Have a language expert improve your writing
Run a free plagiarism check in 10 minutes, generate accurate citations for free.
- Knowledge Base
- Dissertation
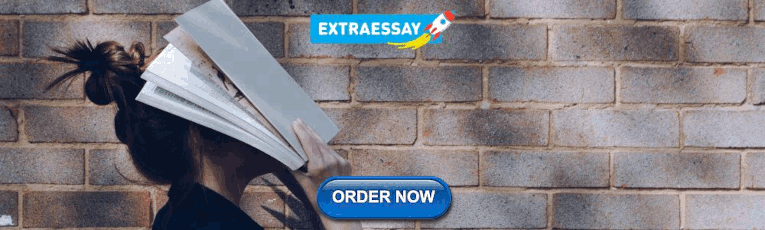
List of Abbreviations | Example, Template & Best Practices
Published on May 23, 2022 by Tegan George . Revised on July 18, 2023.
A list of abbreviations is an alphabetical list of abbreviations that you can add to your thesis or dissertation . If you choose to include it, it should appear at the beginning of your document, just after your table of contents .
Abbreviation lists improve readability, minimizing confusion about abbreviations unfamiliar to your reader. This can be a worthwhile addition to your thesis or dissertation if your dissertation topic or field of study uses a lot of abbreviations in papers.
If you only use a few abbreviations, you don’t necessarily need to include a list. However, it’s never a bad idea to add one if your abbreviations are numerous, or if you think they will not be known to your audience.
You can download our template below in the format of your choice to help you get started.
Download Word doc Download Google doc
Instantly correct all language mistakes in your text
Upload your document to correct all your mistakes in minutes
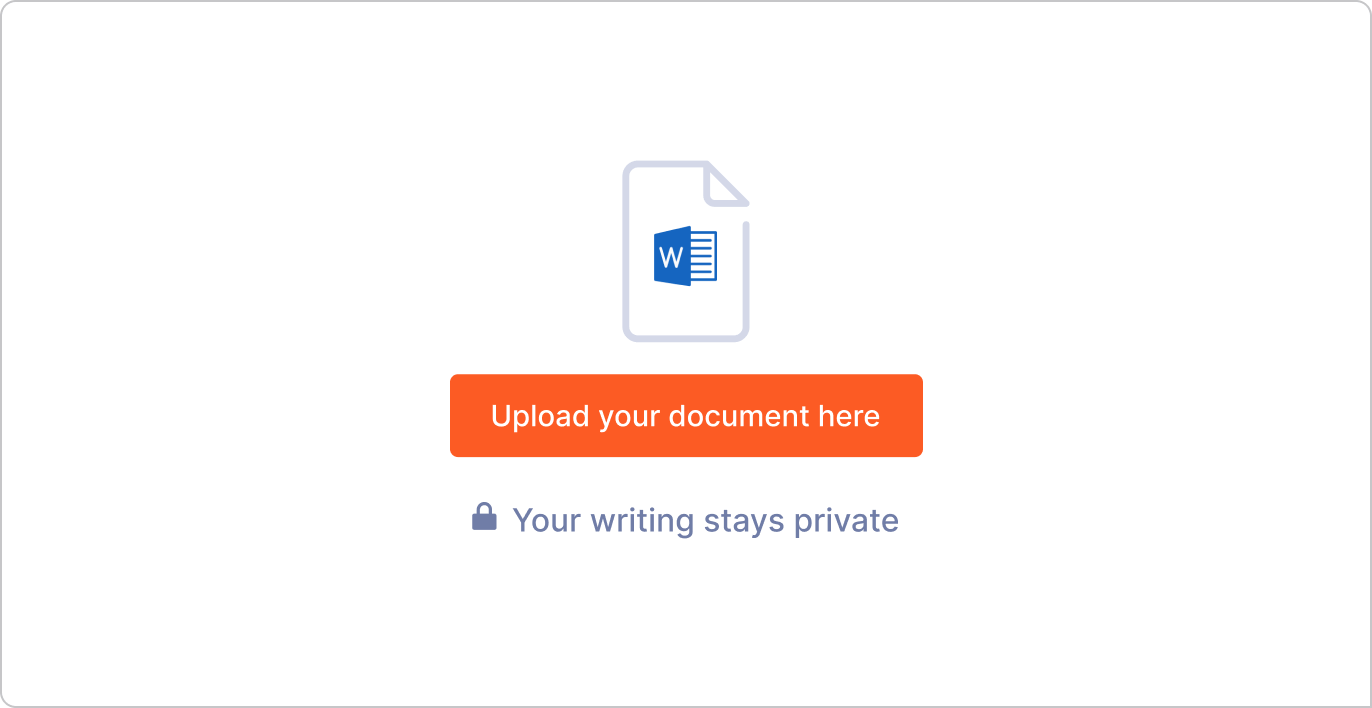
- Table of contents
Example list of abbreviations
Best practices for abbreviations and acronyms, additional lists to include, other interesting articles, frequently asked questions.
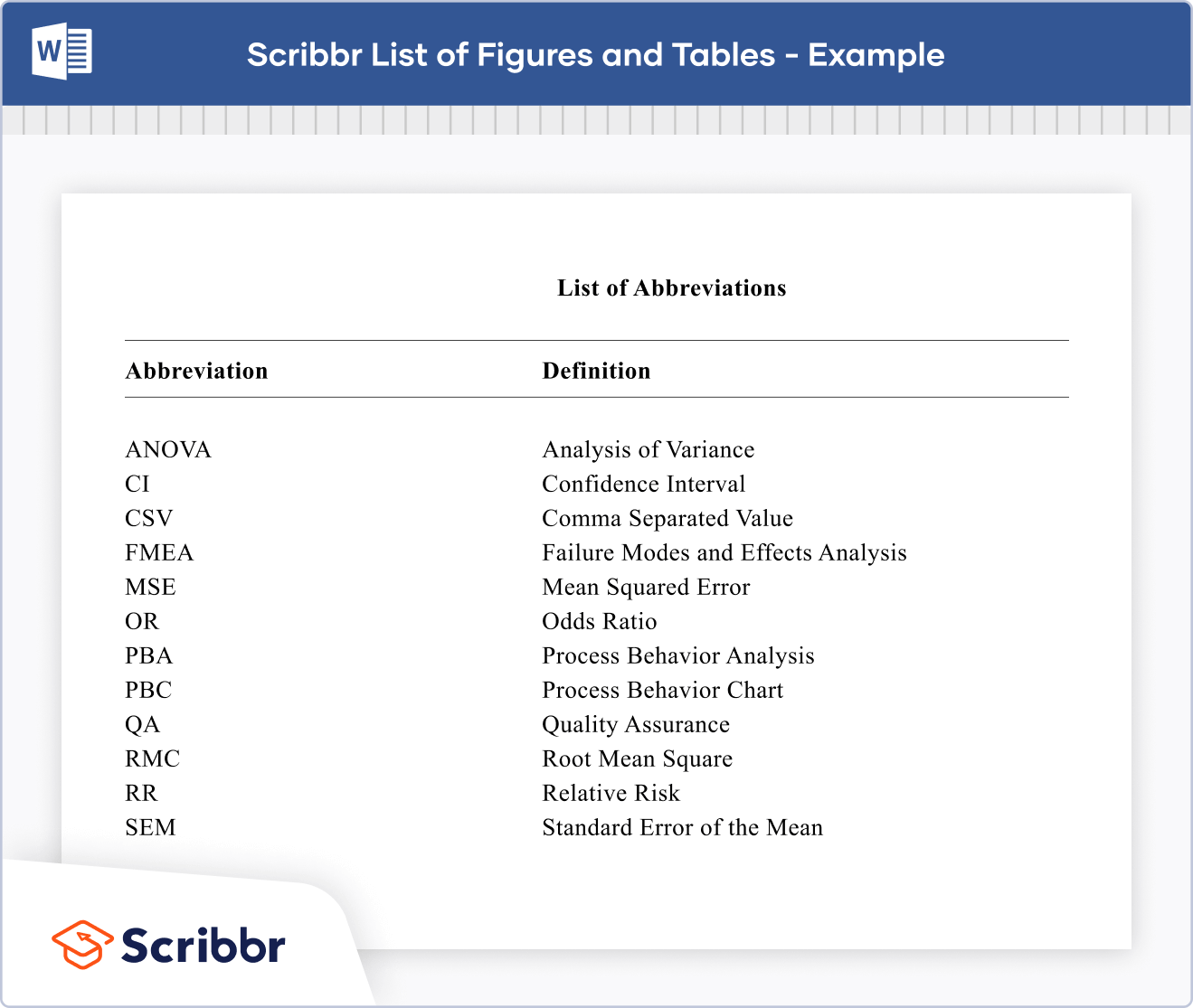
Here's why students love Scribbr's proofreading services
Discover proofreading & editing
There are a few rules to keep in mind about using abbreviations in academic writing. Here are a few tips.
- Acronyms are formed using the first letter of each word in a phrase. The first time you use an acronym, write the phrase in full and place the acronym in parentheses immediately after it. You can then use the acronym throughout the rest of the text.
- The same guidance goes for abbreviations: write the explanation in full the first time you use it, then proceed with the abbreviated version.
- If you’re using very common acronyms or abbreviations, such as USA, PC, or NASA, you can abbreviate them from the get-go. If you’re in doubt, just write it out in full the first time.
As well as the list of abbreviations, you can also use a list of tables and figures and a glossary for your thesis or dissertation.
Include your lists in the following order:
- List of figures and tables
- List of abbreviations
If you want to know more about AI for academic writing, AI tools, or research bias, make sure to check out some of our other articles with explanations and examples or go directly to our tools!
Research bias
- Anchoring bias
- Halo effect
- The Baader–Meinhof phenomenon
- The placebo effect
- Nonresponse bias
- Deep learning
- Generative AI
- Machine learning
- Reinforcement learning
- Supervised vs. unsupervised learning
(AI) Tools
- Grammar Checker
- Paraphrasing Tool
- Text Summarizer
- AI Detector
- Plagiarism Checker
- Citation Generator
The only proofreading tool specialized in correcting academic writing - try for free!
The academic proofreading tool has been trained on 1000s of academic texts and by native English editors. Making it the most accurate and reliable proofreading tool for students.
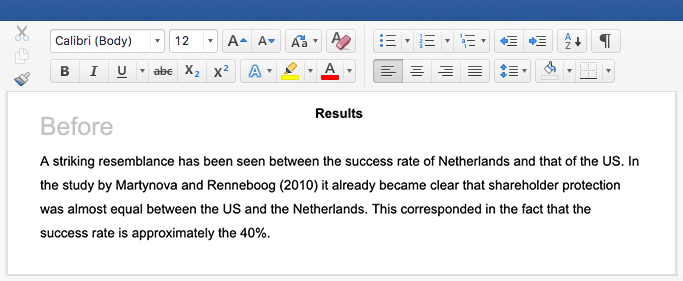
Try for free
As a rule of thumb, write the explanation in full the first time you use an acronym or abbreviation. You can then proceed with the shortened version. However, if the abbreviation is very common (like PC, USA, or DNA), then you can use the abbreviated version from the get-go.
Be sure to add each abbreviation in your list of abbreviations !
If you only used a few abbreviations in your thesis or dissertation , you don’t necessarily need to include a list of abbreviations .
If your abbreviations are numerous, or if you think they won’t be known to your audience, it’s never a bad idea to add one. They can also improve readability, minimizing confusion about abbreviations unfamiliar to your reader.
A list of abbreviations is a list of all the abbreviations that you used in your thesis or dissertation. It should appear at the beginning of your document, with items in alphabetical order, just after your table of contents .
An abbreviation is a shortened version of an existing word, such as Dr. for Doctor. In contrast, an acronym uses the first letter of each word to create a wholly new word, such as UNESCO (an acronym for the United Nations Educational, Scientific and Cultural Organization).
Cite this Scribbr article
If you want to cite this source, you can copy and paste the citation or click the “Cite this Scribbr article” button to automatically add the citation to our free Citation Generator.
George, T. (2023, July 18). List of Abbreviations | Example, Template & Best Practices. Scribbr. Retrieved March 18, 2024, from https://www.scribbr.com/dissertation/list-of-abbreviations/
Is this article helpful?
Tegan George
Other students also liked, dissertation table of contents in word | instructions & examples, figure and table lists | word instructions, template & examples, what is a glossary | definition, templates, & examples, "i thought ai proofreading was useless but..".
I've been using Scribbr for years now and I know it's a service that won't dissappoint. It does a good job spotting mistakes”
Abbreviations in Research: Common Errors in Academic Writing
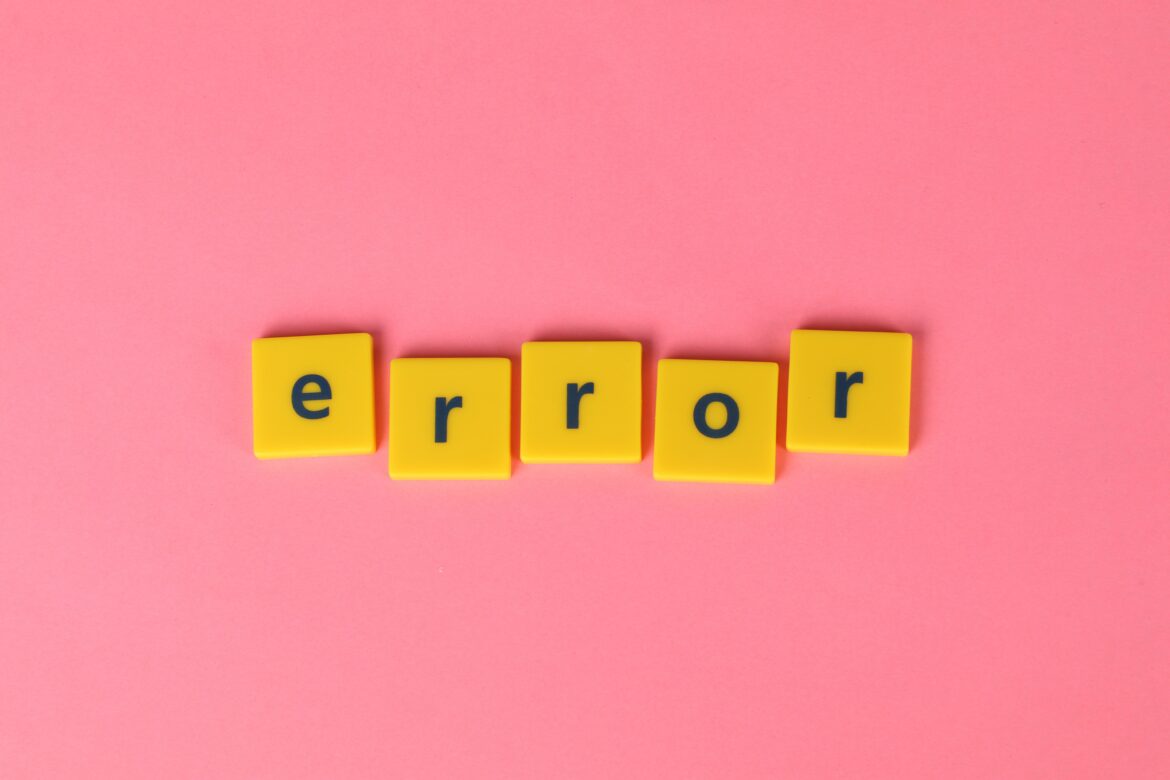
“Provided they are not obscure to the reader, abbreviations communicate more with fewer letters. Writers have only to ensure that the abbreviations they use are too well known to need any introduction, or that they are introduced and explained on their first appearance.”
—From “The Cambridge Guide to English Usage” by Pam Peters 1
David Crystal defines abbreviations as “a major component of the English writing system, not a marginal feature. The largest dictionaries of abbreviations contain well over half a million entries, and their number is increasing all the time.” 2 Students and researchers often use abbreviations in research writing to save space, especially when facing restrictions of page or word limits. Abbreviations in research are also used in place of long or difficult phrases for ease of writing and reading. Exactly how abbreviations in research writing should be used depends on the style guide you follow. For example, in British English, the period (or full stop) is omitted in abbreviations that include the first and last letters of a single word (e.g., “Dr” or “Ms”). But in American English, such abbreviations in writing are followed by a period (e.g., “Dr.” or “Ms.”).
While using abbreviations in academic writing is a common feature in many academic and scientific papers, most journals prefer keeping their use to a minimum or restricting their use to standard abbreviations. As a general rule, all non-standard acronyms/abbreviations in research papers should be written out in full on first use (in both the abstract and the paper itself), followed by the abbreviated form in parentheses, as in the American Psychological Association (APA) style guide.
Table of Contents
- Mistakes to avoid when using acronyms and abbreviations in research writing3
- Tips to using abbreviations in research writing
Frequently Asked Questions (FAQ)
Mistakes to avoid when using acronyms and abbreviations in research writing 3.
- Avoid opening a sentence with an abbreviation in research papers; write the word out.
- Abbreviations such as a.m., p.m., B.C., and A.D. are never spelled out. Unless your style guide says otherwise, use lowercase or small capitals for a.m. and p.m. Use capital letters or small caps for B.C. and A.D. (the periods are optional).
- Avoid RAS Syndrome: RAS Syndrome stands for Redundant Acronym Syndrome…Syndrome. For example, DC Comics—DC already stands for “Detective Comics,” making Comics after DC redundant.
- Avoid Alphabet Soup: Alphabet soup refers to using too many abbreviations in academic writing. Do not abbreviate the words if their frequency of appearance in the document is less than three.
- Do not follow acronyms with a period unless at the end of a sentence.
- When pluralizing acronyms add a lowercase “s” at the end (“three ECGs”); acronyms can be made possessive with an apostrophe followed by a lowercase “s” (“DOD’s acknowledge”).
- Acronyms are treated as singulars, even when they stand for plurals. Therefore, they require a singular verb (“NASA is planning to…”).
- Articles “a” or “an” before an acronym should be based on the opening sound rather than the acronym’s meaning. This depends on whether they are pronounced as words or as a series of letters. Use “an” if a soft vowel sound opens the acronym; else, use “a.” For example, a NATO meeting; an MRI scan.
Tips to using abbreviations in research writing
1. When to abbreviate: Using too many abbreviations in research papers can make the document hard to read. While it makes sense to abbreviate every long word, it’s best to abbreviate terms you use repeatedly.
2. Acronyms and initialisms: Define all acronyms and initialisms on their first use by giving the full terminology followed by the abbreviation in brackets. Once defined, use the shortened version in place of the full term.
3. Contractions: Using contractions (isn’t, can’t, don’t, etc.) in academic writing, such as a research paper, is usually not encouraged because it can make your writing sound informal.
4. Latin abbreviations: Latin abbreviations in research are widely preferred as they contain much meaning in a tiny package. Most style manuals (APA, MLA, and Chicago) suggest limiting the use of Latin abbreviations in the main text. They recommend using etc. , e.g. , and i.e., in parentheses within the body of a text, but others should appear only in footnotes, endnotes, tables, and other forms of documentation. But APA allows using “ et al .” when citing works with multiple authors and v. in the titles of court cases.
5. Capitalization: Abbreviations in writing are in full capital letters (COBOL, HTML, etc.). Exceptions include acronyms such as “radar,” “scuba,” and “lidar,” which have become commonly accepted words.
6. Punctuation: Abbreviations in research can be written without adding periods between each letter. However, when shortening a word, we usually add a period as follows:
Figure → Fig.
Doctor → Dr.
January → Jan.
Note that units of measurement do not require a period after the abbreviation. But, to avoid confusion with the word “ in ,” we write “ inches ” as “ in. ” in documents.
7. Create a list: Make a list of the abbreviations in research as you write. Adding such a list at the start of your document can give the reader and yourself an easy point of reference.
References
- Peters, P. The Cambridge Guide to English Usage. Cambridge University Press (2004).
- Crystal, D. Spell it out: The singular story of English spelling (2013).
- Nordquist, R. 10 Tips for Using Abbreviations Correctly (July 25, 2019). Retrieved from https://www.thoughtco.com/tips-for-using-abbreviations-correctly-1691738
Create error-free research papers with Paperpal’s AI writing tool
Abbreviations in a research paper are shortened forms of words or phrases used to represent specific terms or concepts. They are employed to improve readability and conciseness, especially when there are strict word counts and terms are mentioned frequently throughout the paper. To ensure clarity, it is essential to define each abbreviation when it is first used in the research paper. This is typically done by providing the full term followed by its abbreviation in parentheses.
Some commonly used abbreviations in academic writing include e.g. (exempli gratia), i.e. (id est), et al. (et alia/et alii), etc. (et cetera), cf. (confer), and viz. (videlicet). Additionally, there are several subject-specific abbreviations that are known by and commonly used in a field of study. However, know that abbreviations may mean different things across different fields. This makes it important to consult style guides or specific guidelines provided by the academic institution or target publication to ensure consistent and appropriate use of abbreviations in your academic writing.
An acronym is an abbreviation formed from the initial letters of a series of words and is pronounced as a word itself. For example, NASA (National Aeronautics and Space Administration) and UNESCO (United Nations Educational, Scientific and Cultural Organization) are acronyms. An abbreviation is a shortened form of a word or phrase but they are usually pronounced as individual letters. Examples of abbreviations include “et al.” for “et alia/et alii” and “e.g.” for “exempli gratia.”
Paperpal is a comprehensive AI writing toolkit that helps students and researchers achieve 2x the writing in half the time. It leverages 21+ years of STM experience and insights from millions of research articles to provide in-depth academic writing, language editing, and submission readiness support to help you write better, faster.
Get accurate academic translations, rewriting support, grammar checks, vocabulary suggestions, and generative AI assistance that delivers human precision at machine speed. Try for free or upgrade to Paperpal Prime starting at US$19 a month to access premium features, including consistency, plagiarism, and 30+ submission readiness checks to help you succeed.
Experience the future of academic writing – Sign up to Paperpal and start writing for free!
Related Reads:
- Breaking Down the Difference Between Further and Farther
- Decoding the Difference Between Insure and Ensure
- Principle vs. Principal: How Are They Different?
- Adapt vs. Adopt: Difference, Meaning and Examples Comparison
Academic Translation Simplified! Paperpal Introduces “Translate” Feature Aimed at ESL Researchers
Paperpal’s 7 most read research reads of 2022, you may also like, how to paraphrase research papers effectively, 4 types of transition words for research papers , paraphrasing in academic writing: answering top author queries, sentence length: how to improve your research paper..., navigating language precision: complementary vs. complimentary, climatic vs. climactic: difference and examples, language and grammar rules for academic writing, transitive and intransitive verbs in the world of..., led vs. lead: how to differentiate between the..., academic writing for esl students: 7 tips and strategies....
Have a language expert improve your writing
Run a free plagiarism check in 10 minutes, automatically generate references for free.
- Knowledge Base
- Dissertation
- List of Abbreviations | Example, Template & Best Practices
List of Abbreviations | Example, Template & Best Practices
Published on 23 May 2022 by Tegan George . Revised on 25 October 2022.
A list of abbreviations is an alphabetical list of abbreviations that you can add to your thesis or dissertation. If you choose to include it, it should appear at the beginning of your document, just after your table of contents .
Abbreviation lists improve readability, minimising confusion about abbreviations unfamiliar to your reader. This can be a worthwhile addition to your thesis or dissertation if you find that you’ve used a lot of abbreviations in your paper.
If you only use a few abbreviations, you don’t necessarily need to include a list. However, it’s never a bad idea to add one if your abbreviations are numerous, or if you think they will not be known to your audience.
You can download our template below in the format of your choice to help you get started.
Download Word doc Download Google doc
Instantly correct all language mistakes in your text
Be assured that you'll submit flawless writing. Upload your document to correct all your mistakes.
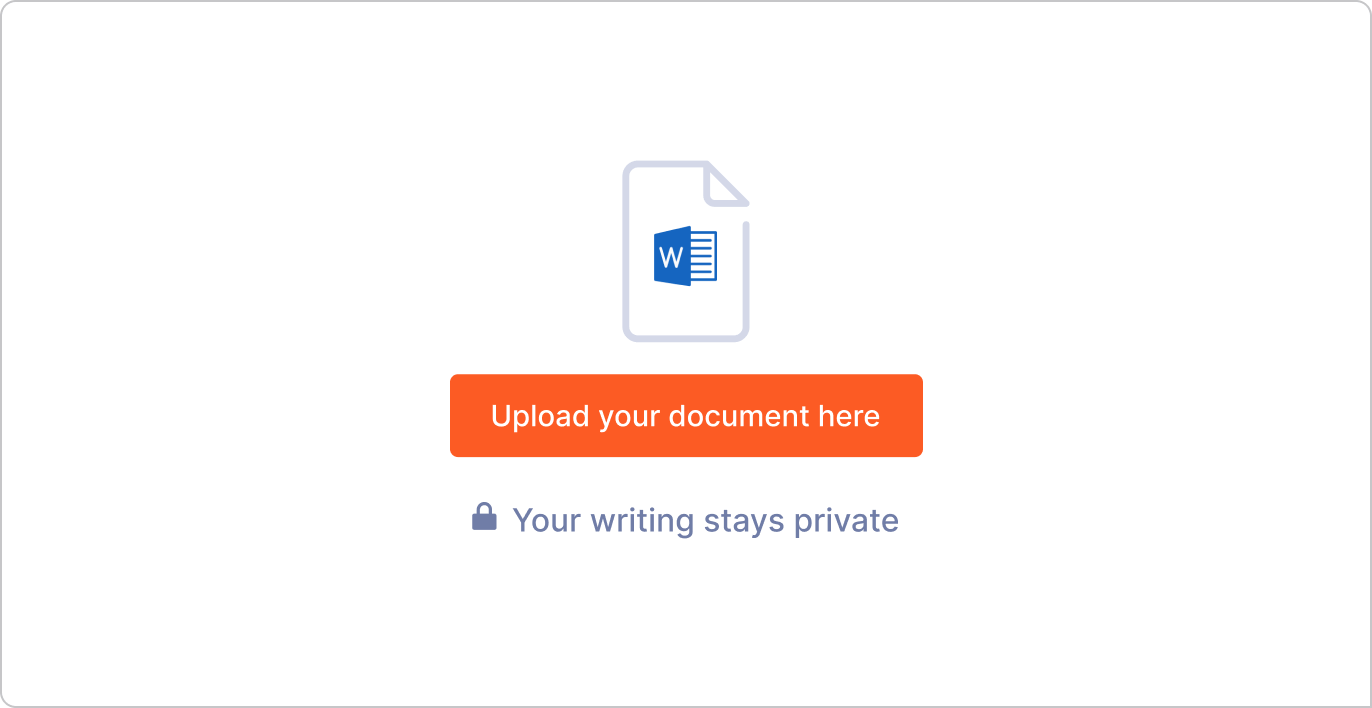
- Table of contents
Example list of abbreviations
Best practices for abbreviations and acronyms, additional lists to include, frequently asked questions.
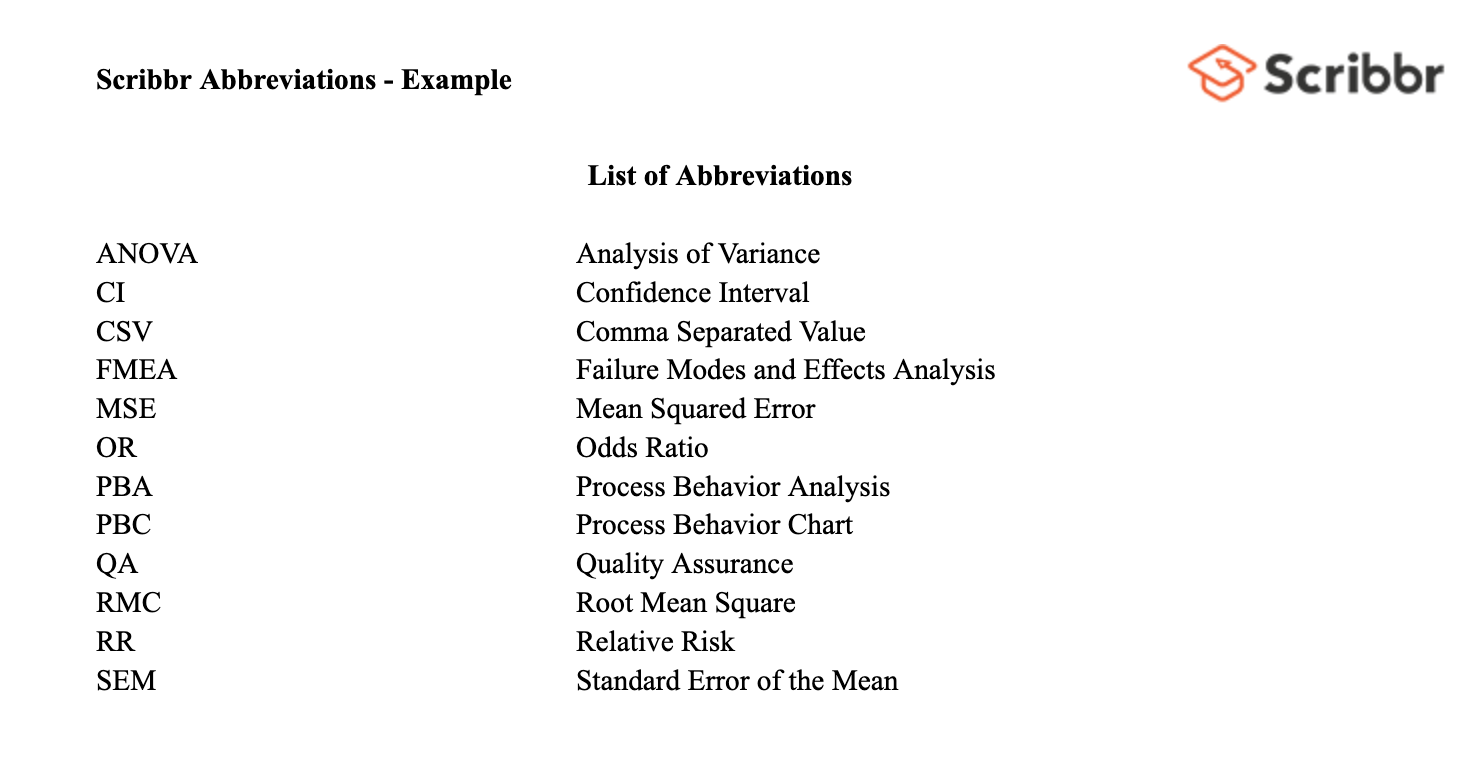
Prevent plagiarism, run a free check.
There are a few rules to keep in mind about using abbreviations in academic writing. Here are a few tips.
- Acronyms are formed using the first letter of each word in a phrase. The first time you use an acronym, write the phrase in full and place the acronym in parentheses immediately after it. You can then use the acronym throughout the rest of the text.
- The same guidance goes for abbreviations: write the explanation in full the first time you use it, then proceed with the abbreviated version.
- If you’re using very common acronyms or abbreviations, such as UK or DNA, you can abbreviate them from the first use. If you’re in doubt, just write it out in full the first time.
As well as the list of abbreviations, you can also use a list of tables and figures and a glossary for your thesis or dissertation.
Include your lists in the following order:
- List of figures and tables
- List of abbreviations
As a rule of thumb, write the explanation in full the first time you use an acronym or abbreviation. You can then proceed with the shortened version. However, if the abbreviation is very common (like UK or PC), then you can just use the abbreviated version straight away.
Be sure to add each abbreviation in your list of abbreviations !
If you only used a few abbreviations in your thesis or dissertation, you don’t necessarily need to include a list of abbreviations .
If your abbreviations are numerous, or if you think they won’t be known to your audience, it’s never a bad idea to add one. They can also improve readability, minimising confusion about abbreviations unfamiliar to your reader.
A list of abbreviations is a list of all the abbreviations you used in your thesis or dissertation. It should appear at the beginning of your document, immediately after your table of contents . It should always be in alphabetical order.
An abbreviation is a shortened version of an existing word, such as Dr for Doctor. In contrast, an acronym uses the first letter of each word to create a wholly new word, such as UNESCO (an acronym for the United Nations Educational, Scientific and Cultural Organization).
Your dissertation sometimes contains a list of abbreviations .
Cite this Scribbr article
If you want to cite this source, you can copy and paste the citation or click the ‘Cite this Scribbr article’ button to automatically add the citation to our free Reference Generator.
George, T. (2022, October 25). List of Abbreviations | Example, Template & Best Practices. Scribbr. Retrieved 18 March 2024, from https://www.scribbr.co.uk/thesis-dissertation/abbreviations-list/
Is this article helpful?
Tegan George
Other students also liked, dissertation table of contents in word | instructions & examples, dissertation title page, research paper appendix | example & templates.

Community Blog
Keep up-to-date on postgraduate related issues with our quick reads written by students, postdocs, professors and industry leaders.
List of Abbreviations for a Thesis or Dissertation

- By DiscoverPhDs
- September 14, 2020
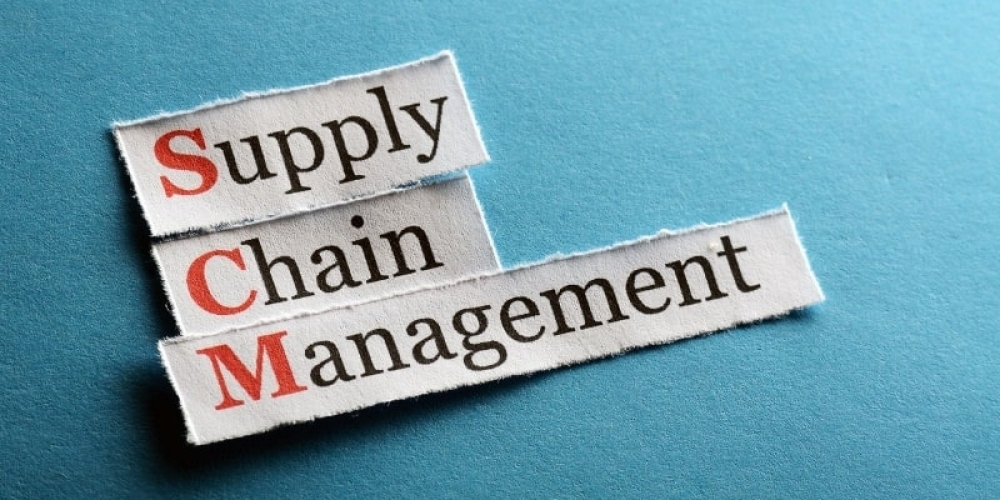
What are Abbreviations and Acronyms?
An abbreviation is a shortened version of a term or phrase, e.g. kg for kilogram or Dr. for doctor.
An acronym is a type of abbreviation constructed from the first letters of a term, e.g. FRP for Fibre Reinforced Polymer or STEM for Science, Technology, Engineering and Maths.
List of Abbreviations in a Thesis or Dissertation
If your thesis or dissertation contains several symbols or abbreviations, it would be beneficial to include a list of abbreviations to assist your reader. This is a list sorted in alphabetical order that gives their definitions.
This will not only help the reader better understand your research, but it will also improve the flow of your paper, as it prevents continually having to define abbreviations in your main text.
Where Does a List of Abbreviations Go?
When including a list of abbreviations, insert them near the start of the report after your table of contents. To make it clear that your document contains an abbreviated list, also add a separate heading to your table of contents.
Note: The page number for your list of abbreviations should continue from the page number that proceeds it; there is no need to reset it for this section.
Rules for Using Abbreviations and Acronyms
The first time you use an abbreviation or acronym, it is good practice to write out the full terminology or phrase followed by the abbreviation or acronym encased in parenthesis.
After defining an abbreviation or acronym for the first time in your main text, you no longer need to use the full term; for example:
Example of Acronyms in a Thesis or Dissertation
This allows the reader to understand your report without having to rely on the list of abbreviations; it is only there to help the reader if they forget what an abbreviation stands for and needs to look it up.
Note: In academic writing, abbreviations that are not listed should always be defined in your thesis text at their first appearance.
Abbreviated Exceptions
Very common abbreviations should not be included in your list because they needlessly overload your list with terms that your readers already know, which discourages them from using it.
Some examples of common abbreviations and acronyms that should not be included in your standard abbreviation list are USA, PhD , Dr. and Ltd. etc.
Example of List of Abbreviations for a Thesis or Dissertation
An example abbreviation list is as follows:

The above example has been extracted from here .
List of Symbols
You can add symbols and their definitions to your list of abbreviations, however, some people like to keep them separate, especially if they have many of them. While this format will come down to personal preference, most STEM students create a separate list of symbols and most non-STEM students incorporate them into their list of abbreviations.
Note: If you are writing your report to APA style, you will need to consider additional requirements when writing your list of abbreviations. You can find further information here .
Further Reading
Whether you’re writing a Ph.D. thesis or a dissertation paper, the following resources will also be of use:
- Title Page for an Academic Paper
- List of Appendices
Finding a PhD has never been this easy – search for a PhD by keyword, location or academic area of interest.
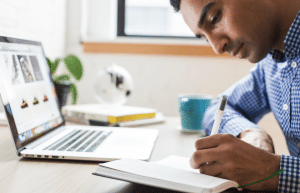
A well written figure legend will explain exactly what a figure means without having to refer to the main text. Our guide explains how to write one.

The term monotonic relationship is a statistical definition that is used to describe the link between two variables.
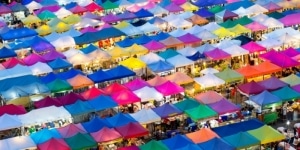
Multistage sampling is a more complex form of cluster sampling for obtaining sample populations. Learn their pros and cons and how to undertake them.
Join thousands of other students and stay up to date with the latest PhD programmes, funding opportunities and advice.
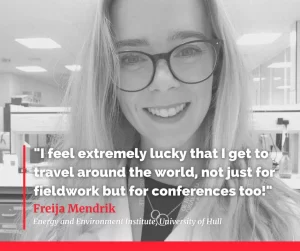
Browse PhDs Now
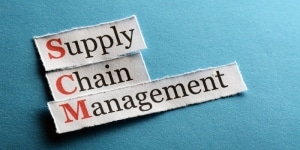
Need to write a list of abbreviations for a thesis or dissertation? Read our post to find out where they go, what to include and how to format them.
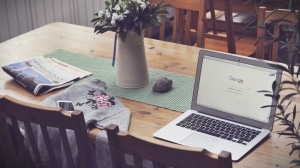
Learn about defining your workspace, having a list of daily tasks and using technology to stay connected, all whilst working from home as a research student.

Elmira is in the third year of her PhD program at the Moscow Institute of Physics and Technology; Engelhardt Institute of Molecular Biology, researching the mechanisms of acute myeloid leukemia cells resistance to targeted therapy.
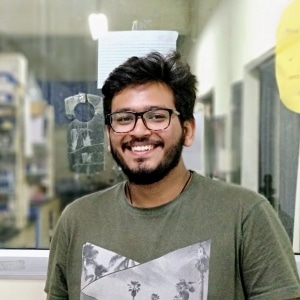
Jay is in the third year of his PhD at Savitribai Phule Pune University, researching the applications of mesenchymal stem cells and nanocarrier for bone tissue engineering.
Join Thousands of Students

OASIS: Writing Center
Other apa guidelines: abbreviations, basics of abbreviations.
Using abbreviations can be an effective way to avoid repeating lengthy, technical terms throughout a piece of writing, but they should be used sparingly to prevent your text from becoming difficult to read.
Many abbreviations take the form of acronyms or initialisms, which are abbreviations consisting of the first letter of each word in a phrase. Examples are National Institute of Mental Health (NIMH) and Better Business Bureau (BBB). Note that the abbreviation uses all capital letters, and there are no periods between the letters.
To use an abbreviation, write out the term or phrase on first use, followed by the abbreviation in parentheses. See these examples:
The patient had been diagnosed with traumatic brain injury (TBI) in March of the previous year. Walden students need to know how to cite information using the American Psychological Association (APA) guidelines.
Using an Abbreviation in a Draft
After introducing the abbreviation, use the abbreviation by itself, without parentheses, throughout the rest of the document.
The patient had been diagnosed with traumatic brain injury (TBI) in March of 2014. According to the Centers for Disease Control and Prevention (CDC, 2015), people with TBI often have difficulty with memory and concentration, physical symptoms such as headaches, emotional symptoms such as sadness and irritability, and difficulty falling asleep. Although the patient explained that she experienced frequent headaches and difficulty concentrating, she had not been regularly taking any medication for her TBI symptoms when she visited the clinic 6 months after her diagnosis.
Note: When introducing an abbreviation within a narrative citation, use a comma between the abbreviation and the year.
Making an Abbreviation Plural
Simply add an “s” to an abbreviation to make it plural. (Do not add an apostrophe.)
I work with five other RNs during a typical shift.
Note: RN is a commonly used acronym found in Merriam-Webster’s Dictionary , so it does not need to be introduced. See the “Exceptions to the Rules” section below for more information about commonly used abbreviations.
Exceptions to the Rules
There are a few exceptions to the basic rules:
- If you use the phrase three times or fewer, it should be written out every time. However, a standard abbreviation for a term familiar in its abbreviated form is clearer and more concise, even if it is used fewer than three times.
- Commonly used acronyms and abbreviations may not need to be written out. If an abbreviation appears as a word in Merriam-Webster’s Collegiate Dictionary , then it does not need to be written it out on first use. Examples include words such as IQ, REM, and HIV.
- Other than abbreviations prescribed by APA in reference list elements (e.g., “ed.” for “edition,” “n.d.” for “no date,” etc.), do not use abbreviations in the references list. For example, a source authored by the Centers for Disease Control and Prevention would not be abbreviated as CDC in the references list.
- If using an abbreviation for a unit of measure with a numerical value, you do not need to write the term out on first use. For example, instead of writing “12 grams;” you can simply use “12 g.” If, however, you use a unit of measure without a numerical value, write the term out (e.g., “several grams”).
- Abbreviations for time, common Latin terms, and statistical abbreviations also follow specific rules. See APA 7, Sections 6.28, 6.29, and 6.44 for more information.
United States and U.S.
In APA style, "United States" should always be spelled out when it is used as a noun or location.
Example: In the United States, 67% reported this experience.
United States can be abbreviated as "U.S." when it is used as an adjective.
Examples: U.S. population and U.S. Census Bureau.
Abbreviations Video
- APA Formatting & Style: Abbreviations (video transcript)
Related Resources

Knowledge Check: Abbreviations
Didn't find what you need? Search our website or email us .
Read our website accessibility and accommodation statement .
- Previous Page: Overview
- Next Page: Active and Passive Voice
- Office of Student Disability Services
Walden Resources
Departments.
- Academic Residencies
- Academic Skills
- Career Planning and Development
- Customer Care Team
- Field Experience
- Military Services
- Student Success Advising
- Writing Skills
Centers and Offices
- Center for Social Change
- Office of Academic Support and Instructional Services
- Office of Degree Acceleration
- Office of Research and Doctoral Services
- Office of Student Affairs
Student Resources
- Doctoral Writing Assessment
- Form & Style Review
- Quick Answers
- ScholarWorks
- SKIL Courses and Workshops
- Walden Bookstore
- Walden Catalog & Student Handbook
- Student Safety/Title IX
- Legal & Consumer Information
- Website Terms and Conditions
- Cookie Policy
- Accessibility
- Accreditation
- State Authorization
- Net Price Calculator
- Contact Walden
Walden University is a member of Adtalem Global Education, Inc. www.adtalem.com Walden University is certified to operate by SCHEV © 2024 Walden University LLC. All rights reserved.
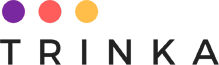
Acronyms and Abbreviations in Academic Writing: A Quick Guide

Abbreviations, acronyms, and Latin expressions can all be found in academic and scientific articles. But as a writer, it can be puzzling to know exactly when and how to use abbreviations and acronyms in formal academic publications. Let’s learn what types of abbreviations there are, what the different style manuals have to say about them, and when you should and shouldn’t include them in your scientific paper.
Table of Content:
What is an abbreviation, using abbreviations properly, how can i use abbreviations in academic writing, how can i avoid making abbreviation mistakes.
An abbreviation is a word that has been shortened from its original longer form. There are several different types of abbreviations.
Acronyms. An acronym is an abbreviation made from the first letter of each word in a name or phrase. Acronyms are pronounced like a word. NASA, which is short for N ational A eronautics and S pace A dministration and pronounced “Nah-suh,” is one well-known acronym. UNICEF, which stands for U nited N ations I nternational C hildren’s E mergency F und and is pronounced “you-nuh-seff,” is another example.
Initialisms. An initialism is similar to an acronym because it is an abbreviation that uses the first letter of each word in a name or phrase. Unlike acronyms, however, the letters of an initialism are pronounced individually. FBI, which is short for F ederal B ureau of I nvestigations, is an initialism. UK (United Kingdom) and USA (United States of America) are also initialisms.
Contractions. A contraction is when two words are combined for ease with an apostrophe. Common examples of contractions include don’t (do not), aren’t (are not), and let’s (let us).
Latin abbreviations. Latin abbreviations are commonly used in academia and law. Common latin abbreviations used in English writing are etc . (et cetera, “and so on”), e.g . (exempli gratia, “for example”), i.e . (id est, “in other words”), and et al . (et alii, “and others”).
Titles, measurements, and dates. Titles ( Dr . for Doctor, Rev . for Reverend), measurement units ( cm for centimeter, kg for kilogram), and days ( Feb . for February, Fri . for Friday) can all be abbreviated.
Before you begin using abbreviations in academic writing, there are a few rules you need to know. There are three main things to keep in mind when using abbreviations in an academic or scientific paper. These are spacing, capitalization, and punctuation . It is important to note that, like many other aspects of writing, the rules are sometimes different in the US and UK variations of English.
- Spacing . For measurement units, there should be a space between the number and the unit. The unit is written after the number.
Correct : Mumbai had received 11 mm of rain by 6 pm . Incorrect : Mumbai had received 11mm of rain by 6pm .
- Capitalization . Initialisms and acronyms should be written in all capital letters.
Correct : The National Health Insurance Service (NHIS) seized Jimin’s $4.7 million dollar apartment for failure to pay premiums. Incorrect : The NHIS (National Health Insurance Service) seized Jimin’s $4.7 million dollar apartment for failure to pay premiums.
Note that if you are abbreviating a phrase that is not a proper noun (name of an institution, organization, etc.), then that phrase should not be capitalized when it is written prior to the abbreviation. For example, if you were introducing BP as an abbreviation for blood pressure, you would write “blood pressure (BP),” not “Blood Pressure (BP).”

Upgrade your writing skills
- Write with Trinka
- Punctuation . Generally, periods are used with latin abbreviations as well as dates . In US English, titles are followed by a period (Dr.), while in UK English, the period is omitted (Dr). Initialisms and acronyms generally don’t use periods (we write USSR rather than U.S.S.R.). Contractions use an apostrophe.
Now that you know how to use abbreviations, you may be wondering if and when you can use abbreviations in academic writing. You definitely can use abbreviations in academic writing if you keep the following rules in mind!
- Spell out the full phrase or term the first time you use it in your paper and include the abbreviation in parentheses. You can use the abbreviation each time after that.
- Don’t abbreviate everything . It is fine to abbreviate technical terms or phrases used repeatedly throughout your writing, but only if you use them more than a few times ! The Chicago Manual of Style advises abbreviating a term when it is used five or more times , while the APA only asks that you use an abbreviation if the term is used three or more times. The AMA guidelines suggest that words that are short and easy to write should not be abbreviated at all.
- Provide a list of abbreviations for your reader. Especially if you are writing a thesis, an academic article or a monograph, including a list of abbreviations used at the beginning will give your reader an easy reference.
- Avoid abbreviations in your paper abstract. While abbreviations are perfectly acceptable in the text of your paper, they should be avoided in your abstract as much as possible.
- Avoid contractions. Contractions in English are considered informal, and should therefore generally be avoided in academic writing.
- Minimize the use of latin abbreviations . It is fine to include parenthetical i.e . and e.g. here and there, but in general, it is preferred to simply write out “for example” or “in other words.” Researchers should specifically note that ending sentences with etc. in academic writing is frowned upon, and frequent use of this term is perceived as lazy writing.
- Know how to make initialisms and acronyms plural . You can pluralize abbreviations by adding a lowercase “s” at the end . CEOs, RNs, and NEATs are all examples of correct plural abbreviations.
- Be consistent! Inconsistent abbreviations can confuse the reader and make your paper appear poorly written. If you abbreviate a word, make sure you use the same abbreviation throughout the text.
In addition to following the tips and tricks above, you should always consult the relevant style manual when you are writing your academic or scientific paper to minimize any abbreviation errors. Another option is to use an AI grammar checker like Trinka. Trinka not only checks for grammar and spelling errors , it can also highlight inconsistent abbreviation use and makes sure that your paper adheres to a specific citation style. Abbreviations are a great way to make your paper more readable or adhere to a limited word count, so don’t be afraid to incorporate them into your academic writing today.
Go beyond grammar & spelling
Falling into a Subjunctive Mood: The Difference Between Was and Were
Canceled or Cancelled? How to Spell it Right Every Time
How to Write Any Type of Letter?
Inquire vs Enquire: What’s the Difference?
Leave A Reply Cancel Reply
Your email address will not be published.
Save my name, email, and website in this browser for the next time I comment.
Subscribe to stay updated with us!
Join thousands of subscribers to get regular updates on industry trends and our blog posts.
Purdue Online Writing Lab Purdue OWL® College of Liberal Arts
MLA Abbreviations
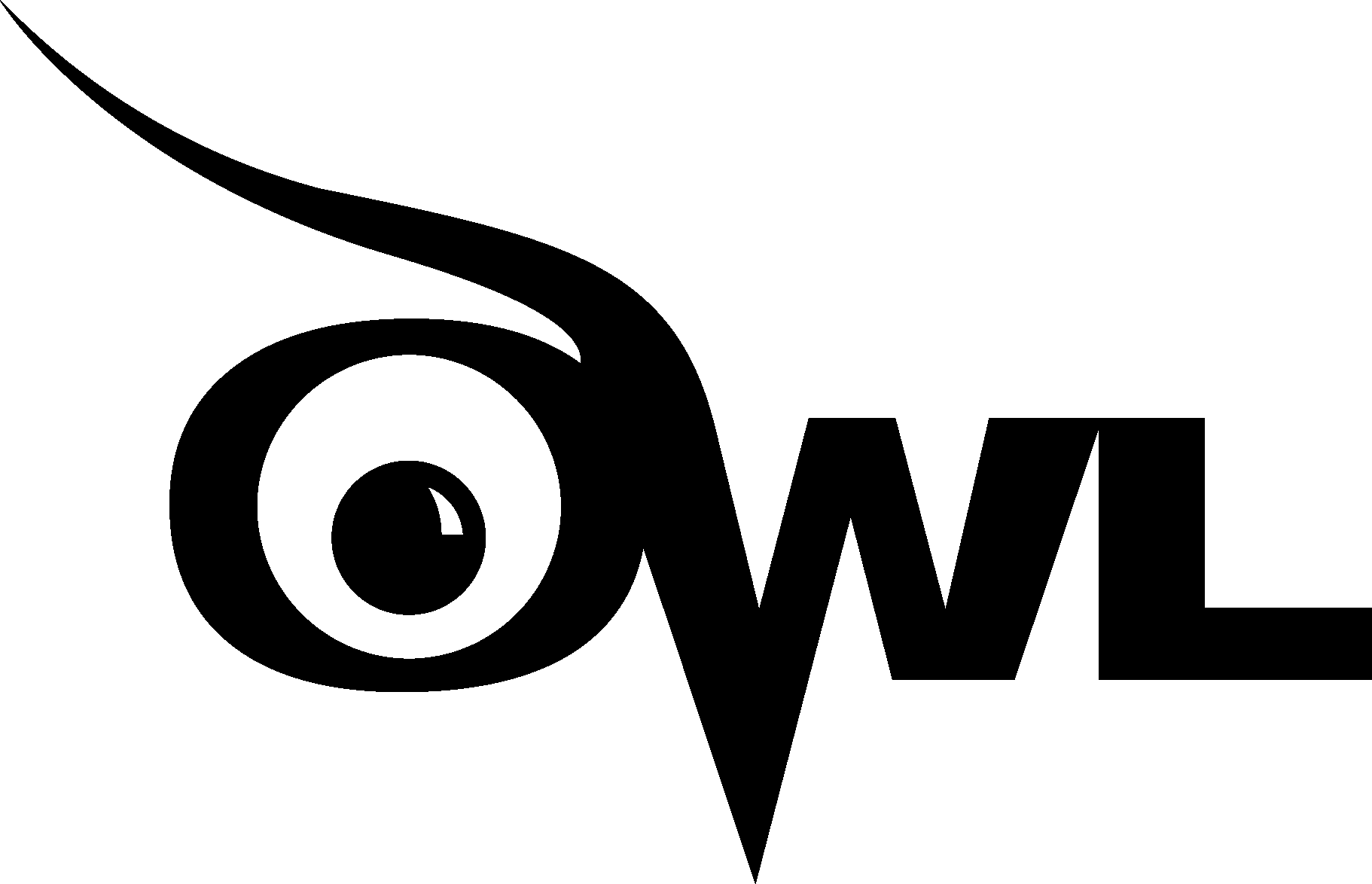
Welcome to the Purdue OWL
This page is brought to you by the OWL at Purdue University. When printing this page, you must include the entire legal notice.
Copyright ©1995-2018 by The Writing Lab & The OWL at Purdue and Purdue University. All rights reserved. This material may not be published, reproduced, broadcast, rewritten, or redistributed without permission. Use of this site constitutes acceptance of our terms and conditions of fair use.
MLA (Modern Language Association) style is most commonly used to write papers and cite sources within the liberal arts and humanities. This resource, updated to reflect the MLA Handbook (9 th ed.), offers examples for the general format of MLA research papers, in-text citations, endnotes/footnotes, and the Works Cited page.
There are a few common trends in abbreviating that you should follow when using MLA, though there are always exceptions to these rules. For a complete list of common abbreviations used in academic writing, see Appendix 1 in the MLA Handbook (9 th ed.).
Uppercase letter abbreviations
Do not use periods or spaces in abbreviations composed solely of capital letters, except in the case of proper names:
unless the name is only composed of initials:
Lowercase letter abbreviations
Use a period if the abbreviation ends in a lowercase letter, unless referring to an Internet suffix, where the period should come before the abbreviation:
Note: Degree names are a notable exception to the lowercase abbreviation rule.
Use periods between letters without spacing if each letter represents a word in common lowercase abbreviations:
Other notable exceptions:
Abbreviations in citations
Condense citations as much as possible using abbreviations.
Time Designations
Remember to follow common trends in abbreviating time and location within citations. Month names longer than four letters used in journal and magazine citations should be abbreviated:
Geographic Names
Use geographic names of states and countries. Abbreviate country, province, and state names.
Scholarly Abbreviations
The MLA Handbook (9 th ed.) encourages users to adhere to the common scholarly abbreviations for both in-text citations and in the works-cited page. Here is the list of common scholarly abbreviations from Appendix 1 of the MLA Handbook (9 th ed.) with a few additions:
- anon. for anonymous
- app. for appendix
- bk. for book
- c. or ca. for circa
- ch. for chapter
- col. for column
- def. for definition
- dept. for department
- e.g. for example
- ed. for edition
- et al. for multiple names (translates to "and others")
- etc. for "and so forth"
- fig. for figure
- fwd. for foreword
- i.e. for that is
- jour. for journal
- lib. for library
- MS, MSS for manuscript(s)
- no. for number
- P for Press (used for academic presses)
- p. for page, pp. for pages
- par. for paragraph when page numbers are unavailable
- qtd. in for quoted in
- rev. for revised
- sec. or sect. for section
- ser. for series
- trans. for translation
- U for University (for example, Purdue U)
- UP for University Press (for example, Yale UP or U of California P)
- vers. for version
- var. for variant
- vol. for volume
Publisher Names
Cite publishers’ names in full as they appear on title or copyright pages. For example, cite the entire name for a publisher (e.g. W. W. Norton or Liveright Publishing).
Exceptions:
- Omit articles and business abbreviations (like Corp., Inc., Co., and Ltd.).
- Use the acronym of the publisher if the company is commonly known by that abbreviation (e.g. MLA, ERIC, GPO). For publishers who are not known by an abbreviation, write the entire name.
- Use only U and P when referring to university presses (e.g. Cambridge UP or U of Arkansas P)
For more information on scholarly abbreviations, see Appendix 1 of the MLA Handbook (9 th ed.) . See also the following examples:
- Subject guides
- Citing and referencing
- Abbreviations used in referencing
Citing and referencing: Abbreviations used in referencing
- In-text citations
- Reference list
- Books and book chapters
- Journals/Periodicals
- Newspapers/Magazines
- Government and other reports
- Legal sources
- Websites and social media
- Audio, music and visual media
- Conferences
- Dictionaries/Encyclopedias/Guides
- Theses/Dissertations
- University course materials
- Company and Industry reports
- Patents and Standards
- Tables and Figures
- Medicine and Health sources
- Foreign language sources
- Music scores
- Journals and periodicals
- Government sources
- News sources
- Web and social media
- Games and apps
- Ancient and sacred sources
- Primary sources
- Audiovisual media and music scores
- Images and captions
- University lectures, theses and dissertations
- Interviews and personal communication
- Archival material
- In-Text Citations: Further Information
- Reference List: Standard Abbreviations
- Data Sheets (inc. Material Safety Data Sheets (MSDS))
- Figures & Tables (inc. Images)
- Lecture Materials (inc. PowerPoint Presentations)
- Reports & Technical Reports
- Theses and Dissertations
- Reference list guidelines
- Journal articles
- Government and industry publications
- Websites, newspaper and social media
- Conference papers, theses and university material
- Video and audio
- Images, graphs, tables, data sets
- Personal communications
- In-text Citations
- Journals / Periodicals
- Encyclopedias and Dictionaries
- Interviews and lectures
- Music Scores / Recordings
- Film / Video Recording
- Television / Radio Broadcast
- Online Communication / Social Media
- Live Performances
- Government and Organisation Publications
- Medicine & health sources
- Government/organisational/technical reports
- Images, graphs, tables, figures & data sets
- Websites newspaper & magazine articles, socia media
- Conferences, theses & university materials
- Personal communication & confidential unpublished material
- Video, audio & other media
- Generative AI
- Indigenous knowledges
APA Contents
- Introduction to APA style
- In-Text Citations
Abbreviations
- Audio and Visual media
- Journals/periodicals
- Tables and figures
- Sample reference list
- Standard abbreviations can be used in your citations.
- Some of the more commonly used examples of abbreviations are listed below.
Compiled or custom textbook
No page numbers, revised edition, translator(s).
Writing academically: Abbreviations
- Academic style
- Personal pronouns
- Contractions
Abbreviations
- Signposting
- Paragraph structure
- Using sources in your writing
Jump to content on this page:
“Quote” Author, Book
Abbreviations and acronyms are shortened forms of words or phrases. Generally, abbreviations are not acceptable in academic writing (with some exceptions, see below) and acronyms are (providing they are used as shown below).
As academic writing is formal in style, most abbreviations should be avoided. Even the common ones shown below:
Some common ones to avoid
Avoid e.g. and i.e. , instead use for example and for instance .
Avoid etc . There isn't really an alternative, so rewrite the sentence.
Avoid dept , govt . Use department , government .
Avoid NB , instead use note that .
Avoid vs or v , instead use versus or against (except in Law reports or cases)
Some acceptable abbreviations
Titles such as Mr. Dr. Prof. are acceptable when using them in conjunction with the individual's name i.e. Dr. Smith.
Some Latin phrases
et al. (short form of et alia - and others is acceptable when giving in text citations with multiple authors. The full stop should always be included afterwards to acknowledge the abbreviation. It does not need to be italicised as it is in common usage.
ibid. (short form of ibidim - in the same place) is acceptable if using footnote references to indicate that a reference is the same as the previous one. Again, always include the full stop to acknowledge the abbreviation. It is the convention to italicise this as it is less commonly used.
sic (short form of sic erat scriptum - thus it was written). This is used to indicate there was an error in something you are quoting (either an interviewee or an author) and it is not a misquote. It is added in square brackets but is neither italicised nor followed by a full stop i.e.
"it'd be great if unis [sic] could develop a person's self-knowledge"
Acronyms are acceptable, but use the name in full on its first use in a particular document (e.g. an assignment), no matter how well known the acronym is. For example, on its first use in an essay you might refer to "the World Health Organisation (WHO)" - it would be fine to simply refer to "the WHO" for the remainder of the essay.
- << Previous: Italics
- Next: Signposting >>
- Last Updated: Nov 10, 2023 4:11 PM
- URL: https://libguides.hull.ac.uk/writing
- Login to LibApps
- Library websites Privacy Policy
- University of Hull privacy policy & cookies
- Website terms and conditions
- Accessibility
- Report a problem
We use cookies to give you the best experience possible. By continuing we’ll assume you’re on board with our cookie policy
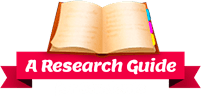
- A Research Guide
- Research Paper Guide
- Common & Uncommon Abbreviations for Research Papers
Common & Uncommon Abbreviations for Research Papers
- Common Abbreviations
Abbreviations of Degrees
Some common abbreviations.
For a complete list of Common Scholarly Abbreviations, please see Section 7.4 in the 6th edition of the MLA Handbook for Writers of Research Papers .
Note: When documenting sources using MLA style, the normal punctuation is omitted for degrees when used in parentheses, tables, works cited, footnotes, endnotes, etc. For example, B.A. is written as BA. Other abbreviations retain the periods if applicable, e.g. acad., bib., misc. Italics = Latin
Also, g reat post to read about US and Canada Map .
Links to Common, Uncommon, and Specialized Abbreviations
Acronym Finder . Look up acronyms, abbreviations and their meanings.
Acronym Search . Your source for acronyms and abbreviations.
Common Abbreviations for posting messages on Bulletin Boards – from BabyCentre.co.uk. Examples: AKA = Also known as, LOL = Laughing out loud, BTW = By the way, CUL = See you later, Yw/Ty = You’re welcome/thank you. Emotions: 🙂 or 🙂 = Smiling (Happy), 🙁 or 🙁 = Frowning (Sad), 😮 = Shouting, xxooxxoo = Love (or hugs) & kisses. See also Shorthand and Meaning from Genealogy.com. (Scroll way down the page to view Shorthand). Examples: = I’m grinning, IMHO = in my humble opinion, FYI = for your information, FWIW = for what it’s worth, ROTFL = rolling on the floor laughing, WTG = way to go, Emotions: 😉 or 😉 = winking, 😀 or 😀 = laughing.
Common Abbreviations from Fact Monster for kids.
Common Abbreviations, Common Symbols, Acronyms for Organizations from Web-based Training Modules funded by the U.S. National Cancer Institute’s Surveillance, Epidemiology and End Results (SEER) Program.
Common Abbreviations from U.S. Department of State.
Common Abbreviations and Acronynms from AllEarsNet.com – Deb’s Unofficial Walt Disney World Information Guide.
Common Abbreviations in Writing.
Common Abbreviations Used in International Narcotics Control Strategy Report.
HyperWar – World War II on the WorldWideWeb – Abbreviations, Acronyms, Codewords, Terms .
Military Abbreviations and Acronyms of the US Armed Forces.
Also, great post to read concerning Abbreviations for Books of the Bible .
By clicking "Log In", you agree to our terms of service and privacy policy . We'll occasionally send you account related and promo emails.
Sign Up for your FREE account

An official website of the United States government
The .gov means it’s official. Federal government websites often end in .gov or .mil. Before sharing sensitive information, make sure you’re on a federal government site.
The site is secure. The https:// ensures that you are connecting to the official website and that any information you provide is encrypted and transmitted securely.
- Publications
- Account settings
Preview improvements coming to the PMC website in October 2024. Learn More or Try it out now .
- Advanced Search
- Journal List
- Saudi J Anaesth
- v.13(Suppl 1); 2019 Apr
Writing the title and abstract for a research paper: Being concise, precise, and meticulous is the key
Milind s. tullu.
Department of Pediatrics, Seth G.S. Medical College and KEM Hospital, Parel, Mumbai, Maharashtra, India
This article deals with formulating a suitable title and an appropriate abstract for an original research paper. The “title” and the “abstract” are the “initial impressions” of a research article, and hence they need to be drafted correctly, accurately, carefully, and meticulously. Often both of these are drafted after the full manuscript is ready. Most readers read only the title and the abstract of a research paper and very few will go on to read the full paper. The title and the abstract are the most important parts of a research paper and should be pleasant to read. The “title” should be descriptive, direct, accurate, appropriate, interesting, concise, precise, unique, and should not be misleading. The “abstract” needs to be simple, specific, clear, unbiased, honest, concise, precise, stand-alone, complete, scholarly, (preferably) structured, and should not be misrepresentative. The abstract should be consistent with the main text of the paper, especially after a revision is made to the paper and should include the key message prominently. It is very important to include the most important words and terms (the “keywords”) in the title and the abstract for appropriate indexing purpose and for retrieval from the search engines and scientific databases. Such keywords should be listed after the abstract. One must adhere to the instructions laid down by the target journal with regard to the style and number of words permitted for the title and the abstract.
Introduction
This article deals with drafting a suitable “title” and an appropriate “abstract” for an original research paper. Because the “title” and the “abstract” are the “initial impressions” or the “face” of a research article, they need to be drafted correctly, accurately, carefully, meticulously, and consume time and energy.[ 1 , 2 , 3 , 4 , 5 , 6 , 7 , 8 , 9 , 10 ] Often, these are drafted after the complete manuscript draft is ready.[ 2 , 3 , 4 , 5 , 9 , 10 , 11 ] Most readers will read only the title and the abstract of a published research paper, and very few “interested ones” (especially, if the paper is of use to them) will go on to read the full paper.[ 1 , 2 ] One must remember to adhere to the instructions laid down by the “target journal” (the journal for which the author is writing) regarding the style and number of words permitted for the title and the abstract.[ 2 , 4 , 5 , 7 , 8 , 9 , 12 ] Both the title and the abstract are the most important parts of a research paper – for editors (to decide whether to process the paper for further review), for reviewers (to get an initial impression of the paper), and for the readers (as these may be the only parts of the paper available freely and hence, read widely).[ 4 , 8 , 12 ] It may be worth for the novice author to browse through titles and abstracts of several prominent journals (and their target journal as well) to learn more about the wording and styles of the titles and abstracts, as well as the aims and scope of the particular journal.[ 5 , 7 , 9 , 13 ]
The details of the title are discussed under the subheadings of importance, types, drafting, and checklist.
Importance of the title
When a reader browses through the table of contents of a journal issue (hard copy or on website), the title is the “ first detail” or “face” of the paper that is read.[ 2 , 3 , 4 , 5 , 6 , 13 ] Hence, it needs to be simple, direct, accurate, appropriate, specific, functional, interesting, attractive/appealing, concise/brief, precise/focused, unambiguous, memorable, captivating, informative (enough to encourage the reader to read further), unique, catchy, and it should not be misleading.[ 1 , 2 , 3 , 4 , 5 , 6 , 9 , 12 ] It should have “just enough details” to arouse the interest and curiosity of the reader so that the reader then goes ahead with studying the abstract and then (if still interested) the full paper.[ 1 , 2 , 4 , 13 ] Journal websites, electronic databases, and search engines use the words in the title and abstract (the “keywords”) to retrieve a particular paper during a search; hence, the importance of these words in accessing the paper by the readers has been emphasized.[ 3 , 4 , 5 , 6 , 12 , 14 ] Such important words (or keywords) should be arranged in appropriate order of importance as per the context of the paper and should be placed at the beginning of the title (rather than the later part of the title, as some search engines like Google may just display only the first six to seven words of the title).[ 3 , 5 , 12 ] Whimsical, amusing, or clever titles, though initially appealing, may be missed or misread by the busy reader and very short titles may miss the essential scientific words (the “keywords”) used by the indexing agencies to catch and categorize the paper.[ 1 , 3 , 4 , 9 ] Also, amusing or hilarious titles may be taken less seriously by the readers and may be cited less often.[ 4 , 15 ] An excessively long or complicated title may put off the readers.[ 3 , 9 ] It may be a good idea to draft the title after the main body of the text and the abstract are drafted.[ 2 , 3 , 4 , 5 ]
Types of titles
Titles can be descriptive, declarative, or interrogative. They can also be classified as nominal, compound, or full-sentence titles.
Descriptive or neutral title
This has the essential elements of the research theme, that is, the patients/subjects, design, interventions, comparisons/control, and outcome, but does not reveal the main result or the conclusion.[ 3 , 4 , 12 , 16 ] Such a title allows the reader to interpret the findings of the research paper in an impartial manner and with an open mind.[ 3 ] These titles also give complete information about the contents of the article, have several keywords (thus increasing the visibility of the article in search engines), and have increased chances of being read and (then) being cited as well.[ 4 ] Hence, such descriptive titles giving a glimpse of the paper are generally preferred.[ 4 , 16 ]
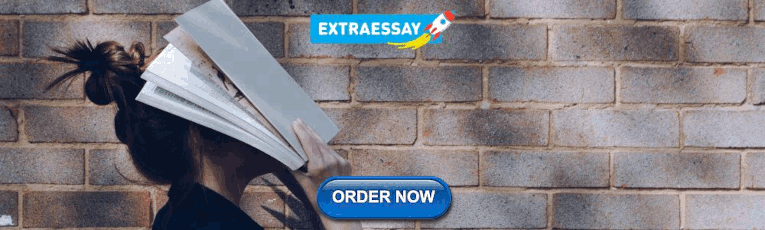
Declarative title
This title states the main finding of the study in the title itself; it reduces the curiosity of the reader, may point toward a bias on the part of the author, and hence is best avoided.[ 3 , 4 , 12 , 16 ]
Interrogative title
This is the one which has a query or the research question in the title.[ 3 , 4 , 16 ] Though a query in the title has the ability to sensationalize the topic, and has more downloads (but less citations), it can be distracting to the reader and is again best avoided for a research article (but can, at times, be used for a review article).[ 3 , 6 , 16 , 17 ]
From a sentence construct point of view, titles may be nominal (capturing only the main theme of the study), compound (with subtitles to provide additional relevant information such as context, design, location/country, temporal aspect, sample size, importance, and a provocative or a literary; for example, see the title of this review), or full-sentence titles (which are longer and indicate an added degree of certainty of the results).[ 4 , 6 , 9 , 16 ] Any of these constructs may be used depending on the type of article, the key message, and the author's preference or judgement.[ 4 ]
Drafting a suitable title
A stepwise process can be followed to draft the appropriate title. The author should describe the paper in about three sentences, avoiding the results and ensuring that these sentences contain important scientific words/keywords that describe the main contents and subject of the paper.[ 1 , 4 , 6 , 12 ] Then the author should join the sentences to form a single sentence, shorten the length (by removing redundant words or adjectives or phrases), and finally edit the title (thus drafted) to make it more accurate, concise (about 10–15 words), and precise.[ 1 , 3 , 4 , 5 , 9 ] Some journals require that the study design be included in the title, and this may be placed (using a colon) after the primary title.[ 2 , 3 , 4 , 14 ] The title should try to incorporate the Patients, Interventions, Comparisons and Outcome (PICO).[ 3 ] The place of the study may be included in the title (if absolutely necessary), that is, if the patient characteristics (such as study population, socioeconomic conditions, or cultural practices) are expected to vary as per the country (or the place of the study) and have a bearing on the possible outcomes.[ 3 , 6 ] Lengthy titles can be boring and appear unfocused, whereas very short titles may not be representative of the contents of the article; hence, optimum length is required to ensure that the title explains the main theme and content of the manuscript.[ 4 , 5 , 9 ] Abbreviations (except the standard or commonly interpreted ones such as HIV, AIDS, DNA, RNA, CDC, FDA, ECG, and EEG) or acronyms should be avoided in the title, as a reader not familiar with them may skip such an article and nonstandard abbreviations may create problems in indexing the article.[ 3 , 4 , 5 , 6 , 9 , 12 ] Also, too much of technical jargon or chemical formulas in the title may confuse the readers and the article may be skipped by them.[ 4 , 9 ] Numerical values of various parameters (stating study period or sample size) should also be avoided in the titles (unless deemed extremely essential).[ 4 ] It may be worthwhile to take an opinion from a impartial colleague before finalizing the title.[ 4 , 5 , 6 ] Thus, multiple factors (which are, at times, a bit conflicting or contrasting) need to be considered while formulating a title, and hence this should not be done in a hurry.[ 4 , 6 ] Many journals ask the authors to draft a “short title” or “running head” or “running title” for printing in the header or footer of the printed paper.[ 3 , 12 ] This is an abridged version of the main title of up to 40–50 characters, may have standard abbreviations, and helps the reader to navigate through the paper.[ 3 , 12 , 14 ]
Checklist for a good title
Table 1 gives a checklist/useful tips for drafting a good title for a research paper.[ 1 , 2 , 3 , 4 , 5 , 6 , 12 ] Table 2 presents some of the titles used by the author of this article in his earlier research papers, and the appropriateness of the titles has been commented upon. As an individual exercise, the reader may try to improvise upon the titles (further) after reading the corresponding abstract and full paper.
Checklist/useful tips for drafting a good title for a research paper
Some titles used by author of this article in his earlier publications and remark/comment on their appropriateness
The Abstract
The details of the abstract are discussed under the subheadings of importance, types, drafting, and checklist.
Importance of the abstract
The abstract is a summary or synopsis of the full research paper and also needs to have similar characteristics like the title. It needs to be simple, direct, specific, functional, clear, unbiased, honest, concise, precise, self-sufficient, complete, comprehensive, scholarly, balanced, and should not be misleading.[ 1 , 2 , 3 , 7 , 8 , 9 , 10 , 11 , 13 , 17 ] Writing an abstract is to extract and summarize (AB – absolutely, STR – straightforward, ACT – actual data presentation and interpretation).[ 17 ] The title and abstracts are the only sections of the research paper that are often freely available to the readers on the journal websites, search engines, and in many abstracting agencies/databases, whereas the full paper may attract a payment per view or a fee for downloading the pdf copy.[ 1 , 2 , 3 , 7 , 8 , 10 , 11 , 13 , 14 ] The abstract is an independent and stand-alone (that is, well understood without reading the full paper) section of the manuscript and is used by the editor to decide the fate of the article and to choose appropriate reviewers.[ 2 , 7 , 10 , 12 , 13 ] Even the reviewers are initially supplied only with the title and the abstract before they agree to review the full manuscript.[ 7 , 13 ] This is the second most commonly read part of the manuscript, and therefore it should reflect the contents of the main text of the paper accurately and thus act as a “real trailer” of the full article.[ 2 , 7 , 11 ] The readers will go through the full paper only if they find the abstract interesting and relevant to their practice; else they may skip the paper if the abstract is unimpressive.[ 7 , 8 , 9 , 10 , 13 ] The abstract needs to highlight the selling point of the manuscript and succeed in luring the reader to read the complete paper.[ 3 , 7 ] The title and the abstract should be constructed using keywords (key terms/important words) from all the sections of the main text.[ 12 ] Abstracts are also used for submitting research papers to a conference for consideration for presentation (as oral paper or poster).[ 9 , 13 , 17 ] Grammatical and typographic errors reflect poorly on the quality of the abstract, may indicate carelessness/casual attitude on part of the author, and hence should be avoided at all times.[ 9 ]
Types of abstracts
The abstracts can be structured or unstructured. They can also be classified as descriptive or informative abstracts.
Structured and unstructured abstracts
Structured abstracts are followed by most journals, are more informative, and include specific subheadings/subsections under which the abstract needs to be composed.[ 1 , 7 , 8 , 9 , 10 , 11 , 13 , 17 , 18 ] These subheadings usually include context/background, objectives, design, setting, participants, interventions, main outcome measures, results, and conclusions.[ 1 ] Some journals stick to the standard IMRAD format for the structure of the abstracts, and the subheadings would include Introduction/Background, Methods, Results, And (instead of Discussion) the Conclusion/s.[ 1 , 2 , 7 , 8 , 9 , 10 , 11 , 12 , 13 , 17 , 18 ] Structured abstracts are more elaborate, informative, easy to read, recall, and peer-review, and hence are preferred; however, they consume more space and can have same limitations as an unstructured abstract.[ 7 , 9 , 18 ] The structured abstracts are (possibly) better understood by the reviewers and readers. Anyway, the choice of the type of the abstract and the subheadings of a structured abstract depend on the particular journal style and is not left to the author's wish.[ 7 , 10 , 12 ] Separate subheadings may be necessary for reporting meta-analysis, educational research, quality improvement work, review, or case study.[ 1 ] Clinical trial abstracts need to include the essential items mentioned in the CONSORT (Consolidated Standards Of Reporting Trials) guidelines.[ 7 , 9 , 14 , 19 ] Similar guidelines exist for various other types of studies, including observational studies and for studies of diagnostic accuracy.[ 20 , 21 ] A useful resource for the above guidelines is available at www.equator-network.org (Enhancing the QUAlity and Transparency Of health Research). Unstructured (or non-structured) abstracts are free-flowing, do not have predefined subheadings, and are commonly used for papers that (usually) do not describe original research.[ 1 , 7 , 9 , 10 ]
The four-point structured abstract: This has the following elements which need to be properly balanced with regard to the content/matter under each subheading:[ 9 ]
Background and/or Objectives: This states why the work was undertaken and is usually written in just a couple of sentences.[ 3 , 7 , 8 , 9 , 10 , 12 , 13 ] The hypothesis/study question and the major objectives are also stated under this subheading.[ 3 , 7 , 8 , 9 , 10 , 12 , 13 ]
Methods: This subsection is the longest, states what was done, and gives essential details of the study design, setting, participants, blinding, sample size, sampling method, intervention/s, duration and follow-up, research instruments, main outcome measures, parameters evaluated, and how the outcomes were assessed or analyzed.[ 3 , 7 , 8 , 9 , 10 , 12 , 13 , 14 , 17 ]
Results/Observations/Findings: This subheading states what was found, is longer, is difficult to draft, and needs to mention important details including the number of study participants, results of analysis (of primary and secondary objectives), and include actual data (numbers, mean, median, standard deviation, “P” values, 95% confidence intervals, effect sizes, relative risks, odds ratio, etc.).[ 3 , 7 , 8 , 9 , 10 , 12 , 13 , 14 , 17 ]
Conclusions: The take-home message (the “so what” of the paper) and other significant/important findings should be stated here, considering the interpretation of the research question/hypothesis and results put together (without overinterpreting the findings) and may also include the author's views on the implications of the study.[ 3 , 7 , 8 , 9 , 10 , 12 , 13 , 14 , 17 ]
The eight-point structured abstract: This has the following eight subheadings – Objectives, Study Design, Study Setting, Participants/Patients, Methods/Intervention, Outcome Measures, Results, and Conclusions.[ 3 , 9 , 18 ] The instructions to authors given by the particular journal state whether they use the four- or eight-point abstract or variants thereof.[ 3 , 14 ]
Descriptive and Informative abstracts
Descriptive abstracts are short (75–150 words), only portray what the paper contains without providing any more details; the reader has to read the full paper to know about its contents and are rarely used for original research papers.[ 7 , 10 ] These are used for case reports, reviews, opinions, and so on.[ 7 , 10 ] Informative abstracts (which may be structured or unstructured as described above) give a complete detailed summary of the article contents and truly reflect the actual research done.[ 7 , 10 ]
Drafting a suitable abstract
It is important to religiously stick to the instructions to authors (format, word limit, font size/style, and subheadings) provided by the journal for which the abstract and the paper are being written.[ 7 , 8 , 9 , 10 , 13 ] Most journals allow 200–300 words for formulating the abstract and it is wise to restrict oneself to this word limit.[ 1 , 2 , 3 , 7 , 8 , 9 , 10 , 11 , 12 , 13 , 22 ] Though some authors prefer to draft the abstract initially, followed by the main text of the paper, it is recommended to draft the abstract in the end to maintain accuracy and conformity with the main text of the paper (thus maintaining an easy linkage/alignment with title, on one hand, and the introduction section of the main text, on the other hand).[ 2 , 7 , 9 , 10 , 11 ] The authors should check the subheadings (of the structured abstract) permitted by the target journal, use phrases rather than sentences to draft the content of the abstract, and avoid passive voice.[ 1 , 7 , 9 , 12 ] Next, the authors need to get rid of redundant words and edit the abstract (extensively) to the correct word count permitted (every word in the abstract “counts”!).[ 7 , 8 , 9 , 10 , 13 ] It is important to ensure that the key message, focus, and novelty of the paper are not compromised; the rationale of the study and the basis of the conclusions are clear; and that the abstract is consistent with the main text of the paper.[ 1 , 2 , 3 , 7 , 9 , 11 , 12 , 13 , 14 , 17 , 22 ] This is especially important while submitting a revision of the paper (modified after addressing the reviewer's comments), as the changes made in the main (revised) text of the paper need to be reflected in the (revised) abstract as well.[ 2 , 10 , 12 , 14 , 22 ] Abbreviations should be avoided in an abstract, unless they are conventionally accepted or standard; references, tables, or figures should not be cited in the abstract.[ 7 , 9 , 10 , 11 , 13 ] It may be worthwhile not to rush with the abstract and to get an opinion by an impartial colleague on the content of the abstract; and if possible, the full paper (an “informal” peer-review).[ 1 , 7 , 8 , 9 , 11 , 17 ] Appropriate “Keywords” (three to ten words or phrases) should follow the abstract and should be preferably chosen from the Medical Subject Headings (MeSH) list of the U.S. National Library of Medicine ( https://meshb.nlm.nih.gov/search ) and are used for indexing purposes.[ 2 , 3 , 11 , 12 ] These keywords need to be different from the words in the main title (the title words are automatically used for indexing the article) and can be variants of the terms/phrases used in the title, or words from the abstract and the main text.[ 3 , 12 ] The ICMJE (International Committee of Medical Journal Editors; http://www.icmje.org/ ) also recommends publishing the clinical trial registration number at the end of the abstract.[ 7 , 14 ]
Checklist for a good abstract
Table 3 gives a checklist/useful tips for formulating a good abstract for a research paper.[ 1 , 2 , 3 , 7 , 8 , 9 , 10 , 11 , 12 , 13 , 14 , 17 , 22 ]
Checklist/useful tips for formulating a good abstract for a research paper
Concluding Remarks
This review article has given a detailed account of the importance and types of titles and abstracts. It has also attempted to give useful hints for drafting an appropriate title and a complete abstract for a research paper. It is hoped that this review will help the authors in their career in medical writing.
Financial support and sponsorship
Conflicts of interest.
There are no conflicts of interest.
Acknowledgement
The author thanks Dr. Hemant Deshmukh - Dean, Seth G.S. Medical College & KEM Hospital, for granting permission to publish this manuscript.
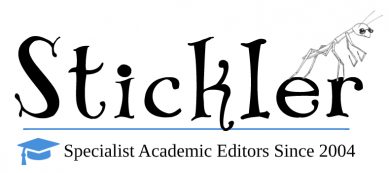
5 Quick Tips for Using Abbreviations and Acronyms in Academic Writing
Whether you’re working on a thesis or a research paper, abbreviations and acronyms are difficult to avoid in academic writing, which is why it’s important that you know how to use them properly. Here’s a quick primer.
1. Spell the word or phrase in full at first mention
When you first use the term that you will be abbreviating, you should usually spell out the actual word or phrase in full and follow that with the abbreviation/acronym in brackets.
For example: “We then conducted an analysis of variance (ANOVA).”
This ensures that your reader understands what your abbreviation means. While some abbreviations may seem common or obvious, different disciplines may use the same shorthand for different phrases. For example, “CAD” could refer to computer-aided design, coronary artery disease, Canadian dollar, current account deficit… You get the point! Abbreviations or acronyms that are extremely common for your audience may not need to be defined, but it’s best to check your style guidelines on this—and if you’re unsure, err on the side of caution and provide the full version at first mention.
2. Make sure abbreviations appear more than once
If you find that you have only used a specific abbreviation once in the entire paper, you should use the full version of the word or phrase instead and delete the abbreviation. This is because the purpose of abbreviations and acronyms is to make the writing more concise and aid comprehension, so—bearing in mind that it’s good practice to spell the abbreviated word or phrase in full at first mention—if it is only used once the abbreviation is redundant.
3. Use abbreviations consistently
Once you have introduced an abbreviation, it should be used consistently throughout the rest of the piece—that is, every time the word or phrase appears following its introduction. This means that once you have defined the abbreviation, you should stick to the same format and not switch back to the full word or a different abbreviation elsewhere in the paper. There are some exceptions to this rule; for example, the original text should be retained in direct quotations and references, and you may wish to use the full version in tables, figures, and section headings, depending on whether your style guide permits or even prescribes this.
4. Don’t go overboard
Abbreviations are a great way to keep writing concise. They can help you meet a tight word count, and enable the reader to focus on important information without getting bogged down by cumbersome phrases (for example, it’s much easier to process “RMSEA” than “root mean square error of approximation”!). However, a good writer will be prudent in their use of abbreviations; if a paper contains too many, that can be distracting and confusing in itself. Bear in mind also that similar constructions should be avoided. For example, if both “CAD” and “CDA” appear in your paper, it may be better to modify one of the abbreviations, or use the full version for one of the terms and do away with that abbreviation altogether.
5. Let us help!
Practice common sense, stick to the rules above, and, as always, feel free to have us check your work prior to submission to make sure your abbreviations are working with your paper rather than against it. Among our usual checks, we’ll ensure abbreviations are appropriate and consistent, and in line with your style guidelines.
- Academic Editing Services
- Theses and Dissertations
- Discounts for High-Volume Clients
- Submit Work and Get an Instant Quote
- Theses & Dissertations
- Translation
- Why We’re Different
- Work for Us
- Learn How to Become a Proofreader
- Academic Resources
- Submit Work & Contact
Thank you for visiting nature.com. You are using a browser version with limited support for CSS. To obtain the best experience, we recommend you use a more up to date browser (or turn off compatibility mode in Internet Explorer). In the meantime, to ensure continued support, we are displaying the site without styles and JavaScript.
- View all journals
- Explore content
- About the journal
- Publish with us
- Sign up for alerts
- Perspective
- Published: 06 March 2024
Artificial intelligence and illusions of understanding in scientific research
- Lisa Messeri ORCID: orcid.org/0000-0002-0964-123X 1 na1 &
- M. J. Crockett ORCID: orcid.org/0000-0001-8800-410X 2 , 3 na1
Nature volume 627 , pages 49–58 ( 2024 ) Cite this article
18k Accesses
3 Citations
697 Altmetric
Metrics details
- Human behaviour
- Interdisciplinary studies
- Research management
- Social anthropology
Scientists are enthusiastically imagining ways in which artificial intelligence (AI) tools might improve research. Why are AI tools so attractive and what are the risks of implementing them across the research pipeline? Here we develop a taxonomy of scientists’ visions for AI, observing that their appeal comes from promises to improve productivity and objectivity by overcoming human shortcomings. But proposed AI solutions can also exploit our cognitive limitations, making us vulnerable to illusions of understanding in which we believe we understand more about the world than we actually do. Such illusions obscure the scientific community’s ability to see the formation of scientific monocultures, in which some types of methods, questions and viewpoints come to dominate alternative approaches, making science less innovative and more vulnerable to errors. The proliferation of AI tools in science risks introducing a phase of scientific enquiry in which we produce more but understand less. By analysing the appeal of these tools, we provide a framework for advancing discussions of responsible knowledge production in the age of AI.
This is a preview of subscription content, access via your institution
Access options
Access Nature and 54 other Nature Portfolio journals
Get Nature+, our best-value online-access subscription
24,99 € / 30 days
cancel any time
Subscribe to this journal
Receive 51 print issues and online access
185,98 € per year
only 3,65 € per issue
Rent or buy this article
Prices vary by article type
Prices may be subject to local taxes which are calculated during checkout
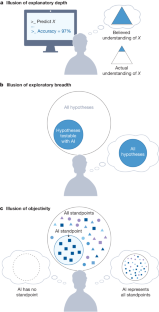
Similar content being viewed by others
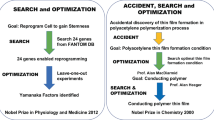
Nobel Turing Challenge: creating the engine for scientific discovery
Hiroaki Kitano
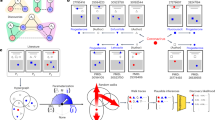
Accelerating science with human-aware artificial intelligence
Jamshid Sourati & James A. Evans
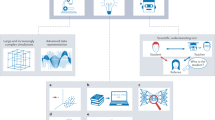
On scientific understanding with artificial intelligence
Mario Krenn, Robert Pollice, … Alán Aspuru-Guzik
Crabtree, G. Self-driving laboratories coming of age. Joule 4 , 2538–2541 (2020).
Article CAS Google Scholar
Wang, H. et al. Scientific discovery in the age of artificial intelligence. Nature 620 , 47–60 (2023). This review explores how AI can be incorporated across the research pipeline, drawing from a wide range of scientific disciplines .
Article CAS PubMed ADS Google Scholar
Dillion, D., Tandon, N., Gu, Y. & Gray, K. Can AI language models replace human participants? Trends Cogn. Sci. 27 , 597–600 (2023).
Article PubMed Google Scholar
Grossmann, I. et al. AI and the transformation of social science research. Science 380 , 1108–1109 (2023). This forward-looking article proposes a variety of ways to incorporate generative AI into social-sciences research .
Gil, Y. Will AI write scientific papers in the future? AI Mag. 42 , 3–15 (2022).
Google Scholar
Kitano, H. Nobel Turing Challenge: creating the engine for scientific discovery. npj Syst. Biol. Appl. 7 , 29 (2021).
Article PubMed PubMed Central Google Scholar
Benjamin, R. Race After Technology: Abolitionist Tools for the New Jim Code (Oxford Univ. Press, 2020). This book examines how social norms about race become embedded in technologies, even those that are focused on providing good societal outcomes .
Broussard, M. More Than a Glitch: Confronting Race, Gender, and Ability Bias in Tech (MIT Press, 2023).
Noble, S. U. Algorithms of Oppression: How Search Engines Reinforce Racism (New York Univ. Press, 2018).
Bender, E. M., Gebru, T., McMillan-Major, A. & Shmitchell, S. On the dangers of stochastic parrots: can language models be too big? in Proc. 2021 ACM Conference on Fairness, Accountability, and Transparency 610–623 (Association for Computing Machinery, 2021). One of the first comprehensive critiques of large language models, this article draws attention to a host of issues that ought to be considered before taking up such tools .
Crawford, K. Atlas of AI: Power, Politics, and the Planetary Costs of Artificial Intelligence (Yale Univ. Press, 2021).
Johnson, D. G. & Verdicchio, M. Reframing AI discourse. Minds Mach. 27 , 575–590 (2017).
Article Google Scholar
Atanasoski, N. & Vora, K. Surrogate Humanity: Race, Robots, and the Politics of Technological Futures (Duke Univ. Press, 2019).
Mitchell, M. & Krakauer, D. C. The debate over understanding in AI’s large language models. Proc. Natl Acad. Sci. USA 120 , e2215907120 (2023).
Kidd, C. & Birhane, A. How AI can distort human beliefs. Science 380 , 1222–1223 (2023).
Birhane, A., Kasirzadeh, A., Leslie, D. & Wachter, S. Science in the age of large language models. Nat. Rev. Phys. 5 , 277–280 (2023).
Kapoor, S. & Narayanan, A. Leakage and the reproducibility crisis in machine-learning-based science. Patterns 4 , 100804 (2023).
Hullman, J., Kapoor, S., Nanayakkara, P., Gelman, A. & Narayanan, A. The worst of both worlds: a comparative analysis of errors in learning from data in psychology and machine learning. In Proc. 2022 AAAI/ACM Conference on AI, Ethics, and Society (eds Conitzer, V. et al.) 335–348 (Association for Computing Machinery, 2022).
Rudin, C. Stop explaining black box machine learning models for high stakes decisions and use interpretable models instead. Nat. Mach. Intell. 1 , 206–215 (2019). This paper articulates the problems with attempting to explain AI systems that lack interpretability, and advocates for building interpretable models instead .
Crockett, M. J., Bai, X., Kapoor, S., Messeri, L. & Narayanan, A. The limitations of machine learning models for predicting scientific replicability. Proc. Natl Acad. Sci. USA 120 , e2307596120 (2023).
Article CAS PubMed PubMed Central Google Scholar
Lazar, S. & Nelson, A. AI safety on whose terms? Science 381 , 138 (2023).
Article PubMed ADS Google Scholar
Collingridge, D. The Social Control of Technology (St Martin’s Press, 1980).
Wagner, G., Lukyanenko, R. & Paré, G. Artificial intelligence and the conduct of literature reviews. J. Inf. Technol. 37 , 209–226 (2022).
Hutson, M. Artificial-intelligence tools aim to tame the coronavirus literature. Nature https://doi.org/10.1038/d41586-020-01733-7 (2020).
Haas, Q. et al. Utilizing artificial intelligence to manage COVID-19 scientific evidence torrent with Risklick AI: a critical tool for pharmacology and therapy development. Pharmacology 106 , 244–253 (2021).
Article CAS PubMed Google Scholar
Müller, H., Pachnanda, S., Pahl, F. & Rosenqvist, C. The application of artificial intelligence on different types of literature reviews – a comparative study. In 2022 International Conference on Applied Artificial Intelligence (ICAPAI) https://doi.org/10.1109/ICAPAI55158.2022.9801564 (Institute of Electrical and Electronics Engineers, 2022).
van Dinter, R., Tekinerdogan, B. & Catal, C. Automation of systematic literature reviews: a systematic literature review. Inf. Softw. Technol. 136 , 106589 (2021).
Aydın, Ö. & Karaarslan, E. OpenAI ChatGPT generated literature review: digital twin in healthcare. In Emerging Computer Technologies 2 (ed. Aydın, Ö.) 22–31 (İzmir Akademi Dernegi, 2022).
AlQuraishi, M. AlphaFold at CASP13. Bioinformatics 35 , 4862–4865 (2019).
Jumper, J. et al. Highly accurate protein structure prediction with AlphaFold. Nature 596 , 583–589 (2021).
Article CAS PubMed PubMed Central ADS Google Scholar
Lee, J. S., Kim, J. & Kim, P. M. Score-based generative modeling for de novo protein design. Nat. Computat. Sci. 3 , 382–392 (2023).
Gómez-Bombarelli, R. et al. Design of efficient molecular organic light-emitting diodes by a high-throughput virtual screening and experimental approach. Nat. Mater. 15 , 1120–1127 (2016).
Krenn, M. et al. On scientific understanding with artificial intelligence. Nat. Rev. Phys. 4 , 761–769 (2022).
Extance, A. How AI technology can tame the scientific literature. Nature 561 , 273–274 (2018).
Hastings, J. AI for Scientific Discovery (CRC Press, 2023). This book reviews current and future incorporation of AI into the scientific research pipeline .
Ahmed, A. et al. The future of academic publishing. Nat. Hum. Behav. 7 , 1021–1026 (2023).
Gray, K., Yam, K. C., Zhen’An, A. E., Wilbanks, D. & Waytz, A. The psychology of robots and artificial intelligence. In The Handbook of Social Psychology (eds Gilbert, D. et al.) (in the press).
Argyle, L. P. et al. Out of one, many: using language models to simulate human samples. Polit. Anal. 31 , 337–351 (2023).
Aher, G., Arriaga, R. I. & Kalai, A. T. Using large language models to simulate multiple humans and replicate human subject studies. In Proc. 40th International Conference on Machine Learning (eds Krause, A. et al.) 337–371 (JMLR.org, 2023).
Binz, M. & Schulz, E. Using cognitive psychology to understand GPT-3. Proc. Natl Acad. Sci. USA 120 , e2218523120 (2023).
Ornstein, J. T., Blasingame, E. N. & Truscott, J. S. How to train your stochastic parrot: large language models for political texts. Github , https://joeornstein.github.io/publications/ornstein-blasingame-truscott.pdf (2023).
He, S. et al. Learning to predict the cosmological structure formation. Proc. Natl Acad. Sci. USA 116 , 13825–13832 (2019).
Article MathSciNet CAS PubMed PubMed Central ADS Google Scholar
Mahmood, F. et al. Deep adversarial training for multi-organ nuclei segmentation in histopathology images. IEEE Trans. Med. Imaging 39 , 3257–3267 (2020).
Teixeira, B. et al. Generating synthetic X-ray images of a person from the surface geometry. In Proc. IEEE Conference on Computer Vision and Pattern Recognition 9059–9067 (Institute of Electrical and Electronics Engineers, 2018).
Marouf, M. et al. Realistic in silico generation and augmentation of single-cell RNA-seq data using generative adversarial networks. Nat. Commun. 11 , 166 (2020).
Watts, D. J. A twenty-first century science. Nature 445 , 489 (2007).
boyd, d. & Crawford, K. Critical questions for big data. Inf. Commun. Soc. 15 , 662–679 (2012). This article assesses the ethical and epistemic implications of scientific and societal moves towards big data and provides a parallel case study for thinking about the risks of artificial intelligence .
Jolly, E. & Chang, L. J. The Flatland fallacy: moving beyond low–dimensional thinking. Top. Cogn. Sci. 11 , 433–454 (2019).
Yarkoni, T. & Westfall, J. Choosing prediction over explanation in psychology: lessons from machine learning. Perspect. Psychol. Sci. 12 , 1100–1122 (2017).
Radivojac, P. et al. A large-scale evaluation of computational protein function prediction. Nat. Methods 10 , 221–227 (2013).
Bileschi, M. L. et al. Using deep learning to annotate the protein universe. Nat. Biotechnol. 40 , 932–937 (2022).
Barkas, N. et al. Joint analysis of heterogeneous single-cell RNA-seq dataset collections. Nat. Methods 16 , 695–698 (2019).
Demszky, D. et al. Using large language models in psychology. Nat. Rev. Psychol. 2 , 688–701 (2023).
Karjus, A. Machine-assisted mixed methods: augmenting humanities and social sciences with artificial intelligence. Preprint at https://arxiv.org/abs/2309.14379 (2023).
Davies, A. et al. Advancing mathematics by guiding human intuition with AI. Nature 600 , 70–74 (2021).
Peterson, J. C., Bourgin, D. D., Agrawal, M., Reichman, D. & Griffiths, T. L. Using large-scale experiments and machine learning to discover theories of human decision-making. Science 372 , 1209–1214 (2021).
Ilyas, A. et al. Adversarial examples are not bugs, they are features. Preprint at https://doi.org/10.48550/arXiv.1905.02175 (2019)
Semel, B. M. Listening like a computer: attentional tensions and mechanized care in psychiatric digital phenotyping. Sci. Technol. Hum. Values 47 , 266–290 (2022).
Gil, Y. Thoughtful artificial intelligence: forging a new partnership for data science and scientific discovery. Data Sci. 1 , 119–129 (2017).
Checco, A., Bracciale, L., Loreti, P., Pinfield, S. & Bianchi, G. AI-assisted peer review. Humanit. Soc. Sci. Commun. 8 , 25 (2021).
Thelwall, M. Can the quality of published academic journal articles be assessed with machine learning? Quant. Sci. Stud. 3 , 208–226 (2022).
Dhar, P. Peer review of scholarly research gets an AI boost. IEEE Spectrum spectrum.ieee.org/peer-review-of-scholarly-research-gets-an-ai-boost (2020).
Heaven, D. AI peer reviewers unleashed to ease publishing grind. Nature 563 , 609–610 (2018).
Conroy, G. How ChatGPT and other AI tools could disrupt scientific publishing. Nature 622 , 234–236 (2023).
Nosek, B. A. et al. Replicability, robustness, and reproducibility in psychological science. Annu. Rev. Psychol. 73 , 719–748 (2022).
Altmejd, A. et al. Predicting the replicability of social science lab experiments. PLoS ONE 14 , e0225826 (2019).
Yang, Y., Youyou, W. & Uzzi, B. Estimating the deep replicability of scientific findings using human and artificial intelligence. Proc. Natl Acad. Sci. USA 117 , 10762–10768 (2020).
Youyou, W., Yang, Y. & Uzzi, B. A discipline-wide investigation of the replicability of psychology papers over the past two decades. Proc. Natl Acad. Sci. USA 120 , e2208863120 (2023).
Rabb, N., Fernbach, P. M. & Sloman, S. A. Individual representation in a community of knowledge. Trends Cogn. Sci. 23 , 891–902 (2019). This comprehensive review paper documents the empirical evidence for distributed cognition in communities of knowledge and the resultant vulnerabilities to illusions of understanding .
Rozenblit, L. & Keil, F. The misunderstood limits of folk science: an illusion of explanatory depth. Cogn. Sci. 26 , 521–562 (2002). This paper provided an empirical demonstration of the illusion of explanatory depth, and inspired a programme of research in cognitive science on communities of knowledge .
Hutchins, E. Cognition in the Wild (MIT Press, 1995).
Lave, J. & Wenger, E. Situated Learning: Legitimate Peripheral Participation (Cambridge Univ. Press, 1991).
Kitcher, P. The division of cognitive labor. J. Philos. 87 , 5–22 (1990).
Hardwig, J. Epistemic dependence. J. Philos. 82 , 335–349 (1985).
Keil, F. in Oxford Studies In Epistemology (eds Gendler, T. S. & Hawthorne, J.) 143–166 (Oxford Academic, 2005).
Weisberg, M. & Muldoon, R. Epistemic landscapes and the division of cognitive labor. Philos. Sci. 76 , 225–252 (2009).
Sloman, S. A. & Rabb, N. Your understanding is my understanding: evidence for a community of knowledge. Psychol. Sci. 27 , 1451–1460 (2016).
Wilson, R. A. & Keil, F. The shadows and shallows of explanation. Minds Mach. 8 , 137–159 (1998).
Keil, F. C., Stein, C., Webb, L., Billings, V. D. & Rozenblit, L. Discerning the division of cognitive labor: an emerging understanding of how knowledge is clustered in other minds. Cogn. Sci. 32 , 259–300 (2008).
Sperber, D. et al. Epistemic vigilance. Mind Lang. 25 , 359–393 (2010).
Wilkenfeld, D. A., Plunkett, D. & Lombrozo, T. Depth and deference: when and why we attribute understanding. Philos. Stud. 173 , 373–393 (2016).
Sparrow, B., Liu, J. & Wegner, D. M. Google effects on memory: cognitive consequences of having information at our fingertips. Science 333 , 776–778 (2011).
Fisher, M., Goddu, M. K. & Keil, F. C. Searching for explanations: how the internet inflates estimates of internal knowledge. J. Exp. Psychol. Gen. 144 , 674–687 (2015).
De Freitas, J., Agarwal, S., Schmitt, B. & Haslam, N. Psychological factors underlying attitudes toward AI tools. Nat. Hum. Behav. 7 , 1845–1854 (2023).
Castelo, N., Bos, M. W. & Lehmann, D. R. Task-dependent algorithm aversion. J. Mark. Res. 56 , 809–825 (2019).
Cadario, R., Longoni, C. & Morewedge, C. K. Understanding, explaining, and utilizing medical artificial intelligence. Nat. Hum. Behav. 5 , 1636–1642 (2021).
Oktar, K. & Lombrozo, T. Deciding to be authentic: intuition is favored over deliberation when authenticity matters. Cognition 223 , 105021 (2022).
Bigman, Y. E., Yam, K. C., Marciano, D., Reynolds, S. J. & Gray, K. Threat of racial and economic inequality increases preference for algorithm decision-making. Comput. Hum. Behav. 122 , 106859 (2021).
Claudy, M. C., Aquino, K. & Graso, M. Artificial intelligence can’t be charmed: the effects of impartiality on laypeople’s algorithmic preferences. Front. Psychol. 13 , 898027 (2022).
Snyder, C., Keppler, S. & Leider, S. Algorithm reliance under pressure: the effect of customer load on service workers. Preprint at SSRN https://doi.org/10.2139/ssrn.4066823 (2022).
Bogert, E., Schecter, A. & Watson, R. T. Humans rely more on algorithms than social influence as a task becomes more difficult. Sci Rep. 11 , 8028 (2021).
Raviv, A., Bar‐Tal, D., Raviv, A. & Abin, R. Measuring epistemic authority: studies of politicians and professors. Eur. J. Personal. 7 , 119–138 (1993).
Cummings, L. The “trust” heuristic: arguments from authority in public health. Health Commun. 29 , 1043–1056 (2014).
Lee, M. K. Understanding perception of algorithmic decisions: fairness, trust, and emotion in response to algorithmic management. Big Data Soc. 5 , https://doi.org/10.1177/2053951718756684 (2018).
Kissinger, H. A., Schmidt, E. & Huttenlocher, D. The Age of A.I. And Our Human Future (Little, Brown, 2021).
Lombrozo, T. Explanatory preferences shape learning and inference. Trends Cogn. Sci. 20 , 748–759 (2016). This paper provides an overview of philosophical theories of explanatory virtues and reviews empirical evidence on the sorts of explanations people find satisfying .
Vrantsidis, T. H. & Lombrozo, T. Simplicity as a cue to probability: multiple roles for simplicity in evaluating explanations. Cogn. Sci. 46 , e13169 (2022).
Johnson, S. G. B., Johnston, A. M., Toig, A. E. & Keil, F. C. Explanatory scope informs causal strength inferences. In Proc. 36th Annual Meeting of the Cognitive Science Society 2453–2458 (Cognitive Science Society, 2014).
Khemlani, S. S., Sussman, A. B. & Oppenheimer, D. M. Harry Potter and the sorcerer’s scope: latent scope biases in explanatory reasoning. Mem. Cognit. 39 , 527–535 (2011).
Liquin, E. G. & Lombrozo, T. Motivated to learn: an account of explanatory satisfaction. Cogn. Psychol. 132 , 101453 (2022).
Hopkins, E. J., Weisberg, D. S. & Taylor, J. C. V. The seductive allure is a reductive allure: people prefer scientific explanations that contain logically irrelevant reductive information. Cognition 155 , 67–76 (2016).
Weisberg, D. S., Hopkins, E. J. & Taylor, J. C. V. People’s explanatory preferences for scientific phenomena. Cogn. Res. Princ. Implic. 3 , 44 (2018).
Jerez-Fernandez, A., Angulo, A. N. & Oppenheimer, D. M. Show me the numbers: precision as a cue to others’ confidence. Psychol. Sci. 25 , 633–635 (2014).
Kim, J., Giroux, M. & Lee, J. C. When do you trust AI? The effect of number presentation detail on consumer trust and acceptance of AI recommendations. Psychol. Mark. 38 , 1140–1155 (2021).
Nguyen, C. T. The seductions of clarity. R. Inst. Philos. Suppl. 89 , 227–255 (2021). This article describes how reductive and quantitative explanations can generate a sense of understanding that is not necessarily correlated with actual understanding .
Fisher, M., Smiley, A. H. & Grillo, T. L. H. Information without knowledge: the effects of internet search on learning. Memory 30 , 375–387 (2022).
Eliseev, E. D. & Marsh, E. J. Understanding why searching the internet inflates confidence in explanatory ability. Appl. Cogn. Psychol. 37 , 711–720 (2023).
Fisher, M. & Oppenheimer, D. M. Who knows what? Knowledge misattribution in the division of cognitive labor. J. Exp. Psychol. Appl. 27 , 292–306 (2021).
Chromik, M., Eiband, M., Buchner, F., Krüger, A. & Butz, A. I think I get your point, AI! The illusion of explanatory depth in explainable AI. In 26th International Conference on Intelligent User Interfaces (eds Hammond, T. et al.) 307–317 (Association for Computing Machinery, 2021).
Strevens, M. No understanding without explanation. Stud. Hist. Philos. Sci. A 44 , 510–515 (2013).
Ylikoski, P. in Scientific Understanding: Philosophical Perspectives (eds De Regt, H. et al.) 100–119 (Univ. Pittsburgh Press, 2009).
Giudice, M. D. The prediction–explanation fallacy: a pervasive problem in scientific applications of machine learning. Preprint at PsyArXiv https://doi.org/10.31234/osf.io/4vq8f (2021).
Hofman, J. M. et al. Integrating explanation and prediction in computational social science. Nature 595 , 181–188 (2021). This paper highlights the advantages and disadvantages of explanatory versus predictive approaches to modelling, with a focus on applications to computational social science .
Shmueli, G. To explain or to predict? Stat. Sci. 25 , 289–310 (2010).
Article MathSciNet Google Scholar
Hofman, J. M., Sharma, A. & Watts, D. J. Prediction and explanation in social systems. Science 355 , 486–488 (2017).
Logg, J. M., Minson, J. A. & Moore, D. A. Algorithm appreciation: people prefer algorithmic to human judgment. Organ. Behav. Hum. Decis. Process. 151 , 90–103 (2019).
Nguyen, C. T. Cognitive islands and runaway echo chambers: problems for epistemic dependence on experts. Synthese 197 , 2803–2821 (2020).
Breiman, L. Statistical modeling: the two cultures. Stat. Sci. 16 , 199–215 (2001).
Gao, J. & Wang, D. Quantifying the benefit of artificial intelligence for scientific research. Preprint at arxiv.org/abs/2304.10578 (2023).
Hanson, B. et al. Garbage in, garbage out: mitigating risks and maximizing benefits of AI in research. Nature 623 , 28–31 (2023).
Kleinberg, J. & Raghavan, M. Algorithmic monoculture and social welfare. Proc. Natl Acad. Sci. USA 118 , e2018340118 (2021). This paper uses formal modelling methods to demonstrate that when companies all rely on the same algorithm to make decisions (an algorithmic monoculture), the overall quality of those decisions is reduced because valuable options can slip through the cracks, even when the algorithm performs accurately for individual companies .
Article MathSciNet CAS PubMed PubMed Central Google Scholar
Hofstra, B. et al. The diversity–innovation paradox in science. Proc. Natl Acad. Sci. USA 117 , 9284–9291 (2020).
Hong, L. & Page, S. E. Groups of diverse problem solvers can outperform groups of high-ability problem solvers. Proc. Natl Acad. Sci. USA 101 , 16385–16389 (2004).
Page, S. E. Where diversity comes from and why it matters? Eur. J. Soc. Psychol. 44 , 267–279 (2014). This article reviews research demonstrating the benefits of cognitive diversity and diversity in methodological approaches for problem solving and innovation .
Clarke, A. E. & Fujimura, J. H. (eds) The Right Tools for the Job: At Work in Twentieth-Century Life Sciences (Princeton Univ. Press, 2014).
Silva, V. J., Bonacelli, M. B. M. & Pacheco, C. A. Framing the effects of machine learning on science. AI Soc. https://doi.org/10.1007/s00146-022-01515-x (2022).
Sassenberg, K. & Ditrich, L. Research in social psychology changed between 2011 and 2016: larger sample sizes, more self-report measures, and more online studies. Adv. Methods Pract. Psychol. Sci. 2 , 107–114 (2019).
Simon, A. F. & Wilder, D. Methods and measures in social and personality psychology: a comparison of JPSP publications in 1982 and 2016. J. Soc. Psychol. https://doi.org/10.1080/00224545.2022.2135088 (2022).
Anderson, C. A. et al. The MTurkification of social and personality psychology. Pers. Soc. Psychol. Bull. 45 , 842–850 (2019).
Latour, B. in The Social After Gabriel Tarde: Debates and Assessments (ed. Candea, M.) 145–162 (Routledge, 2010).
Porter, T. M. Trust in Numbers: The Pursuit of Objectivity in Science and Public Life (Princeton Univ. Press, 1996).
Lazer, D. et al. Meaningful measures of human society in the twenty-first century. Nature 595 , 189–196 (2021).
Knox, D., Lucas, C. & Cho, W. K. T. Testing causal theories with learned proxies. Annu. Rev. Polit. Sci. 25 , 419–441 (2022).
Barberá, P. Birds of the same feather tweet together: Bayesian ideal point estimation using Twitter data. Polit. Anal. 23 , 76–91 (2015).
Brady, W. J., McLoughlin, K., Doan, T. N. & Crockett, M. J. How social learning amplifies moral outrage expression in online social networks. Sci. Adv. 7 , eabe5641 (2021).
Article PubMed PubMed Central ADS Google Scholar
Barnes, J., Klinger, R. & im Walde, S. S. Assessing state-of-the-art sentiment models on state-of-the-art sentiment datasets. In Proc. 8th Workshop on Computational Approaches to Subjectivity, Sentiment and Social Media Analysis (eds Balahur, A. et al.) 2–12 (Association for Computational Linguistics, 2017).
Gitelman, L. (ed.) “Raw Data” is an Oxymoron (MIT Press, 2013).
Breznau, N. et al. Observing many researchers using the same data and hypothesis reveals a hidden universe of uncertainty. Proc. Natl Acad. Sci. USA 119 , e2203150119 (2022). This study demonstrates how 73 research teams analysing the same dataset reached different conclusions about the relationship between immigration and public support for social policies, highlighting the subjectivity and uncertainty involved in analysing complex datasets .
Gillespie, T. in Media Technologies: Essays on Communication, Materiality, and Society (eds Gillespie, T. et al.) 167–194 (MIT Press, 2014).
Leonelli, S. Data-Centric Biology: A Philosophical Study (Univ. Chicago Press, 2016).
Wang, A., Kapoor, S., Barocas, S. & Narayanan, A. Against predictive optimization: on the legitimacy of decision-making algorithms that optimize predictive accuracy. ACM J. Responsib. Comput. , https://doi.org/10.1145/3636509 (2023).
Athey, S. Beyond prediction: using big data for policy problems. Science 355 , 483–485 (2017).
del Rosario Martínez-Ordaz, R. Scientific understanding through big data: from ignorance to insights to understanding. Possibility Stud. Soc. 1 , 279–299 (2023).
Nussberger, A.-M., Luo, L., Celis, L. E. & Crockett, M. J. Public attitudes value interpretability but prioritize accuracy in artificial intelligence. Nat. Commun. 13 , 5821 (2022).
Zittrain, J. in The Cambridge Handbook of Responsible Artificial Intelligence: Interdisciplinary Perspectives (eds. Voeneky, S. et al.) 176–184 (Cambridge Univ. Press, 2022). This article articulates the epistemic risks of prioritizing predictive accuracy over explanatory understanding when AI tools are interacting in complex systems.
Shumailov, I. et al. The curse of recursion: training on generated data makes models forget. Preprint at arxiv.org/abs/2305.17493 (2023).
Latour, B. Science In Action: How to Follow Scientists and Engineers Through Society (Harvard Univ. Press, 1987). This book provides strategies and approaches for thinking about science as a social endeavour .
Franklin, S. Science as culture, cultures of science. Annu. Rev. Anthropol. 24 , 163–184 (1995).
Haraway, D. Situated knowledges: the science question in feminism and the privilege of partial perspective. Fem. Stud. 14 , 575–599 (1988). This article acknowledges that the objective ‘view from nowhere’ is unobtainable: knowledge, it argues, is always situated .
Harding, S. Objectivity and Diversity: Another Logic of Scientific Research (Univ. Chicago Press, 2015).
Longino, H. E. Science as Social Knowledge: Values and Objectivity in Scientific Inquiry (Princeton Univ. Press, 1990).
Daston, L. & Galison, P. Objectivity (Princeton Univ. Press, 2007). This book is a historical analysis of the shifting modes of ‘objectivity’ that scientists have pursued, arguing that objectivity is not a universal concept but that it shifts alongside scientific techniques and ambitions .
Prescod-Weinstein, C. Making Black women scientists under white empiricism: the racialization of epistemology in physics. Signs J. Women Cult. Soc. 45 , 421–447 (2020).
Mavhunga, C. What Do Science, Technology, and Innovation Mean From Africa? (MIT Press, 2017).
Schiebinger, L. The Mind Has No Sex? Women in the Origins of Modern Science (Harvard Univ. Press, 1991).
Martin, E. The egg and the sperm: how science has constructed a romance based on stereotypical male–female roles. Signs J. Women Cult. Soc. 16 , 485–501 (1991). This case study shows how assumptions about gender affect scientific theories, sometimes delaying the articulation of what might be considered to be more accurate descriptions of scientific phenomena .
Harding, S. Rethinking standpoint epistemology: What is “strong objectivity”? Centen. Rev. 36 , 437–470 (1992). In this article, Harding outlines her position on ‘strong objectivity’, by which clearly articulating one’s standpoint can lead to more robust knowledge claims .
Oreskes, N. Why Trust Science? (Princeton Univ. Press, 2019). This book introduces the reader to 20 years of scholarship in science and technology studies, arguing that the tools the discipline has for understanding science can help to reinstate public trust in the institution .
Rolin, K., Koskinen, I., Kuorikoski, J. & Reijula, S. Social and cognitive diversity in science: introduction. Synthese 202 , 36 (2023).
Hong, L. & Page, S. E. Problem solving by heterogeneous agents. J. Econ. Theory 97 , 123–163 (2001).
Sulik, J., Bahrami, B. & Deroy, O. The diversity gap: when diversity matters for knowledge. Perspect. Psychol. Sci. 17 , 752–767 (2022).
Lungeanu, A., Whalen, R., Wu, Y. J., DeChurch, L. A. & Contractor, N. S. Diversity, networks, and innovation: a text analytic approach to measuring expertise diversity. Netw. Sci. 11 , 36–64 (2023).
AlShebli, B. K., Rahwan, T. & Woon, W. L. The preeminence of ethnic diversity in scientific collaboration. Nat. Commun. 9 , 5163 (2018).
Campbell, L. G., Mehtani, S., Dozier, M. E. & Rinehart, J. Gender-heterogeneous working groups produce higher quality science. PLoS ONE 8 , e79147 (2013).
Nielsen, M. W., Bloch, C. W. & Schiebinger, L. Making gender diversity work for scientific discovery and innovation. Nat. Hum. Behav. 2 , 726–734 (2018).
Yang, Y., Tian, T. Y., Woodruff, T. K., Jones, B. F. & Uzzi, B. Gender-diverse teams produce more novel and higher-impact scientific ideas. Proc. Natl Acad. Sci. USA 119 , e2200841119 (2022).
Kozlowski, D., Larivière, V., Sugimoto, C. R. & Monroe-White, T. Intersectional inequalities in science. Proc. Natl Acad. Sci. USA 119 , e2113067119 (2022).
Fehr, C. & Jones, J. M. Culture, exploitation, and epistemic approaches to diversity. Synthese 200 , 465 (2022).
Nakadai, R., Nakawake, Y. & Shibasaki, S. AI language tools risk scientific diversity and innovation. Nat. Hum. Behav. 7 , 1804–1805 (2023).
National Academies of Sciences, Engineering, and Medicine et al. Advancing Antiracism, Diversity, Equity, and Inclusion in STEMM Organizations: Beyond Broadening Participation (National Academies Press, 2023).
Winner, L. Do artifacts have politics? Daedalus 109 , 121–136 (1980).
Eubanks, V. Automating Inequality: How High-Tech Tools Profile, Police, and Punish the Poor (St. Martin’s Press, 2018).
Littmann, M. et al. Validity of machine learning in biology and medicine increased through collaborations across fields of expertise. Nat. Mach. Intell. 2 , 18–24 (2020).
Carusi, A. et al. Medical artificial intelligence is as much social as it is technological. Nat. Mach. Intell. 5 , 98–100 (2023).
Raghu, M. & Schmidt, E. A survey of deep learning for scientific discovery. Preprint at arxiv.org/abs/2003.11755 (2020).
Bishop, C. AI4Science to empower the fifth paradigm of scientific discovery. Microsoft Research Blog www.microsoft.com/en-us/research/blog/ai4science-to-empower-the-fifth-paradigm-of-scientific-discovery/ (2022).
Whittaker, M. The steep cost of capture. Interactions 28 , 50–55 (2021).
Liesenfeld, A., Lopez, A. & Dingemanse, M. Opening up ChatGPT: Tracking openness, transparency, and accountability in instruction-tuned text generators. In Proc. 5th International Conference on Conversational User Interfaces 1–6 (Association for Computing Machinery, 2023).
Chu, J. S. G. & Evans, J. A. Slowed canonical progress in large fields of science. Proc. Natl Acad. Sci. USA 118 , e2021636118 (2021).
Park, M., Leahey, E. & Funk, R. J. Papers and patents are becoming less disruptive over time. Nature 613 , 138–144 (2023).
Frith, U. Fast lane to slow science. Trends Cogn. Sci. 24 , 1–2 (2020). This article explains the epistemic risks of a hyperfocus on scientific productivity and explores possible avenues for incentivizing the production of higher-quality science on a slower timescale .
Stengers, I. Another Science is Possible: A Manifesto for Slow Science (Wiley, 2018).
Lake, B. M. & Baroni, M. Human-like systematic generalization through a meta-learning neural network. Nature 623 , 115–121 (2023).
Feinman, R. & Lake, B. M. Learning task-general representations with generative neuro-symbolic modeling. Preprint at arxiv.org/abs/2006.14448 (2021).
Schölkopf, B. et al. Toward causal representation learning. Proc. IEEE 109 , 612–634 (2021).
Mitchell, M. AI’s challenge of understanding the world. Science 382 , eadm8175 (2023).
Sartori, L. & Bocca, G. Minding the gap(s): public perceptions of AI and socio-technical imaginaries. AI Soc. 38 , 443–458 (2023).
Download references
Acknowledgements
We thank D. S. Bassett, W. J. Brady, S. Helmreich, S. Kapoor, T. Lombrozo, A. Narayanan, M. Salganik and A. J. te Velthuis for comments. We also thank C. Buckner and P. Winter for their feedback and suggestions.
Author information
These authors contributed equally: Lisa Messeri, M. J. Crockett
Authors and Affiliations
Department of Anthropology, Yale University, New Haven, CT, USA
Lisa Messeri
Department of Psychology, Princeton University, Princeton, NJ, USA
M. J. Crockett
University Center for Human Values, Princeton University, Princeton, NJ, USA
You can also search for this author in PubMed Google Scholar
Contributions
The authors contributed equally to the research and writing of the paper.
Corresponding authors
Correspondence to Lisa Messeri or M. J. Crockett .
Ethics declarations
Competing interests.
The authors declare no competing interests.
Peer review
Peer review information.
Nature thanks Cameron Buckner, Peter Winter and the other, anonymous, reviewer(s) for their contribution to the peer review of this work.
Additional information
Publisher’s note Springer Nature remains neutral with regard to jurisdictional claims in published maps and institutional affiliations.
Rights and permissions
Springer Nature or its licensor (e.g. a society or other partner) holds exclusive rights to this article under a publishing agreement with the author(s) or other rightsholder(s); author self-archiving of the accepted manuscript version of this article is solely governed by the terms of such publishing agreement and applicable law.
Reprints and permissions
About this article
Cite this article.
Messeri, L., Crockett, M.J. Artificial intelligence and illusions of understanding in scientific research. Nature 627 , 49–58 (2024). https://doi.org/10.1038/s41586-024-07146-0
Download citation
Received : 31 July 2023
Accepted : 31 January 2024
Published : 06 March 2024
Issue Date : 07 March 2024
DOI : https://doi.org/10.1038/s41586-024-07146-0
Share this article
Anyone you share the following link with will be able to read this content:
Sorry, a shareable link is not currently available for this article.
Provided by the Springer Nature SharedIt content-sharing initiative
This article is cited by
Ai is no substitute for having something to say.
Nature Reviews Physics (2024)
Perché gli scienziati si fidano troppo dell'intelligenza artificiale - e come rimediare
Nature Italy (2024)
Why scientists trust AI too much — and what to do about it
Nature (2024)
By submitting a comment you agree to abide by our Terms and Community Guidelines . If you find something abusive or that does not comply with our terms or guidelines please flag it as inappropriate.
Quick links
- Explore articles by subject
- Guide to authors
- Editorial policies
Sign up for the Nature Briefing newsletter — what matters in science, free to your inbox daily.


- SUGGESTED TOPICS
- The Magazine
- Newsletters
- Managing Yourself
- Managing Teams
- Work-life Balance
- The Big Idea
- Data & Visuals
- Reading Lists
- Case Selections
- HBR Learning
- Topic Feeds
- Account Settings
- Email Preferences
Research Roundup: How the Pandemic Changed Management
- Mark C. Bolino,
- Jacob M. Whitney,
- Sarah E. Henry

Lessons from 69 articles published in top management and applied psychology journals.
Researchers recently reviewed 69 articles focused on the management implications of the Covid-19 pandemic that were published between March 2020 and July 2023 in top journals in management and applied psychology. The review highlights the numerous ways in which employees, teams, leaders, organizations, and societies were impacted and offers lessons for managing through future pandemics or other events of mass disruption.
The recent pandemic disrupted life as we know it, including for employees and organizations around the world. To understand such changes, we recently reviewed 69 articles focused on the management implications of the Covid-19 pandemic. These papers were published between March 2020 and July 2023 in top journals in management and applied psychology.
- Mark C. Bolino is the David L. Boren Professor and the Michael F. Price Chair in International Business at the University of Oklahoma’s Price College of Business. His research focuses on understanding how an organization can inspire its employees to go the extra mile without compromising their personal well-being.
- JW Jacob M. Whitney is a doctoral candidate in management at the University of Oklahoma’s Price College of Business and an incoming assistant professor at Kennesaw State University. His research interests include leadership, teams, and organizational citizenship behavior.
- SH Sarah E. Henry is a doctoral candidate in management at the University of Oklahoma’s Price College of Business and an incoming assistant professor at the University of South Florida. Her research interests include organizational citizenship behaviors, workplace interpersonal dynamics, and international management.
Partner Center
Search form
Doing more, but learning less: the risks of ai in research.

(© stock.adobe.com)
Artificial intelligence (AI) is widely heralded for its potential to enhance productivity in scientific research. But with that promise come risks that could narrow scientists’ ability to better understand the world, according to a new paper co-authored by a Yale anthropologist.
Some future AI approaches, the authors argue, could constrict the questions researchers ask, the experiments they perform, and the perspectives that come to bear on scientific data and theories.
All told, these factors could leave people vulnerable to “illusions of understanding” in which they believe they comprehend the world better than they do.
The paper published March 7 in Nature .
“ There is a risk that scientists will use AI to produce more while understanding less,” said co-author Lisa Messeri, an anthropologist in Yale’s Faculty of Arts and Sciences. “We’re not arguing that scientists shouldn’t use AI tools, but we’re advocating for a conversation about how scientists will use them and suggesting that we shouldn’t automatically assume that all uses of the technology, or the ubiquitous use of it, will benefit science.”
The paper, co-authored by Princeton cognitive scientist M. J. Crockett, sets a framework for discussing the risks involved in using AI tools throughout the scientific research process, from study design through peer review.
“ We hope this paper offers a vocabulary for talking about AI’s potential epistemic risks,” Messeri said.
Added Crockett: “To understand these risks, scientists can benefit from work in the humanities and qualitative social sciences.”
Messeri and Crockett classified proposed visions of AI spanning the scientific process that are currently creating buzz among researchers into four archetypes:
- In study design, they argue, “AI as Oracle” tools are imagined as being able to objectively and efficiently search, evaluate, and summarize massive scientific literatures, helping researchers to formulate questions in their project’s design stage.
- In data collection, “AI as Surrogate” applications, it is hoped, allow scientists to generate accurate stand-in data points, including as a replacement for human study participants, when data is otherwise too difficult or expensive to obtain.
- In data analysis, “AI as Quant” tools seek to surpass the human intellect’s ability to analyze vast and complex datasets.
- And “AI as Arbiter” applications aim to objectively evaluate scientific studies for merit and replicability, thereby replacing humans in the peer-review process.
The authors warn against treating AI applications from these four archetypes as trusted partners, rather than simply tools , in the production of scientific knowledge. Doing so, they say, could make scientists susceptible to illusions of understanding, which can crimp their perspectives and convince them that they know more than they do.
The efficiencies and insights that AI tools promise can weaken the production of scientific knowledge by creating “monocultures of knowing,” in which researchers prioritize the questions and methods best suited to AI over other modes of inquiry, Messeri and Crockett state. A scholarly environment of that kind leaves researchers vulnerable to what they call “illusions of exploratory breadth,” where scientists wrongly believe that they are exploring all testable hypotheses, when they are only examining the narrower range of questions that can be tested through AI.
For example, “Surrogate” AI tools that seem to accurately mimic human survey responses could make experiments that require measurements of physical behavior or face-to-face interactions increasingly unpopular because they are slower and more expensive to conduct, Crockett said.
The authors also describe the possibility that AI tools become viewed as more objective and reliable than human scientists, creating a “monoculture of knowers” in which AI systems are treated as a singular, authoritative, and objective knower in place of a diverse scientific community of scientists with varied backgrounds, training, and expertise. A monoculture, they say, invites “illusions of objectivity” where scientists falsely believe that AI tools have no perspective or represent all perspectives when, in truth, they represent the standpoints of the computer scientists who developed and trained them.
“ There is a belief around science that the objective observer is the ideal creator of knowledge about the world,” Messeri said. “But this is a myth. There has never been an objective ‘knower,’ there can never be one, and continuing to pursue this myth only weakens science.”
There is substantial evidence that human diversity makes science more robust and creative, the authors add.
“ Acknowledging that science is a social practice that benefits from including diverse standpoints will help us realize its full potential,” Crockett said. “Replacing diverse standpoints with AI tools will set back the clock on the progress we’ve made toward including more perspectives in scientific work.”
It is important to remember AI’s social implications, which extend far beyond the laboratories where it is being used in research, Messeri said.
“ We train scientists to think about technical aspects of new technology,” she said. “We don’t train them nearly as well to consider the social aspects, which is vital to future work in this domain.”
Science & Technology
Social Sciences
Media Contact
Bess Connolly : [email protected] ,

Why is fentanyl driving overdose deaths?

Understanding the wiring of the human genome

Connecticut Supreme Court sides with Yale law clinic in police records case

Stewart draws on days in Parliament, and Afghan villages, in new Yale role
- Show More Articles
Suggestions or feedback?
MIT News | Massachusetts Institute of Technology
- Machine learning
- Social justice
- Black holes
- Classes and programs
Departments
- Aeronautics and Astronautics
- Brain and Cognitive Sciences
- Architecture
- Political Science
- Mechanical Engineering
Centers, Labs, & Programs
- Abdul Latif Jameel Poverty Action Lab (J-PAL)
- Picower Institute for Learning and Memory
- Lincoln Laboratory
- School of Architecture + Planning
- School of Engineering
- School of Humanities, Arts, and Social Sciences
- Sloan School of Management
- School of Science
- MIT Schwarzman College of Computing
A noninvasive treatment for “chemo brain”
Press contact :, media download.
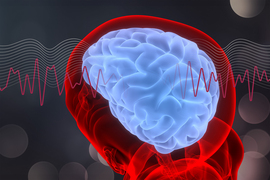
*Terms of Use:
Images for download on the MIT News office website are made available to non-commercial entities, press and the general public under a Creative Commons Attribution Non-Commercial No Derivatives license . You may not alter the images provided, other than to crop them to size. A credit line must be used when reproducing images; if one is not provided below, credit the images to "MIT."
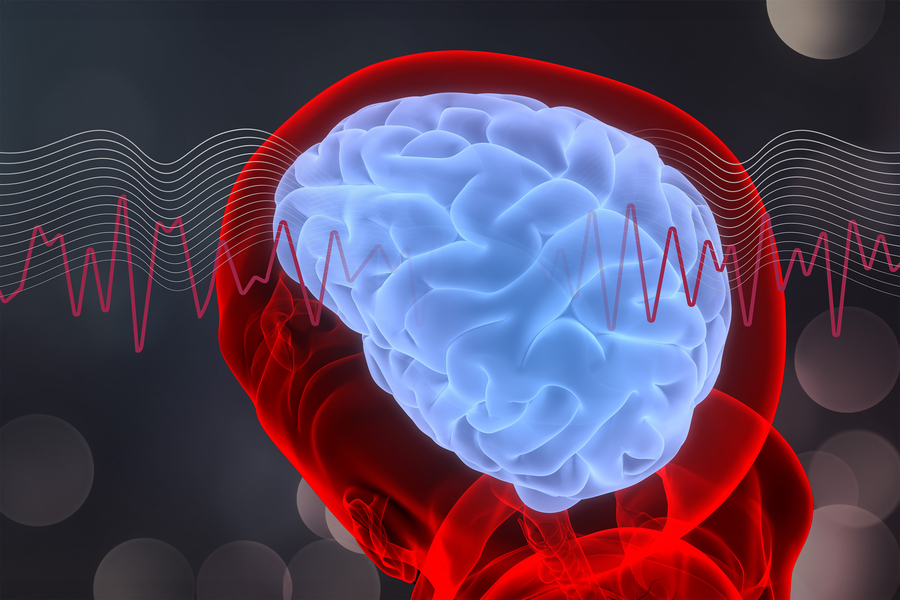
Previous image Next image
Patients undergoing chemotherapy often experience cognitive effects such as memory impairment and difficulty concentrating — a condition commonly known as “chemo brain.”
MIT researchers have now shown that a noninvasive treatment that stimulates gamma frequency brain waves may hold promise for treating chemo brain. In a study of mice, they found that daily exposure to light and sound with a frequency of 40 hertz protected brain cells from chemotherapy-induced damage. The treatment also helped to prevent memory loss and impairment of other cognitive functions.
This treatment, which was originally developed as a way to treat Alzheimer’s disease, appears to have widespread effects that could help with a variety of neurological disorders, the researchers say.
“The treatment can reduce DNA damage, reduce inflammation, and increase the number of oligodendrocytes, which are the cells that produce myelin surrounding the axons,” says Li-Huei Tsai, director of MIT’s Picower Institute for Learning and Memory and the Picower Professor in the MIT Department of Brain and Cognitive Sciences. “We also found that this treatment improved learning and memory, and enhanced executive function in the animals.”
Tsai is the senior author of the new study, which appears today in Science Translational Medicine . The paper’s lead author is TaeHyun Kim, an MIT postdoc.
Protective brain waves
Several years ago, Tsai and her colleagues began exploring the use of light flickering at 40 hertz (cycles per second) as a way to improve the cognitive symptoms of Alzheimer’s disease. Previous work had suggested that Alzheimer’s patients have impaired gamma oscillations — brain waves that range from 25 to 80 hertz (cycles per second) and are believed to contribute to brain functions such as attention, perception, and memory.
Tsai’s studies in mice have found that exposure to light flickering at 40 hertz or sounds with a pitch of 40 hertz can stimulate gamma waves in the brain, which has many protective effects, including preventing the formation of amyloid beta plaques. Using light and sound together provides even more significant protection. The treatment also appears promising in humans: Phase 1 clinical trials in people with early-stage Alzheimer’s disease have found the treatment is safe and does offer some neurological and behavioral benefits.
In the new study, the researchers set out to see whether this treatment could also counteract the cognitive effects of chemotherapy treatment. Research has shown that these drugs can induce inflammation in the brain, as well as other detrimental effects such as loss of white matter — the networks of nerve fibers that help different parts of the brain communicate with each other. Chemotherapy drugs also promote loss of myelin, the protective fatty coating that allows neurons to propagate electrical signals. Many of these effects are also seen in the brains of people with Alzheimer’s.
“Chemo brain caught our attention because it is extremely common, and there is quite a lot of research on what the brain is like following chemotherapy treatment,” Tsai says. “From our previous work, we know that this gamma sensory stimulation has anti-inflammatory effects, so we decided to use the chemo brain model to test whether sensory gamma stimulation can be beneficial.”
As an experimental model, the researchers used mice that were given cisplatin, a chemotherapy drug often used to treat testicular, ovarian, and other cancers. The mice were given cisplatin for five days, then taken off of it for five days, then on again for five days. One group received chemotherapy only, while another group was also given 40-hertz light and sound therapy every day.
After three weeks, mice that received cisplatin but not gamma therapy showed many of the expected effects of chemotherapy: brain volume shrinkage, DNA damage, demyelination, and inflammation. These mice also had reduced populations of oligodendrocytes, the brain cells responsible for producing myelin.
However, mice that received gamma therapy along with cisplatin treatment showed significant reductions in all of those symptoms. The gamma therapy also had beneficial effects on behavior: Mice that received the therapy performed much better on tests designed to measure memory and executive function.
“A fundamental mechanism”
Using single-cell RNA sequencing, the researchers analyzed the gene expression changes that occurred in mice that received the gamma treatment. They found that in those mice, inflammation-linked genes and genes that trigger cell death were suppressed, especially in oligodendrocytes, the cells responsible for producing myelin.
In mice that received gamma treatment along with cisplatin, some of the beneficial effects could still be seen up to four months later. However, the gamma treatment was much less effective if it was started three months after the chemotherapy ended.
The researchers also showed that the gamma treatment improved the signs of chemo brain in mice that received a different chemotherapy drug, methotrexate, which is used to treat breast, lung, and other types of cancer.
“I think this is a very fundamental mechanism to improve myelination and to promote the integrity of oligodendrocytes. It seems that it’s not specific to the agent that induces demyelination, be it chemotherapy or another source of demyelination,” Tsai says.
Because of its widespread effects, Tsai’s lab is also testing gamma treatment in mouse models of other neurological diseases, including Parkinson’s disease and multiple sclerosis. Cognito Therapeutics, a company founded by Tsai and MIT Professor Edward Boyden, has finished a phase 2 trial of gamma therapy in Alzheimer’s patients, and plans to begin a phase 3 trial this year.
“My lab’s major focus now, in terms of clinical application, is Alzheimer’s; but hopefully we can test this approach for a few other indications, too,” Tsai says.
The research was funded by the JPB Foundation, the Ko Hahn Seed Fund, and the National Institutes of Health.
Share this news article on:
Press mentions, new scientist.
MIT scientists have found that a potential treatment for Alzheimer’s disease involving flickering lights and low-pitched sound could also help prevent cognitive problems after cancer treatment, reports Clare Wilson for New Scientist . The treatment is aimed at stimulating 40 Hz brainwaves, which are linked to memory processing. The results suggest targeting such “brainwaves may result in broader benefits for the brain, including increasing the activity of immune cells and, most recently, boosting its drainage system, which could help clear a toxic protein called beta-amyloid.”
Previous item Next item
Related Links
- Li-Huei Tsai
- Picower Institute
- Department of Brain and Cognitive Sciences
Related Topics
- Brain and cognitive sciences
- Pharmaceuticals
Related Articles
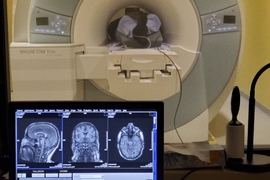
Small studies of 40-hertz sensory stimulation confirm safety, suggest Alzheimer’s benefits
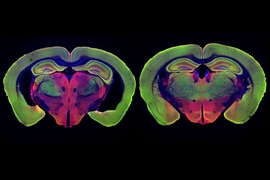
Why visual stimulation may work against Alzheimer’s
![research paper on abbreviation “…[I]f humans behave similarly to mice in response to this treatment, I would say the potential is just enormous, because it’s so noninvasive, and it’s so accessible,” says Li-Huei Tsai, the Picower Professor of Neuroscience, when describing a new treatment for Alzheimer’s disease.](https://news.mit.edu/sites/default/files/styles/news_article__archive/public/images/201612/MIT-li-huei-tsai.jpg?itok=jrC2K2AI)
Unique visual stimulation may be new treatment for Alzheimer’s
More mit news.
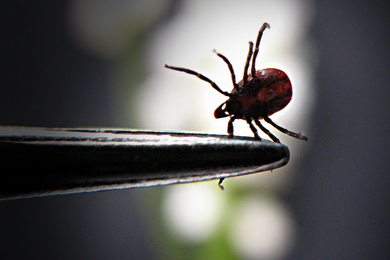
A protein found in human sweat may protect against Lyme disease
Read full story →
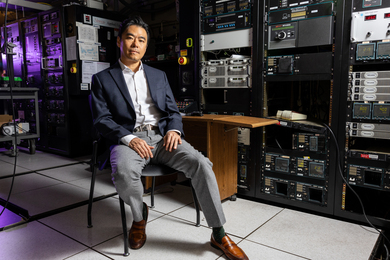
Pushing material boundaries for better electronics
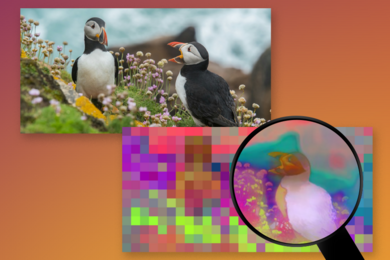
New algorithm unlocks high-resolution insights for computer vision
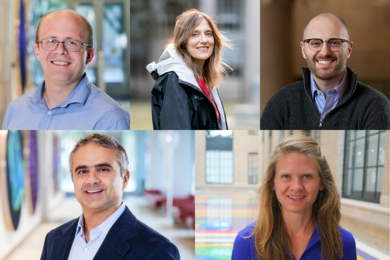
Five MIT faculty members take on Cancer Grand Challenges
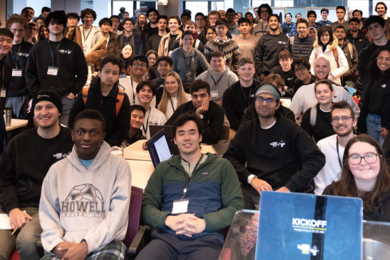
Unlocking the quantum future
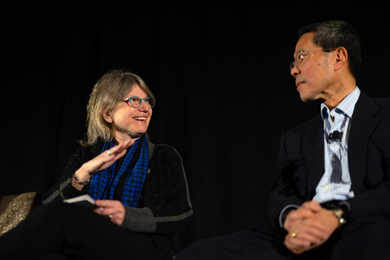
Making the clean energy transition work for everyone
- More news on MIT News homepage →
Massachusetts Institute of Technology 77 Massachusetts Avenue, Cambridge, MA, USA
- Map (opens in new window)
- Events (opens in new window)
- People (opens in new window)
- Careers (opens in new window)
- Accessibility
- Social Media Hub
- MIT on Facebook
- MIT on YouTube
- MIT on Instagram
- Share full article
Advertisement
Supported by
Guest Essay
The Best Way to Find Out if We Can Cool the Planet

By Jeremy Freeman
Dr. Freeman is the executive director of CarbonPlan, a climate research nonprofit.
A few years ago, the idea of deliberately blocking the sun to combat climate change was taboo for scientists. But a lot can change in a short time .
As the disastrous effects of climate change mount, Congress has asked federal scientists for a research plan , private money is flowing and rogue start-ups are attempting experiments — all signs that momentum around solar geoengineering is building fast. The most discussed approach involves spraying tiny particles into the stratosphere to reflect sunlight and cool the planet. Other proposals include injecting sea salt into clouds to increase their reflectivity or using giant space parasols to block the sun .
It might all sound like dystopian science fiction, but some techno-futurists, like OpenAI’s chief executive, Sam Altman, are already normalizing it: “We’re going to have to do something dramatic with climate like geoengineering as a Band-Aid, as a stopgap,” he said in January at the World Economic Forum in Davos.
No one fully understands the risks of these technologies — which could include calamitous disruptions in weather — or how significant the benefits could be. I’m increasingly convinced that we should do more research on solar geoengineering . But such high-stakes science requires extraordinary levels of transparency and accountability to the global public. The alternative is clandestine research controlled by corporations or autocratic regimes, lurching toward deployment without knowing — or knowing and not sharing — the true risks.
The potential risks of solar geoengineering are serious. Spraying reflective particles in one place, for example, could significantly change rainfall patterns elsewhere. What’s more, once anyone starts solar geoengineering at a larger scale, suddenly stopping it could lead to “ termination shock ” — global temperatures rapidly readjusting to where they would have been without geoengineering. With such terrifying points of no return, every accelerating step of research requires global public participation and deliberation.
Most research so far has been tentative and contained to computer simulations . But to know what will happen in the real world, we also need outdoor experiments. By launching an instrument-laden balloon into the stratosphere, for example, researchers could release a tiny amount of particles and measure how they interact with the atmosphere, with minimal environmental risk.
But already we’ve seen a backlash to these kinds of experiments: Harvard geoengineering researchers planned a dry run of their instruments in Sweden in 2021 only to be shut down after the Indigenous Saami Council and local environmental groups protested the tests. A key concern was how such research could redirect attention and investment from more pressing efforts to reduce emissions, thereby becoming a moral hazard . More recently, Mexico banned geoengineering experiments after discovering an American tech entrepreneur had launched a balloon test without permission. And a startup out of Israel has now raised millions of dollars and is planning experiments with little to no transparency. Some assessments suggest that more experiments, and even larger deployments, are increasingly likely. It would be far better if they happened in the open, as in Sweden, rather than in secret.
Even in places where no experiments have been planned, the public is wary. Most people haven’t heard of geoengineering in the United States, but of those who have, 72 percent reported being very concerned we’ll use it before understanding its impact. More broadly, while there’s evidence of support for research , that support is reluctant and conditional. Without transparency and trust, public debate on geoengineering could devolve into conspiracy theories and partisan ideology.
A reluctance to trust scientists is understandable. Science as a profession has for too long pursued prestige at the expense of integrity, and public scientific institutions have been increasingly privatized with minimal accountability. With a long, troubled history that includes eugenics and weapons of war, we cannot pretend that science is either pure or infallible.
But science is fallible precisely because it is a practice , a cooperative human activity. And as the moral philosopher Alasdair MacIntyre reminds us, engaging in a practice well requires exercising its virtues — which for science include transparency, honesty, humility, skepticism and collaboration. For geoengineering, that means disclosing all funding and effectively managing potential conflicts of interests, ensuring the participation of stakeholders from around the world in decision making , avoiding groupthink, sharing early-stage results and data to accelerate research and engaging in radically open science .
Transparency on its own may not lead to the widespread adoption of a new technology. A study on Covid-19 vaccine communication showed that increased transparency, especially about negative outcomes, led to lower vaccine acceptance — but it did increase trust in public health. A potential lesson for solar geoengineering is that transparency is important even when or perhaps especially when it doesn’t result in an outcome scientists initially imagine.
We should be especially wary of ceding control over geoengineering research to the tech industry. Often under the guise of virtue, techno-futurists capitalize on the power that comes from scientific knowledge while exploiting people and the environment, a pattern The Atlantic’s Adrienne LaFrance diagnoses as techno-authoritarianism. We cannot allow private for-profit entities to steer, or covertly fund, solar geoengineering research.
Instead, any research must be done by institutions acting in the public’s interest. If private funding is the only option, scientists will need to choose carefully where they work and defend their integrity against external pressures. They must clearly communicate research findings, positive and negative, and educate the public about what’s possible and what’s at stake. That way the public can in turn hold policymakers, regulators and scientists to account, with everyone working together in pursuit of a common good.
When confronted with the prospect of solar geoengineering, we may wish it had never come to this point. But we can still decide how to move forward responsibly, with and for the public.
Jeremy Freeman is the executive director of CarbonPlan, a climate research nonprofit. Much of CarbonPlan’s work has focused on carbon dioxide removal, another controversial climate technology.
The Times is committed to publishing a diversity of letters to the editor. We’d like to hear what you think about this or any of our articles. Here are some tips . And here’s our email: [email protected] .
Follow the New York Times Opinion section on Facebook , Instagram , TikTok , WhatsApp , X and Threads .
Announcing the NeurIPS 2023 Paper Awards
Communications Chairs 2023 2023 Conference awards , neurips2023
By Amir Globerson, Kate Saenko, Moritz Hardt, Sergey Levine and Comms Chair, Sahra Ghalebikesabi
We are honored to announce the award-winning papers for NeurIPS 2023! This year’s prestigious awards consist of the Test of Time Award plus two Outstanding Paper Awards in each of these three categories:
- Two Outstanding Main Track Papers
- Two Outstanding Main Track Runner-Ups
- Two Outstanding Datasets and Benchmark Track Papers
This year’s organizers received a record number of paper submissions. Of the 13,300 submitted papers that were reviewed by 968 Area Chairs, 98 senior area chairs, and 396 Ethics reviewers 3,540 were accepted after 502 papers were flagged for ethics reviews .
We thank the awards committee for the main track: Yoav Artzi, Chelsea Finn, Ludwig Schmidt, Ricardo Silva, Isabel Valera, and Mengdi Wang. For the Datasets and Benchmarks track, we thank Sergio Escalera, Isabelle Guyon, Neil Lawrence, Dina Machuve, Olga Russakovsky, Hugo Jair Escalante, Deepti Ghadiyaram, and Serena Yeung. Conflicts of interest were taken into account in the decision process.
Congratulations to all the authors! See Posters Sessions Tue-Thur in Great Hall & B1-B2 (level 1).
Outstanding Main Track Papers
Privacy Auditing with One (1) Training Run Authors: Thomas Steinke · Milad Nasr · Matthew Jagielski
Poster session 2: Tue 12 Dec 5:15 p.m. — 7:15 p.m. CST, #1523
Oral: Tue 12 Dec 3:40 p.m. — 4:40 p.m. CST, Room R06-R09 (level 2)
Abstract: We propose a scheme for auditing differentially private machine learning systems with a single training run. This exploits the parallelism of being able to add or remove multiple training examples independently. We analyze this using the connection between differential privacy and statistical generalization, which avoids the cost of group privacy. Our auditing scheme requires minimal assumptions about the algorithm and can be applied in the black-box or white-box setting. We demonstrate the effectiveness of our framework by applying it to DP-SGD, where we can achieve meaningful empirical privacy lower bounds by training only one model. In contrast, standard methods would require training hundreds of models.
Are Emergent Abilities of Large Language Models a Mirage? Authors: Rylan Schaeffer · Brando Miranda · Sanmi Koyejo
Poster session 6: Thu 14 Dec 5:00 p.m. — 7:00 p.m. CST, #1108
Oral: Thu 14 Dec 3:20 p.m. — 3:35 p.m. CST, Hall C2 (level 1)
Abstract: Recent work claims that large language models display emergent abilities, abilities not present in smaller-scale models that are present in larger-scale models. What makes emergent abilities intriguing is two-fold: their sharpness, transitioning seemingly instantaneously from not present to present, and their unpredictability , appearing at seemingly unforeseeable model scales. Here, we present an alternative explanation for emergent abilities: that for a particular task and model family, when analyzing fixed model outputs, emergent abilities appear due to the researcher’s choice of metric rather than due to fundamental changes in model behavior with scale. Specifically, nonlinear or discontinuous metrics produce apparent emergent abilities, whereas linear or continuous metrics produce smooth, continuous, predictable changes in model performance. We present our alternative explanation in a simple mathematical model, then test it in three complementary ways: we (1) make, test and confirm three predictions on the effect of metric choice using the InstructGPT/GPT-3 family on tasks with claimed emergent abilities, (2) make, test and confirm two predictions about metric choices in a meta-analysis of emergent abilities on BIG-Bench; and (3) show how to choose metrics to produce never-before-seen seemingly emergent abilities in multiple vision tasks across diverse deep networks. Via all three analyses, we provide evidence that alleged emergent abilities evaporate with different metrics or with better statistics, and may not be a fundamental property of scaling AI models.
Outstanding Main Track Runner-Ups
Scaling Data-Constrained Language Models Authors : Niklas Muennighoff · Alexander Rush · Boaz Barak · Teven Le Scao · Nouamane Tazi · Aleksandra Piktus · Sampo Pyysalo · Thomas Wolf · Colin Raffel
Poster session 2: Tue 12 Dec 5:15 p.m. — 7:15 p.m. CST, #813
Oral: Tue 12 Dec 3:40 p.m. — 4:40 p.m. CST, Hall C2 (level 1)
Abstract : The current trend of scaling language models involves increasing both parameter count and training dataset size. Extrapolating this trend suggests that training dataset size may soon be limited by the amount of text data available on the internet. Motivated by this limit, we investigate scaling language models in data-constrained regimes. Specifically, we run a large set of experiments varying the extent of data repetition and compute budget, ranging up to 900 billion training tokens and 9 billion parameter models. We find that with constrained data for a fixed compute budget, training with up to 4 epochs of repeated data yields negligible changes to loss compared to having unique data. However, with more repetition, the value of adding compute eventually decays to zero. We propose and empirically validate a scaling law for compute optimality that accounts for the decreasing value of repeated tokens and excess parameters. Finally, we experiment with approaches mitigating data scarcity, including augmenting the training dataset with code data or removing commonly used filters. Models and datasets from our 400 training runs are freely available at https://github.com/huggingface/datablations .
Direct Preference Optimization: Your Language Model is Secretly a Reward Model Authors: Rafael Rafailov · Archit Sharma · Eric Mitchell · Christopher D Manning · Stefano Ermon · Chelsea Finn
Poster session 6: Thu 14 Dec 5:00 p.m. — 7:00 p.m. CST, #625
Oral: Thu 14 Dec 3:50 p.m. — 4:05 p.m. CST, Ballroom A-C (level 2)
Abstract: While large-scale unsupervised language models (LMs) learn broad world knowledge and some reasoning skills, achieving precise control of their behavior is difficult due to the completely unsupervised nature of their training. Existing methods for gaining such steerability collect human labels of the relative quality of model generations and fine-tune the unsupervised LM to align with these preferences, often with reinforcement learning from human feedback (RLHF). However, RLHF is a complex and often unstable procedure, first fitting a reward model that reflects the human preferences, and then fine-tuning the large unsupervised LM using reinforcement learning to maximize this estimated reward without drifting too far from the original model. In this paper, we leverage a mapping between reward functions and optimal policies to show that this constrained reward maximization problem can be optimized exactly with a single stage of policy training, essentially solving a classification problem on the human preference data. The resulting algorithm, which we call Direct Preference Optimization (DPO), is stable, performant, and computationally lightweight, eliminating the need for fitting a reward model, sampling from the LM during fine-tuning, or performing significant hyperparameter tuning. Our experiments show that DPO can fine-tune LMs to align with human preferences as well as or better than existing methods. Notably, fine-tuning with DPO exceeds RLHF’s ability to control sentiment of generations and improves response quality in summarization and single-turn dialogue while being substantially simpler to implement and train.
Outstanding Datasets and Benchmarks Papers
In the dataset category :
ClimSim: A large multi-scale dataset for hybrid physics-ML climate emulation
Authors: Sungduk Yu · Walter Hannah · Liran Peng · Jerry Lin · Mohamed Aziz Bhouri · Ritwik Gupta · Björn Lütjens · Justus C. Will · Gunnar Behrens · Julius Busecke · Nora Loose · Charles Stern · Tom Beucler · Bryce Harrop · Benjamin Hillman · Andrea Jenney · Savannah L. Ferretti · Nana Liu · Animashree Anandkumar · Noah Brenowitz · Veronika Eyring · Nicholas Geneva · Pierre Gentine · Stephan Mandt · Jaideep Pathak · Akshay Subramaniam · Carl Vondrick · Rose Yu · Laure Zanna · Tian Zheng · Ryan Abernathey · Fiaz Ahmed · David Bader · Pierre Baldi · Elizabeth Barnes · Christopher Bretherton · Peter Caldwell · Wayne Chuang · Yilun Han · YU HUANG · Fernando Iglesias-Suarez · Sanket Jantre · Karthik Kashinath · Marat Khairoutdinov · Thorsten Kurth · Nicholas Lutsko · Po-Lun Ma · Griffin Mooers · J. David Neelin · David Randall · Sara Shamekh · Mark Taylor · Nathan Urban · Janni Yuval · Guang Zhang · Mike Pritchard
Poster session 4: Wed 13 Dec 5:00 p.m. — 7:00 p.m. CST, #105
Oral: Wed 13 Dec 3:45 p.m. — 4:00 p.m. CST, Ballroom A-C (level 2)
Abstract: Modern climate projections lack adequate spatial and temporal resolution due to computational constraints. A consequence is inaccurate and imprecise predictions of critical processes such as storms. Hybrid methods that combine physics with machine learning (ML) have introduced a new generation of higher fidelity climate simulators that can sidestep Moore’s Law by outsourcing compute-hungry, short, high-resolution simulations to ML emulators. However, this hybrid ML-physics simulation approach requires domain-specific treatment and has been inaccessible to ML experts because of lack of training data and relevant, easy-to-use workflows. We present ClimSim, the largest-ever dataset designed for hybrid ML-physics research. It comprises multi-scale climate simulations, developed by a consortium of climate scientists and ML researchers. It consists of 5.7 billion pairs of multivariate input and output vectors that isolate the influence of locally-nested, high-resolution, high-fidelity physics on a host climate simulator’s macro-scale physical state. The dataset is global in coverage, spans multiple years at high sampling frequency, and is designed such that resulting emulators are compatible with downstream coupling into operational climate simulators. We implement a range of deterministic and stochastic regression baselines to highlight the ML challenges and their scoring. The data (https://huggingface.co/datasets/LEAP/ClimSim_high-res) and code (https://leap-stc.github.io/ClimSim) are released openly to support the development of hybrid ML-physics and high-fidelity climate simulations for the benefit of science and society.
In the benchmark category :
DecodingTrust: A Comprehensive Assessment of Trustworthiness in GPT Models
Authors: Boxin Wang · Weixin Chen · Hengzhi Pei · Chulin Xie · Mintong Kang · Chenhui Zhang · Chejian Xu · Zidi Xiong · Ritik Dutta · Rylan Schaeffer · Sang Truong · Simran Arora · Mantas Mazeika · Dan Hendrycks · Zinan Lin · Yu Cheng · Sanmi Koyejo · Dawn Song · Bo Li
Poster session 1: Tue 12 Dec 10:45 a.m. — 12:45 p.m. CST, #1618
Oral: Tue 12 Dec 10:30 a.m. — 10:45 a.m. CST, Ballroom A-C (Level 2)
Abstract: Generative Pre-trained Transformer (GPT) models have exhibited exciting progress in capabilities, capturing the interest of practitioners and the public alike. Yet, while the literature on the trustworthiness of GPT models remains limited, practitioners have proposed employing capable GPT models for sensitive applications to healthcare and finance – where mistakes can be costly. To this end, this work proposes a comprehensive trustworthiness evaluation for large language models with a focus on GPT-4 and GPT-3.5, considering diverse perspectives – including toxicity, stereotype bias, adversarial robustness, out-of-distribution robustness, robustness on adversarial demonstrations, privacy, machine ethics, and fairness. Based on our evaluations, we discover previously unpublished vulnerabilities to trustworthiness threats. For instance, we find that GPT models can be easily misled to generate toxic and biased outputs and leak private information in both training data and conversation history. We also find that although GPT-4 is usually more trustworthy than GPT-3.5 on standard benchmarks, GPT-4 is more vulnerable given jailbreaking system or user prompts, potentially due to the reason that GPT-4 follows the (misleading) instructions more precisely. Our work illustrates a comprehensive trustworthiness evaluation of GPT models and sheds light on the trustworthiness gaps. Our benchmark is publicly available at https://decodingtrust.github.io/.
Test of Time
This year, following the usual practice, we chose a NeurIPS paper from 10 years ago to receive the Test of Time Award, and “ Distributed Representations of Words and Phrases and their Compositionality ” by Tomas Mikolov, Ilya Sutskever, Kai Chen, Greg Corrado, and Jeffrey Dean, won.
Published at NeurIPS 2013 and cited over 40,000 times, the work introduced the seminal word embedding technique word2vec. Demonstrating the power of learning from large amounts of unstructured text, the work catalyzed progress that marked the beginning of a new era in natural language processing.
Greg Corrado and Jeffrey Dean will be giving a talk about this work and related research on Tuesday, 12 Dec at 3:05 – 3:25 pm CST in Hall F.
Related Posts
2023 Conference
Announcing NeurIPS 2023 Invited Talks
Reflections on the neurips 2023 ethics review process, neurips newsletter – november 2023.
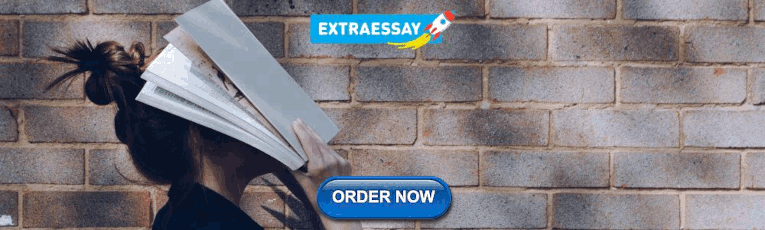
IMAGES
VIDEO
COMMENTS
A list of abbreviations is an alphabetical list of abbreviations that you can add to your thesis or dissertation. If you choose to include it, it should appear at the beginning of your document, just after your table of contents. Abbreviation lists improve readability, minimizing confusion about abbreviations unfamiliar to your reader.
Cheat sheet No time to read? Here's the short version: Avoid contractions like won't, can't, they're, it's. The first time you mention a phrase that can be abbreviated, spell it out in full and provide the abbreviation in parentheses. Use only the abbreviation thereafter. Only abbreviate phrases that occur three or more times in your paper.
An abbreviation is a shortened or contracted form of a word or phrase, such as appt for appointment, parens for parentheses, or lb for pound. Titles are also frequently abbreviated, such as Dr. (Doctor), Rev. (Reverend), and Prof. (Professor). Note that the capitalization of the abbreviation is driven by the original word or phrase.
An abbreviation is a shortened form of a word or phrase. Use abbreviations sparingly and only when they are well-defined, are familiar to readers, and make your writing clearer. Abbreviations listed as words in the dictionary (without the label "abbr.") do not need to be defined in the text. Define all other abbreviations.
There was at least one acronym in 19% of the titles and 73% of the abstracts. Acronym use has also increased over time, but the re-use of acronyms has declined. We found that from more than one ...
As a general rule, all non-standard acronyms/abbreviations in research papers should be written out in full on first use (in both the abstract and the paper itself), followed by the abbreviated form in parentheses, as in the American Psychological Association (APA) style guide. Table of Contents
A list of abbreviations is an alphabetical list of abbreviations that you can add to your thesis or dissertation. If you choose to include it, it should appear at the beginning of your document, just after your table of contents. Abbreviation lists improve readability, minimising confusion about abbreviations unfamiliar to your reader.
Abbreviations are shortened forms of words and phrases and are a common occurrence in research manuscripts as they can help make highly complex technical writing more concise and easier to read. However, they can also cause a lot of confusion, and make communication unclear if they are not used with caution. Consider these sentences:
You should not overuse abbreviations (writing is easier to understand when words are written out); you should also not underuse abbreviations (in general, if you use an abbreviation, it should appear at least three times in your paper, because fewer uses mean it is more likely your reader will forget what the abbreviation refers to).
An abbreviation is a shortened version of a term or phrase, e.g. kg for kilogram or Dr. for doctor. An acronym is a type of abbreviation constructed from the first letters of a term, e.g. FRP for Fibre Reinforced Polymer or STEM for Science, Technology, Engineering and Maths. List of Abbreviations in a Thesis or Dissertation
Basics of Abbreviations Using abbreviations can be an effective way to avoid repeating lengthy, technical terms throughout a piece of writing, but they should be used sparingly to prevent your text from becoming difficult to read.
There are three main things to keep in mind when using abbreviations in an academic or scientific paper. These are spacing, capitalization, and punctuation. It is important to note that, like many other aspects of writing, the rules are sometimes different in the US and UK variations of English.
Scholarly Abbreviations. The MLA Handbook (9 th ed.) encourages users to adhere to the common scholarly abbreviations for both in-text citations and in the works-cited page. Here is the list of common scholarly abbreviations from Appendix 1 of the MLA Handbook (9 th ed.) with a few additions: anon. for anonymous. app. for appendix. bk. for book.
Abbreviations are extensively used in the scientific and medical communities. It is common practice to use abbreviations for long names of many clinical diseases and procedures, and for scientific techniques that have to be repeated many times in medical or scientific papers, posters, and oral presentations.
Abbreviations Notes: Standard abbreviations can be used in your citations. Some of the more commonly used examples of abbreviations are listed below. And others Compiled or custom textbook Edition Editor (s) No date No page numbers Number Page (s) Paragraph Revised edition Translator (s) Volume (s)
Acronyms Abbreviations and acronyms are shortened forms of words or phrases. Generally, abbreviations are not acceptable in academic writing (with some exceptions, see below) and acronyms are (providing they are used as shown below). Abbreviations As academic writing is formal in style, most abbreviations should be avoided.
Is it a good practice to include an abbreviation in a title of a research paper. for example : PC , IDE , RAM Stack Exchange Network Stack Exchange network consists of 183 Q&A communities including Stack Overflow , the largest, most trusted online community for developers to learn, share their knowledge, and build their careers.
Journal Titles and Abbreviations A Acc. Chem. Res. ACH - Models Chem. ACI Mater. J. ACS Symp. Ser. Acta Biochim. Pol. Acta Biotechnol. Acta Chem. Scand.
Abbreviations, particularly those that are not standard, should also be kept to a minimum. ... papers in press, and research datasets that have been assigned a digital object identifier may be ...
For a complete list of Common Scholarly Abbreviations, please see Section 7.4 in the 6th edition of the MLA Handbook for Writers of Research Papers.. Abbreviations of Degrees. Note: When documenting sources using MLA style, the normal punctuation is omitted for degrees when used in parentheses, tables, works cited, footnotes, endnotes, etc.
Introduction. This article deals with drafting a suitable "title" and an appropriate "abstract" for an original research paper. Because the "title" and the "abstract" are the "initial impressions" or the "face" of a research article, they need to be drafted correctly, accurately, carefully, meticulously, and consume time and energy.[1,2,3,4,5,6,7,8,9,10] Often, these ...
Here's a quick primer. 1. Spell the word or phrase in full at first mention. When you first use the term that you will be abbreviating, you should usually spell out the actual word or phrase in full and follow that with the abbreviation/acronym in brackets. For example: "We then conducted an analysis of variance (ANOVA).".
The proliferation of artificial intelligence tools in scientific research risks creating illusions of understanding, where scientists believe they understand more about the world than they ...
Although all of the authors of this paper agree with the need for risk associated with "precarious decisions" in design research, we also recognise that a performative culture is restrictive: demanding that educators have a sense of certainty in what will work in any classroom because it relies on a normalised practice that is somehow believed to be evidence-informed (Biesta, 2007b, 2019 ...
These papers were published between March 2020 and July 2023 in top journals in management and applied psychology. ... His research interests include leadership, teams, and organizational ...
The paper, co-authored by Princeton cognitive scientist M. J. Crockett, sets a framework for discussing the risks involved in using AI tools throughout the scientific research process, from study design through peer review. " We hope this paper offers a vocabulary for talking about AI's potential epistemic risks," Messeri said.
The paper's lead author is TaeHyun Kim, an MIT postdoc. ... "Chemo brain caught our attention because it is extremely common, and there is quite a lot of research on what the brain is like following chemotherapy treatment," Tsai says. "From our previous work, we know that this gamma sensory stimulation has anti-inflammatory effects, so ...
Dr. Freeman is the executive director of CarbonPlan, a climate research nonprofit. A few years ago, the idea of deliberately blocking the sun to combat climate change was taboo for scientists. But ...
Announcing the NeurIPS 2023 Paper Awards By Amir Globerson, Kate Saenko, Moritz Hardt, Sergey Levine and Comms Chair, Sahra Ghalebikesabi . We are honored to announce the award-winning papers for NeurIPS 2023! This year's prestigious awards consist of the Test of Time Award plus two Outstanding Paper Awards in each of these three categories: . Two Outstanding Main Track Papers