- UConn Library
- Literature Review: The What, Why and How-to Guide
- Introduction
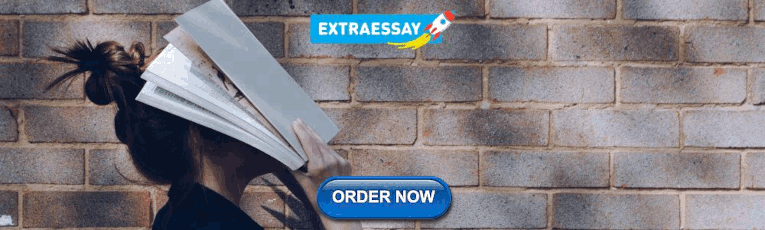
Literature Review: The What, Why and How-to Guide — Introduction
- Getting Started
- How to Pick a Topic
- Strategies to Find Sources
- Evaluating Sources & Lit. Reviews
- Tips for Writing Literature Reviews
- Writing Literature Review: Useful Sites
- Citation Resources
- Other Academic Writings
What are Literature Reviews?
So, what is a literature review? "A literature review is an account of what has been published on a topic by accredited scholars and researchers. In writing the literature review, your purpose is to convey to your reader what knowledge and ideas have been established on a topic, and what their strengths and weaknesses are. As a piece of writing, the literature review must be defined by a guiding concept (e.g., your research objective, the problem or issue you are discussing, or your argumentative thesis). It is not just a descriptive list of the material available, or a set of summaries." Taylor, D. The literature review: A few tips on conducting it . University of Toronto Health Sciences Writing Centre.
Goals of Literature Reviews
What are the goals of creating a Literature Review? A literature could be written to accomplish different aims:
- To develop a theory or evaluate an existing theory
- To summarize the historical or existing state of a research topic
- Identify a problem in a field of research
Baumeister, R. F., & Leary, M. R. (1997). Writing narrative literature reviews . Review of General Psychology , 1 (3), 311-320.
What kinds of sources require a Literature Review?
- A research paper assigned in a course
- A thesis or dissertation
- A grant proposal
- An article intended for publication in a journal
All these instances require you to collect what has been written about your research topic so that you can demonstrate how your own research sheds new light on the topic.
Types of Literature Reviews
What kinds of literature reviews are written?
Narrative review: The purpose of this type of review is to describe the current state of the research on a specific topic/research and to offer a critical analysis of the literature reviewed. Studies are grouped by research/theoretical categories, and themes and trends, strengths and weakness, and gaps are identified. The review ends with a conclusion section which summarizes the findings regarding the state of the research of the specific study, the gaps identify and if applicable, explains how the author's research will address gaps identify in the review and expand the knowledge on the topic reviewed.
- Example : Predictors and Outcomes of U.S. Quality Maternity Leave: A Review and Conceptual Framework: 10.1177/08948453211037398
Systematic review : "The authors of a systematic review use a specific procedure to search the research literature, select the studies to include in their review, and critically evaluate the studies they find." (p. 139). Nelson, L. K. (2013). Research in Communication Sciences and Disorders . Plural Publishing.
- Example : The effect of leave policies on increasing fertility: a systematic review: 10.1057/s41599-022-01270-w
Meta-analysis : "Meta-analysis is a method of reviewing research findings in a quantitative fashion by transforming the data from individual studies into what is called an effect size and then pooling and analyzing this information. The basic goal in meta-analysis is to explain why different outcomes have occurred in different studies." (p. 197). Roberts, M. C., & Ilardi, S. S. (2003). Handbook of Research Methods in Clinical Psychology . Blackwell Publishing.
- Example : Employment Instability and Fertility in Europe: A Meta-Analysis: 10.1215/00703370-9164737
Meta-synthesis : "Qualitative meta-synthesis is a type of qualitative study that uses as data the findings from other qualitative studies linked by the same or related topic." (p.312). Zimmer, L. (2006). Qualitative meta-synthesis: A question of dialoguing with texts . Journal of Advanced Nursing , 53 (3), 311-318.
- Example : Women’s perspectives on career successes and barriers: A qualitative meta-synthesis: 10.1177/05390184221113735
Literature Reviews in the Health Sciences
- UConn Health subject guide on systematic reviews Explanation of the different review types used in health sciences literature as well as tools to help you find the right review type
- << Previous: Getting Started
- Next: How to Pick a Topic >>
- Last Updated: Sep 21, 2022 2:16 PM
- URL: https://guides.lib.uconn.edu/literaturereview

Methodological Approaches to Literature Review
- Living reference work entry
- First Online: 09 May 2023
- Cite this living reference work entry
- Dennis Thomas 2 ,
- Elida Zairina 3 &
- Johnson George 4
606 Accesses
1 Citations
The literature review can serve various functions in the contexts of education and research. It aids in identifying knowledge gaps, informing research methodology, and developing a theoretical framework during the planning stages of a research study or project, as well as reporting of review findings in the context of the existing literature. This chapter discusses the methodological approaches to conducting a literature review and offers an overview of different types of reviews. There are various types of reviews, including narrative reviews, scoping reviews, and systematic reviews with reporting strategies such as meta-analysis and meta-synthesis. Review authors should consider the scope of the literature review when selecting a type and method. Being focused is essential for a successful review; however, this must be balanced against the relevance of the review to a broad audience.
This is a preview of subscription content, log in via an institution to check access.
Access this chapter
Institutional subscriptions
Similar content being viewed by others
Reviewing Literature for and as Research
Discussion and Conclusion
Systematic Reviews in Educational Research: Methodology, Perspectives and Application
Akobeng AK. Principles of evidence based medicine. Arch Dis Child. 2005;90(8):837–40.
Article CAS PubMed PubMed Central Google Scholar
Alharbi A, Stevenson M. Refining Boolean queries to identify relevant studies for systematic review updates. J Am Med Inform Assoc. 2020;27(11):1658–66.
Article PubMed PubMed Central Google Scholar
Arksey H, O’Malley L. Scoping studies: towards a methodological framework. Int J Soc Res Methodol. 2005;8(1):19–32.
Article Google Scholar
Aromataris E MZE. JBI manual for evidence synthesis. 2020.
Google Scholar
Aromataris E, Pearson A. The systematic review: an overview. Am J Nurs. 2014;114(3):53–8.
Article PubMed Google Scholar
Aromataris E, Riitano D. Constructing a search strategy and searching for evidence. A guide to the literature search for a systematic review. Am J Nurs. 2014;114(5):49–56.
Babineau J. Product review: covidence (systematic review software). J Canad Health Libr Assoc Canada. 2014;35(2):68–71.
Baker JD. The purpose, process, and methods of writing a literature review. AORN J. 2016;103(3):265–9.
Bastian H, Glasziou P, Chalmers I. Seventy-five trials and eleven systematic reviews a day: how will we ever keep up? PLoS Med. 2010;7(9):e1000326.
Bramer WM, Rethlefsen ML, Kleijnen J, Franco OH. Optimal database combinations for literature searches in systematic reviews: a prospective exploratory study. Syst Rev. 2017;6(1):1–12.
Brown D. A review of the PubMed PICO tool: using evidence-based practice in health education. Health Promot Pract. 2020;21(4):496–8.
Cargo M, Harris J, Pantoja T, et al. Cochrane qualitative and implementation methods group guidance series – paper 4: methods for assessing evidence on intervention implementation. J Clin Epidemiol. 2018;97:59–69.
Cook DJ, Mulrow CD, Haynes RB. Systematic reviews: synthesis of best evidence for clinical decisions. Ann Intern Med. 1997;126(5):376–80.
Article CAS PubMed Google Scholar
Counsell C. Formulating questions and locating primary studies for inclusion in systematic reviews. Ann Intern Med. 1997;127(5):380–7.
Cummings SR, Browner WS, Hulley SB. Conceiving the research question and developing the study plan. In: Cummings SR, Browner WS, Hulley SB, editors. Designing Clinical Research: An Epidemiological Approach. 4th ed. Philadelphia (PA): P Lippincott Williams & Wilkins; 2007. p. 14–22.
Eriksen MB, Frandsen TF. The impact of patient, intervention, comparison, outcome (PICO) as a search strategy tool on literature search quality: a systematic review. JMLA. 2018;106(4):420.
Ferrari R. Writing narrative style literature reviews. Medical Writing. 2015;24(4):230–5.
Flemming K, Booth A, Hannes K, Cargo M, Noyes J. Cochrane qualitative and implementation methods group guidance series – paper 6: reporting guidelines for qualitative, implementation, and process evaluation evidence syntheses. J Clin Epidemiol. 2018;97:79–85.
Grant MJ, Booth A. A typology of reviews: an analysis of 14 review types and associated methodologies. Health Inf Libr J. 2009;26(2):91–108.
Green BN, Johnson CD, Adams A. Writing narrative literature reviews for peer-reviewed journals: secrets of the trade. J Chiropr Med. 2006;5(3):101–17.
Gregory AT, Denniss AR. An introduction to writing narrative and systematic reviews; tasks, tips and traps for aspiring authors. Heart Lung Circ. 2018;27(7):893–8.
Harden A, Thomas J, Cargo M, et al. Cochrane qualitative and implementation methods group guidance series – paper 5: methods for integrating qualitative and implementation evidence within intervention effectiveness reviews. J Clin Epidemiol. 2018;97:70–8.
Harris JL, Booth A, Cargo M, et al. Cochrane qualitative and implementation methods group guidance series – paper 2: methods for question formulation, searching, and protocol development for qualitative evidence synthesis. J Clin Epidemiol. 2018;97:39–48.
Higgins J, Thomas J. In: Chandler J, Cumpston M, Li T, Page MJ, Welch VA, editors. Cochrane Handbook for Systematic Reviews of Interventions version 6.3, updated February 2022). Available from www.training.cochrane.org/handbook.: Cochrane; 2022.
International prospective register of systematic reviews (PROSPERO). Available from https://www.crd.york.ac.uk/prospero/ .
Khan KS, Kunz R, Kleijnen J, Antes G. Five steps to conducting a systematic review. J R Soc Med. 2003;96(3):118–21.
Landhuis E. Scientific literature: information overload. Nature. 2016;535(7612):457–8.
Lockwood C, Porritt K, Munn Z, Rittenmeyer L, Salmond S, Bjerrum M, Loveday H, Carrier J, Stannard D. Chapter 2: Systematic reviews of qualitative evidence. In: Aromataris E, Munn Z, editors. JBI Manual for Evidence Synthesis. JBI; 2020. Available from https://synthesismanual.jbi.global . https://doi.org/10.46658/JBIMES-20-03 .
Chapter Google Scholar
Lorenzetti DL, Topfer L-A, Dennett L, Clement F. Value of databases other than medline for rapid health technology assessments. Int J Technol Assess Health Care. 2014;30(2):173–8.
Moher D, Liberati A, Tetzlaff J, Altman DG, the PRISMA Group. Preferred reporting items for (SR) and meta-analyses: the PRISMA statement. Ann Intern Med. 2009;6:264–9.
Mulrow CD. Systematic reviews: rationale for systematic reviews. BMJ. 1994;309(6954):597–9.
Munn Z, Peters MDJ, Stern C, Tufanaru C, McArthur A, Aromataris E. Systematic review or scoping review? Guidance for authors when choosing between a systematic or scoping review approach. BMC Med Res Methodol. 2018;18(1):143.
Munthe-Kaas HM, Glenton C, Booth A, Noyes J, Lewin S. Systematic mapping of existing tools to appraise methodological strengths and limitations of qualitative research: first stage in the development of the CAMELOT tool. BMC Med Res Methodol. 2019;19(1):1–13.
Murphy CM. Writing an effective review article. J Med Toxicol. 2012;8(2):89–90.
NHMRC. Guidelines for guidelines: assessing risk of bias. Available at https://nhmrc.gov.au/guidelinesforguidelines/develop/assessing-risk-bias . Last published 29 August 2019. Accessed 29 Aug 2022.
Noyes J, Booth A, Cargo M, et al. Cochrane qualitative and implementation methods group guidance series – paper 1: introduction. J Clin Epidemiol. 2018b;97:35–8.
Noyes J, Booth A, Flemming K, et al. Cochrane qualitative and implementation methods group guidance series – paper 3: methods for assessing methodological limitations, data extraction and synthesis, and confidence in synthesized qualitative findings. J Clin Epidemiol. 2018a;97:49–58.
Noyes J, Booth A, Moore G, Flemming K, Tunçalp Ö, Shakibazadeh E. Synthesising quantitative and qualitative evidence to inform guidelines on complex interventions: clarifying the purposes, designs and outlining some methods. BMJ Glob Health. 2019;4(Suppl 1):e000893.
Peters MD, Godfrey CM, Khalil H, McInerney P, Parker D, Soares CB. Guidance for conducting systematic scoping reviews. Int J Evid Healthcare. 2015;13(3):141–6.
Polanin JR, Pigott TD, Espelage DL, Grotpeter JK. Best practice guidelines for abstract screening large-evidence systematic reviews and meta-analyses. Res Synth Methods. 2019;10(3):330–42.
Article PubMed Central Google Scholar
Shea BJ, Grimshaw JM, Wells GA, et al. Development of AMSTAR: a measurement tool to assess the methodological quality of systematic reviews. BMC Med Res Methodol. 2007;7(1):1–7.
Shea BJ, Reeves BC, Wells G, et al. AMSTAR 2: a critical appraisal tool for systematic reviews that include randomised or non-randomised studies of healthcare interventions, or both. Brit Med J. 2017;358
Sterne JA, Hernán MA, Reeves BC, et al. ROBINS-I: a tool for assessing risk of bias in non-randomised studies of interventions. Br Med J. 2016;355
Stroup DF, Berlin JA, Morton SC, et al. Meta-analysis of observational studies in epidemiology: a proposal for reporting. JAMA. 2000;283(15):2008–12.
Tawfik GM, Dila KAS, Mohamed MYF, et al. A step by step guide for conducting a systematic review and meta-analysis with simulation data. Trop Med Health. 2019;47(1):1–9.
The Critical Appraisal Program. Critical appraisal skills program. Available at https://casp-uk.net/ . 2022. Accessed 29 Aug 2022.
The University of Melbourne. Writing a literature review in Research Techniques 2022. Available at https://students.unimelb.edu.au/academic-skills/explore-our-resources/research-techniques/reviewing-the-literature . Accessed 29 Aug 2022.
The Writing Center University of Winconsin-Madison. Learn how to write a literature review in The Writer’s Handbook – Academic Professional Writing. 2022. Available at https://writing.wisc.edu/handbook/assignments/reviewofliterature/ . Accessed 29 Aug 2022.
Thompson SG, Sharp SJ. Explaining heterogeneity in meta-analysis: a comparison of methods. Stat Med. 1999;18(20):2693–708.
Tricco AC, Lillie E, Zarin W, et al. A scoping review on the conduct and reporting of scoping reviews. BMC Med Res Methodol. 2016;16(1):15.
Tricco AC, Lillie E, Zarin W, et al. PRISMA extension for scoping reviews (PRISMA-ScR): checklist and explanation. Ann Intern Med. 2018;169(7):467–73.
Yoneoka D, Henmi M. Clinical heterogeneity in random-effect meta-analysis: between-study boundary estimate problem. Stat Med. 2019;38(21):4131–45.
Yuan Y, Hunt RH. Systematic reviews: the good, the bad, and the ugly. Am J Gastroenterol. 2009;104(5):1086–92.
Download references
Author information
Authors and affiliations.
Centre of Excellence in Treatable Traits, College of Health, Medicine and Wellbeing, University of Newcastle, Hunter Medical Research Institute Asthma and Breathing Programme, Newcastle, NSW, Australia
Dennis Thomas
Department of Pharmacy Practice, Faculty of Pharmacy, Universitas Airlangga, Surabaya, Indonesia
Elida Zairina
Centre for Medicine Use and Safety, Monash Institute of Pharmaceutical Sciences, Faculty of Pharmacy and Pharmaceutical Sciences, Monash University, Parkville, VIC, Australia
Johnson George
You can also search for this author in PubMed Google Scholar
Corresponding author
Correspondence to Johnson George .
Section Editor information
College of Pharmacy, Qatar University, Doha, Qatar
Derek Charles Stewart
Department of Pharmacy, University of Huddersfield, Huddersfield, United Kingdom
Zaheer-Ud-Din Babar
Rights and permissions
Reprints and permissions
Copyright information
© 2023 Springer Nature Switzerland AG
About this entry
Cite this entry.
Thomas, D., Zairina, E., George, J. (2023). Methodological Approaches to Literature Review. In: Encyclopedia of Evidence in Pharmaceutical Public Health and Health Services Research in Pharmacy. Springer, Cham. https://doi.org/10.1007/978-3-030-50247-8_57-1
Download citation
DOI : https://doi.org/10.1007/978-3-030-50247-8_57-1
Received : 22 February 2023
Accepted : 22 February 2023
Published : 09 May 2023
Publisher Name : Springer, Cham
Print ISBN : 978-3-030-50247-8
Online ISBN : 978-3-030-50247-8
eBook Packages : Springer Reference Biomedicine and Life Sciences Reference Module Biomedical and Life Sciences
- Publish with us
Policies and ethics
- Find a journal
- Track your research

An official website of the United States government
The .gov means it’s official. Federal government websites often end in .gov or .mil. Before sharing sensitive information, make sure you’re on a federal government site.
The site is secure. The https:// ensures that you are connecting to the official website and that any information you provide is encrypted and transmitted securely.
- Publications
- Account settings
Preview improvements coming to the PMC website in October 2024. Learn More or Try it out now .
- Advanced Search
- Journal List
- PLoS Comput Biol
- v.9(7); 2013 Jul

Ten Simple Rules for Writing a Literature Review
Marco pautasso.
1 Centre for Functional and Evolutionary Ecology (CEFE), CNRS, Montpellier, France
2 Centre for Biodiversity Synthesis and Analysis (CESAB), FRB, Aix-en-Provence, France
Literature reviews are in great demand in most scientific fields. Their need stems from the ever-increasing output of scientific publications [1] . For example, compared to 1991, in 2008 three, eight, and forty times more papers were indexed in Web of Science on malaria, obesity, and biodiversity, respectively [2] . Given such mountains of papers, scientists cannot be expected to examine in detail every single new paper relevant to their interests [3] . Thus, it is both advantageous and necessary to rely on regular summaries of the recent literature. Although recognition for scientists mainly comes from primary research, timely literature reviews can lead to new synthetic insights and are often widely read [4] . For such summaries to be useful, however, they need to be compiled in a professional way [5] .
When starting from scratch, reviewing the literature can require a titanic amount of work. That is why researchers who have spent their career working on a certain research issue are in a perfect position to review that literature. Some graduate schools are now offering courses in reviewing the literature, given that most research students start their project by producing an overview of what has already been done on their research issue [6] . However, it is likely that most scientists have not thought in detail about how to approach and carry out a literature review.
Reviewing the literature requires the ability to juggle multiple tasks, from finding and evaluating relevant material to synthesising information from various sources, from critical thinking to paraphrasing, evaluating, and citation skills [7] . In this contribution, I share ten simple rules I learned working on about 25 literature reviews as a PhD and postdoctoral student. Ideas and insights also come from discussions with coauthors and colleagues, as well as feedback from reviewers and editors.
Rule 1: Define a Topic and Audience
How to choose which topic to review? There are so many issues in contemporary science that you could spend a lifetime of attending conferences and reading the literature just pondering what to review. On the one hand, if you take several years to choose, several other people may have had the same idea in the meantime. On the other hand, only a well-considered topic is likely to lead to a brilliant literature review [8] . The topic must at least be:
- interesting to you (ideally, you should have come across a series of recent papers related to your line of work that call for a critical summary),
- an important aspect of the field (so that many readers will be interested in the review and there will be enough material to write it), and
- a well-defined issue (otherwise you could potentially include thousands of publications, which would make the review unhelpful).
Ideas for potential reviews may come from papers providing lists of key research questions to be answered [9] , but also from serendipitous moments during desultory reading and discussions. In addition to choosing your topic, you should also select a target audience. In many cases, the topic (e.g., web services in computational biology) will automatically define an audience (e.g., computational biologists), but that same topic may also be of interest to neighbouring fields (e.g., computer science, biology, etc.).
Rule 2: Search and Re-search the Literature
After having chosen your topic and audience, start by checking the literature and downloading relevant papers. Five pieces of advice here:
- keep track of the search items you use (so that your search can be replicated [10] ),
- keep a list of papers whose pdfs you cannot access immediately (so as to retrieve them later with alternative strategies),
- use a paper management system (e.g., Mendeley, Papers, Qiqqa, Sente),
- define early in the process some criteria for exclusion of irrelevant papers (these criteria can then be described in the review to help define its scope), and
- do not just look for research papers in the area you wish to review, but also seek previous reviews.
The chances are high that someone will already have published a literature review ( Figure 1 ), if not exactly on the issue you are planning to tackle, at least on a related topic. If there are already a few or several reviews of the literature on your issue, my advice is not to give up, but to carry on with your own literature review,

The bottom-right situation (many literature reviews but few research papers) is not just a theoretical situation; it applies, for example, to the study of the impacts of climate change on plant diseases, where there appear to be more literature reviews than research studies [33] .
- discussing in your review the approaches, limitations, and conclusions of past reviews,
- trying to find a new angle that has not been covered adequately in the previous reviews, and
- incorporating new material that has inevitably accumulated since their appearance.
When searching the literature for pertinent papers and reviews, the usual rules apply:
- be thorough,
- use different keywords and database sources (e.g., DBLP, Google Scholar, ISI Proceedings, JSTOR Search, Medline, Scopus, Web of Science), and
- look at who has cited past relevant papers and book chapters.
Rule 3: Take Notes While Reading
If you read the papers first, and only afterwards start writing the review, you will need a very good memory to remember who wrote what, and what your impressions and associations were while reading each single paper. My advice is, while reading, to start writing down interesting pieces of information, insights about how to organize the review, and thoughts on what to write. This way, by the time you have read the literature you selected, you will already have a rough draft of the review.
Of course, this draft will still need much rewriting, restructuring, and rethinking to obtain a text with a coherent argument [11] , but you will have avoided the danger posed by staring at a blank document. Be careful when taking notes to use quotation marks if you are provisionally copying verbatim from the literature. It is advisable then to reformulate such quotes with your own words in the final draft. It is important to be careful in noting the references already at this stage, so as to avoid misattributions. Using referencing software from the very beginning of your endeavour will save you time.
Rule 4: Choose the Type of Review You Wish to Write
After having taken notes while reading the literature, you will have a rough idea of the amount of material available for the review. This is probably a good time to decide whether to go for a mini- or a full review. Some journals are now favouring the publication of rather short reviews focusing on the last few years, with a limit on the number of words and citations. A mini-review is not necessarily a minor review: it may well attract more attention from busy readers, although it will inevitably simplify some issues and leave out some relevant material due to space limitations. A full review will have the advantage of more freedom to cover in detail the complexities of a particular scientific development, but may then be left in the pile of the very important papers “to be read” by readers with little time to spare for major monographs.
There is probably a continuum between mini- and full reviews. The same point applies to the dichotomy of descriptive vs. integrative reviews. While descriptive reviews focus on the methodology, findings, and interpretation of each reviewed study, integrative reviews attempt to find common ideas and concepts from the reviewed material [12] . A similar distinction exists between narrative and systematic reviews: while narrative reviews are qualitative, systematic reviews attempt to test a hypothesis based on the published evidence, which is gathered using a predefined protocol to reduce bias [13] , [14] . When systematic reviews analyse quantitative results in a quantitative way, they become meta-analyses. The choice between different review types will have to be made on a case-by-case basis, depending not just on the nature of the material found and the preferences of the target journal(s), but also on the time available to write the review and the number of coauthors [15] .
Rule 5: Keep the Review Focused, but Make It of Broad Interest
Whether your plan is to write a mini- or a full review, it is good advice to keep it focused 16 , 17 . Including material just for the sake of it can easily lead to reviews that are trying to do too many things at once. The need to keep a review focused can be problematic for interdisciplinary reviews, where the aim is to bridge the gap between fields [18] . If you are writing a review on, for example, how epidemiological approaches are used in modelling the spread of ideas, you may be inclined to include material from both parent fields, epidemiology and the study of cultural diffusion. This may be necessary to some extent, but in this case a focused review would only deal in detail with those studies at the interface between epidemiology and the spread of ideas.
While focus is an important feature of a successful review, this requirement has to be balanced with the need to make the review relevant to a broad audience. This square may be circled by discussing the wider implications of the reviewed topic for other disciplines.
Rule 6: Be Critical and Consistent
Reviewing the literature is not stamp collecting. A good review does not just summarize the literature, but discusses it critically, identifies methodological problems, and points out research gaps [19] . After having read a review of the literature, a reader should have a rough idea of:
- the major achievements in the reviewed field,
- the main areas of debate, and
- the outstanding research questions.
It is challenging to achieve a successful review on all these fronts. A solution can be to involve a set of complementary coauthors: some people are excellent at mapping what has been achieved, some others are very good at identifying dark clouds on the horizon, and some have instead a knack at predicting where solutions are going to come from. If your journal club has exactly this sort of team, then you should definitely write a review of the literature! In addition to critical thinking, a literature review needs consistency, for example in the choice of passive vs. active voice and present vs. past tense.
Rule 7: Find a Logical Structure
Like a well-baked cake, a good review has a number of telling features: it is worth the reader's time, timely, systematic, well written, focused, and critical. It also needs a good structure. With reviews, the usual subdivision of research papers into introduction, methods, results, and discussion does not work or is rarely used. However, a general introduction of the context and, toward the end, a recapitulation of the main points covered and take-home messages make sense also in the case of reviews. For systematic reviews, there is a trend towards including information about how the literature was searched (database, keywords, time limits) [20] .
How can you organize the flow of the main body of the review so that the reader will be drawn into and guided through it? It is generally helpful to draw a conceptual scheme of the review, e.g., with mind-mapping techniques. Such diagrams can help recognize a logical way to order and link the various sections of a review [21] . This is the case not just at the writing stage, but also for readers if the diagram is included in the review as a figure. A careful selection of diagrams and figures relevant to the reviewed topic can be very helpful to structure the text too [22] .
Rule 8: Make Use of Feedback
Reviews of the literature are normally peer-reviewed in the same way as research papers, and rightly so [23] . As a rule, incorporating feedback from reviewers greatly helps improve a review draft. Having read the review with a fresh mind, reviewers may spot inaccuracies, inconsistencies, and ambiguities that had not been noticed by the writers due to rereading the typescript too many times. It is however advisable to reread the draft one more time before submission, as a last-minute correction of typos, leaps, and muddled sentences may enable the reviewers to focus on providing advice on the content rather than the form.
Feedback is vital to writing a good review, and should be sought from a variety of colleagues, so as to obtain a diversity of views on the draft. This may lead in some cases to conflicting views on the merits of the paper, and on how to improve it, but such a situation is better than the absence of feedback. A diversity of feedback perspectives on a literature review can help identify where the consensus view stands in the landscape of the current scientific understanding of an issue [24] .
Rule 9: Include Your Own Relevant Research, but Be Objective
In many cases, reviewers of the literature will have published studies relevant to the review they are writing. This could create a conflict of interest: how can reviewers report objectively on their own work [25] ? Some scientists may be overly enthusiastic about what they have published, and thus risk giving too much importance to their own findings in the review. However, bias could also occur in the other direction: some scientists may be unduly dismissive of their own achievements, so that they will tend to downplay their contribution (if any) to a field when reviewing it.
In general, a review of the literature should neither be a public relations brochure nor an exercise in competitive self-denial. If a reviewer is up to the job of producing a well-organized and methodical review, which flows well and provides a service to the readership, then it should be possible to be objective in reviewing one's own relevant findings. In reviews written by multiple authors, this may be achieved by assigning the review of the results of a coauthor to different coauthors.
Rule 10: Be Up-to-Date, but Do Not Forget Older Studies
Given the progressive acceleration in the publication of scientific papers, today's reviews of the literature need awareness not just of the overall direction and achievements of a field of inquiry, but also of the latest studies, so as not to become out-of-date before they have been published. Ideally, a literature review should not identify as a major research gap an issue that has just been addressed in a series of papers in press (the same applies, of course, to older, overlooked studies (“sleeping beauties” [26] )). This implies that literature reviewers would do well to keep an eye on electronic lists of papers in press, given that it can take months before these appear in scientific databases. Some reviews declare that they have scanned the literature up to a certain point in time, but given that peer review can be a rather lengthy process, a full search for newly appeared literature at the revision stage may be worthwhile. Assessing the contribution of papers that have just appeared is particularly challenging, because there is little perspective with which to gauge their significance and impact on further research and society.
Inevitably, new papers on the reviewed topic (including independently written literature reviews) will appear from all quarters after the review has been published, so that there may soon be the need for an updated review. But this is the nature of science [27] – [32] . I wish everybody good luck with writing a review of the literature.
Acknowledgments
Many thanks to M. Barbosa, K. Dehnen-Schmutz, T. Döring, D. Fontaneto, M. Garbelotto, O. Holdenrieder, M. Jeger, D. Lonsdale, A. MacLeod, P. Mills, M. Moslonka-Lefebvre, G. Stancanelli, P. Weisberg, and X. Xu for insights and discussions, and to P. Bourne, T. Matoni, and D. Smith for helpful comments on a previous draft.
Funding Statement
This work was funded by the French Foundation for Research on Biodiversity (FRB) through its Centre for Synthesis and Analysis of Biodiversity data (CESAB), as part of the NETSEED research project. The funders had no role in the preparation of the manuscript.
- Reserve a study room
- Library Account
- Undergraduate Students
- Graduate Students
- Faculty & Staff
How to Conduct a Literature Review (Health Sciences and Beyond)
What is a literature review, traditional (narrative) literature review, integrative literature review, systematic reviews, meta-analysis, scoping review.
- Developing a Research Question
- Selection Criteria
- Database Search
- Documenting Your Search
- Organize Key Findings
- Reference Management
Ask Us! Health Sciences Library
The health sciences library.
Call toll-free: (844) 352-7399 E-mail: Ask Us More contact information
Related Guides
- Systematic Reviews by Roy Brown Last Updated Oct 17, 2023 684 views this year
- Write a Literature Review by John Glover Last Updated Oct 16, 2023 3472 views this year
A literature review provides an overview of what's been written about a specific topic. There are many different types of literature reviews. They vary in terms of comprehensiveness, types of study included, and purpose.
The other pages in this guide will cover some basic steps to consider when conducting a traditional health sciences literature review. See below for a quick look at some of the more popular types of literature reviews.
For additional information on a variety of review methods, the following article provides an excellent overview.
Grant MJ, Booth A. A typology of reviews: an analysis of 14 review types and associated methodologies. Health Info Libr J. 2009 Jun;26(2):91-108. doi: 10.1111/j.1471-1842.2009.00848.x. Review. PubMed PMID: 19490148.
A traditional (narrative) literature review provides a quick overview of current studies. It helps explain why your study is important in the context of the literature, and can also help you identify areas that need further research. The rest of this guide will cover some basic steps to consider when conducting a traditional literature review. Click on the right thumbnail to see an excerpt from this type of literature review. |
|
Integrative reviews "synthesize findings from different approaches, like experimental and non-experimental studies" ( ). They may or may not be systematic reviews. Click on the right thumbnail to see an excerpt from this type of literature review. |
|
Systematic reviews synthesize high quality empirical information to answer a given research question ( ). Conducting a systematic review involves following rigorous, predefined protocols that "minimise bias and ensure transparency" ( ). See our for more information on what they are and how to conduct one. Click on the right thumbnail to see an excerpt from this type of literature review. |
Meta-analyses are "the statistical integration of separate studies" ( ). They involve identifying similar studies and pooling their data to obtain a more accurate estimate of true effect size. A systematic review can include a meta-analysis. Click on the right thumbnail to see an excerpt from this type of literature review. |
|
A scoping review involves a broad research question that explores the current evidence base ( ). It can help inform areas that are appropriate for a systematic review. Click on the right thumbnail to see an excerpt from this type of literature review. |
|
- Next: Developing a Research Question >>
- Last Updated: Mar 15, 2024 12:22 PM
- URL: https://guides.library.vcu.edu/health-sciences-lit-review

How To Write An A-Grade Literature Review
3 straightforward steps (with examples) + free template.
By: Derek Jansen (MBA) | Expert Reviewed By: Dr. Eunice Rautenbach | October 2019
Quality research is about building onto the existing work of others , “standing on the shoulders of giants”, as Newton put it. The literature review chapter of your dissertation, thesis or research project is where you synthesise this prior work and lay the theoretical foundation for your own research.
Long story short, this chapter is a pretty big deal, which is why you want to make sure you get it right . In this post, I’ll show you exactly how to write a literature review in three straightforward steps, so you can conquer this vital chapter (the smart way).
Overview: The Literature Review Process
- Understanding the “ why “
- Finding the relevant literature
- Cataloguing and synthesising the information
- Outlining & writing up your literature review
- Example of a literature review
But first, the “why”…
Before we unpack how to write the literature review chapter, we’ve got to look at the why . To put it bluntly, if you don’t understand the function and purpose of the literature review process, there’s no way you can pull it off well. So, what exactly is the purpose of the literature review?
Well, there are (at least) four core functions:
- For you to gain an understanding (and demonstrate this understanding) of where the research is at currently, what the key arguments and disagreements are.
- For you to identify the gap(s) in the literature and then use this as justification for your own research topic.
- To help you build a conceptual framework for empirical testing (if applicable to your research topic).
- To inform your methodological choices and help you source tried and tested questionnaires (for interviews ) and measurement instruments (for surveys ).
Most students understand the first point but don’t give any thought to the rest. To get the most from the literature review process, you must keep all four points front of mind as you review the literature (more on this shortly), or you’ll land up with a wonky foundation.
Okay – with the why out the way, let’s move on to the how . As mentioned above, writing your literature review is a process, which I’ll break down into three steps:
- Finding the most suitable literature
- Understanding , distilling and organising the literature
- Planning and writing up your literature review chapter
Importantly, you must complete steps one and two before you start writing up your chapter. I know it’s very tempting, but don’t try to kill two birds with one stone and write as you read. You’ll invariably end up wasting huge amounts of time re-writing and re-shaping, or you’ll just land up with a disjointed, hard-to-digest mess . Instead, you need to read first and distil the information, then plan and execute the writing.
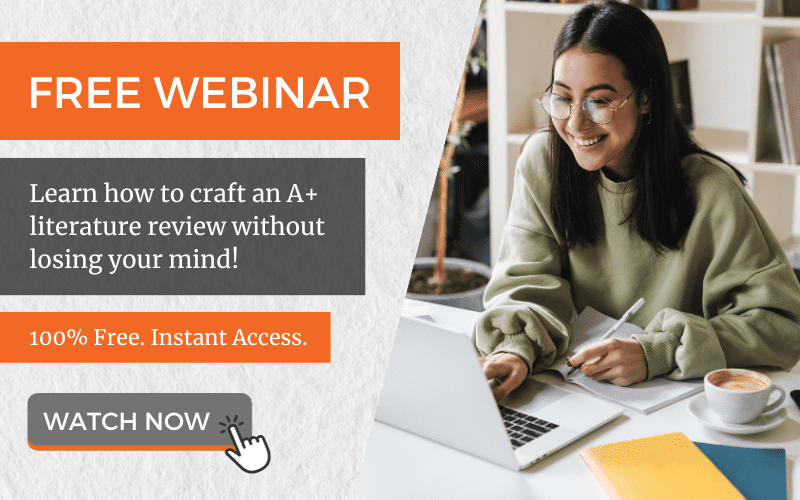
Step 1: Find the relevant literature
Naturally, the first step in the literature review journey is to hunt down the existing research that’s relevant to your topic. While you probably already have a decent base of this from your research proposal , you need to expand on this substantially in the dissertation or thesis itself.
Essentially, you need to be looking for any existing literature that potentially helps you answer your research question (or develop it, if that’s not yet pinned down). There are numerous ways to find relevant literature, but I’ll cover my top four tactics here. I’d suggest combining all four methods to ensure that nothing slips past you:
Method 1 – Google Scholar Scrubbing
Google’s academic search engine, Google Scholar , is a great starting point as it provides a good high-level view of the relevant journal articles for whatever keyword you throw at it. Most valuably, it tells you how many times each article has been cited, which gives you an idea of how credible (or at least, popular) it is. Some articles will be free to access, while others will require an account, which brings us to the next method.
Method 2 – University Database Scrounging
Generally, universities provide students with access to an online library, which provides access to many (but not all) of the major journals.
So, if you find an article using Google Scholar that requires paid access (which is quite likely), search for that article in your university’s database – if it’s listed there, you’ll have access. Note that, generally, the search engine capabilities of these databases are poor, so make sure you search for the exact article name, or you might not find it.
Method 3 – Journal Article Snowballing
At the end of every academic journal article, you’ll find a list of references. As with any academic writing, these references are the building blocks of the article, so if the article is relevant to your topic, there’s a good chance a portion of the referenced works will be too. Do a quick scan of the titles and see what seems relevant, then search for the relevant ones in your university’s database.
Method 4 – Dissertation Scavenging
Similar to Method 3 above, you can leverage other students’ dissertations. All you have to do is skim through literature review chapters of existing dissertations related to your topic and you’ll find a gold mine of potential literature. Usually, your university will provide you with access to previous students’ dissertations, but you can also find a much larger selection in the following databases:
- Open Access Theses & Dissertations
- Stanford SearchWorks
Keep in mind that dissertations and theses are not as academically sound as published, peer-reviewed journal articles (because they’re written by students, not professionals), so be sure to check the credibility of any sources you find using this method. You can do this by assessing the citation count of any given article in Google Scholar. If you need help with assessing the credibility of any article, or with finding relevant research in general, you can chat with one of our Research Specialists .
Alright – with a good base of literature firmly under your belt, it’s time to move onto the next step.
Need a helping hand?
Step 2: Log, catalogue and synthesise
Once you’ve built a little treasure trove of articles, it’s time to get reading and start digesting the information – what does it all mean?
While I present steps one and two (hunting and digesting) as sequential, in reality, it’s more of a back-and-forth tango – you’ll read a little , then have an idea, spot a new citation, or a new potential variable, and then go back to searching for articles. This is perfectly natural – through the reading process, your thoughts will develop , new avenues might crop up, and directional adjustments might arise. This is, after all, one of the main purposes of the literature review process (i.e. to familiarise yourself with the current state of research in your field).
As you’re working through your treasure chest, it’s essential that you simultaneously start organising the information. There are three aspects to this:
- Logging reference information
- Building an organised catalogue
- Distilling and synthesising the information
I’ll discuss each of these below:
2.1 – Log the reference information
As you read each article, you should add it to your reference management software. I usually recommend Mendeley for this purpose (see the Mendeley 101 video below), but you can use whichever software you’re comfortable with. Most importantly, make sure you load EVERY article you read into your reference manager, even if it doesn’t seem very relevant at the time.
2.2 – Build an organised catalogue
In the beginning, you might feel confident that you can remember who said what, where, and what their main arguments were. Trust me, you won’t. If you do a thorough review of the relevant literature (as you must!), you’re going to read many, many articles, and it’s simply impossible to remember who said what, when, and in what context . Also, without the bird’s eye view that a catalogue provides, you’ll miss connections between various articles, and have no view of how the research developed over time. Simply put, it’s essential to build your own catalogue of the literature.
I would suggest using Excel to build your catalogue, as it allows you to run filters, colour code and sort – all very useful when your list grows large (which it will). How you lay your spreadsheet out is up to you, but I’d suggest you have the following columns (at minimum):
- Author, date, title – Start with three columns containing this core information. This will make it easy for you to search for titles with certain words, order research by date, or group by author.
- Categories or keywords – You can either create multiple columns, one for each category/theme and then tick the relevant categories, or you can have one column with keywords.
- Key arguments/points – Use this column to succinctly convey the essence of the article, the key arguments and implications thereof for your research.
- Context – Note the socioeconomic context in which the research was undertaken. For example, US-based, respondents aged 25-35, lower- income, etc. This will be useful for making an argument about gaps in the research.
- Methodology – Note which methodology was used and why. Also, note any issues you feel arise due to the methodology. Again, you can use this to make an argument about gaps in the research.
- Quotations – Note down any quoteworthy lines you feel might be useful later.
- Notes – Make notes about anything not already covered. For example, linkages to or disagreements with other theories, questions raised but unanswered, shortcomings or limitations, and so forth.
If you’d like, you can try out our free catalog template here (see screenshot below).
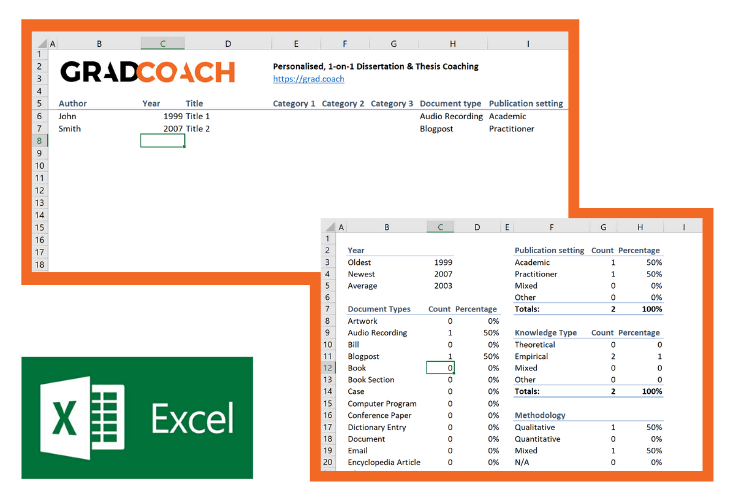
2.3 – Digest and synthesise
Most importantly, as you work through the literature and build your catalogue, you need to synthesise all the information in your own mind – how does it all fit together? Look for links between the various articles and try to develop a bigger picture view of the state of the research. Some important questions to ask yourself are:
- What answers does the existing research provide to my own research questions ?
- Which points do the researchers agree (and disagree) on?
- How has the research developed over time?
- Where do the gaps in the current research lie?
To help you develop a big-picture view and synthesise all the information, you might find mind mapping software such as Freemind useful. Alternatively, if you’re a fan of physical note-taking, investing in a large whiteboard might work for you.
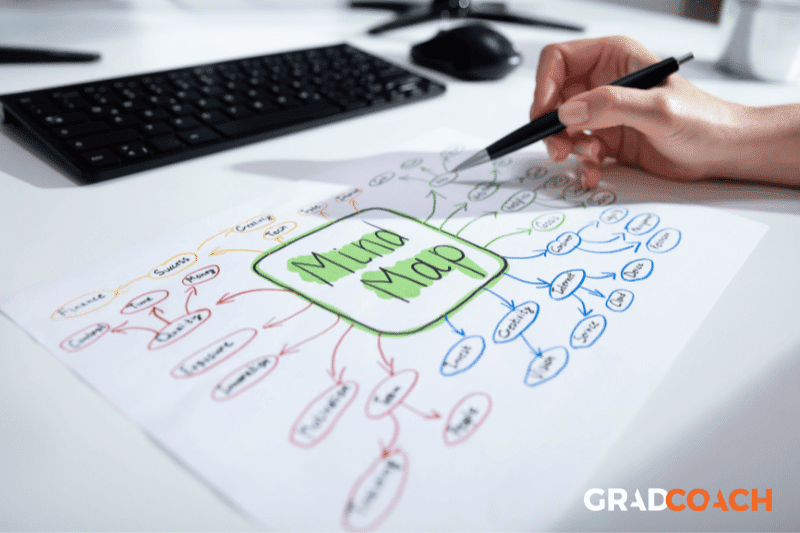
Step 3: Outline and write it up!
Once you’re satisfied that you have digested and distilled all the relevant literature in your mind, it’s time to put pen to paper (or rather, fingers to keyboard). There are two steps here – outlining and writing:
3.1 – Draw up your outline
Having spent so much time reading, it might be tempting to just start writing up without a clear structure in mind. However, it’s critically important to decide on your structure and develop a detailed outline before you write anything. Your literature review chapter needs to present a clear, logical and an easy to follow narrative – and that requires some planning. Don’t try to wing it!
Naturally, you won’t always follow the plan to the letter, but without a detailed outline, you’re more than likely going to end up with a disjointed pile of waffle , and then you’re going to spend a far greater amount of time re-writing, hacking and patching. The adage, “measure twice, cut once” is very suitable here.
In terms of structure, the first decision you’ll have to make is whether you’ll lay out your review thematically (into themes) or chronologically (by date/period). The right choice depends on your topic, research objectives and research questions, which we discuss in this article .
Once that’s decided, you need to draw up an outline of your entire chapter in bullet point format. Try to get as detailed as possible, so that you know exactly what you’ll cover where, how each section will connect to the next, and how your entire argument will develop throughout the chapter. Also, at this stage, it’s a good idea to allocate rough word count limits for each section, so that you can identify word count problems before you’ve spent weeks or months writing!
PS – check out our free literature review chapter template…
3.2 – Get writing
With a detailed outline at your side, it’s time to start writing up (finally!). At this stage, it’s common to feel a bit of writer’s block and find yourself procrastinating under the pressure of finally having to put something on paper. To help with this, remember that the objective of the first draft is not perfection – it’s simply to get your thoughts out of your head and onto paper, after which you can refine them. The structure might change a little, the word count allocations might shift and shuffle, and you might add or remove a section – that’s all okay. Don’t worry about all this on your first draft – just get your thoughts down on paper.
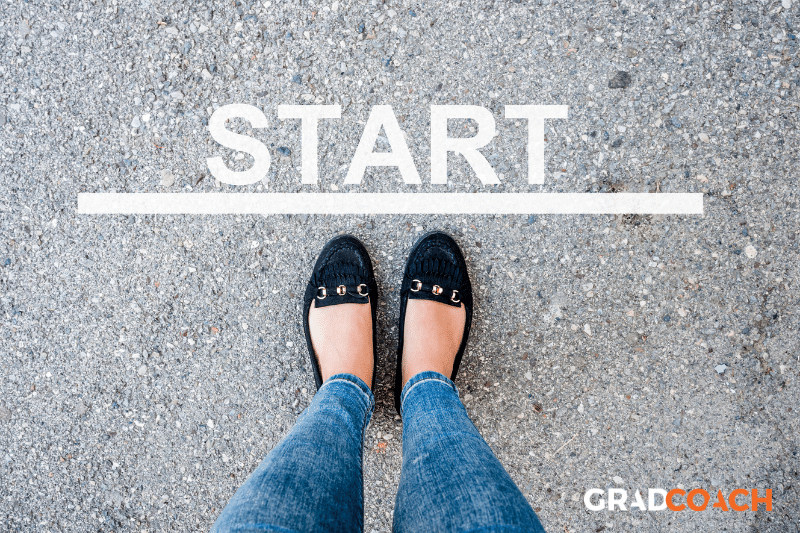
Once you’ve got a full first draft (however rough it may be), step away from it for a day or two (longer if you can) and then come back at it with fresh eyes. Pay particular attention to the flow and narrative – does it fall fit together and flow from one section to another smoothly? Now’s the time to try to improve the linkage from each section to the next, tighten up the writing to be more concise, trim down word count and sand it down into a more digestible read.
Once you’ve done that, give your writing to a friend or colleague who is not a subject matter expert and ask them if they understand the overall discussion. The best way to assess this is to ask them to explain the chapter back to you. This technique will give you a strong indication of which points were clearly communicated and which weren’t. If you’re working with Grad Coach, this is a good time to have your Research Specialist review your chapter.
Finally, tighten it up and send it off to your supervisor for comment. Some might argue that you should be sending your work to your supervisor sooner than this (indeed your university might formally require this), but in my experience, supervisors are extremely short on time (and often patience), so, the more refined your chapter is, the less time they’ll waste on addressing basic issues (which you know about already) and the more time they’ll spend on valuable feedback that will increase your mark-earning potential.
Literature Review Example
In the video below, we unpack an actual literature review so that you can see how all the core components come together in reality.
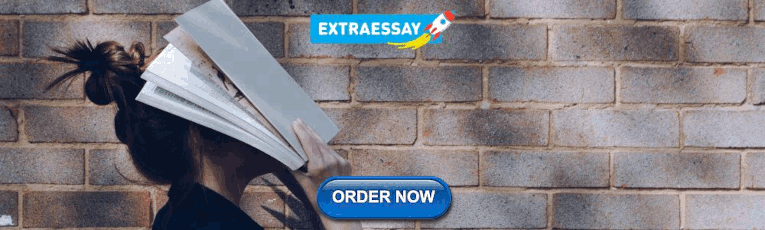
Let’s Recap
In this post, we’ve covered how to research and write up a high-quality literature review chapter. Let’s do a quick recap of the key takeaways:
- It is essential to understand the WHY of the literature review before you read or write anything. Make sure you understand the 4 core functions of the process.
- The first step is to hunt down the relevant literature . You can do this using Google Scholar, your university database, the snowballing technique and by reviewing other dissertations and theses.
- Next, you need to log all the articles in your reference manager , build your own catalogue of literature and synthesise all the research.
- Following that, you need to develop a detailed outline of your entire chapter – the more detail the better. Don’t start writing without a clear outline (on paper, not in your head!)
- Write up your first draft in rough form – don’t aim for perfection. Remember, done beats perfect.
- Refine your second draft and get a layman’s perspective on it . Then tighten it up and submit it to your supervisor.
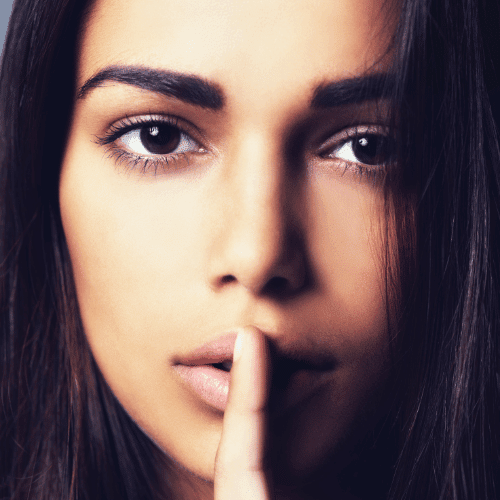
Psst… there’s more!
This post is an extract from our bestselling short course, Literature Review Bootcamp . If you want to work smart, you don't want to miss this .
You Might Also Like:
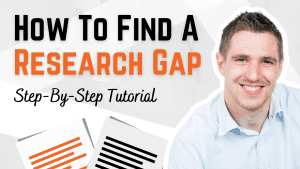
38 Comments
Thank you very much. This page is an eye opener and easy to comprehend.
This is awesome!
I wish I come across GradCoach earlier enough.
But all the same I’ll make use of this opportunity to the fullest.
Thank you for this good job.
Keep it up!
You’re welcome, Yinka. Thank you for the kind words. All the best writing your literature review.
Thank you for a very useful literature review session. Although I am doing most of the steps…it being my first masters an Mphil is a self study and one not sure you are on the right track. I have an amazing supervisor but one also knows they are super busy. So not wanting to bother on the minutae. Thank you.
You’re most welcome, Renee. Good luck with your literature review 🙂
This has been really helpful. Will make full use of it. 🙂
Thank you Gradcoach.
Really agreed. Admirable effort
thank you for this beautiful well explained recap.
Thank you so much for your guide of video and other instructions for the dissertation writing.
It is instrumental. It encouraged me to write a dissertation now.
Thank you the video was great – from someone that knows nothing thankyou
an amazing and very constructive way of presetting a topic, very useful, thanks for the effort,
It is timely
It is very good video of guidance for writing a research proposal and a dissertation. Since I have been watching and reading instructions, I have started my research proposal to write. I appreciate to Mr Jansen hugely.
I learn a lot from your videos. Very comprehensive and detailed.
Thank you for sharing your knowledge. As a research student, you learn better with your learning tips in research
I was really stuck in reading and gathering information but after watching these things are cleared thanks, it is so helpful.
Really helpful, Thank you for the effort in showing such information
This is super helpful thank you very much.
Thank you for this whole literature writing review.You have simplified the process.
I’m so glad I found GradCoach. Excellent information, Clear explanation, and Easy to follow, Many thanks Derek!
You’re welcome, Maithe. Good luck writing your literature review 🙂
Thank you Coach, you have greatly enriched and improved my knowledge
Great piece, so enriching and it is going to help me a great lot in my project and thesis, thanks so much
This is THE BEST site for ANYONE doing a masters or doctorate! Thank you for the sound advice and templates. You rock!
Thanks, Stephanie 🙂
This is mind blowing, the detailed explanation and simplicity is perfect.
I am doing two papers on my final year thesis, and I must stay I feel very confident to face both headlong after reading this article.
thank you so much.
if anyone is to get a paper done on time and in the best way possible, GRADCOACH is certainly the go to area!
This is very good video which is well explained with detailed explanation
Thank you excellent piece of work and great mentoring
Thanks, it was useful
Thank you very much. the video and the information were very helpful.
Good morning scholar. I’m delighted coming to know you even before the commencement of my dissertation which hopefully is expected in not more than six months from now. I would love to engage my study under your guidance from the beginning to the end. I love to know how to do good job
Thank you so much Derek for such useful information on writing up a good literature review. I am at a stage where I need to start writing my one. My proposal was accepted late last year but I honestly did not know where to start
Like the name of your YouTube implies you are GRAD (great,resource person, about dissertation). In short you are smart enough in coaching research work.
This is a very well thought out webpage. Very informative and a great read.
Very timely.
I appreciate.
Very comprehensive and eye opener for me as beginner in postgraduate study. Well explained and easy to understand. Appreciate and good reference in guiding me in my research journey. Thank you
Thank you. I requested to download the free literature review template, however, your website wouldn’t allow me to complete the request or complete a download. May I request that you email me the free template? Thank you.
Submit a Comment Cancel reply
Your email address will not be published. Required fields are marked *
Save my name, email, and website in this browser for the next time I comment.
- Print Friendly
What is a Systematic Literature Review?
A systematic literature review (SLR) is an independent academic method that aims to identify and evaluate all relevant literature on a topic in order to derive conclusions about the question under consideration. "Systematic reviews are undertaken to clarify the state of existing research and the implications that should be drawn from this." (Feak & Swales, 2009, p. 3) An SLR can demonstrate the current state of research on a topic, while identifying gaps and areas requiring further research with regard to a given research question. A formal methodological approach is pursued in order to reduce distortions caused by an overly restrictive selection of the available literature and to increase the reliability of the literature selected (Tranfield, Denyer & Smart, 2003). A special aspect in this regard is the fact that a research objective is defined for the search itself and the criteria for determining what is to be included and excluded are defined prior to conducting the search. The search is mainly performed in electronic literature databases (such as Business Source Complete or Web of Science), but also includes manual searches (reviews of reference lists in relevant sources) and the identification of literature not yet published in order to obtain a comprehensive overview of a research topic.
An SLR protocol documents all the information gathered and the steps taken as part of an SLR in order to make the selection process transparent and reproducible. The PRISMA flow-diagram support you in making the selection process visible.
In an ideal scenario, experts from the respective research discipline, as well as experts working in the relevant field and in libraries, should be involved in setting the search terms . As a rule, the literature is selected by two or more reviewers working independently of one another. Both measures serve the purpose of increasing the objectivity of the literature selection. An SLR must, then, be more than merely a summary of a topic (Briner & Denyer, 2012). As such, it also distinguishes itself from “ordinary” surveys of the available literature. The following table shows the differences between an SLR and an “ordinary” literature review.
- Charts of BSWL workshop (pdf, 2.88 MB)
- Listen to the interview (mp4, 12.35 MB)
Differences to "common" literature reviews
Characteristic | SLR | common literature overview |
---|---|---|
Independent research method | yes | no |
Explicit formulation of the search objectives | yes | no |
Identification of all publications on a topic | yes | no |
Defined criteria for inclusion and exclusion of publications | yes | no |
Description of search procedure | yes | no |
Literature selection and information extraction by several persons | yes | no |
Transparent quality evaluation of publications | yes | no |
What are the objectives of SLRs?
- Avoidance of research redundancies despite a growing amount of publications
- Identification of research areas, gaps and methods
- Input for evidence-based management, which allows to base management decisions on scientific methods and findings
- Identification of links between different areas of researc
Process steps of an SLR
A SLR has several process steps which are defined differently in the literature (Fink 2014, p. 4; Guba 2008, Transfield et al. 2003). We distinguish the following steps which are adapted to the economics and management research area:
1. Defining research questions
Briner & Denyer (2009, p. 347ff.) have developed the CIMO scheme to establish clearly formulated and answerable research questions in the field of economic sciences:
C – CONTEXT: Which individuals, relationships, institutional frameworks and systems are being investigated?
I – Intervention: The effects of which event, action or activity are being investigated?
M – Mechanisms: Which mechanisms can explain the relationship between interventions and results? Under what conditions do these mechanisms take effect?
O – Outcomes: What are the effects of the intervention? How are the results measured? What are intended and unintended effects?
The objective of the systematic literature review is used to formulate research questions such as “How can a project team be led effectively?”. Since there are numerous interpretations and constructs for “effective”, “leadership” and “project team”, these terms must be particularized.
With the aid of the scheme, the following concrete research questions can be derived with regard to this example:
Under what conditions (C) does leadership style (I) influence the performance of project teams (O)?
Which constructs have an effect upon the influence of leadership style (I) on a project team’s performance (O)?
Research questions do not necessarily need to follow the CIMO scheme, but they should:
- ... be formulated in a clear, focused and comprehensible manner and be answerable;
- ... have been determined prior to carrying out the SLR;
- ... consist of general and specific questions.
As early as this stage, the criteria for inclusion and exclusion are also defined. The selection of the criteria must be well-grounded. This may include conceptual factors such as a geographical or temporal restrictions, congruent definitions of constructs, as well as quality criteria (journal impact factor > x).
2. Selecting databases and other research sources
The selection of sources must be described and explained in detail. The aim is to find a balance between the relevance of the sources (content-related fit) and the scope of the sources.
In the field of economic sciences, there are a number of literature databases that can be searched as part of an SLR. Some examples in this regard are:
- Business Source Complete
- ProQuest One Business
- EconBiz
Our video " Selecting the right databases " explains how to find relevant databases for your topic.
Literature databases are an important source of research for SLRs, as they can minimize distortions caused by an individual literature selection (selection bias), while offering advantages for a systematic search due to their data structure. The aim is to find all database entries on a topic and thus keep the retrieval bias low (tutorial on retrieval bias ). Besides articles from scientific journals, it is important to inlcude working papers, conference proceedings, etc to reduce the publication bias ( tutorial on publication bias ).
Our online self-study course " Searching economic databases " explains step 2 und 3.
3. Defining search terms
Once the literature databases and other research sources have been selected, search terms are defined. For this purpose, the research topic/questions is/are divided into blocks of terms of equal ranking. This approach is called the block-building method (Guba 2008, p. 63). The so-called document-term matrix, which lists topic blocks and search terms according to a scheme, is helpful in this regard. The aim is to identify as many different synonyms as possible for the partial terms. A precisely formulated research question facilitates the identification of relevant search terms. In addition, keywords from particularly relevant articles support the formulation of search terms.
A document-term matrix for the topic “The influence of management style on the performance of project teams” is shown in this example .
Identification of headwords and keywords
When setting search terms, a distinction must be made between subject headings and keywords, both of which are described below:
- appear in the title, abstract and/or text
- sometimes specified by the author, but in most cases automatically generated
- non-standardized
- different spellings and forms (singular/plural) must be searched separately
Subject headings
- describe the content
- are generated by an editorial team
- are listed in a standardized list (thesaurus)
- may comprise various keywords
- include different spellings
- database-specific
Subject headings are a standardized list of words that are generated by the specialists in charge of some databases. This so-called index of subject headings (thesaurus) helps searchers find relevant articles, since the headwords indicate the content of a publication. By contrast, an ordinary keyword search does not necessarily result in a content-related fit, since the database also displays articles in which, for example, a word appears once in the abstract, even though the article’s content does not cover the topic.
Nevertheless, searches using both headwords and keywords should be conducted, since some articles may not yet have been assigned headwords, or errors may have occurred during the assignment of headwords.
To add headwords to your search in the Business Source Complete database, please select the Thesaurus tab at the top. Here you can find headwords in a new search field and integrate them into your search query. In the search history, headwords are marked with the addition DE (descriptor).
The EconBiz database of the German National Library of Economics (ZBW – Leibniz Information Centre for Economics), which also contains German-language literature, has created its own index of subject headings with the STW Thesaurus for Economics . Headwords are integrated into the search by being used in the search query.
Since the indexes of subject headings divide terms into synonyms, generic terms and sub-aspects, they facilitate the creation of a document-term matrix. For this purpose it is advisable to specify in the document-term matrix the origin of the search terms (STW Thesaurus for Economics, Business Source Complete, etc.).
Searching in literature databases
Once the document-term matrix has been defined, the search in literature databases begins. It is recommended to enter each word of the document-term matrix individually into the database in order to obtain a good overview of the number of hits per word. Finally, all the words contained in a block of terms are linked with the Boolean operator OR and thereby a union of all the words is formed. The latter are then linked with each other using the Boolean operator AND. In doing so, each block should be added individually in order to see to what degree the number of hits decreases.
Since the search query must be set up separately for each database, tools such as LitSonar have been developed to enable a systematic search across different databases. LitSonar was created by Professor Dr. Ali Sunyaev (Institute of Applied Informatics and Formal Description Methods – AIFB) at the Karlsruhe Institute of Technology.
Advanced search
Certain database-specific commands can be used to refine a search, for example, by taking variable word endings into account (*) or specifying the distance between two words, etc. Our overview shows the most important search commands for our top databases.
Additional searches in sources other than literature databases
In addition to literature databases, other sources should also be searched. Fink (2014, p. 27) lists the following reasons for this:
- the topic is new and not yet included in indexes of subject headings;
- search terms are not used congruently in articles because uniform definitions do not exist;
- some studies are still in the process of being published, or have been completed, but not published.
Therefore, further search strategies are manual search, bibliographic analysis, personal contacts and academic networks (Briner & Denyer, p. 349). Manual search means that you go through the source information of relevant articles and supplement your hit list accordingly. In addition, you should conduct a targeted search for so-called gray literature, that is, literature not distributed via the book trade, such as working papers from specialist areas and conference reports. By including different types of publications, the so-called publication bias (DBWM video “Understanding publication bias” ) – that is, distortions due to exclusive use of articles from peer-reviewed journals – should be kept to a minimum.
The PRESS-Checklist can support you to check the correctness of your search terms.
4. Merging hits from different databases
In principle, large amounts of data can be easily collected, structured and sorted with data processing programs such as Excel. Another option is to use reference management programs such as EndNote, Citavi or Zotero. The Saxon State and University Library Dresden (SLUB Dresden) provides an overview of current reference management programs . Software for qualitative data analysis such as NVivo is equally suited for data processing. A comprehensive overview of the features of different tools that support the SLR process can be found in Bandara et al. (2015).
Our online-self study course "Managing literature with Citavi" shows you how to use the reference management software Citavi.
When conducting an SLR, you should specify for each hit the database from which it originates and the date on which the query was made. In addition, you should always indicate how many hits you have identified in the various databases or, for example, by manual search.
Exporting data from literature databases
Exporting from literature databases is very easy. In Business Source Complete , you must first click on the “Share” button in the hit list, then “Email a link to download exported results” at the very bottom and then select the appropriate format for the respective literature program.
Exporting data from the literature database EconBiz is somewhat more complex. Here you must first create a marked list and then select each hit individually and add it to the marked list. Afterwards, articles on the list can be exported.
After merging all hits from the various databases, duplicate entries (duplicates) are deleted.
5. Applying inclusion and exclusion criteria
All publications are evaluated in the literature management program applying the previously defined criteria for inclusion and exclusion. Only those sources that survive this selection process will subsequently be analyzed. The review process and inclusion criteria should be tested with a small sample and adjustments made if necessary before applying it to all articles. In the ideal case, even this selection would be carried out by more than one person, with each working independently of one another. It needs to be made clear how discrepancies between reviewers are dealt with.
The review of the criteria for inclusion and exclusion is primarily based on the title, abstract and subject headings in the databases, as well as on the keywords provided by the authors of a publication in the first step. In a second step the whole article / source will be read.
You can create tag words for the inclusion and exclusion in your literature management tool to keep an overview.
In addition to the common literature management tools, you can also use software tools that have been developed to support SLRs. The central library of the university in Zurich has published an overview and evaluation of different tools based on a survey among researchers. --> View SLR tools
The selection process needs to be made transparent. The PRISMA flow diagram supports the visualization of the number of included / excluded studies.
Forward and backward search
Should it become apparent that the number of sources found is relatively small, or if you wish to proceed with particular thoroughness, a forward-and-backward search based on the sources found is recommendable (Webster & Watson 2002, p. xvi). A backward search means going through the bibliographies of the sources found. A forward search, by contrast, identifies articles that have cited the relevant publications. The Web of Science and Scopus databases can be used to perform citation analyses.
6. Perform the review
As the next step, the remaining titles are analyzed as to their content by reading them several times in full. Information is extracted according to defined criteria and the quality of the publications is evaluated. If the data extraction is carried out by more than one person, a training ensures that there will be no differences between the reviewers.
Depending on the research questions there exist diffent methods for data abstraction (content analysis, concept matrix etc.). A so-called concept matrix can be used to structure the content of information (Webster & Watson 2002, p. xvii). The image to the right gives an example of a concept matrix according to Becker (2014).
Particularly in the field of economic sciences, the evaluation of a study’s quality cannot be performed according to a generally valid scheme, such as those existing in the field of medicine, for instance. Quality assessment therefore depends largely on the research questions.
Based on the findings of individual studies, a meta-level is then applied to try to understand what similarities and differences exist between the publications, what research gaps exist, etc. This may also result in the development of a theoretical model or reference framework.
Example concept matrix (Becker 2013) on the topic Business Process Management
Article | Pattern | Configuration | Similarities |
---|---|---|---|
Thom (2008) | x | ||
Yang (2009) | x | x | |
Rosa (2009) | x | x |
7. Synthesizing results
Once the review has been conducted, the results must be compiled and, on the basis of these, conclusions derived with regard to the research question (Fink 2014, p. 199ff.). This includes, for example, the following aspects:
- historical development of topics (histogram, time series: when, and how frequently, did publications on the research topic appear?);
- overview of journals, authors or specialist disciplines dealing with the topic;
- comparison of applied statistical methods;
- topics covered by research;
- identifying research gaps;
- developing a reference framework;
- developing constructs;
- performing a meta-analysis: comparison of the correlations of the results of different empirical studies (see for example Fink 2014, p. 203 on conducting meta-analyses)
Publications about the method
Bandara, W., Furtmueller, E., Miskon, S., Gorbacheva, E., & Beekhuyzen, J. (2015). Achieving Rigor in Literature Reviews: Insights from Qualitative Data Analysis and Tool-Support. Communications of the Association for Information Systems . 34(8), 154-204.
Booth, A., Papaioannou, D., and Sutton, A. (2012) Systematic approaches to a successful literature review. London: Sage.
Briner, R. B., & Denyer, D. (2012). Systematic Review and Evidence Synthesis as a Practice and Scholarship Tool. In Rousseau, D. M. (Hrsg.), The Oxford Handbook of Evidenence Based Management . (S. 112-129). Oxford: Oxford University Press.
Durach, C. F., Wieland, A., & Machuca, Jose A. D. (2015). Antecedents and dimensions of supply chain robustness: a systematic literature review . International Journal of Physical Distribution & Logistic Management , 46 (1/2), 118-137. doi: https://doi.org/10.1108/IJPDLM-05-2013-0133
Feak, C. B., & Swales, J. M. (2009). Telling a Research Story: Writing a Literature Review. English in Today's Research World 2. Ann Arbor: University of Michigan Press. doi: 10.3998/mpub.309338
Fink, A. (2014). Conducting Research Literature Reviews: From the Internet to Paper (4. Aufl.). Los Angeles, London, New Delhi, Singapore, Washington DC: Sage Publication.
Fisch, C., & Block, J. (2018). Six tips for your (systematic) literature review in business and management research. Management Review Quarterly, 68, 103–106 (2018). doi.org/10.1007/s11301-018-0142-x
Guba, B. (2008). Systematische Literaturrecherche. Wiener Medizinische Wochenschrift , 158 (1-2), S. 62-69. doi: doi.org/10.1007/s10354-007-0500-0 Hart, C. Doing a literature review: releasing the social science research imagination. London: Sage.
Jesson, J. K., Metheson, L. & Lacey, F. (2011). Doing your Literature Review - traditional and Systematic Techniques . Los Angeles, London, New Delhi, Singapore, Washington DC: Sage Publication.
Page MJ, McKenzie JE, Bossuyt PM, Boutron I, Hoffmann TC, Mulrow CD, et al. The PRISMA 2020 statement: an updated guideline for reporting systematic reviews. BMJ 2021;372:n71. doi: 10.1136/bmj.n71.
Petticrew, M. and Roberts, H. (2006). Systematic Reviews in the Social Sciences: A Practical Guide . Oxford:Blackwell. Ridley, D. (2012). The literature review: A step-by-step guide . 2nd edn. London: Sage.
Chang, W. and Taylor, S.A. (2016), The Effectiveness of Customer Participation in New Product Development: A Meta-Analysis, Journal of Marketing , American Marketing Association, Los Angeles, CA, Vol. 80 No. 1, pp. 47–64.
Tranfield, D., Denyer, D. & Smart, P. (2003). Towards a methodology for developing evidence-informed management knowledge by means of systematic review. British Journal of Management , 14 (3), S. 207-222. doi: https://doi.org/10.1111/1467-8551.00375
Webster, J., & Watson, R. T. (2002). Analyzing the Past to Prepare for the Future: Writing a Literature Review. Management Information Systems Quarterly , 26(2), xiii-xxiii. http://www.jstor.org/stable/4132319
Durach, C. F., Wieland, A. & Machuca, Jose. A. D. (2015). Antecedents and dimensions of supply chain robustness: a systematic literature review. International Journal of Physical Distribution & Logistics Management, 45(1/2), 118 – 137.
What is particularly good about this example is that search terms were defined by a number of experts and the review was conducted by three researchers working independently of one another. Furthermore, the search terms used have been very well extracted and the procedure of the literature selection very well described.
On the downside, the restriction to English-language literature brings the language bias into play, even though the authors consider it to be insignificant for the subject area.
Bos-Nehles, A., Renkema, M. & Janssen, M. (2017). HRM and innovative work behaviour: a systematic literature review. Personnel Review, 46(7), pp. 1228-1253
- Only very specific keywords used
- No precise information on how the review process was carried out (who reviewed articles?)
- Only journals with impact factor (publication bias)
Jia, F., Orzes, G., Sartor, M. & Nassimbeni, G. (2017). Global sourcing strategy and structure: towards a conceptual framework. International Journal of Operations & Production Management, 37(7), 840-864
- Research questions are explicitly presented
- Search string very detailed
- Exact description of the review process
- 2 persons conducted the review independently of each other
Franziska Klatt
+49 30 314-29778
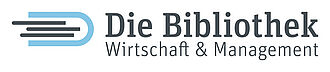
Privacy notice: The TU Berlin offers a chat information service. If you enable it, your IP address and chat messages will be transmitted to external EU servers. more information
The chat is currently unavailable.
Please use our alternative contact options.

- University of Texas Libraries
Literature Reviews
- What is a literature review?
- Steps in the Literature Review Process
- Define your research question
- Determine inclusion and exclusion criteria
- Choose databases and search
- Review Results
- Synthesize Results
- Analyze Results
- Librarian Support
- Artificial Intelligence (AI) Tools
What is a Literature Review?
A literature or narrative review is a comprehensive review and analysis of the published literature on a specific topic or research question. The literature that is reviewed contains: books, articles, academic articles, conference proceedings, association papers, and dissertations. It contains the most pertinent studies and points to important past and current research and practices. It provides background and context, and shows how your research will contribute to the field.
A literature review should:
- Provide a comprehensive and updated review of the literature;
- Explain why this review has taken place;
- Articulate a position or hypothesis;
- Acknowledge and account for conflicting and corroborating points of view
From S age Research Methods
Purpose of a Literature Review
A literature review can be written as an introduction to a study to:
- Demonstrate how a study fills a gap in research
- Compare a study with other research that's been done
Or it can be a separate work (a research article on its own) which:
- Organizes or describes a topic
- Describes variables within a particular issue/problem
Limitations of a Literature Review
Some of the limitations of a literature review are:
- It's a snapshot in time. Unlike other reviews, this one has beginning, a middle and an end. There may be future developments that could make your work less relevant.
- It may be too focused. Some niche studies may miss the bigger picture.
- It can be difficult to be comprehensive. There is no way to make sure all the literature on a topic was considered.
- It is easy to be biased if you stick to top tier journals. There may be other places where people are publishing exemplary research. Look to open access publications and conferences to reflect a more inclusive collection. Also, make sure to include opposing views (and not just supporting evidence).
Source: Grant, Maria J., and Andrew Booth. “A Typology of Reviews: An Analysis of 14 Review Types and Associated Methodologies.” Health Information & Libraries Journal, vol. 26, no. 2, June 2009, pp. 91–108. Wiley Online Library, doi:10.1111/j.1471-1842.2009.00848.x.
Meryl Brodsky : Communication and Information Studies
Hannah Chapman Tripp : Biology, Neuroscience
Carolyn Cunningham : Human Development & Family Sciences, Psychology, Sociology
Larayne Dallas : Engineering
Janelle Hedstrom : Special Education, Curriculum & Instruction, Ed Leadership & Policy
Susan Macicak : Linguistics
Imelda Vetter : Dell Medical School
For help in other subject areas, please see the guide to library specialists by subject .
Periodically, UT Libraries runs a workshop covering the basics and library support for literature reviews. While we try to offer these once per academic year, we find providing the recording to be helpful to community members who have missed the session. Following is the most recent recording of the workshop, Conducting a Literature Review. To view the recording, a UT login is required.
- October 26, 2022 recording
- Last Updated: Jun 18, 2024 1:00 PM
- URL: https://guides.lib.utexas.edu/literaturereviews
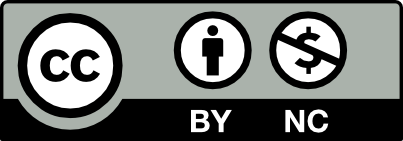
Thank you for visiting nature.com. You are using a browser version with limited support for CSS. To obtain the best experience, we recommend you use a more up to date browser (or turn off compatibility mode in Internet Explorer). In the meantime, to ensure continued support, we are displaying the site without styles and JavaScript.
- View all journals
- Explore content
- About the journal
- Publish with us
- Sign up for alerts
- Open access
- Published: 22 June 2024
Enhancing automated vehicle identification by integrating YOLO v8 and OCR techniques for high-precision license plate detection and recognition
- Hanae Moussaoui 1 ,
- Nabil El Akkad 1 ,
- Mohamed Benslimane 2 ,
- Walid El-Shafai 3 , 4 ,
- Abdullah Baihan 5 ,
- Chaminda Hewage 6 &
- Rajkumar Singh Rathore 6
Scientific Reports volume 14 , Article number: 14389 ( 2024 ) Cite this article
37 Accesses
Metrics details
- Computer science
- Information technology
Vehicle identification systems are vital components that enable many aspects of contemporary life, such as safety, trade, transit, and law enforcement. They improve community and individual well-being by increasing vehicle management, security, and transparency. These tasks entail locating and extracting license plates from images or video frames using computer vision and machine learning techniques, followed by recognizing the letters or digits on the plates. This paper proposes a new license plate detection and recognition method based on the deep learning YOLO v8 method, image processing techniques, and the OCR technique for text recognition. For this, the first step was the dataset creation, when gathering 270 images from the internet. Afterward, CVAT (Computer Vision Annotation Tool) was used to annotate the dataset, which is an open-source software platform made to make computer vision tasks easier to annotate and label images and videos. Subsequently, the newly released Yolo version, the Yolo v8, has been employed to detect the number plate area in the input image. Subsequently, after extracting the plate the k-means clustering algorithm, the thresholding techniques, and the opening morphological operation were used to enhance the image and make the characters in the license plate clearer before using OCR. The next step in this process is using the OCR technique to extract the characters. Eventually, a text file containing only the character reflecting the vehicle's country is generated. To ameliorate the efficiency of the proposed approach, several metrics were employed, namely precision, recall, F1-Score, and CLA. In addition, a comparison of the proposed method with existing techniques in the literature has been given. The suggested method obtained convincing results in both detection as well as recognition by obtaining an accuracy of 99% in detection and 98% in character recognition.
Similar content being viewed by others
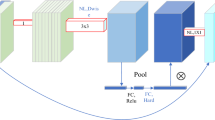
Real-time detection of road manhole covers with a deep learning model

Potholes and traffic signs detection by classifier with vision transformers
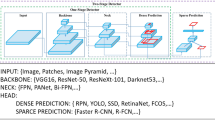
Evaluating YOLO architectures for detecting road killed endangered Brazilian animals
Introduction.
Usually, license plate detection starts with object detection, in which a model is trained to locate license plates in an image or video frame. YOLO (You Only Look Once) 1 and Faster R-CNN are popular object identification frameworks frequently utilized for this task. Afterward, images or frames are often preprocessed to boost contrast, emphasize features, and reduce noise to facilitate the detection model's ability to locate the plates. Moreover, detected zones are frequently filtered according to size, shape, or other factors to eliminate false positives. Afterward, edge and contour detection steps highlight edges and identify contours present in the image by employing several image processing techniques. Another important step in the license plate detection process is the regions of interest (ROIs) extraction, where the main purpose is to identify any potential region that might contain the license plate zone. The current process uses character segmentation and recognition techniques for separating and recognizing the present characters in the extracted license plate. Eventually, the number plate is refined using post-processing methods. Figure 1 below shows the license plate detection steps 2 .

License plate detection flowchart.
Subsequently, identifying the characters on the license plate comes next after it has been recognized, this is referred to as optical character recognition (OCR) 3 for license plates. The characters on the plate are split from the identified plate region to separate individual characters or digits. nonetheless, the fragmented characters are recognized using an OCR engine. One popular open-source OCR engine for text recognition is Tesseract OCR. Deep learning techniques and specialized OCR models 4 can be trained on license plate datasets to increase recognition accuracy. Lastly, post-processing may be applied to the identified characters. This could include fixing mistakes, removing incorrect plates, and ensuring the output satisfies predetermined standards (such as license plate format). Figure 2 below shows the license plate recognition system, which contains the main blocks that should be added to the previous license plate detection process to recognize the characters in the number plate.

License plate recognition flowchart.
Moreover, a large dataset of annotations on license plate images is needed to train the detection and identification models. To ensure the models are robust, these datasets could contain a variety of license plate styles, fonts, and backgrounds. Though the recognition model is trained to recognize characters, the detection model is trained to identify regions on license plates. Afterward, a test dataset is used to assess the system's accuracy and efficiency to ensure it satisfies the necessary specifications and can handle real-world situations. Eventually, a more extensive system, like parking management, security, or traffic management systems, incorporates the detection and recognition components. However, it's important to remember that different license plate techniques, lighting, and environmental circumstances might make detecting and recognizing license plates difficult.
For this reason, creating precise and dependable systems requires robust algorithms and properly annotated information. When implementing such systems, privacy and legal issues also need to be considered. To overcome these difficulties, ALPR systems usually employ deep learning models, image preprocessing, image enhancement, and data augmentation. Furthermore, ongoing research and development are necessary to increase the precision and resilience of license plate detection and identification systems. Accordingly, the proposed method aims at three essential axes: detection, recognition, and assigning each license plate to its original country. The vision of this method is promising and has long-term benefits in many areas, such as security. On the other hand, it's easy to implement and gives satisfactory results.
The rest of the article will be organized as follows, starting with a related work section, where the most relevant previous works in the literature have been gathered. Additionally, a detailed clarification of the proposed method is given in the third part of the article, where the methods used to create the proposed technique were explained point by point, as well as the evaluation metrics and the obtained results. Eventually, a conclusion and future work section is given.
Related works
In computer vision and image processing, license plate detection and recognition (LPR) 5 aims to extract license plates from images or video streams, recognize the characters on the plate, and then process the extracted information. Several methods and approaches have been developed via substantial research and development in this field to increase efficiency and accuracy. Therefore, in the literature, two main approaches exist for license plate detection (LPD) which are the traditional techniques, and the deep learning methods The first approach uses contour analysis, thresholding, and edge detection to find the license plate region in an image. As for the second technique, CNNs 6 remains a popular choice for detecting license plates. Popular real-time license plate identification designs are SSD which refers to Single Shot MultiBox Detector 7 and YOLO. On the other hand, license plate recognition uses optical character recognition that is used to identify the characters on a license plate once the region containing the plate has been identified. Commonly used tools include Tesseract OCR and commercial programs like ABBYY FineReader. Or, it employs deep learning-based optical character recognition such as CNNs and RNNs 8 , that have been used to ameliorate character recognition accuracy. Moreover, several methods have been proposed in the literature, here are some of them:
The authors of the paper (9) 9 presented a method for independently predicting locations; by examining context information, the system produces smoother and more precise detection. It obtained a Hmean of 0.73, a recall of 0.71, and a precision of 0.74.
It is shown in paper (10) 10 that by obtaining high-quality visual data, a pipeline based on convolutional neural networks can enhance text identification and recognition performance. This study employed a pre-trained ResNet-50 network to extract low-level visual features from ImageNet and SynthText.New and improved ReLU layer (new.i.ReLU) blocks are also part of the proposed structure. These blocks have good text component identification capabilities even on curved surfaces and a large receptive field. A new, improved inception layer can produce more effectively a broadly varying-sized text than a linear set of convolution layers.
In the article (11) 11 , the authors provide ReLaText, a novel approach for text detection. It works by redefining text identification as a visual link detection issue. Using a "link" relationship, they first tackle the difficult text-line grouping problem to illustrate the efficacy of this unique formulation.
An improved YOLOv3 network-based scene text detection algorithm is used in the research (12) 12 on scene text detection. First of all, since YOLOv3 relies on the network of the Darknet53 backbone, which has several layers and is unable to train rapidly for a single detection target, this study proposes a technique by replacing the Darknet53 with the Darknet19. Second, the multi-scale detection of the original network was retained, and three different-sized anchors were employed to forecast the bounding boxes.
In the paper (13) 13 , the authors of this work present TextField, a novel text detector for recognizing usual scene texts. Particularly, when each text point should have an orientation field moving away from the closest text boundary. A fully convolutional neural network is used to learn this direction field, which is then represented by a 2D vector image. Unlike typical segmentation-based approaches, it stores the direction information required to discriminate between surrounding text instances as well as the binary text mask.
The authors of the research (14) 14 offer an accurate text region representation method for text localization in scenes. Initially, text suggestions are extracted using a text region proposal network from an input image. A refinement network is then used to verify and enhance these suggestions.
In paper (15) 15 , the authors directly train a cross-modal similitude between each text instance from the natural images and the query text. In particular, they developed an end-to-end trainable network tuned for both scene text detection and cross-modal similarity learning.
The study in the paper (16) 16 presents the Pixel Aggregation Network that the authors refer to as an accurate and efficient arbitrary-shaped text detector. It consists of a learnable post-processing component and a segmentation head with a low computing cost.
In the study (17) 17 , the authors developed a system to test the security of Hindi CAPTCHAS. For this, k-nearest neighbors, support vector machines, and random forest classifiers are used to crack ten distinct colored CAPTCHAs. Two-color schemes have a 90% breaking rate, whereas multi-color schemes have a 93% breaking rate.
In the paper (18) 18 , The authors proposed a technique to evaluate the security of CAPTCHAs based on Devanagari scripts. They selected five distinct monochrome and five grayscale CAPTCHAs for security testing. They produced six different kinds of features for these segmented characters and obtained segmentation rates ranging from 88.13 to 97.6% using these approaches. They employed three classifiers for comparative studies in their categorization process, using k-nearest Neighbor (k-NN), Support Vector Machine (SVM), and Random Forest. They attained a breaking rate of 73–93% for grayscale schemes and 66–85% for monochrome designs.
In the study (19) 19 , the authors suggest a brand-new module Multi-Domain Character Distance Perception to create a position embedding that is both semantically and visually connected. Using the cross-attention method, MDCDP queries both visual and semantic information using the location embedding. They created CDistNet, which guides an increasingly accurate distance modeling by stacking several MDCDPs. Table 1 summarizes the methods in the related works section.
Research gaps
Recent advancements in deep learning, machine learning, and computer vision techniques have contributed to enormous advances in license plate detection and recognition research. There are still some research gaps in this field, such as variable weather, occlusions (partially visible plates due to obstacles or barriers), and lighting conditions. Additionally, current algorithms frequently concentrate on standard license plate formats in particular areas, including alphanumeric plates. Moreover, while specific algorithms perform well when applied to novel datasets or real-world circumstances, their accuracy may decrease when used on other datasets or in highly regulated contexts. Furthermore, efficient algorithms with real-time processing capabilities are required, especially for platforms with limited resources, such as mobile devices and embedded systems. Therefore, by filling these research gaps, license plate detection and recognition systems will function better and be more reliable. They will also be widely used in various applications, such as parking and traffic management, law enforcement, and vehicle identification.
The proposed method
This section discusses the proposed method's central parts, starting with describing the created dataset. Afterward, Yolo v8 detects the license plate in the input image. The detected plate will then be resized and enhanced by using some of the image processing techniques that play the preprocessing stage, such as k-means clustering, thresholding, and morphological operations. This step is crucial when achieving good accuracy in the character recognition part, especially with the noise that may occur during edge detection. Subsequently, the OCR algorithm will be applied to recognize the characters in the image. Afterward, a text file is generated containing only the essential part of the plate that will indicate the car's original country. The suggested method is shown in detail in Figure 3 .
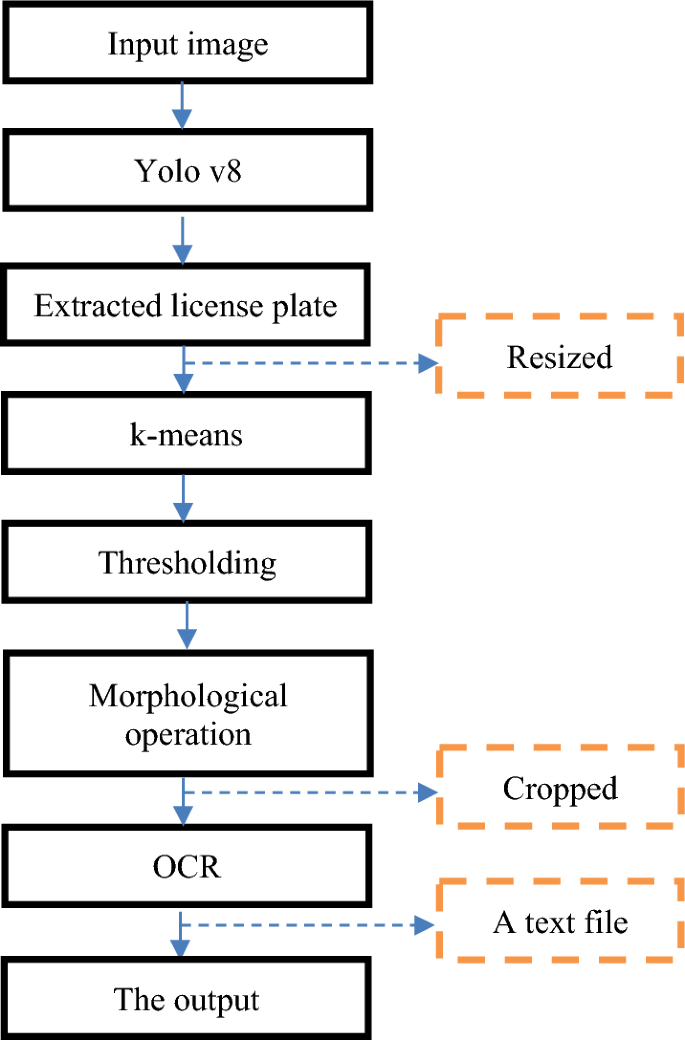
The proposed method flowchart.
To train Yolo v8, a new dataset was created by gathering 270 images from the internet; these images are publicly available and can be downloaded without restriction. Furthermore, this dataset contains cars taken from different angles and under various lighting conditions.
Moreover, to guarantee that a dataset is representative, diversified, and appropriate for the intended purpose, selecting images for it requires careful consideration of several factors, including diversity, by collecting images with different light conditions, scenes, and viewpoints. On the other hand, the created dataset includes images with various instances of the target object, the license plate. Another important criterion is the ethical considerations when selecting and downloading only images that respect people's privacy.
However, these particular requirements may change based on the project's objectives. Eventually, the data was annotated accurately.
Afterward, the CVAT tool was used to annotate the data and generate the annotations. Moreover, the dataset was divided into three categories: train, validation, and test. Figure 4 demonstrates the annotated dataset. Furthermore, at the end of the manuscript, a declaration statement containing the public sources from which the images were downloaded is given.
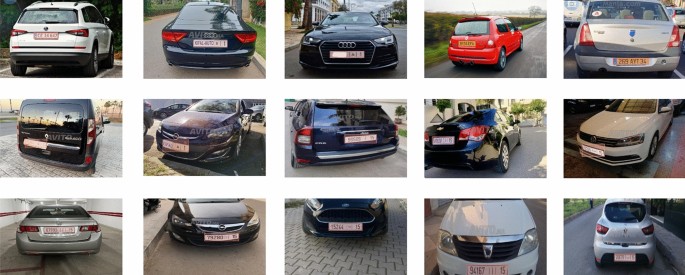
The annotated dataset.
As mentioned earlier, the CVAT annotation tool was chosen to annotate the data used for this study. Here’s a slight clarification about the CVAT annotation process and the steps to follow:
Step 1 Create a free account on the CVAT platform; https://www.cvat.ai/
Step 2 Create a new task
Step 3 Enter the necessary label(s)
Step 4 Upload and submit the data
Step 5 Click on the “Job” section and start annotate
Step 6 When done labeling, export the labeled dataset to a specified format
The inter-annotator agreement
In image processing, inter-annotator agreement describes the level of consistency or agreement across many human annotators that independently label or annotate the same collection of images. This is especially crucial for tasks involving subjective interpretation, like segmentation, object detection, and image classification. Moreover, several annotators may work together to classify images in image processing to provide a ground truth dataset that can be used to train and assess machine learning models. The inter-annotator agreement helps evaluate the consistency and dependability of annotations made by several human annotators. Inter-annotator agreement is typically measured using several metrics, such as Cohen's Kappa coefficient, Fleiss' Kappa, Jaccard index, and dice coefficient. In this study, the annotation process was conducted by two authors (two annotators) using the same annotation tool mentioned earlier.
Moreover, since only one class label is needed in this study, referred to as the license plate label, it was unsuitable to use Cohen's Kappa Coefficient, which requires two or more class labels. Additionally, it needed to be better to implement Fleiss' Kappa, which requires more than two annotators. Instead, the Jaccard index, as well as the Dice coefficient, were used to measure the inter-annotator agreement between the two labeled sets of images given by the two annotators. Therefore, in this case, the Jaccard index determines the IoU by dividing the union of these regions by the intersection of the regions where both annotators label the license plate. To indicate agreement, the overlap between the places where annotators located license plates will be measured by the IoU metric. On the other hand, the Dice Coefficient can be computed similarly to the Jaccard Index by dividing the total size of each zone by twice the intersection of the areas where both annotators label the license plate. Between the license plate regions that the annotators have determined, the Dice Coefficient measures the agreement in terms of overlap.
The obtained values in Table 2 show that the annotators have given a similar annotation which has afterward influenced positively the accuracy of the model that has been used for the license plate detection.
Yolo v8 is Ultralytics' most recent iteration of YOLO. With new features and enhancements for improved performance, flexibility, and efficiency, YOLOv8 20 is a cutting-edge model that builds on the success of earlier iterations. YOLOv8 supports all everyday visual AI tasks, such as tracking, segmentation, posture estimation, detection, and classification. Because of its adaptability, users can use YOLOv8's features in multiple applications and domains. "You Only Look Once" or "YOLO" 21 is a well-known and significant object recognition framework in computer vision and deep learning. Yolo was developed to increase object identification in real-time application speed and accuracy. It deviates from conventional object detection techniques by framing object identification as a regression problem and making predictions for object bounding boxes and class labels in a single forward run of a neural network. The following are some of the main ideas and characteristics of the YOLO algorithm 22 , 23 :
Single-pass Detection: YOLO predicts bounding boxes and class probabilities for objects by processing the entire image or video frame in a single pass. Several additional object-detecting systems, on the other hand, employ multi-stage procedures.
Grid-based Approach: YOLO creates a grid out of the input image, and each grid cell has to guess what kind of object is inside it. YOLO forecasts bounding boxes and associated class probabilities for every cell.
Bounding Box Predictions: Yolo predicts the bounding box coordinates surrounding observed items. It forecasts the bounding boxes' height (h), width (w), and center coordinates (x, y). The forecasts are based on the grid cell's dimensions.
Class Predictions: YOLO further makes predictions about the likelihood that each object it detects will fall into a particular class, such as "car," "person," or "dog." This enables YOLO to categorize items and detect them.
Non-Maximum Suppression: YOLO uses non-maximum suppression (NMS) after formulating predictions to eliminate low-confidence or duplicate detections. The final set of detected objects is refined with the aid of NMS.
The YOLO algorithm applies a set of steps to identify and find objects in images or video frames. The following Figure 5 shows the main steps in the YOLO algorithm:
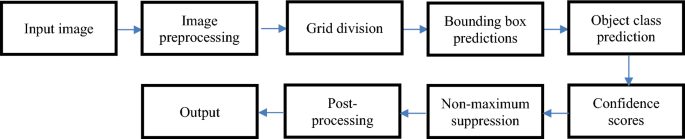
Yolo algorithm process.
Moreover, Yolo's widespread appeal in the computer vision world can be attributed to its exceptional accuracy in real-time object recognition. Researchers and engineers are still working on more improvements and iterations of the YOLO algorithm. Figure 6 below shows the obtained results after applying Yolo v8 to the created dataset:
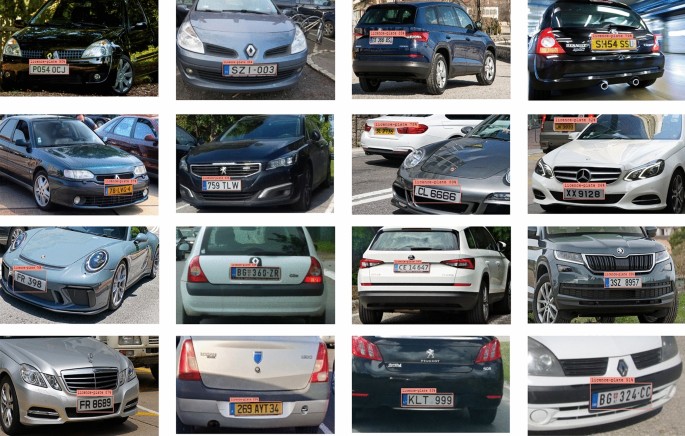
The detected license plates using Yolo v8.
Bounding box predictions are crucial to the success of YOLO in a variety of applications, from autonomous navigation to surveillance, as they enable precise localization, size estimate, as well as immediate evaluation for several objects in images. Moreover, these arguments demonstrate why YOLO v8-based license plate identification systems require bounding box predictions. All of these processes’ verification, tracking, and alphanumeric recognition are made easier by YOLO's ability to locate and identify license plates in a variety of settings and configurations. These processes are essential for automated systems used in traffic control, law enforcement, and vehicle-related services. YOLOv8 offers enhancements to the developer experience as well as architecture. In contrast to its predecessor, YOLOv8 includes:
A brand-new system for anchor-free detection.
Modifications to the model's convolutional blocks.
The mosaic augmentation that was used throughout the training was disabled before the last ten epochs.
Moreover, YOLOv8 includes modifications to enhance the model's development experience. To begin with, the model is now provided in the form of a library that was usually added to the Python code.
The reason behind choosing the Yolo model over the other deep learning techniques is that Yolo is widely known for its ability to process information in real-time. Moreover, it is appropriate for applications where low latency is essential, like license plate recognition in traffic surveillance systems, because it can process images as well as videos quickly. Furthermore, YOLO uses a one-pass architecture, the entire image is processed by the neural network during a single forward pass. This contrasts with several object detection techniques that make use of numerous passes and region proposal networks (RPNs).
On the other hand, and because of its excellent generalization capabilities across several object categories, YOLO is a good choice for a variety of detection tasks, including the identification of license plates. Its architecture can efficiently handle a wide range of object sizes and aspect ratios, also, it successfully strikes a balance between precision and speed. Eventually, for every bounding box prediction, YOLO assigns a score that indicates the probability that the bounding box has an object of interest. This improves the model's detection of meaningful objects and assists in filtering out false positives.
Preprocessing
To increase the recognition system's accuracy and resilience, preprocessing the identified license plate area for character recognition is essential. For this reason, and by using k-means clustering and thresholding, the image is first divided into separate areas according to the intensity of its pixels, in this stage, pixels with comparable brightness are clustered together to help distinguish the characters from the background. Thereafter, a threshold is applied to binarize the image, where character pixels with intensity values exceeding the threshold are categorized as foreground, and background pixels are identified as pixels having intensity values below the threshold. Eventually, a morphological technique, which is the opening operation, is applied to enhance the binary image's quality and eliminate minor noise. Moreover, to eliminate unnecessary background and to concentrate just on the area with the characters, the identified license plate region was cropped.
K-means algorithm
K -means clustering divides data points into K unique, non-overlapping subgroups or clusters 24 , 25 , 26 . Their centroids, or centers, are what characterize these clusters. To find patterns and put related data points together, the technique is frequently used in data analysis, data mining, image segmentation 27 , 28 , 29 , and associated domains. The following Figure 7 presents the required steps that the K -means clustering algorithm uses:
Step 1 Select K initial centroids first. These centroids can be chosen at random from the data points or positioned with more consideration for context.
Step 2 Determine the closest centroid for every data point by applying a distance measure, most commonly the Euclidean distance. In this stage, K clusters are created, and every data point is assigned to the cluster that has the closest centroid.
Step 3 Determine the new centroids for every cluster by averaging all of the data points that belong to that cluster. These new centroids represent the core of each cluster.
Step 4 Assess whether there have been any notable changes to the centroids from the prior iteration. Subsequently, moving to the next stage if the centroids have stabilized and the algorithm has converged.
Step 5 The k cluster centroids and the assignment of every data point to a cluster are the ultimate results of the K -means algorithm. This information can be used to evaluate the data and comprehend the clusters' structure.

K-means clustering process.
It's crucial to remember that the original centroids chosen can have an impact on how well the clustering outcome turns out. It is usual practice to run K -means numerous times with different initializations and choose the optimal outcome according to a criterion like minimizing the total within-cluster variance 30 . This is because random initialization can occasionally result in inadequate solutions.
The k -means clustering mathematical formula is presented below:
J: The objective function
N: The number of the case
K: The number of the cluster
\({c}_{j}\) : The cluster’s j centroid.
\({x}_{i}^{(j)}\) : The I case.
k -means clustering has been used as a part of the whole process of the proposed method for three main reasons:
To distinguish the foreground (the license plate characters) from the background in the image.
Regions related to characters can be identified and segmented based on color using k-means clustering. This helps get the image ready for the next stages of character recognition.
Pixels in grayscale images can be grouped according to intensity using k -means clustering. This can help emphasize areas of the image that will probably contain characters or increase the contrast in the image itself.
For this research, several values for the number of clusters k were tested, with k =2 being selected upon achieving better results.
- Thresholding
Thresholding is a widely used image processing technique 31 in binary images that is employed to separate important objects or features. The threshold value, which is a specific range of values or intensity level, must be determined to convert a grayscale image into a binary format, in which the value of a pixel above the threshold is assigned to one (white). and pixel values that are below the threshold will be set to zero (black). Here's a quick rundown of how thresholding works:
Step 1 The input image is usually converted to grayscale first. This streamlines the thresholding procedure by emphasizing intensity values over color.
Step 2 Selecting a Threshold. Selecting a Limit. It’s required to decide on an appropriate threshold value. The ideal threshold depends on the particular application and the characteristics of the input image. Popular strategies for threshold selection include Otsu's method, which uses image histograms to set thresholds automatically; manual selection; and adaptive thresholding, in which the threshold travels throughout the image to account for local variations in lighting and contrast.
Step 3 Operating Threshold. Once chosen, each pixel in the grayscale image is compared to the threshold value. If the intensity of the pixel is greater than or equal to the threshold, it is set to white; if not, it is turned to black. This results in a binary image where objects of interest are represented in white on a black backdrop.
Figure 8 below presents some of the popular thresholding techniques 32
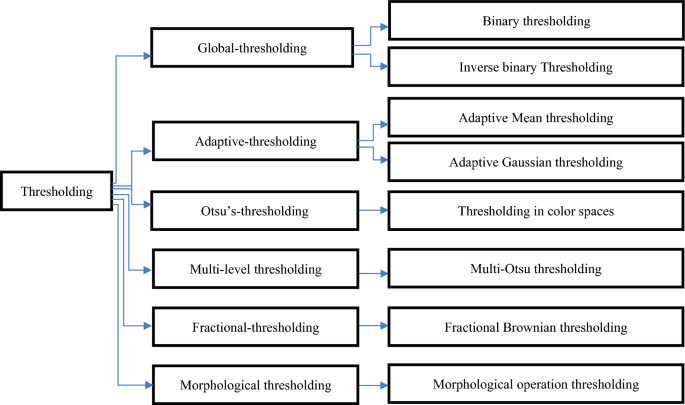
Thresholding algorithm types.
The effectiveness of thresholding depends on several factors, including the input image's quality, the threshold value chosen, and the distinctive characteristics of the background and objects in the image. It can be necessary to carefully consider and test several thresholding strategies and threshold values before deciding on the optimal one for a given task.
The intensity levels of the pixels in an image are compared to a predetermined threshold value in the mathematical method for thresholding.
Let’s assume that t is the threshold value, and intensity (x, y) is the intensity value of a specific pixel at a given position (x, y) in the input image. The mathematical formula of thresholding 33 can be written as below:
Binary thresholding
Inverse binary thresholding
Adaptive mean thresholding
Where t (x, y) is the mean of the values of the pixels around (x,y)
Adaptive Gaussian thresholding
where t (x, y) is the weighted sum of the values of the pixels surrounding (x, y)
For this case study, and after trying several thresholding techniques, the most satisfactory results were achieved when applying the “To zero” thresholding type, more precisely when setting the threshold value to 180. Moreover, in the case of license plate detection and recognition, thresholding remains a crucial method since it streamlines the image processing process, making it easier to extract and identify the license plate's characters. Therefore, Through the enhancement of contrast, reduction of noise, and facilitation of the extraction of important information from the image, thresholding streamlines the image processing involved in license plate identification systems. Therefore, it acts as a prelude to bettering the precision and effectiveness of later processing phases, such as (OCR) and character segmentation.
Morphological operations
Morphological operations 34 are image processing techniques used to manipulate the shape and structure of objects in the image. These methods are very helpful for tasks like image segmentation, noise reduction, and feature extraction. Morphological methods usually use binary images or grayscale. Furthermore, the two most popular morphological processes dilation and erosion can be utilized singly or in combination to achieve a range of image-processing goals. 35
Dilation: In binary images, this is a morphological operation that enlarges the white regions or objects. All of the pixels that a tiny matrix or kernel, which serves as a structural element, scans across the image are colored white. The center of each structuring element is placed over a white pixel in the image at each position where it overlaps with it. Dilation can also be used to join dissimilar objects, thicken things, and fill in gaps. It can be utilized to enhance the notable attributes.
Erosion is the opposite of dilation. It results in the white portions in a binary image shrinking or eroding. Erosion uses a structural element identical to dilation, but instead of changing the selected pixel to white if there is overlap, it only does so if all the pixels below it are white. If there is a black pixel below the structuring element, the target pixel is changed to black. Erosion can be used to remove little artifacts and disconnect related components, and thin objects.
Opening: An opening operation is carried out by combining erosion and dilatation. It assists in removing little details and noises from an image whilst preserving the overall proportions and form of larger objects.
Closing: A closing operation is the reverse of an opening activity. Erosion is the last step after dilatation. It is effective in filling up small gaps in items and connecting nearby components.
Morphological gradient: This technique separates dilation from erosion in the given image. It highlights the edges of the objects in the image.
Top Hat and Black Hat: These techniques involve subtracting the result of a closing or opening operation from the original image. The top-hat procedure draws attention to the lighter portions while the black-hat technique highlights the darker areas.
Hit-or-miss transform: It’s a process that is used to recognize specific patterns or forms in binary images. This technique requires identifying similarities among the two predefined structuring elements in the image: one for the pattern in question and one for its counterpart.
Therefore, the opening technique was chosen for this case study to remove any potential noise from the finished product while maintaining the overall form of the characters. Moreover, artificial intelligence and image analysis frequently use morphological techniques for text extraction processes, image segmentation, object recognition, and other purposes. They can increase image quality, reduce noise in photos, and enhance features for further analysis. The size and form of the structuring element are determined by the specific image processing project at hand as well as the features of the image.
Several document types, including PDF files, digital documents, and electronic photographs, can be transformed into editable and searchable data by using the OCR technology 36 . It is possible to extract, edit, and search for text in these computer files using OCR software and algorithms that evaluate the text and convert it into understandable machine code. The main parts and techniques of OCR are as follows 37 :
Step 1 Since an image is typically used as the given document for OCR, this step happens first. This entails making modifications to the picture, removing noise, and binarizing it (turning it into black and white). These procedures enhance character recognition input quality.
Step 2 OCR often needs to identify the portions of an image containing text. Text localization is the process of identifying the places where text is present.
Step 3 With multiple text lines or paragraphs, OCR systems need to divide the text into individual characters or words. Text segmentation refers to breaking down a text into its constituent parts.
Step 4 OCR systems often require to identification of the text-containing regions of an image. The process of text localization involves identifying the places where text is present.
Step 5 Fundamentally, each segmented character or word is recognized using optical character recognition (OCR), which transforms it into machine-readable text. Character recognition can be accomplished by a variety of techniques, including pattern matching, neural networks, and feature extraction methods.
Step 6 OCR software may utilize methods for post-processing after character recognition to improve error correction and text detection precision. This may include dictionary-based corrections, spell-checking, and context analysis.
Step 7 After identification, the text is made accessible in a way that machines can read such as plain text, searchable PDFs, or other kinds of documents. This allows users to edit, search, and save the content digitally.
Improvements in deep learning and machine learning have led to considerable achievements in OCR technology over time. High-accuracy OCR can be achieved by modern systems, even when dealing with intricate fonts, languages, and document layouts. There is open-source and commercial OCR software available, and OCR APIs are frequently integrated into different services and applications for automated data extraction and document processing.
To convert the characters from the image, different OCR techniques are provided, such as Tesseract OCR, Paddle OCR, Easy OCR, and Keras OCR. Moreover, the EasyOcr technique was chosen for three main reasons.
Quick and effective: EasyOCR can process a lot of images in real time because it is designed to be quick.
Simple to use: Python programs can readily incorporate EasyOCR into their code thanks to its straightforward interface.
Good accuracy: Across a range of OCR criteria, EasyOCR has attained high accuracy.
Yolo v8 and OCR integration
Depending on the use case and requirements, several approaches may be used to integrate Yolov8 along with OCR techniques in particular settings. YOLOv8 can be applied to real-time object detection. For this, the model must be trained using pertinent data to this field to incorporate this capability into a given space. For instance, the model needs to be trained on images of equipment and machinery unique to the manufacturing sector when wanting to recognize items in a manufacturing setting. Subsequently, text can be extracted from objects in an image using optical character recognition (OCR). Applications including document scanning, traffic sign translation, and license plate recognition can all benefit from this technology. Consequently, the results of applying OCR and YOLOv8 to an image can be combined in many ways based on the requirements. For instance, when recognizing license plates, the characters found by OCR can be linked to the cars found by YOLOv8 to determine who the owners of the cars are. On the other hand, in images and video streams, YOLO can recognize traffic indicators like stop signs, speed restriction signs, and traffic signals. Subsequently, OCR methods might be utilized to identify text or symbols on the signs, offering extra context for self-driving cars. Moreover, YOLO v8 and OCR techniques can be found in surveillance footage, where YOLO v8 can identify objects of interest like people, cars, or suspicious objects. Eventually, building intelligent systems that can detect and identify objects and text in images is made achievable by combining these techniques. This opens up a variety of applications in various industries, including document processing, retail, transportation, and surveillance. Typically, it involves preprocessing images, using YOLO v8 for object recognition, OCR technique to extract text, integrating the results, and optimizing performance.
Experiments and results
Several evaluation metrics were employed, which are listed below, to evaluate the results of the suggested approach 38 . Therefore, when it comes to license plate detection, the terms "false positives" and "false negatives" have particular meanings associated with the detection system's accuracy. A false positive happens when the system misidentifies an area of an image as having a license plate when it doesn't. Stated differently, false positives are the results of the system mistakenly identifying a region that is not a license plate for a license plate. Furthermore, when a system is unable to identify the actual license plate area in an image, it results in a false negative for license plate detection. As a result, false negatives are the result of the algorithm missing or failing to detect a region that has a license plate. Additionally, testing the license plate detection on the created dataset yielded a higher recall and precision (99%), with only 2 false positives and no false negatives recorded. Figure 9 illustrates the 2 false positives obtained.
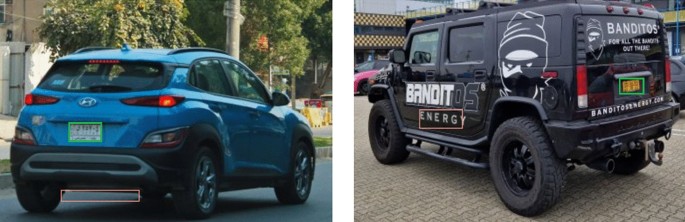
Examples of false positives. Red and green, respectively, outline the expected position as well as the ground truth.
Precision is often used to describe the degree of certainty in machine learning, statistics, and various domain labeling systems or prediction models. It measures the degree to which the system's positive predictions and actual positive events agree. The following formula is used to determine precision when dealing with binary classification, or a simple "yes/no" prediction:
The incidents that were correctly predicted to be positive are the real positives. Furthermore, cases mistakenly classified as positive are known as false positives. The obtained findings are presented in Table 3 below:
Figure 10 below shows the graphic representation of Table 3 :
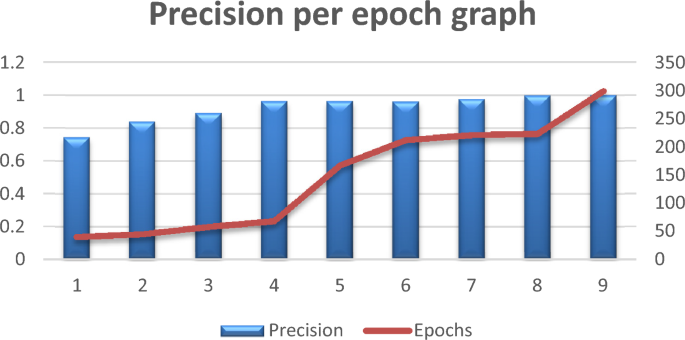
Precision per epoch graph.
From Table 3 and Figure 10 above, it’s noticeable that the precision has a consistent upward trajectory over all epochs and increases continuously. As training continues on the created dataset, it shows that the model is improving in accurately detecting positive cases. This indicates that the model is learning to produce more accurate positive predictions, which is generally a good indication.
Recall, also known as sensitivity or true positive rate, represents a metric used in machine learning and statistics to evaluate a classification model's performance, particularly in binary classification settings. The model's recall measures its ability to recognize each relevant instance in the dataset. The mathematical formula of the recall metric can be written as follows:
The obtained results are given in Table 4 below:
Figure 11 below shows the graphic representation of Table 4 :
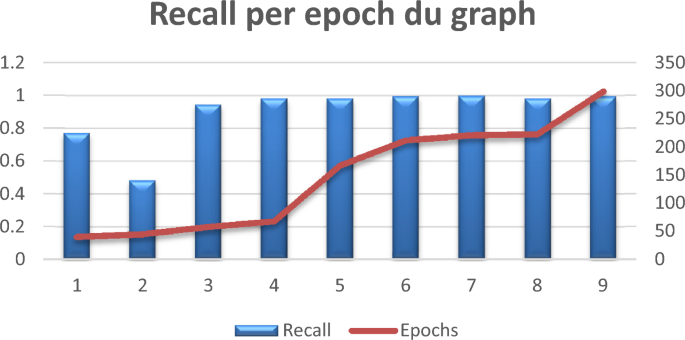
Recall per epoch graph.
In Table 4 and Fig. 11 , a high recall per epoch is noticeable which indicates that the model's ability to distinguish true positive examples from all of the real positive instances in the dataset is either becoming better or changing over training, which means that the model is getting more sensitive to positive occurrences in the data, which is generally a good indication.
F1-score is a commonly used metric in computer learning and statistics that combines recall and precision into an individual number to provide a fair assessment of a model's performance, especially in binary classification problems. The F1-score mathematical formula can be written as follows:
The obtained results are shown in Table 5 below:
Figure 12 below shows the graphic representation of Table 5 :
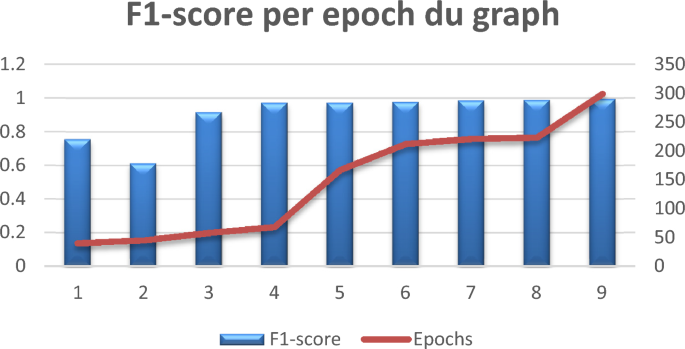
F1-score per epoch graph.
In Table 5 and Fig. 12 , a high F1-score per epoch is noticeable, which means that the model is hitting a good balance between recall and precision. The F1-score is a statistic that takes into account both false positives and false negatives, as it is a harmonic average of precision and recall. it means that as training goes on, the model gets better at correctly classifying both positive and negative instances. This shows that the model has improved the trade-off between recall and precision.
One commonly used metric to evaluate the overall accuracy 39 of a predictive model is the Character-Level Accuracy. It calculates the proportion of each occurrence in the dataset that could have been predicted with accuracy. The mathematical formula of accuracy can be written as follows:
In Table 6 below, the obtained accuracy for character segmentation is displayed:
From the obtained results, it’s seen that the proposed method gives satisfactory results in both detection and recognition of the text in the license plates when achieving an accuracy of 98% in the recognition and 99% in detection. The hybridization of Yolo v8 and the used image processing methods wasn’t arbitrary, these techniques were picked up carefully to have better results even in difficult scenarios where the lightning is bad or the angle at which the image was taken varies. Moreover, to have these results, many other techniques were tested before finding the suitable ones. Furthermore, a comparison with other methods that are given in Table 7 has proved the efficiency of the proposed method.
In paper (1) 40 , the authors used the mask region convolutional neural networks (mask R-CNN) to detect the license plate. afterward, to segment the characters from the detected license plate, they used the Mask R-CNN-based method to classify characters and non-characters. In the paper (2) 41 the authors used a hierarchical Convolutional Neural Network (CNN). The main idea is to use two passes of the same CNN to identify the license plate area. Afterward, a second CNN will be used to recognize the characters. In the paper (3) 42 , the authors proposed a system for detecting and recognizing license plates. For this, they pre-processed the input image using the Median filter in addition to histogram equalization. Afterward, they used Sobel edge detection to detect the license plate as well as labeling the obtained images and separate each object. Subsequently, to segment the characters they used the thresholding technique in addition to extracting the connected components. Finally, they employed the BPNN architecture to recognize the segmented characters.
Figure 13 below is a graphic representation of Table 7 .
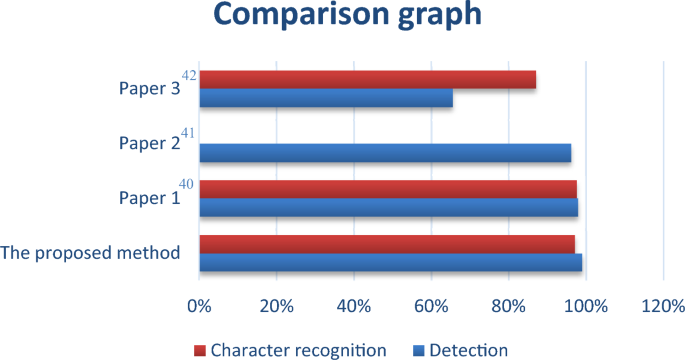
Graphical presentation of the comparison of the proposed method with other techniques.
From the graph above, it’s noticeable that the proposed method outperforms the existing methods for both detection and character recognition.
License plate extraction and recognition is a very promising field of research; many applications tend to implement it for either security or surveillance. However, like any other method, some situations where the proposed technique fell short of expectations were faced, like in the case where images represent low resolution, excessive noise, inadequate lighting, or motion blur. As a result, finding and precisely reading the license plate characters has become difficult. On the other hand, license plates on certain cars might not follow the norms, whether it comes to size, form, or arrangement. These differences may cause the extraction algorithm to become confused, producing unreliable results. Additionally, parts of the license plate may sometimes be obscured by glare from sunshine or reflections from bright surfaces, making it difficult for the recognition algorithm to recognize the characters accurately.
To guarantee reliable performance, a combination of strategies and technologies were employed to address issues such as changing lighting or image quality. To get beyond these challenges, the characters were segmented using the thresholding technique, taking into account changes in brightness in various areas of the image. Additionally, to help differentiate the plate zone from the background by clustering pixels with similar color values, K-means clustering is also used to group pixels within the plate area based on color or intensity similarity. This helps isolate characters from the background. The suggested method's robustness and accuracy were improved by adding morphological processes, especially in situations where the illumination, quality of the image, and character styles varied. Furthermore, touching characters can be separated, or gaps between characters can be closed using operations like opening and closing.
Conclusion and future work
In this paper, a novel technique for detection and recognition has been proposed, where license plates are used as a use case. The proposed method is a hybridization of deep learning by using the Yolo v8 technique for object detection. For this, a new dataset of 270 images was created, and that was annotated using the CVAT tool. Subsequently, after applying the Yolo v8 method, a bunch of machine learning techniques were used to enhance the extracted license plate. Afterward, the final part of the proposed technique was to identify the country of the car by detecting the character in the license plate that refers to its country. Accordingly, the obtained results were very promising and satisfactory.
Moreover, this technique was tested and verified on different illumination conditions, as well as at different angles at which the image was taken; on the other hand, the suggested method aims to increase accuracy, speed, and adaptability to a variety of real-world scenarios. Therefore, in the upcoming research, more reliable, accurate, and efficient systems will be created with a wide range of applications in security, surveillance, transportation, and other fields. We are planning to develop this technique to be applied to different types of license plates, including plates with different symbols, shapes, or colors. Moreover, future work will address more challenging situations, including images taken from various distances, challenging angles, differing illumination conditions, and occlusion.
Ethics approval
All authors are contributing and accepting to submit the current work.
Consent to participate
Consent to publish.
All authors are accepting to submit and publish the submitted work.
Data availability
The data that supports this study is free and the images were downloaded from the following resources that are available in the public domains: [ https://www.avito.ma/ , https://www.caradisiac.com/ , and https://platesmania.com/ ]. All data are available upon request from the corresponding author.
Code availability
The code used in this study can be accessed at the following GitHub link: https://github.com/HanaeMoussaoui/License-plate-detection-and-recognition/tree/main .
Long, X., Deng, K., Wang, G., Zhang, Y., Dang, Q., Gao, Y. & Wen, S. PP-YOLO: An effective and efficient implementation of object detector. arXiv preprint arXiv:2007.12099 . (2020).
Qamar, S., Öberg, R., Malyshev, D. & Andersson, M. A hybrid CNN-Random Forest algorithm for bacterial spore segmentation and classification in TEM images. Sci. Rep. 13 (1), 18758 (2023).
Article CAS PubMed PubMed Central Google Scholar
Memon, J., Sami, M., Khan, R. A. & Uddin, M. Handwritten optical character recognition (OCR): A comprehensive systematic literature review (SLR). IEEE access 8 , 142642–142668 (2020).
Article Google Scholar
Nguyen, T. T. H., Jatowt, A., Coustaty, M. & Doucet, A. Survey of post-OCR processing approaches. ACM Comput. Surveys (CSUR) 54 (6), 1–37 (2021).
Selmi, Z., Halima, M. B., Pal, U. & Alimi, M. A. DELP-DAR system for license plate detection and recognition. Pattern Recogn. Lett. 129 , 213–223 (2020).
Kaur, H., Bansal, S., Kumar, M., Mittal, A. & Kumar, K. Worddeepnet: handwritten gurumukhi word recognition using convolutional neural network. Multimedia Tools Appl. 82 (30), 46763–46788 (2023).
Kaur, H. et al. Bagging: An ensemble approach for recognition of handwritten place names in gurumukhi script. ACM Trans. Asian Low-Res. Lang. Inf. Process. 22 (7), 1–25 (2023).
Moussaoui, H., Benslimane, M. & El Akkad, N. Image segmentation approach based on hybridization between K-means and mask R-CNN. In WITS 2020: Proceedings of the 6th International Conference on Wireless Technologies, Embedded, and Intelligent Systems (pp. 821–830). Springer Singapore. (2022)
Naiemi, F., Ghods, V. & Khalesi, H. A novel pipeline framework for multi oriented scene text image detection and recognition. Expert Syst. Appl. 170 , 114549 (2021).
Ma, C., Sun, L., Zhong, Z. & Huo, Q. ReLaText: Exploiting visual relationships for arbitrary-shaped scene text detection with graph convolutional networks. Pattern Recognition 111 , 107684 (2021).
Wang, H. & Zhang, Z. Text detection algorithm based on improved YOLOv3. In 2019 IEEE 9th International Conference on Electronics Information and Emergency Communication (ICEIEC) (pp. 147–150). IEEE. (2019).
Xu, Y. et al. Textfield: Learning a deep direction field for irregular scene text detection. IEEE Trans. Image Process. 28 (11), 5566–5579 (2019).
Article MathSciNet PubMed Google Scholar
Wang, X., Jiang, Y., Luo, Z., Liu, C. L., Choi, H. & Kim, S. Arbitrary shape scene text detection with adaptive text region representation. In Proceedings of the IEEE/CVF conference on computer vision and pattern recognition (pp. 6449–6458). (2019).
Wang, H., Bai, X., Yang, M., Zhu, S., Wang, J. & Liu, W. Scene text retrieval via joint text detection and similarity learning. In Proceedings of the IEEE/CVF Conference on Computer Vision and Pattern Recognition (pp. 4558–4567). (2021).
Wang, W., Xie, E., Song, X., Zang, Y., Wang, W., Lu, T. & Shen, C. Efficient and accurate arbitrary-shaped text detection with pixel aggregation network. In Proceedings of the IEEE/CVF international conference on computer vision (pp. 8440–8449). (2019).
Neudecker, C., Baierer, K., Gerber, M., Clausner, C., Antonacopoulos, A. & Pletschacher, S. A survey of OCR evaluation tools and metrics. In Proceedings of the 6th International Workshop on Historical Document Imaging and Processing (pp. 13–18). (2021).
Kumar, M., Jindal, M. K. & Kumar, M. An efficient technique for breaking of coloured Hindi CAPTCHA. Soft Comp. 27 (16), 11661–11686 (2023).
Kumar, M., Jindal, M. K. & Kumar, M. A novel attack on monochrome and greyscale Devanagari CAPTCHAs. Trans. Asian Low-Res. Lang. Inf. Process. 20 (4), 1–30 (2021).
Zheng, T., Chen, Z., Fang, S., Xie, H. & Jiang, Y. G. Cdistnet: Perceiving multi-domain character distance for robust text recognition. Int. J. Comp. Vision 132 (2), 300–318 (2024).
Terven, J. & Cordova-Esparza, D. A comprehensive review of YOLO: From YOLOv1 to YOLOv8 and beyond. arXiv 2023. arXiv preprint arXiv:2304.00501 .
Jiang, P., Ergu, D., Liu, F., Cai, Y. & Ma, B. A review of yolo algorithm developments. Procedia Comp. Sci. 199 , 1066–1073 (2022).
Maity, M., Banerjee, S. & Chaudhuri, S. S. Faster r-cnn and yolo based vehicle detection: A survey. In 2021 5th international conference on computing methodologies and communication (ICCMC) (pp. 1442–1447). IEEE. (2021).
Chen, R. C. Automatic license plate recognition via sliding-window darknet-YOLO deep learning. Image Vision Comp. 87 , 47–56 (2019).
Faska, Z., Khrissi, L., Haddouch, K. & El Akkad, N. A robust and consistent stack generalized ensemble-learning framework for image segmentation. J. Eng. Appl. Sci. 70 (1), 74 (2023).
Khrissi, L., El Akkad, N., Satori, H. & Satori, K. Clustering method and sine cosine algorithm for image segmentation. Evolu. Intell. 15 (1), 669–682 (2022).
Khrissi, L., El Akkad, N., Satori, H. & Satori, K. An efficient image clustering technique based on fuzzy c-means and cuckoo search algorithm. Int. J. Adv. Comp. Sci. Appl. 12 (6), 423 (2021).
Google Scholar
Moussaoui, H., Benslimane, M. & El Akkad, N. A novel brain tumor detection approach based on fuzzy c-means and marker watershed algorithm. In International Conference on Digital Technologies and Applications (pp. 871–879). Cham: Springer International Publishing. (2021).
Moussaoui, H., El Akkad, N. & Benslimane, M. Moroccan Carpets Classification Based on SVM Classifier and ORB Features. In International Conference on Digital Technologies and Applications (pp. 446–455). Cham: Springer International Publishing. (2022).
Moussaoui, H. & Benslimane, M. Reinforcement learning: A review. Int. J. Comp. Digital Syst. 13 (1), 1–1 (2023).
Khrissi, L. A. H. B. I. B., El Akkad, N. A. B. I. L., Satori, H. A. S. S. A. N. & Satori, K. H. A. L. I. D. A performant clustering approach based on an improved sine cosine algorithm. Int. J. Comput. 21 (2), 159–168 (2022).
Moussaoui, H., El Akkad, N. & Benslimane, M. A brain tumor segmentation and detection technique based on birch and marker watershed. SN Comput. Sci. 4 (4), 339 (2023).
Moussaoui, H., El Akkad, N. & Benslimane, M. A hybrid skin lesions segmentation approach based on image processing methods. Stat. Optimiz. Inf. Comput. 11 (1), 95–105 (2023).
Houssein, E. H., Helmy, B. E. D., Oliva, D., Elngar, A. A. & Shaban, H. A novel black widow optimization algorithm for multilevel thresholding image segmentation. Expert Syst. Appl. 167 , 114159 (2021).
Yu, J., Xiao, C., Hu, T. & Gao, Y. Selective weighted multi-scale morphological filter for fault feature extraction of rolling bearings. ISA Trans. 132 , 544–556 (2023).
Article PubMed Google Scholar
Pham, D. et al. Robust mapping of spatiotemporal trajectories and cell–cell interactions in healthy and diseased tissues. Nat. Commun. 14 (1), 7739 (2023).
Moussaoui, H., El Akkad, N. & Benslimane, M. A Review of Video Summarization. In International Conference on Digital Technologies and Applications (pp. 516–525). Cham: Springer Nature Switzerland. (2023).
Singh, N., Kumar, M., Singh, B. & Singh, J. DeepSpacy-NER: An efficient deep learning model for named entity recognition for Punjabi language. Evolving Syst. 14 (4), 673–683 (2023).
Zou, Z., Chen, K., Shi, Z., Guo, Y. & Ye, J. Object detection in 20 years: A survey. Proc. IEEE 111 (3), 257–276 (2023).
Li, M., Lv, T., Chen, J., Cui, L., Lu, Y., Florencio, D. & Wei, F. Trocr: Transformer-based optical character recognition with pre-trained models. In Proceedings of the AAAI Conference on Artificial Intelligence (Vol. 37, No. 11, pp. 13094–13102). (2023).
Pham, T. A. Effective deep neural networks for license plate detection and recognition. Visual Comput. 39 (3), 927–941 (2023).
Khan, M. M., Ilyas, M. U., Khan, I. R., Alshomrani, S. M. & Rahardja, S. A review of license plate recognition methods employing neural networks. IEEE Access https://doi.org/10.1109/ACCESS.2023.3254365 (2023).
Lubna, Mufti, N. & Shah, S. A. Automatic number plate Recognition: A detailed survey of relevant algorithms. Sensors 21 (9), 3028 (2021).
Article PubMed PubMed Central Google Scholar
Download references
Acknowledgements
The authors are very grateful to all the institutions in the affiliation list for successfully performing this research work. The authors would like to thank Cybersecurity and Information Networks Centre (CINC), Cardiff School of Technologies, Cardiff Metropolitan University, United Kingdom for their support.
Author information
Authors and affiliations.
Engineering Systems and Applications Laboratory, National School of Applied Sciences, Sidi Mohamed Ben Abdellah University, Fez, Morocco
Hanae Moussaoui & Nabil El Akkad
Laboratory of Industrial Techniques (LTI), EST of Fez, Sidi Mohamed Ben Abdellah University, Fez, Morocco
Mohamed Benslimane
Security Engineering Lab, Computer Science Department, Prince Sultan University, 11586, Riyadh, Saudi Arabia
Walid El-Shafai
Department of Electronics and Electrical Communications Engineering, Faculty of Electronic Engineering, Menoufia University, Menouf, 32952, Egypt
Computer Science Department, Community College, King Saud University, 11437, Riyadh, Saudi Arabia
Abdullah Baihan
Department of Computer Science, Cardiff School of Technologies, Cardiff Metropolitan University, Llandaff Campus, Western Avenue, Cardiff, CF5 2YB, UK
Chaminda Hewage & Rajkumar Singh Rathore
You can also search for this author in PubMed Google Scholar
Contributions
All authors are equally contributed.
Corresponding author
Correspondence to Rajkumar Singh Rathore .
Ethics declarations
Competing interests.
The authors declare no competing interests.
Additional information
Publisher's note.
Springer Nature remains neutral with regard to jurisdictional claims in published maps and institutional affiliations.
Rights and permissions
Open Access This article is licensed under a Creative Commons Attribution 4.0 International License, which permits use, sharing, adaptation, distribution and reproduction in any medium or format, as long as you give appropriate credit to the original author(s) and the source, provide a link to the Creative Commons licence, and indicate if changes were made. The images or other third party material in this article are included in the article's Creative Commons licence, unless indicated otherwise in a credit line to the material. If material is not included in the article's Creative Commons licence and your intended use is not permitted by statutory regulation or exceeds the permitted use, you will need to obtain permission directly from the copyright holder. To view a copy of this licence, visit http://creativecommons.org/licenses/by/4.0/ .
Reprints and permissions
About this article
Cite this article.
Moussaoui, H., Akkad, N.E., Benslimane, M. et al. Enhancing automated vehicle identification by integrating YOLO v8 and OCR techniques for high-precision license plate detection and recognition. Sci Rep 14 , 14389 (2024). https://doi.org/10.1038/s41598-024-65272-1
Download citation
Received : 25 December 2023
Accepted : 18 June 2024
Published : 22 June 2024
DOI : https://doi.org/10.1038/s41598-024-65272-1
Share this article
Anyone you share the following link with will be able to read this content:
Sorry, a shareable link is not currently available for this article.
Provided by the Springer Nature SharedIt content-sharing initiative
- Deep learning
- Image segmentation
- Character recognition
- Morphological operation
By submitting a comment you agree to abide by our Terms and Community Guidelines . If you find something abusive or that does not comply with our terms or guidelines please flag it as inappropriate.
Quick links
- Explore articles by subject
- Guide to authors
- Editorial policies
Sign up for the Nature Briefing: AI and Robotics newsletter — what matters in AI and robotics research, free to your inbox weekly.

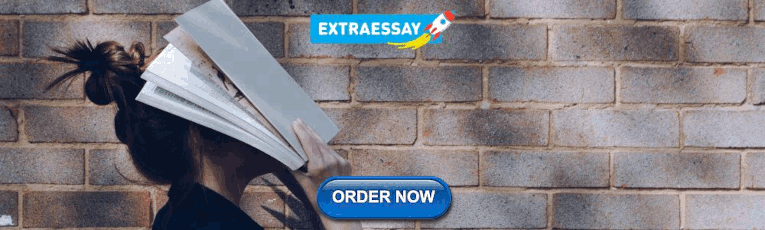
IMAGES
VIDEO
COMMENTS
A literature review is an integrated analysis-- not just a summary-- of scholarly writings and other relevant evidence related directly to your research question.That is, it represents a synthesis of the evidence that provides background information on your topic and shows a association between the evidence and your research question.
Literature reviews play a critical role in scholarship because science remains, first and foremost, a cumulative endeavour (vom Brocke et al., 2009). As in any academic discipline, rigorous knowledge syntheses are becoming indispensable in keeping up with an exponentially growing eHealth literature, assisting practitioners, academics, and graduate students in finding, evaluating, and ...
Example: Predictors and Outcomes of U.S. Quality Maternity Leave: A Review and Conceptual Framework: 10.1177/08948453211037398 ; Systematic review: "The authors of a systematic review use a specific procedure to search the research literature, select the studies to include in their review, and critically evaluate the studies they find." (p. 139).
Abstract. Performing a literature review is a critical first step in research to understanding the state-of-the-art and identifying gaps and challenges in the field. A systematic literature review is a method which sets out a series of steps to methodically organize the review. In this paper, we present a guide designed for researchers and in ...
This book includes steps for students and experienced scholars, with discussion of a variety of literature review types. Conducting research literature reviews:From the Internet to Paper (Fink, 2019). Available resources include Chapters 1 and 2. This edition includes recommendations for organizing literature reviews using online resources.
A literature review is defined as "a critical analysis of a segment of a published body of knowledge through summary, classification, and comparison of prior research studies, reviews of literature, and theoretical articles." (The Writing Center University of Winconsin-Madison 2022) A literature review is an integrated analysis, not just a summary of scholarly work on a specific topic.
A formal literature review is an evidence-based, in-depth analysis of a subject. There are many reasons for writing one and these will influence the length and style of your review, but in essence a literature review is a critical appraisal of the current collective knowledge on a subject. Rather than just being an exhaustive list of all that ...
Literature reviews establish the foundation of academic inquires. However, in the planning field, we lack rigorous systematic reviews. In this article, through a systematic search on the methodology of literature review, we categorize a typology of literature reviews, discuss steps in conducting a systematic literature review, and provide suggestions on how to enhance rigor in literature ...
The best proposals are timely and clearly explain why readers should pay attention to the proposed topic. It is not enough for a review to be a summary of the latest growth in the literature: the ...
lls the reader, and why it is necessary.3.2 Evaluate the nine basic steps taken to wr. te a well-constructed literature review.3.3 Conduct an electronic search using terms, phrases, Boolean operators, and filters.3.4 Evaluate and identify the parts of an empirical research journal article, and use that kn.
A literature review is essential to any scientific research study, which entails an in-depth analysis and synthesis of the existing literature and studies related to the research topic. The ...
In these cases, a literature review provides the basis for building a new conceptual model or theory, and it can be valuable when aiming to map the development of a particular research field over time. However, it is important to note that depending on the goal of the literature review, the method that should be used will vary. 2.1.
In writing the literature review, your purpose is to convey to your reader what knowledge and ideas have been established on a topic, and what their strengths and weaknesses are. As a piece of writing, the literature review must be defined by a guiding concept (e.g., your research objective, the problem or issue you are discussing, or your ...
This. paper discusses literature review as a methodology for conducting research and o ffers an overview of different. types of reviews, as well as some guidelines to how to both conduct and ...
Method details Overview. A Systematic Literature Review (SLR) is a research methodology to collect, identify, and critically analyze the available research studies (e.g., articles, conference proceedings, books, dissertations) through a systematic procedure [12].An SLR updates the reader with current literature about a subject [6].The goal is to review critical points of current knowledge on a ...
3. Evaluate and select literature. 4. Analyze the literature. 5. Plan the structure of your literature review. 6. Write your literature review. Other resources to help you write a successful literature review.
The Literature Review by Diana Ridley The Literature Review is a step-by-step guide to conducting a literature search and writing up the literature review chapter in Masters dissertations and in Ph.D. and professional doctorate theses. The author provides strategies for reading, conducting searches, organizing information and writing the review.
Literature reviews are in great demand in most scientific fields. Their need stems from the ever-increasing output of scientific publications .For example, compared to 1991, in 2008 three, eight, and forty times more papers were indexed in Web of Science on malaria, obesity, and biodiversity, respectively .Given such mountains of papers, scientists cannot be expected to examine in detail every ...
The other pages in this guide will cover some basic steps to consider when conducting a traditional health sciences literature review. See below for a quick look at some of the more popular types of literature reviews. For additional information on a variety of review methods, the following article provides an excellent overview. Grant MJ, Booth A.
Okay - with the why out the way, let's move on to the how. As mentioned above, writing your literature review is a process, which I'll break down into three steps: Finding the most suitable literature. Understanding, distilling and organising the literature. Planning and writing up your literature review chapter.
A systematic literature review (SLR) is an independent academic method that aims to identify and evaluate all relevant literature on a topic in order to derive conclusions about the question under consideration. "Systematic reviews are undertaken to clarify the state of existing research and the implications that should be drawn from this."
A literature or narrative review is a comprehensive review and analysis of the published literature on a specific topic or research question. The literature that is reviewed contains: books, articles, academic articles, conference proceedings, association papers, and dissertations. It contains the most pertinent studies and points to important ...
Conducting the Study. The first step in the scientific process is to identify a problem, which entails all of the following except: A Hypothesis. A researcher conducts a literature review. After completing this review, she states that texting during class can improve student learning. This statement is referred to as:
Step 1 Since an image is typically used as the given document for OCR, this step happens first. This entails making modifications to the picture, removing noise, and binarizing it (turning it into ...