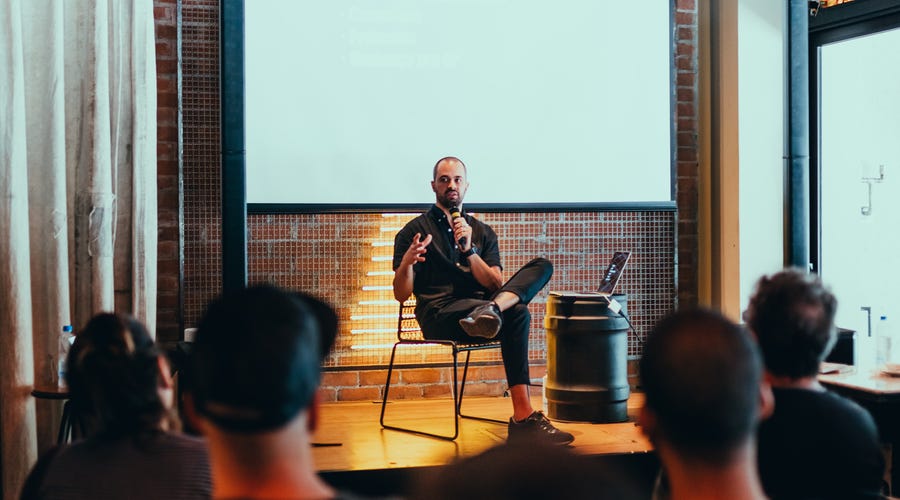
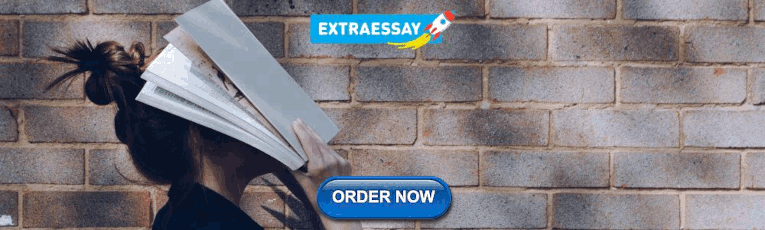
127 big fancy words to sound smart and boost your eloquence

Karolina Assi
Everyone wants to sound smart and come across as someone that can express their thoughts eloquently. And even though you might have this fantastic ability in your native language, you may feel limited doing this in English if you’re beginning your journey in expanding your vocabulary with unusual or rarer words.
Fortunately, the English language has thousands of big words that will make you sound instantly more eloquent and knowledgeable.
These words will help you express yourself in a more elegant way by substituting the basic, everyday words with their more fancy synonyms. Learning those “big” words in English is also a great way to impress those around you - whether it’s at school, at work, or during your next date.
To help you take your English vocabulary to the next level, we’re prepared a list of 120+ big words to sound smart, with their meaning and an example of how to use them in context.
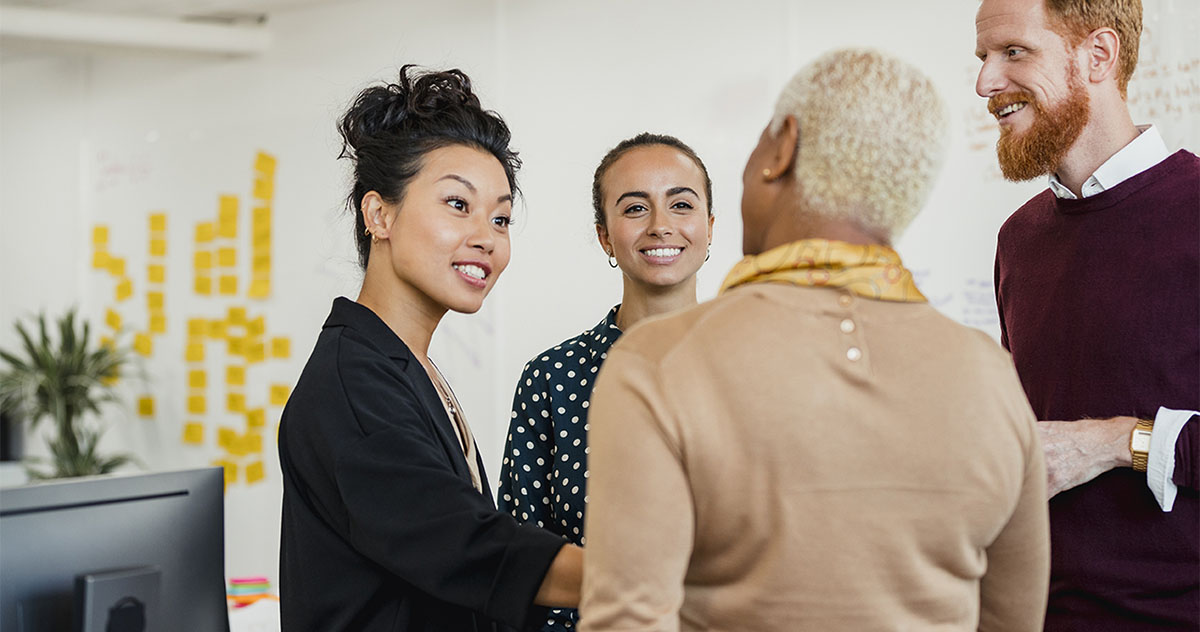
The do’s and don'ts of using big words in English
Throwing in a few fancy words into your conversations or monologue is a good idea to sound more eloquent and impress everyone around you.
It’s also a great way to sound smart when you don’t know what to say on a specific topic but want to make a good impression and appear more knowledgeable than you are (like this English student during his literature class ).
But there’s a fine line between using fancy words that truly make you sound eloquent and those that make you sound like you’re trying too hard.
Sometimes, using big words to sound smart may backfire, especially if you don’t really know what they mean. Then, you may end up saying something that makes no sense and leaving everyone in the room perplexed. Plus, using complex words you don’t understand can make you sound pompous - so tread the line between careful and carefree.
Use them only if you truly understand their meaning and know what context to use them in. But don’t use them mindlessly as it will result in an opposite effect to what you intended.
Aside from learning those fancy words and their meaning, another challenge lies in their pronunciation. If you choose those big words that are also hard to pronounce , like “epitome” or “niche,” you might end up saying something that makes everyone laugh (it wouldn’t be such a bad scenario!).
The point is: if you’re going to use fancy words to sound smart, learn their meaning, understand how to use them in context, and practice their pronunciation first.
Big words to sound smart and their meaning
The smartest way of sounding more eloquent when expressing yourself in English is to change basic, everyday words for their fancier versions. For instance, instead of saying “very big,” say “massive.” Instead of saying “detailed.” say “granular,” and instead of saying “not interesting,” say “banal.”
See? Using the word “granular” in a sentence will inevitably add more elegance to your speech and make you appear more fluent and eloquent.
The words we’ve chosen to include in the tables below follow this exact principle. Most of them are just a fancier version of a basic, simple word you’d normally use. Others are words used in a professional or academic setting that simply add more articulacy to your statement.
Fancy words you can use at work
The question isn’t whether you should learn a couple of fancy words you can use at work to impress your boss and coworkers. The question is, how do you use them without coming across as a pompous know-it-all, irritating everyone around you?
Well, it’s all about using them wisely. Don’t cram 10 fancy words into a simple sentence just to sound smarter. Only use them when they help you get your message across. If they don’t bring any value to your sentence, simply don’t use them.
In other words - don’t force it! Be natural.
With that said, here are some big words you can use at work.
.jpeg)
Ready to further your career with a new language?
Get the language skills, cultural understanding and confidence to open up your world with Berlitz.
Clever words you might use academically
The academic setting does not only encourage you to sound smart. It forces you to. To get higher grades and convince your professors of your knowledge and eloquence, you need to elevate your vocabulary.
Whether it’s in written or spoken assignments, these words will help you express yourself in a more intelligent and elegant way while impressing your colleagues and professors.
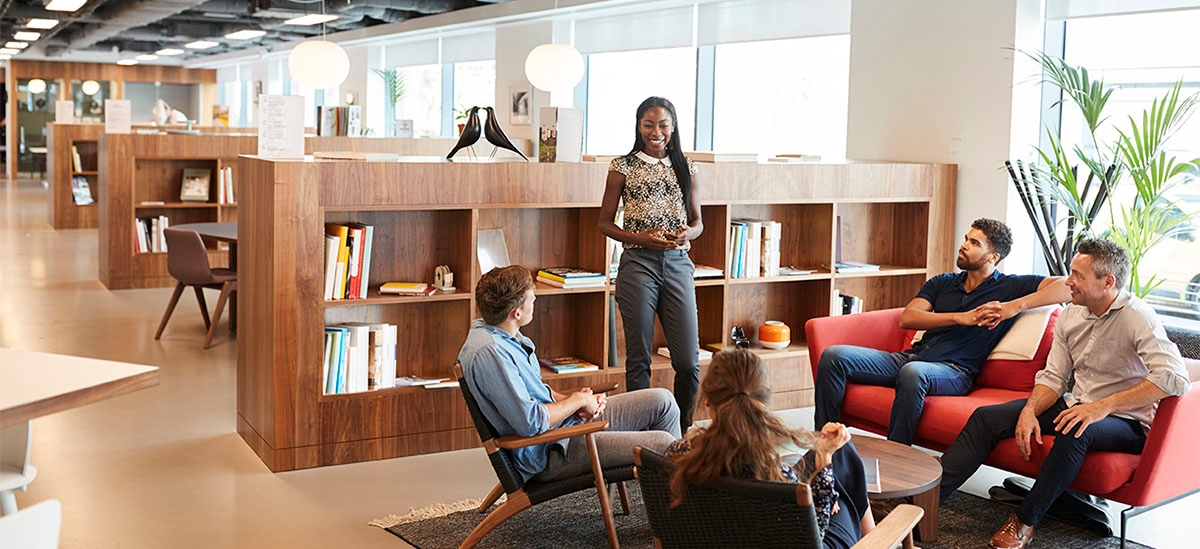
Big interesting words you might use socially
Being the smartest person among your friends is surely a great boost for your ego. It can help you gain their approval, receive compliments, and maybe even get a date or two while hanging out at the bar with your friends.
But the other side of the coin is that using overly sophisticated words in a casual, social setting can make you appear pretentious and out of place. That’s why you need to be careful and not overdo it! If you do, you might only end up humiliating yourself, and that’s a terrible place to be in.
Here are 20+ big words in English you can use in social situations with their meaning and an example of a sentence you could say.
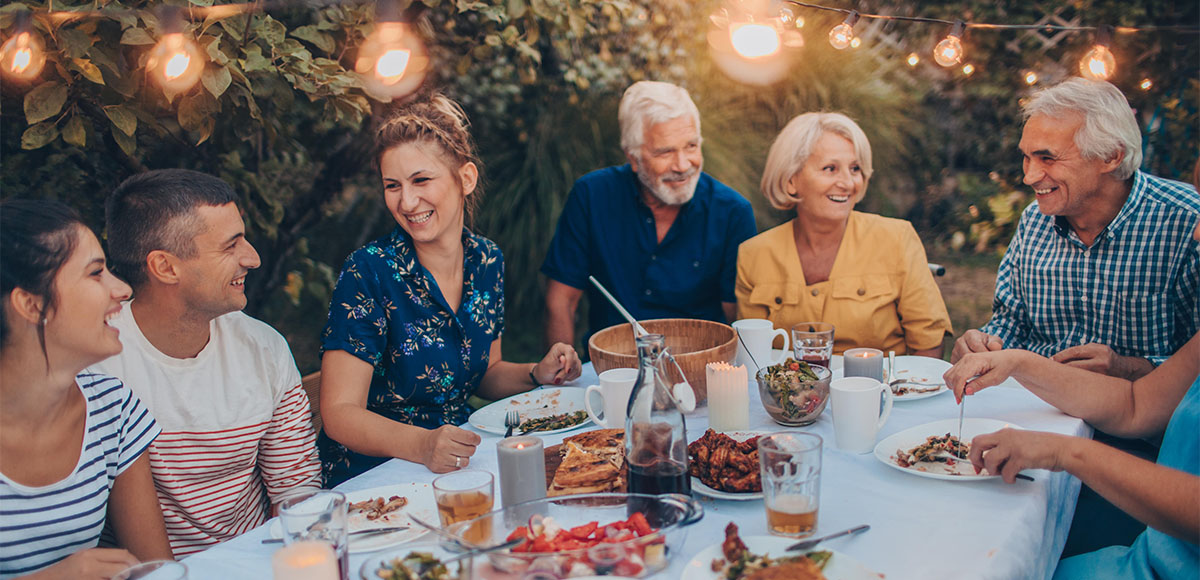
Impressive words you might use romantically
Even if you’re not a very romantic person, some occasions require a bit of romanticism. Using elegant words in your expressions of love and affection can make your romantic conversations and gestures more special and memorable.
Still, don’t use big words if you don’t mean them! You should always be sincere and genuine in your expressions. Remember that words hold tremendous power in inspiring emotions in those who receive them.
With that said, here are 30 big words you can use in a romantic setting to express your love and affection for your significant other or to take your relationship with the person you’re currently dating to the next level (congrats!).
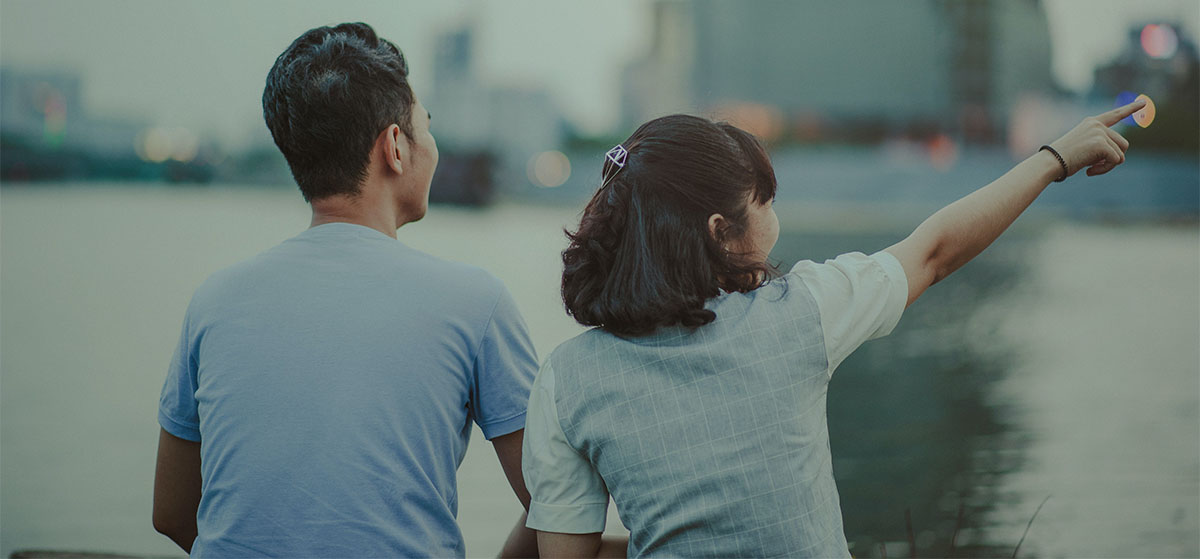
Sophisticated words you might use when discussing art and literature
Are you an art or literature? These two areas often require eloquent vocabulary to describe them. At least, that is the sort of language that people expect to hear from someone who’s an avid reader and art connoisseur.
You might want to express how the allegory in that poem made you feel or the way the plot of the book has enthralled you to keep reading but lack the right words to do it. If so, here’s a list of 20+ words you can use to talk about art and literature in different contexts.
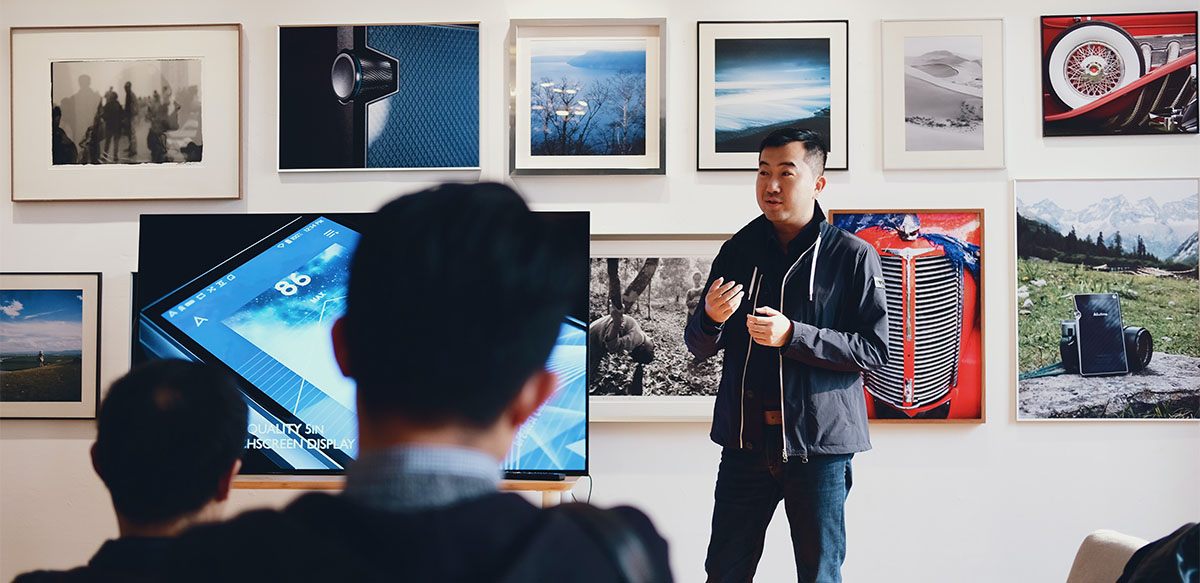
Fancy words you might use when talking about your hobbies
When talking about our hobbies, we want to come across as more knowledgeable than others. After all, they’re our special interests, and we naturally possess a greater deal of expertise in these areas.
Whether you’re into literature, movies, or sports, here are some fancy words you can use to describe your interests.
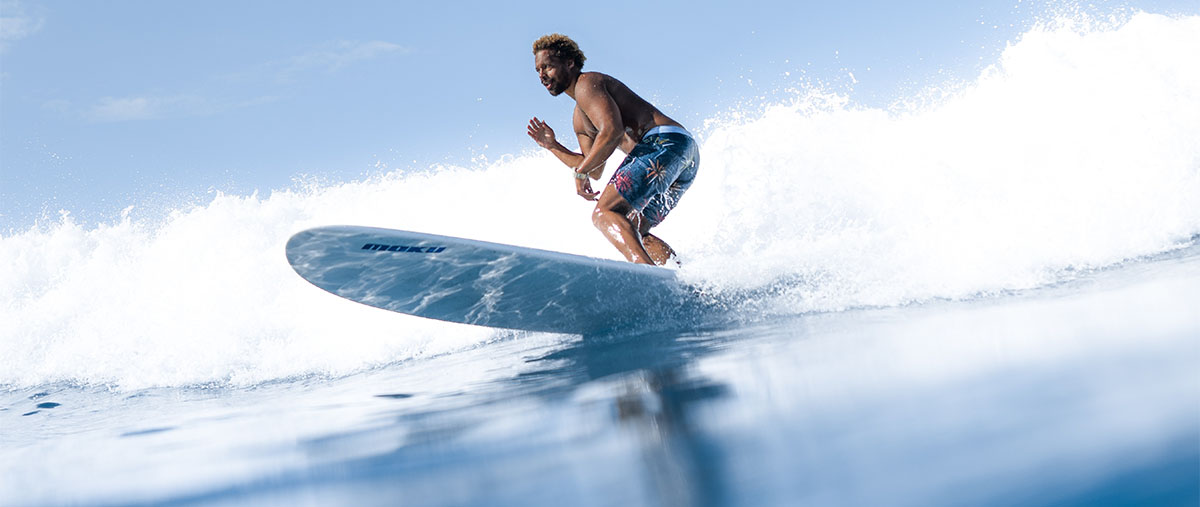
Make the Thesaurus your new best friend
In this article, we’ve only covered 126 big words. Understandably, we can’t include all the fancy words you might need in one article. There are simply too many!
But luckily, there’s a free online tool you can use to find the synonyms of everyday words to expand your vocabulary and make yourself sound smarter.
Can you take a guess?
That’s right - it’s the online Thesaurus . You’ve surely heard about it from your English teacher, but in case you haven’t, Thesaurus is a dictionary of synonyms and related concepts. It’s a great way to find synonyms of different words to spice up your oral or written statements and avoid repeating the same old boring words time and time again.
Choose your words wisely
Whether you’re using simple, everyday words in casual conversations or those big, fancy words in a professional or academic environment, remember one thing: words have power.
They’re spells that you cast (there’s a reason why it’s called “spelling”) onto yourself and those who you speak them to. The words you speak inspire emotions and shape how other people perceive you. But they also influence your own emotions and shape how you perceive yourself.
So choose them wisely.
Learn more about the fascinating English language on our English language blog here.
Related Articles
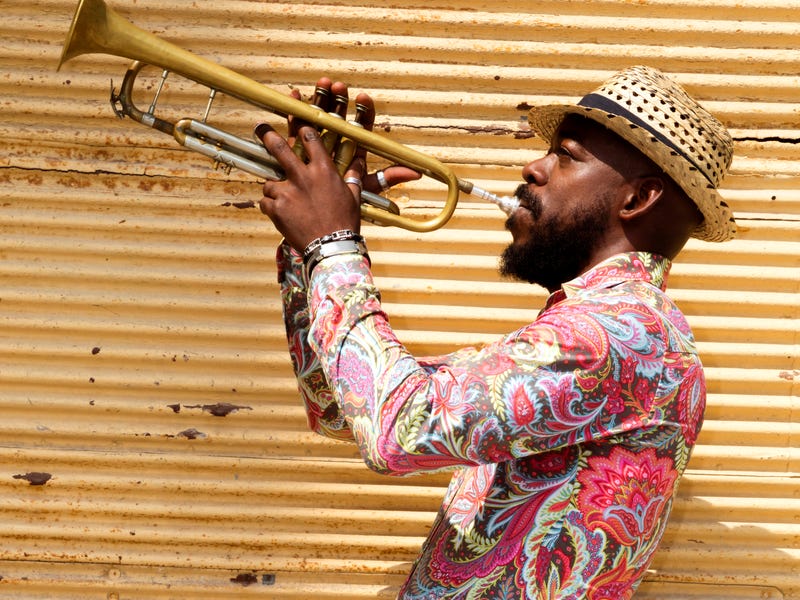
January 31, 2024
15 of the longest words in English and how to pronounce them
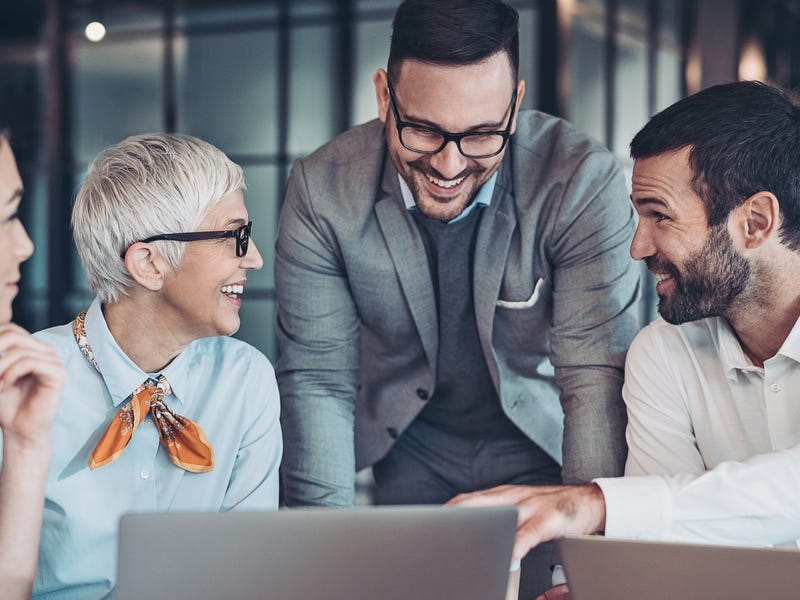
May 23, 2023
127 business English phrases for great business conversations
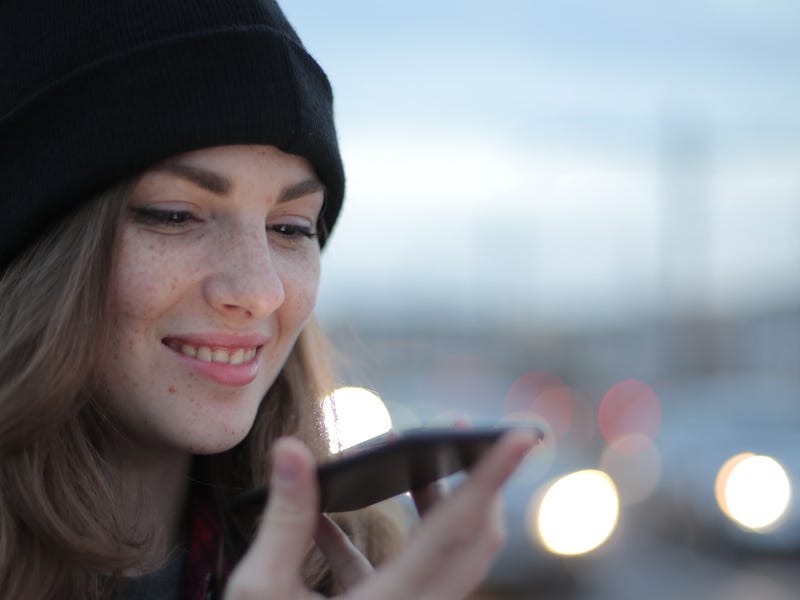
January 31, 2023
252 of the hardest English words to pronounce and spell
1-866-423-7548, find out more.
Fill in the form below and we’ll contact you to discuss your learning options and answer any questions you may have.
I have read the Terms of Use and Privacy Policy
- Privacy Policy
- Terms Of Use

Words for Speaking: 30 Speech Verbs in English (With Audio)

Speaking is amazing, don’t you think?
Words and phrases come out of our mouths — they communicate meaning, and we humans understand each other (well, sometimes)!
But there are countless different ways of speaking.
Sometimes, we express ourselves by speaking quietly, loudly, angrily, unclearly or enthusiastically.
And sometimes, we can express ourselves really well without using any words at all — just sounds.
When we describe what someone said, of course we can say, “He said …” or “She said …”
But there are so many alternatives to “say” that describe the many different WAYS of speaking.
Here are some of the most common ones.
Words for talking loudly in English
Shout / yell / scream.
Sometimes you just need to say something LOUDLY!
Maybe you’re shouting at your kids to get off the climbing frame and come inside before the storm starts.
Or perhaps you’re just one of those people who just shout a lot of the time when you speak. And that’s fine. I’ve got a friend like that. He says it’s because he’s the youngest kid in a family full of brothers and sisters — he had to shout to make sure people heard him. And he still shouts.
Yelling is a bit different. When you yell, you’re probably angry or surprised or even in pain. Yelling is a bit shorter and more “in-the-moment.”
Screaming is similar but usually higher in pitch and full of fear or pain or total fury, like when you’ve just seen a ghost or when you’ve dropped a box of bricks on your foot.

“Stop yelling at me! I’m sorry! I made a mistake, but there’s no need to shout!”
Bark / Bellow / Roar
When I hear these words, I always imagine something like this:

These verbs all feel rather masculine, and you imagine them in a deep voice.
I always think of an army general walking around the room telling people what to do.
That’s probably why we have the phrase “to bark orders at someone,” which means to tell people what to do in an authoritative, loud and aggressive way.
“I can’t stand that William guy. He’s always barking orders at everyone!”
Shriek / Squeal / Screech
Ooooohhh …. These do not sound nice.
These are the sounds of a car stopping suddenly.
Or the sound a cat makes when you tread on her tail.
Or very overexcited kids at a birthday party after eating too much sugar.
These verbs are high pitched and sometimes painful to hear.
“When I heard her shriek , I ran to the kitchen to see what it was. Turned out it was just a mouse.”
“As soon as she opened the box and saw the present, she let out a squeal of delight!”
Wailing is also high pitched, but not so full of energy.
It’s usually full of sadness or even anger.
When I think of someone wailing, I imagine someone completely devastated — very sad — after losing someone they love.
You get a lot of wailing at funerals.
“It’s such a mess!” she wailed desperately. “It’ll take ages to clear up!”
Words for speaking quietly in English
When we talk about people speaking in quiet ways, for some reason, we often use words that we also use for animals.
In a way, this is useful, because we can immediately get a feel for the sound of the word.
This is the sound that snakes make.
Sometimes you want to be both quiet AND angry.
Maybe someone in the theatre is talking and you can’t hear what Hamlet’s saying, so you hiss at them to shut up.
Or maybe you’re hanging out with Barry and Naomi when Barry starts talking about Naomi’s husband, who she split up with last week.
Then you might want to hiss this information to Barry so that Naomi doesn’t hear.
But Naomi wasn’t listening anyway — she was miles away staring into the distance.
“You’ll regret this!” he hissed , pointing his finger in my face.
To be fair, this one’s a little complicated.
Whimpering is a kind of traumatised, uncomfortable sound.
If you think of a frightened animal, you might hear it make some kind of quiet, weak sound that shows it’s in pain or unhappy.
Or if you think of a kid who’s just been told she can’t have an ice cream.
Those sounds might be whimpers.
“Please! Don’t shoot me!” he whimpered , shielding his head with his arms.

Whispering is when you speak, but you bypass your vocal cords so that your words sound like wind.
In a way, it’s like you’re speaking air.
Which is a pretty cool way to look at it.
This is a really useful way of speaking if you’re into gossiping.
“Hey! What are you whispering about? Come on! Tell us! We’ll have no secrets here!”
Words for speaking negatively in English
Ranting means to speak at length about a particular topic.
However, there’s a bit more to it than that.
Ranting is lively, full of passion and usually about something important — at least important to the person speaking.
Sometimes it’s even quite angry.
We probably see rants most commonly on social media — especially by PEOPLE WHO LOVE USING CAPS LOCK AND LOTS OF EXCLAMATION MARKS!!!!!!
Ranting always sounds a little mad, whether you’re ranting about something reasonable, like the fact that there’s too much traffic in the city, or whether you’re ranting about something weird, like why the world is going to hell and it’s all because of people who like owning small, brown dogs.
“I tried to talk to George, but he just started ranting about the tax hike.”
“Did you see Jemima’s most recent Facebook rant ? All about how squirrels are trying to influence the election results with memes about Macaulay Culkin.”
Babble / Blabber / Blather / Drone / Prattle / Ramble

These words all have very similar meanings.
First of all, when someone babbles (or blabbers or blathers or drones or prattles or rambles), it means they are talking for a long time.
And probably not letting other people speak.
And, importantly, about nothing particularly interesting or important.
You know the type of person, right?
You run into a friend or someone you know.
All you do is ask, “How’s life?” and five minutes later, you’re still listening to them talking about their dog’s toilet problems.
They just ramble on about it for ages.
These verbs are often used with the preposition “on.”
That’s because “on” often means “continuously” in phrasal verbs .
So when someone “drones on,” it means they just talk for ages about nothing in particular.
“You’re meeting Aunt Thelma this evening? Oh, good luck! Have fun listening to her drone on and on about her horses.”
Groan / Grumble / Moan
These words simply mean “complain.”
There are some small differences, though.
When you groan , you probably don’t even say any words. Instead, you just complain with a sound.
When you grumble , you complain in a sort of angry or impatient way. It’s not a good way to get people to like you.
Finally, moaning is complaining, but without much direction.
You know the feeling, right?
Things are unfair, and stuff isn’t working, and it’s all making life more difficult than it should be.
We might not plan to do anything about it, but it definitely does feel good to just … complain about it.
Just to express your frustration about how unfair it all is and how you’ve been victimised and how you should be CEO by now and how you don’t get the respect you deserve and …
Well, you get the idea.
If you’re frustrated with things, maybe you just need to find a sympathetic ear and have a good moan.
“Pietor? He’s nice, but he does tend to grumble about the local kids playing football on the street.”
Words for speaking unclearly in English
Mumble / murmur / mutter.
These verbs are all very similar and describe speaking in a low and unclear way, almost like you’re speaking to yourself.
Have you ever been on the metro or the bus and seen someone in the corner just sitting and talking quietly and a little madly to themselves?
That’s mumbling (or murmuring or muttering).
What’s the difference?
Good question!
The differences are just in what type of quiet and unclear speaking you’re doing.
When someone’s mumbling , it means they’re difficult to understand. You might want to ask them to speak more clearly.
Murmuring is more neutral. It might be someone praying quietly to themselves, or you might even hear the murmur of voices behind a closed door.
Finally, muttering is usually quite passive-aggressive and has a feeling of complaining to it.
“I could hear him muttering under his breath after his mum told him off.”

How can you tell if someone’s been drinking too much booze (alcohol)?
Well, apart from the fact that they’re in the middle of trying to climb the traffic lights holding a traffic cone and wearing grass on their head, they’re also slurring — their words are all sort of sliding into each other. Like this .
This can also happen if you’re super tired.
“Get some sleep! You’re slurring your words.”
Stammer / Stutter
Th-th-th-this is wh-wh-when you try to g-g-g-get the words ou-ou-out, but it’s dif-dif-dif-difficu-… hard.
For some people, this is a speech disorder, and the person who’s doing it can’t help it.
If you’ve seen the 2010 film The King’s Speech , you’ll know what I’m talking about.
(Also you can let me know, was it good? I didn’t see it.)
This can also happen when you’re frightened or angry or really, really excited — and especially when you’re nervous.
That’s when you stammer your words.
“No … I mean, yeah … I mean no…” Wendy stammered .
Other words for speaking in English
If you drawl (or if you have a drawl), you speak in a slow way, maaakiiing the voowweeel sounds loooongeer thaan noormaal.
Some people think this sounds lazy, but I think it sounds kind of nice and relaxed.
Some regional accents, like Texan and some Australian accents, have a drawl to them.
“He was the first US President who spoke with that Texan drawl .”
“Welcome to cowboy country,” he drawled .
Grrrrrrrrrrrrrr!
That’s my impression of a dog there.
I was growling.
If you ever go cycling around remote Bulgarian villages, then you’re probably quite familiar with this sound.
There are dogs everywhere, and sometimes they just bark.
But sometimes, before barking, they growl — they make that low, threatening, throaty sound.
And it means “stay away.”
But people can growl, too, especially if they want to be threatening.
“‘Stay away from my family!’ he growled .”
Using speaking verbs as nouns
We can use these speaking verbs in the same way we use “say.”
For example, if someone says “Get out!” loudly, we can say:
“‘Get out!’ he shouted .”
However, most of the verbs we looked at today are also used as nouns. (You might have noticed in some of the examples.)
For example, if we want to focus on the fact that he was angry when he shouted, and not the words he used, we can say:
“He gave a shout of anger.”
We can use these nouns with various verbs, usually “ give ” or “ let out .”
“She gave a shout of surprise.”
“He let out a bellow of laughter.”
“I heard a faint murmur through the door.”
There you have it: 30 alternatives to “say.”
So next time you’re describing your favourite TV show or talking about the dramatic argument you saw the other day, you’ll be able to describe it more colourfully and expressively.
Did you like this post? Then be awesome and share by clicking the blue button below.
8 thoughts on “ Words for Speaking: 30 Speech Verbs in English (With Audio) ”
Always enlighten and fun.. thank you
Great job! Thank you so much for sharing with us. My students love your drawing and teaching very much. So do I of course.
Good news: I found more than 30 verbs for “speaking”. Bad news, only four of them were in your list. That is to say “Good news I’m only 50 I still have plenty of time to learn new things, bad news I’m already 50 and still have so much learn. Thanks for your posts, they’re so interesting and useful!
Excellent. Can I print it?
Thanks Iris.
And yes — Feel free to print it! 🙂
Thanks so much! It was very interesting and helpful❤
Great words, shouts and barks, Gabriel. I’m already writing them down, so I can practise with them bit by bit. Thanks for the lesson!
Thank you so much for sharing with us. .It is very useful
Leave a Reply Cancel reply
Your email address will not be published. Required fields are marked *
Learn New Words 5 Times Faster
Subscribe to our newsletter to get the eBook free!

We only use strictly necessary cookies for this website. Please see the privacy policy for more information. Got it!
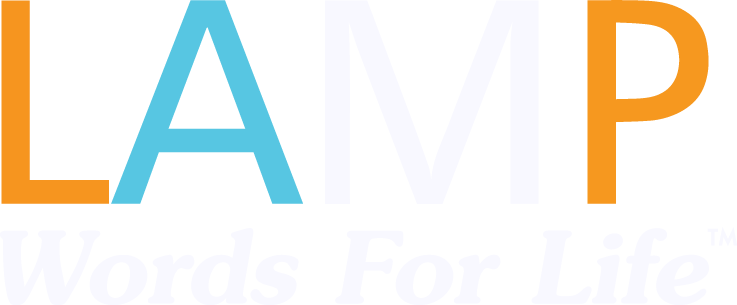
Our phone numbers starting with "800" are currently down. Please use our local numbers starting in "330" instead (Main Number: 330-262-1984, Service: 330-262-0845). We apologize for the inconvenience.
100 High Frequency Core Word List
100 Frequently Used Core Word Starter Set
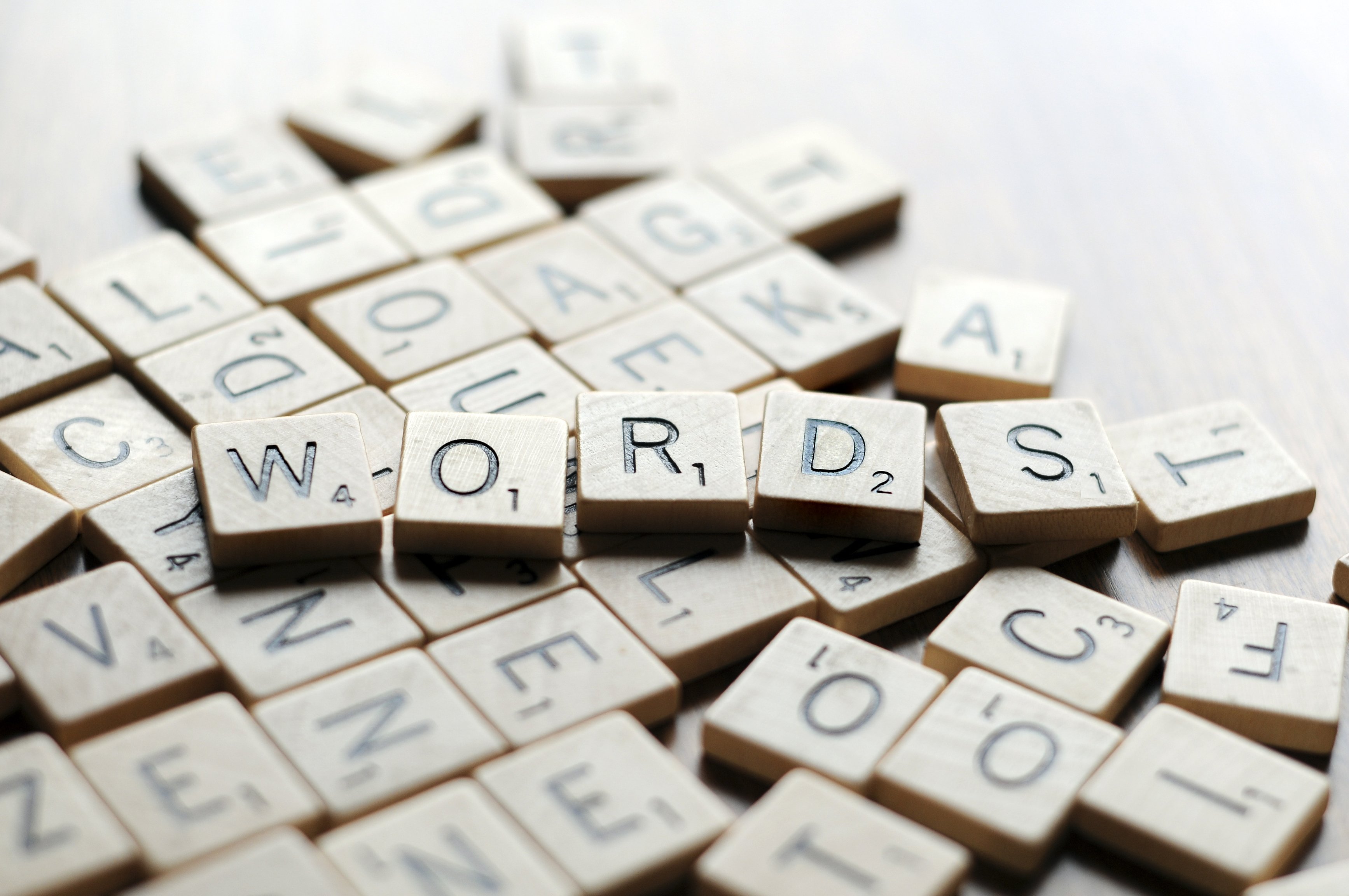
Materials
- 100 High Frequency Word List
- Unity 28 Smart Charts - 100
- Unity 36 Smart Charts - 100
- Unity 45 Smart Charts - 100
- Unity 60 Smart Charts - 100
- LAMP WFL VI Smart Charts - 100
- LAMP Words for Life 84 Smart Charts - 100
- Unity 84 Smart Charts - 100
- WordPower 42 Basic Smart Charts - 100
- WordPower 60 Basic Smart Charts - 100
- WordPower 60 Smart Charts - 100
- WordPower 80 Smart Charts - 100
- WordPower 108 Smart Charts - 100
core word list
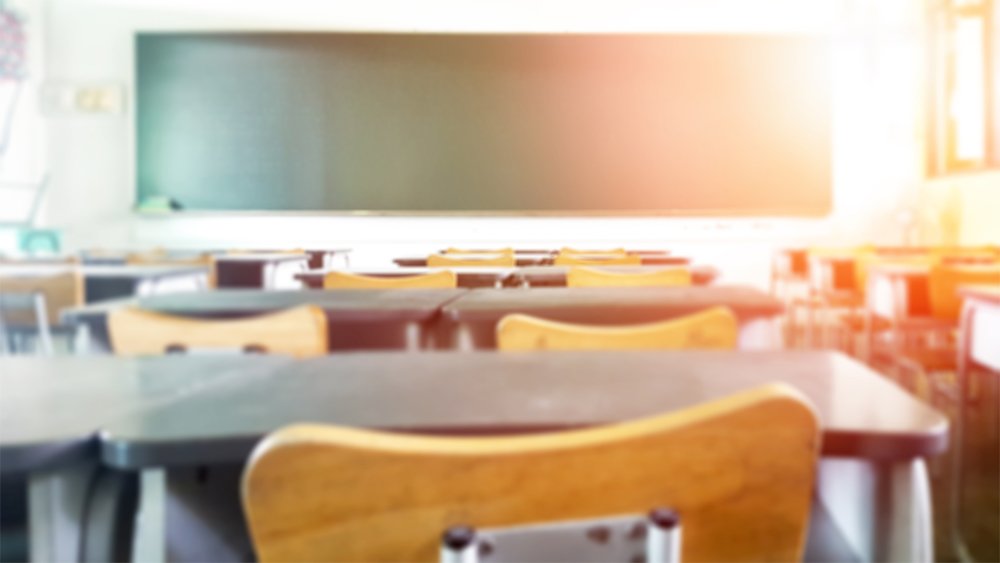
Lesson Plans
View all lesson plans
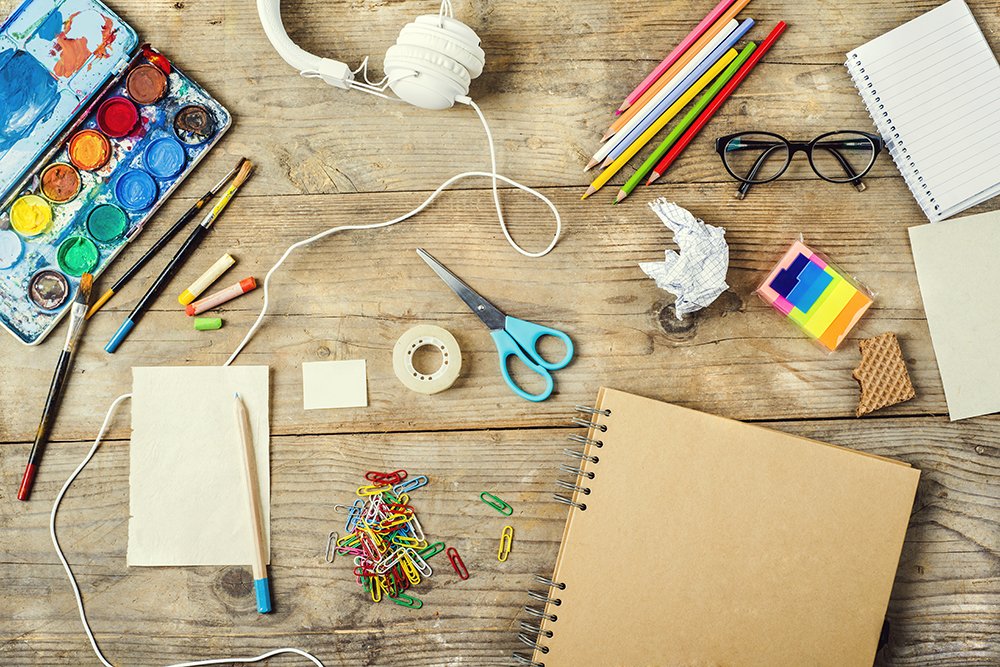
Activities
View all activities
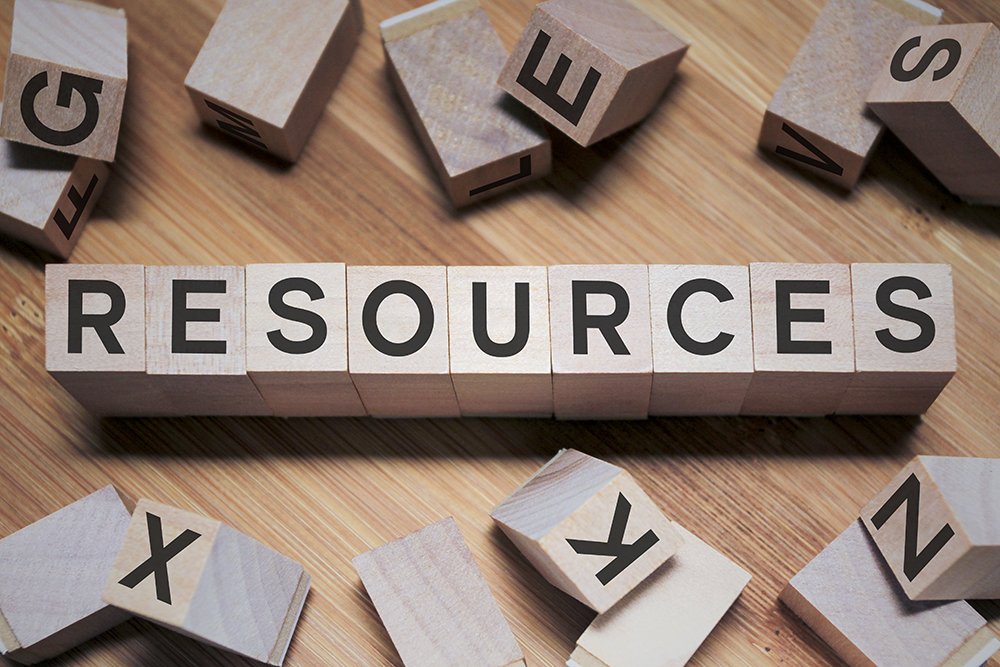
Resources
View all Resources
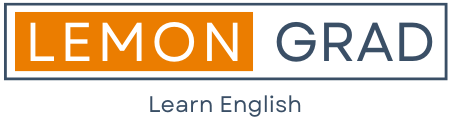
How to Build Vocabulary You Can Actually Use in Speech and Writing?
- Updated on Nov 12, 2023

- shares
This post comes from my experience of adding more than 8,000 words and phrases to my vocabulary in a way that I can actually use them on the fly in my speech and writing. Some words, especially those that I haven’t used for long time, may elude me, but overall the recall & use works quite well.
That’s why you build vocabulary, right? To use in speech and writing. There are no prizes for building list of words you can’t use. (The ultimate goal of vocabulary-building is to use words in verbal communication where you’ve to come up with an appropriate word in split second. It’s not to say that it’s easy to come up with words while writing, but in writing you can at least afford to think.)
This post also adopts couple of best practices such as
- Spaced repetition,
- Deliberate Practice,
- Begin with end in mind, and
- Build on what you already know
In this post, you’ll learn how you too can build such vocabulary, the one you can actually use. However, be warned. It’s not easy. It requires consistent work. But the rewards are more than worth the squeeze.
Since building such vocabulary is one of the most challenging aspects of English Language, you’ll stand out in crowd when you use precise words and, the best part, you can use this sub-skill till you’re in this world, long after you retire professionally. (Doesn’t this sound so much better when weighed against today’s reality where most professional skills get outdated in just few years?)
You may have grossly overestimated the size of your vocabulary
Once your understand the difference between active and passive vocabulary, you’ll realize that size of your vocabulary isn’t what you think it to be.
Active vs. Passive vocabulary
Words that you can use in speech and writing constitute your active vocabulary (also called functional vocabulary). You, of course, understand these words while reading and listening as well. Think of words such as eat , sell , drink , see , and cook .
But how about words such as munch , outsmart , salvage , savagery , and skinny ? Do you use these words regularly while speaking and writing? Unlikely. Do you understand meaning of these words while reading and listening? Highly likely. Such words constitute your passive vocabulary (also called recognition vocabulary). You can understand these words while reading and listening, but you can’t use them while speaking and writing.
Your active vocabulary is a tiny subset of your passive vocabulary:

(While the proportion of the two inner circles – active and passive vocabulary – bears some resemblance to reality, the outer rectangle is not proportionate because of paucity of space. In reality, the outer rectangle is much bigger, representing hundreds of thousands of words.)
Note : Feel free to use the above and other images in the post, using the link of this post for reference/attribution.
Many mistakenly believe that they’ve strong vocabulary because they can understand most words when reading and listening. But the real magic, the real use of vocabulary is when you use words in speech and writing. If you evaluate your vocabulary against this yardstick – active vs. passive – your confidence in your vocabulary will be shaken.
Why build vocabulary – a small exercise?
You would be all too aware of cases where people frequently pause while speaking because they can’t think of words for what they want to say. We can easily spot such extreme cases.
What we fail to spot, however, are less extreme, far more common cases where people don’t pause, but they use imprecise words and long-winding explanations to drive their message.
The bridge was destroyed (or broken) by the flooded river.
The bridge was washed away by the flooded river.
Although both convey the message, the second sentence stands out because of use of precise phrase.
What word(s) best describe what’s happening in the picture below?

Image source
Not the best response.
A better word is ‘emptied’. Even ‘dumped’ is great.
A crisp description of the above action would be: “The dumper emptied (or dumped) the stones on the roadside.”
What about this?

‘Took out grapes’.
‘Plucked grapes’ is far better.
If you notice, these words – wash away , empty , dump , and pluck – are simple. We can easily understand them while reading and listening, but rarely use them (with the possible exception of empty ) in speech or writing. Remember, active vs. passive vocabulary?
If you use such precise words in your communication you’ll stand out in crowd.
Little wonder, studies point to a correlation between strength of vocabulary and professional success. Earl Nightingale, a renowned self-help expert and author, in his 20-year study of college graduates found :
Without a single exception, those who had scored highest on the vocabulary test given in college, were in the top income group, while those who had scored the lowest were in the bottom income group.
He also refers to a study by Johnson O’Connor, an American educator and researcher, who gave vocabulary tests to executive and supervisory personnel in 39 large manufacturing companies. According to this study:
Presidents and vice presidents averaged 236 out of a possible 272 points; managers averaged 168; superintendents, 140; foremen, 114; floor bosses, 86. In virtually every case, vocabulary correlated with executive level and income.
Though there are plenty of studies linking professional success with fluency in English overall, I haven’t come across any study linking professional success with any individual component – grammar and pronunciation, for example – of English language other than vocabulary.
You can make professional success a motivation to improve your active vocabulary.
Let’s dive into the tactics now.
How to build vocabulary you can use in speech and writing?
(In the spirit of the topic of this section, I’ve highlighted words that I’ve shifted from my passive to active vocabulary in red font . I’ve done this for only this section, lest the red font become too distracting.)
Almost all of us build vocabulary through the following two-step process:
Step 1 : We come across new words while reading and listening. Meanings of many of these words get registered in our brains – sometimes vaguely, sometimes precisely – through the context in which we see these words. John Rupert Firth, a leading figure in British linguistics during the 1950s, rightly said , “You shall know a word by the company it keeps.”
Many of these words then figure repeatedly in our reading and listening and gradually, as if by osmosis , they start taking roots in our passive vocabulary.
Step 2 : We start using some of these words in our speech and writing. (They are, as discussed earlier, just a small fraction of our passive vocabulary.) By and large, we stay in our comfort zones, making do with this limited set of words.
Little wonder, we add to our vocabulary in trickle . In his book Word Power Made Easy , Norman Lewis laments the tortoise-like rate of vocabulary-building among adults:
Educational testing indicates that children of ten who have grown up in families in which English is the native language have recognition [passive] vocabularies of over twenty thousand words. And that these same ten-year-olds have been learning new words at a rate of many hundreds a year since the age of four . In astonishing contrast, studies show that adults who are no longer attending school increase their vocabularies at a pace slower than twenty-five to fifty words annually .
Adults improve passive vocabulary at an astonishingly meagre rate of 25-50 words a year. The chain to acquire active vocabulary is getting broken at the first step itself – failure to read or listen enough (see Step 1 we just covered). Most are not even reaching the second step, which is far tougher than the first. Following statistic from National Spoken English Skills Report by Aspiring Minds (sample of more than 30,000 students from 500+ colleges in India) bears this point:

Only 33 percent know such simple words! They’re not getting enough inputs.
Such vocabulary-acquisition can be schematically represented as:

The problem here is at both the steps of vocabulary acquisition:
- Not enough inputs (represented by funnel filled only little) and
- Not enough exploration and use of words to convert inputs into active vocabulary (represented by few drops coming out of the funnel)
Here is what you can do to dramatically improve your active vocabulary:
1. Get more inputs (reading and listening)
That’s a no-brainer. The more you read,
- the more new words you come across and
- the more earlier-seen words get reinforced
If you’ve to prioritize between reading and listening purely from the perspective of building vocabulary, go for more reading, because it’s easier to read and mark words on paper or screen. Note that listening will be a more helpful input when you’re working on your speaking skills .
So develop the habit to read something 30-60 minutes every day. It has benefits far beyond just vocabulary-building .
If you increase your inputs, your vocabulary-acquisition funnel will look something like:
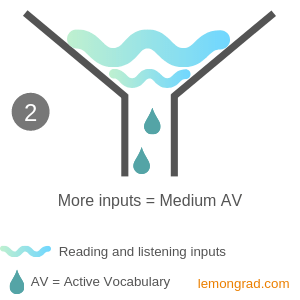
More inputs but no other steps result in larger active vocabulary.
2. Gather words from your passive vocabulary for deeper exploration
The reading and listening you do, over months and years, increase the size of your passive vocabulary. There are plenty of words, almost inexhaustible, sitting underutilized in your passive vocabulary. Wouldn’t it be awesome if you could move many of them to your active vocabulary? That would be easier too because you don’t have to learn them from scratch. You already understand their meaning and usage, at least to some extent. That’s like plucking – to use the word we’ve already overused – low hanging fruits.
While reading and listening, note down words that you’re already familiar with, but you don’t use them (that is they’re part of your passive vocabulary). We covered few examples of such words earlier in the post – pluck , dump , salvage , munch , etc. If you’re like most, your passive vocabulary is already large, waiting for you to shift some of it to your active vocabulary. You can also note down completely unfamiliar words, but only in exceptional cases.
To put what I said in the previous paragraph in more concrete terms, you may ask following two questions to decide which words to note down for further exploration:
- Do you understand the meaning of the word from the context of your reading or listening?
- Do you use this word while speaking and writing?
If the answer is ‘yes’ to the first question and ‘no’ to the second, you can note down the word.
3. Explore the words in an online dictionary
Time to go a step further than seeing words in context while reading.
You need to explore each word (you’ve noted) further in a dictionary. Know its precise meaning(s). Listen to pronunciation and speak it out loud, first individually and then as part of sentences. (If you’re interested in the topic of pronunciation, refer to the post on pronunciation .) And, equally important, see few sentences where the word has been used.
Preferably, note down the meaning(s) and few example sentences so that you can practice spaced repetition and retain them for long. Those who do not know what spaced repetition is, it is the best way to retain things in your long-term memory . There are number of options these days to note words and other details about them – note-taking apps and good-old word document. I’ve been copying-pasting on word document and taking printouts. For details on how I practiced spaced repetition, refer to my experience of adding more than 8,000 words to my vocabulary.
But why go through the drudgery of noting down – and going through, probably multiple times – example sentences? Why not just construct sentences straight after knowing the meaning of the word?
Blachowicz, Fisher, Ogle, and Watts-Taffe, in their paper , point out the yawning gap between knowing the meaning of words and using them in sentences:
Research suggests that students are able to select correct definitions for unknown words from a dictionary, but they have difficulty then using these words in production tasks such as writing sentences using the new words.
If only it was easy. It’s even more difficult in verbal communication where, unlike in writing, you don’t have the luxury of pausing and recalling appropriate words.
That’s why you need to focus on example sentences.
Majority of those who refer dictionary, however, restrict themselves to meaning of the word. Few bother to check example sentences. But they’re at least as much important as meaning of the word, because they teach you how to use words in sentences, and sentences are the building blocks of speech and writing.
If you regularly explore words in a dictionary, your vocabulary-acquisition funnel will look something like:

More inputs combined with exploration of words result in even larger active vocabulary.
After you absorb the meaning and example sentences of a word, it enters a virtuous cycle of consolidation. The next time you read or listen the word, you’ll take note of it and its use more actively , which will further reinforce it in your memory. In contrast, if you didn’t interact with the word in-depth, it’ll pass unnoticed, like thousands do every day. That’s cascading effect.
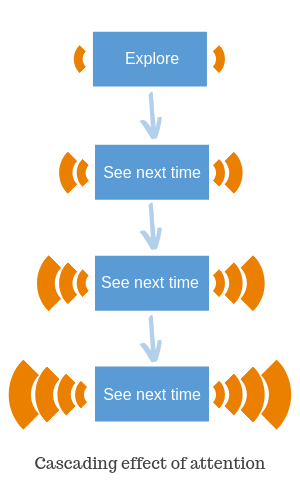
Participate in a short survey
If you’re a learner or teacher of English language, you can help improve website’s content for the visitors through a short survey.
4. Use them
To quote Maxwell Nurnberg and Morris Rosenblum from their book All About Words :
In vocabulary building, the problem is not so much finding new words or even finding out what they mean. The problem is to remember them, to fix them permanently in your mind. For you can see that if you are merely introduced to words, you will forget them as quickly as you forget the names of people you are casually introduced to at a crowded party – unless you meet them again or unless you spend some time with them.
This is the crux. Use it or lose it.
Without using, the words will slowly slip away from your memory.
Without using the words few times, you won’t feel confident using them in situations that matter.
If you use the words you explored in dictionary, your vocabulary-acquisition funnel will look something like:
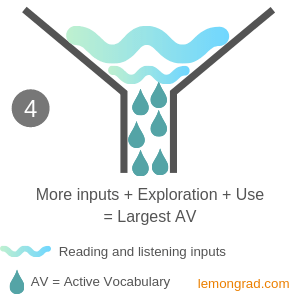
More inputs combined with exploration of words and use of them result in the largest active vocabulary.
Here is a comparison of the four ways in which people acquire active vocabulary:

The big question though is how to use the words you’re exploring. Here are few exercises to accomplish this most important step in vocabulary-building process.
Vocabulary exercises: how to use words you’re learning
You can practice these vocabulary activities for 10-odd minutes every day, preferably during the time you waste such as commuting or waiting, to shift more and more words you’ve noted down to your active vocabulary. I’ve used these activities extensively, with strong results to boot.
1. Form sentences and speak them out during your reviews
When you review the list of words you’ve compiled, take a word as cue without looking at its meaning and examples, recall its meaning, and, most importantly, speak out 4-5 sentences using the word. It’s nothing but a flashcard in work. If you follow spaced repetition diligently, you’ll go through this process at least few times. I recommend reading my experience of building vocabulary (linked earlier) to know how I did this part.
Why speaking out, though? (If the surroundings don’t permit, it can be whisper as well.)
Speaking out the word as part of few sentences will serve the additional purpose of making your vocal cords accustomed to new words and phrases.
2. Create thematic webs
When reviewing, take a word and think of other words related to that word. Web of words on a particular theme, in short, and hence the name ‘thematic web’. These are five of many, many thematic webs I’ve actually come up in my reviews:
(Note: Name of the theme is in bold. Second, where there are multiple words, I’ve underlined the main word.)
If I come across the word ‘gourmet’ in my review, I’ll also quickly recall all the words related with food: tea strainer, kitchen cabinet, sink, dish cloth, wipe dishes, rinse utensils, immerse beans in water, simmer, steam, gourmet food, sprinkle salt, spread butter, smear butter, sauté, toss vegetables, and garnish the sweet dish
Similarly, for other themes:
Prognosis, recuperate, frail, pass away, resting place, supplemental air, excruciating pain, and salubrious
C. Showing off
Showy, gaudy, extravaganza, over the top, ostentatious, and grandstanding
D. Crowd behavior
Restive, expectant, hysteria, swoon, resounding welcome, rapturous, jeer, and cheer
E. Rainfall
Deluge, cats and dogs, downpour, cloudburst, heavens opened, started pouring , submerged, embankment, inundate, waterlogged, soaked to the skin, take shelter, run for a cover, torrent, and thunderbolt
(If you notice, words in a particular theme are much wider in sweep than just synonyms.)
It takes me under a minute to complete dozen-odd words in a theme. However, in the beginning, when you’re still adding to your active vocabulary in tons, you’ll struggle to go beyond 2-3 simple words when thinking out such thematic lists. That’s absolutely fine.
Why thematic web, though?
Because that’s how we recall words when speaking or writing. (If you flip through Word Power Made Easy by Norman Lewis, a popular book on improving vocabulary, you’ll realize that each of its chapters represents a particular idea, something similar to a theme.) Besides, building a web also quickly jogs you through many more words.
3. Describe what you see around
In a commute or other time-waster, look around and speak softly an apt word in a split second for whatever you see. Few examples:
- If you see grass on the roadside, you can say verdant or luxurious .
- If you see a vehicle stopping by the roadside, you can say pull over .
- If you see a vehicle speeding away from other vehicles, you can say pull away .
- If you see a person carrying a load on the road side, you can say lug and pavement .
Key is to come up with these words in a flash. Go for speed, not accuracy. (After all, you’ll have similar reaction time when speaking.) If you can’t think of an appropriate word for what you see instantaneously – and there will be plenty in the beginning – skip it.
This vocabulary exercise also serves an unintended, though important, objective of curbing the tendency to first think in the native language and then translating into English as you speak. This happens because the spontaneity in coming up with words forces you to think directly in English.
Last, this exercise also helps you assess your current level of vocabulary (for spoken English). If you struggle to come up with words for too many things/ situations, you’ve job on your hands.
4. Describe what one person or object is doing
Another vocabulary exercise you can practice during time-wasters is to focus on a single person and describe her/ his actions, as they unfold, for few minutes. An example:
He is skimming Facebook on his phone. OK, he is done with it. Now, he is taking out his earphones. He has plugged them into his phone, and now he is watching some video. He is watching and watching. There is something funny there in that video, which makes him giggle . Simultaneously, he is adjusting the bag slung across his shoulder.
The underlined words are few of the new additions to my active vocabulary I used on the fly when focusing on this person.
Feel free to improvise and modify this process to suit your unique conditions, keeping in mind the fundamentals such as spaced repetition, utilizing the time you waste, and putting what you’re learning to use.
To end this section, I must point out that you need to build habit to perform these exercises for few minutes at certain time(s) of the day. They’re effective when done regularly.
Why I learnt English vocabulary this way?
For few reasons:
1. I worked backwards from the end result to prepare for real-world situations
David H. Freedman learnt Italian using Duolingo , a popular language-learning app, for more than 70 hours in the buildup to his trip to Italy. A week before they were to leave for Rome, his wife put him to test. She asked how would he ask for his way from Rome airport to the downtown. And how would he order in a restaurant?
David failed miserably.
He had become a master of multiple-choice questions in Italian, which had little bearing on the real situations he would face.
We make this mistake all the time. We don’t start from the end goal and work backwards to design our lessons and exercises accordingly. David’s goal wasn’t to pass a vocabulary test. It was to strike conversation socially.
Coming back to the topic of vocabulary, learning meanings and examples of words in significant volume is a challenge. But a much bigger challenge is to recall an apt word in split second while speaking. (That’s the holy grail of any vocabulary-building exercise, and that’s the end goal we want to achieve.)
The exercises I described earlier in the post follow the same path – backwards from the end.
2. I used proven scientific methods to increase effectiveness
Looking at just a word and recalling its meaning and coming up with rapid-fire examples where that word can be used introduced elements of deliberate practice, the fastest way to build neural connection and hence any skill. (See the exercises we covered.) For the uninitiated, deliberate practice is the way top performers in any field practice .
Another proven method I used was spaced repetition.
3. I built on what I already knew to progress faster
Covering mainly passive vocabulary has made sure that I’m building on what I already know, which makes for faster progress.
Don’t ignore these when building vocabulary
Keep in mind following while building vocabulary:
1. Use of fancy words in communication make you look dumb, not smart
Don’t pick fancy words to add to your vocabulary. Use of such words doesn’t make you look smart. It makes your communication incomprehensible and it shows lack of empathy for the listeners. So avoid learning words such as soliloquy and twerking . The more the word is used in common parlance, the better it is.
An example of how fancy words can make a piece of writing bad is this review of movie , which is littered with plenty of fancy words such as caper , overlong , tomfoolery , hectoring , and cockney . For the same reason, Shashi Tharoor’s Word of the Week is not a good idea . Don’t add such words to your vocabulary.
2. Verbs are more important than nouns and adjectives
Verbs describe action, tell us what to do. They’re clearer. Let me explain this through an example.
In his book Start with Why , Simon Sinek articulates why verbs are more effective than nouns:
For values or guiding principles to be truly effective they have to be verbs. It’s not ‘integrity’, it’s ‘always do the right thing’. It’s not ‘innovation’, it’s ‘look at the problem from a different angle’. Articulating our values as verbs gives us a clear idea… we have a clear idea of how to act in any situation.
‘Always do the right thing’ is better than ‘integrity’ and ‘look at the problem from a different angle’ is better than ‘innovation’ because the former, a verb, in each case is clearer.
The same (importance of verb) is emphasized by L. Dee Fink in his book Creating Significant Learning Experiences in the context of defining learning goals for college students.
Moreover, most people’s vocabulary is particularly poor in verbs. Remember, the verbs from the three examples at the beginning of the post – wash away , dump , and pluck ? How many use them? And they’re simple.
3. Don’t ignore simple verbs
You wouldn’t bother to note down words such as slip , give , and move because you think you know them inside out, after all you’ve been using them regularly for ages.
I also thought so… until I explored few of them.
I found that majority of simple words have few common usages we rarely use. Use of simple words for such common usages will stand your communication skills out.
An example:
a. To slide suddenly or involuntarily as on a smooth surface: She slipped on the icy ground .
b. To slide out from grasp, etc.: The soap slipped from my hand .
c. To move or start gradually from a place or position: His hat slipped over his eyes .
d. To pass without having been acted upon or used: to let an opportunity slip .
e. To pass quickly (often followed by away or by): The years slipped by .
f. To move or go quietly, cautiously, or unobtrusively: to slip out of a room .
Most use the word in the meaning (a) and (b), but if you use the word for meaning (c) to (f) – which BTW is common – you’ll impress people.
Another example:
a. Without the physical presence of people in control: an unmanned spacecraft .
b. Hovering near the unmanned iPod resting on the side bar, stands a short, blond man.
c. Political leaders are vocal about the benefits they expect to see from unmanned aircraft.
Most use the word unmanned with a moving object such as an aircraft or a drone, but how about using it with an iPod (see (b) above).
4. Don’t ignore phrasal verbs. Get at least common idioms. Proverbs… maybe
4.1 phrasal verbs.
Phrasal verbs are verbs made from combining a main verb and an adverb or preposition or both. For example, here are few phrasal verbs of verb give :
We use phrasal verbs aplenty:
I went to the airport to see my friend off .
He could see through my carefully-crafted ruse.
I took off my coat.
The new captain took over the reins of the company on June 25.
So, don’t ignore them.
Unfortunately, you can’t predict the meaning of a phrasal verb from the main verb. For example, it’s hard to guess the meaning of take over or take off from take . You’ve to learn each phrasal verb separately.
What about idioms?
Compared to phrasal verbs, idioms are relatively less used, but it’s good to know the common ones. To continue the example of word give , here are few idioms derived from it:
Give and take
Give or take
Give ground
Give rise to
Want a list of common idioms? It’s here: List of 200 common idioms .
4.3 Proverbs
Proverbs are popular sayings that provide nuggets of wisdom. Example: A bird in hand is worth two in the bush.
Compared to phrasal verbs and idioms, they’re much less used in common conversation and therefore you can do without them.
For the motivated, here is a list of common proverbs: List of 200 common proverbs .
5. Steal phrases, words, and even sentences you like
If you like phrases and sentences you come across, add them to your list for future use. I do it all the time and have built a decent repository of phrases and sentences. Few examples (underlined part is the key phrase):
The bondholders faced the prospect of losing their trousers .
The economy behaved more like a rollercoaster than a balloon . [Whereas rollercoaster refers to an up and down movement, balloon refers to a continuous expansion. Doesn’t such a short phrase express such a profound meaning?]
Throw enough spaghetti against the wall and some of it sticks .
You need blue collar work ethic to succeed in this industry.
He runs fast. Not quite .
Time to give up scalpel . Bring in hammer .
Note that you would usually not find such phrases in a dictionary, because dictionaries are limited to words, phrasal verbs, idioms, and maybe proverbs.
6. Commonly-used nouns
One of my goals while building vocabulary has been to learn what to call commonly-used objects (or nouns) that most struggle to put a word to.

To give an example, what would you call the following?
Answer: Tea strainer.
You would sound far more impressive when you say, “My tea strainer has turned blackish because of months of filtering tea.”
Than when you say, “The implement that filters tea has turned blackish because of months of filtering tea.”
What do you say?
More examples:
Saucer (We use it every day, but call it ‘plate’.)
Straight/ wavy/ curly hair
Corner shop
I’ll end with a brief reference to the UIDAI project that is providing unique biometric ID to every Indian. This project, launched in 2009, has so far issued a unique ID (popularly called Aadhaar card) to more than 1.1 billion people. The project faced many teething problems and has been a one big grind for the implementers. But once this massive data of billion + people was collected, so many obstinate, long-standing problems are being eased using this data, which otherwise would’ve been difficult to pull off. It has enabled faster delivery of scores of government and private services, checked duplication on many fronts, and brought in more transparency in financial and other transactions, denting parallel economy. There are many more. And many more are being conceived on top of this data.
At some level, vocabulary is somewhat similar. It’ll take effort, but once you’ve sizable active vocabulary, it’ll strengthen arguably the most challenging and the most impressive part of your communication. And because it takes some doing, it’s not easy for others to catch up.
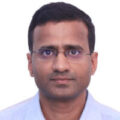
Anil is the person behind content on this website, which is visited by 3,000,000+ learners every year. He writes on most aspects of English Language Skills. More about him here:
Such a comprehensive guide. Awesome…
I am using the note app and inbuilt dictionary of iPhone. I have accumulated over 1400 words in 1 year. Will definitely implement ideas from this blog.
Krishna, thanks. If you’re building vocabulary for using, then make sure you work it accordingly.
Building solid vocabulary is my new year’s resolution and you’ve perfectly captured the issues I’ve been facing, with emphasis on passive vocabulary building. So many vocab apps are multiple choice and thereby useless for this reason. Thanks so much for the exercises! I plan to put them to use!
It was everything that I need to boost my active vocabulary. Thank you so much for sharing all these precious pieces of information.
Anil sir, I am quiet satisfied the way you laid out everything possible that one needs to know from A-Z. Also, thanks for assuring me from your experience that applying this will work.
This post definitely blew me away…. I am impressed! Thank you so much for sharing such valuable information. It was exactly what I needed!
Amazing post! While reading this post, I am thinking about the person who developed this. I wanna give a big hug and thank you so much.
Comments are closed.
Any call to action with a link here?

30 Vocabulary Goals for Speech Therapy (Based on Research)
Need some ideas for vocabulary goals for speech therapy? If you’re feeling stuck, keep on reading! In this post, I’ll provide some suggestions you could use for writing iep goals for vocabulary and semantics. This blog post provides a list of vocabulary-based iep goals that should be modified for each individual student. They can serve as a way to get ideas flowing! Not only that, but I’ll also share some strategies for vocabulary intervention. Vocabulary skills are an important skill to work on in speech therapy!

Goal Bank of Ideas
If you’re a school speech pathologist, then you know you’re going to have a huge pile of paperwork!
We have a lot going on, and it can be helpful to have a suggested list of vocabulary goals that you can modify in order to meet the needs of your students.
Many times, we know what we need to write a goal for, but finding the right wording can be tricky.
Needless to say, it can be very helpful to have a goal bank that can provide a starting point for ideas. *** Please note, the article linked in this paragraph is a general goal bank- keep scrolling for vocabulary-specific goals!
Please note, the goals in the goal bank are just that: ideas. We must always, of course, write goals that are individualized to our students . Which isn’t easy, and takes a lot of your SLP knowledge and expertise into account!
How to Write Measurable IEP Goals
It’s very helpful to learn the SMART framework for writing specific and measurable IEP goals . There are some CEU courses available for SLPs. This ceu course discusses writing SMARTer goals. Likewise, this course also discusses IEP goal writing.
SMART stands for:
Learn more about the SMART framework here .
Reference: Diehm, Emily. “Writing Measurable and Academically Relevant IEP Goals with 80% Accuracy over Three Consecutive Trials.” Perspectives of the ASHA Special Interest Groups , vol. 2, no. 16, 2017, pp. 34–44., https://doi.org/10.1044/persp2.sig16.34.
Reference: staff, n2y. “Tips for Writing and Understanding Smart Iep Goals: N2Y Blog.” n2y , 22 Feb. 2021, https://www.n2y.com/blog/smart-iep-goals/.
Target Vocabulary Words: Where to Start
It can be tricky to know where to begin when it comes to vocabulary intervention! However, vocabulary practice is important!
The first step for some children may be learning core vocabulary . If your student needs to work on functional communication, this is a great place to start. I like to teach core vocabulary during play or throughout a child’s school day.
Both younger children and older children, however, will greatly benefit from exposure and explicit instruction to a variety of Tier II vocabulary words.
What are Tier II vocabulary words? These are words that are used by more advanced language users, and they can be used across a variety of contexts. An example of a tier II vocabulary word is ‘observe’. Research tells us that Tier II vocabulary words are exceptionally important for reading comprehension.
Speech-language pathologists don’t need to wait until a child is older to work on Tier II vocabulary! Even preschool students can benefit from the exposure and explicit instruction during speech therapy sessions. A great activity for younger students might involve using picture books that contain tier II vocabulary words. Or, use a wordless book and the possibilities are endless!
Tier 1 vocabulary words are everyday words that your student likely has had a lot of exposure to naturally. The word ‘table’, for example, is a Tier 1 vocabulary word.
Tier III vocabulary words are domain-specific words. These could be the type of words that are taught during math or science.
References:
Beck, I. L., McKeown, M. G., & Kucan, L. (2002). Bringing words to life: Robust vocabulary instruction . New York, NY: The Guilford.
Boshart, Char. “Exploring Vocabulary Interventions and Activities From Preschool Through Adolescence”. . SpeechTherapyPD.com.
Vocabulary Strategies for Intervention
Need a great way to implement vocabulary instruction? How about 15 great ideas to encourage vocabulary knowledge and development? These best practices for vocabulary building skills are based on research and can be used with a preschool student, an elementary school student, or a middle school or high school student.
Your students with language disorders will no doubt benefit from vocabulary intervention. Vocabulary intervention, along with grammar and sentence structure intervention , is an important component of reading comprehension success.
Vocabulary intervention can- and should- be fun and meaningful. So don’t hesitate to read engaging books, break out a sensory bin, or play games! Check out this list of recommended board games for speech therapy .
15 Effective Vocabulary Strategies Based on Research
The following ways may be fun ways to incorporate vocabulary activities and vocabulary intervention into speech therapy sessions:
- Select a small number of tier II words to focus on during your session, perhaps 3-5.
- Don’t be afraid to repeat those words- repetition is important!
- Keep your student actively engaged. Engaged learners will retain more information!
- If reading a story aloud, stop and have active discussions. It’s okay to take lots of time to finish the story, even across consecutive sessions.
- Have your student say the word aloud multiple times- this is called “phonological rehearsal”.
- Have your student write out the vocabulary target word.
- Have your student draw a picture to explain the definition of the target word. Keep the picture card and collect them and review them.
- Make sure to explain the definition in child-friendly terms.
- Have your student generate their own sentence and definition using the vocabulary word.
- Act out the word’s meaning.
- Don’t forget about the importance of morphological awareness and knowledge. Discuss prefixes, suffixes, and word roots.
- Talk about word relationships, synonyms, antonyms, or multiple-meaning words.
- Discuss similarities and differences between targeted vocabulary words.
- Print out a picture of an object (to represent the target vocabulary word) and color it or paint it!
- Try concept mapping .

Robust is a must | The Informed SLP. (2023). Retrieved 19 March 2023, from https://www.theinformedslp.com/review/robust-is-a-must
Vocabulary intervention: Start here | The Informed SLP. (2023). Retrieved 19 March 2023, from https://www.theinformedslp.com/review/vocabulary-intervention-start-here
Vocabulary intervention for at-risk adolescents | The Informed SLP. (2023). Retrieved 19 March 2023, from https://www.theinformedslp.com/review/vocabulary-intervention-for-at-risk-adolescents
Speech Therapy Goals for Vocabulary and Semantics
Writing goals can be a tough task, but it is so important. Well-written goals and having a structured activity or interactive activity in mind can also be helpful for data collection.
Here are some vocabulary iep goals that a speech therapist might use to generate some ideas for a short-term goal! As a reminder, these are simply ideas. Think of this as an informal iep goal bank. A speech pathologist will modify as needed for an individual student!
Also, don’t hesitate to scroll back up to read about writing measurable goals (i.e. SMART goals). You will want to add information such as the level of accuracy, what types of cues (such as visual cues, or perhaps a verbal cue), and what level of cueing (i.e. minimal cues). Don’t forget how beneficial a graphic organizer can be while working on communication skills!
Vocabulary Goal Bank of Ideas
- using a total communication approach (which may include but is not limited to a communication device, communication board, signing, pictures, gestures, words, or word approximations), Student will imitate single words or simple utterances containing core vocabulary in order to…. (choose a pragmatic function: request, request assistance, describe the location or direction of objects, describe an action, etc.)
- using a total communication approach, generate simple sentences containing core vocabulary in order to… (choose a pragmatic function to finish the objective, such as direct the action of others, request, describe actions, etc.)
- label common objects or pictured objects (nouns)
- label pictured actions (verbs)
- answer basic wh questions to demonstrate comprehension of basic concepts related to…. (location, quantity, quality, time)
- generate semantically and syntactically correct spoken or written sentences for targeted tier II vocabulary words
- use a target tier II vocabulary word in a novel spoken or written sentence
- provide synonyms for targeted vocabulary words
- provide antonyms for targeted vocabulary words
- provide at least two definitions for multiple-meaning vocabulary words
- provide a student-friendly definition for a targeted tier II vocabulary word (i.e. “explain in his own words”)
- identify unfamiliar key words during a read-aloud or structured language activities
- sort objects or pictured objects into piles based on the semantic feature (i.e. category, object function)
- label the category for a named object or pictured object
- state the object function (i.e. what it’s used for)
- describe the appearance of a given item or pictured item
- provide parts or associated parts for a named object or pictured object
- complete analogies related to semantic features (i.e. based on category- dog is to animal as chair is to… furniture)
- identify an item when provided with the category plus 1-2 additional semantic features
- explain similarities and differences between targeted items/ objects
- answer spoken or written questions related to temporal semantic relationships (i.e. time)
- answer spoken or written questions related to spatial semantic relationships (i.e. location)
- answer spoken or written questions related to comparative semantic relationships
- complete spoken or written sentences using appropriate spatial, temporal, or comparative vocabulary
- segment (or divide) words into morphological units (i.e. cats= cat / s)
- create new words by adding prefixes or suffixes to the base
- provide a definition for a targeted affix (prefix or suffix)
- sort words into piles based on targeted affix (prefix or suffix)
- finish a spoken or written analogy using targeted prefixes or suffixes (i.e. Regular is to irregular as responsible is to…)
- provide the part of speech for a targeted tier II vocabulary word (i.e. label it is as verb, adjective, etc.)
5 Recommended Vocabulary Activities for Speech Therapy
Need some ready-to-go vocabulary activities for those busy days? Here are some recommendations for school speech-language pathologists.
- Semantic Relationships Speech Therapy Worksheets
- Describing Digital Task Cards
- Analogy Worksheets
- Weather-Themed Morphology Activities for Speech Therapy
- Prefix and Suffix Worksheets for Speech Therapy

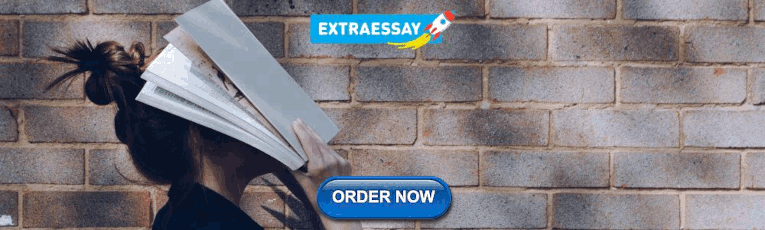
More Speech Therapy Goal Ideas
Are you in a hurry and need this article summed up? To see the vocabulary goals, simply scroll up.
Next, make sure to try out these best-selling vocabulary resources:
Finally, don’t miss these grammar goals for speech therapy .
Similar Posts

3 Easy Final Consonant Deletion Activities for Speech Therapy
If you are a speech language pathologist working with children who have articulation disorders or phonological disorders, chances are you’re always on the lookout for engaging final consonant deletion speech therapy activities! Keep reading, because this article provides suggestions for final consonant deletion activities, as well as some tips and tricks to try out during…
Here’s How I Teach Grammar & Sentence Structure in Speech Therapy
grammar and syntax speech therapy ideas
TH Words for Speech Therapy (Word Lists and Activities)
Need th word lists for speech therapy? Speech pathologists looking for a quick list of initial th words and final th target words to practice during speech therapy, make sure to bookmark this post. You’ll also find some great ideas for making therapy more fun with a variety of engaging games, articulation worksheets, and speech…

The Helpful List of Student Strengths and Weaknesses for IEP Writing
Are you a speech-language pathologist or intervention specialist looking for a list of student strengths and weaknesses for IEP writing? Speech-language pathologists and special education teachers are two examples of professionals who are responsible for the IEP writing process and iep paperwork. Writing an effective IEP is important- but not easy. This blog post provides…

The BEST Free Online Speech Therapy Games, Tools, and Websites
Speech therapists looking for free online speech therapy games will want to check out this blog post! This blog post contains a collection of interactive games and online resources that will be motivating for younger children AND older students! There are links to digital games and reinforcers, such as tic tac toe, online checkers, digital…

3 Practical ST Words Speech Therapy Activities
Are you a speech-language pathologist looking for st words speech therapy activities and word lists? Look no further! This article provides the definition of a phonological disorder, and explains what consonant cluster reduction is. SLPs will want to understand the difference between a phonological disorder and childhood apraxia of speech. Additionally, there are links to…

17 Best Vocabulary Goals for Speech Therapy + Activities
If you’re a speech therapist looking for a great list of vocabulary goals for speech therapy this blog post is for you!
I know what it’s like when you’re constantly trying to come up with iep goals and your brain is simply fried from the stressful workday and goal writing is the last thing you want to do. Okay or maybe ever?
I wanted to take writing goals off your to-do list! I wanted to turn this annoying and sometimes difficult task into a simple copy and paste. I mean who doesn’t love a good copy and paste option? Am I right?
Below is a list of smart goals that you can use for your vocabulary intervention and hopefully make your workday a little less stressful today.
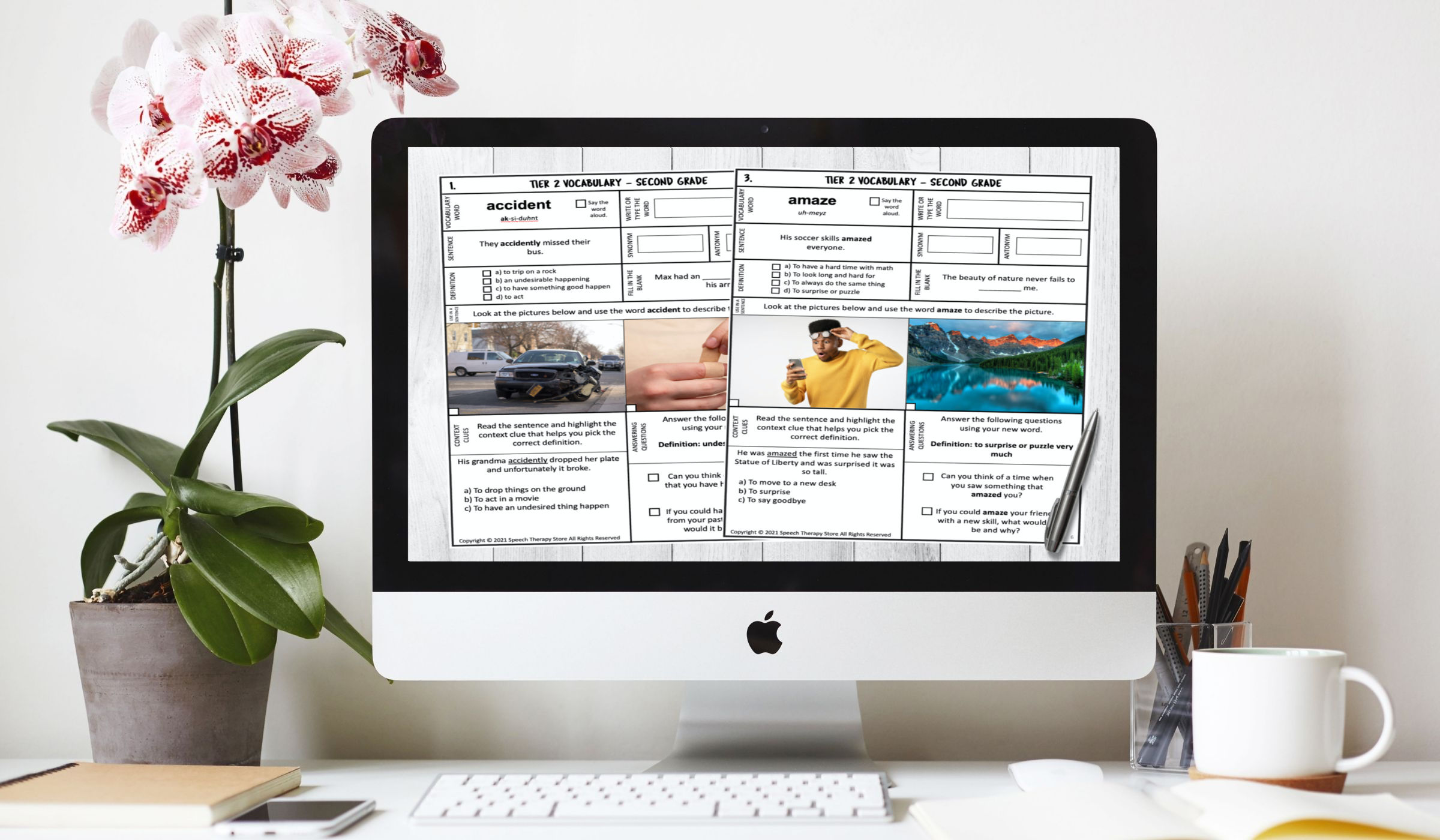
Speech Therapy Goals: Vocabulary
Pick your favorite measurable goal below to have your student start working on their specific communication disorders goal areas today.
Feel free to use any of the following as a long-term goal or break them up to use them as a short-term goal.
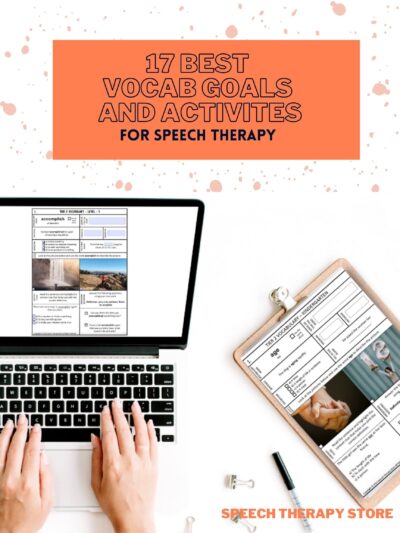
Expressive Language: Vocabulary Goals Speech Therapy
Visual cues.
Given 5 words with visual cues, STUDENT will define the word correctly with 80% accuracy in 4 out of 5 opportunities.
Common Objects or Visual Prompts
Given a common object or visual prompts, STUDENT will use 2-3 critical features to describe the object or picture with 80% accuracy in 4 out of 5 opportunities.
Use New Words
Given an emotional expression picture or story, STUDENT will use vocabulary to clearly describe the feelings, ideas, or experiences with 80% accuracy in 4 out of 5 opportunities.
Identify Similar Words – Synonyms and Antonyms
Given an object, picture, or word, STUDENT will identify synonyms with 80% accuracy in 4 out of 5 opportunities.
Given an object, picture, or word, STUDENT will identify antonyms with 80% accuracy in 4 out of 5 opportunities.
Given 5 identified words in sentences, STUDENT will provide a synonym/antonym with 80% accuracy in 4 out of 5 opportunities.
Given a story with highlighted words, STUDENT will provide a synonym/antonym for each highlighted word with 80% accuracy in 4 out of 5 opportunities.
Given 10 pictures, STUDENT will match opposite pictures in pairs (i.e., happy/sad, up/down) with 80% accuracy in 4 out of 5 opportunities.
Given an object, picture, or word, STUDENT will identify the opposite with 80% accuracy in 4 out of 5 opportunities.
Describe Target Words
Given an object or picture, STUDENT will describe the object or picture by naming the item , identify attributes (color, size, etc.), function , or number with 80% accuracy in 4 out of 5 opportunities.
Reading Passage and Context Clues
Given a reading task, STUDENT will define unfamiliar words using context clues with 80% accuracy in 4 out of 5 opportunities.
Academic: Target Vocabulary Words with Root Words
Given common academic vocabulary, STUDENT will define prefix and/or suffix with 80% accuracy in 4 out of 5 opportunities.
Correct Grammar and Complete Sentence
Given common academic vocabulary, STUDENT will define the vocabulary word using a complete sentence with correct grammar with 80% accuracy in 4 out of 5 opportunities.
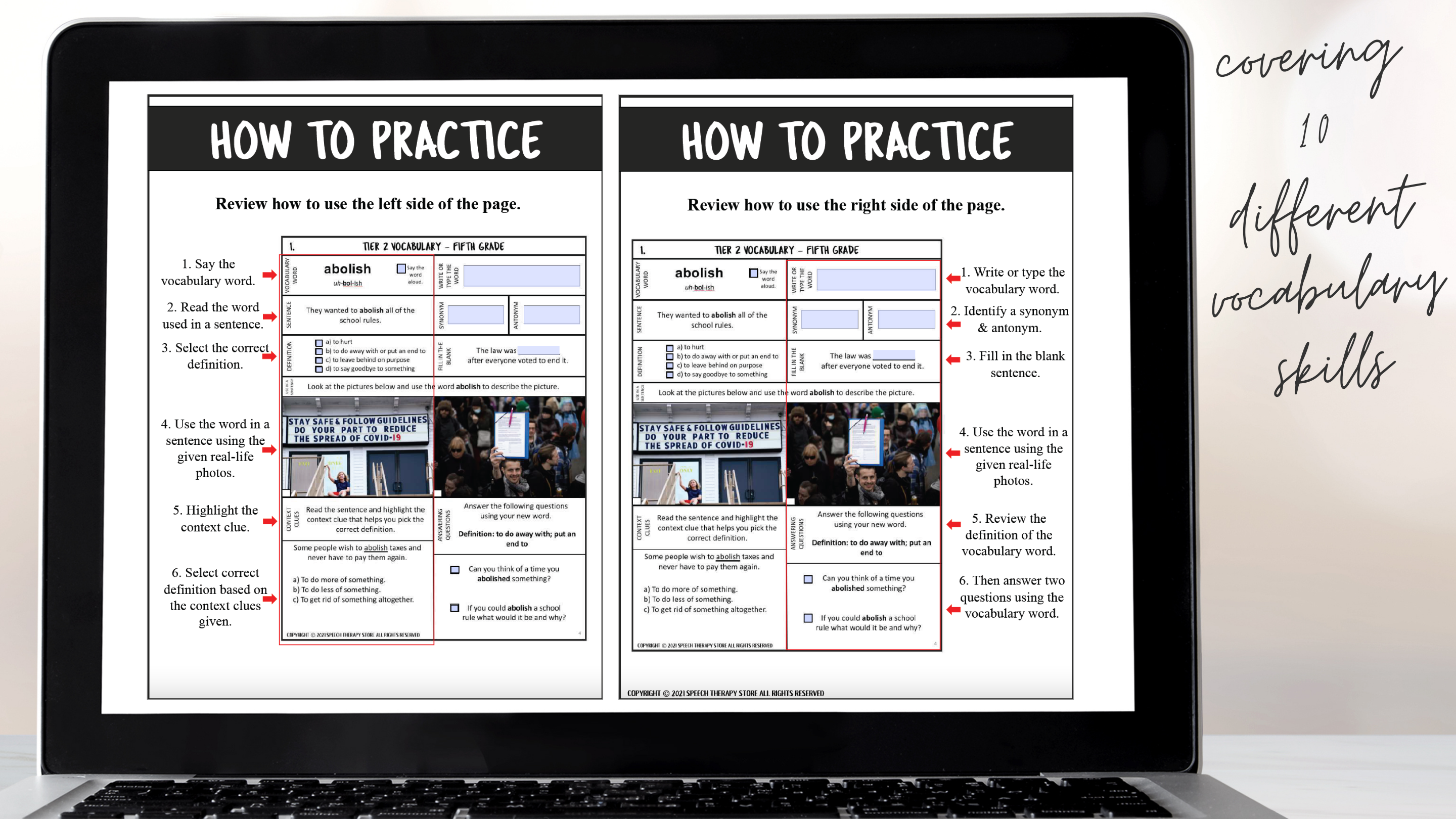
Receptive Language: Vocabulary Goals Speech Therapy
Given 10 common nouns, STUDENT will identify the correct noun by pointing to the appropriate picture with 80% accuracy in 4 out of 5 opportunities.
Given 10 common verbs, STUDENT will identify the correct verb by pointing to the appropriate picture with 80% accuracy in 4 out of 5 opportunities.
Given 10 common adjectives, STUDENT will identify the correct adjective by pointing to the appropriate picture (size, shape, color, texture) with 80% accuracy in 4 out of 5 opportunities.
Given 3 to 5 pictures, STUDENT will identify the category items by pointing/grouping pictures into categories with 80% accuracy in 4 out of 5 opportunities.
SEE ALSO: IEP Goal Bank Posts

Teaching Vocabulary: Speech Therapy Sessions
When it comes to teaching vocabulary in the school setting the best practices are to teach the students the vocabulary strategies and vocabulary knowledge allowing them to learn how to define vocabulary words themselves instead of simply teaching them each new word that they then memorize.
If you’re a speech pathologist, or special education teacher, or parent and you’ve been following me for a while now you know that I love spoiling my community!
And that’s why I’m sharing with you 14 free pages from my newest resource perfect for the elementary age group!
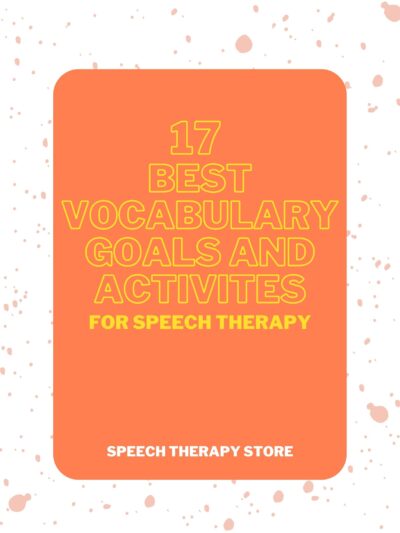
Using Targeted Words
This resource focuses on tier two vocabulary words. Tier two words are common academic words frequently used across multiple subject areas.
Teaching tier two words is an effective method and great way to work on vocabulary that students will come across in multiple textbooks.
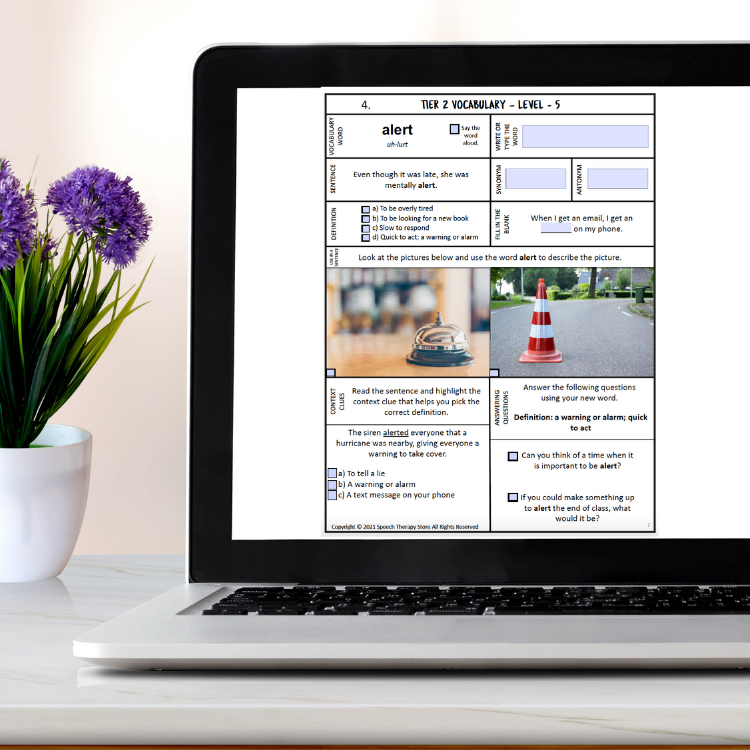
Sentence Level
Have your students practice their word at the sentence level by reading the word in a sentence, adding their word to a fill in the blank sentence, creating a sentence using their word given a visual cue, or practice by answering a question using their new vocabulary word.
Picture Icons
Including picture icons of the words is another fun way to give your students a chance to use their new vocabulary word in a sentence that they get to create.
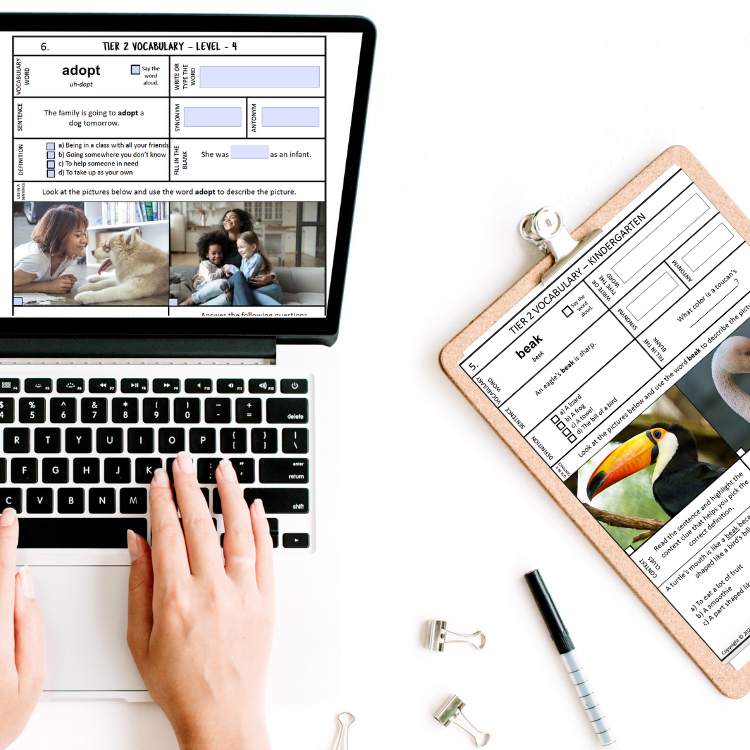
Structured Activity
Using a structured activity with multiple exposures allows the student extra practice with one word at a time.
Consecutive Sessions
Practice over consecutive sessions for additional exposure.
Informal Assessments
The first step when starting a new goal is to collect baseline data. Simply use a couple of these worksheets as a great way to collect an informal assessment of your students’ vocabulary skills.
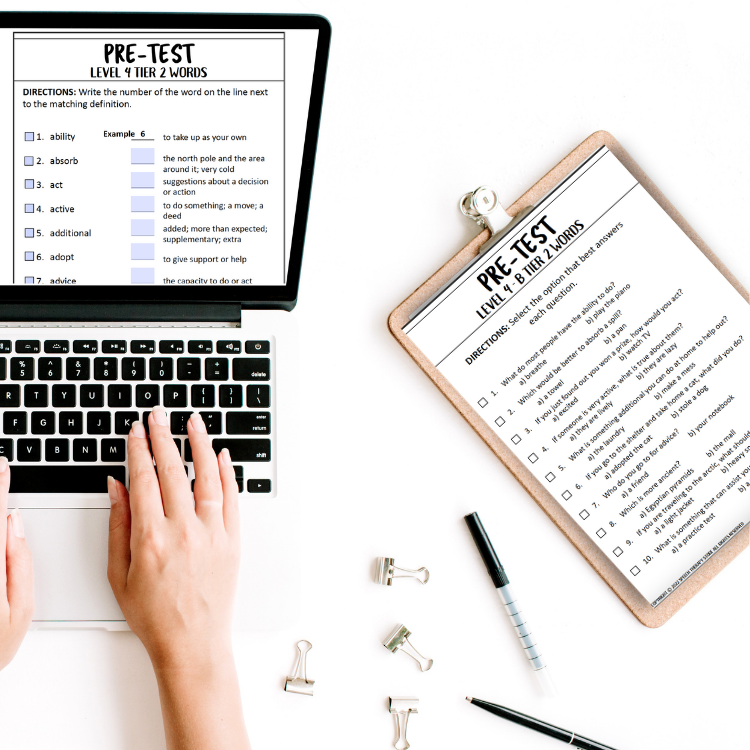
Language Skills
Other language tasks a student could work on are the following:
Do you have a student working on synonyms or antonyms , have a student working on using vocabulary words in a sentence , have a student working on describing a picture , have a student working on context clues , have a student working on defining new vocabulary words , have students answering questions ?
No problem all these students can be working from the same worksheet!
Core Vocabulary Words: Free Activities List
Are you in need of additional free vocabulary activities? I’ve done the searching for you!
After downloading my free 14 vocabulary worksheets above be sure to check out the following resources for even more vocabulary activities to help get you started on your child’s iep vocabulary goals.
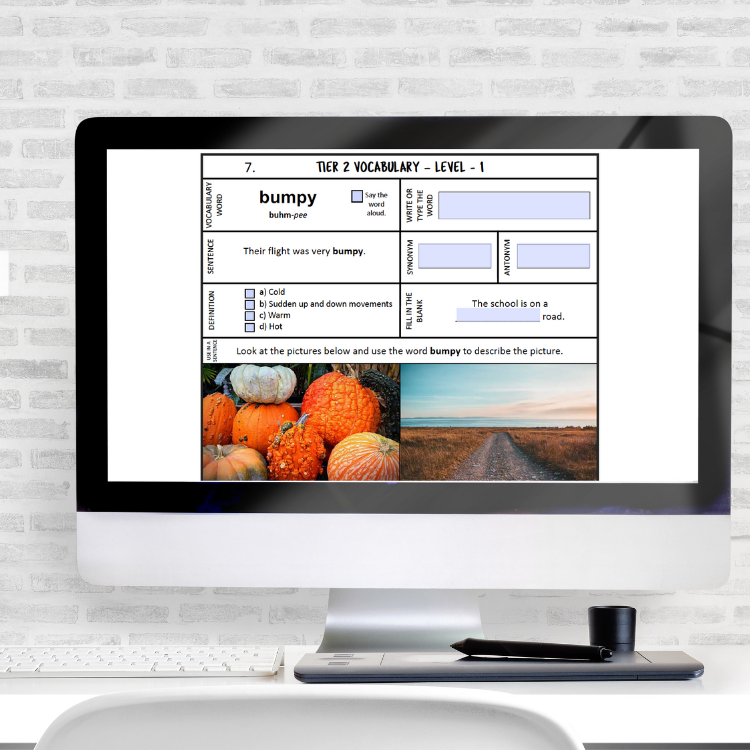
SEE ALSO: 432+ Free Measurable IEP Goals and Objectives Bank
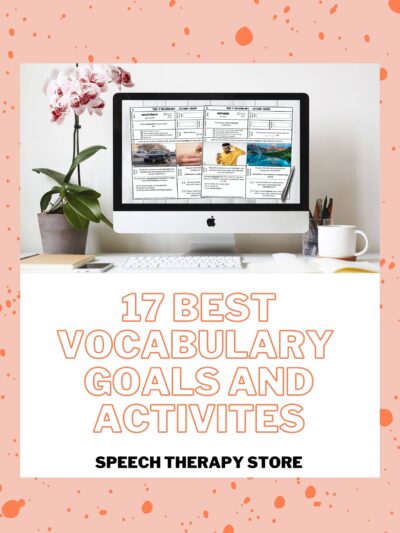
Picture Books
Using picture books can be a fun way to discuss vocabulary words with younger students as you discuss the pictures in the book together.
- Interactive Vocab Book: Mother’s Day Freebie by Jenna Rayburn Kirk – This interactive book uses velcro words so students can match the words to the correct page. There are extra sentence strips to support practicing sentences, describing functions, and describing locations.
- Questions and Vocab, When I was Little: A 4 Year Old’s Memoir of her Youth by Jennifer Trested – This is a great book to use at the end of the year. This freebie includes depth of knowledge questions, vocab, vocabulary pictures, and definitions for each vocabulary word.
- Measurement and Data Vocabulary Book – FREE – Kindergarten Math Center by Keeping my Kinders Busy – This vocab book helps teach vocabulary surrounding the Common Core Kindergarten Measurement and Data Math Unit. It’s easy to prep – just print and the students can trace and color the pictures!
- Interactive Vocabulary Books: Helping at Home by Jenna Rayburn Kirk – This book targets vocabulary, grammar, and language by using velcro pieces to match pictures to words. It keeps little hands busy and is great for preK – first grade!
Correct a Simple Sentence
Practice vocabulary words by correcting a simple sentence to use their vocabulary word correctly.
- Editing Simple Sentences – Winter Sentences by Breaking Barriers – These are winter-themed sentences to help your students learn the editing process. 3 levels help with differentiation and skill-building!
- Concept of Words Simple Sentence Writing by Teachers R US – This activity includes 5 worksheets to help students practice the concept of words, and sight words. It is great for group work or individual work!
Create Complex Sentence
Another fun activity for practicing new vocabulary words is to create a complex sentence with your new words.
- Complex Sentence Vocab! By J-Mar – This is an editable google doc to be used with your vocabulary units. Students can roll a dice that prompts them to use specific conjunction around their vocabulary word.
- Word Work: Practice using Vocab to make Compound and Complex Sentences by Academic Language Central – In these freebies, students are prompted to write compound and complex sentences using their vocab words
Single Word
Practice one word at a time with multiple exposures to using the word in a sentence or to describe a picture.
- Prefix Google Slides Word Search by Literacy Tales – Practice reading, vocabulary and sight words virtually!
- Read and Draw Single Word Vocabulary Printable: PIG by Read & Draw – This is a fun, no-prep activity to help your students remember everyday vocabulary words! (This creator has multiple words!)
- Arctic Animals Word Wall and Vocabulary Matching by ReadingisLove – There are 2 ways to practice vocabulary words in this winter-themed set: a word wall and vocab matching. This is fun and interactive!
Multiple Meaning Words
Using multiple meaning words is another great way to work on your student’s vocabulary skills.
- 193+ Multiple Meaning Words Grouped by Grade + Free Worksheets by Speech Therapy Store – Enjoy this awesome freebie I’ve created with almost 200 multiple meaning words to practice your student’s vocabulary skills.
- Multiple Meaning Word Task Cards – Intermediate Grades! Test Prep by the Owl Spot – This will give your students the chance to practice with word meaning in context. There are 32 task cards and an answer sheet.
- Which Definition Is It? (Multiple Meaning Words w/ Context Clues) by Ciera Harris Teaching – This activity helps students use context clues to figure out the definition of a multiple-meaning word!
Structured Language Activities
You can also work on a language task and simply focus on the vocabulary words as you work through a language activity for more vocabulary practice.
- Follow the Clues: St Patrick’s Day Edition (A Descriptive Language Game) FREEBIE by The Speech Path for Kids – This freebie promotes descriptive language skills while following a St Patricks Day theme!
- What’s Different? Language Activity for Fall by Keeping Speech Simple – This fall-themed, engaging activity promotes descriptive, specific language skills!
Younger Students
Here are a few vocabulary activities that would be perfect for working on with your younger students.
- Back to School Smashmat – Preschool Speech and Language Therapy Activity by Homemade Speech and Language – This freebie targets so many learning areas for PreK and first such as early intervention, speech and language targets, and play skills!
- Caps for Sale Vocabulary by dayle timmons – Read this popular children’s book and identify 6 Tier Two vocabulary words as you read aloud!
- Crossword Puzzle: Animals, Objects, Fruits Vocabulary (Colorful picture clues) by The Mochi Lab – Use these fun, interactive crosswords as an easy way to learn vocabulary!
These would also be perfect for your younger students working on cvc vocabulary tier one words, such as the words cat, bat, or dog.
- Free Phonics Worksheets – Letter Sounds – CVC Words – Beginning Initial Sounds by These are fun, free and interactive worksheets for students to practice CVC words!
- FREE CVC Words Worksheets: No Prep Write Cut and Paste Activity for Word Work by Adapting for Autism – Use these 4 Worksheets to practice reading, writing, vocab and fine motor skills!
- CVC Word Family ‘AT’ No Prep Phonics Printables FREEBIE by Tweet Resources – This freebie focuses on ‘at’ words!
SEE ALSO: 193+ Multiple Meaning Words Grouped by Grade + Free Worksheets
Communication device.
Do you have students using communication devices or boards? Here are a few premade boards to work on different vocabulary words such as expressing likes and dislikes, classroom vocabulary, as well as outside bug vocabulary.
- AAC Core Vocabulary Activities | No Print Speech Therapy | Distance Learning by Speech and Language at Home – Use this core board to have your students discuss their opinions “like” and “don’t like”.
- Core Vocabulary Classroom Labels for Autism and Special Education by The Structured Autism Classroom – Add these core vocabulary words throughout your classroom to help encourage their use throughout the school day.
- AAC Core Vocabulary Freebie | Interactive Books Speech Therapy | Look Outside by Speech and Language at Home – Get outside with your child or student and enjoy working on your student’s bug vocabulary words
Comprehension Questions and WH Questions
Answering comprehension questions and wh questions is another great way to work on vocabulary skills.
- Free Kindergarten Reading Comprehension and Questions by Teaching Biilfizzcend – This is 20 free, fun and interactive reading passages to practice comprehension.
- Free Reading Comprehension Passages & Questions by Mrs. Thompson’s Treasures – This free resource is for grades K- 4, and focuses on engaging students with the text and proving their answers!
- FREEBIE!! WH-Questions Pizza Party! Game for Speech therapy – 90 questions by Miss V’s Speech World – This is a fun and interactive game to get students practicing WH- and HOW- Questions. There are 90 question cards.
Data Collection
If you’re in need of data tracking forms while working on your student’s vocabulary goals for speech therapy then be sure to check out my IEP goal data tracking for progress monitoring forms .
Or if you simply want a list of data sheets to choose from then be sure to check out my list of 35 free speech therapy data sheets roundup .
IEP Goal Bank
Want an even bigger speech therapy goal bank? Don’t worry I’ve got you covered!
Be sure to check out my IEP goal bank made specifically for a speech-language pathologist covering even more language disorder areas such as final consonants or the phonological process of final consonant deletion. Fluency goals using easy onset and slow rate or pragmatic language goals covering communication skills, social interaction, and topic of conversation and so many more educational goals.
In Conclusion: Vocabulary Goals for Speech Therapy
I hope you’ve found this list of vocabulary goals for speech therapy to be helpful in your IEP goal writing!
Be sure to grab your free vocabulary practice pages below by filling out the form below.
Fill Out the Form Below to Download Your Free Sample Pages!
Grab your 14 free sample pages, want even more vocabulary goals for speech therapy resources.
- 430+ Free Multisyllabic Words List Activity Bundle
- 179+ Free Speech Therapy WH Questions Printable
- 133+ Categories List for Speech Therapy
- 33 Most Common Irregular Plurals Flashcards [Freebie]
Want the Best of the Bests?
Be sure to check out our most popular posts below!
- 21 Best Reinforcement Games for Speech Therapy / Teletherapy
- Best IEP Resources
- 71+ Free Social Problem-Solving Scenarios
- 432+ Free Measurable IEP Goals and Objectives Bank
- 279+ Free Speech Therapy Digital Materials
- 179+ Free Speech Therapy Wh-Questions Printable
Sunday 2nd of October 2022
I am looking vocabulary worksheets for Tier 2 kindergarten words
Melissa Berg
Monday 3rd of October 2022
Hi Yukari, You can buy the Kindergarten words here.
Do you have these vocabulary worksheets for all Tier 2 works I can purchase?
Hi Yukari, Yes, I do have a bundle that includes them all for sale. However, I only offer the entire bundle a few times a year. I'm hoping to offer the whole bundle again in November so be sure to keep an eye out for that email. All my best, Melissa
Tuesday 9th of August 2022
Thank you for visiting nature.com. You are using a browser version with limited support for CSS. To obtain the best experience, we recommend you use a more up to date browser (or turn off compatibility mode in Internet Explorer). In the meantime, to ensure continued support, we are displaying the site without styles and JavaScript.
- View all journals
- My Account Login
- Explore content
- About the journal
- Publish with us
- Sign up for alerts
- Open access
- Published: 26 April 2024
Online speech synthesis using a chronically implanted brain–computer interface in an individual with ALS
- Miguel Angrick 1 ,
- Shiyu Luo 2 ,
- Qinwan Rabbani 3 ,
- Daniel N. Candrea 2 ,
- Samyak Shah 1 ,
- Griffin W. Milsap 4 ,
- William S. Anderson 5 ,
- Chad R. Gordon 5 , 6 ,
- Kathryn R. Rosenblatt 1 , 7 ,
- Lora Clawson 1 ,
- Donna C. Tippett 1 , 8 , 9 ,
- Nicholas Maragakis 1 ,
- Francesco V. Tenore 4 ,
- Matthew S. Fifer 4 ,
- Hynek Hermansky 10 , 11 ,
- Nick F. Ramsey 12 &
- Nathan E. Crone 1
Scientific Reports volume 14 , Article number: 9617 ( 2024 ) Cite this article
574 Accesses
14 Altmetric
Metrics details
- Amyotrophic lateral sclerosis
- Neuroscience
Brain–computer interfaces (BCIs) that reconstruct and synthesize speech using brain activity recorded with intracranial electrodes may pave the way toward novel communication interfaces for people who have lost their ability to speak, or who are at high risk of losing this ability, due to neurological disorders. Here, we report online synthesis of intelligible words using a chronically implanted brain-computer interface (BCI) in a man with impaired articulation due to ALS, participating in a clinical trial (ClinicalTrials.gov, NCT03567213) exploring different strategies for BCI communication. The 3-stage approach reported here relies on recurrent neural networks to identify, decode and synthesize speech from electrocorticographic (ECoG) signals acquired across motor, premotor and somatosensory cortices. We demonstrate a reliable BCI that synthesizes commands freely chosen and spoken by the participant from a vocabulary of 6 keywords previously used for decoding commands to control a communication board. Evaluation of the intelligibility of the synthesized speech indicates that 80% of the words can be correctly recognized by human listeners. Our results show that a speech-impaired individual with ALS can use a chronically implanted BCI to reliably produce synthesized words while preserving the participant’s voice profile, and provide further evidence for the stability of ECoG for speech-based BCIs.
Similar content being viewed by others
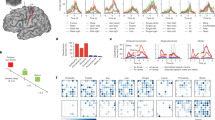
A high-performance speech neuroprosthesis
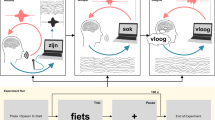
Real-time synthesis of imagined speech processes from minimally invasive recordings of neural activity
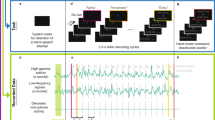
Generalizable spelling using a speech neuroprosthesis in an individual with severe limb and vocal paralysis
Introduction.
A variety of neurological disorders, including amyotrophic lateral sclerosis (ALS), can severely affect speech production and other purposeful movements while sparing cognition. This can result in varying degrees of communication impairments, including Locked-In Syndrome (LIS) 1 , 2 , in which patients can only answer yes/no questions or select from sequentially presented options using eyeblinks, eye movements, or other residual movements. Individuals such as these may use augmentative and alternative technologies (AAT) to select among options on a communication board, but this communication can be slow, effortful, and may require caregiver intervention. Recent advances in implantable brain-computer interfaces (BCIs) have demonstrated the feasibility of establishing and maintaining communication using a variety of direct brain control strategies that bypass weak muscles, for example to control a switch scanner 3 , 4 , a computer cursor 5 , to write letters 6 or to spell words using a hybrid approach of eye-tracking and attempted movement detection 7 . However, these communication modalities are still slower, more effortful, and less intuitive than speech-based BCI control 8 .
Recent studies have also explored the feasibility of decoding attempted speech from brain activity, outputting text or even acoustic speech, which could potentially carry more linguistic information such as intonation and prosody. Previous studies have reconstructed acoustic speech in offline analysis from linear regression models 9 , convolutional 10 and recurrent neural networks 11 , 12 , and encoder-decoder architectures 13 . Concatenative approaches from the text-to-speech synthesis domain have also been explored 14 , 15 , and voice activity has been identified in electrocorticographic (ECoG) 16 and stereotactic EEG recordings 17 . Moreover, speech decoding has been performed at the level of American English phonemes 18 , spoken vowels 19 , 20 , spoken words 21 and articulatory gestures 22 , 23 .
Until now, brain-to-speech decoding has primarily been reported in individuals with unimpaired speech, such as patients temporarily implanted with intracranial electrodes for epilepsy surgery. To date, it is unclear to what extent these findings will ultimately translate to individuals with motor speech impairments, as in ALS and other neurological disorders. Recent studies have demonstrated how neural activity acquired from an ECoG grid 24 or from microelectrodes 25 can be used to recover text from a patient with anarthria due to a brainstem stroke, or from a patient with dysarthria due to ALS, respectively. Prior to these studies, a landmark study allowed a locked-in volunteer to control a real-time synthesizer generating vowel sounds 26 . More recently, Metzger et al. 27 demonstrated in a clinical trial participant diagnosed with quadriplegia and anarthria a multimodal speech-neuroprosthetic system that was capable of synthesizing sentences in a cued setting from silent speech attempts. In our prior work, we presented a ‘plug-and-play’ system that allowed a clinical trial participant living with ALS to issue commands to external devices, such as a communication board, by using speech as a control mechanism 28 .
In related work, BCIs based on non-invasive modalities, such as electroencephalography (EEG), functional near-infrared spectroscopy (fNIRS) or functional magnetic resonance imaging (fMRI) have been investigated for speech decoding applications. These studies have largely focused on imagined speech 29 to avoid contamination by movement artifacts 30 . Recent work by Dash et al., for example, reported speech decoding results for imagined and spoken phrases from 3 ALS patients using magnetoencephalography (MEG) 31 . While speech decoding based on non-invasive methodologies is an important branch in the BCI field as they do not require a surgery and may be adopted by a larger population more easily, their current state of the art comes with disadvantages compared to implantable BCI’s as they lack either temporal or spatial resolution, or are currently not feasible for being used at home.
Here, we show that an individual living with ALS and participating in a clinical trial of an implantable BCI (ClinicalTrials.gov, NCT03567213) was able to produce audible, intelligible words that closely resembled his own voice, spoken at his own pace. Speech synthesis was accomplished through online decoding of ECoG signals generated during overt speech production from cortical regions previously shown to represent articulation and phonation, following similar previous work 11 , 19 , 32 , 33 . Our participant had considerable impairments in articulation and phonation. He was still able to produce some words that were intelligible when spoken in isolation, but his sentences were often unintelligible. Here, we focused on a closed vocabulary of 6 keywords, originally used for decoding spoken commands to control a communication board. Our participant was capable of producing these 6 keywords individually with a high degree of intelligibility. We acquired training data over a period of 6 weeks and deployed the speech synthesis BCI in several separate closed-loop sessions. Since the participant could still produce speech, we were able to easily and reliably time-align the individual’s neural and acoustic signals to enable a mapping between his cortical activity during overt speech production processes and his voice’s acoustic features. We chose to provide delayed rather than simultaneous auditory feedback in anticipation of ongoing deterioration in the patient’s speech due to ALS, with increasing discordance and interference between actual and BCI-synthesized speech. This design choice would be ideal for a neuroprosthetic device that remains capable of producing intelligible words as an individual’s speech becomes increasingly unintelligible, as was expected in our participant due to ALS.
Here, we present a self-paced BCI that translates brain activity directly to acoustic speech that resembles characteristics of the user’s voice profile, with most synthesized words of sufficient intelligibility to be correctly recognized by human listeners. This work makes an important step in adding more evidence that recent speech synthesis from neural signals in patients with intact speech can be translated to individuals with neurological speech impairments, by first focusing on a closed vocabulary that the participant can reliably generate at his own pace, before generalizing towards unseen words. Synthesizing speech from the neural activity associated with overt speech allowed us to demonstrate the feasibility of reproducing the acoustic features of speech when ground truth is available and its alignment with an acoustic target is straightforward, in turn setting a standard for future efforts when ground truth is unavailable, as in the Locked In Syndrome. Moreover, because our speech synthesis model was trained on data that preceded testing by several months, our results also support the stability of ECoG as a basis for speech BCIs.
In order to synthesize acoustic speech from neural signals, we designed a pipeline that consisted of three recurrent neural networks (RNNs) to (1) identify and buffer speech-related neural activity, (2) transform sequences of speech-related neural activity into an intermediate acoustic representation, and (3) eventually recover the acoustic waveform using a vocoder. Figure 1 shows a schematic overview of our approach. We acquired ECoG signals from two electrode grids that covered cortical representations for speech production including ventral sensorimotor cortex and the dorsal laryngeal area (Fig. 1 A). Here, we focused only on a subset of electrodes that had previously been identified as showing significant changes in high-gamma activity associated with overt speech production (see Supplementary Fig. 2 ). From the raw ECoG signals, our closed-loop speech synthesizer extracted broadband high-gamma power features (70–170 Hz) that had previously been demonstrated to encode speech-related information useful for decoding speech (Fig. 1 B) 10 , 14 .
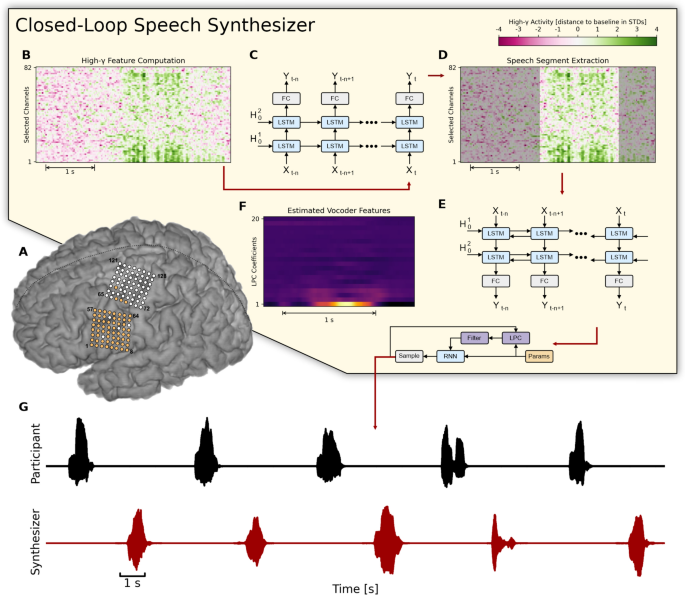
Overview of the closed-loop speech synthesizer. ( A ) Neural activity is acquired from a subset of 64 electrodes (highlighted in orange) from two 8 × 8 ECoG electrode arrays covering sensorimotor areas for face and tongue, and for upper limb regions. ( B ) The closed-loop speech synthesizer extracts high-gamma features to reveal speech-related neural correlates of attempted speech production and propagates each frame to a neural voice activity detection (nVAD) model ( C ) that identifies and extracts speech segments ( D ). When the participant finishes speaking a word, the nVAD model forwards the high-gamma activity of the whole extracted sequence to a bidirectional decoding model ( E ) which estimates acoustic features ( F ) that can be transformed into an acoustic speech signal. ( G ) The synthesized speech is played back as acoustic feedback.
We used a unidirectional RNN to identify and buffer sequences of high-gamma activity frames and extract speech segments (Fig. 1 C,D). This neural voice activity detection (nVAD) model internally employed a strategy to correct misclassified frames based on each frame's temporal context, and additionally included a context window of 0.5 s to allow for smoother transitions between speech and non-speech frames. Each buffered sequence was forwarded to a bidirectional decoding model that mapped high-gamma features onto 18 Bark-scale cepstral coefficients 34 and 2 pitch parameters, henceforth referred to as LPC coefficients 35 , 36 (Fig. 1 E,F). We used a bidirectional architecture to include past and future information while making frame-wise predictions. Estimated LPC coefficients were transformed into an acoustic speech signal using the LPCNet vocoder 36 and played back as delayed auditory feedback (Fig. 1 G).
Synthesis performance
When deployed in sessions with the participant for online decoding, our speech-synthesis BCI was reliably capable of producing acoustic speech that captured many details and characteristics of the voice and pacing of the participant’s natural speech, often yielding a close resemblance to the words spoken in isolation from the participant. Figure 2 A provides examples of original and synthesized waveforms for a representative selection of words time-aligned by subtracting the duration of the extracted speech segment from the nVAD. Onset timings from the reconstructed waveforms indicate that the decoding model captured the flow of the spoken word while also synthesizing silence around utterances for smoother transitions. A comparison between voice activity for spoken and synthesized speech revealed a median Levenstein distance of 235 ms, hinting that the synthesis approach was capable of generating speech that adequately matched the timing of the spoken counterpart. Figure 2 B shows the corresponding acoustic spectrograms for the spoken and synthesized words, respectively. The spectral structures of the original and synthesized speech shared many common characteristics and achieved average correlation scores of 0.67 (± 0.18 standard deviation) suggesting that phoneme and formant-specific information were preserved.
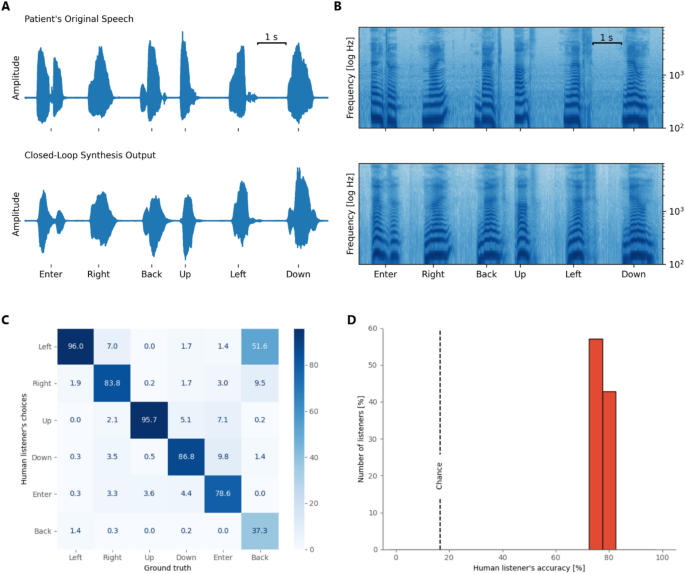
Evaluation of the synthesized words. ( A ) Visual example of time-aligned original and reconstructed acoustic speech waveforms and their spectral representations ( B ) for 6 words that were recorded during one of the closed-loop sessions. Speech spectrograms are shown between 100 and 8000 Hz with a logarithmic frequency range to emphasize formant frequencies. ( C ) The confusion matrix between human listeners and ground truth. ( D ) Distribution of accuracy scores from all who performed the listening test for the synthesized speech samples. Dashed line shows chance performance (16.7%).
We conducted 3 sessions across 3 different days (approximately 5 and a half months after the training data was acquired, each session lasted 6 min) to repeat the experiment with acoustic feedback from the BCI to the participant (see Supplementary Video 1 for an excerpt). Other experiment parameters were not changed. All synthesized words were played back on loudspeakers while simultaneously recorded for evaluation.
To assess the intelligibility of the synthesized words, we conducted listening tests in which human listeners played back individual samples of the synthesized words and selected the word that most closely resembled each sample. Additionally, we mixed in samples that contained the originally spoken words. This allowed us to assess the quality of the participant’s natural speech. We recruited a cohort of 21 native English speakers to listen to all samples that were produced during our 3 closed-loop sessions. Out of 180 samples, we excluded 2 words because the nVAD model did not detect speech activity and therefore no speech output was produced by the decoding model. We also excluded a few cases where speech activity was falsely detected by the nVAD model, which resulted in synthesized silence and remained unnoticed to the participant.
Overall, human listeners achieved an accuracy score of 80%, indicating that the majority of synthesized words could be correctly and reliably recognized. Figure 2 C presents the confusion matrix regarding only the synthesized samples where the ground truth labels and human listener choices are displayed on the X- and Y-axes respectively. The confusion matrix shows that human listeners were able to recognize all but one word at very high rates. “Back” was recognized at low rates, albeit still above chance, and was most often mistaken for “Left”. This could have been due in part to the close proximity of the vowel formant frequencies for these two words. The participant’s weak tongue movements may have deemphasized the acoustic discriminability of these words, in turn resulting in the vocoder synthesizing a version of “back” that was often indistinct from “left”. In contrast, the confusion matrix also shows that human listeners were confident in distinguishing the words “Up” and “Left”. The decoder synthesized an intelligible but incorrect word in only 4% of the cases, and all listeners accurately recognized the incorrect word. Note that all keywords in the vocabulary were chosen for intuitive command and control of a computer interface, for example a communication board, and were not designed to be easily discriminable for BCI applications.
Figure 2 D summarizes individual accuracy scores from all human listeners from the listening test in a histogram. All listeners recognized between 75 and 84% of the synthesized words. All human listeners achieved accuracy scores above chance (16.7%). In contrast, when tested on the participant’s natural speech, our human listeners correctly recognized almost all samples of the 6 keywords (99.8%).
Anatomical and temporal contributions
In order to understand which cortical areas contributed to identification of speech segments, we conducted a saliency analysis 37 to reveal the underlying dynamics in high-gamma activity changes that explain the binary decisions made by our nVAD model. We utilized a method from the image processing domain 38 that queries spatial information indicating which pixels have contributed to a classification task. In our case, this method ranked individual high-gamma features over time by their influence on the predicted speech onsets (PSO). We defined the PSO as the first occurrence when the nVAD model identified spoken speech and neural data started to get buffered before being forwarded to the decoding model. The absolute values of their gradients allowed interpretations of which contributions had the highest or lowest impact on the class scores from anatomical and temporal perspectives.
The general idea is illustrated in Fig. 3 B. In a forward pass, we first estimated for each trial the PSO by propagating through each time step until the nVAD model made a positive prediction. From here, we then applied backpropagation through time to compute all gradients with respect to the model’s input high-gamma features. Relevance scores |R| were computed by taking the absolute value of each partial derivative and the maximum value across time was used as the final score for each electrode 38 . Note that we only performed backpropagation through time for each PSO, and not for whole speech segments.
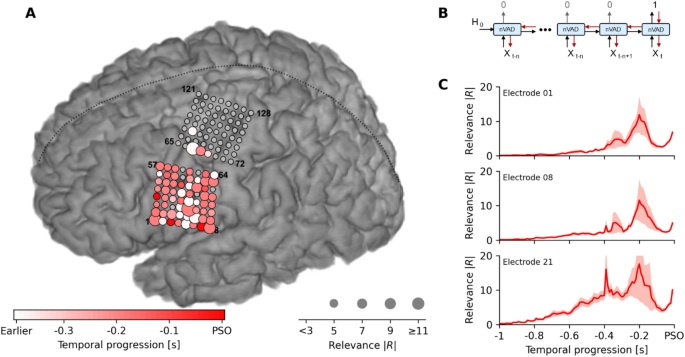
Changes in high-gamma activity across motor, premotor and somatosensory cortices trigger detection of speech output. ( A ) Saliency analysis shows that changes in high-gamma activity predominantly from 300 to 100 ms prior to predicted speech onset (PSO) strongly influenced the nVAD model’s decision. Electrodes covering motor, premotor and somatosensory cortices show the impact of model decisions, while electrodes covering the dorsal laryngeal area only modestly added information to the prediction. Grey electrodes were either not used, bad channels or had no notable contributions. ( B ) Illustration of the general procedure on how relevance scores were computed. For each time step t , relevance scores were computed by backpropagation through time across all previous high-gamma frames X t . Predictions of 0 correspond to no-speech, while 1 represents speech frames. ( C ) Temporal progression of mean magnitudes of the absolute relevance score in 3 selected channels that strongly contributed to PSOs. Shaded areas reflect the standard error of the mean (N = 60). Units of the relevance scores are in 10 –3 .
Results from the saliency analysis are shown in Fig. 3 A. For each channel, we display the PSO-specific relevance scores by encoding the maximum magnitude of the influence in the size of the circles (bigger circles mean stronger influence on the predictions), and the temporal occurrence of that maximum in the respective color coding (lighter electrodes have their maximal influence on the PSO earlier). The color bar at the bottom limits the temporal influence to − 400 ms prior to PSO, consistent with previous reports about speech planning 39 and articulatory representations 19 . The saliency analysis showed that the nVAD model relied on a broad network of electrodes covering motor, premotor and somatosensory cortices whose collective changes in the high-gamma activity were relevant for identifying speech. Meanwhile, voice activity information encoded in the dorsal laryngeal area (highlighted electrodes in the upper grid in Fig. 3 A) 19 only mildly contributed to the PSO.
Figure 3 C shows relevance scores over a time period of 1 s prior to PSO for 3 selected electrodes that strongly contributed to predicting speech onsets. In conjunction with the color coding from Fig. 3 A, the temporal associations were consistent with previous studies that examined phoneme decoding over fixed window sizes of 400 ms 18 and 500 ms 40 , 41 around speech onset times, suggesting that the nVAD model benefited from neural activity during speech planning and phonological processing 39 when identifying speech onset. We hypothesize that the decline in the relevance scores after − 200 ms can be explained by the fact that voice activity information might have already been stored in the long short-term memory of the nVAD model and thus changes in neural activity beyond this time had less influence on the prediction.
Here we demonstrate the feasibility of a closed-loop BCI that is capable of online synthesis of intelligible words using intracranial recordings from the speech cortex of an ALS clinical trial participant. Recent studies 10 , 11 , 13 , 27 suggest that deep learning techniques are a viable tool to reconstruct acoustic speech from ECoG signals. We found an approach consisting of three consecutive RNN architectures that identify and transform neural speech correlates into an acoustic waveform that can be streamed over the loudspeaker as neurofeedback, resulting in an 80% intelligibility score on a closed-vocabulary, keyword reading task.
The majority of human listeners were able to correctly recognize most synthesized words. All words from the closed vocabulary were chosen for a prior study 28 that explored speech decoding for intuitive control of a communication board rather than being constructed to elicit discriminable neural activity that benefits decoder performance. The listening tests suggest that the words “Left” and “Back” were responsible for the majority of misclassified words. These words share very similar articulatory features, and our participant’s speech impairments likely made these words less discriminable in the synthesis process.
Saliency analysis showed that our nVAD approach used information encoded in the high-gamma band across predominantly motor, premotor and somatosensory cortices, while electrodes covering the dorsal laryngeal area only marginally contributed to the identification of speech onsets. In particular, neural changes previously reported to be important for speech planning and phonological processing 19 , 39 appeared to have a profound impact. Here, the analysis indicates that our nVAD model learned a proper representation of spoken speech processes, providing a connection between neural patterns learned by the model and the spatio-temporal dynamics of speech production.
Our participant was chronically implanted with 128 subdural ECoG electrodes, roughly half of which covered cortical areas where similar high-gamma responses have been reliably elicited during overt speech 18 , 19 , 40 , 42 and have been used for offline decoding and reconstruction of speech 10 , 11 . This study and others like it 24 , 27 , 43 , 44 explored the potential of ECoG-based BCIs to augment communication for individuals with motor speech impairments due to a variety of neurological disorders, including ALS and brainstem stroke. A potential advantage of ECoG for BCI is the stability of signal quality over long periods of time 45 . In a previous study of an individual with locked-in syndrome due to ALS, a fully implantable ECoG BCI with fewer electrodes provided a stable switch for a spelling application over a period of more than 3 years 46 . Similarly, Rao et al. reported robust responses for ECoG recordings over the speech-auditory cortex for two drug-resistant epilepsy patients over a period of 1.5 years 47 . More recently, we showed that the same clinical trial participant could control a communication board with ECoG decoding of self-paced speech commands over a period of 3 months without retraining or recalibration 28 . The speech synthesis approach we demonstrated here used training data from five and a half months prior to testing and produced similar results over 3 separate days of testing, with recalibration but no retraining in each session. These findings suggest that the correspondence between neural activity in ventral sensorimotor cortex and speech acoustics were not significantly changed over this time period. Although longitudinal testing over longer time periods will be needed to explicitly test this, our findings provide additional support for the stability of ECoG as a BCI signal source for speech synthesis.
Our approach used a speech synthesis model trained on neural data acquired during overt speech production. This constrains our current approach to patients with speech motor impairments in which vocalization is still possible and in which speech may still be intelligible. Given the increasing use of voice banking among people living with ALS, it may also be possible to improve the intelligibility of synthetic speech using an approach similar to ours, even in participants with unintelligible or absent speech. This speech could be utilized as a surrogate but would require careful alignment to speech attempts. Likewise, the same approach could be used with a generic voice, though this would not preserve the individual’s speech characteristics. Here our results were achieved without the added challenge of absent ground truth, but they serve as an important demonstration that if adequate alignment is achieved, direct synthesis of acoustic speech from ECoG is feasible, accurate, and stable, even in a person with dysarthria due to ALS. Nevertheless, it remains to be seen how long our approach will continue to produce intelligible speech as our patient’s neural responses and articulatory impairments change over time due to ALS. Previous studies of long-term ECoG signal stability and BCI performance in patients with more severe motor impairments suggest that this may be possible 3 , 48 .
Although our approach allowed for online, closed-loop production of synthetic speech that preserved our participant’s individual voice characteristics, the bidirectional LSTM imposed a delay in the audible feedback until after the patient spoke each word. We considered this delay to be not only acceptable, but potentially desirable, given our patient’s speech impairments and the likelihood of these impairments worsening in the future due to ALS. Although normal speakers use immediate acoustic feedback to tune their speech motor output 49 , individuals with progressive motor speech impairments are likely to reach a point at which there is a significant, and distracting, mismatch between the subject’s speech and the synthetic speech produced by the BCI. In contrast, providing acoustic feedback immediately after each utterance gives the user clear and uninterrupted output that they can use to improve subsequent speech attempts, if necessary.
While our results are promising, the approach used here did not allow for synthesis of unseen words. The bidirectional architecture of the decoding model learned variations of the neural dynamics of each word and was capable of recovering their acoustic representations from corresponding sequences of high-gamma frames. This approach did not capture more fine-grained and isolated part-of-speech units, such as syllables or phonemes. However, previous research 11 , 27 has shown that speech synthesis approaches based on bidirectional architectures can generalize to unseen elements that were not part of the training set. Future research will be needed to expand the limited vocabulary used here, and to explore to what extent similar or different approaches are able to extrapolate to words that are not in the vocabulary of the training set.
Our demonstration here builds on previous seminal studies of the cortical representations for articulation and phonation 19 , 32 , 40 in epilepsy patients implanted with similar subdural ECoG arrays for less than 30 days. These studies and others using intraoperative recordings have also supported the feasibility of producing synthetic speech from ECoG high-gamma responses 10 , 11 , 33 , but these demonstrations were based on offline analysis of ECoG signals that were previously recorded in subjects with normal speech, with the exception of the work by Metzger et al. 27 Here, a participant with impaired articulation and phonation was able to use a chronically implanted investigational device to produce acoustic speech that retained his unique voice characteristics. This was made possible through online decoding of ECoG high-gamma responses, using an algorithm trained on data collected months before. Notwithstanding the current limitations of our approach, our findings here provide a promising proof-of-concept that ECoG BCIs utilizing online speech synthesis can serve as alternative and augmentative communication devices for people living with ALS. Moreover, our findings should motivate continued research on the feasibility of using BCIs to preserve or restore vocal communication in clinical populations where this is needed.
Materials and methods
Participant.
Our participant was a male native English speaker in his 60s with ALS who was enrolled in a clinical trial (NCT03567213), approved by the Johns Hopkins University Institutional Review Board (IRB) and by the FDA (under an investigational device exemption) to test the safety and preliminary efficacy of a brain-computer interface composed of subdural electrodes and a percutaneous connection to external EEG amplifiers and computers. All experiments conducted in this study complied with all relevant guidelines and regulations, and were performed according to a clinical trial protocol approved by the Johns Hopkins IRB. Diagnosed with ALS 8 years prior to implantation, our participant’s motor impairments had chiefly affected bulbar and upper extremity muscles and had resulted in motor impairments sufficient to render continuous speech mostly unintelligible (though individual words were intelligible), and to require assistance with most activities of daily living. Our participant’s ability to carry out activities of daily living were assessed using the ALSFRS-R measure 50 , resulting in a score of 26 out of 48 possible points (speech was rated at 1 point, see Supplementary Data S5 ). Furthermore, speech intelligibility and speaking rate were evaluated by a certified speech-language pathologist, whose detailed assessment may be found in the Supplementary Note . The participant gave informed consent after being counseled about the nature of the research and implant-related risks and was implanted with the study device in July 2022. Additionally, the participant gave informed consent for use of his audio and video recordings in publications of the study results.
Study device and implantation
The study device was composed of two 8 × 8 subdural electrode grids (PMT Corporation, Chanhassen, MN) connected to a percutaneous 128-channel Neuroport pedestal (Blackrock Neurotech, Salt Lake City, UT). Both subdural grids contained platinum-iridium disc electrodes (0.76 mm thickness, 2-mm diameter exposed surface) with 4 mm center-to-center spacing and a total surface area of 12.11 cm 2 (36.6 mm × 33.1 mm).
The study device was surgically implanted during a standard awake craniotomy with a combination of local anesthesia and light sedation, without neuromuscular blockade. The device’s ECoG grids were placed on the pial surface of sensorimotor representations for speech and upper extremity movements in the left hemisphere. Careful attention was made to assure that the scalp flap incision was well away from the external pedestal. Cortical representations were targeted using anatomical landmarks from pre-operative structural (MRI) and functional imaging (fMRI), in addition to somatosensory evoked potentials measured intraoperatively. Two reference wires attached to the Neuroport pedestal were implanted in the subdural space on the outward facing surface of the subdural grids. The participant was awoken during the craniotomy to confirm proper functioning of the study device and final placement of the two subdural grids. For this purpose, the participant was asked to repeatedly speak a single word as event-related ECoG spectral responses were noted to verify optimal placement for the implanted electrodes. On the same day, the participant had a post-operative CT which was then co-registered to a pre-operative MRI to verify the anatomical locations of the two grids.
Data recording
During all training and testing sessions, the Neuroport pedestal was connected to a 128-channel NeuroPlex-E headstage that was in turn connected by a mini-HDMI cable to a NeuroPort Biopotential Signal Processor (Blackrock Neurotech, Salt Lake City, UT, USA) and external computers. We acquired neural signals at a sampling rate of 1000 Hz.
Acoustic speech was recorded through an external microphone (BETA® 58A, SHURE, Niles, IL) in a room isolated from external acoustic and electronic noise, then amplified and digitized by an external audio interface (H6-audio-recorder, Zoom Corporation, Tokyo, Japan). The acoustic speech signal was split and forwarded to: (1) an analog input of the NeuroPort Biopotential Signal Processor (NSP) to be recorded at the same frequency and in synchrony with the neural signals, and (2) the testing computer to capture high-quality (48 kHz) recordings. We applied cross-correlation to align the high-quality recordings with the synchronized audio signal from the NSP.
Experiment recordings and task design
Each recording day began with a syllable repetition task to acquire cortical activity to be used for baseline normalization. Each syllable was audibly presented through a loudspeaker, and the participant was instructed to recite the heard stimulus by repeating it aloud. Stimulus presentation lasted for 1 s, and trial duration was set randomly in the range of 2.5 s and 3.5 s with a step size of 80 ms. In the syllable repetition task, the participant was instructed to repeat 12 consonant–vowel syllables (Supplementary Table S4 ), in which each syllable was repeated 5 times. We extracted high-gamma frames from all trials to compute for each day the mean and standard deviation statistics for channel-specific normalization.
To collect data for training our nVAD and speech decoding model, we recorded ECoG during multiple blocks of a speech production task over a period of 6 weeks. During the task, the participant read aloud single words that were prompted on a computer screen, interrupted occasionally by a silence trial in which the participant was instructed to say nothing. The words came from a closed vocabulary of 6 words ("Left", "Right", "Up", "Down", "Enter", "Back", and “…” for silence) that were chosen for a separate study in which these spoken words were decoded from ECoG to control a communication board 28 . In each block, there were ten repetitions of each word (60 words in total) that appeared in a pseudo-randomized order by having a fixed set of seeds to control randomization orders. Each word was shown for 2 s per trial with an intertrial interval of 3 s. The participant was instructed to read the prompted word aloud as soon as it appeared. Because his speech was slow, effortful, and dysarthric, the participant may have sometimes used some of the intertrial interval to complete word production. However, offline analysis verified at least 1 s between the end of each spoken word and the beginning of the next trial, assuring that enough time had passed to avoid ECoG high-gamma responses leaking into subsequent trials. In each block, neural signals and audibly vocalized speech were acquired in parallel and stored to disc using BCI2000 51 .
We recorded training, validation, and test data for 10 days, and deployed our approach for synthesizing speech online five and a half months later. During the online task, the synthesized output was played to the participant while he performed the same keyword reading task as in the training sessions. The feedback from each synthesized word began after he spoke the same word, avoiding any interference with production from the acoustic feedback. The validation dataset was used for finding appropriate hyperparameters to train both nVAD and the decoding model. The test set was used to validate final model generalizability before online sessions. We also used the test set for the saliency analysis. In total, the training set was comprised of 1570 trials that aggregated to approximately 80 min of data (21.8 min are pure speech), while the validation and test set contained 70 trials each with around 3 min of data (0.9 min pure speech). The data in each of these datasets were collected on different days, so that no baseline or other statistics in the training set leaked into the validation or test set.
Signal processing and feature extraction
Neural signals were transformed into broadband high-gamma power features that have been previously reported to closely track the timing and location of cortical activation during speech and language processes 42 , 52 . In this feature extraction process, we first re-referenced all channels within each 64-contact grid to a common-average reference (CAR filtering), excluding channels with poor signal quality in any training session. Next, we selected all channels that had previously shown significant high-gamma responses during the syllable repetition task described above. This included 64 channels (Supplementary Fig. S2 , channels with blue outlines) across motor, premotor and somatosensory cortices, including the dorsal laryngeal area. From here, we applied two IIR Butterworth filters (both with filter order 8) to extract the high-gamma band in the range of 70 to 170 Hz while subsequently attenuating the first harmonic (118–122 Hz) of the line noise. For each channel, we computed logarithmic power features based on windows with a fixed length of 50 ms and a frameshift of 10 ms. To estimate speech-related increases in broadband high-gamma power, we normalized each feature by the day-specific statistics of the high-gamma power features accumulated from the syllable repetition task.
For the acoustic recordings of the participant’s speech, we downsampled the time-aligned high-quality microphone recordings from 48 to 16 kHz. From here, we padded the acoustic data by 16 ms to account for the shift introduced by the two filters on the neural data and estimated the boundaries of speech segments using an energy-based voice activity detection algorithm 53 . Likewise, we computed acoustic features in the LPC coefficient space through the encoding functionality of the LPCNet vocoder. Both voice activity detection and LPC feature encoding were configured to operate on 10 ms frameshifts to match the number of samples from the broadband high-gamma feature extraction pipeline.
Network architectures
Our proposed approach relied on three recurrent neural network architectures: (1) a unidirectional model that identified speech segments from the neural data, (2) a bidirectional model that translated sequences of speech-related high-gamma activity into corresponding sequences of LPC coefficients representing acoustic information, and (3) LPCNet 36 , which converted those LPC coefficients into an acoustic speech signal.
The network architecture of the unidirectional nVAD model was inspired by Zen et al. 54 in using a stack of two LSTM layers with 150 units each, followed by a linear fully connected output layer with two units representing speech or non-speech class target logits (Fig. 4 ). We trained the unidirectional nVAD model using truncated backpropagation through time (BPTT) 55 to keep the costs of single parameter updates manageable. We initialized this algorithm’s hyperparameters k 1 and k 2 to 50 and 100 frames of high-gamma activity, respectively, such that the unfolding procedure of the backpropagation step was limited to 100 frames (1 s) and repeated every 50 frames (500 ms). Dropout was used as a regularization method with a probability of 50% to counter overfitting effects 56 . Comparison between predicted and target labels was determined by the cross-entropy loss. We limited the network training using an early stopping mechanism that evaluated after each epoch the network performance on a held-out validation set and kept track of the best model weights by storing the model weights only when the frame-wise accuracy score was bigger than before. The learning rate of the stochastic gradient descent optimizer was dynamically adjusted in accordance with the RMSprop formula 57 with an initial learning rate of 0.001. Using this procedure, the unidirectional nVAD model was trained for 27,975 update steps, achieving a frame-wise accuracy of 93.4% on held-out validation data. The architecture of the nVAD model had 311,102 trainable weights.
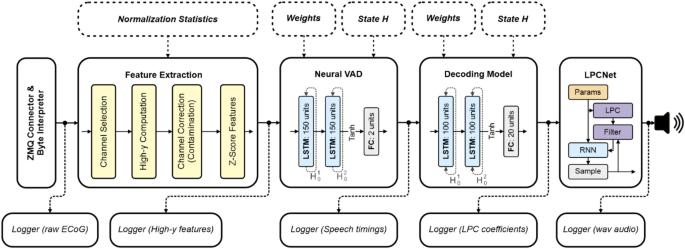
System overview of the closed-loop architecture. The computational graph is designed as a directed acyclic network. Solid shapes represent ezmsg units, dotted ones represent initialization parameters. Each unit is responsible for a self-contained task and distributes their output to all its subscribers. Logger units run in separate processes to not interrupt the main processing chain for synthesizing speech.
The network architecture of the bidirectional decoding model had a very similar configuration to the unidirectional nVAD but employed a stack of bidirectional LSTM layers for sequence modelling 11 to include past and future contexts. Since the acoustic space of the LPC components was continuous, we used a linear fully connected output layer for this regression task. Figure 4 contains an illustration of the network architecture of the decoding model. In contrast to the unidirectional nVAD model, we used standard BPTT to account for both past and future contexts within each extracted segment identified as spoken speech. The architecture of the decoding model had 378,420 trainable weights and was trained for 14,130 update steps using a stochastic gradient descent optimizer. The initial learning rate was set to 0.001 and dynamically updated in accordance with the RMSProp formula. Again, we used dropout with a 50% probability and employed an early stopping mechanism that only updated model weights when the loss on the held-out validation set was lower than before.
Both the unidirectional nVAD and the bidirectional decoding model were implemented within the PyTorch framework. For LPCNet, we used the C-implementation and pretrained model weights by the original authors and communicated with the library via wrapper functions through the Cython programming language.
Closed-loop architecture
Our closed-loop architecture was built upon ezmsg, a general-purpose framework which enables the implementation of streaming systems in the form a directed acyclic network of connected units, which communicate with each other through a publish/subscribe software engineering pattern using asynchronous coroutines. Here, each unit represents a self-contained operation which receives many inputs, and optionally propagates its output to all its subscribers. A unit consists of a settings and state class for enabling initial and updatable configurations and has multiple input and output connection streams to communicate with other nodes in the network. Figure 4 shows a schematic overview of the closed-loop architecture. ECoG signals were received by connecting to BCI2000 via a custom ZeroMQ (ZMQ) networking interface that sent packages of 40 ms over the TCP/IP protocol. From here, each unit interacted with other units through an asynchronous message system that was implemented on top of a shared-memory publish-subscribe multi-processing pattern. Figure 4 shows that the closed-loop architecture was comprised of 5 units for the synthesis pipeline, while employing several additional units that acted as loggers and wrote intermediate data to disc.
In order to play back the synthesized speech during closed-loop sessions, we wrote the bytes of the raw PCM waveform to standard output (stdout) and reinterpreted them by piping them into SoX. We implemented our closed-loop architecture in Python 3.10. To keep the computational complexity manageable for this streamlined application, we implemented several functionalities, such as ringbuffers or specific calculations in the high-gamma feature extraction, in Cython.
Contamination analysis
Overt speech production can cause acoustic artifacts in electrophysiological recordings, allowing learning machines such as neural networks to rely on information that is likely to fail once deployed—a phenomenon widely known as Clever Hans 58 . We used the method proposed by Roussel et al. 59 to assess the risk that our ECoG recordings had been contaminated. This method compares correlations between neural and acoustic spectrograms to determine a contamination index which describes the average correlation of matching frequencies. This contamination index is compared to the distribution of contamination indices resulting from randomly permuting the rows and columns of the contamination matrix—allowing statistical analysis of the risk when assuming that no acoustic contamination is present.
For each recording day among the train, test and validation set, we analyzed acoustic contamination in the high-gamma frequency range. We identified 1 channel (Channel 46) in our recordings that was likely contaminated during 3 recording days (D 5 , D 6 , and D 7 ), and we corrected this channel by taking the average of high-gamma power features from neighboring channels (8-neighbour configuration, excluding the bad channel 38). A detailed report can be found in Supplementary Fig. S1 , where each histogram corresponds to the distribution of permuted contamination matrices, and colored vertical bars indicate the actual contamination index, where green and red indicate the statistical criterion threshold (green: p > 0.05, red: p ≤ 0.05). After excluding the neural data from channel 46, Roussel’s method suggested that the null hypothesis could be rejected, and thus we concluded that no acoustic speech has interfered with neural recording.
Listening test
We conducted a forced-choice listening test similar to Herff et al. 14 in which 21 native English speakers evaluated the intelligibility of the synthesized output and the originally spoken words. Listeners were asked to listen to one word at a time and select which word out of the six options most closely resembled it. Here, the listeners had the opportunity to listen to each sample many times before submitting a choice. We implemented the listening test on top of the BeaqleJS framework 60 . All words that were either spoken or synthesized during the 3 closed-loop sessions were included in the listening test, but were randomly sampled from a uniform distribution for unique randomized sequences across listeners. Supplementary Fig. S3 provides a screenshot of the interface with which the listeners were working.
All human listeners were only recruited through indirect means such as IRB-approved flyers placed on campus sites and had no direct connection to the PI. Anonymous demographic data was collected at the end of the listening test asking for age and preferred gender. Overall, recruited participants were 23.8% male and 61.9% female (14% other or preferred not to answer) ranging between 18 to 30 years old.
Statistical analysis
Original and reconstructed speech spectrograms were compared using Pearson's correlation coefficients for 80 mel-scaled spectral bins. For this, we transformed original and reconstructed waveforms into the spectral domain using the short-time Fourier transform (window size: 50 ms, frameshift: 10 ms, window function: Hanning), applied 80 triangular filters to focus only on perceptual differences for human listeners 61 , and Gaussianized the distribution of the acoustic space using the natural logarithm. Pearson correlation scores were calculated for each sample by averaging the correlation coefficients across frequency bins. The 95% confidence interval (two-sided) was used in the feature selection procedure while the z-criterion was Bonferroni corrected across time points. Lower and upper bounds for all channels and time points can be found in the supplementary data . Contamination analysis is based on permutation tests that use t-tests as their statistical criterion with a Bonferroni corrected significance level of α = 0.05/N, where N represents the number of frequency bins multiplied by the number of selected channels.
Overall, we used the SciPy stats package (version 1.10.1) for statistical evaluation, but the contamination analysis has been done in Matlab with the statistics and machine learning toolbox (version 12.4).
Data availability
Neural data and anonymized speech audio are publicly available at http://www.osf.io/49rt7/ . This includes experiment recordings used as training data and experiment runs from our closed-loop sessions. Additionally, we also included supporting data used for rendering the figures in the main text and in the supplementary material.
Code availability
Corresponding source code for the closed-loop BCI and scripts for generating figures can be obtained from the official Crone Lab Github page at: https://github.com/cronelab/delayed-speech-synthesis . This includes source files for training, inference, and data analysis/evaluation. The ezmsg framework can be obtained from https://github.com/iscoe/ezmsg .
Bauer, G., Gerstenbrand, F. & Rumpl, E. Varieties of the locked-in syndrome. J. Neurol. 221 , 77–91 (1979).
Article CAS PubMed Google Scholar
Smith, E. & Delargy, M. Locked-in syndrome. BMJ 330 , 406–409 (2005).
Article PubMed Central PubMed Google Scholar
Vansteensel, M. J. et al. Fully implanted brain–computer interface in a locked-in patient with ALS. N. Engl. J. Med. 375 , 2060–2066 (2016).
Chaudhary, U. et al. Spelling interface using intracortical signals in a completely locked-in patient enabled via auditory neurofeedback training. Nat. Commun. 13 , 1236 (2022).
Article ADS CAS PubMed Central PubMed Google Scholar
Pandarinath, C. et al. High performance communication by people with paralysis using an intracortical brain–computer interface. eLife 6 , e18554 (2017).
Willett, F. R., Avansino, D. T., Hochberg, L. R., Henderson, J. M. & Shenoy, K. V. High-performance brain-to-text communication via handwriting. Nature 593 , 249–254 (2021).
Oxley, T. J. et al. Motor neuroprosthesis implanted with neurointerventional surgery improves capacity for activities of daily living tasks in severe paralysis: First in-human experience. J. NeuroInterventional Surg. 13 , 102–108 (2021).
Article Google Scholar
Chang, E. F. & Anumanchipalli, G. K. Toward a speech neuroprosthesis. JAMA 323 , 413–414 (2020).
Herff, C. et al. Towards direct speech synthesis from ECoG: A pilot study. In 2016 38th Annual International Conference of the IEEE Engineering in Medicine and Biology Society (EMBC) 1540–1543 (2016).
Angrick, M. et al. Speech synthesis from ECoG using densely connected 3D convolutional neural networks. J. Neural Eng. 16 , 036019 (2019).
Article ADS PubMed Central PubMed Google Scholar
Anumanchipalli, G. K., Chartier, J. & Chang, E. F. Speech synthesis from neural decoding of spoken sentences. Nature 568 , 493–498 (2019).
Wairagkar, M., Hochberg, L. R., Brandman, D. M. & Stavisky, S. D. Synthesizing speech by decoding intracortical neural activity from dorsal motor cortex. In 2023 11th International IEEE/EMBS Conference on Neural Engineering (NER) 1–4 (2023).
Kohler, J. et al. Synthesizing speech from intracranial depth electrodes using an encoder-decoder framework. Neurons Behav. Data Anal. Theory https://doi.org/10.51628/001c.57524 (2022).
Herff, C. et al. Generating natural, intelligible speech from brain activity in motor, premotor, and inferior frontal cortices. Front. Neurosci. https://doi.org/10.3389/fnins.2019.01267 (2019).
Wilson, G. H. et al. Decoding spoken English from intracortical electrode arrays in dorsal precentral gyrus. J. Neural Eng. 17 , 066007 (2020).
Kanas, V. G. et al. Joint spatial-spectral feature space clustering for speech activity detection from ECoG signals. IEEE Trans. Biomed. Eng. 61 , 1241–1250 (2014).
Soroush, P. Z., Angrick, M., Shih, J., Schultz, T. & Krusienski, D. J. Speech activity detection from stereotactic EEG. In 2021 IEEE International Conference on Systems, Man, and Cybernetics (SMC) 3402–3407 (2021).
Mugler, E. M. et al. Direct classification of all American English phonemes using signals from functional speech motor cortex. J. Neural Eng. 11 , 035015 (2014).
Bouchard, K. E., Mesgarani, N., Johnson, K. & Chang, E. F. Functional organization of human sensorimotor cortex for speech articulation. Nature 495 , 327–332 (2013).
Bouchard, K. E. & Chang, E. F. Neural decoding of spoken vowels from human sensory-motor cortex with high-density electrocorticography. In 2014 36th Annual International Conference of the IEEE Engineering in Medicine and Biology Society 6782–6785 (2014).
Kellis, S. et al. Decoding spoken words using local field potentials recorded from the cortical surface. J. Neural Eng. 7 , 056007 (2010).
Mugler, E. M., Goldrick, M., Rosenow, J. M., Tate, M. C. & Slutzky, M. W. Decoding of articulatory gestures during word production using speech motor and premotor cortical activity. In 2015 37th Annual International Conference of the IEEE Engineering in Medicine and Biology Society (EMBC) 5339–5342 (2015).
Mugler, E. M. et al. Differential representation of articulatory gestures and phonemes in precentral and inferior frontal gyri. J. Neurosci. 38 , 9803–9813 (2018).
Article CAS PubMed Central PubMed Google Scholar
Moses, D. A. et al. Neuroprosthesis for decoding speech in a paralyzed person with anarthria. N. Engl. J. Med. 385 , 217–227 (2021).
Willett, F. R. et al. A high-performance speech neuroprosthesis. Nature 620 , 1031–1036 (2023).
Guenther, F. H. et al. A wireless brain–machine interface for real-time speech synthesis. PLoS ONE 4 , e8218 (2009).
Metzger, S. L. et al. A high-performance neuroprosthesis for speech decoding and avatar control. Nature 620 , 1037–1046 (2023).
Luo, S. et al. Stable decoding from a speech BCI enables control for an individual with ALS without recalibration for 3 months. Adv. Sci. 10 , 2304853 (2023).
Cooney, C., Folli, R. & Coyle, D. Neurolinguistics research advancing development of a direct-speech brain–computer interface. iScience 8 , 103–125 (2018).
Herff, C. & Schultz, T. Automatic speech recognition from neural signals: A focused review. Front. Neurosci. https://doi.org/10.3389/fnins.2016.00429 (2016).
Dash, D. et al. Neural Speech Decoding for Amyotrophic Lateral Sclerosis , 2782–2786 (2020). https://doi.org/10.21437/Interspeech.2020-3071 .
Chartier, J., Anumanchipalli, G. K., Johnson, K. & Chang, E. F. Encoding of articulatory kinematic trajectories in human speech sensorimotor cortex. Neuron 98 , 1042-1054.e4 (2018).
Akbari, H., Khalighinejad, B., Herrero, J. L., Mehta, A. D. & Mesgarani, N. Towards reconstructing intelligible speech from the human auditory cortex. Sci. Rep. 9 , 874 (2019).
Moore, B. An introduction to the psychology of hearing: Sixth edition. In An Introduction to the Psychology of Hearing (Brill, 2013).
Taylor, P. Text-to-Speech Synthesis (Cambridge University Press, 2009).
Book Google Scholar
Valin, J.-M. & Skoglund, J. LPCNET: Improving neural speech synthesis through linear prediction. In ICASSP 2019-2019 IEEE International Conference on Acoustics, Speech and Signal Processing (ICASSP) 5891–5895 (2019).
Montavon, G., Samek, W. & Müller, K.-R. Methods for interpreting and understanding deep neural networks. Digit. Signal Process. 73 , 1–15 (2018).
Article MathSciNet Google Scholar
Simonyan, K., Vedaldi, A. & Zisserman, A. Deep inside convolutional networks: Visualising image classification models and saliency maps. In International Conference on Learning Representations (ICLR) (2014).
Indefrey, P. the spatial and temporal signatures of word production components: A critical update. Front. Psychol. https://doi.org/10.3389/fpsyg.2011.00255 (2011).
Ramsey, N. F. et al. Decoding spoken phonemes from sensorimotor cortex with high-density ECoG grids. NeuroImage 180 , 301–311 (2018).
Jiang, W., Pailla, T., Dichter, B., Chang, E. F. & Gilja, V. Decoding speech using the timing of neural signal modulation. In 2016 38th Annual International Conference of the IEEE Engineering in Medicine and Biology Society (EMBC) 1532–1535 (2016).
Crone, N. E. et al. Electrocorticographic gamma activity during word production in spoken and sign language. Neurology 57 , 2045–2053 (2001).
Moses, D. A., Leonard, M. K., Makin, J. G. & Chang, E. F. Real-time decoding of question-and-answer speech dialogue using human cortical activity. Nat. Commun. 10 , 3096 (2019).
Herff, C. et al. Brain-to-text: Decoding spoken phrases from phone representations in the brain. Front. Neurosci. https://doi.org/10.3389/fnins.2015.00217 (2015).
Morrell, M. J. Responsive cortical stimulation for the treatment of medically intractable partial epilepsy. Neurology 77 , 1295–1304 (2011).
Article PubMed Google Scholar
Pels, E. G. M. et al. Stability of a chronic implanted brain–computer interface in late-stage amyotrophic lateral sclerosis. Clin. Neurophysiol. 130 , 1798–1803 (2019).
Rao, V. R. et al. Chronic ambulatory electrocorticography from human speech cortex. NeuroImage 153 , 273–282 (2017).
Silversmith, D. B. et al. Plug-and-play control of a brain–computer interface through neural map stabilization. Nat. Biotechnol. 39 , 326–335 (2021).
Denes, P. B. & Pinson, E. The Speech Chain (Macmillan, 1993).
Google Scholar
Cedarbaum, J. M. et al. The ALSFRS-R: A revised ALS functional rating scale that incorporates assessments of respiratory function. J. Neurol. Sci. 169 , 13–21 (1999).
Schalk, G., McFarland, D. J., Hinterberger, T., Birbaumer, N. & Wolpaw, J. R. BCI2000: A general-purpose brain-computer interface (BCI) system. IEEE Trans. Biomed. Eng. 51 , 1034–1043 (2004).
Leuthardt, E. et al. Temporal evolution of gamma activity in human cortex during an overt and covert word repetition task. Front. Hum. Neurosci. https://doi.org/10.3389/fnhum.2012.00099 (2012).
Povey, D. et al. The kaldi speech recognition toolkit. In IEEE 2011 Workshop on Automatic Speech Recognition and Understanding (IEEE Signal Processing Society, 2011).
Zen, H. & Sak, H. Unidirectional long short-term memory recurrent neural network with recurrent output layer for low-latency speech synthesis. In 2015 IEEE International Conference on Acoustics, Speech and Signal Processing (ICASSP) 4470–4474 (2015).
Sutskever, I. Training Recurrent Neural Networks (University of Toronto, 2013).
Srivastava, N., Hinton, G., Krizhevsky, A., Sutskever, I. & Salakhutdinov, R. Dropout: A simple way to prevent neural networks from overfitting. J. Mach. Learn. Res. 15 , 1929–1958 (2014).
MathSciNet Google Scholar
Ruder, S. An overview of gradient descent optimization algorithms. Preprint at https://arxiv.org/abs/1609.04747 (2016).
Lapuschkin, S. et al. Unmasking Clever Hans predictors and assessing what machines really learn. Nat. Commun. 10 , 1096 (2019).
Roussel, P. et al. Observation and assessment of acoustic contamination of electrophysiological brain signals during speech production and sound perception. J. Neural Eng. 17 , 056028 (2020).
Article ADS PubMed Google Scholar
Kraft, S. & Zölzer, U. BeaqleJS: HTML5 and JavaScript based framework for the subjective evaluation of audio quality. In Linux Audio Conference (2014).
Stevens, S. S., Volkmann, J. & Newman, E. B. A scale for the measurement of the psychological magnitude pitch. J. Acoust. Soc. Am. 8 , 185–190 (1937).
Article ADS Google Scholar
Download references
Acknowledgements
Research reported in this publication was supported by the National Institute Of Neurological Disorders And Stroke of the National Institutes of Health under Award Number UH3NS114439 (PI N.E.C., co-PI N.F.R.). The content is solely the responsibility of the authors and does not necessarily represent the official views of the National Institutes of Health.
Author information
Authors and affiliations.
Department of Neurology, The Johns Hopkins University School of Medicine, Baltimore, MD, USA
Miguel Angrick, Samyak Shah, Kathryn R. Rosenblatt, Lora Clawson, Donna C. Tippett, Nicholas Maragakis & Nathan E. Crone
Department of Biomedical Engineering, The Johns Hopkins University School of Medicine, Baltimore, MD, USA
Shiyu Luo & Daniel N. Candrea
Department of Electrical and Computer Engineering, The Johns Hopkins University, Baltimore, MD, USA
Qinwan Rabbani
Research and Exploratory Development Department, Johns Hopkins Applied Physics Laboratory, Laurel, MD, USA
Griffin W. Milsap, Francesco V. Tenore & Matthew S. Fifer
Department of Neurosurgery, The Johns Hopkins University School of Medicine, Baltimore, MD, USA
William S. Anderson & Chad R. Gordon
Section of Neuroplastic and Reconstructive Surgery, Department of Plastic Surgery, The Johns Hopkins University School of Medicine, Baltimore, MD, USA
Chad R. Gordon
Department of Anesthesiology & Critical Care Medicine, The Johns Hopkins University School of Medicine, Baltimore, MD, USA
Kathryn R. Rosenblatt
Department of Otolaryngology-Head and Neck Surgery, The Johns Hopkins University School of Medicine, Baltimore, MD, USA
Donna C. Tippett
Department of Physical Medicine and Rehabilitation, The Johns Hopkins University School of Medicine, Baltimore, MD, USA
Center for Language and Speech Processing, The Johns Hopkins University, Baltimore, MD, USA
Hynek Hermansky
Human Language Technology Center of Excellence, The Johns Hopkins University, Baltimore, MD, USA
UMC Utrecht Brain Center, Department of Neurology and Neurosurgery, University Medical Center Utrecht, Utrecht, The Netherlands
Nick F. Ramsey
You can also search for this author in PubMed Google Scholar
Contributions
M.A. and N.C. wrote the manuscript. M.A., S.L., Q.R. and D.C. analyzed the data. M.A. and S.S. conducted the listening test. S.L. collected the data. M.A. and G.M. implemented the code for the online decoder and the underlying framework. M.A. made the visualizations. W.A., C.G. and K.R., L.C. and N.M. conducted the surgery/medical procedure. D.T. made the speech and language assessment. F.T. handled the regulatory aspects. H.H. supervised the speech processing methodology. M.F. N.R. and N.C. supervised the study and the conceptualization. All authors reviewed and revised the manuscript.
Corresponding authors
Correspondence to Miguel Angrick or Nathan E. Crone .
Ethics declarations
Competing interests.
The authors declare no competing interests.
Additional information
Publisher's note.
Springer Nature remains neutral with regard to jurisdictional claims in published maps and institutional affiliations.
Supplementary Information
Supplementary information..
Supplementary Video 1.
Supplementary Legends.
Rights and permissions.
Open Access This article is licensed under a Creative Commons Attribution 4.0 International License, which permits use, sharing, adaptation, distribution and reproduction in any medium or format, as long as you give appropriate credit to the original author(s) and the source, provide a link to the Creative Commons licence, and indicate if changes were made. The images or other third party material in this article are included in the article's Creative Commons licence, unless indicated otherwise in a credit line to the material. If material is not included in the article's Creative Commons licence and your intended use is not permitted by statutory regulation or exceeds the permitted use, you will need to obtain permission directly from the copyright holder. To view a copy of this licence, visit http://creativecommons.org/licenses/by/4.0/ .
Reprints and permissions
About this article
Cite this article.
Angrick, M., Luo, S., Rabbani, Q. et al. Online speech synthesis using a chronically implanted brain–computer interface in an individual with ALS. Sci Rep 14 , 9617 (2024). https://doi.org/10.1038/s41598-024-60277-2
Download citation
Received : 19 October 2023
Accepted : 21 April 2024
Published : 26 April 2024
DOI : https://doi.org/10.1038/s41598-024-60277-2
Share this article
Anyone you share the following link with will be able to read this content:
Sorry, a shareable link is not currently available for this article.
Provided by the Springer Nature SharedIt content-sharing initiative
By submitting a comment you agree to abide by our Terms and Community Guidelines . If you find something abusive or that does not comply with our terms or guidelines please flag it as inappropriate.
Quick links
- Explore articles by subject
- Guide to authors
- Editorial policies
Sign up for the Nature Briefing newsletter — what matters in science, free to your inbox daily.

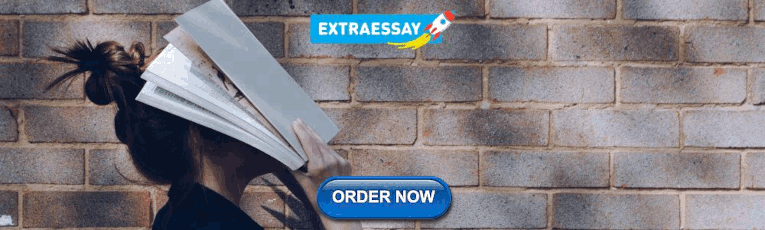
COMMENTS
Whether you're writing an essay or speaking in front of a group, there are certain big words you can use to impress your audience.
Big words to sound smart and their meaning. The smartest way of sounding more eloquent when expressing yourself in English is to change basic, everyday words for their fancier versions. For instance, instead of saying "very big," say "massive.". Instead of saying "detailed." say "granular," and instead of saying "not ...
Babble / Blabber / Blather / Drone / Prattle / Ramble. These words all have very similar meanings. First of all, when someone babbles (or blabbers or blathers or drones or prattles or rambles), it means they are talking for a long time. Too long. And probably not letting other people speak.
100 High Frequency Word List. Unity 28 Smart Charts - 100. Unity 36 Smart Charts - 100. Unity 45 Smart Charts - 100. Unity 60 Smart Charts - 100. LAMP WFL VI Smart Charts - 100. LAMP Words for Life 84 Smart Charts - 100. Unity 84 Smart Charts - 100. WordPower 42 Basic Smart Charts - 100.
a summary that repeats the substance of a longer discussion. The repetition of the matter and the collecting it together, which is. called by the Greeks recapitulation, and by some of the Latins. enumeration, serves for refreshing the judge's memory, for placing the.
brusque. rudely abrupt or blunt in speech or manner. cacophony. loud confusing disagreeable sounds. camaraderie. the quality of affording easy familiarity and sociability. capricious. determined by chance or impulse rather than by necessity. carte blanche.
Many of these words then figure repeatedly in our reading and listening and gradually, as if by osmosis, they start taking roots in our passive vocabulary. Step 2: We start using some of these words in our speech and writing. (They are, as discussed earlier, just a small fraction of our passive vocabulary.)
If you need vocabulary activities, strategies, and ideas for speech therapy, you've come to the right place! Check out my: Vocabulary Worksheets for Speech Therapy (100 vocabulary words! Print-and-go, one-sheet, no-prep vocabulary worksheets that use REAL pictures, available in digital format as well for teletherapy/virtual therapy); Upper Level Vocabulary Worksheets (100 academically ...
A study done by Fallon (2001) found that core words made up to 89% of a preschooler's vocabulary. These words are most commonly "pronouns, verbs, prepositions, and demonstratives". These core words come from studies that compared the most frequently used words in conversation (Banajee et al., 2009, Beukelman et al., 1984).
1. Lead to increased understanding of other words. For example, the word "luxury" was included because it helps you understand other, similar words like "deluxe" and "luxurious". You can use these words to help students understand how the part of speech can change using different endings. 2.
The definition of core vocabulary. Core vocabulary basically means "the most frequently used words in conversation". These are words like "more", "go", "stop", "turn", "on", "off", "that" and "want", to name just a few. Core vocabulary includes adjectives, prepositions, verbs, and pronouns. Core vocabulary ...
Core words comprise 75 - 80% of the words in our day-to-day speech. Core words are useful in a variety of situations. They aren't just nouns; they can be adjectives, verbs, prepositions… words that are high frequency, easy to combined into sentences and they can be used all the time across lots of different routines and settings.
2,000+ Core Tier 2 Vocabulary Words + PDF List. Speech Therapy Store is dedicated to making your speech therapy life easier one resource at a time. To do this, we often partner with companies that share that mission. If you sign up or make a purchase through one of our partners' links, we may receive compensation—at no extra cost to you.
Even preschool students can benefit from the exposure and explicit instruction during speech therapy sessions. A great activity for younger students might involve using picture books that contain tier II vocabulary words. Or, use a wordless book and the possibilities are endless! Tier 1 vocabulary words are everyday words that your student ...
Categories + Word Associations. One facet of your vocabulary, or word-level, therapy should include developing a better, more efficient cognitive organization of vocabulary words. The goal is to build solid semantic networks that link words to other similar words so that a child's vocabulary is better organized, more easily retrieved, and ...
These words are from the speech Gandhi's delivered on August 8, 1942 urging a non-violent fight against British colonial rule. ... 2018, broadly outlining his agenda for the coming year on matters both foreign and domestic. Here are 20 vocabulary words drawn from the President's speech. The full transcript of the speech can be found here. 20 Words.
Depending on the age and needs of our students, the vocabulary goals we target can vary. Below are 5 targets for vocabulary goals to consider, however, they are not the only areas you can target! For more vocabulary goal ideas, head to SLP Now's Goal Bank. 1. Core Vocabulary. For our emergent/early communicators, teaching core vocabulary is ...
Picture Books. Using picture books can be a fun way to discuss vocabulary words with younger students as you discuss the pictures in the book together. Interactive Vocab Book: Mother's Day Freebie by Jenna Rayburn Kirk - This interactive book uses velcro words so students can match the words to the correct page.
a dictionary. or INDEPENDENTLY. VOCABULARY SKILL: use a vocabulary strategy (i.e. context clues, part of speech, affixes/roots, etc.) to infer the meaning of an unknown word. use context clues to determine the meaning of an unknown word. state a word's part of speech. express a definition using the word's prefix, suffix, and/or root.
A vocabulary list featuring The Vocabulary.com Top 1000. The top 1,000 vocabulary words have been carefully chosen to represent difficult but common words that appear in everyday academic and business writing. These words are also the most likely to appear on the SAT, ACT, GRE, and ToEFL. To create this...
Tier 1 includes common words that most students learn through everyday life. They're high frequency and highly functional. Examples of tier 1 vocabulary words are car, blue, cold, drink, or go. Tier 2 includes academic language that can be used across topics and subjects and in a variety of ways. They're more complex, still flexible in their use, and more likely to be found in written text ...
A vocabulary list featuring public speech. ... Practice Answer a few questions about each word. Use this to prep for your next quiz! Vocabulary Jam Compete with other teams in real time to see who answers the most questions correctly! Spelling Bee Test your spelling acumen. Read the definition, listen to the word and try spelling it!
All words from the closed vocabulary were chosen for a prior study 28 that explored speech decoding for intuitive control of a communication board rather than being constructed to elicit ...
A vocabulary list featuring Words Describing Types of Speech. ... Practice Answer a few questions about each word. Use this to prep for your next quiz! Vocabulary Jam Compete with other teams in real time to see who answers the most questions correctly! Spelling Bee Test your spelling acumen. Read the definition, listen to the word and try spelling it!