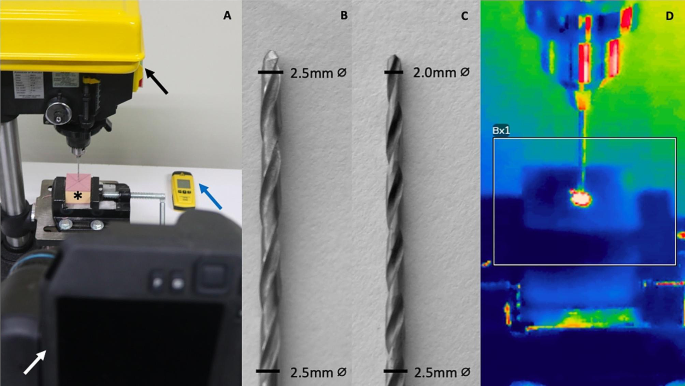 A : Studio with opaque colored background for thermographic tests, containing a vertical drilling machine (VONDER Âź FBV013 1/2 In. 1/3HP) (black arrow), a polyurethane block in a 6-inch bench vise (VONDER Âź ) (asterisk), a digital tachometer (VONDER Âź TDV 100) (blue arrow), and an infrared thermography camera (FLIR Âź T530, Danderyd, Sweden) (white arrow). B : Conventional orthopedic cylindrical drill C : Experimental conical drill. D : Thermographic image of the perforation For this study, the sample was divided into two groups according to the method of drilling, including 25 PB in each group: Group 1 (G1): Drilling with conventional orthopedic cylindrical drill (CCD), 2.5 mm (Fig. 1 B). Group 2 (G2): Drilling with experimental conical drill (ECD), 2.5â2.0 mm (Fig. 1 C). Thermographic assessmentThermographic tests were performed in a studio set up with opaque colored backgrounds and thermally regulated by a temperature conditioning system. It was established that the ambient temperature would be 22 °C and that all equipment, including implants and PBs, would need to be exposed to this temperature for at least four hours, aiming uniformity and thermal stability. Thermographic images were obtained at 30 fps using an infrared thermography camera T530 (FLIR Âź , Danderyd, Sweden) (Fig. 1 A), positioned on a level tripod at a distance of 0.5 m from the PB, with an inclination of 30°, and adjusted to an emissivity of 0.98 for filming. After accommodating and leveling the PB in the vertical drilling machine, a brief thermal stabilization of the PB surface was awaited before drilling was executed, recording the act of drilling and the post-drilling observation period of 40s (Fig. 1 D). The images were analyzed by Thermal Studio software v2.0.6 (FLIR Âź , Danderyd, Sweden), considering perforation time (PT) and maximum temperature during the entire drilling (MT) at the perforation area (rectangle highlighted in Fig. 1 D). In G1, five identical cylindrical drill bits (CCD) made of stainless steel (AISI 420B) (CĂŁomedica Âź , SĂŁo Paulo, Brazil) were used, with the same diameter (2.5 mm) and length (150 mm). The length of the shaft was 100 mm, the length of the helix was 50 mm with an angle of 25° and the point angle was 90°. In G2, five identical experimental conical drills (ECD) were used, made from the same stainless steel (AISI 420B). We also intend to create the same shank profile (100 mm) and helix (50 mm) as the drills used in G1, but due to its conical shape, it presented a progressive reduction in diameter. The helix starts at 2.5 mm, which remains for 15 mm and after that the diameter of the drill begins to progressively decrease until reaching 2.0 mm at its point. Five drill bits were used in each group (G1 and G2); each drilling five PBs, and each drilling was evaluated separately. Polyurethane blocks perforationsTo perforate the PBs, a vertical drilling machine was used (VONDER Âź FBV013 1/2 In. 1/3HP, ParanĂĄ, Brazil) with rpm regulation, installed on a level surface and fixed to the ground. To stabilize the PBs during perforation, a 6-inch bench vise (VONDER Âź , ParanĂĄ, Brazil) was attached (Fig. 1 A). It was established a rotational speed of 1130 rpm, which was checked before each perforation, using a digital tachometer TDV100 (VONDER Âź , ParanĂĄ, Brazil) (Fig. 1 A). The depth of each drilling was standardized based on the length of the drill bit, with the purpose of the end of the drill bit crossing 2 mm into the far cortical of the PB. This measurement was performed using a castroviejo specimeter. The perforations were executed manually and all by the same operator, occurring in groups of ten PBs (five from each group), aiming to maintain the same pattern in all groups, but allowing small individual variations between perforations, as observed in routine surgical procedures. Perforation times (times between the first contact of the drill with the PB until its complete exit) were obtained and MTs were analyzed using Thermal Studio software v2.0.6 (FLIR Âź , Estocolmo, Sweden). Statistical analysisData were tabulated in Microsoft Excel software v.2016 (Microsoft Corp., Washington, USA). The statistical analysis was performed applying a software program (SPSS Statistics v24.0, IBM Inc. Company, New York, USA). The Kolmogorov-Smirnov test was used to evaluate data for normal distribution. Mean and standard deviation (SD) were used to describe quantitative variables and those were compared between the two groups using the Studentâs t-test (MT and PT). Pearsonsâs test was used to access correlation between quantitative variables (MT and PT, MT and number of perforations). A P -valueââ€â0.05 was considered significant for all analysis. Results and discussionThis study evaluated the thermal behavior of perforations in PBs by comparing the use of two orthopedic drill bits with different structural characteristics. Screws inserted in holes drilled by conical drills may have important mechanical potential, and the thermal study of perforations with conical drills is necessary to evaluate the feasibility of their application in future surgical procedures. In the current work, no statistical difference was observed in relation to the mean MT captured during drillings in G1 and G2 (Table 1 ). However, G1 presented the highest MT, comparing to G2 (Fig. 2 A). Recently, Gehrke et al. evaluated the thermal and histological repercussions of using a conical versus a cylindrical drill bit in drilling rabbit tibias and observed that conical drills generated approximately 10% less heat, a statistically significant difference. In the histological comparison, a larger area of new bone formation was observed after 30 days of drilling, better results than those seen in holes made with cylindrical drills [ 19 ]. Shu et al. evaluated in vitro the cellular repercussions of thermal exposure at different temperatures and times for four days, and observed that osteoblasts not only suffered immediate injuries, but also presented important consequences that affected cell viability throughout the follow-up period [ 5 ]. In the present study, all MTs from the perforations of both groups were obtained at the drill exit surface, which can be explained by the accumulation of heat due to friction during drilling [ 5 ]. 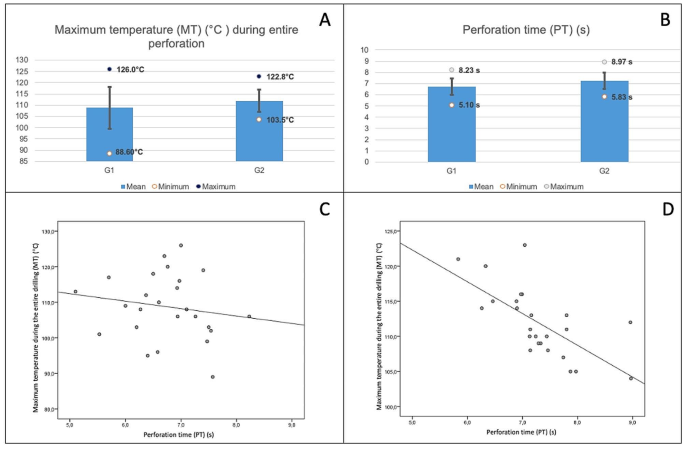 Mean, minimum and maximum values of maximum temperature during the entire drilling (MT) ( A ) and perforation time (PT) ( B ), according to group. The standard deviation is represented by the vertical black bar (A , B ). Correlations between MT and PT in Groups 1 (conventional orthopedic cylindrical drill) ( C ) and 2 (experimental conical drill) ( D ) In the present study, it was observed that there was a statistical difference between the means of the PT of G1 and G2 (Table 1 ; Fig. 2 B). Group 1 had a lower mean PT when compared to G2. However, it is noteworthy that the difference in the mean PT between G1 and G2 was only 0.54s. This time is relatively short when compared to drilling times described by other authors, who evaluated exposure to high temperatures and thermal bone damage from drillings that lasted 15 to 60s [ 4 , 5 , 20 ]. The drill progression speed and, consequently, the PT can influence the drilling temperature. Faster drilling has a shorter heat transfer time to the drilled object. On the other hand, to drill faster, a greater axial force must be applied during drilling, which increases friction, which can lead to an increase in drilling temperature [ 6 , 12 ]. When the PT and MT of the 50 PBs were evaluated together (G1â+âG2), no correlation was observed between these variables ( r =-0.229; p â=â0.11). The same was observed when only G1 perforations were evaluated separately ( r =-0.166; p â=â0.42) (Fig. 2 C), which means, both faster and slower perforations did not influence the MT. Nevertheless, when G2 was evaluated separately, an inversely proportional correlation was observed between PT and MT ( r =-0.687; p â<â0.001) (Fig. 2 D), meaning longer drillings presented lower temperatures compared to faster drillings. Based on these findings, it is suggested that, for ECD, longer drilling times are preferable, as they produce a lower drilling temperature. Shakouri and Nezhad evaluated the CCD drilling temperature in bovine femurs, with different drilling times and different rotational speed, and concluded that faster drillings with higher rpm tend to heat up less, for two main reasons: lower contact time of the drill with the drilled object, and greater capacity to eliminate heat through bone chips [ 4 ]. However, in the present study it was not possible to observe these thermal behaviors during drilling, neither with CCD nor with ECD. Each drill bit from G1 and G2 perforated five PBs. No correlation was found between the number of perforations and MT, even when evaluating the groups together ( r â=â0.047; p â=â0.747) or separately (G1: r â=â0.014; p â=â0.948 and G2: r â=â0.118; p â=â0.576), suggesting that there was no significant wear on the drill bits to influence the perforation temperature over the five perforations of each drill. Alam et al. related the use of worn drills to the need to increase axial force and drilling time, which caused higher temperatures during bone drilling [ 8 ]. However, in the aforementioned work, tests were executed with drills that drilled 50, 100, 150 and 200 times. Therefore, it is feasible to compare the five perforations of each drill in the present study, with no influence on thermal variables depending on the number of perforations. Furthermore, in routine orthopedic procedures, the same drill bit is used to perform several drillings [ 8 ], which allows simulating a surgical reality, with respect to the number of perforations, in the present study. Several studies have evaluated the thermal performance of orthopedic drills and thermal cameras are present in most of these studies, as a non-destructive tool that does not compromise the structure of the drilled component and presents good results [ 4 , 5 , 9 , 13 , 21 ]. On the other hand, thermocouples, which are also equipment used to evaluate drilling temperatures, need to be installed inside the PBs so that it is possible to measure thermal changes through their sensors [ 8 , 19 , 22 ]. Changes to the PB, such as perforations to install thermocouples, can cause areas of structural weakness, preventing other tests, such as biomechanics, to be carried out with the same component [ 17 , 18 ]. In this study, we chose to use the T530 camera (FLIR Âź , Danderyd, Sweden), which applies infrared technology to capture images. Shakouri and Nezhad evaluated the two measurement methodologies in drilling bovine bones and the thermal camera presented reliable results, similar to those of thermocouples [ 4 ]. In conclusion, there was no difference between the means of the maximum temperature during the entire drilling between the two groups (conventional orthopedic cylindrical drill and experimental conical drill), highlighting that, in polyurethane blocks, the experimental conical drill presents similar thermal behavior compared to the conventional orthopedic cylindrical drill. This result encourages biomechanical tests to be conducted with the experimental conical drill. It was also possible to state that longer drilling times with the experimental conical drill resulted in lower drilling temperatures in polyurethane blocks. LimitationsEven though no difference was observed in the mean MT between G1 and G2, and despite ensuring repeatability and homogeneity of PBs, the polyurethane does not have the same thermal characteristics as natural bones [ 23 ], and this highlights the need for further studies to endorse the thermal and mechanical performance of conical drills in surgical procedures. Furthermore, cooling techniques such as irrigation were not used during drilling, due to the characteristics of the polyurethane blocks and the lack of knowledge of their behavior in the face of irrigation. Studies with natural bones and largest samples are necessary to enable such an assessment. Another limiting factor for this type of resource is the possibility of irrigation interfering with the capture of images by the infrared camera. Although most studies use natural bones as a model for evaluating the thermal behavior of orthopedic drills, studies such as those conducted by Pazarcı and GĂŒndoÄdu [ 13 ], Fernandes et al. [ 24 ] and Teixeira et al. [ 25 ] used synthetic bones for this type of evaluation. The statistical difference between the PT of G1 and G2 may have influenced MT, however there was a subtle increase in PT of G2 compared to G1. This can be explained by the fact that the drillings were performed manually. However, this difference was less than one second, being smaller than the standard deviation of both variables, which clinically may not be relevant. Data availabilityThe datasets used and/or analyzed during the current study are available from the corresponding author on reasonable request. AbbreviationsConventional orthopedic cylindrical drill Experimental conical drill Maximum temperature during the entire drilling Polyurethane block Perforation time Kvale E, Kalmukov I, Grassato L, Kalff S, Solano M. Epicondylar plate fixation of humeral condylar fractures in immature French bulldogs: 45 cases (2014â2020). J Small Anim Pract. 2022;63(7):532â41. https://doi.org/10.1111/jsap.13484 Article  CAS  PubMed  Google Scholar  Gilman O, Escauriaza L, Ogden D, Vandenberghe H, Roper D, Oxley B, Granger N. Thoracolumbar spinal stabilization with three dimensional-printed drill guides and pre-contoured polyaxial bone plates. Vet Comp Orthop Traumatol. 2023;36(1):46â52. https://doi.org/10.1055/s-0042-1756514 Article  PubMed  Google Scholar  Kot CCS, Verstraete FJM, Garcia TC, Stover SM, Arzi B. Biomechanical evaluation of locking versus nonlocking 2.0-mm malleable L-miniplate fixation of simulated caudal mandibular fractures in cats. Am J Vet Res. 2022;29(8):ajvr.22.03.0043. https://doi.org/10.2460/ajvr.22.03.0043 Shakouri E, Nezhad MG. An in vitro study of bone drilling: infrared thermography and evaluation of thermal changes of bone and drill bit. Phys Eng Sci Med. 2020;43:247â57. https://doi.org/10.1007/s13246-020-00842-x Article  Google Scholar  Shu L, Bai W, Shimada T, Ying Z, Li S, Sugita N. Thermographic assessment of heat-induced cellular damage during orthopedic surgery. Med Eng Phys. 2020;83:100â5. https://doi.org/10.1016/j.medengphy.2020.05.014 Augustin G, Zigman T, Davila S, Udilljak T, Staroveski T, Brezak D, Babic S. Cortical bone drilling and thermal osteonecrosis. Clin Biomech. 2012;27(4):313â25. https://doi.org/10.1016/j.clinbiomech.2011.10.010 Kumar VK, Prasad K, Sansgiri T, Ranganath K, Shwetha V, Al-Khuteja K. Self-drilling versus self-tapping screws: a 3D finite element analysis. Craniomaxillofac Trauma Reconstr. 2021;14(1):4â10. https://doi.org/10.1177/1943387520904212 Alam K, Qamar SZ, Iqbal M, Piya S, Al-Kindi M, Qureshi A, et al. Effect of drill quality on biological damage in bone drilling. Sci Rep. 2023;13:6234. https://doi.org/10.1038/s41598-023-33381-y Article  CAS  PubMed  PubMed Central  Google Scholar  Soriano J, Garay A, Aristimuño P, Iriarte LM, Eguren JA, Arrazola PJ. Effects of rotational speed, feed rate and tool type on temperatures and cutting forces when drilling bovine cortical bone. Mach Sci Technol. 2013;17(4):611â36. https://doi.org/10.1080/10910344.2013.837353 Fernandes MG, Fonseca EM, Jorge RN, CĂ©spedes MCM, Dias MI. Effect of drill speed on the strain distribution during drilling of bovine and human bones. J Biomech Eng. 2018;2(5):69â74. https://doi.org/10.24243/jmeb/2.5.170 Ghazali M, Roseiro L, Garruço A, Margalho L, Expedito F. Pre-drilling vs. self-drilling of pin bone insertionâa thermography experimental evaluation. In: Tavares J, Natal Jorge R, editors. Lecture Notes in Computation Vision and Biomechanics. 2018. https://doi.org/10.1007/978-3-319-68195-5_118 Timon C, Keady C. Thermal osteonecrosis caused by bone drilling in orthopedic surgery: a literature review. Cureus. 2019;11(7):e5226. https://doi.org/10.7759/cureus.5226 Article  PubMed  PubMed Central  Google Scholar  Pazarcı Ă, GĂŒndoÄdu F. Temperature change during orthopedic drilling procedures: an experimental surgical internal fixation simulation study. J Orthop. 2023;46:58â63. https://doi.org/10.1016/j.jor.2023.10.013 Liu MY, Tsai TT, Lai PL, Hsieh MK, Chen LH, Tai CL. Biomechanical comparison of pedicle screw fixation strength in synthetic bones: effects of screw shape, core/thread profile and cement augmentation. PLoS ONE. 2020;15(2):e0229328. https://doi.org/10.1371/journal.pone.0229328 Hodgkins CW, Fleites J. Fibula nailing: a retrospective review of 110 consecutive FibuLock nails. J Orthop Trauma. 2022;36(7):366â9. https://doi.org/10.1097/BOT.0000000000002329 Shahin M, MassĂ© V, Belzile Ă, BĂ©dard L, Angers M, Vendittoli PA. Midterm results of titanium conical Wagner stem with challenging femoral anatomy: survivorship and unique bone remodeling. Orthop Traumatol Surg Res. 2023;109(1):103242. https://doi.org/10.1016/j.otsr.2022.103242 Associação Brasileira de Normas TĂ©cnicas (ABNT). NBR 15678:2020. Implantes para cirurgia - Espuma rĂgida de poliuretano para ensaio de implantes e instrumentos - Requisitos, classificação e mĂ©todos de ensaio (Implants for surgery - Rigid polyurethane foam for implants and instruments testing - Requirements, classification and test methods). 2020. https://www.abntcatalogo.com.br/pnm.aspx?Q=R3B0TnlLVCt2cTZCMmRzcUg2UVhPZ01IOUsxaGRpMkZJbjJMNW9RWE9kYz0 =. Accessed 9 Dez 2023. Associação Brasileira de Normas TĂ©cnicas (ABNT). NBR 15675-4:2009. Implantes para ortopedia - Parafusos Ăłsseos metĂĄlicos. Parte 4: MĂ©todo de ensaio para determinação da resistĂȘncia ao arrancamento axial (Orthopedics implants - Metallic bone screws. Part 4: Test method for determining the axial pullout strength). 2009. https://www.abntcatalogo.com.br/pnm.aspx?Q=OXBEUm1jMG8rbWxOdFdwbTE0UmRHUFhrR1pDNHNIbkNHeVZ6akMwclRvcz0 =. Accessed 9 Dez 2023. Gehrke SA, Treichel TLE, AramburĂș JĂșnior J, de Aza PN, Prados-Frutos JC. Effects of the technique and drill design used during the osteotomy on the thermal and histological stimulation. Sci Rep. 2020;10:20737. https://doi.org/10.1038/s41598-020-77762-z Eriksson RA, Adell R. Temperatures during drilling for the placement of implants using the osseointegration technique. J Oral Maxillofac Surg. 1986;44(1):4â7. https://doi.org/10.1016/0278-2391(86)90006-6 Scarano A, Lorusso F, Noumbissi S. Infrared thermographic evaluation of temperature modifications induced during implant site preparation with steel vs. Zirconia implant drill. J Clin Med. 2020;9(1):148. https://doi.org/10.3390/jcm9010148 Sui J, Wang C, Sugita N. Experimental study of temperature rise during bone drilling process. Med Eng Phys. 2020;78:64â73. https://doi.org/10.1016/j.medengphy.2020.01.007 Szalma J, LovĂĄsz BV, Vajta L, SoĂłs B, Lempel E, Möhlhenrich SC. The influence of the chosen in vitro bone simulation model on intraosseous temperatures and drilling times. Sci Rep. 2019;9(1):11817. https://doi.org/10.1038/s41598-019-48416-6 Fernandes MGA, Fonseca EMM, Natal. Thermal analysis during bone drilling using rigid polyurethane foams: numerical and experimental methodologies. J Braz Soc Mech Sci Eng. 2016;38:1855â63. https://doi.org/10.1007/s40430-016-0560-4 Article  CAS  Google Scholar  Teixeira TAS, Fernandes MG, Azevedo LD, Fonseca EMM, Jorge RN. Influence of drill bit diameter on drilling of polyurethane foams. Proceedings of the 7th International Conference on Mechanics and Materials in Design. 2017:843â852. Download references AcknowledgementsTo Coordenação de Aperfeiçoamento de Pessoal de NĂvel Superior (CAPES), MinistĂ©rio da Educação, Brazil â Finance code 001. The author M.M.A. is funded by Conselho Nacional de Pesquisa e Desenvolvimento CientĂfico e TecnolĂłgico (CNPq grants #309066/2021-2). This study was financed in part by Coordenação de Aperfeiçoamento de Pessoal de NĂvel Superior, MinistĂ©rio da Educação (CAPES), Brazil â Finance code 001. The author MMA is funded by Conselho Nacional de Pesquisa e Desenvolvimento CientĂfico e TecnolĂłgico (CNPq grants #309066/2021-2). Author informationAuthors and affiliations. Department of Animal Medicine, Federal University of Rio Grande do Sul, Porto Alegre, RS, Brazil InĂĄcio Bernhardt Rovaris, Daniel GuimarĂŁes Gerardi & Marcelo Meller Alievi Department of Veterinary Sciences, Federal University of ParanĂĄ, Palotina, PR, Brazil Anderson Luiz de Carvalho Federal University of Rio Grande do Sul, Porto Alegre, RS, Brazil Gabriel Aardewijn Silva You can also search for this author in PubMed  Google Scholar ContributionsI.B.R. and M.M.A. contributed to the study conception and design. Material preparation and data collection were performed by I.B.R. and G.A.S. Data analyses were performed by I.B.R., A.L.C, D.G.G, G.A.S. and M.M.A. The manuscript was written by I.B.R. and reviewed by M.M.A. All authors read and approved the final manuscript. Corresponding authorCorrespondence to InĂĄcio Bernhardt Rovaris . Ethics declarationsEthics approval and consent to participate. Not applicable. Consent for publicationCompeting interests. The authors declare no competing interests. Additional informationPublisherâs note. Springer Nature remains neutral with regard to jurisdictional claims in published maps and institutional affiliations. Rights and permissionsOpen Access This article is licensed under a Creative Commons Attribution 4.0 International License, which permits use, sharing, adaptation, distribution and reproduction in any medium or format, as long as you give appropriate credit to the original author(s) and the source, provide a link to the Creative Commons licence, and indicate if changes were made. The images or other third party material in this article are included in the articleâs Creative Commons licence, unless indicated otherwise in a credit line to the material. If material is not included in the articleâs Creative Commons licence and your intended use is not permitted by statutory regulation or exceeds the permitted use, you will need to obtain permission directly from the copyright holder. To view a copy of this licence, visit http://creativecommons.org/licenses/by/4.0/ . The Creative Commons Public Domain Dedication waiver ( http://creativecommons.org/publicdomain/zero/1.0/ ) applies to the data made available in this article, unless otherwise stated in a credit line to the data. Reprints and permissions About this articleCite this article. Rovaris, I.B., de Carvalho, A.L., Silva, G.A. et al. Thermographic analysis of perforations in polyurethane blocks performed with experimental conical drill bit in comparison to conventional orthopedic drill bit: a preliminary study. BMC Res Notes 17 , 197 (2024). https://doi.org/10.1186/s13104-024-06862-0 Download citation Received : 21 February 2024 Accepted : 10 July 2024 Published : 17 July 2024 DOI : https://doi.org/10.1186/s13104-024-06862-0 Share this articleAnyone you share the following link with will be able to read this content: Sorry, a shareable link is not currently available for this article. Provided by the Springer Nature SharedIt content-sharing initiative - Bone drilling
- Drill bit design
- Infrared thermography
- Temperature
- Orthopedic drill
BMC Research NotesISSN: 1756-0500  - Open access
- Published: 16 July 2024
Protocol for the development and validation of a Polypharmacy Assessment Score- Jung Yin Tsang  ORCID: orcid.org/0000-0002-0331-2777 1 , 2 , 3 ,
- Matthew Sperrin 2 , 3 ,
- Thomas Blakeman 1 , 2 ,
- Rupert A. Payne 4 &
- Darren M. Ashcroft 2 , 5 Â
Diagnostic and Prognostic Research volume  8 , Article number: 10 ( 2024 ) Cite this article 116 Accesses 4 Altmetric Metrics details An increasing number of people are using multiple medications each day, named polypharmacy. This is driven by an ageing population, increasing multimorbidity, and single disease-focussed guidelines. Medications carry obvious benefits, yet polypharmacy is also linked to adverse consequences including adverse drug events, drug-drug and drug-disease interactions, poor patient experience and wasted resources. Problematic polypharmacy is âthe prescribing of multiple medicines inappropriately, or where the intended benefits are not realisedâ. Identifying people with problematic polypharmacy is complex, as multiple medicines can be suitable for people with several chronic conditions requiring more treatment. Hence, polypharmacy is often potentially problematic, rather than always inappropriate, dependent on clinical context and individual benefit vs risk. There is a need to improve how we identify and evaluate these patients by extending beyond simple counts of medicines to include individual factors and long-term conditions. To produce a Polypharmacy Assessment Score to identify a population with unusual levels of prescribing who may be at risk of potentially problematic polypharmacy. Analyses will be performed in three parts: 1. A prediction model will be constructed using observed medications count as the dependent variable, with age, gender and long-term conditions as independent variables. A â Polypharmacy Assessment Score â will then be constructed through calculating the differences between the observed and expected count of prescribed medications, thereby highlighting people that have unexpected levels of prescribing. Parts 2 and 3 will examine different aspects of validity of the Polypharmacy Assessment Score : 2. To assess âconstruct validityâ, cross-sectional analyses will evaluate high-risk prescribing within populations defined by a range of Polypharmacy Assessment Scores , using both explicit (STOPP/START criteria) and implicit (Medication Appropriateness Index) measures of inappropriate prescribing . 3. To assess âpredictive validityâ, a retrospective cohort study will explore differences in clinical outcomes (adverse drug reactions, unplanned hospitalisation and all-cause mortality) between differing scores . Developing a cross-cutting measure of polypharmacy may allow healthcare professionals to prioritise and risk stratify patients with polypharmacy using unusual levels of prescribing. This would be an improvement from current approaches of either using simple cutoffs or narrow prescribing criteria. Peer Review reports IntroductionPolypharmacy is broadly defined as the use of multiple medicines [ 1 , 2 ]. Over a third of people over 65 are taking more than five regular medicines, with almost a quarter taking eight or more [ 3 ]. This has an ever escalating prevalence, driven by an ageing population, multimorbidity (multiple long-term conditions) and clinical guidance focussed on individual diseases [ 2 , 4 ]. Medications carry clear benefits, yet polypharmacy is linked to adverse consequences including poor patient experience, unplanned hospitalisation and death [ 1 , 3 ]. Adverse reactions and medication errors are directly linked to the number of medicines prescribed, increasing health service utilisation, reducing adherence and decreasing quality of life [ 5 , 6 , 7 ]. In England, it is estimated that 10% of medications are inappropriate and potentially harmful, costing the NHS up to ÂŁ1 billion in medications wastage alone [ 8 ]. Problematic polypharmacy has been defined as âthe prescribing of multiple medications inappropriately, or where the intended benefit of the medication is not realisedâ [ 1 ]. Better methods to identify and evaluate patients with problematic polypharmacy are crucial [ 1 ]. There is no consensus on a definition for polypharmacy, with significant variations in approaches to targeting problematic polypharmacy [ 8 , 9 ]. The World Health Organization defines polypharmacy as four or more medicines, and academic studies most commonly use five or more, with the NHS national polypharmacy indicators starting at eight or more [ 8 , 10 , 11 ]. However, these simple counts or thresholds ignore individual patient factors and clinical appropriateness [ 1 , 12 , 13 ]. This makes it difficult to define and measure outcomes, with interventions being unable to effectively target the optimal population [ 1 , 13 ]. Other targeted approaches frequently adopt drug-specific, explicit prescribing criteria such as STOPP/START or the Beers List [ 13 , 14 , 15 ]. However, these methods focus on individual examples of high-risk prescribing within a limited number of conditions, rather than polypharmacy as a whole [ 1 , 12 ]. There have also been numerous attempts to develop prognostic models for adverse drug reactions, yet to date these have demonstrated inadequacies in performance and clinical application, particularly during external validation [ 16 , 17 ]. Evidence suggests that a comprehensive risk stratification approach is needed to identify and target patients with problematic polypharmacy whilst taking into account individual patient factors [ 1 , 8 , 18 ]. Hence, we propose a novel approach. First, using a regression model, we plan to predict the âexpectedâ count of prescribed medications for each patient, given individual patient characteristics and clinical diagnoses. Then by calculating the discrepancies between the observed and expected count of medications, we can highlight people with unusual levels of prescribing in the context of their clinical and demographic status. This may help prioritise people who are more at risk of problematic polypharmacy. For example, someone on 20 medicines but expected to be taking 5, based on their age and multimorbidity, is likely to require more attention. This protocol describes our approach to the development and validation of a Polypharmacy Assessment Score. Aims and objectivesDevelop a Polypharmacy Assessment Score that accounts for individual patient factors and clinical diagnoses to identify a population with unusual levels of prescribing. Assess the âconstruct validityâ of the score, by estimating the association the Polypharmacy Assessment Score with high-risk prescribing. Estimate âpredictive validityâ of the score, by estimating the risk of adverse outcomes (including adverse drug reactions, hospitalisation and death) within stratified populations of the Polypharmacy Assessment Score . This protocol is guided by the Transparent Reporting of a multivariable prediction model for Individual Prognosis Or Diagnosis (TRIPOD) [ 19 ]. However, although our model uses prediction statistics, there are subtle differences compared with the construction of a traditional prediction model. In particular, as defining whether polypharmacy is actually problematic requires implicit clinical judgement, the principal output of the Polypharmacy Assessment Score is to provide a risk stratification approach to identifying unusual levels of prescribing, akin to funnel plot approaches used for audit (Fig. 1 ) [ 20 ]. This approach allows visualisation of the dispersion of outcomes, allowing prioritisation of a group of patients with unexpected levels of prescribing, defined at an acceptable threshold for representativeness and utility. Therefore, clinical representation and appropriateness need to be carefully considered and balanced with predictive performance. For example, deprivation will be excluded, as a person who is more deprived should receive the same medical care as a person who is less deprived if they have the same clinical characteristics. Also, the dependent variable of our model is observed medication count, rather than a clinical outcome for prognostic prediction. 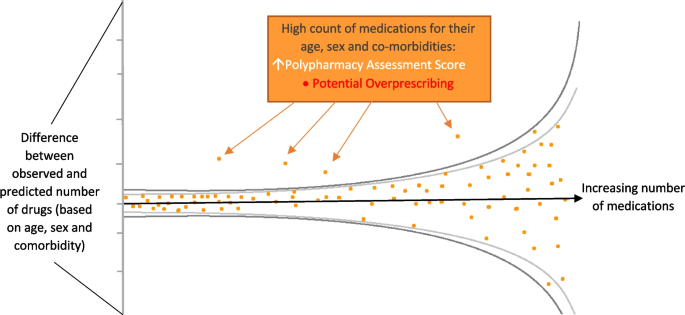 Illustration of the Polypharmacy Assessment Score: a model will determine the predicted count of medications for individual patients given their age, gender and long-term conditions. By then calculating the discrepancy between the observed and predicted number of prescribed medications, the Polypharmacy Assessment Score illustrated by the funnel plot will thus identify those who receive unexpectedly high levels of medicines relative to their multimorbidity Data sourceThe Clinical Practice Research Datalink (CPRD) is a large longitudinal database of general practice electronic healthcare records of over 60 million UK patients. CPRD Aurum data is obtained from the most widely used clinical information system in UK primary care (EMISÂź) [ 21 ]. It includes detailed coded patient-level data on demographics, clinical events, diagnoses and medicines prescribed. The data is nationally representative in terms of age, gender and ethnicity, collated from over 1700 primary care practices in England [ 21 , 22 ]. For additional analyses in objectives 2 and 3, additional patient-level linkages will be requested to English national administrative data on dates and diagnoses for hospital admissions (Hospital Episode Statistics, HES), Office for National Statistics (ONS) mortality records, and small area measures of socioeconomic deprivation (Index of Multiple Deprivation, IMD) based on patient residential postcodes. ParticipantsStudy participants will be aged 40 years or over. Regular medication exposure will be defined by >â90-day prescription length within 180 days and with at least one issue within the last 3 months prior to index date. Participants must have at least 1 year of continuous practice registration before study entry, thus ensuring reliable measures of medication use and baseline covariates. Participants will only be included if records are defined as acceptable for research purposes by CPRD and eligible for HES, ONS and IMD linkages. The study period will be between 1st Jan 2000 and 31st Jan 2020. Random index dates for each patient will be utilised to avoid time-sensitive (e.g. seasonal) variations in prescribing, with a sensitivity analysis performed on fixed calendar time index dates and performing landmark analysis to compare results. Study exit will be defined as ended on the earliest of the following: the patientâs death, the date the patient transferred out of their practice, the last date of data collection from the patientâs practice, or the end of the study period. For objective 1 (prediction model), the primary outcome is the observed count of regular long-term medications. Using this, we can calculate the difference between the observed and predicted count of prescribed medications for individual patients. This difference will represent the Polypharmacy Assessment Score , with a large positive difference (in other words, observed greater than predicted) representing a greater level of potential over-prescribing and a large negative difference representing a greater level of potential under-prescribing (Fig. 1 ). For objective 2 (construct validity), the primary outcome is high-risk prescribing (using both explicit and implicit criteria). For objective 3 (predictive validity), the primary outcome is adverse drug reactions, with secondary outcomes being unplanned hospitalisation and all-cause mortality. Our main predictors include age, gender and long-term conditions. We will include a list of 37 long-term conditions used in CPRD from previous studies and will be guided from by recommendations from the international consensus on definition and measurement multimorbidity [ 4 , 23 , 24 ]. This is due to initial considerations for optimising simplicity, clinical representativeness (e.g. predictors such as deprivation will be intentionally excluded, as explained above) and clinical utility (e.g. other common predictors such as height and weight are likely to have inaccuracies and significant levels of missing data). However, we will explore whether other clinical factors (e.g. smoking, blood pressure) may improve model performance. Sample sizeThe approximate sample size is 8.7 million patients, based on the number of adults aged 40 years and over that are eligible for linkage and limited by random index dates [ 1 ]. Our analyses are exploratory, but following current literature and estimations using a global shrinking factor of 0.9, for a hypothetical model with up to 80 predictors, a standard deviation of 2.5 and an adjusted R-squared of 0.7 based on a previous model on multimorbidity [ 25 ]; an approximate minimum sample of 577 participants (7.21 events per predictor) is required to precisely estimate calibration and discrimination measures for a continuous outcome [ 26 ]. A more conservative estimation, using a lower R-square of 0.5, would require a minimum of 1005 participants (12.6 events per predictor). As such, our large, estimated sample size of 1.5 M participants is sufficient and importantly allows a wide range of different prescribing and patient characteristics to be represented. Missing dataWe will only include patients with complete data on age and gender; this is expected to exclude a negligible number of patients. The absence of a clinical diagnosis code will be taken as the absence of that condition, and so we will not have missing data on conditions. In UK primary care practice, virtually, all prescriptions are issued electronically; missing prescriptions will therefore be assumed to mean no drug has been issued. Partially incomplete prescription data will be handled similar to previous studies by using a stepwise algorithm to impute any missing quantity, dose and duration taking into account previous prescriptions, concurrent prescriptions and other patient and practice prescriptions [ 27 , 28 ]. For objectives 2 and 3, ethnicity and IMD are expected to have small proportions of missing data and will be addressed by creating a missing category. Biological measurements (such as height, weight and blood pressure), smoking status and alcohol use are expected to have missing data. The above variables and other patterns of missing data will be examined, and, depending on the proportion of missing data and established assumptions, missing data will be addressed using a combination of multiple imputation techniques (e.g. predictive mean matching (PMM) for our numeric data and polytomous regression imputation for unordered categorical data), creating a missing category or listwise exclusion for variables containing a small proportion of missing data. Statistical analysis methodsAnalyses will be performed using R software (version 4.3.2) in three parts: Objective 1 (prediction model): A prediction model will be constructed using observed medications count as the dependent variable, with age, gender and long-term conditions as independent variables. A Polypharmacy Assessment Score will then be constructed through calculating the differences between the observed and predicted count of prescribed medications, thereby highlighting people that have unexpected levels of prescribing. We will perform further exploratory analyses to optimise utility of the score, including presenting the score primarily as a continuous scale (e.g. using absolute vs relative differences in observed and predicted medication count) or using an additional categorical output (e.g. drawing thresholds for high, medium and low scores), and this will be further determined based on an analysis on distribution of scores within the population and input from a multidisciplinary panel of experts. Objective 2 (construct validity): Cross-sectional analyses will estimate the prevalence of high-risk prescribing within populations (using both explicit and implicit criteria ) with a range of different Polypharmacy Assessment Scores (e.g. higher, medium and normal scores). Objective 3 (predictive validity): A retrospective cohort study will explore differences in clinical outcomes (adverse drug reactions, unplanned hospitalisation and all-cause mortality) between differing scores of the Polypharmacy Assessment Score , again compared to standard cutoffs of medication count. Handling of predictor variablesThe model will adjust for key predictors including age (continuous variable), gender (binary variable) and multiple long-term conditions (each condition as a binary variable). The model will first derive a âweightâ for each specific long-term condition. As a higher number of medications will be expected for certain clusters of long-term conditions (e.g. diabetes, cardiovascular disease and mental health condition), we will then explore whether accounting for prespecified clusters of conditions may improve the model [ 29 , 30 ]. This will incorporate a range of interaction terms (likely negative, as similar conditions will result in fewer medicines compared to discordant conditions) that need to be included in the model, based on known overlaps in medications for related conditions and a forward selection algorithm to search for further important two-way interactions [ 31 ]. Type of modelWe will use a multivariable Poisson regression model. However, generalised linear frameworks (e.g. quasi-Poisson models), negative binomial regression models and zero-inflated regression models will also be tested for best fit, if there is evidence of overdispersion [ 32 ]. Predictor selection before modellingAs described above, age, gender and multiple long-term conditions have been selected considering clinical representativeness and utility. However, we will explore whether other clinical factors (e.g. smoking, blood pressure, kidney function) may improve model performance. Predictor selection during modellingSelection of terms in the optimal model will be determined using the least absolute shrinkage and selection operator (LASSO) [ 33 ]. To handle specified interactions, we will utilise hierarchical group-lasso regularisation [ 34 ]. Model performancePerformance of the model will be assessed using mean squared error (applied to log counts). Calibration will be assessed using calibration plots and estimation of calibration intercept and slopes along with calibration in the large (CITL) [ 35 ]. Discrimination will be reported using area under the receiver operating characteristics curves (AUROC) reflecting performance at different thresholds, but is not our primary concern as specified above. Internal and external validationObjective 1 (prediction model): We will utilise âinternalâexternal cross validationâ as the most appropriate technique given our large sample size and clustered dataset at practice level [ 36 , 37 ]. This is a recognised method using data from all but one practice to estimate the prediction model and then uses the one remaining practice to evaluate the performance of the model. This process is systematically repeated by rotating the omitted practice to produce multiple estimates of model performance. Unlike standard internal validation methods (e.g. cross-validation and bootstrapping) which compares model reproducibility between individuals from the same population, âinternalâexternal cross validationâ focusses on comparing reproducibility between clusters (in this case â practices) [ 36 , 37 ]. Model performance estimates will be combined using random-effect meta-analysis [ 38 ]. This will evaluate the accuracy of practice-specific performance estimates and also quantify the heterogeneity in model performance across different practices. If the model performs adequately, further external validation may be subsequently performed on external databases, to demonstrate transportability of the Polypharmacy Assessment Score [ 38 ]. Local calibration of the models will still be expected for increased applicability to local contexts during future implementation. Additional analyses for validationTwo further analyses will explore construct and predictive validity of the score: Objective 2 (construct validity): Cross-sectional analyses will first use logistic regression to examine prevalence of high-risk prescribing (composite outcome of drug-drug and drug-disease interactions), using either STOPP/START criteria (the NICE recommended screening tool) for adultsââ„â65 years or the related PROMPT criteria for adultsâ<â65 years, as proxy measures [ 39 , 40 ]. These criteria will be initially analysed as the binary presence of each individual explicit potentially high-risk prescribing criteria. The same random index dates will be utilised as above, and each participant will be followed up for 12 months from index date to estimate prevalence. This will compare the positive predictive value of populations with different levels of Polypharmacy Assessment Score (e.g. higher, medium and normal scores) to standard cutoffs (defined as simple counts of 5, 10 and 15 or more regular medications). A higher positive predictive value detected from patients with higher scores of the Polypharmacy Assessment Score would suggest that it is a better measure of high-risk prescribing than normal scores and simple counts. In addition, a separate cross-sectional analysis will compare a random sample of 30 patients with high and low Polypharmacy Assessment Scores to 30 patients with normal scores (absolute and relative differences evaluated separately) evaluating elements of inappropriate prescribing using the Medication Appropriateness Index, ratified by two independent assessors [ 41 ]. This will enable a further comparison of the sensitivity and specificity of Polypharmacy Assessment Scores in identifying high-risk prescribing. Objective 3 (predictive validity): Several clinical outcomes (primarily adverse drug reactions, but also unplanned hospitalisation, and all-cause mortality) will be assessed in a retrospective cohort study using the above random index dates, with sensitivity analyses on further fixed dates to explore seasonal variations), to examine whether the Polypharmacy Assessment Score predicts relevant outcomes. Exposure will be defined through different levels of Polypharmacy Assessment Scores (e.g. higher vs normal scores). Predictive validity for each outcome will be compared by repeating the cohort study using standard cutoffs (5, 10 or 15 more regular medications) as exposure. Clinical outcomes will be measured at 1- and 5-year follow-up and include adverse drug reactions, unplanned hospitalisation and all-cause mortality using Cox regression. Higher Polypharmacy Assessment Scores are anticipated to exhibit acceptable predictive accuracy for clinical outcomes, and better predictive accuracy for adverse drug reactions compared to normal scores and to lower counts of standard cutoffs (e.g. patients on five or more medications). This would serve as further evidence of validity in relation to outcomes. Compared to current methods, our approach may allow the prioritisation of patients with problematic polypharmacy in a more individualised and holistic manner. Our approach is intentionally pragmatic in adjusting for age, gender and multiple long-term conditions, in order to maximise explainability and implementability, and is designed as a generic measure across medications and conditions for broad applicability. We have further planned qualitative and implementation research with clinical professionals to iteratively explore clinical utility and further develop validity [ 42 ]. There are several limitations to consider. Given the complexities of prescribing decisions, the Polypharmacy Assessment Score will not perfectly identify every individual patient with high-risk prescribing or replace clinical judgement. Some of these complexities will not be captured in routine data, such as the doctor-patient relationship, changing guidelines and differing opinions from specialists [ 43 ]. Although primary care electronic prescription data is reliable, we may miss out exclusively secondary care prescriptions and over-the-counter medications. Whilst not our primary focus, there is also some potential to highlight patients with underprescribing. Appropriateness is not explicitly measured by our score and inherently requires a consultation and shared decision between clinicians and patients [ 1 , 8 ]. Hence, the score only highlights âpotentiallyâ problematic polypharmacy. Therefore, the added value of this score is to prioritise a group of patients with unexpected levels of prescribing given their individual characteristics and multimorbidity, who we hypothesise to be at higher risk of problematic polypharmacy. Availability of data and materialsIn this study, we will use anonymised patient-level data from the CPRD that are not publicly available due to confidentiality considerations. However, researchers can access CPRDâs databases by contacting the CPRD. Details of the application process and conditions of access are available at https://www.cprd.com/data-access . AbbreviationsArea under the receiver operating characteristics curve Calibration-in-the-large Clinical Practice Research Datalink Hospital Episode Statistics Index of Multiple Deprivation Least absolute shrinkage and selection operator National Health Service Office for National Statistics Transparent Reporting of a multivariable prediction model for Individual Prognosis Or Diagnosis Duerden M, Avery T, Payne R. Polypharmacy and medicines optimisation. London: The Kingâs Fund; 2013. Google Scholar  Guthrie B, Makubate B, Hernandez-Santiago V, et al. The rising tide of polypharmacy and drug-drug interactions: population database analysis 1995â2010. BMC Med. 2015;13(1):74. https://doi.org/10.1186/s12916-015-0322-7 . Article  CAS  PubMed  PubMed Central  Google Scholar  Hajjar ER, Cafiero AC, Hanlon JT. Polypharmacy in elderly patients. Am J Geriatr Pharmacother. 2007;5(4):345â51. https://doi.org/10.1016/j.amjopharm.2007.12.002 . Article  PubMed  Google Scholar  Barnett K, Mercer SW, Norbury M, et al. Epidemiology of multimorbidity and implications for health care, research, and medical education: a cross-sectional study. The Lancet. 2012;380(9836):37â43. https://doi.org/10.1016/S0140-6736(12)60240-2 . Article  Google Scholar  Avery AA, Barber N, Ghaleb M, et al. Investigating the prevalence and causes of prescribing errors in general practice: the PRACtICe study. 2012. Gellad WF, Grenard JL, Marcum ZA. A systematic review of barriers to medication adherence in the elderly: looking beyond cost and regimen complexity. Am J Geriatr Pharmacother. 2011;9(1):11â23. https://doi.org/10.1016/j.amjopharm.2011.02.004 . Article  PubMed  PubMed Central  Google Scholar  Nordin Olsson I, Runnamo R, Engfeldt P. Medication quality and quality of life in the elderly, a cohort study. Health Qual Life Outcomes. 2011;9(1):95. https://doi.org/10.1186/1477-7525-9-95 . Article  PubMed Central  Google Scholar  The Department of Health and Social Care. National overprescribing review report: good for you, good for us, good for everybody. 2021. Taghy N, Cambon L, Cohen J-M, et al. Failure to reach a consensus in polypharmacy definition: an obstacle to measuring risks and impactsâresults of a literature review. Ther Clin Risk Manag. 2020;16:57â73. https://doi.org/10.2147/TCRM.S214187 . World Health Organization. Medication without harm: World Health Organization. 2017. NHS Business Services Authority. Medicines Optimisation Polypharmacy Prescribing Comparators. 2017. Gnjidic D, Tinetti M, Allore HG. Assessing medication burden and polypharmacy: finding the perfect measure. Expert Rev Clin Pharmacol. 2017;10(4):345â7. https://doi.org/10.1080/17512433.2017.1301206 . Rankin A, Cadogan CA, Patterson SM, et al. Interventions to improve the appropriate use of polypharmacy for older people. Cochrane Database Syst Rev. 2018;2018(9). https://doi.org/10.1002/14651858.CD008165.pub4 . Cooper JA, Cadogan CA, Patterson SM, et al. Interventions to improve the appropriate use of polypharmacy in older people: a Cochrane systematic review. BMJ Open. 2015;5(12): e009235. https://doi.org/10.1136/bmjopen-2015-009235 . Alldred DP, Kennedy M-C, Hughes C, et al. Interventions to optimise prescribing for older people in care homes. Cochrane Database Syst Rev. 2016;2016(2). https://doi.org/10.1002/14651858.CD009095.pub3 . Stevenson J, Williams JL, Burnham TG, et al. Predicting adverse drug reactions in older adults; a systematic review of the risk prediction models. Clin Interv Aging. 2014:1581. https://doi.org/10.2147/CIA.S65475 . Falconer N, Barras M, Cottrell N. Systematic review of predictive risk models for adverse drug events in hospitalized patients: predictive risk models for adverse drug events in hospitalized patients. Br J Clin Pharmacol. 2018;84(5):846â64. https://doi.org/10.1111/bcp.13514 . Payne RA, Abel GA, Avery AJ, et al. Is polypharmacy always hazardous? A retrospective cohort analysis using linked electronic health records from primary and secondary care: polypharmacy and hospitalization. Br J Clin Pharmacol. 2014;77(6):1073â82. https://doi.org/10.1111/bcp.12292 . Collins GS, Reitsma JB, Altman DG, et al. Transparent reporting of a multivariable prediction model for individual prognosis or diagnosis (TRIPOD): the TRIPOD Statement. BMC Med. 2015;13(1):1. https://doi.org/10.1186/s12916-014-0241-z . Spiegelhalter DJ. Funnel plots for comparing institutional performance. Stat Med. 2005;24(8):1185â202. https://doi.org/10.1002/sim.1970 . Herrett E, Gallagher AM, Bhaskaran K, et al. Data Resource Profile: Clinical Practice Research Datalink (CPRD). Int J Epidemiol. 2015;44(3):827â36. https://doi.org/10.1093/ije/dyv098[publishedOnlineFirst:20150606] . Wolf A, Dedman D, Campbell J, et al. Data resource profile: Clinical Practice Research Datalink (CPRD) Aurum. Int J Epidemiol. 2019;48(6):1740â840. https://doi.org/10.1093/ije/dyz034 . Cassell A, Edwards D, Harshfield A, et al. The epidemiology of multimorbidity in primary care: a retrospective cohort study. Br J Gen Pract. 2018;68(669):e245â51. https://doi.org/10.3399/bjgp18X695465[publishedOnlineFirst:20180312] . Ho ISS, Amaya A-L, Ashley A, et al. Measuring multimorbidity in research: Delphi consensus study. BMJ Medicine. 2022;1(1): e000247. https://doi.org/10.1136/bmjmed-2022-000247 . Payne RA, Mendonca SC, Elliott MN, et al. Development and validation of the Cambridge Multimorbidity Score. CMAJ. 2020;192(5):E107â14. https://doi.org/10.1503/cmaj.190757 . Riley RD, Snell KIE, Ensor J, et al. Minimum sample size for developing a multivariable prediction model: part Iâcontinuous outcomes. Stat Med. 2019;38(7):1262â75. https://doi.org/10.1002/sim.7993 . Pye SR, Sheppard T, Joseph RM, et al. Assumptions made when preparing drug exposure data for analysis have an impact on results: an unreported step in pharmacoepidemiology studies. Pharmacoepidemiol Drug Saf. 2018;27(7):781â8. https://doi.org/10.1002/pds.4440 . Denholm R, Morris R, Payne R. Polypharmacy patterns in the last year of life in patients with dementia. Eur J Clin Pharmacol. 2019;75(11):1583â91. https://doi.org/10.1007/s00228-019-02721-1 . Article  CAS  PubMed  Google Scholar  Forslund T, Carlsson AC, Ljunggren G, et al. Patterns of multimorbidity and pharmacotherapy: a total population cross-sectional study. Fam Pract. 2021;38(2):132â40. https://doi.org/10.1093/fampra/cmaa056 . Zhu Y, Edwards D, Mant J, et al. Characteristics, service use and mortality of clusters of multimorbid patients in England: a population-based study. BMC Med. 2020;18(1):78. https://doi.org/10.1186/s12916-020-01543-8 . Leung TI, Dumontier M. Overlap in drug-disease associations between clinical practice guidelines and drug structured product label indications. J Biomed Semantics. 2016;7:37. https://doi.org/10.1186/s13326-016-0081-1[publishedOnlineFirst:20160608] . Ver Hoef JM, Boveng PL. Quasi-Poisson vs. negative binomial regression: how should we model overdispersed count data? Ecology. 2007;88(11):2766â72. https://doi.org/10.1890/07-0043.1 . Pavlou M, Ambler G, Seaman S, et al. Review and evaluation of penalised regression methods for risk prediction in low-dimensional data with few events. Stat Med. 2016;35(7):1159â77. https://doi.org/10.1002/sim.6782[publishedOnlineFirst:20151029] . Lim M, Hastie T. Learning interactions via hierarchical group-lasso regularization. J Comput Graph Stat. 2015;24(3):627â54. https://doi.org/10.1080/10618600.2014.938812 . Steyerberg EW. Applications of prediction models. New York: Springer Science & Business Media; 2009. Book  Google Scholar  Takada T, Nijman S, Denaxas S, et al. Internal-external cross-validation helped to evaluate the generalizability of prediction models in large clustered datasets. J Clin Epidemiol. 2021;137:83â91. https://doi.org/10.1016/j.jclinepi.2021.03.025 . Debray TP, Vergouwe Y, Koffijberg H, et al. A new framework to enhance the interpretation of external validation studies of clinical prediction models. J Clin Epidemiol. 2015;68(3):279â89. https://doi.org/10.1016/j.jclinepi.2014.06.018[publishedOnlineFirst:20140830] . Debray TP, Moons KG, Ahmed I, et al. A framework for developing, implementing, and evaluating clinical prediction models in an individual participant data meta-analysis. Stat Med. 2013;32(18):3158â80. https://doi.org/10.1002/sim.5732[publishedOnlineFirst:20130111] . OâMahony D, Cherubini A, Guiteras AR, et al. STOPP/START criteria for potentially inappropriate prescribing in older people: version 3. Eur Geriatr Med. 2023;14(4):625â32. Cooper JA, Moriarty F, Ryan C, et al. Potentially inappropriate prescribing in two populations with differing socio-economic profiles: a cross-sectional database study using the PROMPT criteria. Eur J Clin Pharmacol. 2016;72(5):583â91. https://doi.org/10.1007/s00228-015-2003-z . Hanlon JT, Schmader KE. The medication appropriateness index at 20: where it started, where it has been, and where it may be going. Drugs Aging. 2013;30(11):893â900. https://doi.org/10.1007/s40266-013-0118-4 . Tsang JY, Blakeman T, Sperrin M, et al. Identifying, understanding and addressing problematic polypharmacy within multimorbidity in primary care: National Institute for Health and Care Research (NIHR; 2022 [Available from: https://fundingawards.nihr.ac.uk/award/NIHR302624 . Accessed 1 May 2023. Spinewine A, Schmader KE, Barber N, et al. Appropriate prescribing in elderly people: how well can it be measured and optimised? The Lancet. 2007;370(9582):173â84. https://doi.org/10.1016/S0140-6736(07)61091-5 . Download references AcknowledgementsWe would like to acknowledge our patient and public partners for their expert input and ongoing contributions to the work, particularly Manoj Mistry, Philip Bell, Emily Lam and Graham Prestwich. This work was funded by the NIHR Doctoral Fellowship Programme (Ref: NIHR302624). JT, TB and DMA are funded by the NIHR Greater Manchester Patient Safety Research Collaboration. The views expressed in this document are those of the authors and not necessarily those of the NIHR, NHS or the UK Department of Health and Social Care. Author informationAuthors and affiliations. Centre for Primary Care and Health Services Research, School of Health Sciences, University of Manchester, Manchester, M13 9PL, UK Jung Yin Tsang & Thomas Blakeman NIHR Greater Manchester Patient Safety Research Collaboration (GMPSRC), Faculty of Biology, Medicine and Health, Manchester Academic Health Sciences Centre (MAHSC), University of Manchester, Manchester, UK Jung Yin Tsang, Matthew Sperrin, Thomas Blakeman & Darren M. Ashcroft Division of Informatics, Imaging and Data Sciences, School of Health Sciences, University of Manchester, Manchester, UK Jung Yin Tsang & Matthew Sperrin Department of Health and Community Sciences, University of Exeter Medical School, Exeter, UK Rupert A. Payne Division of Pharmacy and Optometry, School of Health Sciences, University of Manchester, Manchester, UK Darren M. Ashcroft You can also search for this author in PubMed  Google Scholar ContributionsAll authors were involved in the conceptualisation and design of the study. JT wrote the first draft of the manuscript. All authors contributed to the review and approval of the final manuscript. Corresponding authorCorrespondence to Jung Yin Tsang . Ethics declarationsEthics approval and consent to participate. This study will be based on data from the CPRD (Clinical Practice Research Datalink) obtained under license from the UK Medicines and Healthcare products Regulatory Agency (MHRA). The data are provided by patients and collected by the National Health Service (NHS) as part of their care and support. The Office for National Statistics and Hospital Episode Statistics data are subject to Crown copyright (2023) protection and reused with the permission of the Health and Social Care Information Centre, all rights reserved. The Office of Population Censuses and Surveys (OPCS) Classification of Interventions and Procedures, codes, terms, and text is Crown copyright (2022) published by The Health and Social Care Information Centre, also known as NHS Digital and licensed under the Open Government License ( www.nationalarchives.gov.uk/doc/open-government-license/open-government-license.htm ). The interpretation and conclusions contained in this study are those of the authors alone, and not necessarily those of the MHRA, NIHR, NHS or the Department of Health and Social Care. The work detailed in this protocol has been approved by the CPRD Research Data Governance (RDG) Process (Ref: 22_002288; 09.02.2023). We would like to acknowledge all the data providers and general practices who make anonymised data available for research. Consent for publicationNot applicable. Competing interestsD. M. A. reports research funding from AbbVie, Almirall, Celgene, Eli Lilly, Novartis, UCB and the LEO Foundation outside the submitted work. The other authors declare that they have no competing interests. Additional informationPublisher's note. Springer Nature remains neutral with regard to jurisdictional claims in published maps and institutional affiliations. Rights and permissionsOpen Access This article is licensed under a Creative Commons Attribution 4.0 International License, which permits use, sharing, adaptation, distribution and reproduction in any medium or format, as long as you give appropriate credit to the original author(s) and the source, provide a link to the Creative Commons licence, and indicate if changes were made. The images or other third party material in this article are included in the article's Creative Commons licence, unless indicated otherwise in a credit line to the material. If material is not included in the article's Creative Commons licence and your intended use is not permitted by statutory regulation or exceeds the permitted use, you will need to obtain permission directly from the copyright holder. To view a copy of this licence, visit http://creativecommons.org/licenses/by/4.0/ . Reprints and permissions About this articleCite this article. Tsang, J.Y., Sperrin, M., Blakeman, T. et al. Protocol for the development and validation of a Polypharmacy Assessment Score. Diagn Progn Res 8 , 10 (2024). https://doi.org/10.1186/s41512-024-00171-7 Download citation Received : 15 November 2023 Accepted : 30 May 2024 Published : 16 July 2024 DOI : https://doi.org/10.1186/s41512-024-00171-7 Share this articleAnyone you share the following link with will be able to read this content: Sorry, a shareable link is not currently available for this article. Provided by the Springer Nature SharedIt content-sharing initiative - Prediction modelling
- Risk calculator
- Polypharmacy
- Multimorbidity
- Overtreatment
- Inappropriate prescribing
Diagnostic and Prognostic ResearchISSN: 2397-7523  Have a language expert improve your writingRun a free plagiarism check in 10 minutes, generate accurate citations for free. Methodology - Types of Research Designs Compared | Guide & Examples
Types of Research Designs Compared | Guide & ExamplesPublished on June 20, 2019 by Shona McCombes . Revised on June 22, 2023. When you start planning a research project, developing research questions and creating a research design , you will have to make various decisions about the type of research you want to do. There are many ways to categorize different types of research. The words you use to describe your research depend on your discipline and field. In general, though, the form your research design takes will be shaped by: - The type of knowledge you aim to produce
- The type of data you will collect and analyze
- The sampling methods , timescale and location of the research
This article takes a look at some common distinctions made between different types of research and outlines the key differences between them. Table of contentsTypes of research aims, types of research data, types of sampling, timescale, and location, other interesting articles. The first thing to consider is what kind of knowledge your research aims to contribute. Type of research | Whatâs the difference? | What to consider | Basic vs. applied | Basic research aims to , while applied research aims to . | Do you want to expand scientific understanding or solve a practical problem? | vs. | Exploratory research aims to , while explanatory research aims to . | How much is already known about your research problem? Are you conducting initial research on a newly-identified issue, or seeking precise conclusions about an established issue? | | aims to , while aims to . | Is there already some theory on your research problem that you can use to develop , or do you want to propose new theories based on your findings? | Prevent plagiarism. Run a free check.The next thing to consider is what type of data you will collect. Each kind of data is associated with a range of specific research methods and procedures. Type of research | Whatâs the difference? | What to consider | Primary research vs secondary research | Primary data is (e.g., through or ), while secondary data (e.g., in government or scientific publications). | How much data is already available on your topic? Do you want to collect original data or analyze existing data (e.g., through a )? | | , while . | Is your research more concerned with measuring something or interpreting something? You can also create a research design that has elements of both. | vs | Descriptive research gathers data , while experimental research . | Do you want to identify characteristics, patterns and or test causal relationships between ? | Finally, you have to consider three closely related questions: how will you select the subjects or participants of the research? When and how often will you collect data from your subjects? And where will the research take place? Keep in mind that the methods that you choose bring with them different risk factors and types of research bias . Biases aren’t completely avoidable, but can heavily impact the validity and reliability of your findings if left unchecked. Type of research | Whatâs the difference? | What to consider | | allows you to , while allows you to draw conclusions . | Do you want to produce knowledge that applies to many contexts or detailed knowledge about a specific context (e.g. in a )? | vs | Cross-sectional studies , while longitudinal studies . | Is your research question focused on understanding the current situation or tracking changes over time? | Field research vs laboratory research | Field research takes place in , while laboratory research takes place in . | Do you want to find out how something occurs in the real world or draw firm conclusions about cause and effect? Laboratory experiments have higher but lower . | Fixed design vs flexible design | In a fixed research design the subjects, timescale and location are begins, while in a flexible design these aspects may . | Do you want to test hypotheses and establish generalizable facts, or explore concepts and develop understanding? For measuring, testing and making generalizations, a fixed research design has higher . | Choosing between all these different research types is part of the process of creating your research design , which determines exactly how your research will be conducted. But the type of research is only the first step: next, you have to make more concrete decisions about your research methods and the details of the study. Read more about creating a research design If you want to know more about statistics , methodology , or research bias , make sure to check out some of our other articles with explanations and examples. - Normal distribution
- Degrees of freedom
- Null hypothesis
- Discourse analysis
- Control groups
- Mixed methods research
- Non-probability sampling
- Quantitative research
- Ecological validity
Research bias - Rosenthal effect
- Implicit bias
- Cognitive bias
- Selection bias
- Negativity bias
- Status quo bias
Cite this Scribbr articleIf you want to cite this source, you can copy and paste the citation or click the âCite this Scribbr articleâ button to automatically add the citation to our free Citation Generator. McCombes, S. (2023, June 22). Types of Research Designs Compared | Guide & Examples. Scribbr. Retrieved July 16, 2024, from https://www.scribbr.com/methodology/types-of-research/ Is this article helpful? Shona McCombesOther students also liked, what is a research design | types, guide & examples, qualitative vs. quantitative research | differences, examples & methods, what is a research methodology | steps & tips, what is your plagiarism score. - Open access
- Published: 15 July 2024
Assessing farmersâ willingness to pay for FMD vaccines and factors influencing payment: a contingent valuation study in central Oromia, Ethiopia- Misgana Lemi Layessa 1 ,
- Endrias Zewdu Gebremedhin 2 ,
- Edilu Jorga Sarba 2 &
- Wakuma Mitiku Bune 2 Â
BMC Veterinary Research volume  20 , Article number: 313 ( 2024 ) Cite this article 247 Accesses Metrics details Foot and mouth disease is a contagious, transboundary, and economically devastating viral disease of cloven-hoofed animals. The disease can cause many consequences, including decreased productivity, limited market access, and elimination of flocks or herds. This study aimed to assess farmersâ willingness to pay (WTP) for foot and mouth disease (FMD) vaccines and identify factors influencing their WTP. A cross-sectional questionnaire survey was conducted on 396 randomly selected livestock-owning farmers from three districts in the central Oromia region (Ambo, Dendi, and Holeta districts. The study utilized the contingent valuation method, specifically employing dichotomous choice bids with double bounds, to evaluate the willingness to pay (WTP) for the FMD vaccine. Mean WTP was assessed using interval regression, and influential factors were identified. The study revealed that the farmerâs mean willingness to pay for a hypothetical foot and mouth disease vaccine was 37.5 Ethiopian Birr (ETB) [95% confidence interval [CI]: 34.5 40.58] in all data, while it was 23.84 (95% CI: 21.47â26.28) in the mixed farming system and 64.87 Ethiopian Birr (95% CI: 58.68 71.15) in the market-oriented farming system. We identified main livelihood, management system, sales income, breed, keeping animals for profit, and foot and mouth disease impact perception score as significant variables ( p ââ€â0.05) determining the farmersâ WTP for the FMD vaccine. Farmers demonstrated a high computed willingness to pay, which can be considered an advantage in the foot and mouth disease vaccination program in central Oromia. Therefore, it is necessary to ensure sufficient vaccine supply services to meet the high demand revealed. Peer Review reports Foot and mouth disease (FMD) is a contagious, transboundary, and economically devastating viral disease of cloven-hoofed animals, including domestic and wildlife species. The disease is caused by foot and mouth disease virus (FMDV), which belongs to the genus Aphtovirus and the family Picornaviridae. The virus comprises seven serotypes (A, O, C, Asia1, SAT1, SAT2, and SAT3) with numerous subtypes. Ethiopia has endemic serotypes of Foot-and-Mouth Disease (FMD), including serotypes O, A, SAT 1, and SAT 2, among the seven known serotypes. Clinically, FMD manifests as vesicular eruptions in the oral cavity, foot, and udder, accompanied by symptoms such as fever, lameness, salivation, and anorexia [ 1 ]. Foot and mouth disease is the most critical global livestock disease regarding its economic impact. In endemic areas, the economic effects of FMD can be separated into direct and indirect losses, and the annual economic impact ranges from USD 6.5 to 21 billion, covering noticeable production losses and immunization expenses. In contrast, outbreaks in FMD-free countries and zones cost USD 1.5 billion each year [ 2 ]. In the crop-livestock mixed farming system of Ethiopia, the economic losses from FMD outbreaks attributable to the disruption of milk production, the loss of draft power, and increased mortality among infected cattle herds were, on average, USD 76. At the same time, it was USD 9.8 per affected head of cattle. In the pastoral system, economic losses of USD 174 and 5.3 per involved herd and head of cattle in the infected herds were estimated. In pastoral systems, the costs associated with these losses are exceptionally high because cattle serve as a crucial source of income and livelihood [ 3 ]. Two major approaches are used to control FMD worldwide: vaccination with or without stamping out, as in continental Europe and parts of South America, and intensive surveillance and eradication, as in the case of North America, Scandinavia, and the United Kingdom. In disease-free developed countries, the stamping-out approach is employed to control the incursion of outbreaks. This method involves swiftly detecting the introduction of the disease and culling both infected and in-contact herds to prevent its spread [ 4 ]. In Ethiopia, various control methods are employed to manage Foot-and-Mouth Disease (FMD), including the implementation of biosecurity measures. These measures encompass conducting vaccination campaigns, implementing disinfection protocols, ensuring safe disposal of carcasses, raising public awareness and education about FMD, and administering antibiotics to combat secondary bacterial complications [ 5 ]. Regular mass vaccinations are commonly used in developing countries to control FMD in endemic regions. However, the use of vaccines that cover multiple serotypes and strains presents challenges due to reduced potency and higher costs compared to single-serotype vaccines [ 4 ]. Achieving effective cross-protection among the various serotypes and strains is also difficult [ 5 ]. In Ethiopia, FMD outbreaks are widespread across all production systems, particularly in mixed crop-livestock, pastoral, and market-oriented districts. The predominant serotypes causing outbreaks in the country are A, O, SAT 1, and SAT 2 [ 6 , 7 ]. Serological studies reveal varying levels of seroprevalence (3.4â72.1%), indicating limitations in the effectiveness of the control program, including planned vaccination, high susceptibility of animals, and inadequate biosecurity measures [ 8 , 9 ]. The National Veterinary Institute (NVI) in Ethiopia manufactures a trivalent Foot-and-Mouth Disease (FMD) vaccine containing inactivated serotypes O, A, and SAT 2 strains, providing six months of immunity. Although the vaccine has an effectiveness falling below the globally indicated 75%, it is recommended for biannual government-administered vaccination [ 10 ]. Its relatively high cost (15 ETB/dose) compared to other livestock vaccines discourages small-scale traditional farmers from using it, leading to low vaccination rates and ongoing outbreaks in villages. Despite falling below the global standard, the existing FMD vaccine still holds value in reducing the incidence and severity of clinical disease in vaccinated cattle [ 10 ]. Researchers can use contingent valuation to determine the WTP for public goods, such as FMD vaccines. This could be for a vaccine under development and yet not marketed [ 6 ] or for existing vaccines that are poorly adapted for a variety of reasons, including price sensitivity [ 7 , 8 ]. Foot-and-mouth disease outbreaks have resulted in significant financial losses for countries, amounting to millions of USD, due to the restrictions or rejection of livestock products. Despite the importance of vaccination in preventing FMD outbreaks and its economic impact, there is a lack of comprehensive understanding regarding farmersâ willingness to invest in FMD vaccines. This study aimed to assess farmersâ WTP for FMD vaccines and identify factors influencing their WTP. Study area and populationThe study was conducted in three districts in the central part of the Oromia region of Ethiopia, namely, the Ambo, Dendi, and Holeta districts (Fig. 1 ), where the mixed crop-livestock farming system is the basis for the communityâs livelihood. There are two main types of production systems practiced in the region: the dominant mixed crop-livestock (MF) production system, which is a subsistence system practiced in rural areas, and a market-oriented (MO) production system, which produces commercial milk in urban and peri-urban areas. The livestock subsector plays an essential role in the livelihood of these areas by providing alternative income sources as a strategy to build emergence to stress and contribute to their food security. Both local and crossbred cattle are raised in the areas. 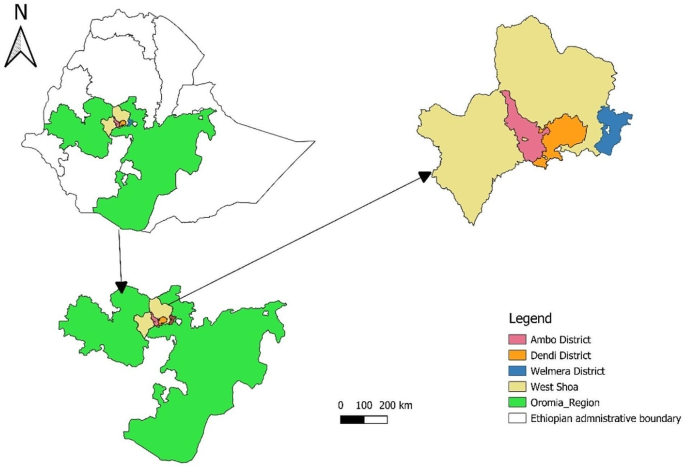 A map showing the study districts in central Oromia, Ethiopia. (Source: QGIS, Version 3.26) We conducted the study on the human population residing in the three selected districts. The study subjects were households in the sedentary farming system engaged in livestock rearing as their primary livelihood or occupation. Study designA cross-sectional study was conducted from January to June 2022 to assess farmersâ WTP for the FMD vaccine. Sampling techniqueThree districts (Ambo, Dendi, and Welmera) and their towns in the West Shoa zone of central Ethiopia were purposefully picked at the outset to ensure the feasibility of the study and accommodate the study area and period. We made this decision based on the observations made among these three districts. Subsequently, we considered six villages from each district, resulting in eighteen villages. We employed a purposive sampling technique for this investigation but did not conduct a formal sample size calculation. This omission was due to the lack of prior knowledge about the joint distribution of the dependent and independent variables [ 9 ]. Since this information was not available before the survey, it was not possible to implement a formal sample size estimate. We randomly selected farmers from urban streets and rural villages within the designated study areas. We randomly selected 22 households from each of the eighteen chosen villages, utilizing household lists provided by development agents and health extension workers in those villages. As a result, a systematic random sampling method was employed to select households and study 396 participants. Contingent valuation method (CVM)Despite having a price, animal disease vaccines offer public advantages by controlling disease transmission within the community, thereby benefiting the well-being of all animals. Hence, contingent valuation is used to determine the WTP for the FMD vaccine [ 6 ]. A survey was designed to produce hypothetical market scenarios that would indicate the value of FMD vaccination using the CVM. Then, the survey respondents were requested to respond to these hypothetical market scenarios. The questionnaire surveyThe survey questions (32 closed-ended) were initially prepared in English, later translated into Afaan Oromo (the language used in the study area), and personally administered to the selected households by the researchers. A pilot survey was carried out in December 2021 in the community before the actual study to assess the instrumentsâ suitability and feasibility and to determine respondentsâ views of possible vaccine prices to pay. For the pilot survey, 60 individuals (30 from mixed farming and 30 from market-oriented livestock-rearing) were randomly selected from the village using field surveying by asking every person who walks a fixed point along a busy pathway to obtain the first sets of bid prices [ 10 ]. In addition, researchers selected WTP sets of bids based on likely vaccine prices available in the market, considering the surveying of bid values in the pilot study. This set of bids obtained from open-ended questionnaire responses was collected as a reference baseline but was not used in the primary analyses. The researchers consulted the National Veterinary Institute (Bishoftu, Ethiopia) to assess the countryâs capacity for producing the vaccines included for FMD control. We conducted the final survey from January to June 2022. The respondents who agreed to participate in the study were adults at least 18 years old, heads of household, and currently owned livestock. The face-to-face survey method was designed to measure potential factors of acceptance of FMD vaccines and WTP for vaccination services. The survey took approximately 30 min for each participant, and households were advised on the animal health and production extension services upon completion of the study to compensate for their time. The double-bounded dichotomous choice method via closed-ended questionnaire format was used in which the respondents were asked a sequence of two questions about their WTP for FMD vaccine at a specific price so that they used to give a âyesâ or ânoâ response to a single bid question. To enhance the reliability of the contingent valuation questionnaire, researchers included a âdo not knowâ response option and a âYes/Noâ response option. To implement this contingent valuation survey effectively, researchers ensured a clear definition of nonmarketed goods (FMD vaccine) and established a credible means of vaccination service provision. They also developed a possible mechanism that facilitated the exchange between the uses of the FMD vaccine and the price of interest. For this purpose, a double-bounded dichotomous questionnaire format was adapted from a previous study in Ethiopia [ 1 ]. In this survey of contingent evaluation elicitation questions, researchers utilized the recommended guidelines prepared by the National Oceanic and Atmospheric Administration (NOAA), which consist of two components [ 11 ]. These guidelines are general in nature and applicable, particularly in developing countries, which depend mainly on the socioeconomic and institutional aspects existing in the study areas [ 12 ]. Following the guidelines outlined, the questionnaire was divided into two components. The questionnaire consisted of two components. The first component included bidding questions directly linked to the WTP for a hypothetical FMD vaccine market price. The second component comprised questions exploring the socioeconomic factors that could impact the farmerâs willingness to pay for the FMD vaccine. Before the two components of the survey, there was a justifying question of whether the respondents knew the disease, which contained a statement of approval for the respondents and some triggering questions to obtain information regarding the respondentâs knowledge about animal health services in addition to FMD disease. Consequently, this double dichotomous choice bidding format consisted of two stages, where respondents were presented with bid amounts and had the option to indicate their WTP as either âyesâ or ânoâ in response to the proposed hypothetical vaccine prices. The initial bid amounts were distributed uniformly and randomly among respondents who were exposed to the questionnaire. Depending on the respondentsâ answer to the initial bid question, the amount of the follow-up bid was increased (premium bid) or decreased (discounted offer) accordingly by a set amount randomized and asked by the respondents, which means that if the response to the initial bid amount was âyes,â the follow-up bid amount would increase by 50%, and if the answer to the initial bid amount was âno,â the follow-up bid amount would decrease by 50% (Table 1 ). In the first and follow-up stage questions, we included an âUndeterminedâ alternative for respondents who could not decide between âYesâ or âNo.â The initial bid set prices proposed in the first component of this survey contained 20, 40, 60, and 80 Ethiopian Birr (ETB) per dose (1 ETBâ=â0.0203 USD at the time of the study) (Table 1 ). Researchers proposed this initial bid price set based on information from an open-ended WTP pilot survey with a price range of 12â100 ETB/annual dose for the same hypothetical vaccine [ 1 ]. This price range was nearly comparable to USD 0.4â3 (ETB 12â88) for diverse types of FMD vaccines reported in the literature in other countries [ 2 , 13 ]. Currently, the Ethiopian government is providing FMD vaccines at a subsidized price of 15 ETB/dose for farmers. The second component of the questionnaire contained the sociodemographic features and management systems (independent variables), which could affect respondentsâ WTP (dependent variable) for a hypothetical FMD vaccine. The key demographic and animal husbandry system variables included were district, sex, age, educational status, household size, main livelihood, tropical livestock unit (TLU) owned, direct livestock income (which is the sum of milk sale and live animal sale), management system, breed, animal isolated for profit, adoption of veterinary service (treatment, vaccination, etc.). FMD impact perception score (perception about FMD impact on livestock), and vaccine knowledge score (knowledge on the use of the vaccine for livestock disease prevention). The present study did not consider all animal-related benefits, such as apiculture, aquaculture, animal byproducts, equines, and pet benefits. Furthermore, we did not consider traits of the vaccine, as they could not be studied using the CVM. Instead, another valuation method called the choice experiment method was employed. We measured the perception of FMD impact and knowledge of vaccines as combined scores of corresponding questions under the two variables. Therefore, we derived the FMD impact perception score from five questions, each with three possible scores, resulting in 15 FMD impact perception scores. Likewise, the knowledge score about livestock vaccines was derived from four vaccine knowledge questions, where a correct response received a score of one and an incorrect response received a score of zero, resulting in a livestock vaccine knowledge score of four [ 1 ]. Data analysisThe data were imported into Microsoft Excel 2007 and manually verified to ensure accuracy. This involved systematically checking for missing values and outliers. All data analyses were performed using STATA software version 14 (Stata Corp. College Station, TX). Descriptive statistics were employed to analyze and summarize the data using various variables. In the interval regression model, the variables considered for the models were checked first for multicollinearity using the variance inflation factor (VIF). We considered a variance inflation factor (VIF) value above ten to indicate collinearity among variables. Consequently, variables such as breed ( r =-0.72) and management system ( r =-0.75) were rejected from the model, and the full models containing all noncollinear variables passed the final analysis, which contained MO and MF together. The model was also developed separately for MO and MF production systems, and the same procedure was followed. The final models were reached by excluding nonsignificant variables with a p-valueâ>â0.05 one at a time until only significant variables were left [ 14 ]. The responses to the double-bounded contingent valuation (CV) questions give four possible discrete outcomes: (1) the household was not willing to pay for FMD vaccines even at the discounted price (âno,â ânoâ) to both bids; (2) the household was not willing to pay for FMD vaccines at the initial price but was willing to buy at the discounted price (âno,â âyesâ); (3) the household was willing to pay for FMD vaccines at the initial price but not the increased, premium price (âyes,â ânoâ); or (4) the household was willing to pay for FMD vaccines at both the initial price and the premium price (âyes,â âyesâ). This double-bounded model allows us to place the householdâs WTP into one of four intervals: (0, Bl), (Bl, Bi), (Bi, Bh), and (Bh, +). where Bi is the initial bid amount, Bl is the lower follow-up bid amount, and Bh is the higher follow-up bid amount. We employed interval regression analysis [ 15 ] to estimate the farmersâ WTP for the FMD vaccine using the double-bounded dichotomous contingent valuation data collected through the questionnaire. This interval regression model gives three types of censoring (left censored, right censored, and interval censored) where the WTP values lie down. After analyzing all interval data, we expressed the values of the STATA output in terms of mean, standard deviation, standard error, percentiles, model coefficients, 95% confidence interval (CI) of coefficients, and P value. Farmersâ WTP for the FMD vaccine was estimated using the interval data estimation method under the assumption that WTP can be modeled as a linear function of the characteristics of respondents along with a standard error [ 16 ]. Respondent characteristicsAmong the 396 farmers surveyed for the dichotomous choice bidding questions, ten answered âDo not knowâ either to both bids or one of the bid questions. Two outliers were found due to their high income. These 12 observations were excluded from the analysis, which gave us 384 cleaned observations from the three districts. Hence, the questionnaire was administered to 396 households surveyed, which was reduced to 384 observations. The average age of the respondents was approximately 43 years old (43.7 for MF and 44.15 for MO), and most were males (94%), with an average household member of 4.7. Almost all respondents mentioned that they know about FMD (locally known as â Kebena , â which means feeling cold ). Approximately 94% of respondents in the market-oriented system kept cattle for profit (livestock products and live animal sales), but only approximately 19% of mixed farming respondents kept cattle for profit. Approximately 94% of the respondents believed that vaccines could prevent livestock diseases, 62% used modern veterinary services, and all had experience using other types of vaccines in their cattle husbandry. Table 2 presents the sociodemographic and cattle husbandry characteristics of the respondents. We used tropical livestock units (TLU) to calculate the total number of different species of livestock kept by respondents. As a result, the average TLU was approximately 12.48 for male farmers (MF) and 9.2 for female farmers (MO) respondents. The average income of respondents from livestock sales in MF and MO was approximately 10,737 ETB and 266,707 ETB, respectively. The mean overall FMD impact perception score out of 15 points was 13.11 for MF and 13.58 for MO system respondents. Similarly, the mean vaccine knowledge score was 2.52 for MF and 3.02 for MO out of 4 points. The respondentsâ WTP for the hypothetical FMD vaccine declined regarding the increase in the bidding amount offered to them, as indicated in Fig. 2 . At the same time, the WTP increased as the bidding amounts decreased. 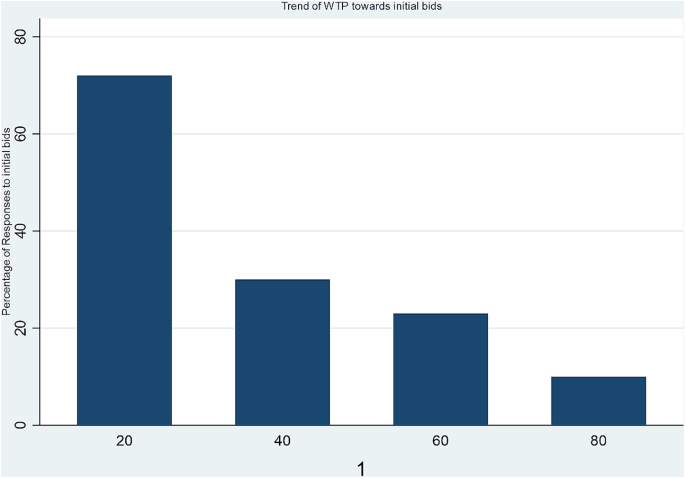 Graph showing the WTP trend regarding the initial bid amount offered to respondents. The â0â and â1â under the graph represent âNoâ and âYesâ responses toward the initial bids provided to respondents, respectively. The respondentsâ WTP (yes response) decreased with the increased bidding amount Among the all-initial bids offered for all respondents, 35.20% received âYesâ responses, and 64.8% received âNoâ answers. The follow-up bids received more âYesâ reactions when the bidding amount was lower. In separate bids, the overall percentage of obedience (yes response) for initial bids of 20 ETB, 40 ETB, 60 ETB, and 80 ETB was 74%, 31%, 24%, and 11%, respectively (Table 3 ). The percentages of WTP (âyesâ responses) in MF respondents for the proposed bids of 20 ETB, 40 ETB, 60 ETB, and 80 ETB were 61%, 9.2%, 4.8%, and 3.7%, respectively (Table 4 ). The percentages of WTP (âyesâ responses) in market-oriented respondents (MO) for the initial bids of 20, 40, 60, and 80 ETBs were 94.7%, 75%, 60.6%, and 20%, respectively (Table 5 ). WTP for FMD vaccine and significant variablesWe obtained the mean estimates of WTP using the interval data method, which distributed the latent WTP between the two bounds (lower and upper bounds). The estimates are directly observed from the model and not derived from other parameters. This is because the estimation procedure effectively involves an interval regression with only an intercept. According to the interval regression analysis, the average WTP per dose, as determined by the constants of the null models (models without any explanatory variables), was estimated at 37.51 ETB (95% CI: 34.53â40.58%) for all respondents. For MF respondents, the average WTP was 23.84 ETB (95% CI: 21.47â26.28), while for MO respondents, it was 64.87 ETB (95% CI: 58.68â71.15). We obtained these WTP estimates from the model without considering other variables that could affect the WTP for the vaccine. The forecasts from the model are presented in Table 6 . The interval regression model assesses the factors influencing respondentsâ WTP for the FMD vaccine. An assessment for multicollinearity using the variance inflation factor (VIF) showed that all the variables had a VIF of less than 10 with a mean VIF of 1.86; hence, except for the production system (collinear with main livelihood), all the variables are not collinear to each other. The variables significantly associated with WTP were the main livelihood, keeping animals for profit, and FMD impact of perception score. The variable main livelihood of the farmers significantly negatively influences the farmersâ WTP for vaccination. Age and sex were not significant and were not included in the multivariable model. The remaining variables, the management system (excluded due to collinearity) and adoption of veterinary service, district, education level, and TLU, were found insignificant in the multivariable analysis ( p â>â0.05) in determining farmersâ WTP for the FMD vaccine. The MF and other respondents were less likely (-26.0 less) to pay for immunization than their MO counterparts. Our model also showed that animals isolated for-profit and FMD impact perception scores had a significant effect on WTP. Accordingly, when the FMD impact perception score increases by one unit, the WTP increases by 1.40 ETB, keeping other variables constant in the interval regression model. Similarly, participants who keep cattle for profit purposes had 16.00 more ETB WTP compared to their counterparts, keeping other variables constant in the interval regression model (Table 7 ). Due to their socioeconomic and husbandry differences, we conducted separate interval regressions for the two production systems (MF and MO). Accordingly, there was a significant difference in WTP for the FMD vaccine, which warranted separate analyses. The factors significantly associated with the WTP of the FMD vaccine in MF were sales income from the animals and the management system. Accordingly, the WTP increased by 0.002 points for each one-unit increase in sales income, whereas farmers handling their cattle under an intensive management system had 6.8 ETB more WTP than those in an extensive system. On the other hand, the factor significantly associated with the WTP of the FMD vaccine in MO was the types of cattle breeds kept. As a result, approximately 13.26 ETB increases were observed in farmers handling exotic breeds compared to those handling local cattle and their crosses (Table 8 ). The study found an overall average willingness to pay of 37.5 ETB for a hypothetical FMD vaccine among farmers. The WTP was 23.84 ETB in the mixed farming system and 64.87 ETB in the market-oriented farming system. In Ethiopia, the utilization of FMD vaccines in all production systems has been limited, primarily because of infrequent vaccination and insufficient awareness regarding the circulating serotypes prior to vaccination. Challenges also arise from the reluctance of livestock producers to vaccinate or bear the cost of vaccination. Jemberu et al. [ 8 ] identified farmersâ perceived cost of vaccination (perceived barrier) as the most crucial perception that significantly influenced the intention to implement vaccination with payment [ 17 ]. The higher average TLU of 12.48 in the MF system, compared to an average TLU of 9.2 in the MO system, could be attributed to the inclusion of other livestock, such as sheep, goats, oxen, and equines within the MF system. In contrast, the MO system primarily focuses on maintaining fewer productive cows for milk production. The study discovered that the estimated WTP for the proposed vaccine price (meanâ=âETB 37.51) exceeded that of the currently available government-produced FMD vaccine in Ethiopia (NVI). This finding was unexpected, as most vaccines, including those for transboundary animal diseases, are typically available at lower prices or free in the study area. The percentage of farmers willing to pay decreased gradually from 74 to 11% as the opening bid values improved from ETB 20 to 80, aligning with the economic principles of market behavior [ 18 ]. The obedience rate (percentage of âyesâ responses) among all respondents was 74% for an initial bid of 20 ETB, 31% for 40 ETB, 24% for 60 ETB, and 11% for 80 ETB. This indicates that the majority of respondents (74%) are willing or inclined to pay the initial bid of 20 ETB, aligning with the typical demand behavior observed in various commodities when prices fluctuate [ 19 ]. The estimated mean WTP for the FMD vaccine computed by the interval regression model from the whole data was ETB 37.51 (95% CI: 34.53â40.58) per year, and this revealed a narrow confidence interval of WTP, which indicates a high level of certainty and shows consistency of this study. This is lower than previous studies on farmersâ WTP for the FMD vaccine in the Amhara region, which was computed as ETB 58.23/dose [ 1 ]. The WTP for the FMD vaccine can vary among regions based on various factors. The possible reasons for such a scenario might be the increased risk of FMD, access to information and awareness, previous experience with the vaccineâs effectiveness, income levels, market opportunities, and access to veterinary services in the Amhara region compared to the current study area. Nevertheless, further research is needed on this issue. Most farmers explained that the stated vaccine price was much lower when compared to the loss of milk production due to FMD. The estimated WTP in MO farming was 64.88 ETB/dose and 23.83 ETB/dose in MF systems, which is relatively high in MO farming. The expected difference in WTP within the MO system was attributed to the comparatively higher income of respondents in this group. However, the calculated WTP in this study significantly surpasses the current price of 15 ETB/dose for the trivalent (O, A, SAT2) vaccine manufactured by the NVI and subsidized by the government. This is consistent with a contingent valuation study for Ethiopiaâs Gumboro and Newcastle disease vaccine programs, which disclosed that farmers recognized the value of vaccine programs and were willing to pay for them [ 20 ]. When using the estimated WTP for practical applications, it is crucial to consider any possible biases related to it. Willingness to pay estimations frequently shows a tendency to overestimate actual market behavior, according to numerous studies on contingent evaluation [ 21 , 22 ]. Therefore, it is essential to exercise caution when applying the projected WTP results to real-world situations. Studies across different countries revealed an estimated WTP between 0.4 and 3 USD per dose in addition to the vaccine delivery service fee [ 2 ]. In Tanzania, a nearly comparable WTP amount, i.e., 1.84 USD, was reported for the cattle FMD vaccine [ 23 ]. Kairu-wanyoike et al. [ 5 ] estimated a mean WTP of 3.03 USD for the contagious bovine pleuropneumonia vaccination in the Narok south district of Kenya. The survey found that approximately 35% of respondents were keen to contribute to FMD control efforts based on the initial bid alone. On the other hand, those unwilling to pay the initial bids constituted a higher percentage (65%) for various reasons, mainly increasing the vaccine price offered. These findings differ significantly from the findings of [ 20 ], who found that 57% of the respondents were willing to pay for vaccination against FMD. None of the sociodemographic variables measured, such as sex, age, education status, and TLU, significantly impacted WTP. Hence, it appears that the main driver for vaccine awareness and WTP is not related to sex, age, TLU, or the education status of the farmer, which is unexpected. Access to livestock extension and benefits-related issues from livestock might play a more significant role in a better WTP [ 24 ]. On the other hand, we found that livestock husbandry-related variables such as main livelihood (whether farmers practice MO or MF), keeping animals for profit, and FMD impact perception score served as essential drivers of WTP for the FMD vaccine. The variable main livelihood had a high effect on the mean WTP, indicating that MO respondents are more likely willing to pay. To describe more, the interval regression model computed that the MO respondents had significantly higher WTP than the MF respondents. This was in line with the findings of other studies, as MO farmers often predominantly generate money, as reported in a previous survey [ 1 ]. In addition, this finding is in accord with the results of other studies, as those with relatively high incomes often matter for the healthcare expenditure of the household [ 19 ]. Hence, the model coefficient at the mean for the main livelihood denoted that the probability that farmers would be willing to pay in MO is 26.0 times higher than that for MF farmers. The difference between the MO and MF systems can be attributed to the income disparity, with the former being wealthier. The farmers practicing the MO cattle farming system predominantly keep exotic cattle breeds or their crosses that are primarily managed under an intensive system, which means they are more affluent and are able to pay for vaccination to prevent any possible loss from FMD diseases, which might affect their income. This is associated with the intention of farmers to rear animals, which is directly related to several benefits gained. Moreover, MO farmers whose livelihood relies on dairy production would keep more exotic crossbred cattle than local breeds with higher WTP for FMD. Knight-Jones and Rushton, in their studies of the economic impact of FMD, found that the health expenditure for livestock is directly related to income from livestock rearing [ 2 ]. Furthermore, farmers who kept animals for profit were more willing to pay (16.0 times) for vaccination than their counterparts who simply rear animals extensively. Those farmers who deliberately raise animals for the sale of animal or animal products such as milk tend to view animal production as a business in which they are eager to invest to mitigate risk and increase the chances of acquiring profits. In their study of FMD outbreak investigation and economic impact assessment in Ethiopia, [ 25 ] found that vaccinating animals decreased production losses due to the outbreak and increased animal production and productivity. As expected, the FMD impact perception score positively influenced farmersâ WTP and was statistically significant at the 4% level. The higher the impact perception score is, the more willing farmers are to contribute to FMD control. Therefore, for every one-unit increase in the farmerâs FMD impact perception score, the WTP for vaccination increased by a factor of 1.40. In line with this, [ 6 ] justified that farmers with high-risk perceptions of bovine tuberculosis have a positive attitude toward WTP for its vaccination. It is psychologically rational that farmers with a good perception of disease impact are willing to pay more to prevent disease. However, there was evidence that prior awareness of or having personal knowledge of the disease did not always lead to higher WTP [ 26 ]. A separate analysis of MO and MF data to determine drivers of WTP resulted in sales income as a significant variable in the MF system. Sales income affected farmersâ WTP (0.002 times), which is consistent with the theoretical concept of positive income elasticity in that wealthier families purchase more commodities than low-income households [ 27 ]. This could be essential for policy implications in Ethiopia, where low-income households often cannot afford proper healthcare for their families and livestock healthcare. Similarly, owning an exotic type of breed showed a significant association with the farmersâ WTP for FMD vaccination in MO respondents. Respondents who have exotic breeds showed 13.26 times more WTP than those who rear locals and their crosses. This finding closely agrees with the study of WTP for the FMD vaccine reported in northern Ethiopia by [ 1 ], which stated that respondents who owned exotic breed cattle and their crosses exhibited a higher WTP compared to those who only owned local cattle breeds. Exotic breeds are valuable investments, motivating farmers to protect them through vaccination. Exotic breeds are more vulnerable to FMD, leading to a greater recognition of the risk and potential economic losses. Those MO farmers often have better financial resources and an understanding of vaccination benefits, allowing them to allocate more funds for disease prevention. In general, WTP for vaccinations was price-sensitive, as expected. As the offered cost per vaccination increased, the expected WTP decreased from 74 to 11%, which may result in insufficient coverage for cost-effective control. However, we also noticed that wealthier households demanded FMD vaccines even at higher prices. Indeed, the vaccination of more affluent populations would benefit poor people due to herd immunity [ 28 ]. Thus, for the full coverage of FMD vaccination in the country, a special fund could be additionally used to subsidize households with lower WTP and support families with lower WTP and those unable to pay. We sampled households in accessible areas, specifically in central Oromia. As a result, we did not implement strict randomization when selecting districts and villages due to the lack of accessibility to certain rural villages in the study areas. Therefore, our survey sample and results might not reflect all relevant drivers of WTP for livestock farmers throughout the country. Moreover, in applying CVM, a possible source of bias might arise because respondents are not purchasing the vaccine in the practical context as in the hypothetical price [ 22 ]. Vaccine traits, a broad concept, were not studied in this choice experiment method. Furthermore, we did not consider herd immunity in the contingent valuation scenario, so our results underestimate the actual value of this particular vaccine. In addition, data of zero protests or those unwilling to pay were not considered in the study, as it was not our objective. Therefore, the above limitations signify possible clues for further research on the above gap. Nevertheless, despite these limitations, the current study tried to identify important factors affecting farmersâ WTP for FMD vaccines. ConclusionsThe present survey of farmersâ WTP indicated that the mean WTP of respondents was high in both the MF and MO systems, even higher than the current market price in the country. Respondents in the MO system showed higher WTP than those in the MF system. The main livelihood, type of breed, sales income, the animal kept for profit, FMD, impact perception score, and the management system are factors affecting the WTP. The findings of this study show that effective control of FMD at the village level requires coordinated action between the state and the community. Addressing the demand for vaccines, strengthening animal health extension services through educating farmers about the effects of the disease and the importance of vaccines, promoting farming as a business, and further research on WTP and vaccine acceptance were suggested. Data availabilitySupplimentary material 1 (questionnaire) is uploaded. AbbreviationsConfidence Interval Contingent Valuation Method Ethiopian Birr Foot and Mouth Disease Foot and Mouth Disease Virus Mixed Crop Livestock Farming Market Oriented National Veterinary Institute South African Territory Tropical Livestock Units United States Dollar Variance Inflation Factor Willingness to Pay Jemberu WT, Molla W, Dagnew T, Rushton J, Hogeveen H. Farmersâ willingness to pay for foot and mouth disease vaccine in different cattle production systems in Amhara region of Ethiopia. PLoS ONE. 2020;15:1â12. Article Google Scholar Knight-Jones TJD, Rushton J. The economic impacts of foot and mouth disease - what are they, how big are they, and where do they occur? Prev Vet Med. 2013;112:161â73. Article CAS PubMed PubMed Central Google Scholar Jemberu WT, Mourits MCM, Woldehanna T, Hogeveen H. Economic impact of foot and mouth disease outbreaks on smallholder farmers in Ethiopia. Prev Vet Med. 2014;116:26â36. Article CAS PubMed Google Scholar Sutmoller P, Barteling SS, Olascoaga RC, Sumption KJ. Control and eradication of foot-and-mouth disease. Virus Res. 2003;91:101â44. Zewdie G, Akalu M, Tolossa W, Belay H, Deresse G. A review of foot-and-mouth disease in Ethiopia: epidemiological aspects, economic implications, and control strategies. Virol J. 2023;20:1â24. https://doi.org/10.1186/s12985-023-02263-0 . Bennett R, Balcombe K. Farmersâ willingness to pay for a tuberculosis cattle vaccine. J Agric Econ. 2012;63:408â24. Kairu-Wanyoike SW, Kaitibie S, Heffernan C, Taylor NM, Gitau GK, Kiara H, et al. Willingness to pay for contagious bovine pleuropneumonia vaccination in Narok South District of Kenya. Prev Vet Med. 2014;115:130â42. Article PubMed PubMed Central Google Scholar Campbell ZA, Otieno L, Shirima GM, Marsh TL, Palmer GH. Drivers of vaccination preferences to protect a low-value livestock resource: willingness to pay for Newcastle disease vaccines by smallholder households. Vaccine. 2019;37:11â8. Hsieh FY, Bloch DA, Larsen MD. A simple method of sample size calculation for linear and logistic regression. Stat Med. 1998;17:1623â34. 3.0.CO;2-S" data-track-item_id="10.1002/(SICI)1097-0258(19980730)17:14 3.0.CO;2-S" data-track-value="article reference" data-track-action="article reference" href="https://doi.org/10.1002%2F%28SICI%291097-0258%2819980730%2917%3A14%3C1623%3A%3AAID-SIM871%3E3.0.CO%3B2-S" aria-label="Article reference 9" data-doi="10.1002/(SICI)1097-0258(19980730)17:14 3.0.CO;2-S">Article CAS PubMed Google Scholar Pearce D, Ăzdemiroǧlu E. Economic valuation with stated preference techniques: Summary guide. Department for Transport, Local Government and the Regions; 2002. Randall A. The NOAA Panel Report: a new beginning or the end of an era? Am J Agric Econ. 1997;79:1489â94. Venkatachalam L. The contingent valuation method: a review. Environ Impact Assess Rev. 2004;24:89â124. Truong DB, Goutard FL, Bertagnoli S, Delabouglise A, Grosbois V, Peyre M. Benefit-cost analysis of foot-and-mouth disease vaccination at the farm-level in South Vietnam. Front Vet Sci. 2018;5:1â11. Jemberu WT, Molla W, Fentie T. A randomized controlled field trial assessing foot and mouth disease vaccine effectiveness in Gondar Zuria district, Northwest Ethiopia. Prev Vet Med. 2020;183 December 2019:105136. Alberini A. Testing willingness-to-pay models of Discrete Choice Contingent Valuation Survey Data. Land Econ. 1995;71:83. Alejandro L. Munich Personal RePEc Archive Introduction to contingent valuation using Stata Introduction to Contingent Valuation using Stata â . MPRA Paper. 2012;:1â16. https://mpra.ub.uni-muenchen.de/id/eprint/41018 . Accessed 3 Apr 2023. Jemberu WT, Mourits MCM, Hogeveen H. Farmersâ intentions to implement foot and mouth disease control measures in Ethiopia. PLoS ONE. 2015;10:1â15. Mitchell RC, Carson T. R. Using surveys to Value Public Goods: the contingent valuation method. Rff; 2013. Mankiw NG. Principles of microeconomics. 7th Editio. 2009. Terfa ZG, Garikipati S, Dessie T, Lynch S, Wigley P, Bettridge JM, et al. Farmersâ willingness to pay for a village poultry vaccine service in Ethiopia: prospect for enhancing rural livelihoods. Food Secur. 2015;7:905â17. Murphy JJ, Allen PG, Stevens TH, Weatherhead D. A meta-analysis of hypothetical bias in stated preference valuation. Environ Resour Econ. 2005;30:313â25. Ryan M, Mentzakis E, Jareinpituk S, Cairns J. External validity of contingent valuation: comparing hypothetical and actual payments. Heal Econ (United Kingdom). 2017;26:1467â73. Google Scholar Railey AF, Lembo T, Palmer GH, Shirima GM, Marsh TL. Spatial and temporal risk as drivers for adoption of foot and mouth disease vaccination. Vaccine. 2018;36:5077â83. Gizaw S, Woldehanna M, Anteneh H, Ayledo G, Awol F, Gebreyohannes G, et al. Animal Health Service Delivery in Crop-Livestock and Pastoral systems in Ethiopia. Front Vet Sci. 2021;8:601878. Tadesse B, Tesfahun A, Molla W, Demisse E, Jemberu WT. Foot and mouth disease outbreak investigation and estimation of its economic impact in selected districts in northwest Ethiopia. Vet Med Sci. 2020;6:122â32. Article PubMed Google Scholar Hou Z, Chang J, Yue D, Fang H, Meng Q, Zhang Y. Determinants of willingness to pay for self-paid vaccines in China. Vaccine. 2014;32:4471â7. Kariyasa K, Dewi YA. Convergence of market concentration: evidence from Czech food processing sectors. J Gend Agric Food Secur. 2011;1:1â22. FAO. the Global Foot and Mouth Disease. In: The Global Foot and Mouth Disease Control Strategy. 2018. p. 2. http://www.fao.org/3/I9857EN/i9857en.PDF . Download references AcknowledgementsThe authors thank the dairy farm owners who participated in the questionnaire survey and generously dedicated their time to providing the requested information. The research has not received any funding. Author informationAuthors and affiliations. West Shewa Zone, Ambo District Veterinary Clinic Office, Ambo, Ethiopia Misgana Lemi Layessa School of Veterinary Medicine, Ambo University, P.O. B 19, Ambo, Ethiopia Endrias Zewdu Gebremedhin, Edilu Jorga Sarba & Wakuma Mitiku Bune You can also search for this author in PubMed Google Scholar ContributionsE.Z.G conceived the idea, supervised the work, and critically revised the manuscript. M.L participated in the design, fieldwork, data analysis, and manuscript drafting. E.J.S and W.M.B assisted with data analysis, results interpretation, and manuscript enrichment. All authors have read and approved the final article. Corresponding authorCorrespondence to Endrias Zewdu Gebremedhin . Ethics declarationsEthics approval and consent to participate. Ambo University Research Ethics Review Committee has approved this study before it is carried out. In the study districts, consent was obtained from all the relevant authorities prior to data collection. Informed oral consent was obtained from each study participant before the interview using the Afaan Oromo language, and the data generated was kept as confidential records. The study participants were informed that the results of the study would be used exclusively for research purposes. This study was performed in line with the principles of the Declaration of Helsinki. Consent for publicationNot applicable. Competing interestsThe authors declare no competing interests. Additional informationPublisherâs note. Springer Nature remains neutral with regard to jurisdictional claims in published maps and institutional affiliations. Electronic supplementary materialBelow is the link to the electronic supplementary material. Supplementary Material 1Rights and permissions. Open Access This article is licensed under a Creative Commons Attribution 4.0 International License, which permits use, sharing, adaptation, distribution and reproduction in any medium or format, as long as you give appropriate credit to the original author(s) and the source, provide a link to the Creative Commons licence, and indicate if changes were made. The images or other third party material in this article are included in the articleâs Creative Commons licence, unless indicated otherwise in a credit line to the material. If material is not included in the articleâs Creative Commons licence and your intended use is not permitted by statutory regulation or exceeds the permitted use, you will need to obtain permission directly from the copyright holder. To view a copy of this licence, visit http://creativecommons.org/licenses/by/4.0/ . The Creative Commons Public Domain Dedication waiver ( http://creativecommons.org/publicdomain/zero/1.0/ ) applies to the data made available in this article, unless otherwise stated in a credit line to the data. Reprints and permissions About this articleCite this article. Layessa, M., Gebremedhin, E.Z., Sarba, E.J. et al. Assessing farmersâ willingness to pay for FMD vaccines and factors influencing payment: a contingent valuation study in central Oromia, Ethiopia. BMC Vet Res 20 , 313 (2024). https://doi.org/10.1186/s12917-024-04169-7 Download citation Received : 26 November 2023 Accepted : 01 July 2024 Published : 15 July 2024 DOI : https://doi.org/10.1186/s12917-024-04169-7 Share this articleAnyone you share the following link with will be able to read this content: Sorry, a shareable link is not currently available for this article. Provided by the Springer Nature SharedIt content-sharing initiative - Central Oromia
- Foot and mouth disease
- Willingness to pay
BMC Veterinary ResearchISSN: 1746-6148  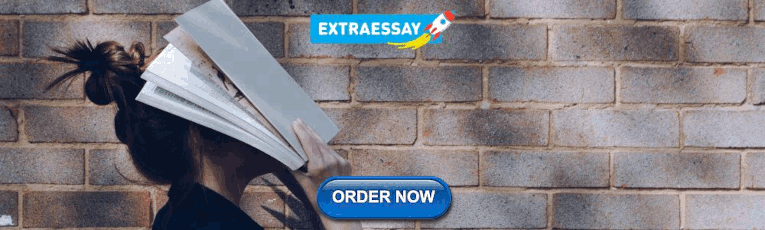 |
IMAGES
VIDEO
COMMENTS
Scientific research: Variables are used in scientific research to understand the relationships between different factors and to make predictions about future outcomes. For example, scientists may study the effects of different variables on plant growth or the impact of environmental factors on animal behavior.
Types of Variables in Research & Statistics | Examples. Published on September 19, 2022 by Rebecca Bevans. Revised on June 21, 2023. In statistical research, a variable is defined as an attribute of an object of study. Choosing which variables to measure is central to good experimental design.
The independent variable is the cause. Its value is independent of other variables in your study. The dependent variable is the effect. Its value depends on changes in the independent variable. Example: Independent and dependent variables. You design a study to test whether changes in room temperature have an effect on math test scores.
Suitable statistical design represents a critical factor in permitting inferences from any research or scientific study.[1] Numerous statistical designs are implementable due to the advancement of software available for extensive data analysis.[1] Healthcare providers must possess some statistical knowledge to interpret new studies and provide up-to-date patient care. We present an overview of ...
The Role of Variables in Research. In scientific research, variables serve several key functions: Define Relationships: Variables allow researchers to investigate the relationships between different factors and characteristics, providing insights into the underlying mechanisms that drive phenomena and outcomes. Establish Comparisons: By manipulating and comparing variables, scientists can ...
In statistical research, a variable is defined as an attribute of an object of study. Choosing which variables to measure is central to good experimental design. Example: Variables If you want to test whether some plant species are more salt-tolerant than others, ...
What is a control variable? In an experimental design, a control variable (or controlled variable) is a variable that is intentionally held constant to ensure it doesn't have an influence on any other variables. As a result, this variable remains unchanged throughout the course of the study. In other words, it's a variable that's not allowed to vary - tough life đ
A solid understanding of variables is the cornerstone in the conceptualization and preparation of a research protocol, and in the framing of study hypotheses. This subject is presented in two parts. This article, Part 1, explains what independent and dependent variables are, how an understanding of these is important in framing hypotheses, and ...
Below is an overview of some of these variables, highlighting their definitions and roles within research studies: Discrete variables: A discrete variable is a quantitative variable that represents quantitative data, such as the number of children in a family or the number of cars in a parking lot. Discrete variables can only take on specific ...
Variables in Research. The definition of a variable in the context of a research study is some feature with the potential to change, typically one that may influence or reflect a relationship or ...
However, it's important that you learn the difference because framing a study using these variables is a common approach to organizing the elements of a social sciences research study in order to discover relevant and meaningful results. Specifically, it is important for these two reasons: ... A variable in research simply refers to a person ...
In research, the independent variable is manipulated to observe its effect, while the dependent variable is the measured outcome. Essentially, the independent variable is the presumed cause, and the dependent variable is the observed effect. Variables provide the foundation for examining relationships, drawing conclusions, and making ...
A variable is any kind of attribute or characteristic that you are trying to measure, manipulate and control in statistics and research. All studies analyze a variable, which can describe a person, place, thing or idea. A variable's value can change between groups or over time.
Research; Moderator Variable: The moderator variable affects the cause-and-effect relationship between the independent and dependent variables. As a result, the influence of the independent variable is in the presence of the moderator variable. Gender; Race; Class; Suppose you want to conduct a study, educational awareness of a specific area.
Variables. What is a variable?[1,2] To put it in very simple terms, a variable is an entity whose value varies.A variable is an essential component of any statistical data. It is a feature of a member of a given sample or population, which is unique, and can differ in quantity or quantity from another member of the same sample or population.
Examples of independent variables in different research contexts. Independent variables can span a wide range of forms, varying from research context to research context. Here are a few examples: In a medical study investigating the effect of a drug on patients' symptoms, the independent variable could be the dosage of the drug administered.
The two main types of variables in psychology are the independent variable and the dependent variable. Both variables are important in the process of collecting data about psychological phenomena. This article discusses different types of variables that are used in psychology research. It also covers how to operationalize these variables when ...
The purpose of Study 1 is to measure the impact of different plant fertilizers on how many fruits apple trees bear. Independent variable: plant fertilizers (chosen by researchers) Dependent variable: fruits that the trees bear (affected by choice of fertilizers) The purpose of Study 2 is to find an association between living in close vicinity ...
For example, in the study of third graders' reading scores, such variables as noise levels in the testing room, the size or lighting or temperature of the room, and whether the children had had a good breakfast, all might be extraneous variables. There is a special class of extraneous variables called confounding variables.
The study revealed that as assessed by the sports officiating officials when they are grouped according to the study's variables, the results show a "high level" in all areas.Furthermore, the ...
"In a research study, the independent variable defines a principal focus of research interest. It is the consequent variable that is presumably affected by one or more independent variables that are either manipulated by the researcher or observed by the researcher and regarded as antecedent conditions that determine the value of the ...
Extraneous variables are not of interest to the study but may influence the dependent variable. For this reason, most quantitative studies attempt to control extraneous variables. The literature should inform you what extraneous variables to account for. There is a special class of extraneous variables called confounding variables.
Variables are any characteristics in the study that can take on different values. The main difference between independent and dependent variables is cause and effect. The independent variable is not expected to be impacted by the study (it's independent), but to cause the difference in the dependent variable. The dependent variable is the ...
Quantitative research involves many kinds of variables. There are four main types discussed in further detail below: Independent variables (IV). Dependent variables (DV). ... In a study of changes in skill levels in a group of caseworkers after a training program, if the follow-up measure is taken relatively late after the training, the simple ...
Research integrity includes: the use of honest and verifiable methods in proposing, performing, and evaluating research reporting research results with particular attention to adherence to rules, regulations, and guidelines following commonly accepted professional codes or norms; Shared values in scientific research
Variables also play a crucial role in the design of research studies. The selection of variables determines the type of research design that will be used, as well as the methods and procedures for collecting and analyzing data. Variables can be independent, dependent, or moderator variables, depending on their role in the research design.
Conical orthopedic drill bits may have the potential to improve the stabilization of orthopedic screws. During perforations, heat energy is released, and elevated temperatures could be related to thermal osteonecrosis. This study was designed to evaluate the thermal behavior of an experimental conical drill bit, when compared to the conventional cylindrical drill, using polyurethane blocks ...
An increasing number of people are using multiple medications each day, named polypharmacy. This is driven by an ageing population, increasing multimorbidity, and single disease-focussed guidelines. Medications carry obvious benefits, yet polypharmacy is also linked to adverse consequences including adverse drug events, drug-drug and drug-disease interactions, poor patient experience and ...
Descriptive research gathers data without controlling any variables, while experimental research manipulates and controls variables to determine cause and effect. ... you have to make more concrete decisions about your research methods and the details of the study. Read more about creating a research design. Other interesting articles.
Study area and population. The study was conducted in three districts in the central part of the Oromia region of Ethiopia, namely, the Ambo, Dendi, and Holeta districts (Fig. 1), where the mixed crop-livestock farming system is the basis for the community's livelihood.There are two main types of production systems practiced in the region: the dominant mixed crop-livestock (MF) production ...