- Resources Home 🏠
- Try SciSpace Copilot
- Search research papers
- Add Copilot Extension
- Try AI Detector
- Try Paraphraser
- Try Citation Generator
- April Papers
- June Papers
- July Papers

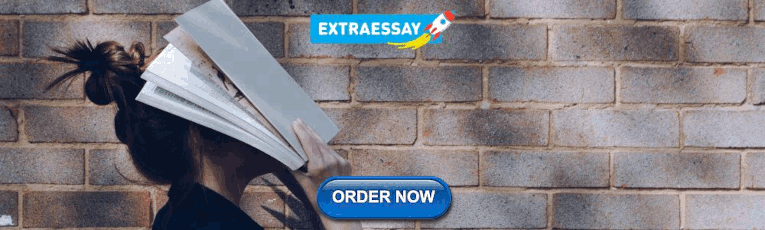
What is a thesis | A Complete Guide with Examples

Table of Contents
A thesis is a comprehensive academic paper based on your original research that presents new findings, arguments, and ideas of your study. It’s typically submitted at the end of your master’s degree or as a capstone of your bachelor’s degree.
However, writing a thesis can be laborious, especially for beginners. From the initial challenge of pinpointing a compelling research topic to organizing and presenting findings, the process is filled with potential pitfalls.
Therefore, to help you, this guide talks about what is a thesis. Additionally, it offers revelations and methodologies to transform it from an overwhelming task to a manageable and rewarding academic milestone.
What is a thesis?
A thesis is an in-depth research study that identifies a particular topic of inquiry and presents a clear argument or perspective about that topic using evidence and logic.
Writing a thesis showcases your ability of critical thinking, gathering evidence, and making a compelling argument. Integral to these competencies is thorough research, which not only fortifies your propositions but also confers credibility to your entire study.
Furthermore, there's another phenomenon you might often confuse with the thesis: the ' working thesis .' However, they aren't similar and shouldn't be used interchangeably.
A working thesis, often referred to as a preliminary or tentative thesis, is an initial version of your thesis statement. It serves as a draft or a starting point that guides your research in its early stages.
As you research more and gather more evidence, your initial thesis (aka working thesis) might change. It's like a starting point that can be adjusted as you learn more. It's normal for your main topic to change a few times before you finalize it.
While a thesis identifies and provides an overarching argument, the key to clearly communicating the central point of that argument lies in writing a strong thesis statement.
What is a thesis statement?
A strong thesis statement (aka thesis sentence) is a concise summary of the main argument or claim of the paper. It serves as a critical anchor in any academic work, succinctly encapsulating the primary argument or main idea of the entire paper.
Typically found within the introductory section, a strong thesis statement acts as a roadmap of your thesis, directing readers through your arguments and findings. By delineating the core focus of your investigation, it offers readers an immediate understanding of the context and the gravity of your study.
Furthermore, an effectively crafted thesis statement can set forth the boundaries of your research, helping readers anticipate the specific areas of inquiry you are addressing.
Different types of thesis statements
A good thesis statement is clear, specific, and arguable. Therefore, it is necessary for you to choose the right type of thesis statement for your academic papers.
Thesis statements can be classified based on their purpose and structure. Here are the primary types of thesis statements:
Argumentative (or Persuasive) thesis statement
Purpose : To convince the reader of a particular stance or point of view by presenting evidence and formulating a compelling argument.
Example : Reducing plastic use in daily life is essential for environmental health.
Analytical thesis statement
Purpose : To break down an idea or issue into its components and evaluate it.
Example : By examining the long-term effects, social implications, and economic impact of climate change, it becomes evident that immediate global action is necessary.
Expository (or Descriptive) thesis statement
Purpose : To explain a topic or subject to the reader.
Example : The Great Depression, spanning the 1930s, was a severe worldwide economic downturn triggered by a stock market crash, bank failures, and reduced consumer spending.
Cause and effect thesis statement
Purpose : To demonstrate a cause and its resulting effect.
Example : Overuse of smartphones can lead to impaired sleep patterns, reduced face-to-face social interactions, and increased levels of anxiety.
Compare and contrast thesis statement
Purpose : To highlight similarities and differences between two subjects.
Example : "While both novels '1984' and 'Brave New World' delve into dystopian futures, they differ in their portrayal of individual freedom, societal control, and the role of technology."
When you write a thesis statement , it's important to ensure clarity and precision, so the reader immediately understands the central focus of your work.
What is the difference between a thesis and a thesis statement?
While both terms are frequently used interchangeably, they have distinct meanings.
A thesis refers to the entire research document, encompassing all its chapters and sections. In contrast, a thesis statement is a brief assertion that encapsulates the central argument of the research.
Here’s an in-depth differentiation table of a thesis and a thesis statement.
Now, to craft a compelling thesis, it's crucial to adhere to a specific structure. Let’s break down these essential components that make up a thesis structure
15 components of a thesis structure
Navigating a thesis can be daunting. However, understanding its structure can make the process more manageable.
Here are the key components or different sections of a thesis structure:
Your thesis begins with the title page. It's not just a formality but the gateway to your research.

Here, you'll prominently display the necessary information about you (the author) and your institutional details.
- Title of your thesis
- Your full name
- Your department
- Your institution and degree program
- Your submission date
- Your Supervisor's name (in some cases)
- Your Department or faculty (in some cases)
- Your University's logo (in some cases)
- Your Student ID (in some cases)
In a concise manner, you'll have to summarize the critical aspects of your research in typically no more than 200-300 words.

This includes the problem statement, methodology, key findings, and conclusions. For many, the abstract will determine if they delve deeper into your work, so ensure it's clear and compelling.
Acknowledgments
Research is rarely a solitary endeavor. In the acknowledgments section, you have the chance to express gratitude to those who've supported your journey.

This might include advisors, peers, institutions, or even personal sources of inspiration and support. It's a personal touch, reflecting the humanity behind the academic rigor.
Table of contents
A roadmap for your readers, the table of contents lists the chapters, sections, and subsections of your thesis.
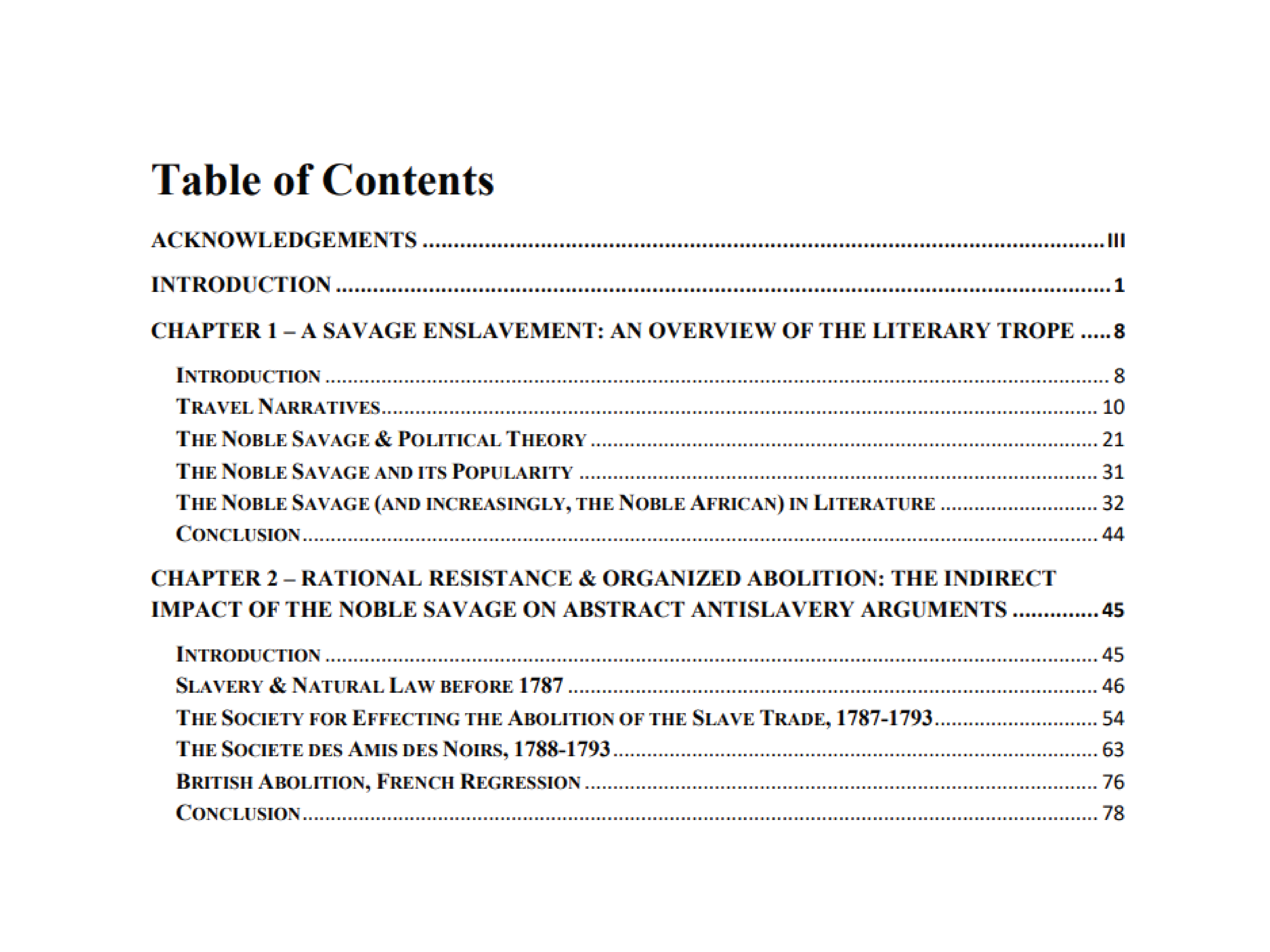
By providing page numbers, you allow readers to navigate your work easily, jumping to sections that pique their interest.
List of figures and tables
Research often involves data, and presenting this data visually can enhance understanding. This section provides an organized listing of all figures and tables in your thesis.

It's a visual index, ensuring that readers can quickly locate and reference your graphical data.
Introduction
Here's where you introduce your research topic, articulate the research question or objective, and outline the significance of your study.

- Present the research topic : Clearly articulate the central theme or subject of your research.
- Background information : Ground your research topic, providing any necessary context or background information your readers might need to understand the significance of your study.
- Define the scope : Clearly delineate the boundaries of your research, indicating what will and won't be covered.
- Literature review : Introduce any relevant existing research on your topic, situating your work within the broader academic conversation and highlighting where your research fits in.
- State the research Question(s) or objective(s) : Clearly articulate the primary questions or objectives your research aims to address.
- Outline the study's structure : Give a brief overview of how the subsequent sections of your work will unfold, guiding your readers through the journey ahead.
The introduction should captivate your readers, making them eager to delve deeper into your research journey.
Literature review section
Your study correlates with existing research. Therefore, in the literature review section, you'll engage in a dialogue with existing knowledge, highlighting relevant studies, theories, and findings.
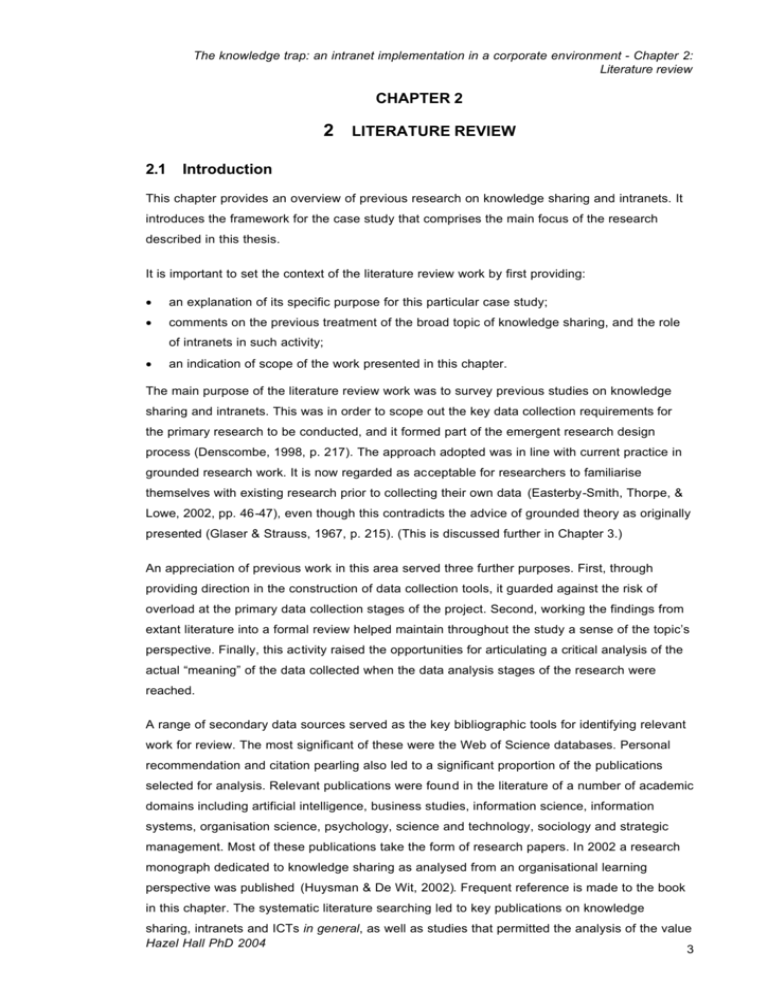
It's here that you identify gaps in the current knowledge, positioning your research as a bridge to new insights.
To streamline this process, consider leveraging AI tools. For example, the SciSpace literature review tool enables you to efficiently explore and delve into research papers, simplifying your literature review journey.
Methodology
In the research methodology section, you’ll detail the tools, techniques, and processes you employed to gather and analyze data. This section will inform the readers about how you approached your research questions and ensures the reproducibility of your study.
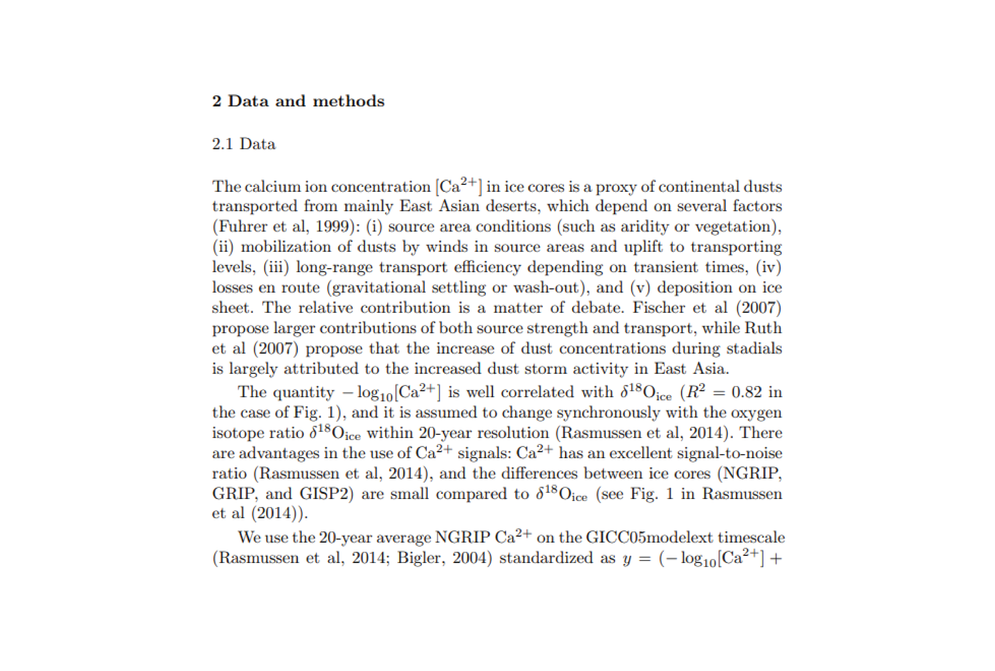
Here's a breakdown of what it should encompass:
- Research Design : Describe the overall structure and approach of your research. Are you conducting a qualitative study with in-depth interviews? Or is it a quantitative study using statistical analysis? Perhaps it's a mixed-methods approach?
- Data Collection : Detail the methods you used to gather data. This could include surveys, experiments, observations, interviews, archival research, etc. Mention where you sourced your data, the duration of data collection, and any tools or instruments used.
- Sampling : If applicable, explain how you selected participants or data sources for your study. Discuss the size of your sample and the rationale behind choosing it.
- Data Analysis : Describe the techniques and tools you used to process and analyze the data. This could range from statistical tests in quantitative research to thematic analysis in qualitative research.
- Validity and Reliability : Address the steps you took to ensure the validity and reliability of your findings to ensure that your results are both accurate and consistent.
- Ethical Considerations : Highlight any ethical issues related to your research and the measures you took to address them, including — informed consent, confidentiality, and data storage and protection measures.
Moreover, different research questions necessitate different types of methodologies. For instance:
- Experimental methodology : Often used in sciences, this involves a controlled experiment to discern causality.
- Qualitative methodology : Employed when exploring patterns or phenomena without numerical data. Methods can include interviews, focus groups, or content analysis.
- Quantitative methodology : Concerned with measurable data and often involves statistical analysis. Surveys and structured observations are common tools here.
- Mixed methods : As the name implies, this combines both qualitative and quantitative methodologies.
The Methodology section isn’t just about detailing the methods but also justifying why they were chosen. The appropriateness of the methods in addressing your research question can significantly impact the credibility of your findings.
Results (or Findings)
This section presents the outcomes of your research. It's crucial to note that the nature of your results may vary; they could be quantitative, qualitative, or a mix of both.

Quantitative results often present statistical data, showcasing measurable outcomes, and they benefit from tables, graphs, and figures to depict these data points.
Qualitative results , on the other hand, might delve into patterns, themes, or narratives derived from non-numerical data, such as interviews or observations.
Regardless of the nature of your results, clarity is essential. This section is purely about presenting the data without offering interpretations — that comes later in the discussion.
In the discussion section, the raw data transforms into valuable insights.
Start by revisiting your research question and contrast it with the findings. How do your results expand, constrict, or challenge current academic conversations?
Dive into the intricacies of the data, guiding the reader through its implications. Detail potential limitations transparently, signaling your awareness of the research's boundaries. This is where your academic voice should be resonant and confident.
Practical implications (Recommendation) section
Based on the insights derived from your research, this section provides actionable suggestions or proposed solutions.
Whether aimed at industry professionals or the general public, recommendations translate your academic findings into potential real-world actions. They help readers understand the practical implications of your work and how it can be applied to effect change or improvement in a given field.
When crafting recommendations, it's essential to ensure they're feasible and rooted in the evidence provided by your research. They shouldn't merely be aspirational but should offer a clear path forward, grounded in your findings.
The conclusion provides closure to your research narrative.
It's not merely a recap but a synthesis of your main findings and their broader implications. Reconnect with the research questions or hypotheses posited at the beginning, offering clear answers based on your findings.

Reflect on the broader contributions of your study, considering its impact on the academic community and potential real-world applications.
Lastly, the conclusion should leave your readers with a clear understanding of the value and impact of your study.
References (or Bibliography)
Every theory you've expounded upon, every data point you've cited, and every methodological precedent you've followed finds its acknowledgment here.

In references, it's crucial to ensure meticulous consistency in formatting, mirroring the specific guidelines of the chosen citation style .
Proper referencing helps to avoid plagiarism , gives credit to original ideas, and allows readers to explore topics of interest. Moreover, it situates your work within the continuum of academic knowledge.
To properly cite the sources used in the study, you can rely on online citation generator tools to generate accurate citations!
Here’s more on how you can cite your sources.
Often, the depth of research produces a wealth of material that, while crucial, can make the core content of the thesis cumbersome. The appendix is where you mention extra information that supports your research but isn't central to the main text.

Whether it's raw datasets, detailed procedural methodologies, extended case studies, or any other ancillary material, the appendices ensure that these elements are archived for reference without breaking the main narrative's flow.
For thorough researchers and readers keen on meticulous details, the appendices provide a treasure trove of insights.
Glossary (optional)
In academics, specialized terminologies, and jargon are inevitable. However, not every reader is versed in every term.
The glossary, while optional, is a critical tool for accessibility. It's a bridge ensuring that even readers from outside the discipline can access, understand, and appreciate your work.

By defining complex terms and providing context, you're inviting a wider audience to engage with your research, enhancing its reach and impact.
Remember, while these components provide a structured framework, the essence of your thesis lies in the originality of your ideas, the rigor of your research, and the clarity of your presentation.
As you craft each section, keep your readers in mind, ensuring that your passion and dedication shine through every page.
Thesis examples
To further elucidate the concept of a thesis, here are illustrative examples from various fields:
Example 1 (History): Abolition, Africans, and Abstraction: the Influence of the ‘Noble Savage’ on British and French Antislavery Thought, 1787-1807 by Suchait Kahlon.
Example 2 (Climate Dynamics): Influence of external forcings on abrupt millennial-scale climate changes: a statistical modelling study by Takahito Mitsui · Michel Crucifix
Checklist for your thesis evaluation
Evaluating your thesis ensures that your research meets the standards of academia. Here's an elaborate checklist to guide you through this critical process.
Content and structure
- Is the thesis statement clear, concise, and debatable?
- Does the introduction provide sufficient background and context?
- Is the literature review comprehensive, relevant, and well-organized?
- Does the methodology section clearly describe and justify the research methods?
- Are the results/findings presented clearly and logically?
- Does the discussion interpret the results in light of the research question and existing literature?
- Is the conclusion summarizing the research and suggesting future directions or implications?
Clarity and coherence
- Is the writing clear and free of jargon?
- Are ideas and sections logically connected and flowing?
- Is there a clear narrative or argument throughout the thesis?
Research quality
- Is the research question significant and relevant?
- Are the research methods appropriate for the question?
- Is the sample size (if applicable) adequate?
- Are the data analysis techniques appropriate and correctly applied?
- Are potential biases or limitations addressed?
Originality and significance
- Does the thesis contribute new knowledge or insights to the field?
- Is the research grounded in existing literature while offering fresh perspectives?
Formatting and presentation
- Is the thesis formatted according to institutional guidelines?
- Are figures, tables, and charts clear, labeled, and referenced in the text?
- Is the bibliography or reference list complete and consistently formatted?
- Are appendices relevant and appropriately referenced in the main text?
Grammar and language
- Is the thesis free of grammatical and spelling errors?
- Is the language professional, consistent, and appropriate for an academic audience?
- Are quotations and paraphrased material correctly cited?
Feedback and revision
- Have you sought feedback from peers, advisors, or experts in the field?
- Have you addressed the feedback and made the necessary revisions?
Overall assessment
- Does the thesis as a whole feel cohesive and comprehensive?
- Would the thesis be understandable and valuable to someone in your field?
Ensure to use this checklist to leave no ground for doubt or missed information in your thesis.
After writing your thesis, the next step is to discuss and defend your findings verbally in front of a knowledgeable panel. You’ve to be well prepared as your professors may grade your presentation abilities.
Preparing your thesis defense
A thesis defense, also known as "defending the thesis," is the culmination of a scholar's research journey. It's the final frontier, where you’ll present their findings and face scrutiny from a panel of experts.
Typically, the defense involves a public presentation where you’ll have to outline your study, followed by a question-and-answer session with a committee of experts. This committee assesses the validity, originality, and significance of the research.
The defense serves as a rite of passage for scholars. It's an opportunity to showcase expertise, address criticisms, and refine arguments. A successful defense not only validates the research but also establishes your authority as a researcher in your field.
Here’s how you can effectively prepare for your thesis defense .
Now, having touched upon the process of defending a thesis, it's worth noting that scholarly work can take various forms, depending on academic and regional practices.
One such form, often paralleled with the thesis, is the 'dissertation.' But what differentiates the two?
Dissertation vs. Thesis
Often used interchangeably in casual discourse, they refer to distinct research projects undertaken at different levels of higher education.
To the uninitiated, understanding their meaning might be elusive. So, let's demystify these terms and delve into their core differences.
Here's a table differentiating between the two.
Wrapping up
From understanding the foundational concept of a thesis to navigating its various components, differentiating it from a dissertation, and recognizing the importance of proper citation — this guide covers it all.
As scholars and readers, understanding these nuances not only aids in academic pursuits but also fosters a deeper appreciation for the relentless quest for knowledge that drives academia.
It’s important to remember that every thesis is a testament to curiosity, dedication, and the indomitable spirit of discovery.
Good luck with your thesis writing!
Frequently Asked Questions
A thesis typically ranges between 40-80 pages, but its length can vary based on the research topic, institution guidelines, and level of study.
A PhD thesis usually spans 200-300 pages, though this can vary based on the discipline, complexity of the research, and institutional requirements.
To identify a thesis topic, consider current trends in your field, gaps in existing literature, personal interests, and discussions with advisors or mentors. Additionally, reviewing related journals and conference proceedings can provide insights into potential areas of exploration.
The conceptual framework is often situated in the literature review or theoretical framework section of a thesis. It helps set the stage by providing the context, defining key concepts, and explaining the relationships between variables.
A thesis statement should be concise, clear, and specific. It should state the main argument or point of your research. Start by pinpointing the central question or issue your research addresses, then condense that into a single statement, ensuring it reflects the essence of your paper.
You might also like

AI for Meta Analysis — A Comprehensive Guide
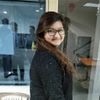
Cybersecurity in Higher Education: Safeguarding Students and Faculty Data

How To Write An Argumentative Essay

Writing the Research Methodology Section of Your Thesis
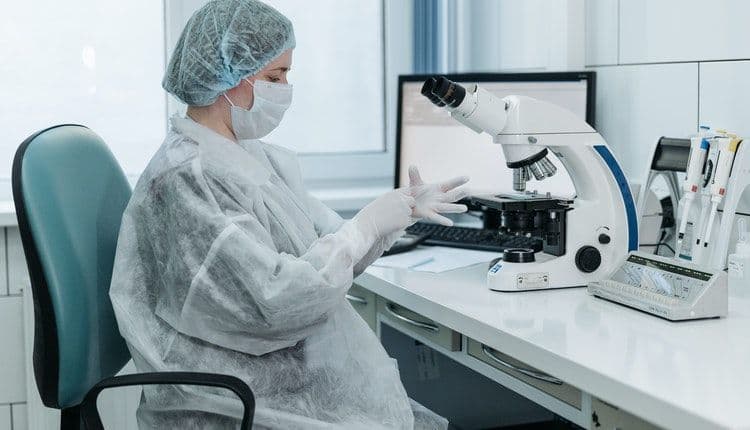
This article explains the meaning of research methodology and the purpose and importance of writing a research methodology section or chapter for your thesis paper. It discusses what to include and not include in a research methodology section, the different approaches to research methodology that can be used, and the steps involved in writing a robust research methodology section.
What is a thesis research methodology?
A thesis research methodology explains the type of research performed, justifies the methods that you chose by linking back to the literature review , and describes the data collection and analysis procedures. It is included in your thesis after the Introduction section . Most importantly, this is the section where the readers of your study evaluate its validity and reliability.
What should the research methodology section in your thesis include?
- The aim of your thesis
- An outline of the research methods chosen (qualitative, quantitative, or mixed methods)
- Background and rationale for the methods chosen, explaining why one method was chosen over another
- Methods used for data collection and data analysis
- Materials and equipment used—keep this brief
- Difficulties encountered during data collection and analysis. It is expected that problems will occur during your research process. Use this as an opportunity to demonstrate your problem-solving abilities by explaining how you overcame all obstacles. This builds your readers’ confidence in your study findings.
- A brief evaluation of your research explaining whether your results were conclusive and whether your choice of methodology was effective in practice
What should not be included in the research methodology section of your thesis?
- Irrelevant details, for example, an extensive review of methodologies (this belongs in the literature review section) or information that does not contribute to the readers’ understanding of your chosen methods
- A description of basic procedures
- Excessive details about materials and equipment used. If an extremely long and detailed list is necessary, add it as an appendix
Types of methodological approaches
The choice of which methodological approach to use depends on your field of research and your thesis question. Your methodology should establish a clear relationship with your thesis question and must also be supported by your literature review . Types of methodological approaches include quantitative, qualitative, or mixed methods.
Quantitative studies generate data in the form of numbers to count, classify, measure, or identify relationships or patterns. Information may be collected by performing experiments and tests, conducting surveys, or using existing data. The data are analyzed using statistical tests and presented as charts or graphs. Quantitative data are typically used in the Sciences domain.
For example, analyzing the effect of a change, such as alterations in electricity consumption by municipalities after installing LED streetlights.
The raw data will need to be prepared for statistical analysis by identifying variables and checking for missing data and outliers. Details of the statistical software program used (name of the package, version number, and supplier name and location) must also be mentioned.
Qualitative studies gather non-numerical data using, for example, observations, focus groups, and in-depth interviews. Open-ended questions are often posed. This yields rich, detailed, and descriptive results. Qualitative studies are usually subjective and are helpful for investigating social and cultural phenomena, which are difficult to quantify. Qualitative studies are typically used in the Humanities and Social Sciences (HSS) domain.
For example, determining customer perceptions on the extension of a range of baking utensils to include silicone muffin trays.
The raw data will need to be prepared for analysis by coding and categorizing ideas and themes to interpret the meaning behind the responses given.
Mixed methods use a combination of quantitative and qualitative approaches to present multiple findings about a single phenomenon. T his enables triangulation: verification of the data from two or more sources.
Data collection
Explain the rationale behind the sampling procedure you have chosen. This could involve probability sampling (a random sample from the study population) or non-probability sampling (does not use a random sample).
For quantitative studies, describe the sampling procedure and whether statistical tests were used to determine the sample size .
Following our example of analyzing the changes in electricity consumption by municipalities after installing LED streetlights, you will need to determine which municipal areas will be sampled and how the information will be gathered (e.g., a physical survey of the streetlights or reviewing purchase orders).
For qualitative research, describe how the participants were chosen and how the data is going to be collected.
Following our example about determining customer perceptions on the extension of a range of baking utensils to include silicone muffin trays, you will need to decide the criteria for inclusion as a study participant (e.g., women aged 20–70 years, bakeries, and bakery supply shops) and how the information will be collected (e.g., interviews, focus groups, online or in-person questionnaires, or video recordings) .
Data analysis
For quantitative research, describe what tests you plan to perform and why you have chosen them. Popular data analysis methods in quantitative research include:
- Descriptive statistics (e.g., means, medians, modes)
- Inferential statistics (e.g., correlation, regression, structural equation modeling)
For qualitative research, describe how the data is going to be analyzed and justify your choice. Popular data analysis methods in qualitative research include:
- Qualitative content analysis
- Thematic analysis
- Discourse analysis
- Narrative analysis
- Grounded theory
- Interpretative phenomenological analysis (IPA)
Evaluate and justify your methodological choices
You need to convince the reader that you have made the correct methodological choices. Once again, this ties back to your thesis question and literature review . Write using a persuasive tone, and use rhetoric to convince the reader of the quality, reliability, and validity of your research.
Ethical considerations
- The young researcher should maintain objectivity at all times
- All participants have the right to privacy and anonymity
- Research participation must be voluntary
- All subjects have the right to withdraw from the research at any time
- Consent must be obtained from all participants before starting the research
- Confidentiality of data provided by individuals must be maintained
- Consider how the interpretation and reporting of the data will affect the participants
Tips for writing a robust thesis research methodology
- Determine what kind of knowledge you are trying to uncover. For example, subjective or objective, experimental or interpretive.
- A thorough literature review is the best starting point for choosing your methods.
- Ensure that there is continuity throughout the research process. The authenticity of your research depends upon the validity of the research data, the reliability of your data measurements, and the time taken to conduct the analysis.
- Choose a research method that is achievable. Consider the time and funds available, feasibility, ethics, and access and availability of equipment to measure the phenomenon or answer your thesis question correctly.
- If you are struggling with a concept, ask for help from your supervisor, academic staff members, or fellow students.
A thesis methodology justifies why you have chosen a specific approach to address your thesis question. It explains how you will collect the data and analyze it. Above all, it allows the readers of your study to evaluate its validity and reliability.
A thesis is the most crucial document that you will write during your academic studies. For professional thesis editing and thesis proofreading services, visit Enago Thesis Editing for more information.
Editor’s pick
Get free updates.
Subscribe to our newsletter for regular insights from the research and publishing industry!
Review Checklist
Introduce your methodological approach , for example, quantitative, qualitative, or mixed methods.
Explain why your chosen approach is relevant to the overall research design and how it links with your thesis question.
Justify your chosen method and why it is more appropriate than others.
Provide background information on methods that may be unfamiliar to readers of your thesis.
Introduce the tools that you will use for data collection , and explain how you plan to use them (e.g., surveys, interviews, experiments, or existing data).
Explain how you will analyze your results. The type of analysis used depends on the methods you chose. For example, exploring theoretical perspectives to support your explanation of observed behaviors in a qualitative study or using statistical analyses in a quantitative study.
Mention any research limitations. All studies are expected to have limitations, such as the sample size, data collection method, or equipment. Discussing the limitations justifies your choice of methodology despite the risks. It also explains under which conditions the results should be interpreted and shows that you have taken a holistic approach to your study.
What is the difference between methodology and methods? +
Methodology refers to the overall rationale and strategy of your thesis project. It involves studying the theories or principles behind the methods used in your field so that you can explain why you chose a particular method for your research approach. Methods , on the other hand, refer to how the data were collected and analyzed (e.g., experiments, surveys, observations, interviews, and statistical tests).
What is the difference between reliability and validity? +
Reliability refers to whether a measurement is consistent (i.e., the results can be reproduced under the same conditions). Validity refers to whether a measurement is accurate (i.e., the results represent what was supposed to be measured). For example, when investigating linguistic and cultural guidelines for administration of the Preschool Language Scales, Fifth Edition (PLS5) in Arab-American preschool children, the normative sample curves should show the same distribution as a monolingual population, which would indicate that the test is valid. The test would be considered reliable if the results obtained were consistent across different sampling sites.
What tense is used to write the methods section? +
The methods section is written in the past tense because it describes what was done.
What software programs are recommended for statistical analysis? +
Recommended programs include Statistical Analysis Software (SAS) , Statistical Package for the Social Sciences (SPSS) , JMP , R software, MATLAB , Microsoft Excel, GraphPad Prism , and Minitab .

Qualitative Data Analysis Methods 101:
The “big 6” methods + examples.
By: Kerryn Warren (PhD) | Reviewed By: Eunice Rautenbach (D.Tech) | May 2020 (Updated April 2023)
Qualitative data analysis methods. Wow, that’s a mouthful.
If you’re new to the world of research, qualitative data analysis can look rather intimidating. So much bulky terminology and so many abstract, fluffy concepts. It certainly can be a minefield!
Don’t worry – in this post, we’ll unpack the most popular analysis methods , one at a time, so that you can approach your analysis with confidence and competence – whether that’s for a dissertation, thesis or really any kind of research project.
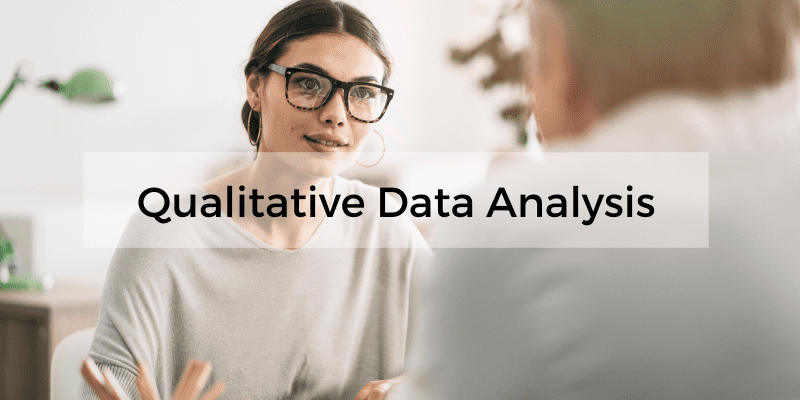
What (exactly) is qualitative data analysis?
To understand qualitative data analysis, we need to first understand qualitative data – so let’s step back and ask the question, “what exactly is qualitative data?”.
Qualitative data refers to pretty much any data that’s “not numbers” . In other words, it’s not the stuff you measure using a fixed scale or complex equipment, nor do you analyse it using complex statistics or mathematics.
So, if it’s not numbers, what is it?
Words, you guessed? Well… sometimes , yes. Qualitative data can, and often does, take the form of interview transcripts, documents and open-ended survey responses – but it can also involve the interpretation of images and videos. In other words, qualitative isn’t just limited to text-based data.
So, how’s that different from quantitative data, you ask?
Simply put, qualitative research focuses on words, descriptions, concepts or ideas – while quantitative research focuses on numbers and statistics . Qualitative research investigates the “softer side” of things to explore and describe , while quantitative research focuses on the “hard numbers”, to measure differences between variables and the relationships between them. If you’re keen to learn more about the differences between qual and quant, we’ve got a detailed post over here .
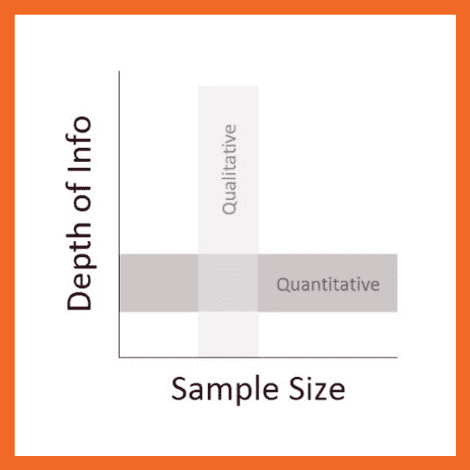
So, qualitative analysis is easier than quantitative, right?
Not quite. In many ways, qualitative data can be challenging and time-consuming to analyse and interpret. At the end of your data collection phase (which itself takes a lot of time), you’ll likely have many pages of text-based data or hours upon hours of audio to work through. You might also have subtle nuances of interactions or discussions that have danced around in your mind, or that you scribbled down in messy field notes. All of this needs to work its way into your analysis.
Making sense of all of this is no small task and you shouldn’t underestimate it. Long story short – qualitative analysis can be a lot of work! Of course, quantitative analysis is no piece of cake either, but it’s important to recognise that qualitative analysis still requires a significant investment in terms of time and effort.
Need a helping hand?
In this post, we’ll explore qualitative data analysis by looking at some of the most common analysis methods we encounter. We’re not going to cover every possible qualitative method and we’re not going to go into heavy detail – we’re just going to give you the big picture. That said, we will of course includes links to loads of extra resources so that you can learn more about whichever analysis method interests you.
Without further delay, let’s get into it.
The “Big 6” Qualitative Analysis Methods
There are many different types of qualitative data analysis, all of which serve different purposes and have unique strengths and weaknesses . We’ll start by outlining the analysis methods and then we’ll dive into the details for each.
The 6 most popular methods (or at least the ones we see at Grad Coach) are:
- Content analysis
- Narrative analysis
- Discourse analysis
- Thematic analysis
- Grounded theory (GT)
- Interpretive phenomenological analysis (IPA)
Let’s take a look at each of them…
QDA Method #1: Qualitative Content Analysis
Content analysis is possibly the most common and straightforward QDA method. At the simplest level, content analysis is used to evaluate patterns within a piece of content (for example, words, phrases or images) or across multiple pieces of content or sources of communication. For example, a collection of newspaper articles or political speeches.
With content analysis, you could, for instance, identify the frequency with which an idea is shared or spoken about – like the number of times a Kardashian is mentioned on Twitter. Or you could identify patterns of deeper underlying interpretations – for instance, by identifying phrases or words in tourist pamphlets that highlight India as an ancient country.
Because content analysis can be used in such a wide variety of ways, it’s important to go into your analysis with a very specific question and goal, or you’ll get lost in the fog. With content analysis, you’ll group large amounts of text into codes , summarise these into categories, and possibly even tabulate the data to calculate the frequency of certain concepts or variables. Because of this, content analysis provides a small splash of quantitative thinking within a qualitative method.
Naturally, while content analysis is widely useful, it’s not without its drawbacks . One of the main issues with content analysis is that it can be very time-consuming , as it requires lots of reading and re-reading of the texts. Also, because of its multidimensional focus on both qualitative and quantitative aspects, it is sometimes accused of losing important nuances in communication.
Content analysis also tends to concentrate on a very specific timeline and doesn’t take into account what happened before or after that timeline. This isn’t necessarily a bad thing though – just something to be aware of. So, keep these factors in mind if you’re considering content analysis. Every analysis method has its limitations , so don’t be put off by these – just be aware of them ! If you’re interested in learning more about content analysis, the video below provides a good starting point.
QDA Method #2: Narrative Analysis
As the name suggests, narrative analysis is all about listening to people telling stories and analysing what that means . Since stories serve a functional purpose of helping us make sense of the world, we can gain insights into the ways that people deal with and make sense of reality by analysing their stories and the ways they’re told.
You could, for example, use narrative analysis to explore whether how something is being said is important. For instance, the narrative of a prisoner trying to justify their crime could provide insight into their view of the world and the justice system. Similarly, analysing the ways entrepreneurs talk about the struggles in their careers or cancer patients telling stories of hope could provide powerful insights into their mindsets and perspectives . Simply put, narrative analysis is about paying attention to the stories that people tell – and more importantly, the way they tell them.
Of course, the narrative approach has its weaknesses , too. Sample sizes are generally quite small due to the time-consuming process of capturing narratives. Because of this, along with the multitude of social and lifestyle factors which can influence a subject, narrative analysis can be quite difficult to reproduce in subsequent research. This means that it’s difficult to test the findings of some of this research.
Similarly, researcher bias can have a strong influence on the results here, so you need to be particularly careful about the potential biases you can bring into your analysis when using this method. Nevertheless, narrative analysis is still a very useful qualitative analysis method – just keep these limitations in mind and be careful not to draw broad conclusions . If you’re keen to learn more about narrative analysis, the video below provides a great introduction to this qualitative analysis method.
QDA Method #3: Discourse Analysis
Discourse is simply a fancy word for written or spoken language or debate . So, discourse analysis is all about analysing language within its social context. In other words, analysing language – such as a conversation, a speech, etc – within the culture and society it takes place. For example, you could analyse how a janitor speaks to a CEO, or how politicians speak about terrorism.
To truly understand these conversations or speeches, the culture and history of those involved in the communication are important factors to consider. For example, a janitor might speak more casually with a CEO in a company that emphasises equality among workers. Similarly, a politician might speak more about terrorism if there was a recent terrorist incident in the country.
So, as you can see, by using discourse analysis, you can identify how culture , history or power dynamics (to name a few) have an effect on the way concepts are spoken about. So, if your research aims and objectives involve understanding culture or power dynamics, discourse analysis can be a powerful method.
Because there are many social influences in terms of how we speak to each other, the potential use of discourse analysis is vast . Of course, this also means it’s important to have a very specific research question (or questions) in mind when analysing your data and looking for patterns and themes, or you might land up going down a winding rabbit hole.
Discourse analysis can also be very time-consuming as you need to sample the data to the point of saturation – in other words, until no new information and insights emerge. But this is, of course, part of what makes discourse analysis such a powerful technique. So, keep these factors in mind when considering this QDA method. Again, if you’re keen to learn more, the video below presents a good starting point.
QDA Method #4: Thematic Analysis
Thematic analysis looks at patterns of meaning in a data set – for example, a set of interviews or focus group transcripts. But what exactly does that… mean? Well, a thematic analysis takes bodies of data (which are often quite large) and groups them according to similarities – in other words, themes . These themes help us make sense of the content and derive meaning from it.
Let’s take a look at an example.
With thematic analysis, you could analyse 100 online reviews of a popular sushi restaurant to find out what patrons think about the place. By reviewing the data, you would then identify the themes that crop up repeatedly within the data – for example, “fresh ingredients” or “friendly wait staff”.
So, as you can see, thematic analysis can be pretty useful for finding out about people’s experiences , views, and opinions . Therefore, if your research aims and objectives involve understanding people’s experience or view of something, thematic analysis can be a great choice.
Since thematic analysis is a bit of an exploratory process, it’s not unusual for your research questions to develop , or even change as you progress through the analysis. While this is somewhat natural in exploratory research, it can also be seen as a disadvantage as it means that data needs to be re-reviewed each time a research question is adjusted. In other words, thematic analysis can be quite time-consuming – but for a good reason. So, keep this in mind if you choose to use thematic analysis for your project and budget extra time for unexpected adjustments.
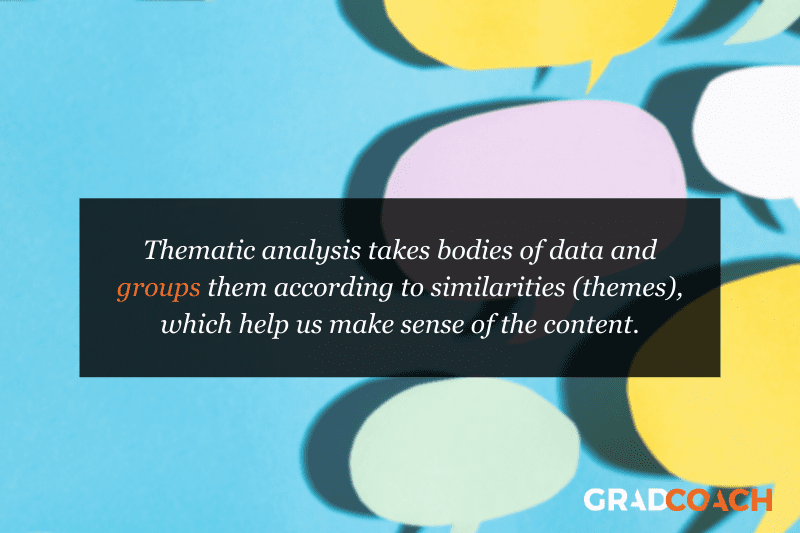
QDA Method #5: Grounded theory (GT)
Grounded theory is a powerful qualitative analysis method where the intention is to create a new theory (or theories) using the data at hand, through a series of “ tests ” and “ revisions ”. Strictly speaking, GT is more a research design type than an analysis method, but we’ve included it here as it’s often referred to as a method.
What’s most important with grounded theory is that you go into the analysis with an open mind and let the data speak for itself – rather than dragging existing hypotheses or theories into your analysis. In other words, your analysis must develop from the ground up (hence the name).
Let’s look at an example of GT in action.
Assume you’re interested in developing a theory about what factors influence students to watch a YouTube video about qualitative analysis. Using Grounded theory , you’d start with this general overarching question about the given population (i.e., graduate students). First, you’d approach a small sample – for example, five graduate students in a department at a university. Ideally, this sample would be reasonably representative of the broader population. You’d interview these students to identify what factors lead them to watch the video.
After analysing the interview data, a general pattern could emerge. For example, you might notice that graduate students are more likely to read a post about qualitative methods if they are just starting on their dissertation journey, or if they have an upcoming test about research methods.
From here, you’ll look for another small sample – for example, five more graduate students in a different department – and see whether this pattern holds true for them. If not, you’ll look for commonalities and adapt your theory accordingly. As this process continues, the theory would develop . As we mentioned earlier, what’s important with grounded theory is that the theory develops from the data – not from some preconceived idea.
So, what are the drawbacks of grounded theory? Well, some argue that there’s a tricky circularity to grounded theory. For it to work, in principle, you should know as little as possible regarding the research question and population, so that you reduce the bias in your interpretation. However, in many circumstances, it’s also thought to be unwise to approach a research question without knowledge of the current literature . In other words, it’s a bit of a “chicken or the egg” situation.
Regardless, grounded theory remains a popular (and powerful) option. Naturally, it’s a very useful method when you’re researching a topic that is completely new or has very little existing research about it, as it allows you to start from scratch and work your way from the ground up .
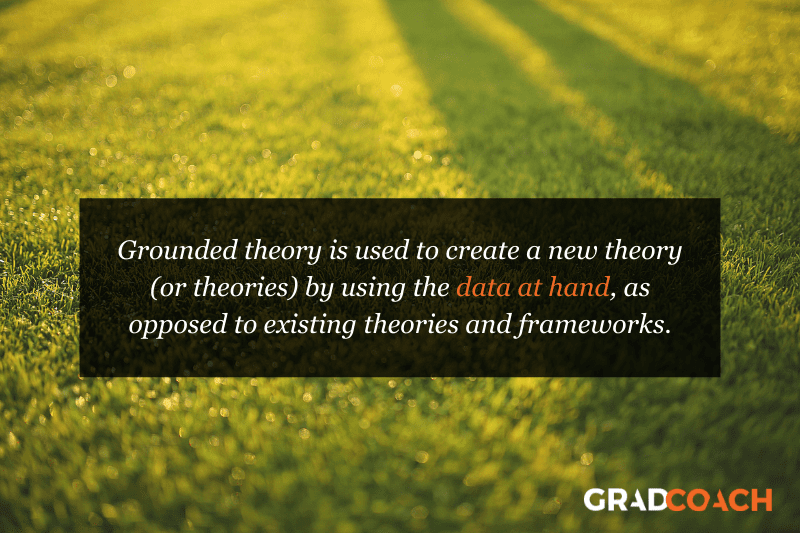
QDA Method #6: Interpretive Phenomenological Analysis (IPA)
Interpretive. Phenomenological. Analysis. IPA . Try saying that three times fast…
Let’s just stick with IPA, okay?
IPA is designed to help you understand the personal experiences of a subject (for example, a person or group of people) concerning a major life event, an experience or a situation . This event or experience is the “phenomenon” that makes up the “P” in IPA. Such phenomena may range from relatively common events – such as motherhood, or being involved in a car accident – to those which are extremely rare – for example, someone’s personal experience in a refugee camp. So, IPA is a great choice if your research involves analysing people’s personal experiences of something that happened to them.
It’s important to remember that IPA is subject – centred . In other words, it’s focused on the experiencer . This means that, while you’ll likely use a coding system to identify commonalities, it’s important not to lose the depth of experience or meaning by trying to reduce everything to codes. Also, keep in mind that since your sample size will generally be very small with IPA, you often won’t be able to draw broad conclusions about the generalisability of your findings. But that’s okay as long as it aligns with your research aims and objectives.
Another thing to be aware of with IPA is personal bias . While researcher bias can creep into all forms of research, self-awareness is critically important with IPA, as it can have a major impact on the results. For example, a researcher who was a victim of a crime himself could insert his own feelings of frustration and anger into the way he interprets the experience of someone who was kidnapped. So, if you’re going to undertake IPA, you need to be very self-aware or you could muddy the analysis.
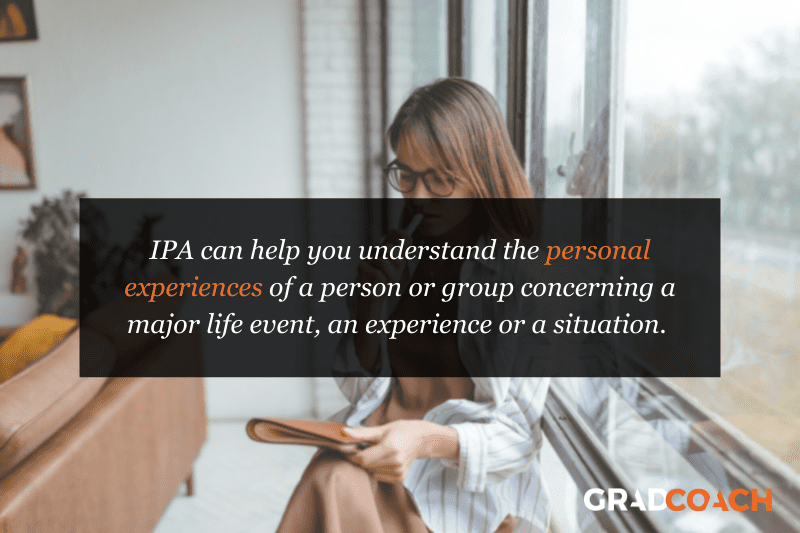
How to choose the right analysis method
In light of all of the qualitative analysis methods we’ve covered so far, you’re probably asking yourself the question, “ How do I choose the right one? ”
Much like all the other methodological decisions you’ll need to make, selecting the right qualitative analysis method largely depends on your research aims, objectives and questions . In other words, the best tool for the job depends on what you’re trying to build. For example:
- Perhaps your research aims to analyse the use of words and what they reveal about the intention of the storyteller and the cultural context of the time.
- Perhaps your research aims to develop an understanding of the unique personal experiences of people that have experienced a certain event, or
- Perhaps your research aims to develop insight regarding the influence of a certain culture on its members.
As you can probably see, each of these research aims are distinctly different , and therefore different analysis methods would be suitable for each one. For example, narrative analysis would likely be a good option for the first aim, while grounded theory wouldn’t be as relevant.
It’s also important to remember that each method has its own set of strengths, weaknesses and general limitations. No single analysis method is perfect . So, depending on the nature of your research, it may make sense to adopt more than one method (this is called triangulation ). Keep in mind though that this will of course be quite time-consuming.
As we’ve seen, all of the qualitative analysis methods we’ve discussed make use of coding and theme-generating techniques, but the intent and approach of each analysis method differ quite substantially. So, it’s very important to come into your research with a clear intention before you decide which analysis method (or methods) to use.
Start by reviewing your research aims , objectives and research questions to assess what exactly you’re trying to find out – then select a qualitative analysis method that fits. Never pick a method just because you like it or have experience using it – your analysis method (or methods) must align with your broader research aims and objectives.
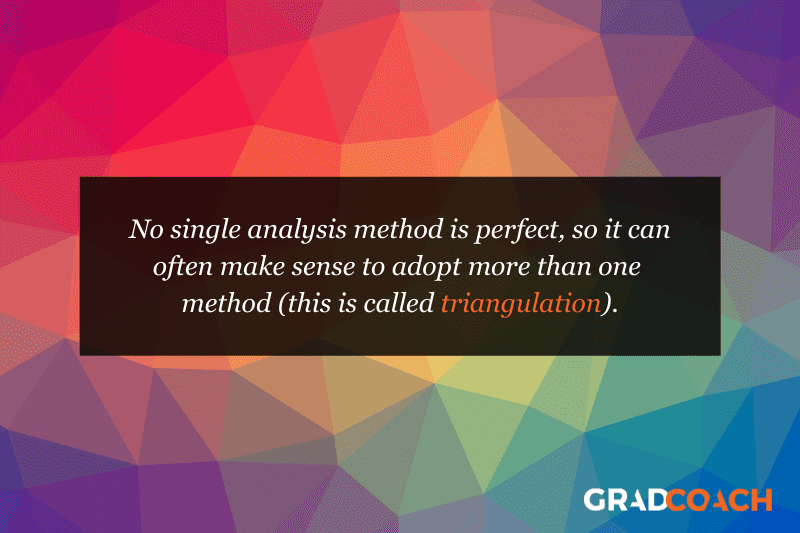
Let’s recap on QDA methods…
In this post, we looked at six popular qualitative data analysis methods:
- First, we looked at content analysis , a straightforward method that blends a little bit of quant into a primarily qualitative analysis.
- Then we looked at narrative analysis , which is about analysing how stories are told.
- Next up was discourse analysis – which is about analysing conversations and interactions.
- Then we moved on to thematic analysis – which is about identifying themes and patterns.
- From there, we went south with grounded theory – which is about starting from scratch with a specific question and using the data alone to build a theory in response to that question.
- And finally, we looked at IPA – which is about understanding people’s unique experiences of a phenomenon.
Of course, these aren’t the only options when it comes to qualitative data analysis, but they’re a great starting point if you’re dipping your toes into qualitative research for the first time.
If you’re still feeling a bit confused, consider our private coaching service , where we hold your hand through the research process to help you develop your best work.
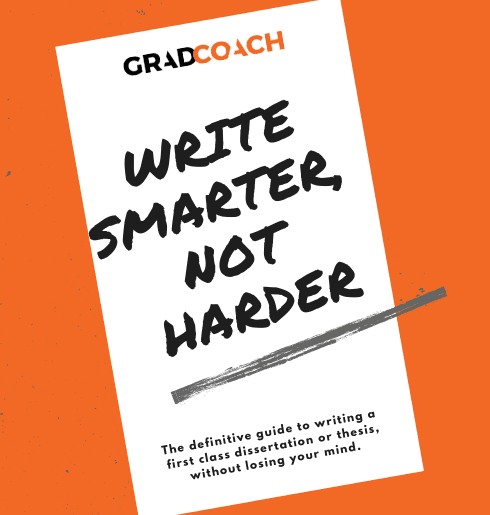
Psst… there’s more (for free)
This post is part of our dissertation mini-course, which covers everything you need to get started with your dissertation, thesis or research project.
You Might Also Like:
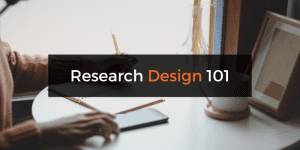
84 Comments
This has been very helpful. Thank you.
Thank you madam,
Thank you so much for this information
I wonder it so clear for understand and good for me. can I ask additional query?
Very insightful and useful
Good work done with clear explanations. Thank you.
Thanks so much for the write-up, it’s really good.
Thanks madam . It is very important .
thank you very good
This has been very well explained in simple language . It is useful even for a new researcher.
Great to hear that. Good luck with your qualitative data analysis, Pramod!
This is very useful information. And it was very a clear language structured presentation. Thanks a lot.
Thank you so much.
very informative sequential presentation
Precise explanation of method.
Hi, may we use 2 data analysis methods in our qualitative research?
Thanks for your comment. Most commonly, one would use one type of analysis method, but it depends on your research aims and objectives.
You explained it in very simple language, everyone can understand it. Thanks so much.
Thank you very much, this is very helpful. It has been explained in a very simple manner that even a layman understands
Thank nicely explained can I ask is Qualitative content analysis the same as thematic analysis?
Thanks for your comment. No, QCA and thematic are two different types of analysis. This article might help clarify – https://onlinelibrary.wiley.com/doi/10.1111/nhs.12048
This is my first time to come across a well explained data analysis. so helpful.
I have thoroughly enjoyed your explanation of the six qualitative analysis methods. This is very helpful. Thank you!
Thank you very much, this is well explained and useful
i need a citation of your book.
Thanks a lot , remarkable indeed, enlighting to the best
Hi Derek, What other theories/methods would you recommend when the data is a whole speech?
Keep writing useful artikel.
It is important concept about QDA and also the way to express is easily understandable, so thanks for all.
Thank you, this is well explained and very useful.
Very helpful .Thanks.
Hi there! Very well explained. Simple but very useful style of writing. Please provide the citation of the text. warm regards
The session was very helpful and insightful. Thank you
This was very helpful and insightful. Easy to read and understand
As a professional academic writer, this has been so informative and educative. Keep up the good work Grad Coach you are unmatched with quality content for sure.
Keep up the good work Grad Coach you are unmatched with quality content for sure.
Its Great and help me the most. A Million Thanks you Dr.
It is a very nice work
Very insightful. Please, which of this approach could be used for a research that one is trying to elicit students’ misconceptions in a particular concept ?
This is Amazing and well explained, thanks
great overview
What do we call a research data analysis method that one use to advise or determining the best accounting tool or techniques that should be adopted in a company.
Informative video, explained in a clear and simple way. Kudos
Waoo! I have chosen method wrong for my data analysis. But I can revise my work according to this guide. Thank you so much for this helpful lecture.
This has been very helpful. It gave me a good view of my research objectives and how to choose the best method. Thematic analysis it is.
Very helpful indeed. Thanku so much for the insight.
This was incredibly helpful.
Very helpful.
very educative
Nicely written especially for novice academic researchers like me! Thank you.
choosing a right method for a paper is always a hard job for a student, this is a useful information, but it would be more useful personally for me, if the author provide me with a little bit more information about the data analysis techniques in type of explanatory research. Can we use qualitative content analysis technique for explanatory research ? or what is the suitable data analysis method for explanatory research in social studies?
that was very helpful for me. because these details are so important to my research. thank you very much
I learnt a lot. Thank you
Relevant and Informative, thanks !
Well-planned and organized, thanks much! 🙂
I have reviewed qualitative data analysis in a simplest way possible. The content will highly be useful for developing my book on qualitative data analysis methods. Cheers!
Clear explanation on qualitative and how about Case study
This was helpful. Thank you
This was really of great assistance, it was just the right information needed. Explanation very clear and follow.
Wow, Thanks for making my life easy
This was helpful thanks .
Very helpful…. clear and written in an easily understandable manner. Thank you.
This was so helpful as it was easy to understand. I’m a new to research thank you so much.
so educative…. but Ijust want to know which method is coding of the qualitative or tallying done?
Thank you for the great content, I have learnt a lot. So helpful
precise and clear presentation with simple language and thank you for that.
very informative content, thank you.
You guys are amazing on YouTube on this platform. Your teachings are great, educative, and informative. kudos!
Brilliant Delivery. You made a complex subject seem so easy. Well done.
Beautifully explained.
Thanks a lot
Is there a video the captures the practical process of coding using automated applications?
Thanks for the comment. We don’t recommend using automated applications for coding, as they are not sufficiently accurate in our experience.
content analysis can be qualitative research?
THANK YOU VERY MUCH.
Thank you very much for such a wonderful content
do you have any material on Data collection
What a powerful explanation of the QDA methods. Thank you.
Great explanation both written and Video. i have been using of it on a day to day working of my thesis project in accounting and finance. Thank you very much for your support.
very helpful, thank you so much
Submit a Comment Cancel reply
Your email address will not be published. Required fields are marked *
Save my name, email, and website in this browser for the next time I comment.
- Print Friendly

Enter the URL below into your favorite RSS reader.
Using Quantitative Analytical Methods to Support Qualitative Data Analysis: Lessons Learnt During a PhD Study
- Citation (BibTeX)
View more stats
Qualitative studies produce rich insights on accounting phenomena in complex social environments. However, the data analysis phase presents many challenges and places heavy demands on the individual researcher. This paper describes in detail, the application of quantitative analytical protocols to support qualitative data analysis during a PhD study. The protocols were developed to manage the task of analysing large volumes of data in a complete and unbiased way. The paper provides useful insights for qualitative researchers on the steps involved in implementing this approach and considers both its merits and problems.
1. Introduction
Qualitative studies are popular among accounting scholars. This context-rich approach expands our understanding of how accounting phenomena are created, experienced and interpreted within a complex social environment (Mason , 2012) . However, qualitative research places heavy demands on the individual researcher particularly during the data analysis phase (Ahrens & Dent , 1998; O’Dwyer , 2008) with one such challenge being the large volume of data commonly generated. Analysis of large bodies of narrative text is time-consuming, resource-intensive and subject to interpretation bias reducing the external validity of such research (Indulska et al. , 2012) .
The objective of this paper is to encourage researchers, particularly doctoral students, to consider an analytical protocol that combines quantitative methods alongside traditional qualitative approaches. The major focus of the paper is to provide a detailed explanation of a systematic analytical protocol designed to reveal key connections between phenomena of interest. Rather than a conceptual discussion, the protocol is described within the context of the author’s own data analysis experience while undertaking a doctoral study. This provides a context to consider how the analytical protocol, namely C-Ratios (which quantify the relative strength of interactions between constructs by normalising the frequency of data coding co-occurrences), supported the research objectives. C-Ratios are an effective technique in managing the analysis of large volumes of narrative data and ensuring consideration is given to all data in interpreting data and drawing conclusions.
The following section discusses the separation that has emerged between quantitative and qualitative research. Section 3 discusses some challenges faced by qualitative researchers during data analysis. Section 4 details the application of quantitative analytical methods to qualitative data. Section 5 provides a detailed description of the use of C-Ratios as a key element of data analysis in one study. Finally, the paper concludes with a retrospective evaluation of the use of the technique and considers its merits and the problems encountered.
2. Separation between Quantitative and Qualitative Research
From a methodological perspective, research is commonly classified as either qualitative or quantitative and both approaches are valid (Myers , 2009) . The distinctions between approaches are frequently stereotyped, for example: theory builders or theory testers; creators of horizontal or vertical knowledge; addressing questions regarding ‘how & why’ or ‘how often & how many’ (Malina et al. , 2011; Pratt et al. , 2020) . In the context of accounting, studies tend to adopt either quantitative or qualitative methods (Ihantola & Kihn , 2011) and both methods fruitfully advance our understanding of accounting phenomena. However, accounting scholars have highlighted the worrying trend of separation between methodological camps (Euske et al. , 2011; Modell , 2005 , 2007) . This has resulted in researchers being stereotyped as: ‘number crunchers’ or ‘naval gazers’; concerned with either ‘hard or squishy’ (Malina et al. , 2011 , p. 60) . This divide has resulted in “methodological camps that do not communicate well to refine or modify our incomplete theories and knowledge of practice” (Malina et al. , 2011 , p. 60) . As Modell & Humphrey (2008 , p. 93) argue, the “dividing lines …. might have been over-drawn”. Research is fundamentally a process of building on prior knowledge and advances in knowledge depend on researchers extending ideas, results and procedures of peers in their research communities (Euske et al. , 2011) . The existence of separate methodological camps is concerning as it may potentially hamper scholarly advances.
Quantitative methods can be traced back to the natural sciences and are widely used in the social sciences and include for example, survey methods or experiments (Myers , 2009) . These methods are appropriate for large data samples where the researcher is drawing on pre-existing research instruments to generate statistical testable data (Cresswell , 2009) . Emphasis is placed on testing theory and hence the relationship between theory and research tends to be viewed as deductive. Studies of this type tend to focus on explaining phenomenon variance through confirmatory testing, making systematic comparisons, measuring and analysing causal relationships (Pratt et al. , 2020; Silverman , 2005) . The trends and patterns that emerge can then be generalised to a wider population (Cresswell , 2009; Myers , 2009) . In this tradition, methodological transparency is critical to demonstrate trustworthiness (Pratt et al. , 2020) as findings should be replicable.
In contrast, qualitative approaches emerged from the traditions of anthropology and sociology (Myers , 2009; Pelto & Pelto , 1978) and includes, for example, case studies and field studies. This type of research relaxes the rigours of quantitative methods and permits the researcher to explore complex relationships and to discover rather than confirm or test (Corbin & Strauss , 2008) . According to Moll et al. (2006) , the philosophical origins of qualitative methods stress the value of understanding human behaviours and social interactions in an organisational context. From an accounting perspective, qualitative studies “seek a holistic understanding and critique of lived experiences, social settings and behaviours, through researchers’ engagement with the everyday” (Parker , 2012 , p. 55) . Qualitative studies are motivated towards addressing in-depth issues and addressing ‘how’ and ‘why’ questions. Qualitative data has several strengths. For instance, it captures naturally occurring events within their natural setting providing ‘real-life’ understanding, rom which ‘thick descriptions’ emerge (Denzin , 1994) and it permits an exploration of underlying processes. Qualitative data is potentially rich, vivid and holistic which offers potential to uncover real-world complexities. However, from a practical perspective, balancing between the richness while providing the means to establish an adequate level of trustworthiness in the findings that emerge can be challenging (Modell & Humphrey , 2008) . While qualitative and quantitative studies differ in the types of questions they address and how they are designed, both are widely used by accounting researchers. In the following section, attention focuses on challenges that arise at the data-analysis stage of qualitative studies.
3. Challenges Faced by Qualitative Researchers During Data Analysis
The data-analysis phase is onerous on qualitative researchers (Ahrens & Dent , 1998; O’Dwyer , 2008) . One of the foremost challenges, particularly for inexperienced qualitative researchers, lies in how to manage and analyse large volumes of qualitative data collected (Yin , 2009) . As it is not uncommon for interviews to transcribe to hundreds or even a few thousand pages of transcribed text, such volumes of evidence can quickly become overwhelming (Mason , 2012; O’Dwyer , 2008) . Referring to the vast quantities of data gathered in case study research, Pettigrew (1988 , p. 98) fittingly warns: “there is an ever-present danger of death by data asphyxiation” . This is concerning as Faust (1982) observes that humans struggle to process large volumes of data as it overloads our information-processing capabilities. A perceived lack of progress can lead the researcher to feeling disheartened and studies becoming stalled (McQueen & Knussen , 2002) . Furthermore, researchers may respond by arriving at rushed, partial and unfounded conclusions (Miles & Huberman , 2014) as they strive to reduce complexity into more manageable configurations.
Qualitative data is often perceived as lacking a standardised structure (Eisenhardt , 1989; Lillis , 1999 , 2006; Smith , 2015) taking the form of extended narratives characterised as dispersed, bulky and sequential rather than simultaneous (Miles & Huberman , 2014) . This point is particularly relevant when data is collected through semi-structured interviews (Mason , 2012) . The lack of structure reflects the inherent flexibility of qualitative data collection techniques. For example, the extent to which an interviewer needs to probe specific issues to ensure a complete understanding is likely to vary at each interview.
These challenges are further amplified by the absence of established techniques to ensure that qualitative data analysis is carried out in a way that is impartial and complete. It is important that all data collected is analysed and considered equally (Lillis , 1999 , 2006; Miles & Huberman , 2014) . It is tempting to be drawn towards data that corresponds seamlessly with theory; or quotes that encapsulate the essence of what we are studying (Lillis , 2006) . We instinctively recall more clearly vivid events, contextually rich stories and exciting descriptions in contrast to more mundane passages (McQueen & Knussen , 2002; Tversky & Kahneman , 1973) . This is problematic if such quotes are not representative of the entire data set and may lead to the introduction of bias. Further potential for bias exists as qualitative data-analysis is dependent on coding classifications prescribed by researchers themselves (Lillis , 1999) .
In addition, readers of qualitative research have limited opportunities to confirm the accuracy of the process of analysis and interpretation. As Marginson (2008 , p. 334) points out, only researchers possess the entire data set from which conclusions have been drawn. In contrast, readers have limited scope to confirm the accuracy of researchers’ analysis. Researchers must gain the readers’ trust by convincing them that the data analysis has been systematically constructed (Seale , 1999) , and conducted in a consistent manner. Both the underlying logic of analytical choices made and the procedures and practices followed must be well-explained (Mason , 2012) . I discuss strategies to manage qualitative data analysis challenges in the next section.
4. The Application of Quantitative Analytical Methods to Assist in Analysing Qualitative Data
“In qualitative research, numbers tend to get ignored. After all, the hallmark of qualitative research is that it goes beyond how much there is of something to tell us about its essential qualities. However, a lot of counting goes on in the background when judgments of qualities are being made. When we identify a theme or a pattern, we’re isolating something that (a) happens a number of times and (b) consistently happens in specific ways. The “numbers of times” and “consistency” judgments are based on counting. When we make a generalization, we amass a swarm of particulars and decide, almost unconsciously, which particulars are there more often, matter more than others, go together, and so on. When we say something is “important” or “significant” or “recurrent” we have come to that estimate, in part, by making counts, comparisons, and weights.” (Miles & Huberman , 2014 , p. 253)
A strategy to overcome the challenges associated with analysing qualitative data is to look beyond traditional qualitative methods of analysis and consider quantitative techniques as a means of providing support. Miles & Huberman (2014) advocate, quantification and ‘numbers’ to complement analysis. In practice, this means combining qualitative analysis with quantitative analysis either concurrently or sequentially (Cresswell , 2009) . Advocates suggest that a broader analytical approach enhances completeness, reduces the potential for bias, improves reliability resulting in more convincing and accurate conclusions emerging (Cresswell , 2009; Ihantola & Kihn , 2011; Lillis , 2006) . Quantification underpins several qualitative analytical protocols used by qualitative researchers: content analysis, matrices and C-Ratios each of which I discuss in turn.
4.1 Content Analysis
Accounting researchers have successfully used the technique of words and phrases (or both) counts or frequency for content analysis in the analysis of archival data (Smith , 2015) . This provides a systematic method to analyse the content of text (Steenkamp & Northcott , 2007) . The technique involves counting key words, phrases or both. Frequencies are then analysed thereby introducing elements of quantification into the process of analysing qualitative data (Easterby-Smith et al. , 2002) . For example, Smith & Taffler (2000) use a key word ratio variable (number of common occurrences of keyword/total number of words in the narrative section of the chairman’s statement) to indicate the perceived importance of keywords from a selection of chairman’s statements.
According to Malina et al. (2011) , content analysis has used widely by accounting scholars, particularly in the area of corporate reporting and finance (for example, in examining the narrative sections of annual reports and corporate communications (Abrahamson & Amir , 1996; Edgar et al. , 2018; Merkl-Davies et al. , 2011; Moreno et al. , 2019; Tennyson et al. , 1990) ). However, quantifying the relative importance of words, phrases or both, such as the approach used in Smith & Taffler (2000) , tends to be confined to analysing archival data or documentary evidence rather than interview data.
4.2 Matrices
To overcome the challenges inherent in making sense of masses of qualitative data, Lillis (1999) encourages the use of a systematic analytical approach of structured data display as described by Miles & Huberman (2014) . Matrices are a form of data display, defined as the “crossing of two or more main dimensions to see how they interact” (Miles & Huberman , 2014 , p. 239) . They suggest that the process of creating matrices is both creative and systematic. Mason (2012) observes that the selection of dimensions represented on each axis represent important decisions, as they reflect the application of interpretative principles.
The technique aids analytical thinking, making it easier to identify connections or relationships that exist within data (Mason , 2012) . Lillis (1999) points to the usefulness of matrix displays in enhancing trust by: establishing an audit trail from interview transcriptions to results ensuring that all cases are evaluated, and assist in revealing new empirical-based propositions. Reflecting on her own use of matrix displays, Lillis (1999) observes how the matrix data displays establish a disciplined approach that enhance completeness and impartiality. Similarly, O’Dwyer (2008) writes positively of his own experiences using matrices. Specifically, detailed coding combined with overviews obtained through matrices, facilitates a holistic view of the data while also bringing to light interrelationships and contradictions in the data. Furthermore, O’Dwyer (2008) observes how the thoroughness of his data analysis protocol instilled confidence allowing him to be more convincing in articulating his arguments.
4.3 Co-occurrences and C-Ratio
Quantifying the overlap between interview data coded to multiple codes can assist researchers in assessing the strength of the relation between constructs of interest. Overlap is measured through co-occurrences or C-Ratio which are proxies for the level of interaction, between empirical data, coded by researchers as relating to two or more constructs of interest. The range of possible C-Ratio is between 0 (indicating that codes do not co-occur) and 1 (indicating these two codes completely co-occur). Therefore, interrelationships with a high (low) C-Ratio reflect a high (low) interaction between constructs. C-Ratios are calculated using the formula ( https://doc.atlasti.com/ManualWin.v9/ATLAS.ti_ManualWin.v9.pdf ):
The calculation of the C-Ratio is based on approaches borrowed from quantitative content analysis. Code co-occurrences are typically displayed within a data matrix to produce a ‘Code Co-Occurrence Table’. Data analysis software packages, such as Atlas-ti or NVivo, allows users to drill down to retrieve the actual quotations underlying the matrix. In the context of accounting, only a small number of studies have applied this technique. An overview of each follows.
Malina and Selto (2004) examine a large manufacturing company’s efforts to improve profitability through the design and use of a performance measurement model. They coded interview data using data analysis software (Altas-ti) according to whether the interview comments were positive or negative with respect to specified desirable attributes of performance measures identified in the literature. The authors present the co-occurrences between favourable comments relating to specific attributes and unfavourable comments related to other attributes within the same interview text (for measures that earlier analysis identified as being omitted from the design of the performance measurement model). Stronger co-occurrences pointed to a greater trading-off effect and the authors could identify the key trade-offs between attributes. For example, within the data, the attribute of improved decision making was considered subservient (and omitted) relative to objectivity and accuracy attributes.
Malina & Selto (2015) utilises C-Ratios to quantify the interaction between empirical data constructs to identify key factors associated with performance measure model longevity. They first code data to Ferreria and Otley’s (2009) management control framework (key performance measures, target setting, performance evaluation, reward system and information flows) and second, to three behavioural-economic nudges (anchoring and adjustment, availability, conformity and framing).
In the Malina and Selto (2015) study data analysis focused on examining the use of behavioural-economic nudges in an enduring performance measurement model. The authors summarised the frequency of interview excerpts coded to constructs (1,541 in total), within Ferreria and Otley’s (2009) framework (718) and the behavioural economic nudges (877). They argued that code frequencies are proxies “for the overall perceived importance of posited constructs” (p. 35). Analysis proceeded to present a matrix summarising the frequencies which the same text is coded to both categories of constructs resulting in 1,721 co-occurring codes: “Co-occurrences are proxies for interactions of concepts underlying the codes. High co-occurrence frequencies indicate the importance of interacting concepts” p. 36. The authors use C-Ratio (as defined above) to measure the intensity of the interaction between behavioural-economics nudges and the design and use of a performance measurement model. While acknowledging that there is no standard significant level of C-Ratio, the authors focus on interactions with C-Ratios of 0.25 or greater as they suggest, interactions which a relatively higher C-Ratio, reflect the most likely drivers of performance measurement models’ longevity. The evidence regarding co-occurrence within interview content identified four behavioural nudges present in the design and use of the performance measurement model.
Lillis et al. (2017) employ C-Ratios during data analysis to tease out how subjectivity emerges and become informative within performance measurement and reward systems. Interview data was coded to concepts from existing theoretical frameworks using NVivo. The authors perform two subsequent rounds of coding. In the first round, data is coded to informativeness criteria identified in the incentive contracting literature (effort intensity, effort direction, isolating agent effort and congruity). Following this, data is coded to subjective interventions (subjective measures, subjective initial rating, subjective final rating and subjective rewards) with 1,952 data codes assigned to subjectivity, of these 870 related to informativeness codes and 1,082 related to subjective intervention codes. For a more insightful analysis, Lillis et al. (2017) employ a Co-occurrence and C-Ratio technique.
“Evidence in qualitative studies is generally conveyed through the use of quotations along with researcher interpretations of broader patterns in data. It is difficult to convey the ‘weight’ of evidence using this approach, and quotations can only constitute examples from extensive narratives that form the field study data base. To address the challenge of how to present the ‘weight’ of evidence in qualitative data, we adopt a technique which allows us to quantify patterns in the data.” (Lillis et al. , 2017 , p. 19)
Using capabilities within the qualitative data software, Lillis et al. (2017) produce a “matrix of the frequencies of which all code pairing was applied to the same narrative” (p. 18) these Co-occurrences act as proxies for interactions of concepts underlying the codes. This reveals a total of 1,009 co-occurring narratives among informativeness codes and subjectivity codes. The co-occurrences were translated into C-Ratios. High (or low) C-Ratios indicate the importance of interacting concepts (low interaction) in interviewee narratives. The authors set an arbitrary C-Ratio cut-off of 0.20. Six interactions (pairings or cells) meet this cut-off and the findings section is structured around each of these salient interactions (for example one interaction involved subjective measures and effort intensity, while another interaction consisted of subjective initial rating and effort direction). This approach supports Lillis et al. (2017) to focus on “co-occurring codes most worthy of further investigation” (p. 19).
Identifying key interactions between data constructs is a common data-analysis objective in Malina & Selto (2004 , 2015) and Lillis et al. (2017) . These studies all use Co-occurrence and C-Ratios to systematically measure the presence and extent of overlap in data coded to multiple codes. More importantly, C-Ratios permit easy identification of important interactions between constructs of interest. Section 5 focuses on the application of C-Ratios to support data analysis in the context of a doctoral research study.
5. Application of the Structured Analytical Approach and C-Ratio Protocol in A Phd Study
Co-occurrences and the C-Ratio technique described in Section 4.3 formed a key component of data analysis in a PhD study (Martyn , 2018) . This section provides a detailed description of the use of Co-Occurrences and C-Ratios as part of the data analysis process. The broad objective of the PhD was to examine how management control systems guide middle managers’ actions. The management literature suggests that organisational performance is primarily driven by what happens at the middle rather than at top levels of organisational hierarchies (Currie & Procter , 2005) . From a management control perspective only a handful of studies have investigated how management control systems are used to steer the work of middle managers. Both the management literature and the management control systems literature share a related primary interest, improving organisational performance, yet the two streams of literature remain largely discrete. The motivation for the study was to draw together these two streams of literature to advance understanding of how management control systems guide middle managers’ efforts in contemporary organisations. The study draws on two theoretical frames. First, Simons’ (1995) levers of control framework which conceptualises four levers of control that senior managers use to realise strategy: beliefs system, boundary system, control systems used in an interactive manner and control systems used in a diagnostic manner. Second, Floyd and Wooldridges’ (1992 , 1997) middle managers’ strategic roles typology, which categorises the span of middle managers influence on strategy: implementing deliberate strategy, championing alternatives, synthesising information and facilitating adaptability.
Using a multiple case study design (two firms from the medical device sector and two firms in the information technology sector), 43 in-depth semi-structured interviews were conducted with middle managers working in different functional areas. One of the objectives of the study was to examine how modes of control characterised in the levers of control (beliefs systems, boundary systems, interactive control systems and diagnostic control systems) steer different middle manager activities identified in the management literature (implementation of deliberate strategy, synthesising information, championing alternatives and facilitating adaptability). Determining the level of interaction between control levers and middle manager strategic roles was a key concern during data analysis. In addition, the intensity of the interactions between control levers and middle manager strategic roles reveals the extent to which individual control levers steers specific strategic action at middle management level. Applying quantitative analytical procedures, discussed in section 4.3, aligned well with the intent underpinning data analysis in the study.
The structured data analysis process compromised of ten phases of analysis. For completeness, all ten distinct phases of analysis are included, which broadly consist of three concurrent activities (data reduction, data display and conclusion drawing) identified by Miles & Huberman (2014) . Less emphasis is given to phases 1-7 as they are relatively standard across qualitative studies. In contrast, more detailed attention is given to phases 8-10 to provide a detailed account of the application of the C-Ratio technique in an empirical study.
Phase 1: Initial Engagement with the Data
In line with Eisenhardt (1989) and Bryman & Bell (2003) , interview transcripts were read several times and on-site field notes were reviewed to develop an intimate knowledge of the data (O’Dwyer , 2008) .
Phase 2: Creation of Database of Transcripts
Given the large volume of narrative data (interview transcripts totalled 1,290 pages of text) collected during this study, a database of interview transcripts, audio files and field notes were created within NVivo version 10 (qualitative data analysis software package). In addition, both case and interviewee attributes (industry sector, gender and role categorisation) were also recorded.
Phase 3: First Round of Coding
The initial round of coding followed a deductive analysis approach (Moll et al. , 2006) reflecting the study’s strongly theory-driven nature (relying on two theories: levers of control and middle managers’ strategic role typology). Data was coded to category codes constructed from the levers of control framework and broad participant-driven codes.
Phase 4: Descriptive Write-ups
Following Eisenhardt (2002) , descriptive write-ups (including extensive use of summary tables) organised around case and theoretical properties were prepared. Extracting data from NVivo, based on code categories identified in Phase 3 supported this process. The descriptive write-ups helped to identify: recurring themes and patterns; similarities and differences between cases; and how the levers of control were influencing interviewees.
Phase 5: Second Round of Coding
Coding expanded to create data-driven sub-categories to capture behaviours and implications. Using the reporting functionality within NVivo, data was extracted within identified code categories and was subsequently used to prepare data summary tables.
Phase 6: Third Round of Coding
Round three of coding mapped interview data against the characteristics of the middle management strategic involvement typology (Floyd & Wooldridge , 1992 , 1997) identified in the literature. Patterns in how the levers of control could be linked to specific middle manager strategic roles began to emerge.
Phase 7: Review of Volume of Data
By this point, the researcher had spent a prolonged period immersed in analysing the large body of evidence gathered from the field. It was time to reflect on the progress to date and challenges experienced. While the three rounds of coding had certainly aided the researcher in gaining insights, coping with the large volume of data was becoming overwhelming and ‘data asphyxiation’ (Pettigrew , 1988) was setting in.
Phase 8: Adoption of Matrix Approach
To overcome the challenge of analysing large volume of data gathered from four case studies, the researcher applied a structured data display (matrix) approach recommended by Miles & Huberman (2014) as a data reduction tool. As discussed in Section 4.2, this technique aids analytical thinking, the identification of connections or relationships that exist within data, rendering it a suitable approach for the study. Matrices would support the analysis by crossing dimensions, in this case the individual control levers, against middle management strategic roles. Lillis (1999) suggests that research questions should guide matrix design parameters. This recommendation was adhered to in this study: matrix columns represented each of the four levers of control (Beliefs Systems, Boundary Systems, Diagnostic Control Systems and Interactive Control Systems) while matrix rows corresponded to middle management strategic roles (Facilitating, Implementing, Championing and Synthesising). Selecting dimensions represented on each axis is not analytically neutral; the researcher had to reflect on how this would affect the process of interpreting the data and its appropriateness in terms of addressing the questions posed in the study.
Based on the iterative coding process (phases 3, 5 and 6), significant segments of interview narrative were coded to both the levers of control and middle manager strategic role codes in NVivo. More in-depth analysis of these coding overlaps was key to gaining focused insights relevant to addressing the research objective. Using NVivo’s Crosstab functionality, the researcher constructed a two-way matrix template. Table 1 illustrates the outline of the template matrix structure (unpopulated). Using this matrix template, matrices were generated from interview data coded in NVivo for each case firm, role categorisation (marketing, finance, manufacturing) and industry sector (IT and medical device). As the matrices crossed two dimensions (levers of control and middle manager strategic roles), they permitted a greater understanding of the extent to which the two dimensions interact revealing, as Lillis (2006) suggests, links between empirical observations and theory.
Phase 9: Use of C-Ratios
To build further on this line of analysis further and assess the strength of the interaction between the individual control levers and middle manager strategic roles, the researcher applied a systematic approach to reveal the “weight of evidence” following a procedure used in previous studies outlined in Section 4.3 (Lillis et al. , 2017; Malina & Selto , 2004 , 2015) . This approach involved calculating C-Ratios to quantify the relative strength of interactions between the two dimensions of interest in this study. Several steps were required. First, using NVivo’s Query functionality, the researcher counted instances of narratives coded to levers of control and middle manager dimensions to create a code frequency table ( Table 2 ).
Second, the researcher developed a two-way crosstab display in NVivo to summarise the co-occurrences between levers of control and middle manager strategic roles, forming a matrix of coding co-occurrences as illustrated in Table 3 . Essentially, this summarised the frequency that discrete narratives had been coded to each code pairing (meaning the narrative was coded to both the lever of control and the middle manager strategic role during phases 3, 5 and 6). For example, 105 discrete narratives were coded to both beliefs systems and middle management’s facilitating role.
Third, by translating co-occurrence frequency measures into a relative measure, the researcher was able to evaluate the strength of specific interactions. To normalise the coding co-occurrence frequency (Lillis et al. , 2017) absolute co-occurrence measures were adjusted to C-Ratios using the formula: C 12 =n 12 /(n 1 +n 2 -n 12 ). The C-Ratio between beliefs system and middle managers’ facilitating role is calculated as follows: 105/(777+300-105) = 0.11 where 105 (shaded in Table 3 ) is the co-occurrence between beliefs systems and facilitating role, 777 (shaded in Table 2 ) is the narrative coding frequency of facilitating and 300 (shaded in Table 2 ) is the narrative coding frequency of beliefs system.
As highlighted in Section 4.3, a C-Ratio has a value between 0 (indicating no co-occurrence) and 1 (indicating complete co-occurrence). Therefore, interrelationships with a high (low) C-Ratio reflect a high (low) interaction between underlying dimensions. For instance, in Table 4 , the data reveals a strong interaction (C-Ratio of 0.38) between narratives coded to the diagnostic control system lever and middle managements’ implementing strategy role. In contrast, there is little interaction (C-Ratio of 0.02) between narratives coded to the beliefs systems and middle managements’ synthesising role.
Phase 10: Final Write-up of Findings
Matrices aided the researcher in identifying the linkages in the empirical evidence, specifically which control levers interacted with particular middle management roles, and perhaps more importantly, the C-Ratio technique quantified the strength of the interaction or what Lillis et al. (2017) refer to as the weight of the evidence. As this analysis procedure was applied to the entire dataset and in turn to cross-sections of data (each firm, each role category, and each industry sector), this enabled the researcher to find patterns, make contrasts and comparisons. Using this technique, salient interactions were immediately evident (higher C-Ratios) and this served as a strong guide during the write up process. For example, the C-Ratio analysis revealed a strong interaction (0.37) between middle managers’ synthesising strategic role and control systems used in an interactive manner. This suggest that interactive control systems are a salient mode of control in guiding middle managers’ synthesising activities. Similarly, the relatively high C-Ratio (0.38) observed between diagnostic control systems and middle managers’ role in implementing strategy indicates that diagnostic control systems exert a strong influence on middle managers as they implement organisational strategy.
The C-Ratios displayed in Table 4 summarise the relative weight of interaction between code pairings, the researcher could, at the ‘press of a button’ in NVivo, drill down into the detailed narratives permitting ready access to relevant quotations. The ease with which the researcher could switch between the ‘high level’ and drill down into granular detail aided the write up process.
6. Conclusions and Lessons Learned
This paper describes ten phases of data analysis in a structured analytical approach to analyse qualitative data using matrices (Miles & Huberman , 2014) and calculation of C-Ratios. The study sought to enhance understanding of how different modes of control steer middle managers to fulfil several strategic roles. The C-Ratio technique, in quantifying the frequency and strength of the association between the two constructs, helped to illuminate on how this happens in practice. Furthermore, the process signalled associations that were, as Miles & Huberman (2014) suggest, ‘important’ and ‘significant’. This ‘signposting’ helped to verify the researcher’s intuitions about key linkages within the data.
On reflection, the analytical protocol followed was useful for several reasons. First, the protocol helped to alleviate the challenge of analysing substantial volumes of interview data. The process of constructing the matrices followed a structured procedure. This contrasted with the more reflective nature of the preceding phases. The two approaches, reflective and quantification, combined well, allowing scope for rich descriptions to emerge while preserving focus on key connections. Second, the analytical protocol was also helpful in pacing the analysis. Each cell in the matrices represented an interaction to potentially tease out further, albeit that some warranted more attention than others. This configuration provided a natural way to structure deeper analysis, one interaction at a time. Working through each interaction in a paced approach made the task at hand far more manageable and served as a reference to assess progress. O’Dwyer (2008) observes that, irrespective of the process of analysis, significant perseverance is necessary. A cell at a time tactic served to regulate the researcher’s perseverance. Third, the researcher was aware of the requirement to analyse the data in a complete and unbiased way. The process adopted supported these aims. Matrices effectively responded to the issue of completeness as all data was evaluated. As Lillis (2002 , p. 511) points out, analysis is “built on interpretation…. therefore potentially subject to considerable bias”. The ability of the C-Ratios to capture the strength of interaction (weight of the evidence) between dimensions helped prevent bias. Fourth, the task of making inferences and drawing conclusions rests firmly with the researcher. The C-Ratios bolstered intuitive insights and enabled the researcher to be more confident in the interpretation and claims made (Miles & Huberman , 2014) . Learning to construct convincing arguments is essential during the PhD journey. The analytical process supported that aim.
In summary, the analytical choices made were matched with the objectives of the research as focus rested on understanding the interconnection between specific control levers and specific middle manager strategic roles. The importance of designing an analytical approach that supports the researcher in addressing their research questions cannot be over stated. While this study drew on a quantitative technique to support qualitative data analysis, the author does not claim the use of this method of data analysis as superior to others; merely that it was appropriate and justified in the context of this PhD study.
Some drawbacks in using co-occurrences and C-Ratios surfaced. First, constructing the matrices and C-Ratios together, with the associated preparatory work, represented a considerable investment in time. The process was repetitive; prepared at an overall summary level (illustrated in Tables 1 to 4 ) but also for each of the four case firms, each of the five role categories and two industry categories. Second, the structure of the analysis did not easily reveal how control levers worked in combination to steer middle managers’ strategic efforts. It is widely recognised that, in practice, individual control mechanisms are interrelated. Examining an interaction between one control lever and one strategic role did not capture this and further analysis was necessary. The process is heavily reliant on a well thought through and consistently executed coding structure. In addition, the use of the technique required a detailed explanation in the methods chapter of the thesis. Explaining in detail what was done, and why it was done in the selected way (Modell & Humphrey , 2008) , was necessary to ensure that the reader could trust the research.
Sharing the analytical approach in this paper is motivated by several aims. First, to encourage researchers to consider, where appropriate, supplementing qualitative analysis methods with protocols that would more traditionally be associated with the quantitative domain. Second, the paper illustrates a technique for managing large volumes of data typically gathered in a qualitative study at doctoral level. This may be helpful to other PhD researchers who find themselves becoming overwhelmed at the critical data-analysis stage. Furthermore, advances in Artificial Intelligence technology in the area of auto-transcribing means that volumes of interview data can now be transcribed relatively easily, making interview data potentially more attractive as a data source for doctoral students. Third, the analytical approach struck a balance between, on one hand, the need to allow rich findings (the creative) to emerge, while on the other hand, simultaneously providing the means to establish adequate trust in the findings (Modell & Humphrey , 2008) .
Acknowledgements
I am grateful to Breda Sweeney for her comments on an earlier version of this paper. Furthermore, I appreciate the helpful feedback of the editor and two anonymous reviewers. I gratefully acknowledge the guidance given by Anne Lillis in applying the C-Ratio technique.
Think of yourself as a member of a jury, listening to a lawyer who is presenting an opening argument. You'll want to know very soon whether the lawyer believes the accused to be guilty or not guilty, and how the lawyer plans to convince you. Readers of academic essays are like jury members: before they have read too far, they want to know what the essay argues as well as how the writer plans to make the argument. After reading your thesis statement, the reader should think, "This essay is going to try to convince me of something. I'm not convinced yet, but I'm interested to see how I might be."
An effective thesis cannot be answered with a simple "yes" or "no." A thesis is not a topic; nor is it a fact; nor is it an opinion. "Reasons for the fall of communism" is a topic. "Communism collapsed in Eastern Europe" is a fact known by educated people. "The fall of communism is the best thing that ever happened in Europe" is an opinion. (Superlatives like "the best" almost always lead to trouble. It's impossible to weigh every "thing" that ever happened in Europe. And what about the fall of Hitler? Couldn't that be "the best thing"?)
A good thesis has two parts. It should tell what you plan to argue, and it should "telegraph" how you plan to argue—that is, what particular support for your claim is going where in your essay.
Steps in Constructing a Thesis
First, analyze your primary sources. Look for tension, interest, ambiguity, controversy, and/or complication. Does the author contradict himself or herself? Is a point made and later reversed? What are the deeper implications of the author's argument? Figuring out the why to one or more of these questions, or to related questions, will put you on the path to developing a working thesis. (Without the why, you probably have only come up with an observation—that there are, for instance, many different metaphors in such-and-such a poem—which is not a thesis.)
Once you have a working thesis, write it down. There is nothing as frustrating as hitting on a great idea for a thesis, then forgetting it when you lose concentration. And by writing down your thesis you will be forced to think of it clearly, logically, and concisely. You probably will not be able to write out a final-draft version of your thesis the first time you try, but you'll get yourself on the right track by writing down what you have.
Keep your thesis prominent in your introduction. A good, standard place for your thesis statement is at the end of an introductory paragraph, especially in shorter (5-15 page) essays. Readers are used to finding theses there, so they automatically pay more attention when they read the last sentence of your introduction. Although this is not required in all academic essays, it is a good rule of thumb.
Anticipate the counterarguments. Once you have a working thesis, you should think about what might be said against it. This will help you to refine your thesis, and it will also make you think of the arguments that you'll need to refute later on in your essay. (Every argument has a counterargument. If yours doesn't, then it's not an argument—it may be a fact, or an opinion, but it is not an argument.)
This statement is on its way to being a thesis. However, it is too easy to imagine possible counterarguments. For example, a political observer might believe that Dukakis lost because he suffered from a "soft-on-crime" image. If you complicate your thesis by anticipating the counterargument, you'll strengthen your argument, as shown in the sentence below.
Some Caveats and Some Examples
A thesis is never a question. Readers of academic essays expect to have questions discussed, explored, or even answered. A question ("Why did communism collapse in Eastern Europe?") is not an argument, and without an argument, a thesis is dead in the water.
A thesis is never a list. "For political, economic, social and cultural reasons, communism collapsed in Eastern Europe" does a good job of "telegraphing" the reader what to expect in the essay—a section about political reasons, a section about economic reasons, a section about social reasons, and a section about cultural reasons. However, political, economic, social and cultural reasons are pretty much the only possible reasons why communism could collapse. This sentence lacks tension and doesn't advance an argument. Everyone knows that politics, economics, and culture are important.
A thesis should never be vague, combative or confrontational. An ineffective thesis would be, "Communism collapsed in Eastern Europe because communism is evil." This is hard to argue (evil from whose perspective? what does evil mean?) and it is likely to mark you as moralistic and judgmental rather than rational and thorough. It also may spark a defensive reaction from readers sympathetic to communism. If readers strongly disagree with you right off the bat, they may stop reading.
An effective thesis has a definable, arguable claim. "While cultural forces contributed to the collapse of communism in Eastern Europe, the disintegration of economies played the key role in driving its decline" is an effective thesis sentence that "telegraphs," so that the reader expects the essay to have a section about cultural forces and another about the disintegration of economies. This thesis makes a definite, arguable claim: that the disintegration of economies played a more important role than cultural forces in defeating communism in Eastern Europe. The reader would react to this statement by thinking, "Perhaps what the author says is true, but I am not convinced. I want to read further to see how the author argues this claim."
A thesis should be as clear and specific as possible. Avoid overused, general terms and abstractions. For example, "Communism collapsed in Eastern Europe because of the ruling elite's inability to address the economic concerns of the people" is more powerful than "Communism collapsed due to societal discontent."
Copyright 1999, Maxine Rodburg and The Tutors of the Writing Center at Harvard University
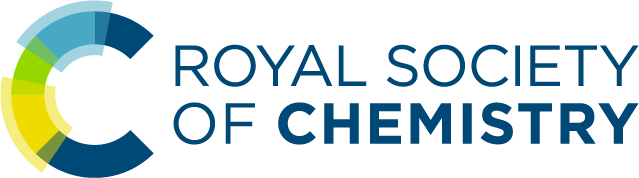
Journals, books & databases
- Our journals
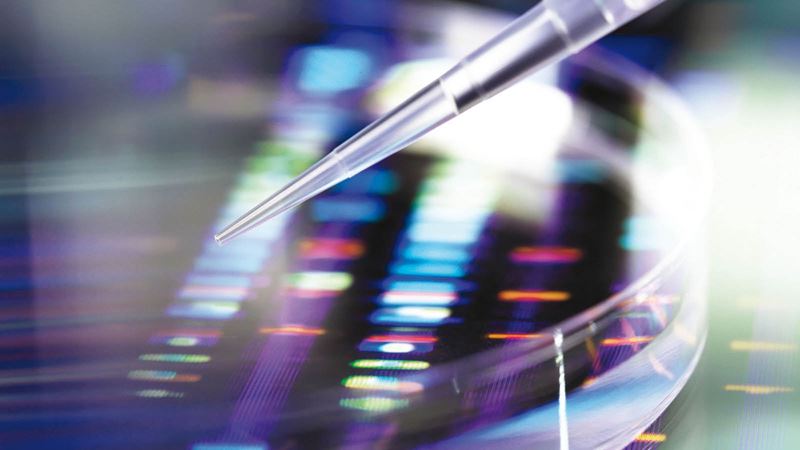
Analytical Methods
Early applications of new analytical methods and technology demonstrating potential for societal impact
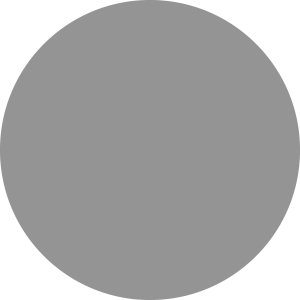
You can find details about how to access information remotely in this step-by-step guide . The guide will also help if for any reason you have difficulty accessing the content you want.
What would you like to know about this journal?
Analytical Methods is a Transformative Journal and Plan S compliant
Impact factor: 3.1*
Time to first decision (all decisions): 11.0 days**
Time to first decision (peer reviewed only): 30.0 days***
Editor-in-Chief: B. Jill Venton
Indexed in MEDLINE
Open access publishing options available
Read this journal
Submit an article
Sign up for regular email alerts
View all journal metrics
Publish open access
Meet the team
Journal scope
Analytical Methods welcomes early applications of new analytical and bioanalytical methods and technology demonstrating the potential for societal impact.
We require that methods and technology reported in the journal are sufficiently innovative, robust, accurate, and compared to other available methods for the intended application. Developments with interdisciplinary approaches are particularly welcome. Systems should be proven with suitably complex and analytically challenging samples.
We encourage developments within, but not limited to, the following technologies and applications:
- global health, point-of-care and molecular diagnostics
- biosensors and bioengineering
- drug development and pharmaceutical analysis
- applied microfluidics and nanotechnology
- omics studies, such as proteomics, metabolomics or glycomics
- environmental, agricultural and food science
- neuroscience
- biochemical and clinical analysis
- forensic analysis
- industrial process and method development
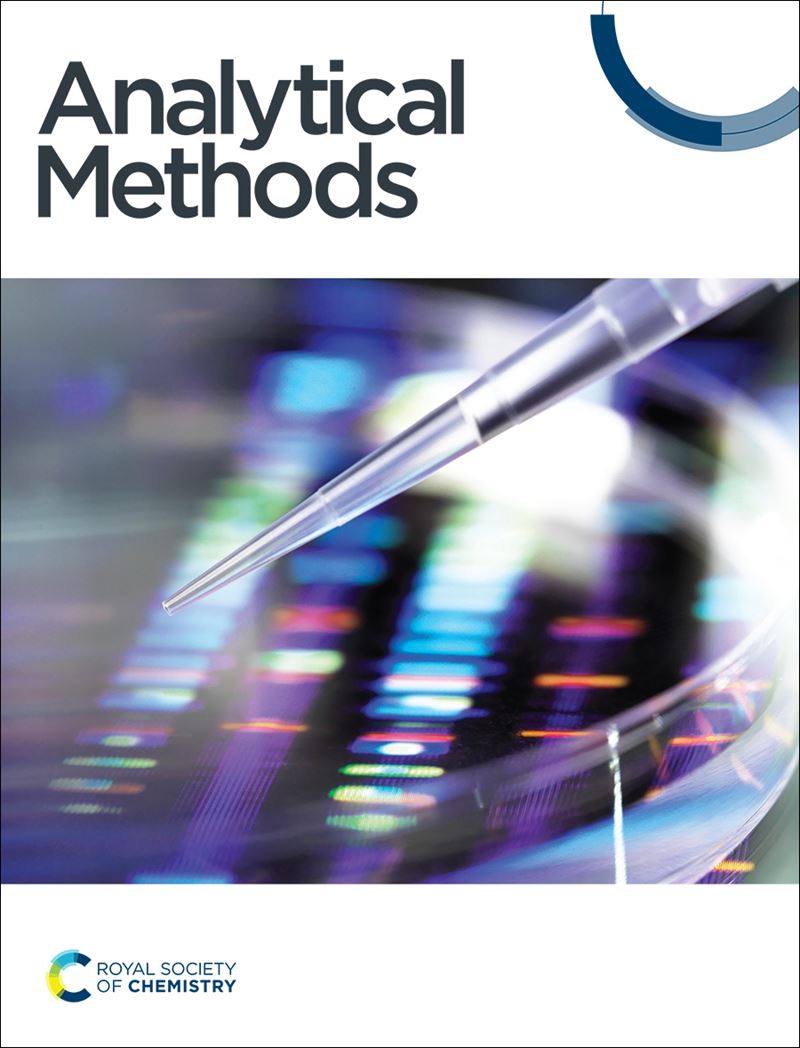
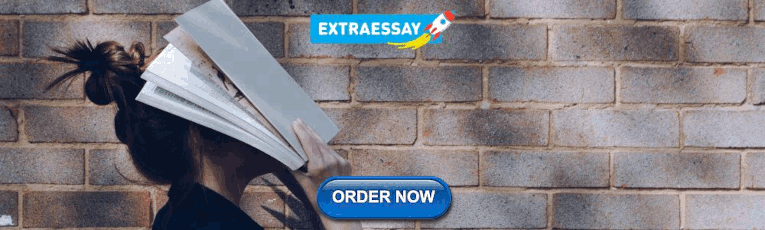
Meet the editorial team
Find out who is on the editorial and advisory boards for the Analytical Methods journal.
Editor-in-chief
B. Jill Venton , University of Virginia, USA
Associate editors
Martina Catani , University of Ferrara, Italy
Wendell Coltro , Federal University of Goiás, Brazil
Juan F García-Reyes , University of Jaén, Spain
Tony Killard , Reviews Editor, University of West England, UK
Zhen Liu , Nanjing University, China
Matthew Lockett , University of North Carolina at Chapel Hill, USA
Chao Lu , Beijing University of Chemical Technology, China
Fiona Regan , Dublin City University, Ireland
Jailson de Andrade , Universidade Federal da Bahia, Brazil
Lane Baker , Indiana University, USA
Craig Banks , Manchester Metropolitan University, UK
Jonas Bergquist , Uppsala University, Sweden
Emanuel Carrilho , São Carlos, Brazil
James Chapman , The University of Queensland, Australia
Yi Chen , Chinese Academy of Sciences, China
Christopher J. Easley , Auburn University, USA
Anthony Gachanja , Jomo Kenyatta University of Agriculture and Technology, Kenya
Amanda Hummon , Ohio State University, USA
Lauro Kubota , Instituto de Química, Brazil
Ally Lewis , University of York, UK
Juewen Liu , University of Waterloo, Canada
Susan Lunte, University of Kansas, USA
Jim Luong , Dow Chemical Canada ULC, Canada
Scott Martin , Saint Louis University, USA
Susheel Mittal , Thapar University, India
Antonio Molina-Díaz , University of Jaén, Spain
Koji Otsuka , Kyoto University, Japan
Brett Paull , University of Tasmania, Australia
Michael Roper , Florida State University, USA
Zachary Schultz , Ohio State University, USA
Sabeth Verpoorte , University of Groningen, Netherlands
Guobao Xu , Changchun Institute of Applied Chemistry, China
Rebecca Garton , Executive Editor
Alice Smallwood , Deputy Editor
Celeste Brady , Development Editor
David Lake , Development Editor
Jason Woolford , Editorial Production Manager
Gabriel Clarke , Publishing Editor
Derya Kara-Fisher , Publishing Editor
Emma Stephen , Publishing Editor
Ziva Whitelock , Publishing Editor
Leo Curtis , Editorial Assistant
Andrea Whiteside , Publishing Assistant
Jeanne Andres , Publisher
Article types
Analytical Methods publishes:
Communications
Full papers, technical notes, critical reviews, minireviews, tutorial reviews.
These must report preliminary research findings that are highly original, of immediate interest and are likely to have a high impact. Communications are given priority treatment, are fast-tracked through the publication process and appear prominently at the front of the journal.
The key aim of Communications is to present innovative concepts with important analytical implications. As such, Communications need only demonstrate 'proof of principle': it is not expected that the analytical figures of merit will necessarily surpass those of existing, highly refined analytical techniques.
At the time of submission, authors should also provide a justification for urgent publication as a Communication. Ideally, a Full paper should follow each Communication in an appropriate primary journal.
There is no page limit for communications in Analytical Methods , however the length should be commensurate with scientific content. Authors are encouraged to make full use of electronic supplementary information (ESI) in order to present more concise articles.
These must describe science that will be of benefit to the community in the particular field of analysis and are judged according to originality, quality of scientific content and contribution to existing knowledge.
Although there is no page limit for Full papers, appropriateness of length to content of new science will be taken into consideration.
These should be brief descriptions of developments, techniques or applications that offer definite advantages over those already available. Technical notes should offer practical solutions to problems that are of interest to the readership and merit publication, but where a Full paper is not justified.
Technical notes should be as brief as possible; wherever appropriate authors should use references to the established technique, explaining in full only what is novel about the proposed approach.
Critical reviews are definitive, comprehensive reviews but must also provide a critical evaluation of the chosen topic area. Authors should try to be selective in the choice of material, whilst still aim to cover all the important work in the field, also indicating possible future developments.
Minireviews are highlights or summaries of research in an emerging area of analytical science covering approximately the last two-three years. Given topics should review work no more than approximately 36 months old, and articles should cover only the most interesting/significant developments in that specific subject area.
The articles should be highly critical and selective in referencing published work. A small amount of speculation (one or two paragraphs) of possible future developments may also be appropriate in the Conclusions section.
Written from a personal point of view, these ideally should be the first review of a new significant area, bringing together the results of various primary publications.
Tutorial reviews are intended to interest a large number of readers and should be written at a level that could be understood by an advanced undergraduate student.
The intention is to increase awareness and understanding of the chosen topic area for workers/researchers already involved in the field, workers changing the direction/emphasis of their work and a broad based non-specialist (graduate and post-graduate) audience, with a view to informing them of the most recent developments in the area.
Potential writers should contact the editorial office before embarking on their work.
Comments and Replies are a medium for the discussion and exchange of scientific opinions between authors and readers concerning material published in Analytical Methods .
For publication, a Comment should present an alternative analysis of and/or new insight into the previously published material. Any Reply should further the discussion presented in the original article and the Comment. Comments and Replies that contain any form of personal attack are not suitable for publication.
Comments that are acceptable for publication will be forwarded to the authors of the work being discussed, and these authors will be given the opportunity to submit a Reply. The Comment and Reply will both be subject to rigorous peer review in consultation with the journal’s Editorial Board where appropriate. The Comment and Reply will be published together.
Journal specific guidelines
On submission, authors are required to submit a short significance statement for their manuscript. This statement should address the technological advance and/or significance of the methods and applications in the presented work (1–2 sentences maximum). This information will help the Editor and reviewers assess the article.
How do Analyst and Analytical Methods compare? From discovery to recovery – Analyst and Analytical Methods working together for the analytical community Analyst , 2011, 136 , 429 DOI: 10.1039/c0an90013c
Open access publishing options
Analytical Methods is a hybrid (transformative) journal and gives authors the choice of publishing their research either via the traditional subscription-based model or instead by choosing our gold open access option. Find out more about our Transformative Journals. which are Plan S compliant .
Gold open access
For authors who want to publish their article gold open access , Analytical Methods charges an article processing charge (APC) of £2,750 (+ any applicable tax). Our APC is all-inclusive and makes your article freely available online immediately, permanently, and includes your choice of Creative Commons licence (CC BY or CC BY-NC) at no extra cost. It is not a submission charge, so you only pay if your article is accepted for publication.
Learn more about publishing open access .
Read & Publish
If your institution has a Read & Publish agreement in place with the Royal Society of Chemistry, APCs for gold open access publishing in Analytical Methods may already be covered.
Check if your institution is already part of our Read & Publish community .
Please use your official institutional email address to submit your manuscript; this helps us to identify if you are eligible for Read & Publish or other APC discounts.
Traditional subscription model
Authors can also publish in Analytical Methods via the traditional subscription model without needing to pay an APC. Articles published via this route are available to institutions and individuals who subscribe to the journal. Our standard licence allows you to make the accepted manuscript of your article freely available after a 12-month embargo period. This is known as the green route to open access.
Learn more about green open access .
Peer review
Analytical Methods follows a single-anonymised peer review process and articles are typically sent to at least two independent reviewers for evaluation. A dynamic and high-quality team of associate editors is responsible for peer review and associated editorial decisions. Authors may choose their preferred choice of associate editor upon submission.
Please note that it may not always be possible for the author's first choice associate editor to be selected. In situations where this is not possible the editorial office will assign the most suitable alternative.
On submission to the journal, all manuscripts are assigned to an external (academic) Associate Editor. Each submission is assessed for quality, scope, and impact. Those that do not meet the criteria based on these factors are rejected without further peer review. Otherwise, the article is sent to at least two external reviewers with expertise in the article topic for confidential review. An editorial decision to reject or accept the article is made on these reports.
More reviewers may be consulted in cases of opposing reports or when more clarification is needed. Articles needing significant revisions may be sent for further peer review before acceptance. Authors may appeal a rejection via communication with the Associate Editor. Our processes and policies can provide full details of the initial assessment process.
Readership information
Readership is cross-disciplinary and Analytical Methods appeals to readers across academia and industry, who have an interest in the advancement of measurement science and the breadth of application of analytical and bioanalytical methodologies.
These include, but are not restricted to, analytical and environmental scientists; biochemists and biotechnologists; process and industrial scientists; biomedical and clinical scientists; forensic and heritage scientists; agriculture, food, safety and product technologists; pharmaceutical scientists and toxicologists.
Subscription information
Analytical Methods is part of RSC Gold and Analytical Science subscription packages. Online only 2024 : ISSN 1759-9679 £2,513 / $4,425
*2022 Journal Citation Reports (Clarivate Analytics, 2023)
**The median time from submission to first decision including manuscripts rejected without peer review from the previous calendar year
***The median time from submission to first decision for peer-reviewed manuscripts from the previous calendar year
Advertisement
Module 8: Analysis and Synthesis
Analytical thesis statements, learning objective.
- Describe strategies for writing analytical thesis statements
- Identify analytical thesis statements
In order to write an analysis, you want to first have a solid understanding of the thing you are analyzing. Remember, when you are analyzing as a writer, you are:
- Breaking down information or artifacts into component parts
- Uncovering relationships among those parts
- Determining motives, causes, and underlying assumptions
- Making inferences and finding evidence to support generalizations
You may be asked to analyze a book, an essay, a poem, a movie, or even a song. For example, let’s suppose you want to analyze the lyrics to a popular song. Pretend that a rapper called Escalade has the biggest hit of the summer with a song titled “Missing You.” You listen to the song and determine that it is about the pain people feel when a loved one dies. You have already done analysis at a surface level and you want to begin writing your analysis. You start with the following thesis statement:
Escalade’s hit song “Missing You” is about grieving after a loved one dies.
There isn’t much depth or complexity to such a claim because the thesis doesn’t give much information. In order to write a better thesis statement, we need to dig deeper into the song. What is the importance of the lyrics? What are they really about? Why is the song about grieving? Why did he present it this way? Why is it a powerful song? Ask questions to lead you to further investigation. Doing so will help you better understand the work, but also help you develop a better thesis statement and stronger analytical essay.
Formulating an Analytical Thesis Statement
When formulating an analytical thesis statement in college, here are some helpful words and phrases to remember:
- What? What is the claim?
- How? How is this claim supported?
- So what? In other words, “What does this mean, what are the implications, or why is this important?”
Telling readers what the lyrics are might be a useful way to let them see what you are analyzing and/or to isolate specific parts where you are focusing your analysis. However, you need to move far beyond “what.” Instructors at the college level want to see your ability to break down material and demonstrate deep thinking. The claim in the thesis statement above said that Escalade’s song was about loss, but what evidence do we have for that, and why does that matter?
Effective analytical thesis statements require digging deeper and perhaps examining the larger context. Let’s say you do some research and learn that the rapper’s mother died not long ago, and when you examine the lyrics more closely, you see that a few of the lines seem to be specifically about a mother rather than a loved one in general.
Then you also read a recent interview with Escalade in which he mentions that he’s staying away from hardcore rap lyrics on his new album in an effort to be more mainstream and reach more potential fans. Finally, you notice that some of the lyrics in the song focus on not taking full advantage of the time we have with our loved ones. All of these pieces give you material to write a more complex thesis statement, maybe something like this:
In the hit song “Missing You,” Escalade draws on his experience of losing his mother and raps about the importance of not taking time with family for granted in order to connect with his audience.
Such a thesis statement is focused while still allowing plenty of room for support in the body of your paper. It addresses the questions posed above:
- The claim is that Escalade connects with a broader audience by rapping about the importance of not taking time with family for granted in his hit song, “Missing You.”
- This claim is supported in the lyrics of the song and through the “experience of losing his mother.”
- The implications are that we should not take the time we have with people for granted.
Certainly, there may be many ways for you to address “what,” “how,” and “so what,” and you may want to explore other ideas, but the above example is just one way to more fully analyze the material. Note that the example above is not formulaic, but if you need help getting started, you could use this template format to help develop your thesis statement.
Through ________________(how?), we can see that __________________(what?), which is important because ___________________(so what?). [1]
Just remember to think about these questions (what? how? and so what?) as you try to determine why something is what it is or why something means what it means. Asking these questions can help you analyze a song, story, or work of art, and can also help you construct meaningful thesis sentences when you write an analytical paper.
Key Takeaways for analytical theses
Don’t be afraid to let your claim evolve organically . If you find that your thinking and writing don’t stick exactly to the thesis statement you have constructed, your options are to scrap the writing and start again to make it fit your claim (which might not always be possible) or to modify your thesis statement. The latter option can be much easier if you are okay with the changes. As with many projects in life, writing doesn’t always go in the direction we plan, and strong analysis may mean thinking about and making changes as you look more closely at your topic. Be flexible.
Use analysis to get you to the main claim. You may have heard the simile that analysis is like peeling an onion because you have to go through layers to complete your work. You can start the process of breaking down an idea or an artifact without knowing where it will lead you or without a main claim or idea to guide you. Often, careful assessment of the pieces will bring you to an interesting interpretation of the whole. In their text Writing Analytically , authors David Rosenwasser and Jill Stephen posit that being analytical doesn’t mean just breaking something down. It also means constructing understandings. Don’t assume you need to have deeper interpretations all figured out as you start your work.
When you decide upon the main claim, make sure it is reasoned . In other words, if it is very unlikely anyone else would reach the same interpretation you are making, it might be off base. Not everyone needs to see an idea the same way you do, but a reasonable person should be able to understand, if not agree, with your analysis.
Look for analytical thesis statements in the following activity.
Using Evidence
An effective analytical thesis statement (or claim) may sound smart or slick, but it requires evidence to be fully realized. Consider movie trailers and the actual full-length movies they advertise as an analogy. If you see an exciting one-minute movie trailer online and then go see the film only to leave disappointed because all the good parts were in the trailer, you feel cheated, right? You think you were promised something that didn’t deliver in its execution. A paper with a strong thesis statement but lackluster evidence feels the same way to readers.
So what does strong analytical evidence look like? Think again about “what,” “how,” and “so what.” A claim introduces these interpretations, and evidence lets you show them. Keep in mind that evidence used in writing analytically will build on itself as the piece progresses, much like a good movie builds to an interesting climax.
Key Takeaways about evidence
Be selective about evidence. Having a narrow thesis statement will help you be selective with evidence, but even then, you don’t need to include any and every piece of information related to your main claim. Consider the best points to back up your analytic thesis statement and go deeply into them. (Also, remember that you may modify your thesis statement as you think and write, so being selective about what evidence you use in an analysis may actually help you narrow down what was a broad main claim as you work.) Refer back to our movie theme in this section: You have probably seen plenty of films that would have been better with some parts cut out and more attention paid to intriguing but underdeveloped characters and/or ideas.
Be clear and explicit with your evidence. Don’t assume that readers know exactly what you are thinking. Make your points and explain them in detail, providing information and context for readers, where necessary. Remember that analysis is critical examination and interpretation, but you can’t just assume that others always share or intuit your line of thinking. Need a movie analogy? Think back on all the times you or someone you know has said something like “I’m not sure what is going on in this movie.”
Move past obvious interpretations. Analyzing requires brainpower. Writing analytically is even more difficult. Don’t, however, try to take the easy way out by using obvious evidence (or working from an obvious claim). Many times writers have a couple of great pieces of evidence to support an interesting interpretation, but they feel the need to tack on an obvious idea—often more of an observation than analysis—somewhere in their work. This tendency may stem from the conventions of the five-paragraph essay, which features three points of support. Writing analytically, though, does not mean writing a five-paragraph essay (not much writing in college does). Develop your other evidence further or modify your main idea to allow room for additional strong evidence, but avoid obvious observations as support for your main claim. One last movie comparison? Go take a look at some of the debate on predictable Hollywood scripts. Have you ever watched a movie and felt like you have seen it before? You have, in one way or another. A sharp reader will be about as interested in obvious evidence as he or she will be in seeing a tired script reworked for the thousandth time.
One type of analysis you may be asked to write is a literary analysis, in which you examine a piece of text by breaking it down and looking for common literary elements, such as character, symbolism, plot, setting, imagery, and tone.
The video below compares writing a literary analysis to analyzing a team’s chances of winning a game—just as you would look at various factors like the weather, coaching, players, their record, and their motivation for playing. Similarly, when analyzing a literary text you want to look at all of the literary elements that contribute to the work.
The video takes you through the story of Cinderalla as an example, following the simplest possible angle (or thesis statement), that “Dreams can come true if you don’t give up.” (Note that if you were really asked to analyze Cinderella for a college class, you would want to dig deeper to find a more nuanced and interesting theme, but it works well for this example.) To analyze the story with this theme in mind, you’d want to consider the literary elements such as imagery, characters, dialogue, symbolism, the setting, plot, and tone, and consider how each of these contribute to the message that “Dreams can come true if you don’t give up.”
You can view the transcript for “How to Analyze Literature” here (opens in new window) .
Contribute!
Improve this page Learn More
- UCLA Undergraduate Writing Center. "What, How and So What?" Approaching the Thesis as a Process. https://wp.ucla.edu/wp-content/uploads/2016/01/UWC_handouts_What-How-So-What-Thesis-revised-5-4-15-RZ.pdf ↵
- Keys to Successful Analysis. Authored by : Guy Krueger. Provided by : University of Mississippi. License : CC BY-SA: Attribution-ShareAlike
- Thesis Statement Activity. Authored by : Excelsior OWL. Located at : https://owl.excelsior.edu/research/thesis-or-focus/thesis-or-focus-thesis-statement-activity/ . License : CC BY: Attribution
- What is Analysis?. Authored by : Karen Forgette. Provided by : University of Mississippi. License : CC BY: Attribution
- How to Analyze Literature. Provided by : HACC, Central Pennsylvania's Community College. Located at : https://www.youtube.com/watch?v=pr4BjZkQ5Nc . License : Other . License Terms : Standard YouTube License
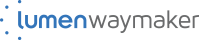
- Open access
- Published: 13 July 2021
QbD approach to HPLC method development and validation of ceftriaxone sodium
- Krunal Y. Patel 1 ,
- Zarna R. Dedania 1 ,
- Ronak R. Dedania 1 &
- Unnati Patel 2
Future Journal of Pharmaceutical Sciences volume 7 , Article number: 141 ( 2021 ) Cite this article
19k Accesses
31 Citations
Metrics details
Quality by design (QbD) refers to the achievement of certain predictable quality with desired and predetermined specifications. A quality-by-design approach to method development can potentially lead to a more robust/rugged method due to emphasis on risk assessment and management than traditional or conventional approach. An important component of the QbD is the understanding of dependent variables, various factors, and their interaction effects by a desired set of experiments on the responses to be analyzed. The present study describes the risk based HPLC method development and validation of ceftriaxone sodium in pharmaceutical dosage form.
An efficient experimental design based on central composite design of two key components of the RP-HPLC method (mobile phase and pH) is presented. The chromatographic conditions were optimized with the Design Expert software 11.0 version, i.e., Phenomenex ODS column C18 (250 mm × 4.6 mm, 5.0 μ), mobile phase used acetonitrile to water (0.01% triethylamine with pH 6.5) (70:30, v/v), and the flow rate was 1 ml/min with retention time 4.15 min. The developed method was found to be linear with r 2 = 0.991 for range of 10–200 μg/ml at 270 nm detection wavelength. The system suitability test parameters, tailing factor and theoretical plates, were found to be 1.49 and 5236. The % RSD for intraday and inter day precision was found to be 0.70–0.94 and 0.55–0.95 respectively. The robustness values were less than 2%. The assay was found to be 99.73 ± 0.61%. The results of chromatographic peak purity indicate the absence of any coeluting peaks with the ceftriaxone sodium peak. The method validation parameters were in the prescribed limit as per ICH guidelines.
The central composite design experimental design describes the interrelationships of mobile phase and pH at three different level and responses to be observed were retention time, theoretical plates, and peak asymmetry with the help of the Design Expert 11.0 version. Here, a better understanding of the factors that influence chromatographic separation with greater confidence in the ability of the developed HPLC method to meet their intended purposes is done. The QbD approach to analytical method development was used for better understanding of method variables with different levels.
A QbD is defined as “A systemic approach to the method development that begins with predefined objectives and emphasizes product and process understanding and process control, based on sound science and quality risk management [ 1 ].” The QbD approach emphasizes product and process understanding with quality risk management and controls, resulting in higher assurance of product quality, regulatory flexibility, and continual improvement. The QbD method was based on the understanding and implementation of guidelines ICH Q8 Pharmaceutical Development, ICH Q9 Quality Risk Management, and ICH Q10 Pharmaceutical Quality System [ 2 , 3 , 4 ]. Analytical science is considered to be an integral part of pharmaceutical product development and hence go simultaneously during the entire product life cycle. Analytical QbD defined as a science and risk-based paradigm for analytical method development, endeavoring for understanding the predefined objectives to control the critical method variables affecting the critical method attributes to achieve enhanced method performance, high robustness, ruggedness, and flexibility for continual improvement [ 5 , 6 ]. The result of analytical QbD is well known, fit for purpose, and robust method that reliably delivers the intended output over its lifecycle, similar to the process QbD [ 7 , 8 ]. For QbD, HPLC methods, robustness, and ruggedness should be tested earlier in the development stage of the method to ensure the efficiency of the method over the lifetime of the product [ 9 ]. Otherwise, it can take considerable time and energy to redevelop, revalidate, and retransfer analytical methods if a non-robust or non-rugged system is adapted. The major objective of AQbD has been to identify failure modes and establish robust method operable design region or design space within meaningful system suitability criteria and continuous life cycle management. Literature survey reveals QbD approaches for HPLC method were reported [ 10 , 11 , 12 , 13 ].
The current work intends to develop and optimize the HPLC method for ceftriaxone sodium in pharmaceutical dosage form by quality-by-design approach.
Ceftriaxone sodium was procured as gift sample Salvavidas Pharmaceutical Pvt. Ltd., Surat, Gujarat. All other reagents and chemicals used were of analytical grade, and solvents were used were of HPLC grade. The marketed formulations MONOCEF 250 mg by Aristo were used for assay.
Instruments and reference standards
The HPLC WATERS-2695 with Detector-UV VIS Dual Absorbance Detector WATERS-2487. C-18 column (150 mm × 4.6 mm × 5 μm particle size) was used at ambient temperature.
Chromatographic conditions
The Phenomenex C-18 column (250 mm × 4.6 mm having 5.0 μm particle size equilibrated with a mobile phase consisting of acetonitrile to water (70:30, v/v)) was used. The mobile phase pH 6.5 was adjusted with 0.01% triethylamine. The flow rate was kept at 1 ml/min, and column was set at ambient temperature. Eluents were supervised using a PDA detector at 270.0 nm. A satisfactory separation and peak symmetry for the drug were obtained with the above chromatographic condition. The HPLC method for ceftriaxone sodium was optimized for various parameters: mobile phase and pH as two variables at three different levels using central composite design.
Preparation of reference standard solution
The 1000 μg/ml standard stock solution was prepared by dissolving an accurately 25 mg of ceftriaxone sodium in 25 ml methanol. The stock solution was further diluted to a sub-stock 100 μg/ml. The 10 μg/ml solution was prepared by diluting 1 ml of sub-stock solution to 10 ml with methanol.
Selection of detection wavelength
Ten μg/ml ceftriaxone sodium was scanned in the range of 200–400 nm, and wavelength maxima 270 nm was selected as detection wavelength.
HPLC method development by QbD approach
HPLC method development by Analytical QbD was as follows.
Selection of quality target product profile
The QTPP plays an important role for identifying the variables that affect the QTPP parameters. The retention time, theoretical plates, and peak asymmetry were identified as QTPP for proposed HPLC method [ 14 , 15 ].
Determine critical quality attributes
The CQAs are the method parameters that are directly affect the QTPP. The mobile phase composition and pH of buffer were two critical method parameters required to be controlled to maintain the acceptable response range of QTPP [ 16 ].
Factorial design
After defining the QTPP and CQAs, the central composite experimental design was applied to optimization and selection of two key components: mobile phase and pH of HPLC method. The various interaction effects and quadratic effects of the mobile phase composition and pH of buffer solution on the retention time, theoretical plates, and peak asymmetry was studied using central composite statistical screening design.
A 2-factor, mobile phase composition and pH of buffer solution at 3 different levels, design was used with Design Expert® (Version 11.0, Stat-Ease Inc., and M M), the best suited response for second-order polynomial exploring quadratic response surfaces [ 15 ].
where A and B are independent variables coded for levels, Y is the measured response associated with each combination of factor level, β0 is an intercept, and β1 to β22 are regression coefficients derived from experimental runs of the observed experimental values of Y. Interaction and quadratic terms respectively represent the terms AB, A2, and B2.
Since multivariable interaction of variables and process parameter have been studied, the factors were selected based on preliminary analysis [ 17 ]. As independent variables, mobile phase composition and pH of buffer were chosen and shown in Table 1 . The dependent variables were retention time, peak area, and peak asymmetry as dependent variables for proposed independent variables [ 18 ].
Evaluation of experimental results and selection of final method conditions
Using the CCD approach, these method conditions were assessed. At the first step, the conditions for retention time, theoretical plates, and peak asymmetry were evaluated. For ceftriaxone sodium, this resulted in distinct chromatographic conditions. The proven acceptable ranges from robust regions where the deliberate variations in the method parameters do not affect the quality. This ensures that the method does not fail downstream during validation testing. If the modeling experiments do not have the desired response, the variable needs to be optimized at different levels until the responses were within the acceptable ranges [ 19 ]. The best suited chromatographic conditions shall be optimized using the Design Expert tools.
Risk assessment
The optimized final method is selected against the attributes of the method like that the developed method is efficient and will remain operational throughout the product’s lifetime. A risk-based approach based on the QbD principles set out in ICH Q8 and ICH Q9 guidelines was applied to the evaluation of method to study the robustness and ruggedness [ 20 ]. The parameters of the method or its performance under several circumstances, such as various laboratories, chemicals, analysts, instruments, reagents, and days, were evaluated for robustness and ruggedness studies [ 21 ].
Implement a control strategy
A control strategy should be implemented after the development of method. The analytical target profile was set for the development of the analytical control strategy. The analytical control strategy is the planned set of controls that was derived from the understanding of the various parameters, i.e., fitness for purpose, analytical procedure, and risk management. All these parameters ensure that both performance of the method and quality outputs are within the planned analytical target profile. Analytical control strategy was planned for sample preparation, measurement, and replicate control operations [ 22 ].
Continual improvement for managing analytical life cycle
The best way in the management of analysis lifecycle is doing a continual improvement that can be implemented by monitoring the quality consistency and periodic maintenance of HPLC instrument, computers, and updating of software and other related instrument and apparatus can be done within laboratory [ 23 ].
Analytical method validation
Method validation is a documented evidence which provides a high degree of assurance for a specific method that the process used to confirm the analytical process is suitable for its intended use. The developed HPLC method for estimation ceftriaxone sodium was validated as per ICH Q2 (R1) guidelines [ 24 ].
The linearity of ceftriaxone sodium was evaluated by analyzing 5 independent levels concentration range of 10–200 μg/ml. The calibration curve was constructed by plotting peak area on y axis versus concentration on x-axis. The regression line equation and correlation coefficient values were determined.
Repeatability calculated by the measurement of six samples 100 μg/ml ceftriaxone sodium. The intraday and interday precision were determined by analyzing three different concentrations of ceftriaxone sodium 100, 150, and 200 μg/ml concentrations at three times, on the same day at an interval of 2 h and for three different days. The acceptance limit for % RSD was less than 2.
The accuracy of the method was determined by calculating by recovery study from marketed formulation by at three levels 80%, 100%, and 120% of standard addition. The % recovery of ceftriaxone sodium was calculated. The acceptance limit for % recovery as per ICH guidelines was 98–102% of standard addition.
LOD and LOQ
The lowest drug concentration that can be accurately identified and separated from the background is referred to as a detection limit (LOD) and that can be quantified at the lowest concentration is referred to as LOQ, i.e., the quantification limit. The following equation was used to measure LOD and LOQ according to ICH guidelines.
where σ is the standard deviation of the y-intercept of the regression line, and SD is the slope of the calibration curve.
Robustness and ruggedness studies
The method’s robustness was calculated by subjecting the method to a minor change in the state of the method, such as pump flow rate and pH of mobile phase composition. The ruggedness studies were determined by changing the analyst as extraneous influencing factor. The acceptance limit for calculated %RSD of peak area was less than 2.
System suitability studies
The system suitability was evaluated by six replicate analyses of ceftriaxone sodium. The retention time, column efficiency, peak asymmetry, and theoretical plates were calculated for standard solutions.
Twenty tablets were weighed and powdered. Weigh an accurately about powder equivalent to 100 mg of ceftriaxone sodium, and transfer to 100 ml of volumetric flask. Add 25 ml of methanol, and perform sonication for 15 min until the powder dissolves. Then, make up the volume up to the mark with mobile phase. Filter the resulting solution with 0.42 μ Whatman filter paper. From the filtrate, dilute 0.5 ml to 10 ml to have a concentration of 100 μg/ml. The solution was analyzed by HPLC with same chromatographic condition as linearity. The mean of 3 different assay were used for calculation.
Initially, a mobile phase acetonitrile to water, 50:50 v/v, was tried; the peak was observed at far retention time. No single peak was observed with mobile phase acetonitrile to water, 80:20 v/v. The further mobile phase tried was acetonitrile to water, 40:60 v/v. The improvement of peak shape and symmetry was done by adjusting the buffer pH. The system suitability test parameters were satisfied with optimized chromatographic condition. The optimized mobile phase consisting of acetonitrile to water, 70:30 v/v, and pH 6.5 adjusted with 0.01% triethylamine. The central composite design was used further for the optimization of various parameters within the design space.
HPLC method development by QbD approach [ 25 ]
Quality target product profile
The QTPP selected were retention time, theoretical plates, and peak asymmetry for optimization of HPLC chromatographic condition.
Critical quality attributes
The mobile phase composition acetonitrile to water, 70:30, and pH of buffer solution adjusted with 0.01% triethylamine were identified.
Factorial design [ 21 ]
The CCD central composite design was selected for proposed HPLC method development. The optimization of various parameters is shown in Table 2 .
Design space
The response surface study type, central composite design, and quadric design model with 11 runs were used. The proposed CCD experimental design was applied, and the evaluation of mobile phase composition and pH of buffer was done against the three responses, retention time, theoretical plates, and peak asymmetry, and the result was summarized.
From Fig. 1 and equation retention time (for actual values) = 56.75 + 0.028 × A − 19.01 × B − 0.010 × AB + 0.000343 × A 2 + 1.70458 × B 2 , it was concluded that as β 1 positive coefficient (0.028) suggests that as the amount of acetonitrile in the mobile phase (A) increases and β 2 negative coefficient (− 19.01) suggests that as pH of buffer (B) decreases, the value of retention time was increased.
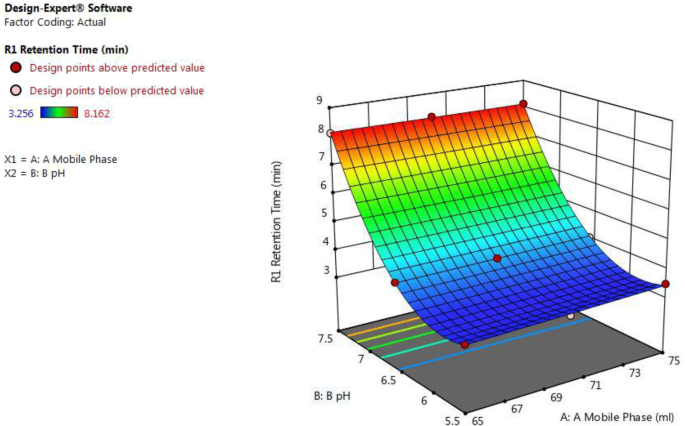
3D surface plot for effect of combination of factors on R1 retention time of ceftriaxone sodium by using central composite design
From Fig. 2 and equation theoretical plates (for actual values) = − 16774.36 − 4220.40 × A + 53225.20 × B + 56.05 × A × B + 26.83 × A 2 − 4380.60 × B 2 , it was concluded that as β 1 negative coefficient (− 4220.40) suggests that as the amount of acetonitrile in the mobile phase (A) decreases and β 2 positive coefficient (53225.20) suggests that as pH of buffer (B) increases, the value of theoretical plates was increased
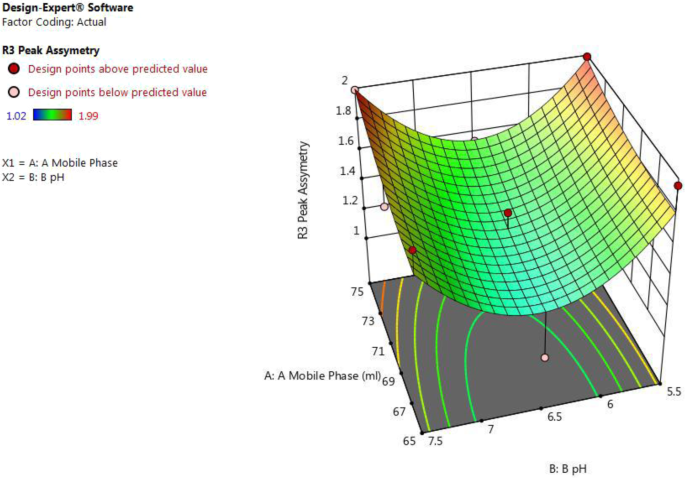
3D surface plot for effect of combination of factors on R2 theoretical plate ceftriaxone sodium by using central composite design
From Fig. 3 and equation peak asymmetry (for actual values) = 31.13 − 0.31 × A − 5.98 × B + 0.0055 × A × B + 0.0021 × A 2 + 0.429 × B 2 , it was concluded that as β 1 negative coefficient (− 0.31) suggests that as the amount of acetonitrile in the mobile phase (A) decreases and β 2 negative coefficient (− 5.98) suggests that as pH of buffer (B) decreases, the value of peak asymmetry was increased.
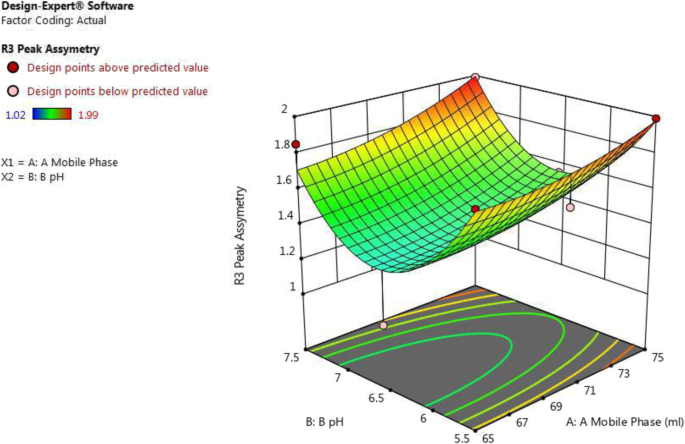
3D surface plot for effect of combination of factors on R3 peak asymmetry of ceftriaxone sodium by using central composite design
Optimized condition obtained
It was obtained by studying all responses in different experimental conditions using the Design expert 11.0 software, and optimized HPLC conditions and predicted responses are shown in Table 3 .
The observed value for responses was calculated by running the HPLC chromatogram for given set of mobile phase and pH of buffer and then compared with the predicted values to evaluate for % prediction error.
Method validation
System suitability.
The system suitability test was applied to a representative chromatogram to check the various parameters such as the retention time which was found to be 4.15 min, theoretical plates were 5263, peak asymmetry was 1.49, and % RSD of six replicate injections was 0.82. The 3D surface plot of desirability for obtaining optimized formulation is shown Fig. 4 .
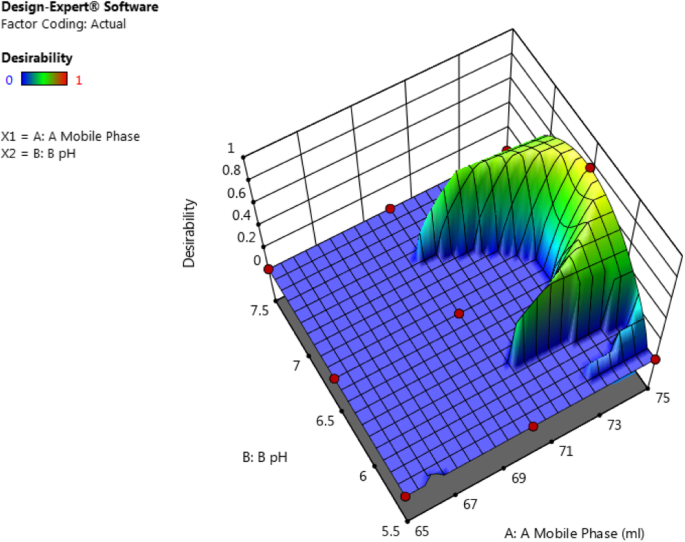
3D surface plot of desirability for obtaining optimized formulation
The constructed calibration curve for ceftriaxone sodium was linear over the concentration range of 10–200 μg/ml shown in Fig. 2 and Table 4 . Typically, the regression equation for the calibration curve was found to be y = 35441x + 60368 with a 0.991 correlation coefficient when graph was plotted with peak area verses concentration (Fig. 5 ).
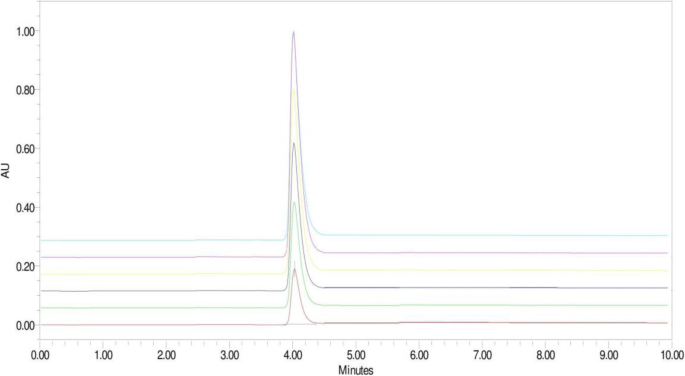
Linearity of 10–200 μg/ml ceftriaxone sodium
The % RSD for repeatability for ceftriaxone sodium based on six times the measurement of the same concentration (100 μg/ml) was found to be less than 0.082. Interday and intraday precisions were shown in Table 5 . The % RSD value less than 2 indicated that the developed method was found to be precise.
The accuracy was done by recovery study. Sample solutions were prepared by spiking at 3 levels, i.e., 80%, 100%, and 120%. The % recovery data obtained by the proposed HPLC method are shown in Table 6 . The % of recovery within 98–102% justify the developed method was accurate as per the ICH Q2 (R1) guidelines.
For robustness and ruggedness studies 100 μg/ml solution of ceftriaxone sodium was used. The robustness was studied by the slight but deliberate change in intrinsic method parameters like pH of mobile phase and flow rate. The ruggedness was studied by change in analyst as extraneous influencing factor. The % RSD for peak area were found to be less than 2 by change in pH of mobile phase, flow rate, and analyst.
The LOD and LOQ for ceftriaxone sodium based on standard deviation of slope and intercept were found to be 0.22 μg/ml and 0.67 μg/ml respectively.
The optimized chromatogram ceftriaxone sodium showed a resolved peak at retention time 4.15 min when performed assay from tablets. The % assay of drug content was found to be 99.73 ± 0.61 (n = 3) for label claim of ceftriaxone sodium. The assay result indicated the method’s ability to measure accurately and specifically in presence of excipients presents in tablet powder.
The analytical quality-by-design HPLC method for the estimation of ceftriaxone sodium in pharmaceutical formulation has been developed. The analytical target product profile were retention time, theoretical plates, and peak asymmetry for the analysis of ceftriaxone sodium by HPLC. The two variables namely the mobile phase composition and pH of buffer solution were identified as the critical quality attributes that affect the analytical target product profile. The central composite design was applied for two factors at three different levels with the use of the Design Expert Software Version 11.0. The risk assessment study identified the critical variables that have impact on analytical target profile [ 26 , 27 , 28 ]. In chromatographic separation, the variability in column selection, instrument configuration, and injection volume was kept controlled while variables such as pH of mobile phase, flow rate, and column temperature were assigned to robustness study.
The quality-by-design approach successfully developed the HPLC method for ceftriaxone sodium. The optimized RP-HPLC method for determination of ceftriaxone sodium used Phenomenex C18 column (250 × 4.6 mm, 5 μm particle size) and mobile phase consist of acetonitrile to water, 70:30 v/v, pH adjusted to 6.5 with 0.01% triethylamine buffer. The retention time for ceftriaxone sodium was found to be 4.15 min. The method was linear in the range of 10–200 μg/ml with 0.991 correlation coefficient. The % RSD for repeatability, intraday, and inter day precision was found to be less that 2% indicating the optimized method was precise. The LOD and LOQ were 0.22 μg/ml and 0.67 μg/ml, respectively. The % recovery of spiked samples was found to be 99.57 ± 1.47 to 100.79 ± 1.73 as per the acceptance criteria of the ICH guidelines. The method was developed as per the ICH guidelines.
A quality-by-design approach to HPLC method development has been described. The method goals are clarified based on the analytical target product profile. The experimental design describes the scouting of the key HPLC method components including mobile phase and pH. The analytical QbD concepts were extended to the HPLC method development for ceftriaxone sodium, and to determine the best performing system and the final design space, a multivariant study of several important process parameters such as the combination of 2 factors namely the mobile phase composition and pH of buffer at 3 different levels was performed. Their interrelationships were studied and optimized at different levels using central composite design. Here, a better understanding of the factors influencing chromatographic separation in the ability of the methods to meet their intended purposes is done. This approach offers a practical knowledge understanding that help for the development of a chromatographic optimization that can be used in the future. All the validated parameters were found within the acceptance criteria. The validated method was found to be linear, precise, accurate, specific, robust, and rugged for determination of ceftriaxone sodium. The QbD approach to method development has helped to better understand the method variables hence leading to less chance of failure during method validation and transfer. The automated QbD method development approach using the Design Expert software has provided a better performing more robust method in less time compared to manual method development. The statistical analysis of data indicates that the method is reproducible, selective, accurate, and robust. This method will be used further for routine analysis for quality control in pharmaceutical industry.
Availability of data and materials
All data and material are available upon request.
Abbreviations
- Quality by design
Active pharmaceutical ingredient
Central composite design
Critical quality attribute
High-performance liquid chromatography
Reverse phase high-performance liquid chromatography
Limit of quantitation
Limit of detection
Relative standard deviation
Sandipan R (2012) Quality by design: A holistic concept of building quality in pharmaceuticals. Int J Pharm Biomed Res 3:100–108
Google Scholar
The International Conference on Harmonisation ICH Technical Requirements for Registration of Pharmaceuticals for Human Use on Pharmaceutical Development Q8(R2) (2009) https://database.ich.org/sites/default/files/Q8%28R2%29%20Guideline.pdf
The International Conference on Harmonisation ICH Technical Requirements for Registration of Pharmaceuticals for Human Use on Quality Risk Management Q9 (2005) https://database.ich.org/sites/default/files/Q9%20Guideline.pdf
The International Conference on Harmonisation ICH Technical Requirements for Registration of Pharmaceuticals for Human Use on Pharmaceutical Quality System Q10 (2008) https://database.ich.org/sites/default/files/Q10%20Guideline.pdf
Borman P, Nethercote P, Chatfield M, Thompson D, Truman K (2007) The application of quality by design to analytical methods. Pharm Tech 31:142–152
Schweitzer M, Pohl M, Hanna BM, Nethercote P, Borman P, Hansen G, Smith K, Larew J (2010) Implications and opportunities of applying QbD principles to analytical measurements. Pharm Tech 34:52–59
CAS Google Scholar
Galen WE (2004) Analytical Instrumentation Handbook 2nd edn. Marcel Dekker Inc, New York
Snyder LR, Kirkland JJ, Glajch LJ (1997) Practical HPLC method development; 2nd edn. John Wiley & Sons Inc, New York. https://doi.org/10.1002/9781118592014
Book Google Scholar
Bhatt D, Rane S (2011) QbD approach to analytical RP-HPLC method development and its validation. Int J Pharm Pharm Sci 3:79–187
Rajkotwala A, Shaikh S, Dedania Z, Dedania R, Vijyendraswamy S (2016) QbD approach to analytical method development and validation of piracetam by HPLC. World J Pharmacy Pharmaceutical Sci 5:1771–1784
Singh P, Maurya J, Dedania Z, Dedania R (2017) QbD Approach for stability indicating HPLC method for determination of artemether and lumefantrine in combined dosage form. Int J Drug Reg Affairs 5:44–59
Article CAS Google Scholar
Prajapati R, Dedania Z, Jain V, Sutariya V, Dedania R, Chisti Z (2019) QbD approach to HPLC method development and validation for estimation of fluoxetine hydrochloride and olanzapine in pharmaceutical dosage form. J Emerging Tech Innovative Res 6:179–195
Dhand V, Dedania Z, Dedania R, Nakarani K (2020) QbD approach to method development and validation of orciprenaline sulphate by HPLC. J Global Trends Pharm Sci 11:8634–8640
Krull I, Swartz M, Turpin J, Lukulay P, Verseput R (2008) A quality-by-design methodology for rapid LC method development, part I. Liq Chroma Gas Chroma N Am 26:1190–1197
Myers R, Montgomery D, Anderson-Cook C (2016) Response surface methodology: process and product optimization using designed experiments. 4th edn. New York: Wiley
Yubing T (2011) Quality by design approaches to analytical methods- FDA perspective. https://www.fda.gov/files/about%20fda/published/Quality-by-Design-Approaches-to-Analytical-Methods%2D%2D%2D%2DFDA-Perspective%2D%2DYubing-Tang%2D%2DPh.D.%2D%2DOctober%2D%2D2011%2D%2DAAPS-Annual-Meeting.pdf . Accessed 15 Dec 2018.
Krull I, Swartz M, Turpin J, Lukulay P, Verseput R (2009) A quality-by-design methodology for rapid LC method development part II. Liq Chroma Gas Chroma N Am 27:48–69
Reid G, Morgado J, Barnett K, Harrington B, Wang J, Harwood J, Fortin D (2013) Analytical QbD in pharmaceutical development. https://www.waters.com/nextgen/in/en/library/application-notes/2019/analytical-quality-by-design-based-method-development-for-the-analysis-of-formoterol-budesonide-and-related-compounds-using-uhplc-ms.html . Accessed 10 June 2018.
Molnar RH, Monks K (2010) Aspects of the “Design Space” in high pressure liquid chromatography method development. J Chromatogra A 1217(19):3193–3200. https://doi.org/10.1016/j.chroma.2010.02.001
Monks K, Molnar I, Rieger H, Bogati B, Szabo E (2012) Quality by design: multidimensional exploration of the design space in high performance liquid chromatography method development for better robustness before validation. J Chromatogra A 1232:218–230. https://doi.org/10.1016/j.chroma.2011.12.041
Ramalingam P, Kalva B, Reddy Y (2015) Analytical quality by design: a tool for regulatory flexibility and robust analytics. Int J Ana Chem. https://doi.org/10.1155/2015/868727
The International Conference on Harmonisation ICH Technical Requirements for Registration of Pharmaceuticals for Human Use on Development and Manufacture of Drug Substances (Chemical Entities and Biotechnological/Biological Entities) Q11 (2012) https://database.ich.org/sites/default/files/Q11%20Guideline.pdf
Orlandini S, Pinzauti S, Furlanetto S (2013) Application of quality by design to the development of analytical separation methods. Ana Bioana Chem 405(2-3):443–450. https://doi.org/10.1007/s00216-012-6302-2
The International Conference on Harmonisation ICH Technical Requirements for Registration of Pharmaceuticals for Human Use on Validation of Analytical Procedures: Text and Methodology Q2(R1) (2005) https://database.ich.org/sites/default/files/Q2%28R1%29%20Guideline.pdf
Reid G, Cheng G, Fortin D (2013) Reversed-phase liquid chromatographic method development in an analytical quality by design framework. J Liq Chrom Related Tech 36(18):2612–2638. https://doi.org/10.1080/10826076.2013.765457
Elder P, Borman P (2013) Improving analytical method reliability across the entire product lifecycle using QbD approaches. Pharmaceu Outsourcing, 14:14–19. http://www.pharmoutsourcing.com/Featured-Articles/142484-Improving-Analytical-Method-Reliability-Across-the-Entire-Product-Lifecycle-Using-QbD-Approaches/ . Accessed 2019.
Smith J, Jones M Jr, Houghton L (1999) Future of health insurance. N Engl J Med 965:325–329
Schweitzer M, Pohl M, Hanna-Brown M (2010) Implications and opportunities of applying QbD principles to analytical measurements. Pharmaceu Tech 34:52–59
Download references
Acknowledgements
All authors are very thankful to the Bhagwan Mahavir College of Pharmacy, Surat, for providing necessary facilities to carry out the research work.
This research did not receive any specific grant from funding agencies in the public, commercial, or not-for-profit sectors.
Author information
Authors and affiliations.
Department of Quality Assurance, Bhagwan Mahavir College of Pharmacy, Vesu, Surat, Gujarat, India
Krunal Y. Patel, Zarna R. Dedania & Ronak R. Dedania
Department of Chemistry, The University of Alabama in Huntsville, 301 Sparkman Dr, Huntsville, AL-35899, USA
Unnati Patel
You can also search for this author in PubMed Google Scholar
Contributions
All authors associated with this research work declared that there is no conflict of interest for publication of work. All authors have read and approved the manuscript. The contribution of each author is mentioned below. KP: He is a M Pharm (Quality Assurance) Research Student and the above work has been carried out by him as dissertation work. ZD: She is Research Guide and HOD, Department of Quality Assurance and under her noble guidance the QbD approach for HPLC method has been developed and validated as per ICH guidelines. She is also giving training for ease of operation sophisticated instrument and involved in interpretation of data. RD: He is a co-guide and under his noble guidance student can understand the Design Expert Software and interpretation of statistical data. UP: She is a graduate teaching assistant at University of Alabama at Huntsville, USA and she has contributed for preparing the manuscript.
Corresponding author
Correspondence to Zarna R. Dedania .
Ethics declarations
Ethics approval and consent to participate.
Not applicable
Consent for publication
Competing interests.
No competing interests to declare.
Additional information
Publisher’s note.
Springer Nature remains neutral with regard to jurisdictional claims in published maps and institutional affiliations.
Rights and permissions
Open Access This article is licensed under a Creative Commons Attribution 4.0 International License, which permits use, sharing, adaptation, distribution and reproduction in any medium or format, as long as you give appropriate credit to the original author(s) and the source, provide a link to the Creative Commons licence, and indicate if changes were made. The images or other third party material in this article are included in the article's Creative Commons licence, unless indicated otherwise in a credit line to the material. If material is not included in the article's Creative Commons licence and your intended use is not permitted by statutory regulation or exceeds the permitted use, you will need to obtain permission directly from the copyright holder. To view a copy of this licence, visit http://creativecommons.org/licenses/by/4.0/ .
Reprints and permissions
About this article
Cite this article.
Patel, K.Y., Dedania, Z.R., Dedania, R.R. et al. QbD approach to HPLC method development and validation of ceftriaxone sodium. Futur J Pharm Sci 7 , 141 (2021). https://doi.org/10.1186/s43094-021-00286-4
Download citation
Received : 04 December 2020
Accepted : 18 June 2021
Published : 13 July 2021
DOI : https://doi.org/10.1186/s43094-021-00286-4
Share this article
Anyone you share the following link with will be able to read this content:
Sorry, a shareable link is not currently available for this article.
Provided by the Springer Nature SharedIt content-sharing initiative
- Ceftriaxone sodium
- Design approach
Have a language expert improve your writing
Run a free plagiarism check in 10 minutes, generate accurate citations for free.
- Knowledge Base
Methodology
- How to Do Thematic Analysis | Step-by-Step Guide & Examples
How to Do Thematic Analysis | Step-by-Step Guide & Examples
Published on September 6, 2019 by Jack Caulfield . Revised on June 22, 2023.
Thematic analysis is a method of analyzing qualitative data . It is usually applied to a set of texts, such as an interview or transcripts . The researcher closely examines the data to identify common themes – topics, ideas and patterns of meaning that come up repeatedly.
There are various approaches to conducting thematic analysis, but the most common form follows a six-step process: familiarization, coding, generating themes, reviewing themes, defining and naming themes, and writing up. Following this process can also help you avoid confirmation bias when formulating your analysis.
This process was originally developed for psychology research by Virginia Braun and Victoria Clarke . However, thematic analysis is a flexible method that can be adapted to many different kinds of research.
Table of contents
When to use thematic analysis, different approaches to thematic analysis, step 1: familiarization, step 2: coding, step 3: generating themes, step 4: reviewing themes, step 5: defining and naming themes, step 6: writing up, other interesting articles.
Thematic analysis is a good approach to research where you’re trying to find out something about people’s views, opinions, knowledge, experiences or values from a set of qualitative data – for example, interview transcripts , social media profiles, or survey responses .
Some types of research questions you might use thematic analysis to answer:
- How do patients perceive doctors in a hospital setting?
- What are young women’s experiences on dating sites?
- What are non-experts’ ideas and opinions about climate change?
- How is gender constructed in high school history teaching?
To answer any of these questions, you would collect data from a group of relevant participants and then analyze it. Thematic analysis allows you a lot of flexibility in interpreting the data, and allows you to approach large data sets more easily by sorting them into broad themes.
However, it also involves the risk of missing nuances in the data. Thematic analysis is often quite subjective and relies on the researcher’s judgement, so you have to reflect carefully on your own choices and interpretations.
Pay close attention to the data to ensure that you’re not picking up on things that are not there – or obscuring things that are.
Here's why students love Scribbr's proofreading services
Discover proofreading & editing
Once you’ve decided to use thematic analysis, there are different approaches to consider.
There’s the distinction between inductive and deductive approaches:
- An inductive approach involves allowing the data to determine your themes.
- A deductive approach involves coming to the data with some preconceived themes you expect to find reflected there, based on theory or existing knowledge.
Ask yourself: Does my theoretical framework give me a strong idea of what kind of themes I expect to find in the data (deductive), or am I planning to develop my own framework based on what I find (inductive)?
There’s also the distinction between a semantic and a latent approach:
- A semantic approach involves analyzing the explicit content of the data.
- A latent approach involves reading into the subtext and assumptions underlying the data.
Ask yourself: Am I interested in people’s stated opinions (semantic) or in what their statements reveal about their assumptions and social context (latent)?
After you’ve decided thematic analysis is the right method for analyzing your data, and you’ve thought about the approach you’re going to take, you can follow the six steps developed by Braun and Clarke .
The first step is to get to know our data. It’s important to get a thorough overview of all the data we collected before we start analyzing individual items.
This might involve transcribing audio , reading through the text and taking initial notes, and generally looking through the data to get familiar with it.
Next up, we need to code the data. Coding means highlighting sections of our text – usually phrases or sentences – and coming up with shorthand labels or “codes” to describe their content.
Let’s take a short example text. Say we’re researching perceptions of climate change among conservative voters aged 50 and up, and we have collected data through a series of interviews. An extract from one interview looks like this:
In this extract, we’ve highlighted various phrases in different colors corresponding to different codes. Each code describes the idea or feeling expressed in that part of the text.
At this stage, we want to be thorough: we go through the transcript of every interview and highlight everything that jumps out as relevant or potentially interesting. As well as highlighting all the phrases and sentences that match these codes, we can keep adding new codes as we go through the text.
After we’ve been through the text, we collate together all the data into groups identified by code. These codes allow us to gain a a condensed overview of the main points and common meanings that recur throughout the data.
Next, we look over the codes we’ve created, identify patterns among them, and start coming up with themes.
Themes are generally broader than codes. Most of the time, you’ll combine several codes into a single theme. In our example, we might start combining codes into themes like this:
At this stage, we might decide that some of our codes are too vague or not relevant enough (for example, because they don’t appear very often in the data), so they can be discarded.
Other codes might become themes in their own right. In our example, we decided that the code “uncertainty” made sense as a theme, with some other codes incorporated into it.
Again, what we decide will vary according to what we’re trying to find out. We want to create potential themes that tell us something helpful about the data for our purposes.
Now we have to make sure that our themes are useful and accurate representations of the data. Here, we return to the data set and compare our themes against it. Are we missing anything? Are these themes really present in the data? What can we change to make our themes work better?
If we encounter problems with our themes, we might split them up, combine them, discard them or create new ones: whatever makes them more useful and accurate.
For example, we might decide upon looking through the data that “changing terminology” fits better under the “uncertainty” theme than under “distrust of experts,” since the data labelled with this code involves confusion, not necessarily distrust.
Now that you have a final list of themes, it’s time to name and define each of them.
Defining themes involves formulating exactly what we mean by each theme and figuring out how it helps us understand the data.
Naming themes involves coming up with a succinct and easily understandable name for each theme.
For example, we might look at “distrust of experts” and determine exactly who we mean by “experts” in this theme. We might decide that a better name for the theme is “distrust of authority” or “conspiracy thinking”.
Finally, we’ll write up our analysis of the data. Like all academic texts, writing up a thematic analysis requires an introduction to establish our research question, aims and approach.
We should also include a methodology section, describing how we collected the data (e.g. through semi-structured interviews or open-ended survey questions ) and explaining how we conducted the thematic analysis itself.
The results or findings section usually addresses each theme in turn. We describe how often the themes come up and what they mean, including examples from the data as evidence. Finally, our conclusion explains the main takeaways and shows how the analysis has answered our research question.
In our example, we might argue that conspiracy thinking about climate change is widespread among older conservative voters, point out the uncertainty with which many voters view the issue, and discuss the role of misinformation in respondents’ perceptions.
If you want to know more about statistics , methodology , or research bias , make sure to check out some of our other articles with explanations and examples.
- Normal distribution
- Measures of central tendency
- Chi square tests
- Confidence interval
- Quartiles & Quantiles
- Cluster sampling
- Stratified sampling
- Discourse analysis
- Cohort study
- Peer review
- Ethnography
Research bias
- Implicit bias
- Cognitive bias
- Conformity bias
- Hawthorne effect
- Availability heuristic
- Attrition bias
- Social desirability bias
Cite this Scribbr article
If you want to cite this source, you can copy and paste the citation or click the “Cite this Scribbr article” button to automatically add the citation to our free Citation Generator.
Caulfield, J. (2023, June 22). How to Do Thematic Analysis | Step-by-Step Guide & Examples. Scribbr. Retrieved April 6, 2024, from https://www.scribbr.com/methodology/thematic-analysis/
Is this article helpful?
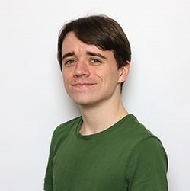
Jack Caulfield
Other students also liked, what is qualitative research | methods & examples, inductive vs. deductive research approach | steps & examples, critical discourse analysis | definition, guide & examples, unlimited academic ai-proofreading.
✔ Document error-free in 5minutes ✔ Unlimited document corrections ✔ Specialized in correcting academic texts

An official website of the United States government
The .gov means it’s official. Federal government websites often end in .gov or .mil. Before sharing sensitive information, make sure you’re on a federal government site.
The site is secure. The https:// ensures that you are connecting to the official website and that any information you provide is encrypted and transmitted securely.
- Publications
- Account settings
Preview improvements coming to the PMC website in October 2024. Learn More or Try it out now .
- Advanced Search
- Journal List
- Turk J Pharm Sci
- v.18(3); 2021 Jun

Development and Validation of an HPLC Method Using an Experimental Design for Analysis of Amlodipine Besylate and Enalapril Maleate in a Fixed-dose Combination
Diren sarisaltik yasin.
1 Dicle University Faculty of Pharmacy, Department of Pharmaceutical Technology, Diyarbakır, Turkey
Alev ARSLANTÜRK BİNGÜL
2 Dicle University Faculty of Science, Department of Chemistry, Diyarbakır, Turkey
Alptuğ KARAKÜÇÜK
3 Gazi University Faculty of Pharmacy, Department of Pharmaceutical Technology, Ankara, Turkey
4 Ankara Medipol University Faculty of Pharmacy, Department of Pharmaceutical Technology, Ankara, Turkey
Zeynep Şafak TEKSİN
Objectives:.
The aim of this study was to develop and optimize a simple, cost-effective, and robust high-performance liquid chromatography (HPLC) method by taking an experimental design approach to the assay and dissolution analysis of amlodipine besylate and enalapril maleate from a fixed-dose combination tablet.
Materials and Methods:
The chromatographic analysis was performed on a C18 column (4.6x250 mm id., particle size of 5 μm). The injection volume was 5 μL, and the detection wavelength was 215 nm. A Box-Behnken design was used to test the robustness of the method. The flow rate (1, 1.2, and 1.4 mL/min), column temperature (25°C, 30°C, and 35°C), methanol ratio of the mobile phase (5, 10, and 15%), and pH of the mobile phase (2.8, 3, and 3.2) were selected as independent variables. The method was validated according to International Conference on Harmonization guidelines. Dissolution of the tablets was performed by using USP apparatus 2 and analyzed using the optimized HPLC method. Multivariate linear regression analysis and ANOVA were used in the statistical evaluation.
Linear models were fitted for all variables. The flow rate was the most significant factor affecting the APIs’ concentrations. The optimized method included the following parameters: Column temperature of 25°C, 10% methanol as the mobile phase, pH of 2.95, and flow rate of 1.205 mL/min. Retention times were 3.8 min and 7.9 min for enalapril and amlodipine, respectively. The method was found to be linear in the range of 0.8-24 μg/mL (R 2 >0.999) and 1.6-48 μg/mL (R 2 >0.999) for amlodipine and enalapril, respectively. Both APIs were dissolved more than 85% within 10 min.
Conclusion:
The experimental design was proved as a useful tool for the determination and separation of enalapril maleate and amlodipine besylate in dosage forms. The optimized method can be used for in vitro performance and quality control tests of fixed-dose tablet combinations containing enalapril maleate and amlodipine besylate.
INTRODUCTION
At the early stages of the treatment of hypertension, it can be useful to choose monotherapy to observe the effect and the side effects of the drug. However, monotherapy can be insufficient to reach the target blood pressure in a majority of patients. 1 , 2 , 3 A greater therapeutic benefit can be achieved with two or even more antihypertensive drugs. 4 Therefore, fixed-dose combinations (FDCs) are frequently used in cardiovascular diseases such as hypertension. In order to develop an FDC product including two drugs, certain conditions must be met. For instance, a synergistic effect can be observed using two drugs together, or a side effect related to a drug may be eliminated using the other drug concurrently. 5 In the treatment of hypertension, there is a synergistic effect between calcium channel blockers (CCBs) and angiotensin-converting enzyme inhibitors (ACEIs). In addition, ACEIs such as enalapril prevent peripheral edema caused by CCBs such as amlodipine. 6
Amlodipine is a long-acting CCB that inhibits the transmembrane influx of calcium ions into vascular smooth muscle and cardiac muscle. It is indicated for the treatment of hypertension and coronary artery disease when used alone or in combination with another antihypertensive agent. 7 Amlodipine is given orally as besylate in general, but doses are calculated in terms of amlodipine base. A dose of 6.94 mg of amlodipine besylate is equivalent to 5 mg of amlodipine base. The recommended dose of amlodipine is 5-10 mg once daily. 8 Since amlodipine is a weak base, it exhibits high solubility in physiological pH values. Although the bioavailability of amlodipine is approximately 60%-65%, it is defined as a highly permeable drug because of the 90%-95% excretion rate as an inactive metabolite in the urine Shohin et al. 9 Amlodipine is a class 1 drug according to the Biopharmaceutics Classification System (BCS). 9 , 10 , 11
Enalapril is the ethyl ester of enalaprilat, an ACEI indicated for the treatment of hypertension and heart failure. Enalapril is available as maleate salt in the drug market. Enalapril maleate is a white crystalline powder sparingly soluble in water. Although the solubility is 25 mg/mL at pH 3.5, it increases to 200 mg/mL at pH 7.0. It is defined as BCS class 3 with high solubility but low permeability properties. 12
There are high-performance liquid chromatography (HPLC) methods recommended in United States Pharmacopeia (USP42) for analysis of amlodipine besylate 13 and enalapril maleate, 14 separately and a few liquid chromatography methods are available in the literature for analyses of amlodipine, 15 and enalapril, 16 , 17 individually or in combination with other drugs. 18 , 19 , 20 , 21 , 22 , 23 However, these methods are not suitable for the separation of amlodipine and enalapril in the same dosage unit. Nevertheless, there are three published articles for HPLC analysis of amlodipine besylate and enalapril maleate together in dosage forms. 24 , 25 , 26 However these methods contain a high ratio of organic solvents in the mobile phase, which is environmentally inappropriate according to the green chemistry approach. An important principle of green chemistry is to reduce toxic organic solvents and to consume safer chemicals. 27 , 28 Relating to the green analytical chemistry approach, Korany et al. 27 recommended reducing the acetonitrile amount in the methods and using multiparameter methods such as design of experiment (DOE) instead of the one factor at a time (OFAT) approach. 28 In the method developed by Chaudhari 24 , the mobile phase contains 50% acetonitrile and 40% methanol and a higher injection volume (20 µL), which increases the consumption of mobile phase and the linearity range was comparatively narrow (0.5-6 µg/mL and 0.5-8 µg/mL for enalapril and amlodipine, respectively). In another method, the mobile phase includes 60% acetonitrile, the injection volume was 20 µL, and the linearity range was not suitable for lower concentrations (20-100 µg/mL), which might be essential for the initial points of the dissolution tests. 25 In the method developed by Masih et al. 26 , 50% 1N HCl and 50% methanol were included in the mobile phase, and the injection volume was 10 µL. Additionally, none of the studies include the application of DOE in robustness testing in validation for amlodipine besylate and enalapril maleate. Furthermore, there is no dissolution analysis of enalapril and amlodipine in the combined dosage form in the literature.
DOE is a well-defined mathematical methodology to demonstrate how to obtain maximum reliable and valuable scientific information by performing minimal experiments. 29 In this technique, the effects of multiple variations on one or more responses can be investigated at the same time, instead of changing OFAT. Although conventional developmental approaches are mainly empirical and are often conducted using the changing OFAT method, DOE provides the facility of performing systematic and multivariate experiments in order to entirely understand the process and to assess the statistical significance of the variables. 30 , 31 By creating experimental matrix, DOE allows faster visualization and determination of more factors at a time. 32 Besides, in OFAT approach factors are evaluated independently, so it is assumed that the factors do not influence each other. However, the potential interactions between the factors can be identified using the appropriate DOE model. 33 , 34 In the pharmaceutical field, DOE helps to understand the effects of the critical formulation and process variables on the final product. 35 , 36 DOE can be used for factor screening and characterization of a new system or optimization of a characterized system. Factors are independent variables that might affect the results of critical responses. For instance, in an analytical method development process, the flow rate can be an independent factor that has potential effects on the peak area of the analyte. In a screening design it is aimed to investigate numerous factors that might affect the response and to discover the factor which has the most significant influence on the responses. 37 On the other hand, in an optimization process, the main objective of which is to define the optimal conditions and settings for the factors. 38 In case more than one factor must be examined, the multivariate optimization designs can be reasonable in order to evaluate different factors at the same time and to determine if interactions exist between factors. 37 , 38
In analytical chemistry, DOE can be used for chromatographic analytical method development to optimize the sampling preparational, column, detector, instrumental, or environmental factors. 31 , 39 Similarly, analytical method validation parameters such as accuracy, linearity, precision, or robustness can be performed by experimental design approaches. 29 , 40 , 41 , 42 , 43 , 44 , 45 , 46 Using DOE in validation studies is recommended in the International Conference on Harmonization (ICH) guidelines. 27 , 47 There have been many studies in which DOE was applied to robustness. 31 , 32 , 43 , 48 , 49 Experimental design targeting robustness is a good approach to fully understand the factors with effects on the responses and provide maximum information about the method in a short time. Robustness should be built into methods in the pre-validation stages; otherwise, a robustness test performed too late has a risk of obtaining inappropriate results which can cause redevelopment and revalidation. 50 Therefore, a robustness test in the earlier stage of the method development process leads to a saving of effort, time, and money. Experimental data obtained from early stages can aid in performance method evaluation and can be used to guide further method development. 51
Optimization can be performed by using response surface methodology (RSM) designs such as the Box-Behnken design (BBD) and the central composite design (CCD). 49 , 52 The BBD is a second-order design that allows investigation of numerous factors with three levels. It is preferable to the CCD because it prevents an unrealistic extreme scenario by creating the experimental matrix without containing extreme points in the same experiment. 33 , 52 BBD is used in analytical method optimization in many studies. 6 , 48 , 53 , 54 , 55 , 56 , 57 , 58 , 59 , 60 , 61 , 62 , 63 , 64 , 65
In this study, a simple, rapid and robust HPLC method with photodiode array (PDA) detection at 215 nm was developed for the determination and separation of amlodipine besylate and enalapril maleate in FDC tablets. This method, which is available for assay and dissolution studies, was fast, environmentally friendly, and more cost-effective than the earlier published methods. 24 , 25 , 26 In this study, DOE was adapted to the robustness parameter of the analytical method for determining amlodipine and enalapril together. DOE principles were used in the method development of amlodipine and enalapril for the first time. The validation of the method was performed according to the ICH Q2 (R1) guideline. 47 The BBD was used for the optimization of the method. The optimized HPLC method was applied to dissolution and assay analysis of an in-house FDC tablet including amlodipine and enalapril.
MATERIALS AND METHODS
Materials and reagents.
HPLC-grade methanol, o-phosphoric acid and hydrochloric acid 37% were obtained from Merck, Germany. Amlodipine besylate (Hetero Drugs, India) and enalapril maleate (Zheijiang Huahai, China) were kindly gifted by Nobel Pharma, Turkey.
The FDC tablet contains 6.94 mg of amlodipine besylate and 10 mg of enalapril maleate as APIs.
The HPLC system was a Shimadzu chromatographic system (Japan) with LC-20AD pump, SPD-M20A PDA detector at a wavelength of 215 nm, a reversed phase C18 column (4.6x250 mm id., particle size of 5 µm) from Waters ® (USA). The HPLC system was controlled by LC Solution Software. Design Expert ® Version 9 (Stat-Ease Inc, USA) was used for the experimental design and statistical analysis of data. A pH meter (PASS1 P11-BNC-Bante, England) was used to control the aqueous buffer. Dissolution test was performed with Pharmatest ® Dissolution System (Germany).
Chromatographic conditions
The mobile phase was a mixture of methanol and water (pH adjusted to 3.0 with o-phosphoric acid) in the proportion of 10:90 (v:v). The injection volume of the samples was 5 µL. The flow rate was 1.2 mL/min. The detector wavelength was 215 nm and the column temperature was 30°C.
Preparation of standard solutions
The standard solution was prepared according to the following process: 6.94 mg of amlodipine besylate (equivalent to 5 mg amlodipine base) and 10 mg of enalapril maleate were weighed and transferred to a 50 mL volumetric flask and diluted to the appropriate volume with 0.1N HCl. This solution included 0.1 mg/mL of amlodipine base and 0.2 mg/mL of enalapril maleate. The calculations were performed considering amlodipine base and enalapril as maleate salts because of the dose proportionality in market products .
Calibration procedure
Calibration series were prepared in volumetric flasks by the appropriate dilution of standard solution with 0.1N HCl. The calibration curve was plotted with eight concentrations in the range of 0.8-24 µg/mL for amlodipine and 1.6-48 µg/mL for enalapril (as maleate). The experiments were performed in three replicates for each level. The linearity of the calibration curve was evaluated by the linear regression statistics of concentrations against peak area.
Statistical analysis
Experimental design.
Experimental plan, data analysis and optimization process were executed in Design Expert ® Version 9 by using the BBD. The BBD is a three-level and multi-factor design which is a combination of 2K factorial and balanced incomplete block designs. In this study, four factors with three levels for each were determined as given in Table 1 .

The significant factors in the model were determined by multivariate linear regression analysis and ANOVA F-test and its lack of fit with a confidence interval of 95% for each response. Significant factors were determined by the probability level that the p value is less than 0.05 and one-factor graphs.
Assay in FDC tablets
The FDC tablet containing amlodipine besylate and enalapril maleate was prepared by using direct compression method. For assay of the tablets, 10 tablets for each product were selected at random and weighed. Then these tablets were powdered, and a quantity of the powder (equivalent to 5 mg of amlodipine and 10 mg of enalapril maleate) was accurately weighed and transferred to a 50 mL volumetric flask. A 30 mL volume of diluent solution (0.1N HCl) was added and mixed for 15 min in magnetic stirrer. Then, it was diluted with the same solution to the volume and mixed in an ultrasonic bath for 10 min. A 4 mL volume of this solution was transferred to a 25 mL volumetric flask and diluted to the volume using the same solvent and was held in an ultrasonic bath for 5 min. The samples were filtered through a syringe tip filter of 0.45-µm pore size and then analyzed using HPLC.
Dissolution studies
Dissolution studies were performed using USP apparatus II (paddle method) in 0.1N HCl (pH 1.2). The dissolution volume was 900 mL, and the temperature was 37°C±0.5°C. The paddle rotational speed was 75 rpm. Samples (2 mL) were withdrawn at 10, 20, 30, 45, and 60 min, and the same amount of fresh media was replaced. The samples were filtered through 0.45-µm membrane filters to vials and analyzed by the optimized HPLC method. The dissolution profiles were evaluated as the cumulative drug dissolved (%) over time. All experiments were performed in n=3 and the cumulative amounts were evaluated as the mean ± standard deviation (SD).
RESULTS AND DISCUSSION
The chromatograms of diluent (blank) and those obtained from the standard solutions of amlodipine and enalapril are given in Figure 1 , ,2 2 respectively. The initial method provided good separation in a short time of 3.8 min for enalapril and 7.9 min for amlodipine. This level of separation is acceptable in a conventional method development process. A robustness study with DOE was also performed.

Chromatogram of the placebo (blank medium) for specificity testing
PDA: Photodiode array

Chromatogram of enalapril (8 μg/mL, as maleate) and amlodipine (4 μg/mL) in the initial method
Robustness with DOE principles
According to the ICH Q2 (R1), in a robust method, small variations in certain method parameters do not affect the reliability and results of the method. 47 These small variations are important for the pharmaceutical industry in terms of the transfer of the analytical method from research and development to the quality control laboratory or from one company to another. In other words, it is the indication of the strength of the method. 51 In order to assess the concurrent influences of the changes in factors on the defined responses, a multivariate analysis by DOE is recommended in robustness studies. 43 DOE is used in analytical method development for two main purposes: To determine the most significant factor influencing the response of the study and to discover the optimized value of the factors for best results for the response. 37
The DOE plan in a robustness test includes the following stages: 31
Selection of factors and their levels
Robustness studies are an excellent opportunity to apply statistical experimental design to provide data-based control of the method. 51 Since there are many factors that might affect the method, it is vital to choose the right factors. In robustness studies of liquid chromatography, the most frequently preferred factors are the pH of the mobile phase, analysis time, flow rate, column type, temperature, composition of the mobile phase, detection wavelength, chosen filters, or the variations in sample preparation such as dilution, shaking time, or heating temperature. 39 , 51 It should be noted that there are no absolute truths in selecting factors in a DOE process; the chosen factors should comply with the purpose. According to ICH Q2 (R1), the following variations were recommended for the robustness test of HPLC methods: 1) pH of the mobile phase, 2) composition of the mobile phase, 3) column type, 4) temperature, and 5) flow rate. Except for the column type, all recommended factors (mobile phase ratio, pH, flow rate, and column temperature) were investigated in this study. The chosen factors and their pre-defined levels have the potential to affect the method depending on the analyst, laboratory or equipment, and environmental conditions. 47
After selecting the factors, it is necessary to define their levels. In a two-level model such as Plackett-Burman Design (PBD) or two-level factorial designs, a maximum and a minimum limit are required for the factor values. In three-level designs, additional middle values, which generally represent the target or the expected value, are added to the design. Defining the levels is a critical step in experimental design. Particularly in two-level designs in which inappropriate levels were used, inaccurate and low-quality results can be obtained. 33 In order to avoid this problem, a three-level BBD design is preferred. The levels of the factors are usually defined symmetrically around the nominal level, which is the middle level in a three-level design. The interval chosen between the levels is generally decided according to the operator’s personal experiences or anticipated changes from one laboratory to another. For example, if the developed method will be transferred to another laboratory, the pH can be measured using a pH meter with a small deviation, so pH should be considered as critical. The pH of a solution varies with a deviation of 0.02 with a confidence limit of 95%. 50 Therefore, this limit is acceptable for the pH in a robustness test. The interval of pH was ±0.02 in this study. The levels of column temperature were decided ±5°C as recommended in the article by Vander-Heyden et al. 50 , which was aimed to guide a robustness parameter in method development. The levels of other factors, selected as 5% for mobile phase composition and 0.2 mL/min for flow rate, were in agreement with previous similar studies. 32 , 43 , 65
Defining responses to be investigated
In the HPLC studies where robustness was investigated by DOE, various responses such as peak area, peak height, determined concentration, retention time, tailing factor, theoretical plate number, and resolution were used. The most important selection criterion for a response to use in factor evaluation is ease of measurement. 39 Additionally, using a large number of responses can lead to confusion when interpreting the results. Therefore, API concentrations calculated from the peak areas were selected as responses in this study.
Choosing an experimental design
A suitable experimental design should be selected based on the aim of the study. In case a large number of factors might affect the method, the aim can be to discard some factors that have no significant effect on the response. For this purpose, a screening design such as PBD can be used. On the other hand, if the main objective is to investigate the effects of the relatively lower number of factors deeply, or optimize the most effective factors, optimization designs should be preferred. 31 Generally, optimization is carried out following determination of the most significant factors by screening design. In case there is a factor known to be highly effective in the separation (such a flow rate or temperature), optimization designs can be preferred directly. 37 In this study, factors that may affect the results, such as the column temperature, flow rate, and composition of the mobile phase, were chosen with the purpose of performing an optimization. Another reason for choosing an RSM design is to observe any interaction between the factors.
The most used RSM designs are CCD and BBD. BBD requires the fewest experiments among the RSM designs because it does not contain values that are maximum or minimum values in the experimental matrix. 33 Since BBD requires fewer experiments, and the experimental matrix does not contain the highest or lowest level in the combination, this experimental design prevents an unrealistic extreme scenario. Therefore, the experiment number, time, and cost are reduced. BBD can evaluate the linear and non-linear effects of factors. 34 , 66 Thus, BBD was selected for the experimental plan, data analysis and optimization process using the Design Expert ® Version 9 software.
Execution of experiments
Experimental executions were computed by Design Expert Software. Robustness was assessed by using BBD with 29 runs. Experimental design and calculated concentrations of enalapril (as maleate) and amlodipine and the corresponding responses are given in Table 2 .

Statistical evaluation of responses and their interpretations
The best fit model was linear for all factors and their responses. In the literature, linear analysis is frequently indicated and recommended in robustness tests. 29 , 30 Therefore, our results were as expected. Linear models are used to show the main effects of factors.
The equation model for Y 1 (enalapril concentration) and Y 2 (amlodipine concentration) was as follows:
Y 1 =32.32+0.079X 1 -5.32X 2 +0.11X 3 +0.51X 4 (Equation 1)
Y 2 =16.19+0.12X 1 -2.72X 2 +0.020X 3 +0.021X 4 (Equation 2)
Where, X 1 is column temperature, X 2 is flow rate, X 3 is the methanol ratio in the mobile phase, and X 4 is the pH of the mobile phase.
The ANOVA results are given in Table 3 . The significant effects showed a p value less than 0.05, a low SD (CV %), and a high adjusted R-square (adj R 2 ) value indicating a good relationship between the experimental data and those of the fitted model. The predicted R-square (pred R 2 ) value was in agreement with the adj R 2 for all responses.

The one-factor graphs ( Figure 3 , ,4) 4 ) demonstrated that the flow rate was the most significant factor on the responses; inverse proportionality was found (p<0.05). It was revealed that the most critical factor in robustness is the flow rate. The methanol ratio in mobile phase, temperature, and pH had no significant effect on the calculated concentrations of amlodipine and enalapril in defined levels. Kovacs et al. 30 have evaluated the same factors in their robustness test with different responses such as peak asymmetry and retention time. They found that the proportion of methanol in the mobile phase had a significant effect on the retention time of strontium ranelate. Similarly, Dhumal et al. 32 found that the proportion of methanol in the mobile phase and the flow rate had a negative effect, while the pH had a positive effect on the peak area and the determined tapentadol concentration. In another study, in which the same factors and different responses (tailing factor, retention time and theoretical plate) were used, the most effective factors were found to be the methanol composition and pH. 45 However, the significance of factors depends on the APIs and chromatographic conditions. If we had defined our levels more broadly for other factors (methanol ratio, temperature, and pH) or if we had assessed more responses such as tailing factor or resolution we might have observed a meaningful effect with other factors. However, this was not considered to be an error in the design because the DOE is specific to the purpose. In this study, we would like to see how possible rational changes would affect the analytical results, rather than creating a design space based on the extreme values of factors.

A-D) One-factor graphs of the main effects of the factors on amlodipine concentration

A-D) One-factor graphs of the main effects of the factors on enalapril concentration
Two-way interactions between independent variables were found to be insignificant (p>0.05). Therefore, a simple screening design, such as a PBD, which is the most popular design in robustness evaluation, might be used in this study. 37 However, since PBD is a two-level design, it can cause inaccurate statistical evaluations when unsuitable factor levels are selected or when there might be an interaction between the factors. If an experimental model is needed to determine tolerable variations, an optimization design is recommended by Sahu et al. 31 For this reason, as discussed before, we preferred a BBD that contained a third level (target middle level) and provided more information about the method. There have been similar studies with other drugs in which calculated drug concentrations were the only response and flow rate was the only significant factor in the response. 43 , 46
Optimization
Following linear model fitting, an optimization run was performed, and factor settings were defined using the prediction spreadsheet of the software ( Figure 5 ). The final optimized parameters were a flow rate of 1.205 mL/min, pH of 2.95, and column temperature of 25°C. The factors described in the optimization were very close to the nominal levels in the BBD design. Non-etheless, these minor changes caused a better peak shape for amlodipine and a lower tailing factor (from 1.417 to 1.164, p<0.05) ( Figure 6 ). Retention times were not changed in the method with 3.8 min and 7.9 min for enalapril and amlodipine, respectively.

Optimization conditions of independent variables according to the Design Expert ® Software

Chromatograms of enalapril (8 μg/mL, as maleate) and amlodipine (4 μg/mL) in the optimized method
The optimized method was validated based on international guidelines.
The linearity of the peak area versus concentration was shown in the range of 0.8-24 µg/mL for amlodipine and 1.6-48 µg/mL for enalapril (as maleate). Linearity results were given in Table 4 . The linearity range was kept wider than the previously published methods. 24 , 25 , 26 The lower concentrations are considered for the first minutes of the dissolution study, and higher values are for the assay.

Accuracy was demonstrated using six different solutions, containing 1.39, 2.78, 5.56, 12, 16, and 19.2 µg/mL of amlodipine and 2.78, 5.56, 11.12, 24, 32, and 38.4 µg/mL of enalapril maleate. Recovery values were obtained within the range of 98.6%-101.6%. The low value of relative standard deviation (RSD) less than 1% indicates that the proposed method is accurate. Results are presented in Table 5 .

Repeatability
Repeatability is also termed intraday precision and provides information about the precision under the same operating conditions in a short time interval. 47 Repeatability was assessed using 10 determinations of the solutions including 16 µg/mL of amlodipine and 32 µg/mL of enalapril maleate. The recovery values were 99.9±0.31% and 100±0.07% for amlodipine and enalapril maleate, respectively.
The RSDs were 0.307% and 0.0711% for amlodipine and enalapril maleate, respectively.
Intermediate precision
Intermediate precision was assessed using the interday variations. Two different concentrations (4 and 16 µg/mL for amlodipine and 8 and 32 µg/mL for enalapril maleate) were analyzed on three consecutive days. The RSD values of interday precision were less than 1%, confirming the method precision. The results are given in Table 6 .

The low RSD value for intermediate precision and repeatability of the method as well as within-day and day-to-day variation suggested that the method was precise within the range of measurement.
Limit of detection (LOD) and limit of quantification (LOQ)
LOD and LOQ were calculated based on the SD of the response and the slope by using the equations below:
L O D = 3 . 3 × σ S ( Equation 3 )
L O Q = 10 × σ S ( Equation 4 )
where s is the SD of the response, and S is the slope of the calibration curve. According to the equations, LOD values were 0.0631 µg/mL and 0.0424 µg/mL and LOQ were 0.19 µg/mL and 0.129 µg/mL for amlodipine and enalapril maleate, respectively.
The LOD and LOQ results suggested that the method was highly sensitive.
The drugs dissolved in 0.1N HCl were stable when stored at 25°C for 72 hours. After 72 hours, drug recovery values were 99.7% for amlodipine and 99.4% for enalapril maleate.
Assay in tablets
The optimized method was used for the assay of amlodipine and enalapril in FDC tablets. An additional peak from excipients was not observed. The results were in the range of the labeled amount ±5% for both drugs ( Table 7 ).

Dissolution
Dissolution was performed with the in-house FDC tablet by using USP apparatus II in 0.1N HCl. 0.1N HCl was selected as the model dissolution medium. The proposed HPLC method was available for dissolution of FDC tablets. Both amlodipine and enalapril were dissolved more than 85% within 10 min. Dissolution profiles of amlodipine and enalapril were given in Figure 7 . The dissolution media of 0.1N HCl replaces the artificial stomach medium that is frequently used with the purpose of formulation development and quality control. For using this analytical method for other dissolution media such as pH 4.5 or pH 6.8 there might be small modifications in chromatographic conditions.

Dissolution results of amlodipine and enalapril in an in-house FDC product (n=3)
FDC: Fixed-dose combination
In conclusion, an accurate, precise, specific, and environmentally appropriate HPLC method was developed and validated for amlodipine besylate and enalapril maleate in the typical dosage unit. The BBD, an optimization design, was used to evaluate the operational factors in a robustness test, and validation was performed according to international guidelines. The developed method was more economic and suitable for green chemistry with less solvent consumption, which improved column performance. The method was applied to assay and dissolution studies and was found suitable for quality control tests and in vitro performance of pharmaceutical dosage forms for a fixed-dose tablet combination containing amlodipine besylate and enalapril maleate for the treatment of hypertension.
Acknowledgments
The authors would like to thank Nobel Pharma (Turkey) for providing amlodipine besylate and enalapril maleate as gift samples.
Conflicts of interest: No conflict of interest was declared by the authors. The authors alone are responsible for the content and writing of the paper.
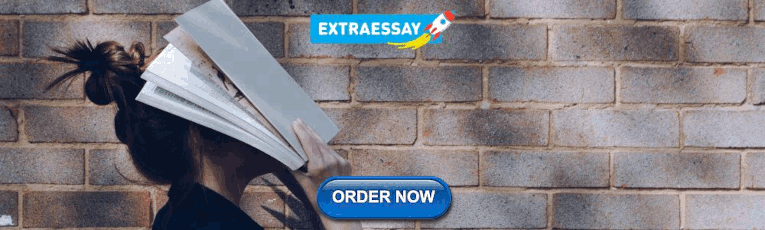
IMAGES
VIDEO
COMMENTS
In shorter scientific papers, where the aim is to report the findings of a specific study, you might simply describe what you did in a methods section. In a longer or more complex research project, such as a thesis or dissertation , you will probably include a methodology section , where you explain your approach to answering the research ...
Summary of Methods Chapter Strategies ! Most important: Explain each of your methodology choices by linking it to what you want to learn. Show how your methods are the best way to answer your research question - how various methodological choices you made (e.g., decision to do multiple site comparison) provided leverage for understanding
A thesis is an in-depth research study that identifies a particular topic of inquiry and presents a clear argument or perspective about that topic using evidence and logic. Writing a thesis showcases your ability of critical thinking, gathering evidence, and making a compelling argument. Integral to these competencies is thorough research ...
Thesis. Your thesis is the central claim in your essay—your main insight or idea about your source or topic. Your thesis should appear early in an academic essay, followed by a logically constructed argument that supports this central claim. A strong thesis is arguable, which means a thoughtful reader could disagree with it and therefore ...
It is very important to choose the right research methodology and methods for your thesis, as your research is the base that your entire thesis will rest on. It will be difficult for me to choose a research method for you. You will be the best judge of the kind of methods that work for your research. However, I can guide you on how you can ...
A thesis research methodology explains the type of research performed, justifies the methods that you chose by linking back to the literature review, and describes the data collection and analysis procedures.It is included in your thesis after the Introduction section.Most importantly, this is the section where the readers of your study evaluate its validity and reliability.
This chapter provides a detailed description of the research methods used to gather and analyze data. It should explain the research design, the sampling method, data collection techniques, and data analysis procedures. ... Submit the Thesis: Finally, ... Writing a thesis requires extensive research and analytical skills. It helps to develop ...
Good analytic thesis statements require digging deeper and often looking into the context. Let's say you do some research and learn that the rapper's mother died not long ago, and when you examine the lyrics more closely, you see that a few of the lines seem to be specifically about a mother rather than a loved one in general ("why"). ...
QDA Method #3: Discourse Analysis. Discourse is simply a fancy word for written or spoken language or debate. So, discourse analysis is all about analysing language within its social context. In other words, analysing language - such as a conversation, a speech, etc - within the culture and society it takes place.
In addition, the use of the technique required a detailed explanation in the methods chapter of the thesis. Explaining in detail what was done, and why it was done in the selected way (Modell & Humphrey, 2008), was necessary to ensure that the reader could trust the research. Sharing the analytical approach in this paper is motivated by several ...
It is certified that PhD Thesis titled "Development and Validation of Analytical Methods for Estimation of Anti-Diabetic Drugs" by Ms. Nidhi Chhabilkumar Kotecha has been examined by us. We undertake the following: a. Thesis has significant new work / knowledge as compared already published or are under consideration to be published elsewhere.
Descriptive Analytics. Definition: Descriptive analytics focused on describing or summarizing raw data and making it interpretable. This type of analytics provides insight into what has happened in the past. It involves the analysis of historical data to identify patterns, trends, and insights. Descriptive analytics often uses visualization ...
Content analysis is a research method used to identify patterns in recorded communication. To conduct content analysis, you systematically collect data from a set of texts, which can be written, oral, or visual: Books, newspapers and magazines. Speeches and interviews. Web content and social media posts. Photographs and films.
A good thesis has two parts. It should tell what you plan to argue, and it should "telegraph" how you plan to argue—that is, what particular support for your claim is going where in your essay. Steps in Constructing a Thesis. First, analyze your primary sources. Look for tension, interest, ambiguity, controversy, and/or complication.
Journal scope. Analytical Methods welcomes early applications of new analytical and bioanalytical methods and technology demonstrating the potential for societal impact. We require that methods and technology reported in the journal are sufficiently innovative, robust, accurate, and compared to other available methods for the intended application.
Identify analytical thesis statements. In order to write an analysis, you want to first have a solid understanding of the thing you are analyzing. Remember, when you are analyzing as a writer, you are: Breaking down information or artifacts into component parts. Uncovering relationships among those parts.
develop newer analytical methods for such drug s. The method development provides the following. requirements to the analyst so as to enab le him to. estimate the drug. The required data for a ...
The QbD approach to analytical method development was used for better understanding of method variables with different levels. Background A QbD is defined as "A systemic approach to the method development that begins with predefined objectives and emphasizes product and process understanding and process control, based on sound science and ...
How to Do Thematic Analysis | Step-by-Step Guide & Examples. Published on September 6, 2019 by Jack Caulfield.Revised on June 22, 2023. Thematic analysis is a method of analyzing qualitative data.It is usually applied to a set of texts, such as an interview or transcripts.The researcher closely examines the data to identify common themes - topics, ideas and patterns of meaning that come up ...
This method, which is available for assay and dissolution studies, was fast, environmentally friendly, and more cost-effective than the earlier published methods. 24,25,26 In this study, DOE was adapted to the robustness parameter of the analytical method for determining amlodipine and enalapril together. DOE principles were used in the method ...
The Shodhganga@INFLIBNET Centre provides a platform for research students to deposit their Ph.D. theses and make it available to the entire scholarly community in open access. Shodhganga@INFLIBNET. Jawaharlal Nehru Technological University, Anantapuram. Department of Pharmaceutical Sciences.