
Statistics Made Easy
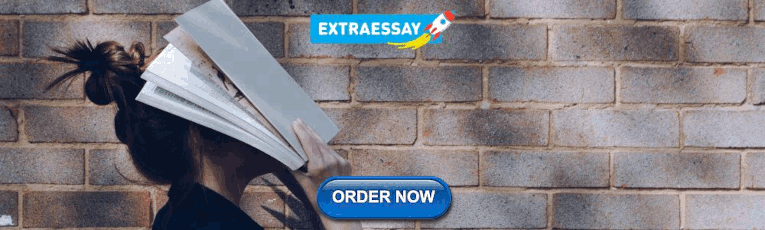
Extraneous Variable: Definition & Examples
The whole point of conducting an experiment is to determine whether or not changing the values of some independent variable has an effect on a dependent variable.
An extraneous variable is any variable you’re not interested in studying that could also have some effect on the dependent variable.

For example, we might want to know how the number of hours that a basketball player trains per week affects their average points per game. However, an extraneous variable that could also affect their points per game is the number of hours they spend stretching each week.

We would need to make sure that we control for this extraneous variable so that we can draw reliable conclusions about the effect that hours spent training has on average points per game.
Types of Extraneous Variables
There are four types of extraneous variables:
1. Situational Variables
These are aspects of the environment that could affect the way an individual behaves in an experiment. Examples include:
- Lighting conditions
- Visual distractions
- Temperature
2. Experimenter Bias
This refers to ways in which the experimenter may accidently bias the way that individuals behave in an experiment. Examples include:
- Using certain phrasing to hint at the way researchers want individuals to behave.
- Speaking in a positive or negative tone when providing instructions to individuals.
3. Demand Characteristics
This refers to any clues in the experiment that could give away the true purpose of the research to the individuals. Examples include:
- Their surroundings.
- Their interpretation of what is happening in the situation.
- The researcher’s behavior.
4. Participant Variables
This refers to the natural variance among individuals and the ways in which this could affect the results of the experiment. Examples include:
- An individual’s natural intelligence.
- An individuals’ mood that day.
- An individual’s physical abilities.
- An individual’s ability to concentrate.
How to Control Extraneous Variables
There are four main ways to control for extraneous variables in an experiment:
1. Consistent environment.
Each individual should be able to participate in an experiment in the exact same environment, e.g. with the same lighting conditions, same noise levels, same temperature, and same number of potential distractions. This should minimize the effect of situational variables and demand characteristics.
2. Experimental design.
By designing an experiment such that individuals are randomly assigned to treatment groups and such that researchers are also blind to which individuals belong to which group, this should minimize the problem of experimental bias.
3. Randomization.
By randomly assigning individuals to treatments (e.g. high training volume vs. low training volume), any differences in participant ability between groups should be roughly evenly distributed. This should minimize the problem of participant variables.
By using a consistent environment, experimental design, and randomization, researchers can minimize the effect that potential extraneous variables can have on experiment.
Thus, when researchers draw conclusions about the effect that an independent variable has on a dependent variable, they can be sure that no extraneous variables are actually causing the true effect.
Additional Resources
What is a Lurking Variable? What is a Confounding Variable?
Featured Posts

Hey there. My name is Zach Bobbitt. I have a Masters of Science degree in Applied Statistics and I’ve worked on machine learning algorithms for professional businesses in both healthcare and retail. I’m passionate about statistics, machine learning, and data visualization and I created Statology to be a resource for both students and teachers alike. My goal with this site is to help you learn statistics through using simple terms, plenty of real-world examples, and helpful illustrations.
2 Replies to “Extraneous Variable: Definition & Examples”
Question – theoretically, the lurking and concomitant variables would be extraneous variables , correct?
Excellent! Thanks so much for this very detailed clarification!
Leave a Reply Cancel reply
Your email address will not be published. Required fields are marked *
What is an extraneous variable?
Last updated
6 February 2023
Reviewed by
Cathy Heath
Independent
Intervening
Quantitative
Qualitative
Confounding
An extraneous variable is any variable not being investigated that has the potential to affect the outcome of a research study. In other words, it is any factor not considered an independent variable that can affect the dependent variables or controlled conditions.
For example, in a study of physical performance (independent variable), the effect of a specific athletic shoe (dependent variable) may be tested. Extraneous variables in this example might include:
Demographics such as age and gender
Testing environment
Time of day of testing
Make research less tedious
Dovetail streamlines research to help you uncover and share actionable insights
- Why do extraneous variables matter?
Extraneous variables provide alternative explanations for test results. Because of this, they can affect and threaten the internal validity of a study.
When not accounted for, extraneous variables often introduce selection biases to research, such as:
Attrition bias: the unequal loss of participants from a study group when those participants who drop out of the study are systematically different from those who stay.
Nonresponsive bias: when participants who do not respond to a survey are significantly different from those who do respond.
Ascertainment, or sampling, bias: when certain members of the intended population are less likely to be included than others.
Undercoverage bias: when some members of the population are not represented in the sample.
Survivorship bias: when conclusions are drawn by focusing only on examples of successful individuals (survivors) rather than the entire group.
Extraneous variables matter because of the effect they can have on the outcome of a study.
- Extraneous vs. confounding variables
An extraneous variable is anything that could influence the dependent variable. A confounding variable is an extraneous variable associated with both independent and dependent variables . The confounding variable influences the dependent variable and correlates with the independent variable.
In a conceptual framework diagram, the confounding variable is connected by an arrow to both the independent and dependent variables. Extraneous variables are only connected to a dependent variable .
- How extraneous variables work
Uncontrolled extraneous variables make it hard to determine the exact effects of the independent variable on the dependent variable, because they may mask them. They can also make it seem as though there is a true effect of the independent variable in the experiment when there is none.
- Types of extraneous variables
Extraneous variables are categorized into four major types. These categories help researchers to select the best methods of control for their research.
Experimenter effects
Unintentional actions by researchers that influence study outcomes are called experimenter effects. These include experimenters' interactions with study participants that can unintentionally affect their behaviors and cause errors in measurement, analysis, observation, or interpretation that might change the study results. Examples include:
Speaking in a positive or negative tone while providing experiment instructions
Using certain phrasing to hint at how experimenters want participants to behave
The experimenter must maintain an unbiased presence for test results to be valid.
Situational variables
Variables that can alter participants' behaviors in study environments, such as temperature or lighting, are called situational variables. Factors such as these are the sources of random variation, or random errors, in measurements.
The effect of situational variables must be eliminated or reduced to understand the true relationship between independent and dependent variables.
Participant variables
Participant variables are characteristics or aspects of a study participant's background that could affect study results. Examples of participant variables are:
Gender identity
Marital status
Religious affiliation
Because these differences can lead to different outcomes, it's important to measure and analyze these variables.
Demand characteristics
Demand characteristics are cues that encourage participants to conform to researchers' behavioral expectations. These occur when study subjects infer the intentions of a research study from the materials or research setting and use these hints to act in ways consistent with research hypotheses.
Demand characteristics can bias study outcomes and reduce the external validity of the results.
- How to control extraneous variables
An important aspect of experimental design is the control of extraneous variables.
Standardized procedures
One way to avoid experimenter effects is to implement masking to hide the hypothesis from the participants and the researchers. In such a double-blind study, researchers cannot bias participants towards acting in expected ways or selectively interpret results to suit the hypothesis.
Keeping the variables constant throughout the study or statistically accounting for them in analyses is the best way to avoid situational variables from influencing study outcomes.
To avoid demand characteristics, researchers might try making it difficult for participants to guess the aim of the study. Asking participants to perform unrelated filler tasks or fill out plausibly relevant surveys can lead them away from the true nature of the study.
Random assignment
Randomly assigning the participants to control and experimental groups will prevent participant variables. This makes groups comparable by evenly distributing participant characteristics between them.
Controlling an extraneous variable turns it into a control variable.
Extraneous variables introduce research bias to a study. When left uncontrolled, they might lead to inaccurate conclusions about the relationship between independent and dependent variables.
What are some examples of extraneous variables?
In a study researching whether memory capacity is related to test performance, extraneous variables might include:
The time of day the test is taken
Test anxiety experienced by participants
The level of stress caused by the test
Researching whether sleep deprivation affects driving ability could have extraneous variables such as:
Years of driving experience
Road conditions
Noise levels in the vehicle
What is the difference between independent and extraneous variables?
Independent variables are controllable variables. They are changed or varied during a study to influence the dependent variable. The independent variable is the feature, attribute, or factor manipulated by the researcher so that its effect on the dependent variables can be measured.
Extraneous variables also affect the dependent variable, but the researcher cannot manipulate these. They are uncontrolled variables that present themselves during a study and provide alternative explanations for the test results.
What extraneous variables can arise from a telephone survey method?
In the telephone survey method of conducting research, extraneous variables might include:
Background noise
Distractions, such as a television or other people in the room
Integrity of the telephone connection
The interviewee's ability to hear and understand questions
These extraneous variables can influence the validity of study results.
Editor’s picks
Last updated: 11 January 2024
Last updated: 6 October 2023
Last updated: 25 November 2023
Last updated: 12 May 2023
Last updated: 15 February 2024
Last updated: 30 April 2024
Last updated: 18 May 2023
Last updated: 10 April 2023
Latest articles
Related topics, .css-je19u9{-webkit-align-items:flex-end;-webkit-box-align:flex-end;-ms-flex-align:flex-end;align-items:flex-end;display:-webkit-box;display:-webkit-flex;display:-ms-flexbox;display:flex;-webkit-flex-direction:row;-ms-flex-direction:row;flex-direction:row;-webkit-box-flex-wrap:wrap;-webkit-flex-wrap:wrap;-ms-flex-wrap:wrap;flex-wrap:wrap;-webkit-box-pack:center;-ms-flex-pack:center;-webkit-justify-content:center;justify-content:center;row-gap:0;text-align:center;max-width:671px;}@media (max-width: 1079px){.css-je19u9{max-width:400px;}.css-je19u9>span{white-space:pre;}}@media (max-width: 799px){.css-je19u9{max-width:400px;}.css-je19u9>span{white-space:pre;}} decide what to .css-1kiodld{max-height:56px;display:-webkit-box;display:-webkit-flex;display:-ms-flexbox;display:flex;-webkit-align-items:center;-webkit-box-align:center;-ms-flex-align:center;align-items:center;}@media (max-width: 1079px){.css-1kiodld{display:none;}} build next, decide what to build next.

Users report unexpectedly high data usage, especially during streaming sessions.

Users find it hard to navigate from the home page to relevant playlists in the app.

It would be great to have a sleep timer feature, especially for bedtime listening.

I need better filters to find the songs or artists I’m looking for.
Log in or sign up
Get started for free

Want to create or adapt books like this? Learn more about how Pressbooks supports open publishing practices.
6.1 Experiment Basics
Learning objectives.
- Explain what an experiment is and recognize examples of studies that are experiments and studies that are not experiments.
- Explain what internal validity is and why experiments are considered to be high in internal validity.
- Explain what external validity is and evaluate studies in terms of their external validity.
- Distinguish between the manipulation of the independent variable and control of extraneous variables and explain the importance of each.
- Recognize examples of confounding variables and explain how they affect the internal validity of a study.
What Is an Experiment?
As we saw earlier in the book, an experiment is a type of study designed specifically to answer the question of whether there is a causal relationship between two variables. Do changes in an independent variable cause changes in a dependent variable? Experiments have two fundamental features. The first is that the researchers manipulate, or systematically vary, the level of the independent variable. The different levels of the independent variable are called conditions. For example, in Darley and Latané’s experiment, the independent variable was the number of witnesses that participants believed to be present. The researchers manipulated this independent variable by telling participants that there were either one, two, or five other students involved in the discussion, thereby creating three conditions. The second fundamental feature of an experiment is that the researcher controls, or minimizes the variability in, variables other than the independent and dependent variable. These other variables are called extraneous variables. Darley and Latané tested all their participants in the same room, exposed them to the same emergency situation, and so on. They also randomly assigned their participants to conditions so that the three groups would be similar to each other to begin with. Notice that although the words manipulation and control have similar meanings in everyday language, researchers make a clear distinction between them. They manipulate the independent variable by systematically changing its levels and control other variables by holding them constant.
Internal and External Validity
Internal validity.
Recall that the fact that two variables are statistically related does not necessarily mean that one causes the other. “Correlation does not imply causation.” For example, if it were the case that people who exercise regularly are happier than people who do not exercise regularly, this would not necessarily mean that exercising increases people’s happiness. It could mean instead that greater happiness causes people to exercise (the directionality problem) or that something like better physical health causes people to exercise and be happier (the third-variable problem).
The purpose of an experiment, however, is to show that two variables are statistically related and to do so in a way that supports the conclusion that the independent variable caused any observed differences in the dependent variable. The basic logic is this: If the researcher creates two or more highly similar conditions and then manipulates the independent variable to produce just one difference between them, then any later difference between the conditions must have been caused by the independent variable. For example, because the only difference between Darley and Latané’s conditions was the number of students that participants believed to be involved in the discussion, this must have been responsible for differences in helping between the conditions.
An empirical study is said to be high in internal validity if the way it was conducted supports the conclusion that the independent variable caused any observed differences in the dependent variable. Thus experiments are high in internal validity because the way they are conducted—with the manipulation of the independent variable and the control of extraneous variables—provides strong support for causal conclusions.
External Validity
At the same time, the way that experiments are conducted sometimes leads to a different kind of criticism. Specifically, the need to manipulate the independent variable and control extraneous variables means that experiments are often conducted under conditions that seem artificial or unlike “real life” (Stanovich, 2010). In many psychology experiments, the participants are all college undergraduates and come to a classroom or laboratory to fill out a series of paper-and-pencil questionnaires or to perform a carefully designed computerized task. Consider, for example, an experiment in which researcher Barbara Fredrickson and her colleagues had college students come to a laboratory on campus and complete a math test while wearing a swimsuit (Fredrickson, Roberts, Noll, Quinn, & Twenge, 1998). At first, this might seem silly. When will college students ever have to complete math tests in their swimsuits outside of this experiment?
The issue we are confronting is that of external validity. An empirical study is high in external validity if the way it was conducted supports generalizing the results to people and situations beyond those actually studied. As a general rule, studies are higher in external validity when the participants and the situation studied are similar to those that the researchers want to generalize to. Imagine, for example, that a group of researchers is interested in how shoppers in large grocery stores are affected by whether breakfast cereal is packaged in yellow or purple boxes. Their study would be high in external validity if they studied the decisions of ordinary people doing their weekly shopping in a real grocery store. If the shoppers bought much more cereal in purple boxes, the researchers would be fairly confident that this would be true for other shoppers in other stores. Their study would be relatively low in external validity, however, if they studied a sample of college students in a laboratory at a selective college who merely judged the appeal of various colors presented on a computer screen. If the students judged purple to be more appealing than yellow, the researchers would not be very confident that this is relevant to grocery shoppers’ cereal-buying decisions.
We should be careful, however, not to draw the blanket conclusion that experiments are low in external validity. One reason is that experiments need not seem artificial. Consider that Darley and Latané’s experiment provided a reasonably good simulation of a real emergency situation. Or consider field experiments that are conducted entirely outside the laboratory. In one such experiment, Robert Cialdini and his colleagues studied whether hotel guests choose to reuse their towels for a second day as opposed to having them washed as a way of conserving water and energy (Cialdini, 2005). These researchers manipulated the message on a card left in a large sample of hotel rooms. One version of the message emphasized showing respect for the environment, another emphasized that the hotel would donate a portion of their savings to an environmental cause, and a third emphasized that most hotel guests choose to reuse their towels. The result was that guests who received the message that most hotel guests choose to reuse their towels reused their own towels substantially more often than guests receiving either of the other two messages. Given the way they conducted their study, it seems very likely that their result would hold true for other guests in other hotels.
A second reason not to draw the blanket conclusion that experiments are low in external validity is that they are often conducted to learn about psychological processes that are likely to operate in a variety of people and situations. Let us return to the experiment by Fredrickson and colleagues. They found that the women in their study, but not the men, performed worse on the math test when they were wearing swimsuits. They argued that this was due to women’s greater tendency to objectify themselves—to think about themselves from the perspective of an outside observer—which diverts their attention away from other tasks. They argued, furthermore, that this process of self-objectification and its effect on attention is likely to operate in a variety of women and situations—even if none of them ever finds herself taking a math test in her swimsuit.
Manipulation of the Independent Variable
Again, to manipulate an independent variable means to change its level systematically so that different groups of participants are exposed to different levels of that variable, or the same group of participants is exposed to different levels at different times. For example, to see whether expressive writing affects people’s health, a researcher might instruct some participants to write about traumatic experiences and others to write about neutral experiences. The different levels of the independent variable are referred to as conditions , and researchers often give the conditions short descriptive names to make it easy to talk and write about them. In this case, the conditions might be called the “traumatic condition” and the “neutral condition.”
Notice that the manipulation of an independent variable must involve the active intervention of the researcher. Comparing groups of people who differ on the independent variable before the study begins is not the same as manipulating that variable. For example, a researcher who compares the health of people who already keep a journal with the health of people who do not keep a journal has not manipulated this variable and therefore not conducted an experiment. This is important because groups that already differ in one way at the beginning of a study are likely to differ in other ways too. For example, people who choose to keep journals might also be more conscientious, more introverted, or less stressed than people who do not. Therefore, any observed difference between the two groups in terms of their health might have been caused by whether or not they keep a journal, or it might have been caused by any of the other differences between people who do and do not keep journals. Thus the active manipulation of the independent variable is crucial for eliminating the third-variable problem.
Of course, there are many situations in which the independent variable cannot be manipulated for practical or ethical reasons and therefore an experiment is not possible. For example, whether or not people have a significant early illness experience cannot be manipulated, making it impossible to do an experiment on the effect of early illness experiences on the development of hypochondriasis. This does not mean it is impossible to study the relationship between early illness experiences and hypochondriasis—only that it must be done using nonexperimental approaches. We will discuss this in detail later in the book.
In many experiments, the independent variable is a construct that can only be manipulated indirectly. For example, a researcher might try to manipulate participants’ stress levels indirectly by telling some of them that they have five minutes to prepare a short speech that they will then have to give to an audience of other participants. In such situations, researchers often include a manipulation check in their procedure. A manipulation check is a separate measure of the construct the researcher is trying to manipulate. For example, researchers trying to manipulate participants’ stress levels might give them a paper-and-pencil stress questionnaire or take their blood pressure—perhaps right after the manipulation or at the end of the procedure—to verify that they successfully manipulated this variable.
Control of Extraneous Variables
An extraneous variable is anything that varies in the context of a study other than the independent and dependent variables. In an experiment on the effect of expressive writing on health, for example, extraneous variables would include participant variables (individual differences) such as their writing ability, their diet, and their shoe size. They would also include situation or task variables such as the time of day when participants write, whether they write by hand or on a computer, and the weather. Extraneous variables pose a problem because many of them are likely to have some effect on the dependent variable. For example, participants’ health will be affected by many things other than whether or not they engage in expressive writing. This can make it difficult to separate the effect of the independent variable from the effects of the extraneous variables, which is why it is important to control extraneous variables by holding them constant.
Extraneous Variables as “Noise”
Extraneous variables make it difficult to detect the effect of the independent variable in two ways. One is by adding variability or “noise” to the data. Imagine a simple experiment on the effect of mood (happy vs. sad) on the number of happy childhood events people are able to recall. Participants are put into a negative or positive mood (by showing them a happy or sad video clip) and then asked to recall as many happy childhood events as they can. The two leftmost columns of Table 6.1 “Hypothetical Noiseless Data and Realistic Noisy Data” show what the data might look like if there were no extraneous variables and the number of happy childhood events participants recalled was affected only by their moods. Every participant in the happy mood condition recalled exactly four happy childhood events, and every participant in the sad mood condition recalled exactly three. The effect of mood here is quite obvious. In reality, however, the data would probably look more like those in the two rightmost columns of Table 6.1 “Hypothetical Noiseless Data and Realistic Noisy Data” . Even in the happy mood condition, some participants would recall fewer happy memories because they have fewer to draw on, use less effective strategies, or are less motivated. And even in the sad mood condition, some participants would recall more happy childhood memories because they have more happy memories to draw on, they use more effective recall strategies, or they are more motivated. Although the mean difference between the two groups is the same as in the idealized data, this difference is much less obvious in the context of the greater variability in the data. Thus one reason researchers try to control extraneous variables is so their data look more like the idealized data in Table 6.1 “Hypothetical Noiseless Data and Realistic Noisy Data” , which makes the effect of the independent variable is easier to detect (although real data never look quite that good).
Table 6.1 Hypothetical Noiseless Data and Realistic Noisy Data
One way to control extraneous variables is to hold them constant. This can mean holding situation or task variables constant by testing all participants in the same location, giving them identical instructions, treating them in the same way, and so on. It can also mean holding participant variables constant. For example, many studies of language limit participants to right-handed people, who generally have their language areas isolated in their left cerebral hemispheres. Left-handed people are more likely to have their language areas isolated in their right cerebral hemispheres or distributed across both hemispheres, which can change the way they process language and thereby add noise to the data.
In principle, researchers can control extraneous variables by limiting participants to one very specific category of person, such as 20-year-old, straight, female, right-handed, sophomore psychology majors. The obvious downside to this approach is that it would lower the external validity of the study—in particular, the extent to which the results can be generalized beyond the people actually studied. For example, it might be unclear whether results obtained with a sample of younger straight women would apply to older gay men. In many situations, the advantages of a diverse sample outweigh the reduction in noise achieved by a homogeneous one.
Extraneous Variables as Confounding Variables
The second way that extraneous variables can make it difficult to detect the effect of the independent variable is by becoming confounding variables. A confounding variable is an extraneous variable that differs on average across levels of the independent variable. For example, in almost all experiments, participants’ intelligence quotients (IQs) will be an extraneous variable. But as long as there are participants with lower and higher IQs at each level of the independent variable so that the average IQ is roughly equal, then this variation is probably acceptable (and may even be desirable). What would be bad, however, would be for participants at one level of the independent variable to have substantially lower IQs on average and participants at another level to have substantially higher IQs on average. In this case, IQ would be a confounding variable.
To confound means to confuse, and this is exactly what confounding variables do. Because they differ across conditions—just like the independent variable—they provide an alternative explanation for any observed difference in the dependent variable. Figure 6.1 “Hypothetical Results From a Study on the Effect of Mood on Memory” shows the results of a hypothetical study, in which participants in a positive mood condition scored higher on a memory task than participants in a negative mood condition. But if IQ is a confounding variable—with participants in the positive mood condition having higher IQs on average than participants in the negative mood condition—then it is unclear whether it was the positive moods or the higher IQs that caused participants in the first condition to score higher. One way to avoid confounding variables is by holding extraneous variables constant. For example, one could prevent IQ from becoming a confounding variable by limiting participants only to those with IQs of exactly 100. But this approach is not always desirable for reasons we have already discussed. A second and much more general approach—random assignment to conditions—will be discussed in detail shortly.
Figure 6.1 Hypothetical Results From a Study on the Effect of Mood on Memory

Because IQ also differs across conditions, it is a confounding variable.
Key Takeaways
- An experiment is a type of empirical study that features the manipulation of an independent variable, the measurement of a dependent variable, and control of extraneous variables.
- Studies are high in internal validity to the extent that the way they are conducted supports the conclusion that the independent variable caused any observed differences in the dependent variable. Experiments are generally high in internal validity because of the manipulation of the independent variable and control of extraneous variables.
- Studies are high in external validity to the extent that the result can be generalized to people and situations beyond those actually studied. Although experiments can seem “artificial”—and low in external validity—it is important to consider whether the psychological processes under study are likely to operate in other people and situations.
- Practice: List five variables that can be manipulated by the researcher in an experiment. List five variables that cannot be manipulated by the researcher in an experiment.
Practice: For each of the following topics, decide whether that topic could be studied using an experimental research design and explain why or why not.
- Effect of parietal lobe damage on people’s ability to do basic arithmetic.
- Effect of being clinically depressed on the number of close friendships people have.
- Effect of group training on the social skills of teenagers with Asperger’s syndrome.
- Effect of paying people to take an IQ test on their performance on that test.
Cialdini, R. (2005, April). Don’t throw in the towel: Use social influence research. APS Observer . Retrieved from http://www.psychologicalscience.org/observer/getArticle.cfm?id=1762 .
Fredrickson, B. L., Roberts, T.-A., Noll, S. M., Quinn, D. M., & Twenge, J. M. (1998). The swimsuit becomes you: Sex differences in self-objectification, restrained eating, and math performance. Journal of Personality and Social Psychology, 75 , 269–284.
Stanovich, K. E. (2010). How to think straight about psychology (9th ed.). Boston, MA: Allyn & Bacon.
Research Methods in Psychology Copyright © 2016 by University of Minnesota is licensed under a Creative Commons Attribution-NonCommercial-ShareAlike 4.0 International License , except where otherwise noted.

Research Variables 101
Independent variables, dependent variables, control variables and more
By: Derek Jansen (MBA) | Expert Reviewed By: Kerryn Warren (PhD) | January 2023
If you’re new to the world of research, especially scientific research, you’re bound to run into the concept of variables , sooner or later. If you’re feeling a little confused, don’t worry – you’re not the only one! Independent variables, dependent variables, confounding variables – it’s a lot of jargon. In this post, we’ll unpack the terminology surrounding research variables using straightforward language and loads of examples .
Overview: Variables In Research
What (exactly) is a variable.
The simplest way to understand a variable is as any characteristic or attribute that can experience change or vary over time or context – hence the name “variable”. For example, the dosage of a particular medicine could be classified as a variable, as the amount can vary (i.e., a higher dose or a lower dose). Similarly, gender, age or ethnicity could be considered demographic variables, because each person varies in these respects.
Within research, especially scientific research, variables form the foundation of studies, as researchers are often interested in how one variable impacts another, and the relationships between different variables. For example:
- How someone’s age impacts their sleep quality
- How different teaching methods impact learning outcomes
- How diet impacts weight (gain or loss)
As you can see, variables are often used to explain relationships between different elements and phenomena. In scientific studies, especially experimental studies, the objective is often to understand the causal relationships between variables. In other words, the role of cause and effect between variables. This is achieved by manipulating certain variables while controlling others – and then observing the outcome. But, we’ll get into that a little later…
The “Big 3” Variables
Variables can be a little intimidating for new researchers because there are a wide variety of variables, and oftentimes, there are multiple labels for the same thing. To lay a firm foundation, we’ll first look at the three main types of variables, namely:
- Independent variables (IV)
- Dependant variables (DV)
- Control variables
What is an independent variable?
Simply put, the independent variable is the “ cause ” in the relationship between two (or more) variables. In other words, when the independent variable changes, it has an impact on another variable.
For example:
- Increasing the dosage of a medication (Variable A) could result in better (or worse) health outcomes for a patient (Variable B)
- Changing a teaching method (Variable A) could impact the test scores that students earn in a standardised test (Variable B)
- Varying one’s diet (Variable A) could result in weight loss or gain (Variable B).
It’s useful to know that independent variables can go by a few different names, including, explanatory variables (because they explain an event or outcome) and predictor variables (because they predict the value of another variable). Terminology aside though, the most important takeaway is that independent variables are assumed to be the “cause” in any cause-effect relationship. As you can imagine, these types of variables are of major interest to researchers, as many studies seek to understand the causal factors behind a phenomenon.
Need a helping hand?
What is a dependent variable?
While the independent variable is the “ cause ”, the dependent variable is the “ effect ” – or rather, the affected variable . In other words, the dependent variable is the variable that is assumed to change as a result of a change in the independent variable.
Keeping with the previous example, let’s look at some dependent variables in action:
- Health outcomes (DV) could be impacted by dosage changes of a medication (IV)
- Students’ scores (DV) could be impacted by teaching methods (IV)
- Weight gain or loss (DV) could be impacted by diet (IV)
In scientific studies, researchers will typically pay very close attention to the dependent variable (or variables), carefully measuring any changes in response to hypothesised independent variables. This can be tricky in practice, as it’s not always easy to reliably measure specific phenomena or outcomes – or to be certain that the actual cause of the change is in fact the independent variable.
As the adage goes, correlation is not causation . In other words, just because two variables have a relationship doesn’t mean that it’s a causal relationship – they may just happen to vary together. For example, you could find a correlation between the number of people who own a certain brand of car and the number of people who have a certain type of job. Just because the number of people who own that brand of car and the number of people who have that type of job is correlated, it doesn’t mean that owning that brand of car causes someone to have that type of job or vice versa. The correlation could, for example, be caused by another factor such as income level or age group, which would affect both car ownership and job type.
To confidently establish a causal relationship between an independent variable and a dependent variable (i.e., X causes Y), you’ll typically need an experimental design , where you have complete control over the environmen t and the variables of interest. But even so, this doesn’t always translate into the “real world”. Simply put, what happens in the lab sometimes stays in the lab!
As an alternative to pure experimental research, correlational or “ quasi-experimental ” research (where the researcher cannot manipulate or change variables) can be done on a much larger scale more easily, allowing one to understand specific relationships in the real world. These types of studies also assume some causality between independent and dependent variables, but it’s not always clear. So, if you go this route, you need to be cautious in terms of how you describe the impact and causality between variables and be sure to acknowledge any limitations in your own research.
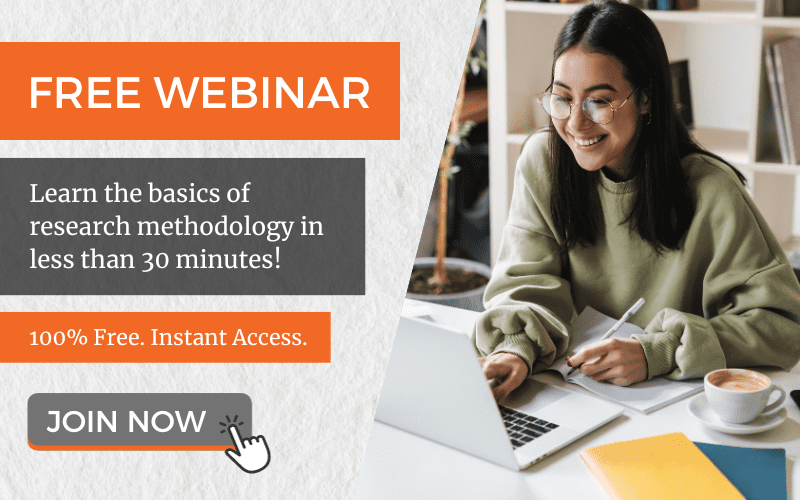
What is a control variable?
In an experimental design, a control variable (or controlled variable) is a variable that is intentionally held constant to ensure it doesn’t have an influence on any other variables. As a result, this variable remains unchanged throughout the course of the study. In other words, it’s a variable that’s not allowed to vary – tough life 🙂
As we mentioned earlier, one of the major challenges in identifying and measuring causal relationships is that it’s difficult to isolate the impact of variables other than the independent variable. Simply put, there’s always a risk that there are factors beyond the ones you’re specifically looking at that might be impacting the results of your study. So, to minimise the risk of this, researchers will attempt (as best possible) to hold other variables constant . These factors are then considered control variables.
Some examples of variables that you may need to control include:
- Temperature
- Time of day
- Noise or distractions
Which specific variables need to be controlled for will vary tremendously depending on the research project at hand, so there’s no generic list of control variables to consult. As a researcher, you’ll need to think carefully about all the factors that could vary within your research context and then consider how you’ll go about controlling them. A good starting point is to look at previous studies similar to yours and pay close attention to which variables they controlled for.
Of course, you won’t always be able to control every possible variable, and so, in many cases, you’ll just have to acknowledge their potential impact and account for them in the conclusions you draw. Every study has its limitations , so don’t get fixated or discouraged by troublesome variables. Nevertheless, always think carefully about the factors beyond what you’re focusing on – don’t make assumptions!
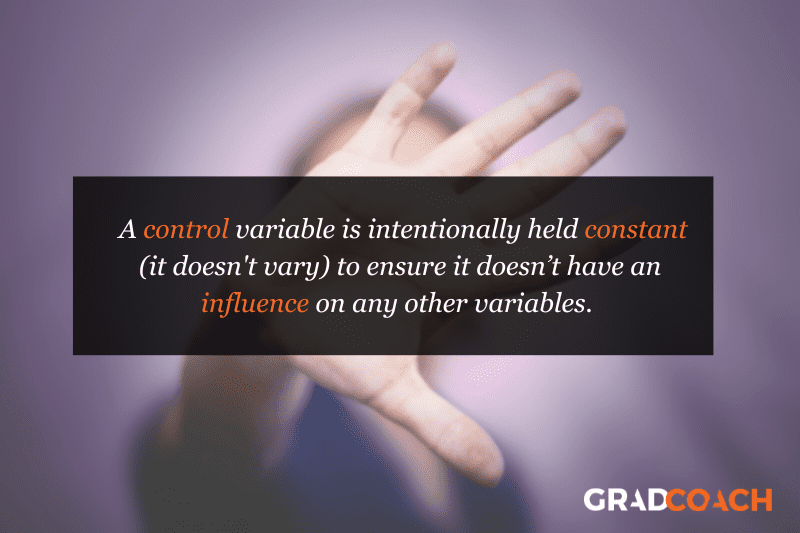
Other types of variables
As we mentioned, independent, dependent and control variables are the most common variables you’ll come across in your research, but they’re certainly not the only ones you need to be aware of. Next, we’ll look at a few “secondary” variables that you need to keep in mind as you design your research.
- Moderating variables
- Mediating variables
- Confounding variables
- Latent variables
Let’s jump into it…
What is a moderating variable?
A moderating variable is a variable that influences the strength or direction of the relationship between an independent variable and a dependent variable. In other words, moderating variables affect how much (or how little) the IV affects the DV, or whether the IV has a positive or negative relationship with the DV (i.e., moves in the same or opposite direction).
For example, in a study about the effects of sleep deprivation on academic performance, gender could be used as a moderating variable to see if there are any differences in how men and women respond to a lack of sleep. In such a case, one may find that gender has an influence on how much students’ scores suffer when they’re deprived of sleep.
It’s important to note that while moderators can have an influence on outcomes , they don’t necessarily cause them ; rather they modify or “moderate” existing relationships between other variables. This means that it’s possible for two different groups with similar characteristics, but different levels of moderation, to experience very different results from the same experiment or study design.
What is a mediating variable?
Mediating variables are often used to explain the relationship between the independent and dependent variable (s). For example, if you were researching the effects of age on job satisfaction, then education level could be considered a mediating variable, as it may explain why older people have higher job satisfaction than younger people – they may have more experience or better qualifications, which lead to greater job satisfaction.
Mediating variables also help researchers understand how different factors interact with each other to influence outcomes. For instance, if you wanted to study the effect of stress on academic performance, then coping strategies might act as a mediating factor by influencing both stress levels and academic performance simultaneously. For example, students who use effective coping strategies might be less stressed but also perform better academically due to their improved mental state.
In addition, mediating variables can provide insight into causal relationships between two variables by helping researchers determine whether changes in one factor directly cause changes in another – or whether there is an indirect relationship between them mediated by some third factor(s). For instance, if you wanted to investigate the impact of parental involvement on student achievement, you would need to consider family dynamics as a potential mediator, since it could influence both parental involvement and student achievement simultaneously.
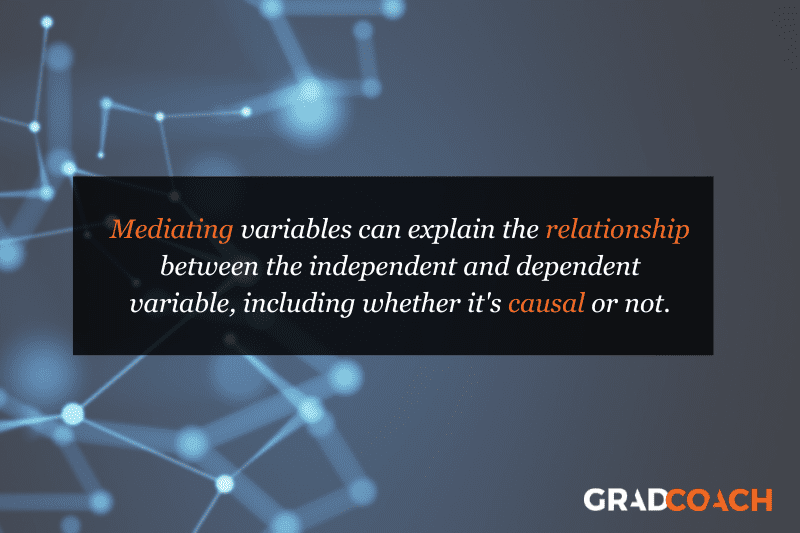
What is a confounding variable?
A confounding variable (also known as a third variable or lurking variable ) is an extraneous factor that can influence the relationship between two variables being studied. Specifically, for a variable to be considered a confounding variable, it needs to meet two criteria:
- It must be correlated with the independent variable (this can be causal or not)
- It must have a causal impact on the dependent variable (i.e., influence the DV)
Some common examples of confounding variables include demographic factors such as gender, ethnicity, socioeconomic status, age, education level, and health status. In addition to these, there are also environmental factors to consider. For example, air pollution could confound the impact of the variables of interest in a study investigating health outcomes.
Naturally, it’s important to identify as many confounding variables as possible when conducting your research, as they can heavily distort the results and lead you to draw incorrect conclusions . So, always think carefully about what factors may have a confounding effect on your variables of interest and try to manage these as best you can.
What is a latent variable?
Latent variables are unobservable factors that can influence the behaviour of individuals and explain certain outcomes within a study. They’re also known as hidden or underlying variables , and what makes them rather tricky is that they can’t be directly observed or measured . Instead, latent variables must be inferred from other observable data points such as responses to surveys or experiments.
For example, in a study of mental health, the variable “resilience” could be considered a latent variable. It can’t be directly measured , but it can be inferred from measures of mental health symptoms, stress, and coping mechanisms. The same applies to a lot of concepts we encounter every day – for example:
- Emotional intelligence
- Quality of life
- Business confidence
- Ease of use
One way in which we overcome the challenge of measuring the immeasurable is latent variable models (LVMs). An LVM is a type of statistical model that describes a relationship between observed variables and one or more unobserved (latent) variables. These models allow researchers to uncover patterns in their data which may not have been visible before, thanks to their complexity and interrelatedness with other variables. Those patterns can then inform hypotheses about cause-and-effect relationships among those same variables which were previously unknown prior to running the LVM. Powerful stuff, we say!
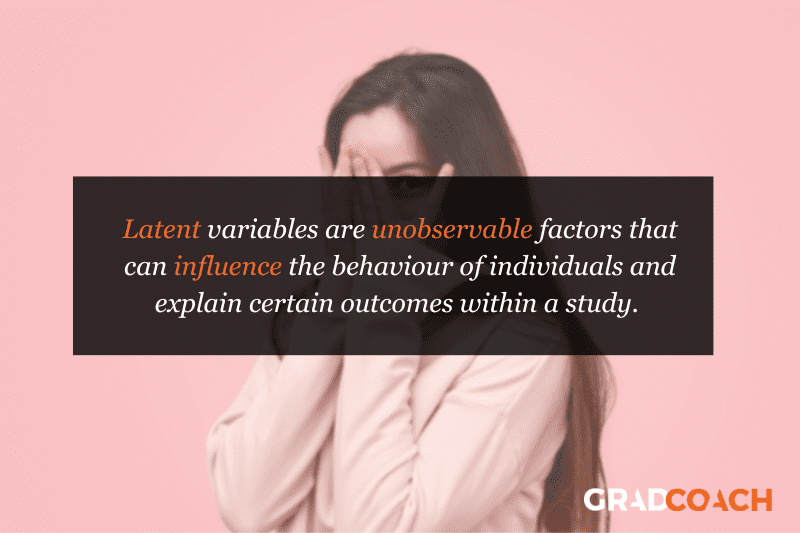
Let’s recap
In the world of scientific research, there’s no shortage of variable types, some of which have multiple names and some of which overlap with each other. In this post, we’ve covered some of the popular ones, but remember that this is not an exhaustive list .
To recap, we’ve explored:
- Independent variables (the “cause”)
- Dependent variables (the “effect”)
- Control variables (the variable that’s not allowed to vary)
If you’re still feeling a bit lost and need a helping hand with your research project, check out our 1-on-1 coaching service , where we guide you through each step of the research journey. Also, be sure to check out our free dissertation writing course and our collection of free, fully-editable chapter templates .
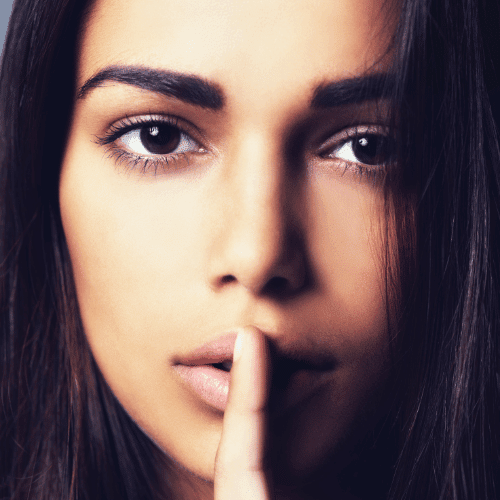
Psst... there’s more!
This post was based on one of our popular Research Bootcamps . If you're working on a research project, you'll definitely want to check this out ...
You Might Also Like:
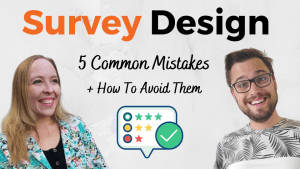
Very informative, concise and helpful. Thank you
Helping information.Thanks
practical and well-demonstrated
Very helpful and insightful
Submit a Comment Cancel reply
Your email address will not be published. Required fields are marked *
Save my name, email, and website in this browser for the next time I comment.
- Print Friendly
Have a language expert improve your writing
Run a free plagiarism check in 10 minutes, automatically generate references for free.
- Knowledge Base
- Methodology
- External Validity | Types, Threats & Examples
External Validity | Types, Threats & Examples
Published on 3 May 2022 by Pritha Bhandari . Revised on 18 December 2023.
External validity is the extent to which you can generalise the findings of a study to other situations, people, settings, and measures. In other words, can you apply the findings of your study to a broader context?
The aim of scientific research is to produce generalisable knowledge about the real world. Without high external validity, you cannot apply results from the laboratory to other people or the real world.
In qualitative studies , external validity is referred to as transferability.
Table of contents
Types of external validity, trade-off between external and internal validity, threats to external validity and how to counter them, frequently asked questions about external validity.
There are two main types of external validity: population validity and ecological validity.
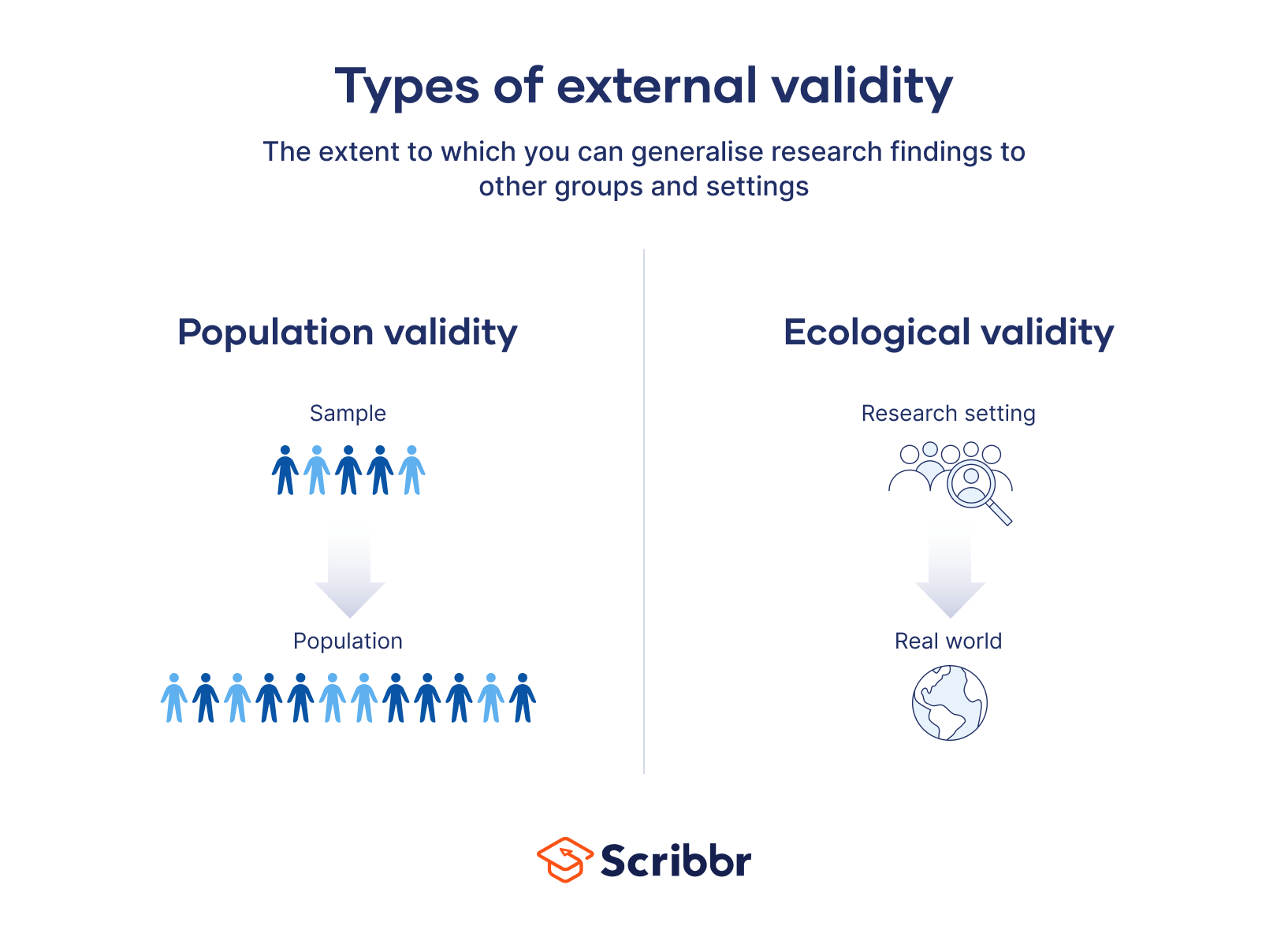
Population validity
Population validity refers to whether you can reasonably generalise the findings from your sample to a larger group of people (the population).
Population validity depends on the choice of population and on the extent to which the study sample mirrors that population. Non-probability sampling methods are often used for convenience. With this type of sampling, the generalisability of results is limited to populations that share similar characteristics with the sample.
You recruit over 200 participants. They are science and engineering students; most of them are British, male, 18–20 years old, and from a high socioeconomic background. In a laboratory setting, you administer a mathematics and science test and then ask them to rate how well they think performed. You find that the average participant believes they are smarter than 66% of their peers.
Here, your sample is not representative of the whole population of students at your university. The findings can only reasonably be generalised to populations that share characteristics with the participants, e.g. university-educated men studying STEM subjects.
For higher population validity, your sample would need to include people with different characteristics (e.g., women, nonbinary people, and students from different fields, countries, and socioeconomic backgrounds).
Samples like this one, from Western, educated, industrialised, rich, and democratic (WEIRD) countries, are used in an estimated 96% of psychology studies , even though they represent only 12% of the world’s population. As outliers in terms of visual perception, moral reasoning, and categorisation (among many other topics), WEIRD samples limit broad population validity in the social sciences.
Ecological validity
Ecological validity refers to whether you can reasonably generalise the findings of a study to other situations and settings in the ‘real world’.
In a laboratory setting, you set up a simple computer-based task to measure reaction times. Participants are told to imagine themselves driving around the racetrack and double-click the mouse whenever they see an orange cat on the screen. For one round, participants listen to a podcast. In the other round, they do not need to listen to anything.
In the example above, it is difficult to generalise the findings to real-life driving conditions. A computer-based task using a mouse does not resemble real-life driving conditions with a steering wheel. Additionally, a static image of an orange cat may not represent common real-life hurdles when driving.
To improve ecological validity in a lab setting, you could use an immersive driving simulator with a steering wheel and foot pedal instead of a computer and mouse. This increases psychological realism by more closely mirroring the experience of driving in the real world.
Alternatively, for higher ecological validity, you could conduct the experiment using a real driving course.
Prevent plagiarism, run a free check.
Internal validity is the extent to which you can be confident that the causal relationship established in your experiment cannot be explained by other factors.
There is an inherent trade-off between external and internal validity ; the more applicable you make your study to a broader context, the less you can control extraneous factors in your study.
Threats to external validity are important to recognise and counter in a research design for a robust study.
Participants are given a pretest and a post-test measuring how often they experienced anxiety in the past week. During the study, all participants are given an individual mindfulness training and asked to practise mindfulness daily for 15 minutes in the morning.
How to counter threats to external validity
There are several ways to counter threats to external validity:
- Replications counter almost all threats by enhancing generalisability to other settings, populations and conditions.
- Field experiments counter testing and situation effects by using natural contexts.
- Probability sampling counters selection bias by making sure everyone in a population has an equal chance of being selected for a study sample.
- Recalibration or reprocessing also counters selection bias using algorithms to correct weighting of factors (e.g., age) within study samples.
The external validity of a study is the extent to which you can generalise your findings to different groups of people, situations, and measures.
The two types of external validity are population validity (whether you can generalise to other groups of people) and ecological validity (whether you can generalise to other situations and settings).
There are seven threats to external validity : selection bias , history, experimenter effect, Hawthorne effect , testing effect, aptitude-treatment, and situation effect.
Attrition bias can skew your sample so that your final sample differs significantly from your original sample. Your sample is biased because some groups from your population are underrepresented.
With a biased final sample, you may not be able to generalise your findings to the original population that you sampled from, so your external validity is compromised.
Cite this Scribbr article
If you want to cite this source, you can copy and paste the citation or click the ‘Cite this Scribbr article’ button to automatically add the citation to our free Reference Generator.
Bhandari, P. (2023, December 18). External Validity | Types, Threats & Examples. Scribbr. Retrieved 6 May 2024, from https://www.scribbr.co.uk/research-methods/external-validity-explained/
Is this article helpful?
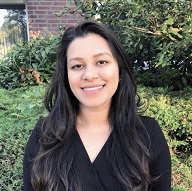
Pritha Bhandari
Other students also liked, the 4 types of validity | types, definitions & examples, internal validity | definition, threats & examples, reliability vs validity in research | differences, types & examples.
- Privacy Policy

Home » Variables in Research – Definition, Types and Examples
Variables in Research – Definition, Types and Examples
Table of Contents

Variables in Research
Definition:
In Research, Variables refer to characteristics or attributes that can be measured, manipulated, or controlled. They are the factors that researchers observe or manipulate to understand the relationship between them and the outcomes of interest.
Types of Variables in Research
Types of Variables in Research are as follows:
Independent Variable
This is the variable that is manipulated by the researcher. It is also known as the predictor variable, as it is used to predict changes in the dependent variable. Examples of independent variables include age, gender, dosage, and treatment type.
Dependent Variable
This is the variable that is measured or observed to determine the effects of the independent variable. It is also known as the outcome variable, as it is the variable that is affected by the independent variable. Examples of dependent variables include blood pressure, test scores, and reaction time.
Confounding Variable
This is a variable that can affect the relationship between the independent variable and the dependent variable. It is a variable that is not being studied but could impact the results of the study. For example, in a study on the effects of a new drug on a disease, a confounding variable could be the patient’s age, as older patients may have more severe symptoms.
Mediating Variable
This is a variable that explains the relationship between the independent variable and the dependent variable. It is a variable that comes in between the independent and dependent variables and is affected by the independent variable, which then affects the dependent variable. For example, in a study on the relationship between exercise and weight loss, the mediating variable could be metabolism, as exercise can increase metabolism, which can then lead to weight loss.
Moderator Variable
This is a variable that affects the strength or direction of the relationship between the independent variable and the dependent variable. It is a variable that influences the effect of the independent variable on the dependent variable. For example, in a study on the effects of caffeine on cognitive performance, the moderator variable could be age, as older adults may be more sensitive to the effects of caffeine than younger adults.
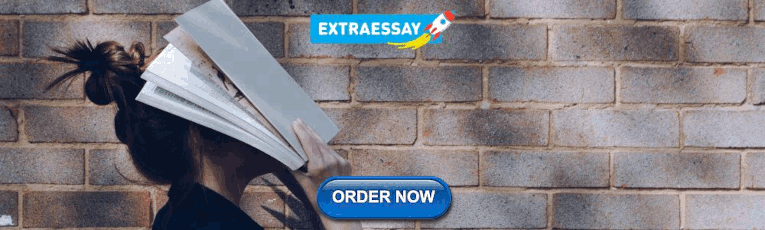
Control Variable
This is a variable that is held constant or controlled by the researcher to ensure that it does not affect the relationship between the independent variable and the dependent variable. Control variables are important to ensure that any observed effects are due to the independent variable and not to other factors. For example, in a study on the effects of a new teaching method on student performance, the control variables could include class size, teacher experience, and student demographics.
Continuous Variable
This is a variable that can take on any value within a certain range. Continuous variables can be measured on a scale and are often used in statistical analyses. Examples of continuous variables include height, weight, and temperature.
Categorical Variable
This is a variable that can take on a limited number of values or categories. Categorical variables can be nominal or ordinal. Nominal variables have no inherent order, while ordinal variables have a natural order. Examples of categorical variables include gender, race, and educational level.
Discrete Variable
This is a variable that can only take on specific values. Discrete variables are often used in counting or frequency analyses. Examples of discrete variables include the number of siblings a person has, the number of times a person exercises in a week, and the number of students in a classroom.
Dummy Variable
This is a variable that takes on only two values, typically 0 and 1, and is used to represent categorical variables in statistical analyses. Dummy variables are often used when a categorical variable cannot be used directly in an analysis. For example, in a study on the effects of gender on income, a dummy variable could be created, with 0 representing female and 1 representing male.
Extraneous Variable
This is a variable that has no relationship with the independent or dependent variable but can affect the outcome of the study. Extraneous variables can lead to erroneous conclusions and can be controlled through random assignment or statistical techniques.
Latent Variable
This is a variable that cannot be directly observed or measured, but is inferred from other variables. Latent variables are often used in psychological or social research to represent constructs such as personality traits, attitudes, or beliefs.
Moderator-mediator Variable
This is a variable that acts both as a moderator and a mediator. It can moderate the relationship between the independent and dependent variables and also mediate the relationship between the independent and dependent variables. Moderator-mediator variables are often used in complex statistical analyses.
Variables Analysis Methods
There are different methods to analyze variables in research, including:
- Descriptive statistics: This involves analyzing and summarizing data using measures such as mean, median, mode, range, standard deviation, and frequency distribution. Descriptive statistics are useful for understanding the basic characteristics of a data set.
- Inferential statistics : This involves making inferences about a population based on sample data. Inferential statistics use techniques such as hypothesis testing, confidence intervals, and regression analysis to draw conclusions from data.
- Correlation analysis: This involves examining the relationship between two or more variables. Correlation analysis can determine the strength and direction of the relationship between variables, and can be used to make predictions about future outcomes.
- Regression analysis: This involves examining the relationship between an independent variable and a dependent variable. Regression analysis can be used to predict the value of the dependent variable based on the value of the independent variable, and can also determine the significance of the relationship between the two variables.
- Factor analysis: This involves identifying patterns and relationships among a large number of variables. Factor analysis can be used to reduce the complexity of a data set and identify underlying factors or dimensions.
- Cluster analysis: This involves grouping data into clusters based on similarities between variables. Cluster analysis can be used to identify patterns or segments within a data set, and can be useful for market segmentation or customer profiling.
- Multivariate analysis : This involves analyzing multiple variables simultaneously. Multivariate analysis can be used to understand complex relationships between variables, and can be useful in fields such as social science, finance, and marketing.
Examples of Variables
- Age : This is a continuous variable that represents the age of an individual in years.
- Gender : This is a categorical variable that represents the biological sex of an individual and can take on values such as male and female.
- Education level: This is a categorical variable that represents the level of education completed by an individual and can take on values such as high school, college, and graduate school.
- Income : This is a continuous variable that represents the amount of money earned by an individual in a year.
- Weight : This is a continuous variable that represents the weight of an individual in kilograms or pounds.
- Ethnicity : This is a categorical variable that represents the ethnic background of an individual and can take on values such as Hispanic, African American, and Asian.
- Time spent on social media : This is a continuous variable that represents the amount of time an individual spends on social media in minutes or hours per day.
- Marital status: This is a categorical variable that represents the marital status of an individual and can take on values such as married, divorced, and single.
- Blood pressure : This is a continuous variable that represents the force of blood against the walls of arteries in millimeters of mercury.
- Job satisfaction : This is a continuous variable that represents an individual’s level of satisfaction with their job and can be measured using a Likert scale.
Applications of Variables
Variables are used in many different applications across various fields. Here are some examples:
- Scientific research: Variables are used in scientific research to understand the relationships between different factors and to make predictions about future outcomes. For example, scientists may study the effects of different variables on plant growth or the impact of environmental factors on animal behavior.
- Business and marketing: Variables are used in business and marketing to understand customer behavior and to make decisions about product development and marketing strategies. For example, businesses may study variables such as consumer preferences, spending habits, and market trends to identify opportunities for growth.
- Healthcare : Variables are used in healthcare to monitor patient health and to make treatment decisions. For example, doctors may use variables such as blood pressure, heart rate, and cholesterol levels to diagnose and treat cardiovascular disease.
- Education : Variables are used in education to measure student performance and to evaluate the effectiveness of teaching strategies. For example, teachers may use variables such as test scores, attendance, and class participation to assess student learning.
- Social sciences : Variables are used in social sciences to study human behavior and to understand the factors that influence social interactions. For example, sociologists may study variables such as income, education level, and family structure to examine patterns of social inequality.
Purpose of Variables
Variables serve several purposes in research, including:
- To provide a way of measuring and quantifying concepts: Variables help researchers measure and quantify abstract concepts such as attitudes, behaviors, and perceptions. By assigning numerical values to these concepts, researchers can analyze and compare data to draw meaningful conclusions.
- To help explain relationships between different factors: Variables help researchers identify and explain relationships between different factors. By analyzing how changes in one variable affect another variable, researchers can gain insight into the complex interplay between different factors.
- To make predictions about future outcomes : Variables help researchers make predictions about future outcomes based on past observations. By analyzing patterns and relationships between different variables, researchers can make informed predictions about how different factors may affect future outcomes.
- To test hypotheses: Variables help researchers test hypotheses and theories. By collecting and analyzing data on different variables, researchers can test whether their predictions are accurate and whether their hypotheses are supported by the evidence.
Characteristics of Variables
Characteristics of Variables are as follows:
- Measurement : Variables can be measured using different scales, such as nominal, ordinal, interval, or ratio scales. The scale used to measure a variable can affect the type of statistical analysis that can be applied.
- Range : Variables have a range of values that they can take on. The range can be finite, such as the number of students in a class, or infinite, such as the range of possible values for a continuous variable like temperature.
- Variability : Variables can have different levels of variability, which refers to the degree to which the values of the variable differ from each other. Highly variable variables have a wide range of values, while low variability variables have values that are more similar to each other.
- Validity and reliability : Variables should be both valid and reliable to ensure accurate and consistent measurement. Validity refers to the extent to which a variable measures what it is intended to measure, while reliability refers to the consistency of the measurement over time.
- Directionality: Some variables have directionality, meaning that the relationship between the variables is not symmetrical. For example, in a study of the relationship between smoking and lung cancer, smoking is the independent variable and lung cancer is the dependent variable.
Advantages of Variables
Here are some of the advantages of using variables in research:
- Control : Variables allow researchers to control the effects of external factors that could influence the outcome of the study. By manipulating and controlling variables, researchers can isolate the effects of specific factors and measure their impact on the outcome.
- Replicability : Variables make it possible for other researchers to replicate the study and test its findings. By defining and measuring variables consistently, other researchers can conduct similar studies to validate the original findings.
- Accuracy : Variables make it possible to measure phenomena accurately and objectively. By defining and measuring variables precisely, researchers can reduce bias and increase the accuracy of their findings.
- Generalizability : Variables allow researchers to generalize their findings to larger populations. By selecting variables that are representative of the population, researchers can draw conclusions that are applicable to a broader range of individuals.
- Clarity : Variables help researchers to communicate their findings more clearly and effectively. By defining and categorizing variables, researchers can organize and present their findings in a way that is easily understandable to others.
Disadvantages of Variables
Here are some of the main disadvantages of using variables in research:
- Simplification : Variables may oversimplify the complexity of real-world phenomena. By breaking down a phenomenon into variables, researchers may lose important information and context, which can affect the accuracy and generalizability of their findings.
- Measurement error : Variables rely on accurate and precise measurement, and measurement error can affect the reliability and validity of research findings. The use of subjective or poorly defined variables can also introduce measurement error into the study.
- Confounding variables : Confounding variables are factors that are not measured but that affect the relationship between the variables of interest. If confounding variables are not accounted for, they can distort or obscure the relationship between the variables of interest.
- Limited scope: Variables are defined by the researcher, and the scope of the study is therefore limited by the researcher’s choice of variables. This can lead to a narrow focus that overlooks important aspects of the phenomenon being studied.
- Ethical concerns: The selection and measurement of variables may raise ethical concerns, especially in studies involving human subjects. For example, using variables that are related to sensitive topics, such as race or sexuality, may raise concerns about privacy and discrimination.
About the author
Muhammad Hassan
Researcher, Academic Writer, Web developer
You may also like

Control Variable – Definition, Types and Examples

Moderating Variable – Definition, Analysis...

Categorical Variable – Definition, Types and...

Independent Variable – Definition, Types and...

Ratio Variable – Definition, Purpose and Examples

Ordinal Variable – Definition, Purpose and...
- Bipolar Disorder
- Therapy Center
- When To See a Therapist
- Types of Therapy
- Best Online Therapy
- Best Couples Therapy
- Best Family Therapy
- Managing Stress
- Sleep and Dreaming
- Understanding Emotions
- Self-Improvement
- Healthy Relationships
- Student Resources
- Personality Types
- Guided Meditations
- Verywell Mind Insights
- 2024 Verywell Mind 25
- Mental Health in the Classroom
- Editorial Process
- Meet Our Review Board
- Crisis Support
Types of Variables in Psychology Research
Examples of Independent and Dependent Variables
Kendra Cherry, MS, is a psychosocial rehabilitation specialist, psychology educator, and author of the "Everything Psychology Book."
:max_bytes(150000):strip_icc():format(webp)/IMG_9791-89504ab694d54b66bbd72cb84ffb860e.jpg)
James Lacy, MLS, is a fact-checker and researcher.
:max_bytes(150000):strip_icc():format(webp)/James-Lacy-1000-73de2239670146618c03f8b77f02f84e.jpg)
Dependent and Independent Variables
- Intervening Variables
- Extraneous Variables
- Controlled Variables
- Confounding Variables
- Operationalizing Variables
Frequently Asked Questions
Variables in psychology are things that can be changed or altered, such as a characteristic or value. Variables are generally used in psychology experiments to determine if changes to one thing result in changes to another.
Variables in psychology play a critical role in the research process. By systematically changing some variables in an experiment and measuring what happens as a result, researchers are able to learn more about cause-and-effect relationships.
The two main types of variables in psychology are the independent variable and the dependent variable. Both variables are important in the process of collecting data about psychological phenomena.
This article discusses different types of variables that are used in psychology research. It also covers how to operationalize these variables when conducting experiments.
Students often report problems with identifying the independent and dependent variables in an experiment. While this task can become more difficult as the complexity of an experiment increases, in a psychology experiment:
- The independent variable is the variable that is manipulated by the experimenter. An example of an independent variable in psychology: In an experiment on the impact of sleep deprivation on test performance, sleep deprivation would be the independent variable. The experimenters would have some of the study participants be sleep-deprived while others would be fully rested.
- The dependent variable is the variable that is measured by the experimenter. In the previous example, the scores on the test performance measure would be the dependent variable.
So how do you differentiate between the independent and dependent variables? Start by asking yourself what the experimenter is manipulating. The things that change, either naturally or through direct manipulation from the experimenter, are generally the independent variables. What is being measured? The dependent variable is the one that the experimenter is measuring.
Intervening Variables in Psychology
Intervening variables, also sometimes called intermediate or mediator variables, are factors that play a role in the relationship between two other variables. In the previous example, sleep problems in university students are often influenced by factors such as stress. As a result, stress might be an intervening variable that plays a role in how much sleep people get, which may then influence how well they perform on exams.
Extraneous Variables in Psychology
Independent and dependent variables are not the only variables present in many experiments. In some cases, extraneous variables may also play a role. This type of variable is one that may have an impact on the relationship between the independent and dependent variables.
For example, in our previous example of an experiment on the effects of sleep deprivation on test performance, other factors such as age, gender, and academic background may have an impact on the results. In such cases, the experimenter will note the values of these extraneous variables so any impact can be controlled for.
There are two basic types of extraneous variables:
- Participant variables : These extraneous variables are related to the individual characteristics of each study participant that may impact how they respond. These factors can include background differences, mood, anxiety, intelligence, awareness, and other characteristics that are unique to each person.
- Situational variables : These extraneous variables are related to things in the environment that may impact how each participant responds. For example, if a participant is taking a test in a chilly room, the temperature would be considered an extraneous variable. Some participants may not be affected by the cold, but others might be distracted or annoyed by the temperature of the room.
Other extraneous variables include the following:
- Demand characteristics : Clues in the environment that suggest how a participant should behave
- Experimenter effects : When a researcher unintentionally suggests clues for how a participant should behave
Controlled Variables in Psychology
In many cases, extraneous variables are controlled for by the experimenter. A controlled variable is one that is held constant throughout an experiment.
In the case of participant variables, the experiment might select participants that are the same in background and temperament to ensure that these factors don't interfere with the results. Holding these variables constant is important for an experiment because it allows researchers to be sure that all other variables remain the same across all conditions.
Using controlled variables means that when changes occur, the researchers can be sure that these changes are due to the manipulation of the independent variable and not caused by changes in other variables.
It is important to also note that a controlled variable is not the same thing as a control group . The control group in a study is the group of participants who do not receive the treatment or change in the independent variable.
All other variables between the control group and experimental group are held constant (i.e., they are controlled). The dependent variable being measured is then compared between the control group and experimental group to see what changes occurred because of the treatment.
Confounding Variables in Psychology
If a variable cannot be controlled for, it becomes what is known as a confounding variabl e. This type of variable can have an impact on the dependent variable, which can make it difficult to determine if the results are due to the influence of the independent variable, the confounding variable, or an interaction of the two.
Operationalizing Variables in Psychology
An operational definition describes how the variables are measured and defined in the study. Before conducting a psychology experiment , it is essential to create firm operational definitions for both the independent variable and dependent variables.
For example, in our imaginary experiment on the effects of sleep deprivation on test performance, we would need to create very specific operational definitions for our two variables. If our hypothesis is "Students who are sleep deprived will score significantly lower on a test," then we would have a few different concepts to define:
- Students : First, what do we mean by "students?" In our example, let’s define students as participants enrolled in an introductory university-level psychology course.
- Sleep deprivation : Next, we need to operationally define the "sleep deprivation" variable. In our example, let’s say that sleep deprivation refers to those participants who have had less than five hours of sleep the night before the test.
- Test variable : Finally, we need to create an operational definition for the test variable. For this example, the test variable will be defined as a student’s score on a chapter exam in the introductory psychology course.
Once all the variables are operationalized, we're ready to conduct the experiment.
Variables play an important part in psychology research. Manipulating an independent variable and measuring the dependent variable allows researchers to determine if there is a cause-and-effect relationship between them.
A Word From Verywell
Understanding the different types of variables used in psychology research is important if you want to conduct your own psychology experiments. It is also helpful for people who want to better understand what the results of psychology research really mean and become more informed consumers of psychology information .
Independent and dependent variables are used in experimental research. Unlike some other types of research (such as correlational studies ), experiments allow researchers to evaluate cause-and-effect relationships between two variables.
Researchers can use statistical analyses to determine the strength of a relationship between two variables in an experiment. Two of the most common ways to do this are to calculate a p-value or a correlation. The p-value indicates if the results are statistically significant while the correlation can indicate the strength of the relationship.
In an experiment on how sugar affects short-term memory, sugar intake would be the independent variable and scores on a short-term memory task would be the independent variable.
In an experiment looking at how caffeine intake affects test anxiety, the amount of caffeine consumed before a test would be the independent variable and scores on a test anxiety assessment would be the dependent variable.
Just as with other types of research, the independent variable in a cognitive psychology study would be the variable that the researchers manipulate. The specific independent variable would vary depending on the specific study, but it might be focused on some aspect of thinking, memory, attention, language, or decision-making.
American Psychological Association. Operational definition . APA Dictionary of Psychology.
American Psychological Association. Mediator . APA Dictionary of Psychology.
Altun I, Cınar N, Dede C. The contributing factors to poor sleep experiences in according to the university students: A cross-sectional study . J Res Med Sci . 2012;17(6):557-561. PMID:23626634
Skelly AC, Dettori JR, Brodt ED. Assessing bias: The importance of considering confounding . Evid Based Spine Care J . 2012;3(1):9-12. doi:10.1055/s-0031-1298595
- Evans, AN & Rooney, BJ. Methods in Psychological Research. Thousand Oaks, CA: SAGE Publications; 2014.
- Kantowitz, BH, Roediger, HL, & Elmes, DG. Experimental Psychology. Stamfort, CT: Cengage Learning; 2015.
By Kendra Cherry, MSEd Kendra Cherry, MS, is a psychosocial rehabilitation specialist, psychology educator, and author of the "Everything Psychology Book."
Our websites may use cookies to personalize and enhance your experience. By continuing without changing your cookie settings, you agree to this collection. For more information, please see our University Websites Privacy Notice .
Neag School of Education
Educational Research Basics by Del Siegle
External validity.
Note to EPSY 5601 Students: An understanding of the difference between population and ecological validity is sufficient. Mastery of the sub categories for each is not necessary for this course.
External Validity (Generalizability) –to whom can the results of the study be applied–
There are two types of study validity: internal (more applicable with experimental research) and external. This section covers external validity.
External validity involves the extent to which the results of a study can be generalized (applied) beyond the sample. In other words, can you apply what you found in your study to other people (population validity) or settings (ecological validity). A study of fifth graders in a rural school that found one method of teaching spelling was superior to another may not be applicable with third graders (population) in an urban school (ecological).
Threats to External Validity
Population Validity the extent to which the results of a study can be generalized from the specific sample that was studied to a larger group of subjects
- the extent to which one can generalize from the study sample to a defined population– If the sample is drawn from an accessible population, rather than the target population, generalizing the research results from the accessible population to the target population is risky. 2. the extent to which personological variables interact with treatment effects– If the study is an experiment, it may be possible that different results might be found with students at different grades (a personological variable).
Ecological Validity the extent to which the results of an experiment can be generalized from the set of environmental conditions created by the researcher to other environmental conditions (settings and conditions).
- Explicit description of the experimental treatment (not sufficiently described for others to replicate) If the researcher fails to adequately describe how he or she conducted a study, it is difficult to determine whether the results are applicable to other settings.
- Multiple-treatment interference (catalyst effect) If a researcher were to apply several treatments, it is difficult to determine how well each of the treatments would work individually. It might be that only the combination of the treatments is effective.
- Hawthorne effect (attention causes differences) Subjects perform differently because they know they are being studied. “…External validity of the experiment is jeopardized because the findings might not generalize to a situation in which researchers or others who were involved in the research are not present” (Gall, Borg, & Gall, 1996, p. 475)
- Novelty and disruption effect (anything different makes a difference) A treatment may work because it is novel and the subjects respond to the uniqueness, rather than the actual treatment. The opposite may also occur, the treatment may not work because it is unique, but given time for the subjects to adjust to it, it might have worked.
- Experimenter effect (it only works with this experimenter) The treatment might have worked because of the person implementing it. Given a different person, the treatment might not work at all.
- Pretest sensitization (pretest sets the stage) A treatment might only work if a pretest is given. Because they have taken a pretest, the subjects may be more sensitive to the treatment. Had they not taken a pretest, the treatment would not have worked.
- Posttest sensitization (posttest helps treatment “fall into place”) The posttest can become a learning experience. “For example, the posttest might cause certain ideas presented during the treatment to ‘fall into place’ ” (p. 477). If the subjects had not taken a posttest, the treatment would not have worked.
- Interaction of history and treatment effec t (…to everything there is a time…) Not only should researchers be cautious about generalizing to other population, caution should be taken to generalize to a different time period. As time passes, the conditions under which treatments work change.
- Measurement of the dependent variable (maybe only works with M/C tests) A treatment may only be evident with certain types of measurements. A teaching method may produce superior results when its effectiveness is tested with an essay test, but show no differences when the effectiveness is measured with a multiple choice test.
- Interaction of time of measurement and treatment effect (it takes a while for the treatment to kick in) It may be that the treatment effect does not occur until several weeks after the end of the treatment. In this situation, a posttest at the end of the treatment would show no impact, but a posttest a month later might show an impact.
Bracht, G. H., & Glass, G. V. (1968). The external validity of experiments. American Education Research Journal, 5, 437-474. Gall, M. D., Borg, W. R., & Gall, J. P. (1996). Educational research: An introduction. White Plains, NY: Longman.
Del Siegle, Ph.D. Neag School of Education – University of Connecticut [email protected] www.delsiegle.com
Internal vs. External Validity In Psychology
Julia Simkus
Editor at Simply Psychology
BA (Hons) Psychology, Princeton University
Julia Simkus is a graduate of Princeton University with a Bachelor of Arts in Psychology. She is currently studying for a Master's Degree in Counseling for Mental Health and Wellness in September 2023. Julia's research has been published in peer reviewed journals.
Learn about our Editorial Process
Saul Mcleod, PhD
Editor-in-Chief for Simply Psychology
BSc (Hons) Psychology, MRes, PhD, University of Manchester
Saul Mcleod, PhD., is a qualified psychology teacher with over 18 years of experience in further and higher education. He has been published in peer-reviewed journals, including the Journal of Clinical Psychology.
Olivia Guy-Evans, MSc
Associate Editor for Simply Psychology
BSc (Hons) Psychology, MSc Psychology of Education
Olivia Guy-Evans is a writer and associate editor for Simply Psychology. She has previously worked in healthcare and educational sectors.
Internal validity centers on demonstrating clear casual relationships within the bounds of a specific study and external validity relates to demonstrating the applicability of findings beyond that original study situation or population.
Researchers have to weigh these considerations in designing methodologically rigorous and generalizable studies.

Internal Validity
Internal validity refers to the degree of confidence that the causal relationship being tested exists and is trustworthy.
It tests how likely it is that your treatment caused the differences in results that you observe. Internal validity is largely determined by the study’s experimental design and methods .
Studies that have a high degree of internal validity provide strong evidence of causality, so it makes it possible to eliminate alternative explanations for a finding.
Studies with low internal validity provide weak evidence of causality. The less chance there is for confounding or extraneous variables , the higher the internal validity and the more confident we can be in our findings.
In order to assume cause and effect in a research study, the cause must precede the effect in terms of time, the cause and effect must vary together, and there must be no other explanations for the relationship observed. If these three criteria are observed, you can be confident that a study is internally valid.
An example of a study with high internal validity would be if you wanted to run an experiment to see if using a particular weight-loss pill will help people lose weight.
To test this hypothesis, you would randomly assign a sample of participants to one of two groups: those who will take the weight-loss pill and those who will take a placebo pill.
You can ensure that there is no bias in how participants are assigned to the groups by blinding the research assistants , so they don’t know which participants are in which groups during the experiment. The participants are also blinded, so they do not know whether they are receiving the intervention or not.
If participants drop out of the study, their characteristics are examined to ensure there is no systematic bias regarding who left.
It is important to have a well-thought-out research procedure to mitigate the threats to internal validity.
External Validity
External validity refers to the extent to which the results of a research study can be applied or generalized to another context.
This is important because if external validity is established, the studies’ findings can be generalized to a larger population as opposed to only the relatively few subjects who participated in the study. Unlike internal validity, external validity doesn’t assess causality or rule out confounders.
There are two types of external validity: ecological validity and population validity.
- Ecological validity refers to whether a study’s findings can be generalized to other situations or settings. A high ecological validity means that there is a high degree of similarity between the experimental setting and another setting, and thus we can be confident that the results will generalize to that other setting.
- Population validity refers to how well the experimental sample represents other populations or groups. Using random sampling techniques , such as stratified sampling or cluster sampling, significantly helps increase population validity.
An example of a study with high external validity would be if you hypothesize that practicing mindfulness two times per week will improve the mental health of those diagnosed with depression.
You recruit people who have been diagnosed with depression for at least a year and are between 18–29 years old. Choosing this representative sample with a clearly defined population of interest helps ensure external validity.
You give participants a pre-test and a post-test measuring how often they experienced symptoms of depression in the past week.
During the study, all participants were given individual mindfulness training and asked to practice mindfulness daily for 15 minutes as part of their morning routine.
You can also replicate the study’s results using different methods of mindfulness or different samples of participants.
Trade-off Between Internal and External Validity
There tends to be a negative correlation between internal and external validity in experimental research. This means that experiments that have high internal validity will likely have low external validity and vice versa.
This happens because experimental conditions that produce higher degrees of internal validity (e.g., artificial labs) tend to be highly unlikely to match real-world conditions. So, the external validity is weaker because a lab environment is much different than the real world.
On the other hand, to produce higher degrees of external validity, you want experimental conditions that match a real-world setting (e.g., observational studies ).
However, this comes at the expense of internal validity because these types of studies increase the likelihood of confounding variables and alternative explanations for differences in outcomes.
A solution to this trade-off is replication! You want to conduct the research in multiple environments and settings – first in a controlled, artificial environment to establish the existence of a causal relationship and then in a “real-world” setting to analyze if the results are generalizable.
Threats to Internal Validity
Attrition refers to the loss of study participants over time. Participants might drop out or leave the study which means that the results are based solely on a biased sample of only the people who did not choose to leave.
Differential rates of attrition between treatment and control groups can skew results by affecting the relationship between your independent and dependent variables and thus affect the internal validity of a study.
Confounders
A confounding variable is an unmeasured third variable that influences, or “confounds,” the relationship between an independent and a dependent variable by suggesting the presence of a spurious correlation.
Confounders are threats to internal validity because you can’t tell whether the predicted independent variable causes the outcome or if the confounding variable causes it.
Participant Selection Bias
This is a bias that may result from the selection or assignment of study groups in such a way that proper randomization is not achieved.
If participants are not randomly assigned to groups, the sample obtained might not be representative of the population intended to be studied. For example, some members of a population might be less likely to be included than others due to motivation, willingness to take part in the study, or demographics.
Experimenter Bias
Experimenter bias occurs when an experimenter behaves in a different way with different groups in a study, impacting the results and threatening internal validity. This can be eliminated through blinding.
Social Interaction (Diffusion)
Diffusion refers to when the treatment in research spreads within or between treatment and control groups. This can happen when there is interaction or observation among the groups.
Diffusion poses a threat to internal validity because it can lead to resentful demoralization. This is when the control group is less motivated because they feel resentful over the group that they are in.
Historical Events
Historical events might influence the outcome of studies that occur over longer periods of time. For example, changes in political leadership, natural disasters, or other unanticipated events might change the conditions of the study and influence the outcomes.
Instrumentation
Instrumentation refers to any change in the dependent variable in a study that arises from changes in the measuring instrument used. This happens when different measures are used in the pre-test and post-test phases.
Maturation refers to the impact of time on a study. If the outcomes of the study vary as a natural result of time, it might not be possible to determine whether the effects seen in the study were due to the study treatment or simply due to the impact of time.
Statistical Regression
Regression to the mean refers to the fact that if one sample of a random variable is extreme, the next sampling of the same random variable is likely going to be closer to its mean.
This is a threat to internal validity as participants at extreme ends of treatment can naturally fall in a certain direction due to the passage of time rather than being a direct effect of an intervention.
Repeated Testing
Testing your research participants repeatedly with the same measures will influence your research findings because participants will become more accustomed to the testing. Due to familiarity, or awareness of the study’s purpose, many participants might achieve better results over time.
Threats to External Validity
Sample features.
If some feature(s) of the sample used were responsible for the effect, this could lead to limited generalizability of the findings.
This is a bias that may result from the selection or assignment of study groups in such a way that proper randomization is not achieved. If participants are not randomly assigned to groups, the sample obtained might not be representative of the population intended to be studied.
For example, some members of a population might be less likely to be included than others due to motivation, willingness to take part in the study, or demographics.
Situational Factors
Factors such as the setting, time of day, location, researchers’ characteristics, noise, or the number of measures might affect the generalizability of the findings.
Aptitude-Treatment Interaction → Aptitude-Treatment Interaction to the concept that some treatments are more or less effective for particular individuals depending upon their specific abilities or characteristics.
Hawthorne Effect
The Hawthorne Effect refers to the tendency for participants to change their behaviors simply because they know they are being studied.
Experimenter Effect
Experimenter bias occurs when an experimenter behaves in a different way with different groups in a study, impacting the results and threatening the external validity.
John Henry Effect
The John Henry Effect refers to the tendency for participants in a control group to actively work harder because they know they are in an experiment and want to overcome the “disadvantage” of being in the control group.
Factors that Improve Internal Validity
Blinding refers to a practice where the participants (and sometimes the researchers) are unaware of what intervention they are receiving.
This reduces the influence of extraneous factors and minimizes bias, as any differences in outcome can thus be linked to the intervention and not to the participant’s knowledge of whether they were receiving a new treatment or not.
Random Sampling
Using random sampling to obtain a sample that represents the population that you wish to study will improve internal validity.
Random Assignment
Using random assignment to assign participants to control and treatment groups ensures that there is no systematic bias among the research groups.
Strict Study Protocol
Highly controlled experiments tend to improve internal validity. Experiments that occur in lab settings tend to have higher validity as this reduces variability from sources other than the treatment.
Experimental Manipulation
Manipulating an independent variable in a study as opposed to just observing an association without conducting an intervention improves internal validity.
Factors that Improve External Validity
Replication.
Conducting a study more than once with a different sample or in a different setting to see if the results will replicate can help improve external validity.
If multiple studies have been conducted on the same topic, a meta-analysis can be used to determine if the effect of an independent variable can be replicated, thus making it more reliable.
Replication is the strongest method to counter threats to external validity by enhancing generalizability to other settings, populations, and conditions.
Field Experiments
Conducting a study outside the laboratory, in a natural, real-world setting will improve external validity (however, this will threaten the internal validity)
Probability Sampling
Using probability sampling will counter selection bias by making sure everyone in a population has an equal chance of being selected for a study sample.
Recalibration
Recalibration is the use of statistical methods to maintain accuracy, standardization, and repeatability in measurements to assure reliable results.
Reweighting groups, if a study had uneven groups for a particular characteristic (such as age), is an example of calibration.
Inclusion and Exclusion Criteria
Setting criteria as to who can be involved in the research and who cannot be involved will ensure that the population being studied is clearly defined and that the sample is representative of the population.
Psychological Realism
Psychological realism refers to the process of making sure participants perceive the experimental manipulations as real events so as to not reveal the purpose of the study and so participants don’t behave differently than they would in real life based on knowing the study’s goal.

Posted May 6, 2024
At 4:38 PM UTC
The big DOJ antitrust trial over Google Search revealed last week that Big G pays Apple $20 billion a year to be the default search on iOS. That’s over 20 percent of the “services” revenue Tim Cook loves to talk about on earnings calls , but hey, where is all that money on the balance sheet?
BI’s Peter Kafka found out: Apple categorizes it as “advertising.”
[ Business Insider ]
The best new browser for Windows
Better siri is coming: what apple’s research says about its ai plans, bluesky confirms jack dorsey is no longer on its board, the eta aquarid meteor shower peaks tonight — here’s how to see it, tesla plans to charge some model y owners to unlock more range, more from this stream us v. google: all the news from the search antitrust showdown, as google’s antitrust trial wraps, doj seeks sanctions over missing messages, multibillion-dollar apple deal looms large in google antitrust trial, google paid apple $20 billion in 2022 to be safari’s default search engine., a google witness let slip just how much it pays apple for safari search.
- Share full article
Advertisement
Supported by
Canadian Arrests Highlight Alleged Gang Role in India’s Intelligence Operations
India’s external spy agency, the Research and Analysis Wing, has long been accused of tapping into criminal networks to carry out operations in South Asia. Is the agency now doing similar operations in the West?

By Mujib Mashal and Suhasini Raj
Reporting from New Delhi
Months after Prime Minister Justin Trudeau of Canada accused India’s government of plotting a murder on Canadian soil — plunging diplomatic relations between the two countries to their lowest level ever — the first arrests in the killing, which came on Friday, did little to demystify the basis of his claim.
The police didn’t offer clues or present any evidence that India had orchestrated the killing of Hardeep Singh Nijjar, a Sikh nationalist leader who was gunned down at the temple he led in Surrey, British Columbia, in June. What they did say was that three Indian men had committed the killing and that an investigation into India’s role was ongoing.
Before the arrests, Indian officials had maintained that Canada was trying to drag New Delhi into what it described as essentially a rivalry between gangs whose members were long wanted for crimes back in India.
After the arrests, a report from the CBC, Canada’s public broadcasting corporation , based on anonymous sources, also said the suspects belonged to an Indian criminal gang.
But analysts and former officials said that the possible role of a gang in the killing does not necessarily mean the Indian government was not involved in the crime.
India’s external spy agency, the Research and Analysis Wing, or RAW, has long been suspected of tapping into criminal networks to carry out operations in its immediate neighborhood in South Asia while maintaining deniability.
Canada’s accusation, if proven, that India orchestrated the Nijjar killing — and a similar accusation made soon after by the United States in a different case — may suggest that RAW is now extending its playbook of working with criminals to carry out operations in Western countries, analysts said.
U.S. officials have produced strong evidence in their accusation that an agent of the Indian government participated in a foiled attempt to assassinate a dual American-Canadian citizen. And Canada and allied officials have maintained that Canada has evidence supporting Mr. Trudeau’s claim that Indian agents carried out Mr. Nijjar’s killing.
But the Canadian failure to reveal any evidence that India took part, nine months after Mr. Trudeau’s explosive allegation, leaves the killing of Mr. Nijjar in the realm of accusations and counter-accusation in what is a highly tense political environment in both countries, analysts said.
Prime Minister Narendra Modi has been flexing his muscles as a nationalist strongman, pitching himself during his ongoing campaign for a third-term in office as a protector of India who would go as far as it takes to target security threats.
During speeches, he has boasted about how his government eliminates enemies by “descending in their homes.” While he has made those references in relation to the country’s archenemy — Pakistan — right wing accounts on social media had celebrated the slaying of Mr. Nijjar in Canada as a similar reach of Mr. Modi’s long arm.
Mr. Trudeau, on the other hand, had been facing criticism of weakness in the face of Chinese election interference activities on Canadian soil, and his getting ahead of the Nijjar killing was seen as compensating for that.
Canadian police announced on Friday that they had arrested the three Indian men in Edmonton, Alberta, the same day and charged them with first-degree murder and conspiracy to commit murder in the killing of Mr. Nijjar. The suspects had been living in Canada for three to five years but were not permanent residents of Canada, the police said.
The gang that the CBC reported that the hit-men are connected to is led by Lawrence Bishnoi, 31, who is accused of several cases of murder, extortion and narcotics trafficking. He has orchestrated much of it from an Indian jail, where he has been held since 2014 . His members are seen as being behind the murder of a popular Punjabi rapper, and threats of attacks on Bollywood celebrities.
Indian security officials have frequently arrested criminals connected to Mr. Bishnoi, often with allegations that the gang’s network stretched as far as Canada and overlapped with those promoting from Canadian soil the cause of Khalistan, a once deeply violent separatist movement with the goal of carving out the Indian state of Punjab as an independent nation.
A large Sikh diaspora resides in Canada, many of them having migrated there after a violent and often indiscriminate crackdown by the Indian government in the 1980s against the movement for an independent Khalistan. While the cause has largely died down inside India, it continues to have supporters among some segments of the diaspora. The Indian government has accused Canada, and several other Western countries, of not doing enough to crack down on the separatists.
Analysts and former security officials said that in India’s immediate geographic neighborhood, RAW has often been willing to venture into murky spaces to recruit killers. Senior officials of Mr. Modi’s administration, including Ajit Doval, the storied former spymaster who now serves as his longtime national security adviser, have in the past been accused of reaching into the underworld to find hit men willing to go after targets both inside the country as well as abroad.
Mr. Bishnoi has demonstrated enormous power from behind bars, even giving a television interview from jail last year to pitch himself as a nationalist warrior rather than a criminal mastermind. That, one former security official said, was a signal of his trying to align himself with the spirit of nationalism for a potential deal.
“I am a nationalist,” Mr. Bishnoi said in that interview. “I am against Khalistan. I am against Pakistan.”
Ajai Sahni, a security analyst who runs the South Asia Terrorism Portal in New Delhi, said the exploitation of criminal gangs by spy agencies to carry out operations with deniability was something that “happens all over the world.”
“It is definitely possible for agencies like RAW to use gang rivalries instead of exposing their own covert operators,” Mr. Sahni added. “But just because that is generally how one would expect it to be done, it doesn’t necessarily mean we know this is exactly the case in Nijjar’s killing.”
The failed plot on American soil had some of the sloppy hallmarks of an agency trying to extend an old playbook into a different, unfamiliar space.
A U.S. indictment in November laid out evidence, including electronic communication and cash transactions between the hired hit man — who turned out to be an undercover cop — a boastful middleman, and an Indian intelligence handler whom The Washington Post recently identified as Vikram Yadav .
The Indian government’s response suggested worry: India’s top diplomat said the action was not government policy, while the government announced an investigation into the matter and promised cooperation with the United States.
Canada’s case has played out very differently. The country has not publicly disclosed any evidence backing up Mr. Trudeau’s clam, even as allied officials said in September that Canadian officials had found a “smoking gun”: intercepted communications of Indian diplomats in Canada indicating involvement in the plot.
Indian officials have pushed back against Mr. Trudeau’s claims with the kind of aggression that suggested it either wasn’t involved or that it was confident of its deniability.
The Indian government expelled Canadian diplomats , and doubled down by putting out a list of individuals on Canadian soil that it said were long wanted as part of what it described as a crime and terror nexus.
Last week, officials in Mr. Modi’s government jumped on scenes of an event that Mr. Trudeau had attended to say it showed his accusations were simply to appease what they say is a Sikh vote bank for him. They pointed to videos of an event where Mr. Trudeau was the chief guest and where chants of “long live Khalistan” were shouted. Mr. Trudeau, in his speech, said he will always be there “to protect your rights and your freedoms, and we will always defend your community against hatred.”
After the speech, the Indian foreign ministry summoned Canada’s second highest ranking diplomat in New Delhi to lodge a complaint.
“His remarks to us illustrates once again the kind of political space that has been given in Canada to separatism, extremism and people who practice violence,” Randhir Jaiswal, the foreign ministry spokesman, said at a news conference.
Mujib Mashal is the South Asia bureau chief for The Times, helping to lead coverage of India and the diverse region around it, including Bangladesh, Sri Lanka, Nepal and Bhutan. More about Mujib Mashal
Suhasini Raj is a reporter based in New Delhi who has covered India for The Times since 2014. More about Suhasini Raj
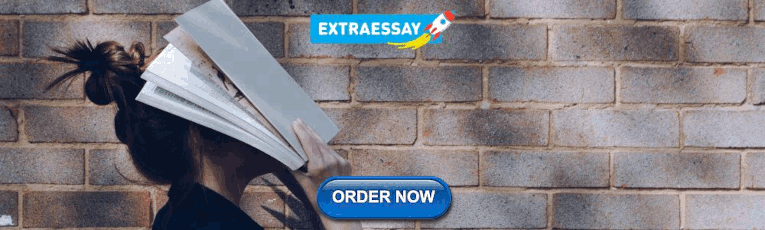
COMMENTS
A confounding variable is a type of extraneous variable that is associated with both the independent and dependent variables. An extraneous variable is anything that could influence the dependent variable. A confounding variable influences the dependent variable, and also correlates with or causally affects the independent variable.
2. Participant Variable. This refers to the ways in which each participant varies from the other and how this could affect the results, e.g., mood, intelligence, anxiety, nerves, concentration, etc. For example, if a participant that has performed a memory test was tired, dyslexic, or had poor eyesight, this could affect their performance and ...
Examples. Discrete variables (aka integer variables) Counts of individual items or values. Number of students in a class. Number of different tree species in a forest. Continuous variables (aka ratio variables) Measurements of continuous or non-finite values. Distance.
An extraneous variable is any variable you're not interested in studying that could also have some effect on the dependent variable. ... This refers to any clues in the experiment that could give away the true purpose of the research to the individuals. Examples include: Their surroundings. Their interpretation of what is happening in the ...
Here are some examples of extraneous variables: Age: Age is a common extraneous variable that can affect many different types of studies. For example, if a study is examining the effects of a new drug on blood pressure, age may be an extraneous variable that needs to be controlled. Older individuals tend to have higher blood pressure, so ...
A variable in research is a characteristic, number, or quantity that may assume different values. Variables may be: Dependent. Independent. Intervening. Moderating. Quantitative. Qualitative. Composite. Confounding. Extraneous. An extraneous variable is any variable not being investigated that has the potential to affect the outcome of a research study.
Trade-off between external and internal validity. Internal validity is the extent to which you can be confident that the causal relationship established in your experiment cannot be explained by other factors.. There is an inherent trade-off between external and internal validity; the more applicable you make your study to a broader context, the less you can control extraneous factors in your ...
External Validity. At the same time, the way that experiments are conducted sometimes leads to a different kind of criticism. Specifically, the need to manipulate the independent variable and control extraneous variables means that experiments are often conducted under conditions that seem artificial or unlike "real life" (Stanovich, 2010).
A confounding variable (also known as a third variable or lurking variable) is an extraneous factor that can influence the relationship between two variables being studied. Specifically, for a variable to be considered a confounding variable, it needs to meet two criteria: It must be correlated with the independent variable (this can be causal ...
External validity is the extent to which you can generalise the findings of a study to other situations, people, settings and measures. ... Interactions between characteristics of the group and individual variables together influence the dependent variable. Interactions between certain characteristics of the participants with depression (e.g ...
The Role of Variables in Research. In scientific research, variables serve several key functions: Define Relationships: Variables allow researchers to investigate the relationships between different factors and characteristics, providing insights into the underlying mechanisms that drive phenomena and outcomes. Establish Comparisons: By manipulating and comparing variables, scientists can ...
Categorical Variable. This is a variable that can take on a limited number of values or categories. Categorical variables can be nominal or ordinal. Nominal variables have no inherent order, while ordinal variables have a natural order. Examples of categorical variables include gender, race, and educational level.
The essential difference between internal validity and external validity is that internal validity refers to the structure of a study (and its variables) while external validity refers to the universality of the results. But there are further differences between the two as well. For instance, internal validity focuses on showing a difference ...
The two main types of variables in psychology are the independent variable and the dependent variable. Both variables are important in the process of collecting data about psychological phenomena. This article discusses different types of variables that are used in psychology research. It also covers how to operationalize these variables when ...
External Validity. (Generalizability) -to whom can the results of the study be applied-. There are two types of study validity: internal (more applicable with experimental research) and external. This section covers external validity. External validity involves the extent to which the results of a study can be generalized (applied) beyond ...
There tends to be a negative correlation between internal and external validity in experimental research. This means that experiments that have high internal validity will likely have low external validity and vice versa. ... A confounding variable is an unmeasured third variable that influences, or "confounds," the relationship between an ...
INTRODUCTION. Scientific research is usually initiated by posing evidenced-based research questions which are then explicitly restated as hypotheses.1,2 The hypotheses provide directions to guide the study, solutions, explanations, and expected results.3,4 Both research questions and hypotheses are essentially formulated based on conventional theories and real-world processes, which allow the ...
Internal and external validity are two ways of testing cause-and-effect relationships. Internal validity refers to the degree of confidence that the causal relationship being tested is trustworthy and not influenced by other factors or variables. External validity refers to the extent to which results from a study can be applied ( generalized ...
The validity of a research study includes two domains: internal and external validity. Internal validity is defined as the extent to which the observed results represent the truth in the population we are studying and, thus, are not due to methodological errors. In our example, if the authors can support that the study has internal validity ...
Variables in Research. The definition of a variable in the context of a research study is some feature with the potential to change, typically one that may influence or reflect a relationship or ...
An extraneous variable is also known as a confounding or external variable. These terms are interchangeable in many instances and they refer to something that emerges unexpectedly during the course of a study or experiment which may affect the results. These variables primarily affect the dependent variables within an experiment.
The big DOJ antitrust trial over Google Search revealed last week that Big G pays Apple $20 billion a year to be the default search on iOS. That's over 20 percent of the "services" revenue ...
Apetoh's research at the Brown Center is part of his ongoing investigation on T-cells, which are pivotal players in the body's immune response against disease, notably cancer. He and his fellow researchers are trying to improve the efficacy and power of these white blood cells to make chemotherapy more effective and to better predict how ...
In research that investigates a potential cause-and-effect relationship, a confounding variable is an unmeasured third variable that influences both the supposed cause and the supposed effect. It's important to consider potential confounding variables and account for them in your research design to ensure your results are valid .
Nearly 19,000 students from nine Indiana University campuses across the state are about to graduate, 70% of whom are earning bachelor's degrees. Many of them started during the height of the COVID-19 pandemic. Despite these challenges, almost 1,000 students are graduating with a 4.0 GPA. Five undergrads share how their experiences have set ...
India's external spy agency, the Research and Analysis Wing, has long been accused of tapping into criminal networks to carry out operations in South Asia. Is the agency now doing similar ...