
- SUGGESTED TOPICS
- The Magazine
- Newsletters
- Managing Yourself
- Managing Teams
- Work-life Balance
- The Big Idea
- Data & Visuals
- Reading Lists
- Case Selections
- HBR Learning
- Topic Feeds
- Account Settings
- Email Preferences
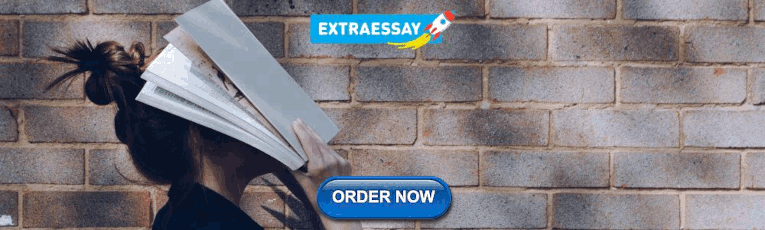
Time Management Is About More Than Life Hacks
- Erich C. Dierdorff

Your productivity hinges on these three skills.
There is certainly no shortage of advice — books and blogs, hacks and apps — all created to boost time management with a bevy of ready-to-apply tools. Yet, the frustrating reality for individuals trying to improve their time management is that tools alone won’t work. You have to develop your time management skills in three key areas: awareness, arrangement, and adaptation. The author offers evidence-based tactics to improve in all three areas.
Project creep, slipping deadlines, and a to-do list that seems to get longer each day — these experiences are all too common in both life and work. With the New Year’s resolution season upon us, many people are boldly trying to fulfill goals to “manage time better,” “be more productive,” and “focus on what matters.” Development goals like these are indeed important to career success. Look no further than large-scale surveys that routinely find time management skills among the most desired workforce skills, but at the same time among the rarest skills to find.

- Erich C. Dierdorff is a professor of management and entrepreneurship at the Richard H. Driehaus College of Business at DePaul University and is currently an associate editor at Personnel Psychology.
Partner Center
- Browse All Articles
- Newsletter Sign-Up
TimeManagement →
No results found in working knowledge.
- Were any results found in one of the other content buckets on the left?
- Try removing some search filters.
- Use different search filters.
College Students’ Time Management: a Self-Regulated Learning Perspective
- Review Article
- Published: 27 October 2020
- Volume 33 , pages 1319–1351, ( 2021 )
Cite this article
- Christopher A. Wolters ORCID: orcid.org/0000-0002-8406-038X 1 &
- Anna C. Brady 1
25k Accesses
59 Citations
10 Altmetric
Explore all metrics
Despite its recognized importance for academic success, much of the research investigating time management has proceeded without regard to a comprehensive theoretical model for understanding its connections to students’ engagement, learning, or achievement. Our central argument is that self-regulated learning provides the rich conceptual framework necessary for understanding college students’ time management and for guiding research examining its relationship to their academic success. We advance this larger purpose through four major sections. We begin by describing work supporting the significance of time management within post-secondary contexts. Next, we review the limited empirical findings linking time management and the motivational and strategic processes viewed as central to self-regulated learning. We then evaluate conceptual ties between time management and processes critical to the forethought, performance, and post-performance phases of self-regulated learning. Finally, we discuss commonalities in the antecedents and contextual determinants of self-regulated learning and time management. Throughout these sections, we identify avenues of research that would contribute to a greater understanding of time management and its fit within the framework of self-regulated learning. Together, these efforts demonstrate that time management is a significant self-regulatory process through which students actively manage when and for how long they engage in the activities deemed necessary for reaching their academic goals.
This is a preview of subscription content, log in via an institution to check access.
Access this article
Price includes VAT (Russian Federation)
Instant access to the full article PDF.
Rent this article via DeepDyve
Institutional subscriptions
Similar content being viewed by others
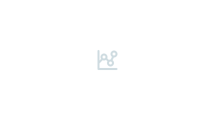
Examining the relations of time management and procrastination within a model of self-regulated learning
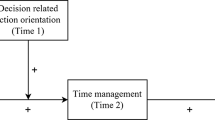
Willing, able, and engaged: roles of action-state orientation, intrinsic academic motivation, and time management on academic engagement
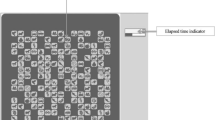
Are Mastery-Oriented College Students Better Time Managers?
Adams, G. A., & Jex, S. M. (1999). Relationships between time management, control, work family conflict, and strain. Journal of Occupational Health Psychology, 4 (1), 72–77.
Google Scholar
Aeon, B., & Aguinis, H. (2017). It’s about time: new perspectives and insights on time management. Academy of Management Perspectives, 31 , 309–330.
Ames, C. (1992). Classrooms: goals, structures, and student motivation. Journal of Educational Psychology, 84 , 261–271.
Asikainen, H., & Gijbels, D. (2017). Do students develop towards more deep approaches to learning during studies? A systematic review on the development of students’ deep and surface approaches to learning in higher education. Educational Psychology Review, 29 , 205–234.
Balduf, M. (2009). Underachievement among college students. Journal of Advanced Academics, 20 , 274–294.
Banahan, L., & Mullendore, R. (2014). Navigating the first college year. A guide for parents and families . Columbia: University of South Carolina, National Resource Center for The First-Year Experience and Students in Transition.
Bandura, A. (1986). The explanatory and predictive scope of self-efficacy theory. Journal of Social and Clinical Psychology, 4 , 359–373.
Bartels, J. M., Magun-Jackson, S., & Ryan, J. J. (2010). Dispositional approach-avoidance achievement motivation and cognitive self-regulated learning: the mediation of achievement goals. Individual Differences Research, 8 , 97–110.
Basila, C. (2014). Good time management and motivation level predict student academic success in college on-line courses. International Journal of Cyber Behavior, Psychology and Learning, 4 , 45–52.
Bembenutty, H. (2009). Academic delay of gratification, self-regulation of learning, gender differences, and expectancy-value. Personality and Individual Differences, 46 , 347–352.
Beuhler, R., Griffin, D., & Peetz, J. (2010). The planning fallacy: cognitive, motivational, and social origins. Advances in Experimental Social Psychology, 43 , 1–62.
Bidjerano, T., & Dai, D. Y. (2007). The relationship between the big-five model of personality and self-regulated learning strategies. Learning and Individual Differences, 17 , 69–81.
Boekaerts, M. (1996). Self-regulated learning at the junction of cognition and motivation. European Psychologist, 1 , 100–112.
Boekaerts, M., & Corno, L. (2005). Self-regulation in the classroom: a perspective on assessment and intervention. Applied Psychology: An International Review, 54 , 199–231.
Bond, M. J., & Feather, N. T. (1988). Some correlates of structure and purpose in the use of time. Journal of Personality and Social Psychology, 55 , 321–329.
Britton, B. K., & Glynn, S. M. (1989). Mental management and creativity: a cognitive model of time management for intellectual productivity. In J. Glover, R. Ronning, & C. Reynolds (Eds.), Handbook of creativity (pp. 429–440). New York: Plenum.
Britton, B. K., & Tesser, A. (1991). Effects of time-management practices on college grades. Journal of Educational Psychology, 83 , 405–410.
Buehler, R., & Griffin, D. (2015). When plans lead to optimistic forecasts. In M. D. Mumford & M. Frese (Eds.), The psychology in planning in organizations: research and applications (pp. 31–57). New York: Routledge.
Burlison, J. D., Murphy, C. S., & Dwyer, W. O. (2009). Evaluation of the Motivated Strategies for Learning Questionnaire for predicting academic performance in college students of varying scholastic aptitude. College Student Journal, 43 , 1313–1323.
Burnette, J., O’Boyle, E., VanEpps, E., Pollack, J., & Finkel, E. (2013). Mind-sets matter: a meta-analytic review of implicit theories and self-regulation. Psychological Bulletin, 139 (3), 655–701.
Burt, C. D., Weststrate, A., Brown, C., & Champion, F. (2010). Development of the time management environment (TiME) scale. Journal of Managerial Psychology, 25 , 649–668.
Butler, D., & Winne, P. (1995). Feedback and self-regulated learning: a theoretical synthesis. Review of Educational Research, 65 , 245–281.
Cano, F. (2006). An in-depth analysis of the Learning and Study Strategies Inventory (LASSI). Educational and Psychological Measurement, 66 , 1023–1038.
Capdeferro, N., Romero, M., & Barberà, E. (2014). Polychronicity: review of the literature and a new configuration for the study of this hidden dimension of online learning. Distance Education, 35 , 294–310.
Cassidy, S. (2011). Self-regulated learning in higher education: identifying key component processes. Studies in Higher Education, 36 , 989–1000.
Chang, A., & Nguyen, L. T. (2011). The mediating effects of time structure on the relationships between time management behaviour, job satisfaction, and psychological well-being. Australian Journal of Psychology, 63 , 187–197.
Choi, B. (2016). How people learn in an asynchronous online learning environment: the relationships between graduate students’ learning strategies and learning satisfaction. Canadian Journal of Learning and Technology, 42 , 1–15.
Chuderski, A. (2016). Time pressure prevents relational learning. Learning and Individual Differences, 49 , 361–365.
Claessens, B. J. C., van Eerde, W., Rutte, C. G., & Roe, R. A. (2007). A review of the time management literature. Personnel Review, 36 , 255–276.
Conti, R. (2001). Time flies: investigating the connection between intrinsic motivation and the experience of time. Journal of Personality, 69 (1), 1–26.
Corno, L., Cronbach, L. J., Kupermintz, H., Lohman, D. F., Mandinach, E. B., Porteus, A. W., & Talbert, J. E. (2002). Remaking the concept of aptitude: extending the legacy of Richard E Snow . Mahwah: Lawrence Erlbaum Associates Publishers.
Crede, M., & Kuncel, N. R. (2008). Study habits, skills, and attitudes: the third pillar supporting collegiate academic performance. Perspectives on Psychological Science, 3 (6), 425–453.
Crede, M., & Phillips, L. A. (2011). A meta-analytic review of the Motivated Strategies for Learning Questionnaire. Learning and Individual Differences, 21 , 337–346.
Crede, M., Roch, S. G., & Kieszczynka, U. M. (2010). Class attendance in college: a meta-analytic review of the relationship of class attendance with grades and student characteristics. Review of Educational Research, 80 , 272–295.
Csikszentmihalyi, M. (1996). Creativity: flow and the psychology of discovery and invention . New York: HarperCollins Publishers.
Dembo, M. H., & Eaton, M. J. (2000). Self-regulation of academic learning in middle-level schools. The Elementary School Journal, 100 , 473–490.
Dent, A., & Koenka, A. (2016). The relation between self-regulated learning and academic achievement across childhood and adolescence: a meta-analysis. Educational Psychology Review, 28 , 425–474.
Díaz-Morales, J. F., Ferrari, J. R., & Cohen, J. R. (2008). Indecision and avoidant procrastination: the role of morningness—eveningness and time perspective in chronic delay lifestyles. The Journal of General Psychology, 135 (3), 228–240.
Donker, A., de Boer, H., Kostons, D., Dignath van Ewijk, C., & van der Werf, M. (2014). Effectiveness of learning strategy instruction on academic performance: a meta-analysis. Educational Psychology Review, 11 , 1–26.
Douglas, H. E., Bore, M., & Munro, D. (2016). Coping with university education: the relationships of time management behaviour and work engagement with the five factor model aspects. Learning and Individual Differences, 45 , 268–274.
Doumen, S., Broeckmans, J., & Masui, C. (2014). The role of self-study time in freshmen’s achievement. Educational Psychology, 34 , 385–402.
Duncan, T. G., & McKeachie, W. J. (2005). The making of the motivated strategies for learning questionnaire. Educational Psychologist, 40 , 117–128.
Duncheon, J. C., & Tierney, W. G. (2013). Changing conceptions of time: implications for educational research and practice. Review of Educational Research, 83 , 236–272.
Dunlosky, J., & Ariel, R. (2011). Self-regulated learning and the allocation of study time. In B. Ross (Ed.), Psychology of learning and motivation (Vol. 54, pp. 103–140). San Diego: Academic Press.
Dunning, D., Heath, C., & Suls, J. (2004). Flawed self-assessments. Psychological Science in the Public Interest, 5 (3), 69–106.
Efklides, A. (2011). Interactions of metacognition with motivation and affect in self-regulated learning: the MASRL model. Educational Psychologist, 46 , 6–25.
Eilam, B., & Aharon, I. (2003). Students’ planning in the process of self-regulated learning. Contemporary Educational Psychology, 28 , 304–334.
Ferrari, J. R., & Díaz-Morales, J. F. (2007). Procrastination: different time orientations reflect different motives. Journal of Research in Personality, 41 , 707–714.
Flake, J. K., Barron, K. E., Hulleman, C., McCoach, B. D., & Welsh, M. E. (2015). Measuring cost: the forgotten component of expectancy-value theory. Contemporary Educational Psychology, 41 , 232–244.
Flanigan, A. E., & Kiewra, K. A. (2018). What college instructors can do about student cyber-slacking. Educational Psychology Review, 30 , 585–597.
Francis-Smythe, J. A., & Robertson, I. T. (1999a). On the relationship between time management and time estimation. British Journal of Psychology, 90 , 333–347.
Francis-Smythe, J. A., & Robertson, I. T. (1999b). Time-related individual differences. Time & Society, 8 , 273–292.
Fromme, K., Corbin, W., & Kruse, M. (2008). Behavioral risks during the transition from high school to college. Developmental Psychology, 44 (5), 1497–1504.
Gable, P. A., & Poole, B. D. (2012). Time flies when you’re having approach-motivated fun: effects of motivational intensity on time perception. Psychological Science, 23 (8), 879–886.
Gevers, J. M., Rutte, C. G., & Van Eerde, W. (2006). Meeting deadlines in work groups: implicit and explicit mechanisms. Applied Psychology, 55 , 52–72.
Green, P., & Skinner, D. (2005). Does time management training work? An evaluation. International Journal of Training and Development, 9 , 124–139.
Gulec, M., Selvi, Y., Boysan, M., Aydin, A., Oral, E., & Aydin, E. F. (2013). Chronotype effects on general well-being and psychopathology levels in healthy young adults. Biological Rhythm Research, 44 , 457–468.
Guzman, G., Goldberg, T., & Swanson, H. (2018). A meta-analysis of self-monitoring on reading performance of K-12 students. School Psychology Quarterly, 33 (1), 160–168.
Hadwin, A., & Oshige, M. (2011). Self-regulation, coregulation, and socially shared regulation: exploring perspectives of social in self-regulated learning theory. Teachers College Record, 113 , 240–264.
Hafner, A., Stock, A., Pinneker, L., & Strohle, S. (2014). Stress prevention through a time management training intervention: an experimental study. Educational Psychology, 34 , 403–416.
Hahn, C., Cowell, J. M., Wiprzycka, U. J., Goldstein, D., Ralph, M., Hasher, L., & Zelazo, P. D. (2012). Circadian rhythms in executive function during the transition to adolescence: the effect of synchrony between chronotype and time of day. Developmental Science, 15 (3), 408–416.
Harkin, B., Webb, T. L., Chang, B. P. I., Prestwich, A., Conner, M., Kellar, I., et al. (2016). Does monitoring goal progress promote goal attainment? A meta-analysis of the experimental evidence. Psychological Bulletin, 142 (2), 198–229.
Hattie, J., Biggs, J., & Purdie, N. (1996). Effects of learning skills interventions on student learning: a meta-analysis. Review of Educational Research, 66 , 99–136.
Haynes, N., Comer, J., & Hamilton-Lee, M. (1988). Gender and achievement status differences on learning factors among Black high school students. Journal of Educational Research, 81 , 233–237.
Hensley, L. C., Wolters, C. A., Won, S., & Brady, A. C. (2018). Academic probation, time management, and time use in a college success course. Journal of College Reading and Learning, 48 , 105–123.
Hicks, T., & Heastie, S. (2008). High school to college transition: a profile of the stressors, physical and psychological health issues that affect the first-year on-campus college students. Journal of Cultural Diversity, 15 (3), 143–147.
Hilbrecht, M., Zuzanek, J., & Mannell, R. C. (2008). Time use, time pressure and gendered behavior in early and late adolescence. Sex Roles, 58 , 342–357.
Hofer, B. K., Yu, S. L., & Pintrich, P. R. (1998). Teaching college students to be self-regulated learners. In D. H. Schunk & B. J. Zimmerman (Eds.), Self-regulated learning: from teaching to self-reflective practice (pp. 57–85). New York: Guilford Press.
Horstmanshof, L., & Zimitat, C. (2007). Future time orientation predicts academic engagement among first-year university students. British Journal of Educational Psychology, 77 (Pt 3), 703–718.
Huie, F. C., Winsler, A., & Kitsantas, A. (2014). Employment and first-year college achievement: the role of self-regulation and motivation. Journal of Education and Work, 27 , 110–135.
Husman, J., & Lens, W. (1999). The role of the future in student motivation. Educational Psychologist, 34 , 113–125.
Kanfer, R., Frese, M., & Johnson, R. (2017). Motivation related to work: a century of progress. Journal of Applied Psychology, 102 (3), 338–355.
Kantrowitz, T. M., Grelle, D. M., Beaty, J. C., & Wolf, M. B. (2012). Time is money: polychronicity as a predictor of performance across job levels. Human Performance, 25 , 114–137.
Kauderer, S., & Randler, C. (2013). Differences in time use among chronotypes in adolescents. Biological Rhythm Research, 44 , 601–608.
Kaufman-Scarborough, C., & Lindquist, J. D. (1999). Time management and polychronicity: comparisons, contrasts, and insights for the workplace. Journal of Managerial Psychology, 14 , 288–312.
Keating, D. P. (2012). Cognitive and brain development in adolescence. Enfance, 64 , 267–279.
Kesici, Ş., Baloğlu, M., & Deniz, M. E. (2011). Self-regulated learning strategies in relation with statistics anxiety. Learning and Individual Differences, 21 , 472–477.
Kim, K. R., & Seo, E. H. (2015). The relationship between procrastination and academic performance: a meta-analysis. Personality and Individual Differences, 82 , 26–33.
Kitsantas, A., Winsler, A., & Huie, F. (2008). Self-regulation and ability predictors of academic success during college: a predictive validity study. Journal of Advanced Academics, 20 , 42–68.
Koch, C. J., & Kleinmann, M. (2002). A stitch in time saves nine: behavioural decision-making explanations for time management problem. European Journal of Work and Organizational Psychology, 11 , 199–217.
Komarraju, M., Karau, S. J., & Schmeck, R. R. (2009). Role of the Big Five personality traits in predicting college students’ academic motivation and achievement. Learning and Individual Differences, 19 , 47–52.
Konig, C. J., & Waller, M. J. (2010). Time for reflection: a critical examination of polychronicity. Human Performance, 23 , 173–190.
Kooij, D. T. A. M., Kanfer, R., Betts, M., & Rudolph, C. W. (2018). Future time perspective: a systematic review and meta-analysis. Journal of Applied Psychology, 103 (8), 867–893.
Krumrei-Mancuso, E. J., Newton, F. B., Kim, E., & Wilcox, D. (2013). Psychosocial factors predicting first-year college student success. Journal of College Student Development, 54 , 247–266.
Landrum, R. E., Turrisi, R., & Brandel, J. M. (2006). College students’ study time: course level, time of semester, and grade earned. Psychological Reports, 98 (3), 675–682.
Liborius, P., Bellhauser, H., & Schmitz, B. (2017). What makes a good study day? An intraindividual study on university students’ time investment by means of time-series analyses. Learning and Instruction.
Linnenbrink-Garcia, L., & Patall, E. (2016). Motivation. In L. Corno & E. Anderman (Eds.), Handbook of educational psychology (3rd ed., pp. 91–1030). New York: Routledge.
Liu, O. L., Rijmen, F., MacCann, C., & Roberts, R. (2009). The assessment of time management in middle-school students. Personality and Individual Differences, 47 , 174–179.
Locke, E., & Latham, G. (2019). The development of goal setting theory: a half-century retrospective. Motivation Science, 5 , 93–105.
Loureiro, F., & Garcia-Marques, T. (2015). Morning or evening person? Which type are you? Self-assessment of chronotype. Personality and Individual Differences, 86 , 168–171.
Lynch, D. J. (2010). Application of online discussion and cooperative learning strategies to online and blended college courses. College Student Journal, 44 , 920–927.
Macan, T. H. (1994). Time management: test of a process model. Journal of Applied Psychology, 79 , 381–391.
Macan, T. H., Shahani, C., Dipboye, R. L., & Phillips, A. P. (1990). College students’ time management: correlations with academic performance and stress. Journal of Educational Psychology, 82 , 760–768.
MacCann, C., & Roberts, R. D. (2010). Do time management, grit, and self-control relate to academic achievement independently of conscientiousness? In R. E. Hicks (Ed.), Personality and individual differences: current directions (pp. 79–90). Bowen Hills: Australian Academic Press.
MacCann, C., Fogarty, G. J., & Roberts, R. D. (2012). Strategies for success in education: time management is more important for part-time than full-time community college students. Learning and Individual Differences, 22 , 618–623.
McCrae, R., & Lockenhoff, C. (2010). Self-regulation and the five-factor model of personality traits. In R. Hoyle (Ed.), Handbook of personality and self-regulation (pp. 143–168). New York: Wiley.
McInerney, D., & King, R. (2018). Culture and self-regulation in educational contexts. In D. Schunk & J. Greene (Eds.), Handbook of self-regulation of learning and performance (2nd ed., pp. 485–502). New York: Routledge.
Meeuwisse, M., Born, M. P., & Severiens, S. E. (2013). Academic performance differences among ethnic groups: do the daily use and management of time offer explanations? Social Psychology of Education, 16 , 599–615.
Melancon, J. G. (2002). Reliability, structure, and correlates of Learning and Study Strategies Inventory scores. Educational and Psychological Measurement, 62 , 1020–1027.
Miele, D. B., & Scholer, A. A. (2018). The role of metamotivational monitoring in motivation regulation. Educational Psychologist, 53 , 1–21.
Miller, R. B., & Brickman, S. J. (2004). A model of future-oriented motivation and self-regulation. Educational Psychology Review, 16 , 9–33.
Muis, K. R. (2007). The role of epistemic beliefs in self-regulated learning. Educational Psychologist, 42 , 173–190.
Nelson, T., & Narens, L. (1990). Metamemory: a theoretical framework and some new findings. In G. H. Bower (Ed.), The psychology of learning and motivation (pp. 125–173). New York: Academic Press.
Nonis, S., Teng, J., & Ford, C. (2005). A cross-cultural investigation of time management practices and job outcomes. International Journal of Intercultural Relations, 29 , 409–428.
Nonis, S. A., Philhours, M. J., & Hudson, G. I. (2006). Where does the time go? A diary approach to business and marketing students’ time use. Journal of Marketing Education, 28 , 121–134.
Olaussen, B. S., & Bråten, I. (1998). Identifying latent variables measured by the Learning and Study Strategies Inventory (LASSI) in Norwegian college students. The Journal of Experimental Education, 67 , 82–96.
Olejnik, S., & Nist, S. L. (1992). Identifying latent variables measured by the Learning and Study Strategies Inventory (LASSI). The Journal of Experimental Education, 60 , 151–159.
Panadero, E. (2017). A review of self-regulated learning: six models and four directions for research. Frontiers in Psychology, 8 , 1–28.
Panadero, E., Brown, G., & Strijbos, J. (2016). The future of student self-assessment: a review of known and unknowns and potential directions. Educational Psychology Review, 28 (803), 830.
Panek, E. (2014). Left to their own devices: college students’ “guilty pleasure” media use and time management. Communication Research, 41 , 561–577.
Pekrun, R., Goetz, T., Titz, W., & Perry, R. P. (2002). Academic emotions in students’ self-regulated learning and achievement: a program of qualitative and quantitative research. Educational Psychologist, 37 , 91–105.
Pintrich, P. R. (2000). The role of goal orientation in self-regulated learning. In M. Boekaerts, P. R. Pintrich, & M. Zeidner (Eds.), Handbook of self-regulation (pp. 451–502). San Diego: Academic.
Pintrich, P. R. (2004). A conceptual framework for assessing motivation and self-regulated learning in college students. Educational Psychology Review, 16 , 385–407.
Pintrich, P. R., & Zusho, A. (2007). Student motivation and self-regulated learning in the college classroom. In R. P. Perry & J. C. Smart (Eds.), The scholarship of teaching and learning in higher education: an evidence based perspective (pp. 731–810). New York: Springer.
Pintrich, P. R., Smith, D. A., Garcia, T., & McKeachie, W. J. (1993). Reliability and predictive validity of the Motivated Strategies for Learning Questionnaire. Educational and Psychological Measurement, 53 , 801–813.
Pintrich, P. R., Wolters, C. A., & Baxter, G. P. (2000). Assessing metacognition and self-regulated learning. In G. Schraw & J. Impara (Eds.), Issues in the measurement of metacognition (pp. 43–97). Lincoln: Buros Institute of Mental Measurement.
Plant, E. A., Ericsson, K. A., Hill, L., & Asberg, K. (2005). Why study time does not predict grade point average across college students: implications of deliberate practice for academic performance. Contemporary Educational Psychology, 30 , 96–116.
Prevatt, F., Petscher, Y., Proctor, B. E., Hurst, A., & Adams, K. (2006). The revised Learning and Study Strategies Inventory: an evaluation of competing models. Educational and Psychological Measurement, 66 (3), 448–458.
Pychyl, T. A., Morin, R. W., & Salmon, B. R. (2000). Procrastination and the planning fallacy: an examination of the study habits of university students. Journal of Social Behavior and Personality, 15 , 135–150.
Ranellucci, J., Hall, N. C., & Goetz, T. (2015). Achievement goals, emotions, learning, and performance: a process model. Motivation Science, 1 , 98–120.
Rhodes, M., & Tauber, S. (2011). The influence of delaying judgments of learning on metacognitive accuracy: a meta-analytic review. Psychological Bulletin, 137 (1), 131–148.
Richards, J. H. (1987). Time management—a review. Work & Stress, 1 , 73–78.
Rickert, N. P., Meras, I. L., & Witkow, M. R. (2014). Theories of intelligence and students’ daily self-handicapping behaviors. Learning and Individual Differences, 36 , 1–8.
Roskes, M., Elliot, A. J., Nijstad, B. A., & De Dreu, C. K. (2013). Time pressure undermines performance more under avoidance than approach motivation. Personality and Social Psychology Bulletin, 39 , 803–813.
Rytkonen, H., Parpala, A., Lindblom-Ylanne, S., Virtanen, V., & Postareff, L. (2012). Factors affecting bioscience students’ academic achievement. Instructional Science, 40 , 241–256.
Sanders, L., Reedy, D., & Frizell, M. (Eds.). (2018). Learning centers in the 21 st century: a modern guide for learning assistance professionals in higher education . Bentonville: AR. Iona Press.
Sansgiry, S. S., Bhosle, M., & Sail, K. (2006). Factors that affect academic performance among pharmacy students. American Journal of Pharmaceutical Education, 70 , 1–9.
Schell, K. L., & Conte, J. M. (2008). Associations among polychronicity, goal orientation, and error orientation. Personality and Individual Differences, 44 , 288–298.
Schraw, G. (2006). Knowledge: structures and processes. In P. Alexander & P. Winne (Eds.), Handbook of educational psychology (2nd ed., pp. 245–263). Mahwah: Erlbaum Associates.
Schunk, D. H., & Zimmerman, B. J. (1997). Social origins of self-regulatory competence. Educational Psychologist, 32 , 195–208.
Schunk, D. H., & Zimmerman, B. J. (Eds.). (1998). Self-regulated learning: from teaching to self-reflective practice . New York: Guilford Press.
Sen, S., & Yilmaz, A. (2016). Devising a structural equation model of relationships between preservice teachers’ time and study environment management, effort regulation, self-efficacy, control of learning beliefs, and metacognitive self-regulation. Science Education International, 27 , 301–316.
Shaunessy-Dedrick, E., Suldo, S. M., Roth, R. A., & Fefer, S. A. (2015). Students’ perceptions of factors that contribute to risk and success in accelerated high school courses. The High School Journal, 98 , 109–137.
Shell, D. F., & Husman, J. (2001). The multivariate dimensionality of personal control and future time perspective beliefs in achievement and self-regulation. Contemporary Educational Psychology, 26 (4), 481–506.
Simons, J., Vansteenkiste, M., Lens, W., & Lacante, M. (2004). Placing motivation and future time perspective theory in a temporal perspective. Educational Psychology Review, 16 , 121–139.
Sinatra, G., Kienhues, D., & Hofer, B. (2014). Addressing challenges to public understanding of science: epistemic cognition, motivated reasoning, and conceptual change. Educational Psychologist, 49 , 123–138.
Sirois, F. M. (2014). Procrastination and stress: exploring the role of self-compassion. Self and Identity, 13 , 128–145.
Sitzmann, T., & Ely, K. (2011). A meta-analysis of self-regulated learning in work-related training and educational attainment: what we know and where we need to go. Psychological Bulletin, 137 (3), 421–442.
Sitzmann, T., Ely, K., Brown, K., & Bauer, K. (2010). Self-assessment of knowledge: a cognitive learning or affective measure? Academy of Management Learning & Education, 9 , 169–191.
Snow, R. (1989). Cognitive-conative aptitude interactions in learning. In R. Kanfer, P. Ackerman, & R. Cudeck (Eds.), Abilities, motivation, and methodology: the Minnesota symposium on learning and individual differences (pp. 435–474). Hillsdale: Erlbaum Associates.
Snow, R. E., Corno, L., & Jackson III, D. (1996). Individual differences in affective and conative functions. In D. C. Berliner & R. C. Calfee (Eds.), Handbook of educational psychology (pp. 243–310). New York: Macmillan Library Reference.
Soderstrom, N. C., & Bjork, R. A. (2014). Testing facilitates the regulation of subsequent study time. Journal of Memory and Language, 73 , 99–115.
Steel, P. (2007). The nature of procrastination: a meta-analytic and theoretical review of quintessential self-regulatory failure. Psychological Bulletin, 133 (1), 65–94.
Stevens, T., & Tallent-Runnels, M. K. (2004). The learning and study strategies inventory-high school version: issues of factorial invariance across gender and ethnicity. Educational and Psychological Measurement, 64 , 332–346.
Strunk, K. K., Cho, Y., Steele, M. R., & Bridges, S. L. (2013). Development and validation of a 2× 2 model of time-related academic behavior: procrastination and timely engagement. Learning and Individual Differences, 25 , 35–44.
Tanner, J. R., Stewart, G., Maples, G. M., & Totaro, M. W. (2009). How business students spend their time—do they really know? Research in Higher Education Journal, 3 , 1–9.
Terenzini, P., Rendon, L., Upcraft, M. L., Millar, S., Allison, K., Gregg, P., & Jalomo, R. (1994). The transition to college: diverse students, diverse stories. Research in Higher Education, 35 , 57–73.
Trueman, M., & Hartley, J. (1996). A comparison between the time-management skills and academic performance of mature and traditional-entry university students. Higher Education, 32 , 199–215.
Truschel, J., & Reedy, D. L. (2009). National survey—what is a learning center in the 21st century? Learning Assistance Review, 14 , 9–22.
Tsai, H. C., & Liu, S. H. (2015). Relationships between time-management skills, Facebook interpersonal skills and academic achievement among junior high school students. Social Psychology of Education, 18 , 503–516.
U.S. Department of Education, Institute of Education Sciences, What Works Clearinghouse (2016). Studies of interventions for students in developmental education intervention report: first year experience courses for students in developmental education . Retrieved from http://whatworks.ed.gov . Accessed 20 Jan 2020.
Urdan, T., & Schoenfelder, E. (2006). Classroom effects on student motivation: goal structures, social relationships, and competence beliefs. Journal of School Psychology, 44 , 331–349.
Usher, E. L., & Pajares, F. (2008). Sources of self-efficacy in school: critical review of the literature and future directions. Review of Educational Research, 78 , 751–796.
van Den Hurk, M. (2006). The relation between self-regulated strategies and individual study time, prepared participation and achievement in a problem-based curriculum. Active Learning in Higher Education, 7 , 155–169.
van der Meer, J., Jansen, E., & Torenbeek, M. (2010). ‘It’s almost a mindset that teachers need to change’: first-year students’ need to be inducted into time management. Studies in Higher Education, 35 , 777–791.
van Eerde, W. (2015). Time management and procrastination. In M. D. Mumford & M. Frese (Eds.), The psychology of planning in organizations: research and applications (pp. 312–333). New York: Routledge.
Wagner, P., Schober, B., & Spiel, C. (2008). Time students spend working at home for school. Learning and Instruction, 18 , 309–320.
Weiner, B. (2005). Motivation from an attribution perspective and the social psychology of perceived competence. In A. Elliot & C. Dweck (Eds.), Handbook of competence and motivation (pp. 73–84). New York: Guildford.
Weinstein, C. E., Palmer, D. R., & Acee, T. W. (2016). LASSI user’s manual (3th ed.). Clearwater: H & H Pub.
Winne, P. H. (1995). Inherent details in self-regulated learning. Educational Psychologist, 30 , 173–187.
Winne, P. H., & Baker, R. (2013). The potentials of educational data mining for researching metacognition, motivation, and self-regulated learning. Journal of Educational Data Mining, 5 , 1–8.
Winne, P., & Hadwin, A. (1998). Studying as self-regulated learning. In D. Hacker, J. Dunlosky, & A. Graesser (Eds.), Metacognition in educational theory and practice (pp. 277–304). Mahwah: Erlbaum.
Winne, P. H., & Hadwin, A. F. (2008). The weave of motivation and self-regulated learning. Motivation and self-regulated learning: theory, research, and applications (pp. 297–314). New York: Taylor & Francis.
Winne, P., & Perry, N. (2000). Measuring self-regulated learning. In M. Boekaerts, P. Pintrich, & M. Zeidner (Eds.), Handbook of self-regulation (pp. 531–566). San Diego: Academic Press.
Witkow, M. R. (2009). Academic achievement and adolescents’ daily time use in the social and academic domains. Journal of Research on Adolescence, 19 , 151–172.
Wolters, C. A. (2003). Regulation of motivation: evaluating an underemphasized aspect of self-regulated learning. Educational Psychologist, 38 , 189–205.
Wolters, C. A. (2011). Regulation of motivation: contextual and social aspects. Teachers College Record, 113 , 265–283.
Wolters, C. A., & Gonzalez, A. L. (2008). Classroom climate and motivation: a step toward integration. Advances in Motivation and Achievement: Social Psychological Influences, 15 , 493–519.
Wolters, C. A., & Hoops, L. D. (2015). Self-regulated learning interventions for motivationally disengaged college students. In T. L. Cleary (Ed.), Self-regulated learning interventions with at-risk youth: Enhancing adaptability, performance, and well-being (pp. 67–88). Washington: American Psychological Association.
Wolters, C., & Taylor, D. (2012). A self-regulated learning perspective on student engagement. In S. L. Christenson, A. Reschly, & C. Wylie (Eds.), Handbook of research on student engagement (pp. 635–651). New York: Springer.
Wolters, C. A., & Won, S. (2018). Validity and the use of self-report questionnaires to assess self-regulated learning. In D. H. Schunk & J. A. Greene (Eds.), Handbook of self-regulation of learning and performance (pp. 307–322). New York: Routledge.
Wolters, C. A., Won, S., & Hussain, M. (2017). Examining the relations of time management and procrastination within a model of self-regulated learning. Metacognition and Learning, 12 , 381–399.
Won, S., & Yu, S. L. (2018). Relations of perceived parental autonomy support and control with adolescents’ academic time management and procrastination. Learning and Individual Differences, 61 , 205–215.
Woolfolk, A. E., & Woolfolk, R. L. (1986). Time management: an experimental investigation. Journal of School Psychology, 24 , 267–275.
Xie, K., Heddy, B. C., & Greene, B. A. (2019). Affordances of using mobile technology to support experience-sampling method in examining college students’ engagement. Computers & Education, 128 , 183–198.
Xu, J. (2008). Models of secondary school students’ interest in homework: a multilevel analysis. American Educational Research Journal, 45 , 1180–1205.
Xu, J. (2010). Predicting homework time management at the secondary school level: a multilevel analysis. Learning and Individual Differences, 20 , 34–39.
Xu, J., Du, J., & Fan, X. (2013). “Finding our time”: predicting students’ time management in online collaborative groupwork. Computers & Education, 69 , 139–147.
Xu, J., Yuan, R., Xu, B., & Xu, M. (2014). Modeling students’ time management in math homework. Learning and Individual Differences, 34 , 33–42.
Yamada, M., Goda, Y., Matsuda, T., Saito, Y., Kato, H., & Miyagawa, H. (2016). How does self-regulated learning relate to active procrastination and other learning behaviors? Journal of Computing in Higher Education, 28 , 326–343.
Young, D. G., & Hopp, J. M. (2014). 2012-2013 national survey of first-year seminars: exploring high-impact practices in the first college year (Research report No. 4) . Columbia: University of South Carolina.
Zimbardo, P., & Boyd, J. (1999). Putting time in perspective: a valid, reliable individual-differences metric. Journal of Personality and Social Psychology, 77 , 1271–1288.
Zimmerman, B. J. (1989). A social cognitive view of self-regulated academic learning. Journal of Educational Psychology, 81 , 329–339.
Zimmerman, B. J. (2000). Attaining self-regulation: a social cognitive perspective. In M. Boekaerts, P. R. Pintrich, & M. Zeidner (Eds.), Handbook of self-regulation: theory, research, and applications (pp. 13–29). San Diego: Academic Press.
Zimmerman, B. J., & Risemberg, R. (1997). Becoming a self-regulated writer: a social cognitive perspective. Contemporary Educational Psychology, 22 , 73–101.
Zimmerman, B. J., & Schunk, D. (2012). Motivation: an essential dimension of self-regulated learning. In D. Schunk & B. Zimmerman (Eds.), Motivation and self-regulated learning: theory, research and applications (pp. 1–30). New York: Routledge.
Zimmerman, B. J., Greenberg, D., & Weinstein, C. E. (1994). Self-regulating academic study time: a strategy approach. In D. H. Schunk & B. J. Zimmerman (Eds.), Self-regulation of learning and performance: issues and educational applications (pp. 181–199). Hillsdale: Erlbaum.
Zusho, A. (2017). Toward an integrated model of student learning in the college classroom. Educational Psychology Review, 29 , 301–324.
Zusho, A., & Pintrich, P. (2003). Skill and will: the role of motivation and cognition in the learning of college chemistry. International Journal of Science Education, 25 , 1081–1094.
Download references
Author information
Authors and affiliations.
Dennis Learning Center, The Ohio State University, 250B Younkin Success Building, 1640 Neil Ave., Columbus, OH, 43201, USA
Christopher A. Wolters & Anna C. Brady
You can also search for this author in PubMed Google Scholar
Corresponding author
Correspondence to Christopher A. Wolters .
Ethics declarations
Conflict of interest.
The authors declare that they have no conflict of interest.
Additional information
Publisher’s note.
Springer Nature remains neutral with regard to jurisdictional claims in published maps and institutional affiliations.
Rights and permissions
Reprints and permissions
About this article
Wolters, C.A., Brady, A.C. College Students’ Time Management: a Self-Regulated Learning Perspective. Educ Psychol Rev 33 , 1319–1351 (2021). https://doi.org/10.1007/s10648-020-09519-z
Download citation
Published : 27 October 2020
Issue Date : December 2021
DOI : https://doi.org/10.1007/s10648-020-09519-z
Share this article
Anyone you share the following link with will be able to read this content:
Sorry, a shareable link is not currently available for this article.
Provided by the Springer Nature SharedIt content-sharing initiative
- Self-regulated learning
- Time management
- Postsecondary students
- Find a journal
- Publish with us
- Track your research
Accessibility Links
- Skip to content
- Skip to search IOPscience
- Skip to Journals list
- Accessibility help
- Accessibility Help
Click here to close this panel.
Purpose-led Publishing is a coalition of three not-for-profit publishers in the field of physical sciences: AIP Publishing, the American Physical Society and IOP Publishing.
Together, as publishers that will always put purpose above profit, we have defined a set of industry standards that underpin high-quality, ethical scholarly communications.
We are proudly declaring that science is our only shareholder.
The Impact of Time Management on Students' Academic Achievement
S N A M Razali 1 , M S Rusiman 1 , W S Gan 1 and N Arbin 2
Published under licence by IOP Publishing Ltd Journal of Physics: Conference Series , Volume 995 , International Seminar on Mathematics and Physics in Sciences and Technology 2017 (ISMAP 2017) 28–29 October 2017, Hotel Katerina, Malaysia Citation S N A M Razali et al 2018 J. Phys.: Conf. Ser. 995 012042 DOI 10.1088/1742-6596/995/1/012042
Article metrics
113017 Total downloads
Share this article
Author affiliations.
1 Department of Mathematics and Statistic, Faculty of Applied Science and Technology University Tun Hussein Onn Malaysia, Batu Pahat, Johor, Malaysia.
2 Department of Mathematic, Faculty of Science and Mathematics, Universiti Pendidikan Sultan Idris, 35900 Perak, Malaysia.
Buy this article in print
Time management is very important and it may actually affect individual's overall performance and achievements. Students nowadays always commented that they do not have enough time to complete all the tasks assigned to them. In addition, a university environment's flexibility and freedom can derail students who have not mastered time management skills. Therefore, the aim of this study is to determine the relationship between the time management and academic achievement of the students. The factor analysis result showed three main factors associated with time management which can be classified as time planning, time attitudes and time wasting. The result also indicated that gender and races of students show no significant differences in time management behaviours. While year of study and faculty of students reveal the significant differences in the time management behaviours. Meanwhile, all the time management behaviours are significantly positively related to academic achievement of students although the relationship is weak. Time planning is the most significant correlated predictor.
Export citation and abstract BibTeX RIS
Content from this work may be used under the terms of the Creative Commons Attribution 3.0 licence . Any further distribution of this work must maintain attribution to the author(s) and the title of the work, journal citation and DOI.
EMPIRICAL STUDY article
Self-regulation of time: the importance of time estimation accuracy.
A correction has been applied to this article in:
Corrigendum: Self-regulation of time: The importance of time estimation accuracy
- Read correction
- 1 Department of Curriculum, Foundations, and Reading, College of Education, Georgia Southern University, Statesboro, GA, United States
- 2 Dennis Learning Center, Department of Educational Studies, College of Education and Human Ecology, The Ohio State University, Columbus, OH, United States
- 3 Department of Educational Studies, College of Education and Human Ecology, The Ohio State University, Columbus, OH, United States
Time management is one central aspect of students’ self-regulated learning. In addition, biased time estimation seems to be central to students’ self-regulation of their time. In this study, we explored college students’ time estimation bias. In addition, we were interested in whether the activation of task beliefs influenced students’ time estimation bias and how specific beliefs about task difficulty influence time estimation bias. Findings suggested that students tended to demonstrate bias in their estimations of the time their academic tasks would take. Additionally, the activation of task beliefs did not influence students’ time estimation accuracy. Finally, both prior task difficulty and anticipated difficulty influenced students’ time estimation bias. These findings highlight the complexity of students’ time estimation bias and point to the opportunities for future directions.
Introduction
Life as a college student includes a wide range of activities and responsibilities. The effective management of tasks, particularly academic tasks, is essential for college students’ success ( Zimmerman et al., 1994 ). One way that students manage their academic tasks and other responsibilities is through time management strategies. Researchers have suggested that the process of time management is important for academic success ( Claessens et al., 2007 ; Kitsantas et al., 2008 ; Aeon and Aguinis, 2017 ). Due to the clear link between time management and academic performance, researchers have turned their attention toward understanding the specific processes involved in time management ( Wolters and Brady, 2021 ). The purpose of the present study was to investigate one process related to time management: time estimation bias. Specifically, the present study investigated college students’ tendency to accurately estimate the amount of time it would take them to complete academic tasks. Using a quasi-experimental approach, we also examined whether activating task beliefs influences students’ time estimation bias. In addition, we examined the impact of perceived difficulty on students’ time estimation.
Time estimation
Time estimation is the process of approximating the amount of time a task will take prior to beginning the task ( Buehler et al., 1994 ). To appropriately plan for the completion of academic tasks, a student must be able to realistically identify how much time a task will take ( Wolters and Brady, 2021 ). The majority of research focused on time estimation has investigated time estimation accuracy and bias (as reviewed by Halkjelsvik and Jørgensen, 2012 ; Buehler and Griffin, 2015 ). Although research tends to suggest that people are biased in their time estimation predictions, there is not agreement on whether individuals tend to overestimate or underestimate their time on tasks ( Halkjelsvik and Jørgensen, 2012 ). Some researchers have argued that people tend to underestimate how long tasks will take ( Buehler et al., 1997 ; Roy et al., 2005 ; Boltz and Yum, 2010 ; Buehler and Griffin, 2015 ), while others have noted that the body of work focused on time estimation varies in whether people over or underestimate their time on tasks ( Halkjelsvik and Jørgensen, 2012 ). Early research on this topic termed individuals’ biased estimations as the “planning fallacy” ( Kahneman and Tversky, 1977 ).
People’s time estimation has been commonly investigated using two different research designs: naturalistic studies focused on long-term tasks and experimental studies focused on short-term tasks. In addition, researchers have distinguished between performance time predictions (i.e., estimating how many minutes a task will take) and completion time predictions (i.e., estimating the particular date or time when a task will be completed; Halkjelsvik and Jørgensen, 2012 ). Naturalistic studies focus on authentic academic and non-academic tasks that individuals plan to complete by asking individuals to estimate the date by which they plan to complete a particular task. Typically, these studies focus on multifaceted and important longer-term tasks that individuals plan to complete over multiple episodes of engagement ( Buehler et al., 1994 ; Weick and Guinote, 2010 ). In addition, in these studies researchers do not typically examine the number of minutes tasks will take; rather, they investigate inconsistencies in the date by which individuals expect to complete their task. For example, Buehler et al. (1994) asked undergraduate students who were completing an honors thesis to estimate the date on which they planned to submit their thesis. They found that students tended to demonstrate inaccuracy in the date they planned to submit their honors thesis by; they estimated submitting it earlier than the date they actually submitted their thesis ( Buehler et al., 1994 ). A different study conducted by Weick and Guinote (2010) asked 20 undergraduate students to estimate their completion of a final course project in their psychology course. Students reported when they expected to complete their first draft of the course assignment, their final draft, and when they expected to submit their course project. Researchers followed up on actual completion dates 2 weeks later and found that students tended to estimate submitting their course project earlier than the actual date they submitted their course project.
While these studies are useful in understanding the way students might estimate time for their long-term goals on important tasks, they might not be as useful in understanding how students plan for short-term routine tasks. Because long-term tasks, such as an honors thesis or the rough draft of a course project, tend to be composed of smaller, everyday tasks, it is of critical importance for researchers also to consider students’ time estimation for short-term academic tasks. For example, an honors thesis is composed of many smaller tasks. While writing a thesis, a student must complete each smaller section of the paper (e.g., literature review, method, results, discussion). In addition, in these naturalistic studies, researchers tend to examine time estimation bias by focusing on the deadline by which students submit their projects. The time estimation on these smaller sections is likely important for the time estimation on the larger task.
Researchers who have focused on short-term tasks (i.e., tasks that can be completed in one relatively brief episode) have tended to adopt an experimental design. Typically, during these studies, college students are asked to estimate how long a novel task or a series of novel tasks will take in a laboratory. Then, researchers time how long the task or series of tasks takes to understand students’ time estimation accuracy. For example, in one study researchers asked students to estimate how long it would take them to sort a pile of 100 psychology journals in chronological order ( Boltz and Yum, 2010 ). Boltz and Yum (2010) offered support for an individual’s tendency to underestimate the time these tasks would take; 72% of participants displayed an underestimation bias. In a different study, undergraduate students were asked to estimate how long it would take them to sort 500 sheets of paper into 10 equal stacks ( Roy et al., 2008 ). The results from this study suggested that students tended to underestimate how long the sorting task would take.
Although research conducted within a laboratory offers a better understanding of time estimation bias on short-term tasks, this design is not necessarily representative of the way students typically complete academic activities or the types of academic tasks students face. When college students complete academic tasks, they must organize their schedules on their own accord. For each academic task, they can often choose when to complete the task and how much time they plan to spend on the academic task. Additionally, the tasks that they complete do not tend to be novel. Students are likely to have had some level of previous experience to inform their time estimations. For instance, most students write many papers throughout their college career. Therefore, the process of writing papers becomes a routine academic task.
Taken together, it is clear that an important next step in time estimation research is to examine students’ time estimation bias on authentic, short-term tasks. In order to address this need, our study focuses on actual academic tasks that students planned to complete in the coming week. In doing so, the present study seeks to understand whether activation of task beliefs might influence time estimation bias.
Factors related to time estimation
Because much of the previous literature has suggested that individuals tend to demonstrate bias in their time estimations ( Buehler et al., 1997 ; Roy et al., 2008 ; Boltz and Yum, 2010 ; Weick and Guinote, 2010 ), researchers have turned their attention toward understanding factors that might influence time estimation accuracy. One line of research has examined how feedback and reflections on previous tasks can prompt more accurate time estimation ( Roy et al., 2005 , 2008 ; König et al., 2015 ), while a second line of research has examined the impact of individual’s motivational beliefs on time estimation bias ( Buehler et al., 1997 ).
One factor thought to influence time estimation bias for current tasks is the activation of metacognitive knowledge of time estimation. Researchers have examined how the feedback on previous instances of time estimation relates to time estimation on future tasks. Roy et al. (2008) conducted three experimental studies which manipulated whether individuals were given the number of minutes it took them to complete the task previously or the number of minutes it took another person to complete the task previously. When individuals were given information about how they performed on previous tasks or how others performed on the task, they tended to be more accurate in their estimations. These findings were consistent for tasks that were novel, lab tasks (e.g., sorting papers) and tasks that were commonplace (e.g., pumping gas). This suggests that feedback on inaccurate time estimations might prompt more accurate time estimation. A different study suggested that consideration of previous incorrect estimations of dissimilar tasks can prompt students to be more accurate on future tasks ( König et al., 2015 ). In this study, two dissimilar tasks were completed in an hour-and-a-half long session. Undergraduate students were more accurate in their time estimations when completing the second task compared to the first. This suggests that activation of incorrect experiences of time estimation might affect students’ future time estimation accuracy. In a review of research, Roy et al. (2005) highlighted “remembering the past” as one intervention researchers have used to improve the accuracy and bias of people’s time estimation (pp. 747). In general, this manipulation has not successfully improved estimation accuracy. Roy et al. (2005) argues that this could be because people are not accurately remembering their past durations. Taken together, there is inconsistency in how reflections on prior experiences influence future estimations. This could be rooted in the focus of the reflection. Studies have tended to focus on reflecting on prior task durations, but it could be important to consider other aspects of the academic task, such as task difficulty.
A second line of research focused on understanding time estimation has investigated the impact of motivation. Research has suggested a negative relationship between motivation and time estimation accuracy ( Buehler et al., 1997 ). Indeed, Buehler et al. (1997) conducted two studies that examined the relationship between motivation and time estimation. In the first study, individuals were asked when they planned to submit their tax return, then were later surveyed on the date they actually submitted their return. The authors considered the amount of money individuals were receiving in their tax return as a proxy for motivation to get the task completed. Results indicated that those who had larger tax refunds tended to be less accurate in predicting when they would finish the task compared to individuals who had smaller tax refunds. In study two, Buehler et al. (1997) replicated this finding with experimental methods by using money as incentives for early completion of a word generation task. Undergraduates who were offered money for early task completion tended to underestimate how long the planned tasks would take.
While these studies offer important implications for the impact of monetary incentives on individual’s time estimation accuracy, the assumption that these monetary incentives impacted individual’s motivation for the task is debateable. For example, those who have larger tax returns might also have more complex tax returns, thus making it more challenging to estimate the date they planned to submit their returns. The present study seeks to add to this work integrating the two lines of research presented above. We examined how the activation of task difficulty beliefs (i.e., prior task difficulty and anticipated task difficulty) may influence students’ time estimations. In doing so, we add to the literature by elaborating on the aspects of time estimation that might be most important for students to consider.
A self-regulated learning view of time estimation
Although time estimation has tended to receive attention outside of the framework of time management, the process of time estimation is likely an important part of the larger system through which students control the amount of time they devote to academic tasks ( Burt and Kemp, 1994 ; Francis-Smythe and Robertson, 1999 ; Wolters and Brady, 2021 ). In general, self-regulated learning can be defined as the active processes that students engage in as they complete academic tasks to regulate their learning ( Pintrich and Zusho, 2007 ). Typically, self-regulated learning frameworks identify phases that occur before, during, and after students engage in an academic task ( Panadero, 2017 ). During these phases, students can plan, monitor, control, and reflect on aspects of their learning ( Zimmerman, 1990 ; Pintrich and Zusho, 2007 ). Self-regulated learning researchers describe time management as a series of processes that individuals can engage to plan, monitor, control, and reflect on their time ( Wolters and Brady, 2021 ). Researchers have suggested that time estimation may be particularly important as students create plans for their task completion ( Wolters and Brady, 2021 ). Of course, time estimation represents just one foundational aspect of time management. Time management involves additional subprocesses and is influenced by a range of factors. As an example, people might experience the passage of time differently ( Reunanen, 2015 ).
Self-regulated learning is not the only framework that works to explain students’ goal attainment and academic engagement. For example, Gollwitzer (1999) suggested that people may create implementation attentions, where they create conditional statements explaining when, where, and how they will engage in particular processes. These implementation intentions are informed by goals. Prior research has suggested that the creation of implementation intentions improves the likelihood that individuals will complete their goals and are less likely to demonstrate unrealistic optimism (calculated using predicted and actual duration of the task; Koole and Van’t Spijker, 2000 ). Similarly, Taylor et al. (1998) argued that mental simulation can reduce the likelihood that individuals demonstrate biased task duration predictions. In addition, one study rooted in frameworks of self-regulated learning examined a five-week intervention intended to improve students’ study time calibration ( Follmer et al., 2022 ). This study suggests that providing opportunities for students to practice estimating their time on academic tasks may lead to less biased estimations.
Central to frameworks self-regulated learning is the idea that prior task experiences influence future task experiences. Indeed, following the completion of an academic task, students may reflect on their task experience and consider effectiveness of their approaches ( Zimmerman, 1990 ). This information is later used in future self-regulated learning cycles when students active their prior metacognitive knowledge about a particular task. Thus, students’ time estimation bias may be influenced by the activation of prior experiences with similar academic tasks. Based on frameworks of self-regulated learning, the process of time estimation might involve thoughtful consideration of a student’s (1) previous experience with the task, (2) expectations about the task, and (3) the obstacles or challenges a student anticipates when completing the task.
Present study
The goal of this study was to expand on previous literature by investigating college students’ time estimation bias on routine, short-term tasks. First, we were interested in the time estimation bias on these academic tasks. Consistent with previous research ( Buehler et al., 1997 ; Boltz and Yum, 2010 ; Buehler and Griffin, 2015 ), we hypothesized that students’ time estimations would be inaccurate. Specifically, we expected students to underestimate how long their academic tasks would take. Second, we investigated whether reflecting on aspects of the task would prompt students to be more accurate in their time estimation. Because research has suggested a link between previous experience with tasks and future experiences with tasks ( Roy et al., 2008 ; König et al., 2015 ), we hypothesized that students who activated their task beliefs would be more accurate in their time estimation compared to students who did not. Finally, we examined the impact of students’ task related beliefs on their time estimation accuracy. Specifically, we were interested in the connection between students’ perceptions of similarity to previous tasks, difficulty of previous tasks, and difficulty of the current task to time estimation accuracy. We expected the difficulty of the previous and future task to exacerbate students’ inaccurate time estimation. This was based on previous research focused on the impact of students’ motivation on time estimation ( Buehler et al., 1997 ). The specific research questions we examined were: (1) To what extent are college students time estimations biased?, (2) How does activation of task beliefs impact students’ time estimation bias?, and (3) How does perceived difficulty of prior tasks and anticipated difficulty of the current task relate to time estimation bias?
Materials and methods
Participants.
Participants ( N = 210, 58% male) were undergraduates enrolled in a learning to learn course at a large Midwestern university. All academic ranks were represented (13% first-years, 34% second-years, 26% third-years, 27% fourth-years). Students’ racial and ethnic background were based on university records; students were white (62%), Asian (11%), Black or African American (11%), two or more races (7%), or other (9%).
As a part of a unit focused on time management during the fifth week of the semester, all students in the course completed a two-part assignment on time estimation. However, only students who provided consent to use their data for research were included in our analyses. The assignment was accessed through the course’s learning management system. It directed students to choose an academic task that they planned to complete during the coming week and to report information about that task (i.e., type of task and goal statement). Students were specifically instructed to choose a task that they expected to complete in one sitting. After describing the task, students were randomly and surreptitiously assigned to one of two groups. Students assigned to the experimental group ( n = 107) were required to complete four questions intended to activate their task beliefs ( Table 1 ). Questions focused on their past experiences with the type of task they had selected, their expectations about the task, and the obstacles they expected to face while completing the task. Students in the Control group ( n = 103) did not complete these questions. Hence, the one difference between the two groups was students’ engagement in a short, structured experience reflecting on their past experiences with similar types of tasks. Following this manipulation, all students were asked to estimate where they planned to complete the task, the day they planned to complete the task, and the time they planned to begin their task. Additionally, all students were asked to estimate how many minutes they expected the task to take. All of this information was entered into the course assignment. At the end of part 1 of the time estimation assignment, students were asked to track their actual location, day, time, and number of minutes associated with their task.
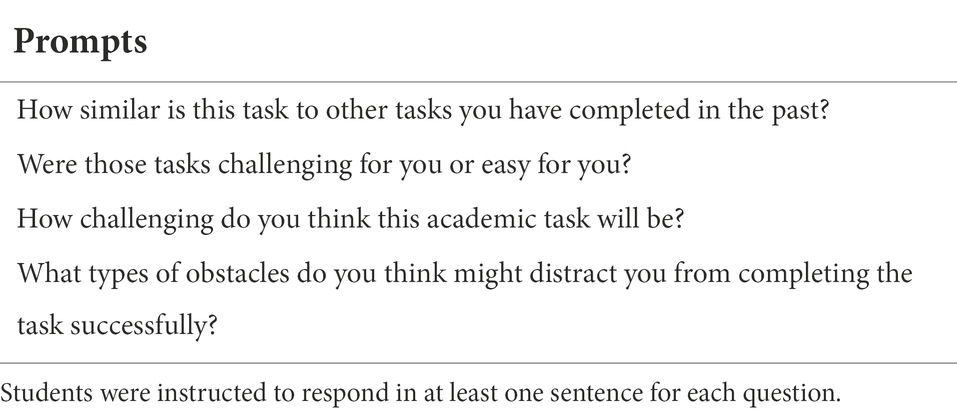
Table 1 . Prompts completed by the experimental condition during time estimation assignment part 1.
Students were instructed to complete part 2 of the assignment after they had finished their chosen task. Part 2 of the time estimation assignment was the same for all students. Part 2 of the time estimation assignment prompted students to provide information about their actual experiences completing their academic task. The first prompt asked students if they had, in fact, completed the academic task they had selected for the assignment. If students reported that they did not complete the task they were directed to open-ended questions focused on time management and time estimation and removed from our study. If students did report that they completed their academic task, they were asked to report the location, day, and time they completed their chosen task. Additionally, they were asked to report the number of minutes the task took. For the final portion of the assignment, students were asked open-ended questions focused on time management and time estimation.
Task beliefs
Students’ prior perceived difficulty and anticipated difficulty with the task they had selected to use for the assignment were assessed using separate open-ended questions that were coded using steps outlined by Saldaña (2016) . The first author and an undergraduate research assistant completed the coding process. First, the first author read all responses and created a coding system. Then, the research assistant was trained in the coding system. Both the first author and the research assistant independently coded a portion of responses ( n = 27) to assess interrater reliability.
We used one of the activation questions (i.e., “Were those tasks challenging for you or easy for you?”) from the assignment to assess perceived prior difficulty. Students’ open-ended responses were categorized using codes for easy, moderately challenging, and very challenging. The interrater reliability between coders was 81%.
Anticipated difficulty was assessed using a second activation question (i.e., “How challenging do you think this academic task will be?”). Similar to previous challenge, students’ responses were coded into three categories easy, moderately challenging, and very challenging. The interrater reliability was 78%.
In part 1 of the assignment, students reported the predicted task duration (i.e., how many minutes they expected the task to take). Approximately 1 week later and after the task had been completed, they reported the actual task duration (i.e., the number of minutes the task actually took). Similar to previous studies ( Boltz and Yum, 2010 ), a time estimation ratio was calculated as the predicted duration of task divided by actual duration of task. If a students’ ratio was less than 1, they had underestimated how long a task would take. A ratio above 1 indicated that students overestimated how long a task would take. Finally, a ratio of 1 indicated that a student’s predicted and actual time duration were the same.
To what extent were students’ time estimations accurate?
The range, means, and standard deviations for students’ time estimation ratios, predicted task durations, and actual task durations are presented in Table 2 . Students’ time estimation ratio scores ranged from.29 to 12. In terms of frequency, more students underestimated how long their selected tasks would take (47.1%) than overestimated how long their tasks would take (31.9%). A notable and surprising percentage of students (21%) reported that the actual time they devoted to the task was exactly as they had predicted. The average time estimation ratio was 1.27 ( SD = 1.04). The time estimation ratio was not normally distributed; thus a box-cox transformation ( Box and Cox, 1964 ) was conducted to allow for parametric analyses. The range, means, and standard deviations for the transformed time estimation ratios is presented in Table 2 . All further analyses were conducted on the transformed time estimation ratio. 1
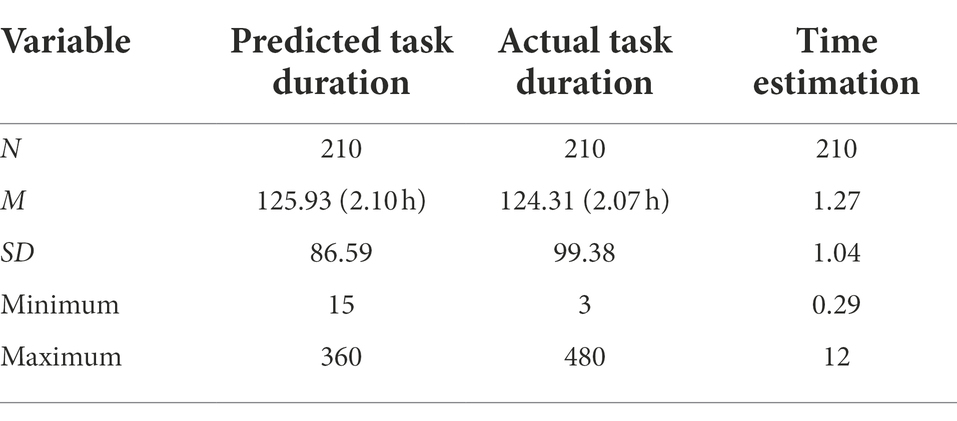
Table 2 . Means, standard deviations, and range of minutes spent on tasks and time estimation ratios.
Students chose the specific academic task that they planned to complete in the coming week. To ensure that there were no significant differences in time estimation bias based the particular type of task students completed, we coded tasks into three categories: reading, homework assignment, and studying for an exam. Based on results from a one-way ANOVA, there were no significant differences in students transformed time estimation ratios based on the type of task (Welch’s F (98.61, 2) = 2.29, p = 0.106).
Does reflecting on tasks improve time estimation accuracy?
The transformed time estimation ratios for the experimental group were compared to those for the control group. A one-way ANOVA indicated no significant differences in time estimation ratios between the experimental and the control group (Welch’s F (198.27, 1) = 3.53, p = 0.06). Thus, the hypothesis that the activation of task beliefs would impact students’ time estimation bias was not supported.
Do perceptions of the task difficulty relate to time estimation accuracy?
Analyses focused on understanding the relationship between the perceptions of task difficulty and time estimation bias included exclusively students in the experimental group ( n = 107). A one-way ANOVA was conducted to determine whether there were differences in students’ transformed time estimation ratios based on the reported difficulty of prior tasks.
As depicted in Table 3 , results indicated that students’ time estimations were significantly different based on prior difficulty (Welch’s F (65.58, 2) = 5.03, p = 0.009). Pairwise comparisons were performed using the Games-Howell procedure. This post hoc analysis indicated significant differences in transformed time estimation ratios for students whose prior academic tasks were easy ( M = −0.01) versus challenging ( M = 0.29; p = 0.007). This finding suggests that prior challenge influences students’ time estimation bias.
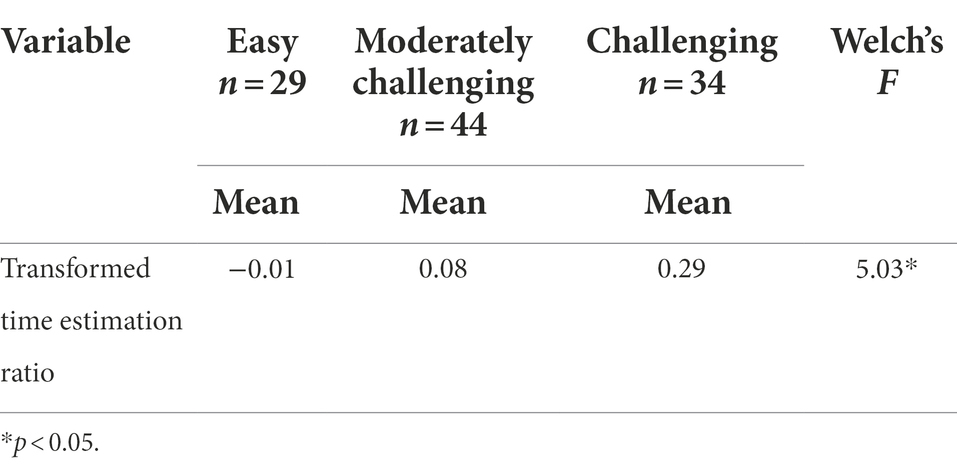
Table 3 . One-way ANOVA comparing transformed time estimation ratios based on reported difficulty of previous academic task.
Similarly, a one-way ANOVA was conducted to examine differences in students’ transformed time estimation ratios based on anticipated task difficulty ( Table 4 ). Results indicated significant differences in transformed time estimation ratios based on students’ anticipated difficulty (Welch’s F (46.95, 2) = 3.97, p = 0.03). Subsequently, Games-Howell was used to perform pairwise comparisons. Post hoc analyses indicated significant differences between tasks that were expected to be easy ( M = 0.03) and challenging ( M = 0.34; p = 0.03). In addition, there were significant differences between tasks that were expected to be moderately challenging ( M = 0.06) and challenging ( M = 0.34; p = 0.04). This finding suggests that anticipated challenge influences students’ time estimation accuracy.
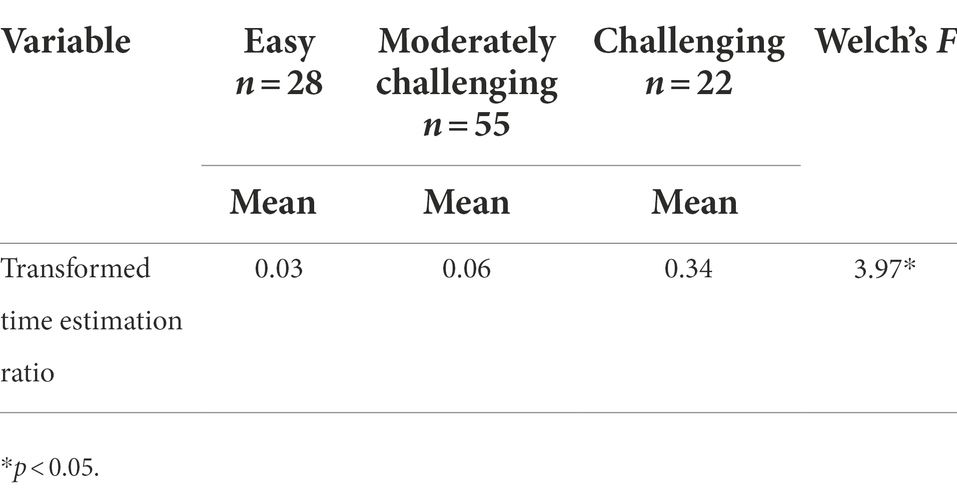
Table 4 . One-way ANOVA comparing transformed time estimation ratios based on reported anticipated difficulty.
The ability to manage time effectively is an essential skill for college students ( Kitsantas et al., 2008 ; Wolters et al., 2017 ). One process related to time management that could be especially important is students’ time estimation. Previous research has suggested that individuals tend to be biased in their time estimations ( Buehler and Griffin, 2015 ). Because of this bias, researchers have worked to examine factors that might improve individual’s time estimation accuracy ( Buehler et al., 1997 ; Roy et al., 2008 ; König et al., 2015 ). The purpose of the present study was to investigate whether the activation of task beliefs influenced students’ time estimation.
Time estimation bias
The majority of students demonstrated biased time estimations. When comparing students who underestimated their time on academic tasks and those who overestimated their time on academic tasks, a greater number of students underestimated their time on academic tasks in the present study. This finding is in line with prior studies that have suggested that people tend to underestimate their time on tasks ( Buehler and Griffin, 2015 ).
We hypothesized that the majority of students would underestimate the amount of time it would take to complete academic tasks, thus it was interesting that a large group of students overestimated their time on academic tasks. There are at least two potential explanations for this overestimation. First, it could be that when college students consider short-term, typical tasks they are more likely to overestimate the amount of time tasks will take rather than underestimate. Previous studies tended to find underestimation when individuals were asked to report on novel, experimental tasks ( Roy et al., 2008 ; Boltz and Yum, 2010 ) or when individuals completed typical, long-term tasks ( Buehler et al., 1994 , 1997 ). In contrast to previous studies, the students in this study chose tasks with which they tended to be familiar. Even when the content of tasks was not similar to previous tasks, the types of tasks students completed were routine college tasks (e.g., reading, studying for exams, and completing homework assignments). Additionally, students chose tasks that they planned to complete in one episode, rather than tasks that would take place over multiple episodes.
A second reason why students might have overestimated the amount of time for their academic tasks could be related to the context of the study. Students completed this assignment during the time management unit of a learning to learn course ( Hofer et al., 1998 ). Receiving direct time management instruction might have encouraged some students to be more deliberate when estimating their time on academic tasks. These students might have strategically reported predicted task durations that were longer than expected to ensure they had enough time to engage in their academic task. When comparing overestimation and underestimation, the consequences of planning too few minutes for an academic task seem to outweigh the consequences of planning too many minutes for an academic task. For example, if a student is planning to complete a lab report and allots one hour of time, if that student does not finish the lab report in the allotted time, then they might not turn in a completed lab report. In contrast, if a student allots two hours to finish their lab report and finishes the lab report in one and a half hours, then the student is able to turn in a completed lab report.
Future researchers should continue to assess the time estimation bias of college students. In particular, to clarify the reasons why students might tend to overestimate or underestimate, additional studies should use process-oriented approaches to examine the way students estimate their time. For example, think-aloud protocols could be a useful way to examine why students might overestimate or underestimate how long tasks would take. This would help researchers better understand the underlying mechanism of time estimation.
Influences on time estimation bias
A second major finding from this study was that students who activated their task beliefs and students who did not activate their beliefs showed similar patterns in their time estimation bias. This was surprising based on previous literature that suggested that consideration of prior experiences completing similar tasks may be a viable way to improve the accuracy of students’ time estimation ( Roy et al., 2008 ; König et al., 2015 ) and literature suggesting the importance of activation of beliefs during self-regulated learning ( Pintrich and Zusho, 2007 ).
There are at least two potential reasons why activation of beliefs about prior difficulty, anticipated obstacles, and anticipated difficulty might not have influenced time estimation in this study. First, it could be that the activation questions did not stimulate deep enough thought about students’ beliefs. In the present study, the assignment instructions asked students to respond to each question in at least one sentence. Many students who responded to the prompts did not provide one sentence, rather they provided a couple of words. For example, in response to the question “How similar is this task to other tasks you have completed in the past?” many students responded simply “similar.” When students provided one-word responses, they might not have been thinking deeply enough about their past task experiences. For instance, although students considered the task difficulty, it might have been more useful to contemplate the specific aspects of the task that they perceived as most difficult.
A second reason why the activation questions might not have prompted more accurate time estimations is due to the focus of the questions. It might be more important for students to consider different task-related beliefs (i.e., beyond prior difficulty, anticipated obstacles, and anticipated difficulty) to encourage more effective time estimation. For example, a question focused on the specific struggles students faced during previous academic tasks might have provided additional information to appropriately plan for the current academic task, thus impacting students’ time estimation accuracy. Additionally, it could be that students’ reflections prompted the recall of inaccurate memories about prior task experiences ( Roy et al., 2005 ). Biased or inaccurate memories may have led to biased or inaccurate plans.
To continue to develop an understanding of the impact of the activation of task beliefs on students’ time estimation accuracy, future researchers could prompt deeper engagement in reflections. For instance, rather than asking students to provide a one-sentence reflection their prior difficulty, anticipated obstacles, and anticipated difficulty, researchers might ask students to respond in one paragraph or require them to address prompts for specific information that demand greater reflection and recollection of past experiences. Questions could focus on other beliefs held by students (e.g., motivational beliefs) and other perceptions of the task (e.g., task complexity).
The importance of perceived difficulty
A third major finding of the present study related to students’ specific beliefs about their academic tasks. Findings suggested differences in students’ time estimation ratios based on perceived prior and anticipated task difficulty. In addition to emphasizing the importance of perceived difficulty, this finding also may suggest that variation in students’ time estimation biases could be due to the specific beliefs they hold rather than whether those beliefs are explicitly activated prior to beginning an academic task. In addition, these findings suggest that difficulty could be especially important as students enact their time management processes.
In general, this seems to be in line with prior research that suggests that individual’s perceptions influence their time estimation accuracy ( Buehler et al., 1997 ) and that task beliefs influence student’s self-regulated learning processes ( Pintrich and Zusho, 2007 ). Of course, students’ perceptions of prior and anticipated difficulty are likely closely connected to their task experiences. As an example, difficulty may be related to students’ familiarity with a task. As prior research has suggested relationships between time estimation bias and familiarity with tasks ( Boltz et al., 1998 ; Roy and Christenfeld, 2007 ), future research is needed to disentangle the task experiences and beliefs that relate to time estimation bias.
Limitations and future directions
When considering the implications of this study, it is important to acknowledge a couple of limitations. First, all students who participated in the present study were enrolled in a learning-to-learn course, which focused on improving students’ use of self-regulated learning strategies. Future research should explore similar research questions with students who are not enrolled in a learning-to-learn course.
In addition, there are a few minor aspects of the time estimation assignment directions that might have affected the study results. First, the instructions asked students to choose a task that they planned to complete in the coming week. Although we gave students an opportunity to indicate that they did not end up completing the academic task, the majority of students might have been more likely to stick to their time estimation plan because they felt like it was part of their course assignment. This is particularly true for students who completed more flexible assignments, like reading or studying for an exam. In addition, factors outside of the scope of this study may influence time estimation and, more broadly, time management. Future studies should investigate college students’ time estimation bias outside of a learning to learn course assignment. This might alleviate additional pressure students feel to follow-through with their plan because of course assignment, making the study more ecologically valid. This may also allow researchers to explore additional factors that influence time management.
In light of the limitations associated with this study, future research should continue to explore time estimation accuracy in ecologically valid contexts with different groups of students. Exploring the factors that impact students’ time estimation continues to be an important goal because of the potential connection to students’ self-regulated learning and time management. Viewing time estimation through a lens of self-regulated learning offers specific factors that could be investigated in relation to time estimation accuracy, like students’ motivational beliefs, other time management strategies, or the tendency to procrastinate. Better understanding the relationship between time estimation and other aspects of self-regulated learning would provide insights into the role of time estimation.
Time management has been viewed as an important process for college students’ academic success ( Zimmerman et al., 1994 ; Claessens et al., 2007 ; Kitsantas et al., 2008 ; Wolters et al., 2017 ). Time estimation is one potentially important aspect of time management. This study contributes to previous work focused on time management and time estimation by investigating students’ time estimation accuracy for typical short-term academic tasks. Findings indicate that students tend to be biased in their time estimations, and that reflecting on previous tasks does not influence students’ time estimation bias. In addition, findings highlight that both prior task difficulty and anticipated difficulty may influence students’ time estimation bias.
Data availability statement
The datasets presented in this article are not readily available because we did not receive IRB approval to share the data sets. Requests to access the datasets should be directed to [email protected] .
Ethics statement
The studies involving human participants were reviewed and approved by The Ohio State University - IRB. The patients/participants provided their written informed consent to participate in this study.
Author contributions
AB collected and analyzed the data and completed the majority of the writing. CW helped with the study design, data analysis approach, and revised writing. SY helped with the study design and revised the writing. All authors contributed to the article and approved the submitted version.
Acknowledgments
The authors would like to thank Katherine Kachnowski for her help with data analysis.
Conflict of interest
The authors declare that the research was conducted in the absence of any commercial or financial relationships that could be construed as a potential conflict of interest.
Publisher’s note
All claims expressed in this article are solely those of the authors and do not necessarily represent those of their affiliated organizations, or those of the publisher, the editors and the reviewers. Any product that may be evaluated in this article, or claim that may be made by its manufacturer, is not guaranteed or endorsed by the publisher.
1. ^ All analyses were also conducted using Kruskal–Wallis H -tests, the nonparametric version of the ANOVA. The Kruskal–Wallis H -tests produced similar results.
Aeon, B., and Aguinis, H. (2017). It’s about time: new perspectives and insights on time management. Acad. Manag. Perspect. 31, 309–330. doi: 10.5465/amp.2016.0166
CrossRef Full Text | Google Scholar
Boltz, M. G., Kupperman, C., and Dunne, J. (1998). The role of learning in remembered duration. Mem. Cogn. 26, 903–921. doi: 10.3758/BF03201172
PubMed Abstract | CrossRef Full Text | Google Scholar
Boltz, M. G., and Yum, Y. N. (2010). Temporal concepts and predicted duration judgments. J. Exp. Soc. Psychol. 46, 895–904. doi: 10.1016/j.jesp.2010.07.002
Box, G. E., and Cox, D. R. (1964). An analysis of transformations. J. R. Stat. Soc. Series B Methodol. 26, 211–243.
Google Scholar
Buehler, R., and Griffin, D. (2015). “When plans lead to optimistic forecasts,” in The Psychology in Planning in Organizations: Research and Applications . eds. M. D. Mumford and M. Frese (New York: Routledge), 31–57.
Buehler, R., Griffin, D., and MacDonald, H. (1997). The role of motivated reasoning in optimistic time predictions. Personal. Soc. Psychol. Bull. 23, 238–247. doi: 10.1177/0146167297233003
Buehler, R., Griffin, D., and Ross, M. (1994). Exploring the “planning fallacy”: why people underestimate their task completion times. J. Pers. Soc. Psychol. 67, 366–381. doi: 10.1037/0022-3514.67.3.366
Burt, C. D. B., and Kemp, S. (1994). Construction of activity duration and time management potential. Appl. Cogn. Psychol. 8, 155–168. doi: 10.1002/acp.2350080206
Claessens, B. J. C., van Eerde, W., Rutte, C. G., and Roe, R. A. (2007). A review of the time management literature. Pers. Rev. 36, 255–276. doi: 10.1108/00483480710726136
Follmer, D. J., Patchan, M., and Spitznogle, R. (2022). Supporting college learners’ study time calibration: relations to course achievement and self-regulated learning skills. J. Coll. Reading Learn. 52, 75–96. doi: 10.1080/10790195.2022.2033646
Francis-Smythe, J. A., and Robertson, I. T. (1999). On the relationship between time management and time estimation. Br. J. Psychol. 90, 333–347. doi: 10.1086/250095
Gollwitzer, P. M. (1999). Implementation intentions: strong effects of simple plans. Am. Psychol 54, 493–503. doi: 10.1037/0003-066X.54.7.493
Halkjelsvik, T., and Jørgensen, M. (2012). From origami to software development: a review of studies on judgment-based predictions of performance time. Psychol. Bull. 138, 238–271. doi: 10.1037/a0025996
Hofer, B. K., Yu, S. L., and Pintrich, P. R. (1998). “Teaching college students to be self-regulated learners,” in Self-Regulated Learning: From Teaching to Self-Reflective Practice . eds. D. H. Schunk and B. J. Zimmerman (New York: Guilford), 57–85.
Kahneman, D., and Tversky, A. (1977). Intuitive Prediction: Biases and Corrective Procedures . Decisions and designs Inc. McLean, VA.
Kitsantas, A., Winsler, A., and Huie, F. (2008). Self-regulation and ability predictors of academic success during college: a predictive validity study. J. Adv. Acad. 20, 42–68. doi: 10.4219/jaa-2008-867
König, C. J., Wirz, A., Thomas, K. E., and Weidmann, R.-Z. (2015). The effects of previous misestimation of task duration on estimating future task duration. Curr. Psychol. 34, 1–13. doi: 10.1007/s12144-014-9236-3
Koole, S., and Van’t Spijker, M. (2000). Overcoming the planning fallacy through willpower: effects of implementation intentions on actual and predicted task-completion times. Eur. J. Soc. Psychol. 30, 873–888. doi: 10.1002/1099-0992(200011/12)30:6<873::AID-EJSP22>3.0.CO;2-U
Panadero, E. (2017). A review of self-regulated learning: six models and four directions for research. Front. Psychol. 8:422. doi: 10.3389/fpsyg.2017.00422
Pintrich, P. R., and Zusho, A. (2007). “Student motivation and self-regulated learning in the college classroom,” in The Scholarship of Teaching and Learning in Higher Education: An Evidence-Based Practice . ed. R. Murray (New York: Open University Press), 731–810.
Reunanen, T. (2015). Human factor in time management. Procedia Manuf. 3, 709–716. doi: 10.1016/j.promfg.2015.07.311
Roy, M. M., and Christenfeld, N. J. S. (2007). Bias in memory predicts bias in estimation of future task duration. Mem. Cogn. 35, 557–564. doi: 10.3758/BF03193294
Roy, M. M., Christenfeld, N. J. S., and McKenzie, C. R. M. (2005). Underestimating the duration of future events: memory incorrectly used or memory bias? Psychol. Bull. 131, 738–756. doi: 10.1037/0033-2909.131.5.738
Roy, M. M., Mitten, S. T., and Christenfeld, N. J. S. (2008). Correcting memory improves accuracy of predicted task duration. J. Exp. Psychol. Appl. 14, 266–275. doi: 10.1037/1076-898X.14.3.266
Saldaña, J. (2016). The Coding Manual for Qualitative Researchers . London, England: SAGE.
Taylor, S. E., Pharn, L. B., Rivkin, I. D., and Armor, D. A. (1998). Harnessing the imagination: mental stimulation, self-regulation, and coping. Am. Psychol. 53, 429–439. doi: 10.1037/0003-066X.53.4.429
Weick, M., and Guinote, A. (2010). How long will it take? Power biases time predictions. J. Exp. Soc. Psychol. 46, 595–604. doi: 10.1016/j.jesp.2010.03.005
Wolters, C. A., and Brady, A. C. (2021). College students’ time management: a self-regulated learning perspective. Educ. Psychol. Rev. 33, 1319–1351. doi: 10.1007/s10648-020-09519-z
Wolters, C. A., Won, S., and Hussain, M. (2017). Examining the relations of time management and procrastination within a model of self-regulated learning. Metacogn. Learn. 12, 381–399. doi: 10.1007/s11409-017-9174-1
Zimmerman, B. J. (1990). Self-regulated learning and academic achievement: an overview. Educ. Psychol. 25, 3–17. doi: 10.1207/s15326985ep2501
Zimmerman, B. J., Greenberg, D., and Weinstein, C. E. (1994). “Self-regulating academic study time: a strategy approach,” in Self-Regulation of Learning and Performance: Issues in Educational Applications . eds. D. H. Schunk and B. J. Zimmerman (New Jersey: Lawrence Erlbaum Associates), vol. 195, W299–W301.
Keywords: self-regulated learning, time management, time estimation, planning fallacy, college students
Citation: Brady AC, Wolters CA and Yu SL (2022) Self-regulation of time: The importance of time estimation accuracy. Front. Psychol . 13:925812. doi: 10.3389/fpsyg.2022.925812
Received: 21 April 2022; Accepted: 05 October 2022; Published: 24 October 2022.
Reviewed by:
Copyright © 2022 Brady, Wolters and Yu. This is an open-access article distributed under the terms of the Creative Commons Attribution License (CC BY) . The use, distribution or reproduction in other forums is permitted, provided the original author(s) and the copyright owner(s) are credited and that the original publication in this journal is cited, in accordance with accepted academic practice. No use, distribution or reproduction is permitted which does not comply with these terms.
*Correspondence: Anna C. Brady, [email protected]
Disclaimer: All claims expressed in this article are solely those of the authors and do not necessarily represent those of their affiliated organizations, or those of the publisher, the editors and the reviewers. Any product that may be evaluated in this article or claim that may be made by its manufacturer is not guaranteed or endorsed by the publisher.
Users agree that automated translations may not effectively convert the intended design, meaning, and/or context of the website, may not translate images or PDF content, and may not take into account regional language differences. Any person that uses the translated site does so at that person’s own risk. UGA Extension is not responsible for any damages, costs, liability, or risk associated with any use, functionality, and/or content of the website translations.
For more information, visit the Language Translation page.
Time Management: 10 Strategies for Better Time Management
The term Time Management is a misnomer. You cannot manage time; you manage the events in your life in relation to time. You may often wish for more time, but you only get 24 hours, 1,440 minutes, or 86,400 seconds each day. How you use that time depends on skills learned through self-analysis, planning, evaluation, and self-control. Much like money, time is both valuable and limited. It must be protected, used wisely, and budgeted.
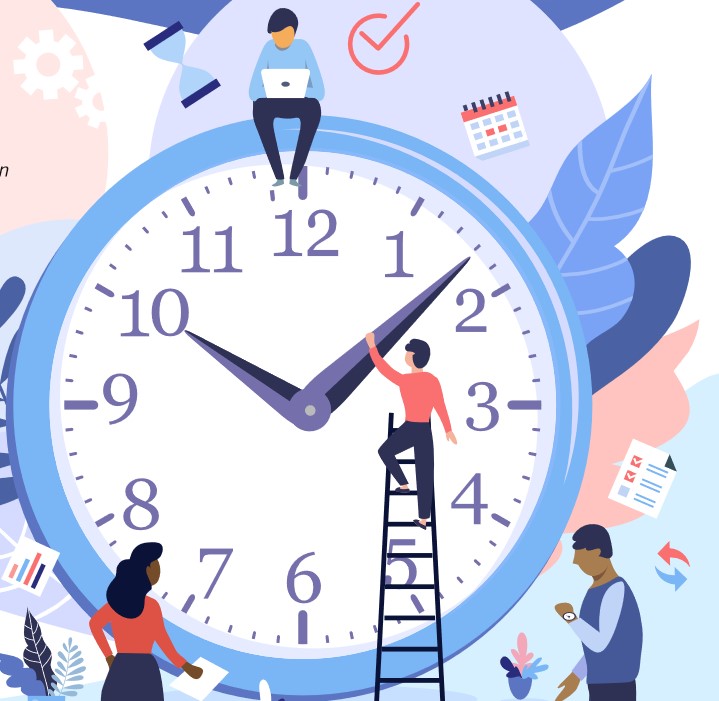
- Are more productive.
- Have more energy for things they need to accomplish.
- Feel less stressed.
- Have more free time to do the things they want.
- Get more things done.
- Relate more positively to others.
- Feel better about themselves. (Dodd and Subdheim, 2005)
Finding a time management strategy that works best for you depends on your personality, ability to self-motivate, and level of self-discipline. By incorporating some, or all the ten strategies below, you can more effectively manage your time.
1. Know How You Spend Your Time
A time log is a helpful way to determine how you use your time. Record what you are doing in 15-minute intervals for a week or two. Evaluate the results:
- Did everything you needed to do get done?
- Which tasks require the most time?
- What time of day when you are most productive?
- Where is most of your time is devoted (i.e. job, family, personal, recreation)?
Identifying your most time-consuming tasks and determining whether you are investing your time in the most important activities can help you to determine a course of action. Having a good sense of the time required for routine tasks can help you be more realistic in planning and estimating how much time is available for other activities. Many apps exist to help you keep track of your time, as mentioned in Strategy 3.
2. Set Priorities
Managing your time effectively requires a distinction between what is important and what is urgent (MacKenzie, 1990). Experts agree that the most important tasks usually aren’t the most urgent tasks. However, we tend to let the urgent tasks dominate our lives. Covey, Merrill, and Merrill (1994) categorize activities into four quadrants in their Time Management Matrix: urgent, not urgent, important, and not important. While activities that are both urgent and important must be done, Covey et al. suggests spending less time on activities that are not important (regardless of their urgency) to gain time for activities that are not urgent but important. Focusing on these important activities allows you to gain greater control over your time and may reduce the number of important tasks that become urgent.
Creating a "to do” list is an easy way to prioritize. Whether you need a daily, weekly, or monthly list depends on your lifestyle. Be careful to keep list-making from getting out of control. List manageable tasks rather than goals or multi-step plans. Rank the items on your “to do” list in order of priority (both important and urgent). You may choose to group items in categories such as high priority, medium priority, or low priority; number them in order of priority; or use a color-coding system. The goal is not to mark off the most items, but to mark off the highest priority items (MacKenzie, 1990). A prioritized “to do” list allows you to set boundaries so you can say “no” to activities that may be interesting or provide a sense of achievement but do not fit your basic priorities.
3. Use a Planning Tool
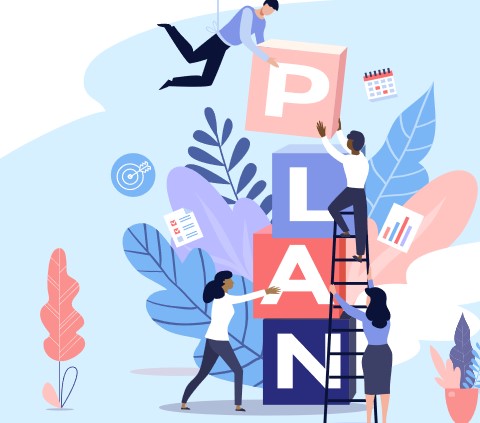
When using a planning tool:
- Always record your information on the tool itself. Jotting notes elsewhere that must be transferred later is inefficient and wastes more time.
- Review your planning tool daily.
- Keep a list of your priorities in your planning tool and refer to it often.
- Keep planning tools synchronized. If you keep more than one, make sure your phone, computer, and paper planning tools match.
- Keep a back-up system.
Apps on your phone can be great planning tools. Apps typically fall into one of the following categories:
- Time Trackers – Gain an awareness of how you spend your time.
- Time Savers – Increase productivity and break time-wasting habits.
- Task Managers – Prioritize and organize tasks to improve time management.
- Habit Developers – Create healthy habits to encourage time management.
4. Get Organized
Disorganization leads to poor time management. Research has shown that clutter has a strong negative impact on perceived well-being (Roster, 2016). To improve your time management, get organized.
Set up three boxes (or corners of a room) labeled "Keep," "Give Away," and "Toss." Sort items into these boxes. Discard items in your “Toss” box. Your "Give Away" box may include items you want to sell, donate, or discard.
The next step is to improve the time you spend processing information. For example, tasks such as email can eat up your day. To combat wasted time, implement an email organization system that allows you to process the information in each email as efficiently as possible. Use folders, flagging, or a color-coded system to keep track of what’s what.
5. Schedule Appropriately
Scheduling is more than just recording what must be done (e.g., meetings and appointments). Be sure to build in time for the things you want to do. Effective scheduling requires you to know yourself. Your time log should help you to identify times when you are most productive and alert. Plan your most challenging tasks for when you have the most energy. Block out time for your high priority activities first and protect that time from interruptions.
Schedule small tasks such as drafting an email, creating a grocery shopping list, reading, watching webinars or listening to podcasts for long commutes or when waiting for a call or appointment. Capitalize on what would otherwise be time lost. Avoid nonproductive activities, such as playing games or scrolling through social media. Limit scheduled time to about three-fourths of your day to allow for creative activities such as planning, dreaming, and thinking.
6. Delegate: Get Help from Others
Delegating means assigning responsibility for a task to someone else, freeing up your time for tasks that require your expertise. Identify tasks others can do and select the appropriate person(s) to do them. Select someone with the appropriate skills, experience, interest, and authority needed to accomplish the task. Be specific. Define the task and your expectations while allowing the person some freedom to personalize the task. Check how well the person is progressing periodically and provide any assistance, being careful not to take over the responsibility. Finally, reward the person for a job well done or make suggestions for improvements if needed. (Dodd and Sundheim, 2005). Another way to get help is to “buy” time by obtaining goods or services that save time. For example, paying someone to mow your lawn or clean your house, or joining a carpool for your children’s extracurricular activities frees time for other activities. The time-savings from hiring someone for specialized projects is often worth the cost.
7. Stop Procrastinating
People put off tasks for a variety of reasons. Perhaps the task seems overwhelming or unpleasant. To help stop procrastination, consider “eating the big frog first.” A quote commonly attributed to Mark Twain says, “If it’s your job to eat a frog today, it’s best to do it first thing in the morning. And if it’s your job to eat two frogs, it’s best to eat the big frog first.” Unpleasant tasks we procrastinate completing are “big frogs.” Complete these tasks as your first action of the day to get them out of the way. Another option is to “snowball” your tasks by breaking them down into smaller segments, completing preparatory tasks, and eventually completing the larger task at hand. Whether you choose the “big frog first” or “snowball” method, try building in a reward system for completed tasks to help stay motivated.
8. Manage Time-Wasters
Reduce or eliminate time spent in these activities by implementing some simple tips.
Handheld Devices
- Take advantage of voice-to-text features such as transcribed voicemails or to make notes or draft emails and text messages when you are on the go.
- Avoid small talk. Stay focused.
- Take any necessary action immediately following a call.
- Impose screen time limits and regularly monitor your digital wellness (see Strategy 10).
- Schedule breaks from your devices.
- Set aside a specific time to view and respond to email, but don’t let it accumulate to the point it becomes overwhelming to sort.
- Turn off notifications for email.
- Handle each item only once if possible.
- Immediately delete or unsubscribe from junk emails.
- Keep address books up-to-date and organized.
- Utilize built-in shortcuts to sort email.
Unexpected Visitors
- Schedule time for face-to-face visits.
- Inform visitors of your time constraints and politely offer to reschedule.
- Set a mutually agreeable time limit for the visit.
- When someone comes to the door, stand up and have your meeting standing to help keep it brief.
In-Person and Virtual Meetings
- Know the purpose of the meeting in advance.
- Arrive early.
- Start and end the meeting on time.
- Prepare an agenda and stick to it. Use a timed agenda, if necessary.
- Don’t schedule meetings unless they are necessary and have a specific purpose or agenda.
- Use recording software or designate a note-taker.
Family Obligations
- Use and sync virtual calendars for easy sharing between busy family members.
- Make each family member responsible for consulting the master calendar for potential conflicts.
- Create a central area or agreed upon app for posting communications such as appointment reminders, announcements, and messages.
9. Avoid Multi-tasking
Psychological studies have shown that multi-tasking does not save time. In fact, the opposite is often true. You lose time when switching from one task to another, resulting in a loss of productivity (Rubinsteim, Meyer, and Evans, 2001). Routine multi-tasking may lead to difficulty in concentrating and maintaining focus. Do your best to focus on just one task at a time by keeping your area clear of distractions, including turning off notifications on your devices, and set aside dedicated time for specific tasks.
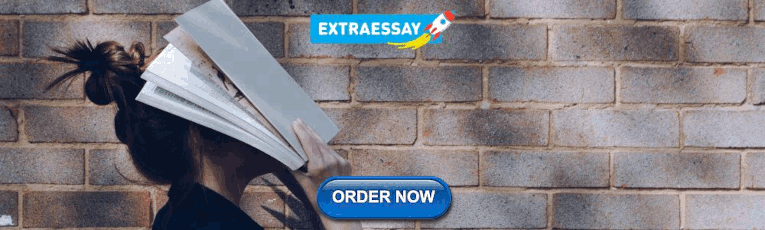
10. Stay Healthy
The care and attention you give yourself is an important investment of time. Scheduling time to relax or do nothing helps you rejuvenate physically and mentally, enabling you to accomplish tasks more quickly and easily. Be sure to monitor your screen time as a part of your digital well-being, setting boundaries to stay healthy. A study conducted by Google showed that four out of five study participants who took steps to improve their digital well-being believe their overall well-being was positively impacted as well (Google, 2019). To improve your digital well-being, set time limits or utilizing built-in software on electronic devices such as phones and tablets to help maintain your digital wellness. Blue light blockers and grayscale mode may also help you improve your digital well-being. Set a time each night to shut off all digital devices to give your mind time to relax; this can also help improve your sleep schedule.
Unfortunately, poor time management and too much screen time can result in fatigue, moodiness, and more frequent illness. To reduce stress, reward yourself for time management successes. Take time to recognize that you have accomplished a major task or challenge before moving on to the next activity.
Whatever time management strategies you use, take time to evaluate how they have worked for you. Do you have a healthy balance between work and home life? Are you accomplishing the tasks that are most important in your life? Are you investing enough time in your own personal well being? If the answer is “no” to any of these questions, then reevaluate your time management strategies and transition to ones that will work better for you. Successful time management leads to greater personal happiness, more accomplishments at home and at work, and a more satisfying future.
Previously updated by: Roxie Price, University of Georgia Extension Dana Carney, University of Georgia Extension Rachael Clews, K-State Research and Extension
Originally written by: Sue W. Chapman, retired, UGA Extension Michael Rupured, retired, UGA Extension
Covey, S. R., Merrill, A. R., & Merrill, R. R. (1994). First things first: To live, to love, to learn, to leave a legacy . Simon & Schuster.
Dodd, P., & Sundheim, D. (2005). The 25 best time management tools and techniques: How to get more done without driving yourself crazy . Peak Performance Press, Inc.
Google, Global (DE, ES, FR, IT, PL, U.K., U.S.). (2019). Digital wellbeing survey (General population, 18+ years, n=97).
MacKenzie, A. (1990). The time trap (3rd ed.). American Management Association.
Roster, C., Ferrari, J., & Jurkat, M. (2016, March 16). The dark side of home: Assessing possession ‘clutter’ on subjective well-being. Journal of Environmental Psychology , 46 , 32–41. https://doi.org/10.1016/j.jenvp.2016.03.003
Rubinsteim, J., Meyer, D., & Evans, J. (2001). Executive control of cognitive processes in task switching. Journal of Experimental Psychology: Human Perception and Performance, 27 (4), 763–797. https://doi.org/10.1037/0096-1523.27.4.763
Status and Revision History Published with Full Review on Apr 25, 2014 Published with Minor Revisions on Aug 26, 2020 Published with Full Review on Feb 19, 2024
Have a question?
Related publications.
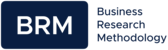
The Importance of Time Management
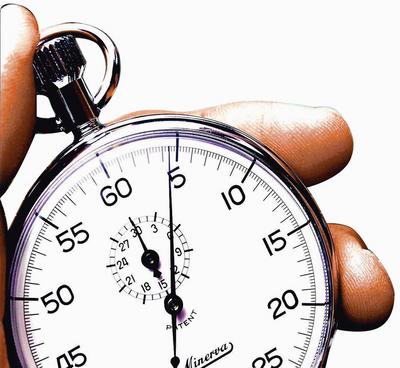
The definition of the concept of time has been associated with ambiguities and even contradictions. For instance, time has been described as “time nothing more than the occurrence of events in sequence, on after another” (Olpin and Hessen, 2012, p.167), and “a resource that, when lost or misplaced, is gone forever” (Kerzner, 2009, p.286).
The definition of time management has been offered as “the process of skilfully applying time to finish and perfect a specific activity within time constraint” (Harris, 2008, p.22). However, there is no single definition of time that fully captures all aspects of the concept.
Becker and Mustric (2008) divide time into two categories: quantitative and qualitative. It can be specified that “quantitative time is seen to represent time as quantities, that is, time can be measured and counted into seconds, minutes, days, weeks, months, and years” (Wu, 2009, p.5). Qualitative time, on the other hand, is associated with a meaning of an activity the time is spent on.
Similar to the concept of time, there are varying opinions amongst secondary data authors regarding the definition and role of time management. For instance, it has been noted that “time management is about taking charge, carefully, consciously, purposefully – not shrinking from difficulties, but engaging them” (Kristan, 2010, p.4). Moreover, some secondary data authors also note that “time management is not an end itself. It is the means to an end. When linked with setting and meeting goals it provided a way of getting really high achievement out of individuals and others with whom they come into contact” (Pearson and Thomas, 2010, p.49).
Marquis and Huston (2009), on the other hand, disagree with the term of time management, and argue that time cannot be managed in any ways, but rather individuals can manage events in their lives in relation to time.
At the same time, it has been warned by some secondary data authors that “time management must not be seen as only concerned with packing more activity on the available time, though this may be part of it; it must be in ensuring that objectives are met” (Forsyth, 2010, p.13). Moreover, it has also been stated that “time management is not about creating more time but rather about making the best use of the time we have” (Hansen, 2011, p.3).
The majority of publications devoted to the issues of time management discuss the advantages of effective management of time and offer techniques that can be used in order to achieve this objective.
For instance it has been stated that “when we manage our time more effectively and efficiently, we reduce stress and frustration, we become more confident, and we feel better” (Mancini, 2007, p.viii). Moreover, some secondary data authors convincingly argue that “with proper planning and some self-discipline, many urgent matters disappear altogether or, when they do surface, your planning and discipline makes them less urgent and easier to deal with” (Rivera, 2007, p.51).
Moreover, authors state that “the benefits of time management are many. Rather than running around in a state of frenzy, you feel a sense of empowerment from getting things done and having the time to enjoy life’s simple pleasures” (Seaward, 2011, p.134).
Furthermore, the additional advantages of time management have been highlighted by secondary data authors as being more productive (Green and Skinner, 2005, Alexander and Dobson, 2008), having more energy to accomplish tasks (Limoncelli, 2006, Becker and Mustric, 2008, Christie, 2009), feeling less stressed (Bhugra and Howes, 2007, Brott, 2008, Christie, 2009), possibility to pursue personal interests (Felton and Sims, 2009), getting more things done (Green and Skinner, 2005, Mustric, 2008), relating to others in more positive manner (Bhugra and Howes, 2007), and feeling better about self (Brott, 2008).
Secondary data authors stress that time management principles can be and have to be mastered by everyone regardless the nature of the their employment, age, religion or any other demographic or other variables. Namely, it has been said that “given the right intention, and motivation, it is possible for anyone to improve their time utilisation and to do so markedly of you have not thought about it recently” (Forsyth, 2010, p.8).
Major Concepts and Ideas Associated with Time Management
First of all, it is important to note that “in spite of all popular attention to managing time, relatively little research has been conducted on the process involved in using one’s time effectively (e.g. by using “prime time” to carry out important tasks) and completing work within deadlines” (Claessens et al, 2007, p.256)
According to Fleming (2011) an effective time management is closely associated with an understanding the difference between efficiency and effectiveness. Fleming (2011) clarifies that while efficiency involves completing specified tasks in a right manner, effectiveness relates to identifying the right tasks to be accomplished in the first place.
A similar viewpoint is expressed by Fleming (2011) as well, who argues that the practice of identification of strategic tasks in an appropriate manner, and the selection of appropriate tools and techniques for the completion of those tasks can be considered the most basic conditions of effective time management.
Singh (2008) points to two fundamental aspects associated with the concept of time:
a) The sequence, and more precisely, the order of the changes;
b) The duration of the changes or of the period between them.
Felton and Sims (2009), on the other hand, closely associate the concept of time management with one’s self management. The authors assert that “the focus of time management is priorities and stems from self-management. Self-management is the powerhouse of time management” (Felton and Sims, 2009, p.21).
Zeller (2008) expands this idea and stresses the importance of having an effective personal system of time management along with a comprehensive Specifically, the author reasons that “effective time management requires more than good intent and self-knowledge” (Zeller, 2008, p.14), and a good system consisting of specific processes need to be put in place in order to engaged in time management in an efficient manner. The specific processes involved in time management are specified by Downs (2008) as setting priorities, analysing, filtering, scheduling and executing.
The importance of environmental factors in effective management of time has been mentioned by Singh (2008), Forsyth (2010), and Silvis (2011). All of these authors give credit to Schematic Model of Factors Affecting Time Use proposed by Robinson (1977) in terms of classification and presentation of environmental factors affecting use of time in an effective manner. Moreover, Singh (2008) argues that despite the fact that more than three decades have passed from the introduction of Schematic Model of Factors Affecting Time Use the importance of the model has not been compromised.
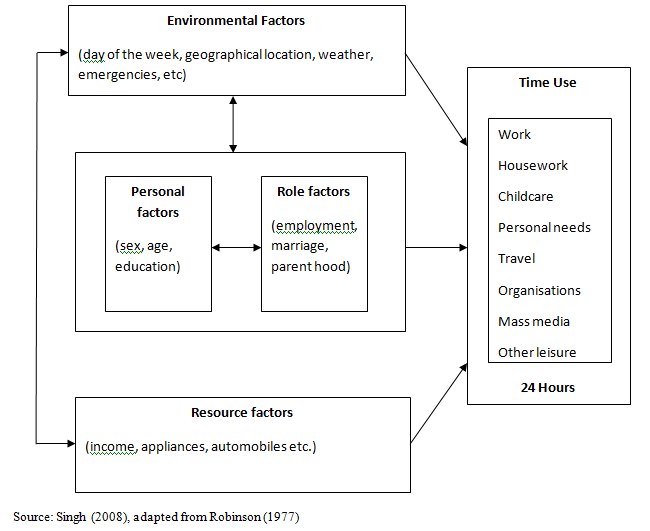
Adair, J & Allen, M. (1999) “Time Management and Personal Development” Hawksmere Alexander, R & Dobson, M.S. (2008) “Real-world time management” AMACOM Barling, J., Kelloway, E. K. & Cheung, D. (1996). “Time management and achievement striving interact to predict car sale performance” Journal of Applied Psychology , 81 , 821-826. Becker, H & Mustric, F. (2008) “Can I Have 5 Minutes of Your Time?” Morgan James Publishing Beevers, R. (2006) “Customer Service Excellence in the Public Sector”, Northern Housing Consortium Bhugra, D. & Howes, O. (2007) “Handbook of Psychiatric Trainees” RCPsych Publications Blaxter, L, Hughes,C & Tight, M. (2006) “How To Research” 3 rd edition, McGraw-Hill Bond, M. & Feather, N. (1988) “Some correlates of structure and purpose in the use of time” Journal of Personality and Social Psychology Boone, LE & Kurtz, DL (2010) “Contemporary Business” John Wiley & Sons Brink, H, Walt, C.V & Rensburg, G.V. (2005) “Fundamentals of Research Methodology for Helath-Care Professionals” Juta Online Brott, R. (2008) “Successful Time Management: Be the Productive Person You Want to Be” ANC Book Publishing Buchbinder, S.B & Thompson, J.M. (2010) “Career opportunities in health care management” Jones and Bartlett Learning Butler, G & Hope, T. (2007) “Managing Your Mind: The Mental Fitness Guide” Oxford Christie, S. (2009) “Effective time management for doctors” Developmedica Claessens, J.C., Eerde, W. & Rutte, C.G. (2007) “A Review of the time management literature” Personnel Review , Vol. 36, pp. 255 – 276 Covey, S. (1990) “ The Seven Habits of Highly Effective People” Fireside Denscombe, M. (2010) “The Good Research Guide for Small-Scale Social Research Projects” fourth edition. Denhardt, R.B. (2010) “Theories of Public Organisation” Cengage Learning Decrop, A. (2006) “Vacation decision making” CABI Dodd, P & Sundheim, D. (2011) “The 25 Best Time Management Tools and Techniques: How to Get More Done Without Driving Yourself Crazy” John Wiley & Sons Downs, L.J. (2008) “Time Management Training2 ASTD Engel, R.J. & Schutt, R.K. (2009) “Fundamentals of Social Work Research” SAGE Publications Evans, C. (2008) “Time Management for Dummies” John Wiley & Sons Felton, S & Sims, M. (2009) “Organising Your Day: Time Management Techniques That Will Work for You” Revell Fleming, I. (2011) “The Time Management Pocketbook” 6 th edition, Management Pocketbooks Flynn, N. (2007) “Public Sector Management” 5 th edition, SAGE Publications Forsyth, P. (2010) “Successful Time Management” Kogan Page Green, P & Skinner, D. (2005) “Does time management training work? An evaluation” International Journal of Training and Development Volume 9, Issue 2, pp. 124 – 139
Harris, J. (2008) “Time Management 100 Success Secrets: The 100 Most Asked Questions on Skills, Tips, Training, Tools and Techniques for Effective Time Management” Lulu Publications
Hillestad, S.G & Berkowitz, E.N. (2004) “Health care market strategy: from planning to action” Jones and Bartlett Learning Kassel, D.S. (2010) “Managing Public Sector Projects: A Strategic Framework for Success in an Era of Downsized Government” Taylor & Francis
Kerzner, H. (2009) “Project Management: A Systems Approach to Planning, Scheduling, and Controlling” John Wiley & Sons
Kristan, P. (2010) “Awakening in Time: Practical Time Management for Those on a Spiritual Path” Dog Ear Publishing
Lakein, A. (1973) “How to Get Control of Your Time and Your Life” P.H. Wyden New York Limoncelli, T. (2006) “Time management for system administrators” O’Reilly Macan, T. H. (1996 “Time management training: Effects on time behaviours, attitudes, and job performance” Journal of Psychology , 130 , 229-236 Managing Interruptions. (2011) Available at: http://www.time-management-success.com/managing-interruptions.html Accessed November 13, 2011
Marquis, B.L. & Huston, C.J. (2009) “Leadership roles and management functions in nursing: theory and application” Wolters Kluwer Health
Matsuo, M. (2005) “The role of internal competition in knowledge creation: an empirical study of Japanese firms” Peter Lang Mancini, M. (2007) “Time Management: 24 Techniques to Make Each Minute Work” McGrow-Hill Mudrack, P. E. (1997) “The structure of perceptions of time” Educational and Psychological Measurement , 57 , 222-240
Olpin, M. & Hesson, M. (2012) “Stress Management for Life: A Research – Based Experiential Approach” Cengage Learning Randel, J.(2010) “The Skinny on Time Management: How to Maximise Your 24-Hour Gift” RAND Media Co Rivera, C. (2007) “Time Management” Global Media Seaward, B.L. (2011) “Essentials of Managing Stress” Jones and Bartlett Learning Seaward, B.L & Seaward, B. (2011) “Managing Stress: Principles and Strategies for Health and Well-Being” Jones and Bartlett Learning Secunda, A. (1999) “ The 15 second principle : short, simple steps to achieving long-term goals ” Berkley Books Silvis, C. (2011) “Time Management and Organisation for Writers” in 2012 Writer’s Market Simmons, S. (2011) “9 Ways to Handle Interruptions Like a Pro” Available at: http://www.lifehack.org/articles/lifehack/9-ways-to-handle-interruptions-like-a-pro.html Accessed December, 2, 2011. Singh, U.B. (2008) “Time Management: A Study of HRD Managers” Kalpaz Publications Starling, G. (2010) “Managing the Public Sector” Wadsworth Tobis, M & Tobis, I. (2002) “Managing multiple projects” McGraw-Hill Professional Tracy, B. (2007) “Eat That Frog! 21 Great Ways to Stop Procrastinating and Get More Done in Less Time” Berrett-Koehler Store Walsh, R. (2008) “Time Management: Proven Techniques for Making Every Minute Count” Adams Media Waterworth, S. (2003) “Time management strategies in nursing practice” Journal of Advanced Nursing, Volume 43, Issue 5, pp. 432 – 440 Wirick, D.W. (2009) “Public-Sector Project Management: Meeting the Challenges and Achieving the Results” John Wiles & Sons
Wu, D. (2009) “Temporal Structures in Individual Time Management: Practices to Enhance Calendar Tool Design” IGI
Wysocki, D.K. (2007) “Readings in Social Research Methods” Cengage Learning Yager, J. (2008) “Work Less, Do More: The 14-Day Productivity Makeover, Barnes & Noble Zapico-Goni, E & Wholey, J. (2007) “Monitoring Performance in the Public Sector,” Transaction Books Zeller, D. (2008) “Successful Time Management for Dummies” For Dummies
- Submit your COVID-19 Pandemic Research
- Research Leap Manual on Academic Writing
- Conduct Your Survey Easily
- Research Tools for Primary and Secondary Research
- Useful and Reliable Article Sources for Researchers
- Tips on writing a Research Paper
- Stuck on Your Thesis Statement?
- Out of the Box
- How to Organize the Format of Your Writing
- Argumentative Versus Persuasive. Comparing the 2 Types of Academic Writing Styles
- Very Quick Academic Writing Tips and Advices
- Top 4 Quick Useful Tips for Your Introduction
- Have You Chosen the Right Topic for Your Research Paper?
- Follow These Easy 8 Steps to Write an Effective Paper
- 7 Errors in your thesis statement
- How do I even Write an Academic Paper?
- Useful Tips for Successful Academic Writing
Transformative Forces: Social Entrepreneurship as Key Competency
Virtual learning experiences among postgraduate students in namibia, collaborative governance in government administration in the field of state security along the republic of indonesia (ri)-malaysia border area.
- IT Service Management System Practices in Kenya
- Introduction Economic and Psychological Well-Being During COVID-19 Pandemic in Albania, A Need for Sustainability
- Designing a Framework for Assessing Agripreneurship Action for the Green Scheme Irrigation Projects, Namibia
- The Potential Utilisation of Artificial Intelligence (AI) in Enterprises
- Case Study – Developing a National Research and Evidence Base for The Health and Wellbeing Chapter of The Welsh Government’s 2023 Innovation Strategy for Wales
- Slide Share

The importance of effective time management in research: Strategies for improving productivity
Research is a crucial aspect of academic and professional life, but it can be challenging to balance research responsibilities with other commitments. Effective time management is essential for researchers to be productive and achieve their goals without sacrificing their personal lives. In this article, we will explore the importance of time management in research and provide strategies for improving productivity.
Importance of Time Management in Research:
Effective time management is critical in research for several reasons. First, research requires a significant amount of time, and effective time management ensures that this time is used efficiently. Poor time management can result in missed deadlines, incomplete projects, and wasted resources. Second, time management can help researchers achieve a healthy work-life balance, which is essential for maintaining well-being and avoiding burnout. Third, time management can improve research outcomes by allowing researchers to focus on their work without distractions.
Strategies for Improving Productivity:
Here are some strategies for improving productivity through effective time management:
Plan Ahead: Effective time management begins with planning. Researchers should create a research plan that includes their goals, deadlines, and milestones. Planning helps researchers stay organized and avoid last-minute rushes.
Prioritize Tasks: Not all tasks in research are equally important. Researchers should prioritize tasks based on their importance and urgency. Tasks that are urgent and important should be given priority over tasks that are less critical.
Use Time-Blocking: Time-blocking is a technique that involves scheduling specific blocks of time for different tasks. Researchers can allocate time for specific activities, such as writing, data analysis, or meetings. Time-blocking can help researchers stay focused and avoid distractions.
Take Breaks: Taking breaks is essential for maintaining productivity. Researchers should take regular breaks to rest their minds and recharge their energy. Short breaks can help researchers stay refreshed and focused.
Avoid Multitasking: Multitasking can reduce productivity and increase stress. Researchers should focus on one task at a time and avoid distractions. Switching between tasks can reduce efficiency and increase the risk of errors.
Use Productivity Tools: There are several productivity tools available that can help researchers manage their time more effectively. Tools such as calendar apps, to-do lists, and project management software can help researchers stay organized and on track.
Delegate Tasks: Delegating tasks can help researchers save time and reduce their workload. Researchers can delegate tasks to research assistants or collaborators to free up their time for more critical activities.
Effective time management is critical for researchers to achieve their goals and maintain a healthy work-life balance. By planning ahead, prioritizing tasks, using time-blocking, taking breaks, avoiding multitasking, using productivity tools, and delegating tasks, researchers can improve their productivity and achieve their research objectives. Adopting these strategies can help researchers achieve their full potential and succeed in their research endeavors.
Suggested Articles

The Role of Primary and Secondary Research Tools in Your Survey Try a New Method…

Research collaborations have become increasingly popular in recent years, with more and more researchers recognizing…

How to organize your research paper effectively Wondering about organizing your paper wisely? And here…

In today's digital age, data is an invaluable asset for researchers. However, managing research data…
Related Posts

Comments are closed.

Migrated Content
Working time around the world
According to a new ILO report, innovative working time arrangements, such as those introduced during the COVID-19 crisis, can bring benefits for economies, businesses and workers, including greater productivity and improved work-life balance.
6 January 2023
There is a substantial amount of evidence that work–life balance policies provide significant benefits to enterprises, supporting the argument that such policies are a ‘win-win’ for both employers and employees,” the report states.
- Working-time laws and regulations on maximum daily hours of work and statutory rest periods are achievements that contribute to the long-term health and well-being of a society and must not be put at risk.
- Longer hours of work are generally associated with lower unit labour productivity, while shorter hours of work are linked with higher productivity.
- Countries should make use of the experiences they developed with working-time reduction and flexibility during the COVID-19 crisis. Inclusive short-time work schemes with the highest possible allowances not only maintain employment but also sustain purchasing power and create the possibility of cushioning the effects of economic crises.
- Public policy responses are needed to promote reductions in hours of work in many countries, to promote both a healthy work-life balance and improved productivity.
- Teleworking helps maintain employment and creates new scope for employee autonomy. However, these and other types of flexible working arrangements need regulating, to contain their potential negative effects, through policies such as what is often called a “right to disconnect” from work.
Related content

Working Time and Work-Life Balance Around the World
Appointments at Mayo Clinic
- Weight loss
Exercise for weight loss: Calories burned in 1 hour
Being active can help you lose weight and keep it off. Find out how much you need.
Being active is vital to losing weight and keeping it off. When active, the body uses more energy in the form of calories. And burning more calories than you take in leads to weight loss.
To lose weight, most people need to cut the number of calories they eat and move more. This is according to the 2020-2025 Dietary Guidelines for Americans. Most often, that means cutting daily calories by 500 to 750 to lose 1 1/2 pounds (0.7 kilograms) a week.
Other factors might be involved in losing weight. Because of changes to the body over time, you might need to cut calories more as you age to keep losing weight or to stay at the same weight.
Diet or exercise: Does one matter more?
Both are important. Diet affects weight loss more than physical activity does. Physical activity, including exercise, has a stronger effect in keeping weight from coming back after weight loss.
Losing weight with diet alone and without physical activity can make people weaker. This is because of age-related losses in bone density and muscle mass. Adding resistance training and aerobic exercise to a weight-loss program helps prevent the loss of bone and muscle.
These are the exercise guidelines for most healthy adults from the U.S. Department of Health and Human Services:
Aerobic activity. Get at least 150 minutes of moderate aerobic activity a week. Or get 75 minutes of vigorous aerobic activity a week. You also can get an equal mix of the two types.
Aim to exercise most days of the week. For even more health benefits, strive for 300 minutes a week or more of moderate aerobic activity or 150 minutes of vigorous activity. Exercising this much may help with weight loss or keeping off lost weight. But even small amounts of physical activity can be helpful. Being active for short periods of time during the day can add up and give you great health benefits.
- Strength training. Do strength training exercises for all major muscle groups at least two times a week. One set of each exercise is enough for health and fitness benefits. Use a weight or resistance level heavy enough to tire your muscles after about 12 to 15 repetitions.
Moderate aerobic exercise includes activities such as brisk walking, biking, swimming and mowing the lawn.
Vigorous aerobic exercise includes activities such as running, swimming hard laps, heavy yardwork and aerobic dancing.
Strength training can include use of weights or weight machines, your own body weight, resistance tubing, or activities such as rock climbing.
How much am I burning?
This list shows about how many calories are burned while doing certain exercises for one hour. This is based on a person who weighs 160 pounds (73 kilograms). The calories you burn depend on the exercise you do, how hard you do it, how much you weigh and other factors.
Based on Ainsworth BE, et al. 2011 compendium of physical activities: A second update of codes and MET values. Medicine & Science in Sports & Exercise. 2011;43:1575.
Remember, to lose weight or to keep weight from creeping up on you as you age, you need to eat less and move more. Moving more means adding more physical activity into your life.
There is a problem with information submitted for this request. Review/update the information highlighted below and resubmit the form.
From Mayo Clinic to your inbox
Sign up for free and stay up to date on research advancements, health tips, current health topics, and expertise on managing health. Click here for an email preview.
Error Email field is required
Error Include a valid email address
To provide you with the most relevant and helpful information, and understand which information is beneficial, we may combine your email and website usage information with other information we have about you. If you are a Mayo Clinic patient, this could include protected health information. If we combine this information with your protected health information, we will treat all of that information as protected health information and will only use or disclose that information as set forth in our notice of privacy practices. You may opt-out of email communications at any time by clicking on the unsubscribe link in the e-mail.
Thank you for subscribing!
You'll soon start receiving the latest Mayo Clinic health information you requested in your inbox.
Sorry something went wrong with your subscription
Please, try again in a couple of minutes
- Physical Activity Guidelines for Americans. 2nd ed. U.S. Department of Health and Human Services. https://health.gov/paguidelines/second-edition. Accessed March 13, 2024.
- Physical activity for a healthy weight. Centers for Disease Control and Prevention. https://www.cdc.gov/healthyweight/physical_activity/index.html. Accessed March 13, 2024.
- Ainsworth BE, et al. 2011 compendium of physical activities: A second update of codes and MET values. Medicine & Science in Sports & Exercise. 2011;43:1575.
- 2020-2025 Dietary Guidelines for Americans. U.S. Department of Health and Human Services and U.S. Department of Agriculture. https://www.dietaryguidelines.gov. Accessed March 13, 2024.
- Perreault L, et al. Obesity in adults: Role of physical activity and exercise. https://www.uptodate.com/contents/search. Accessed March 13, 2024.
- AskMayoExpert. Physical activity (adult). Mayo Clinic; 2022.
Products and Services
- A Book: The Mayo Clinic Diet Bundle
- The Mayo Clinic Diet Online
- Aerobic exercise
- Hate to exercise? Try these tips
- Strength training basics
- Walking for weight loss
- Walking for fitness
Mayo Clinic does not endorse companies or products. Advertising revenue supports our not-for-profit mission.
- Opportunities
Mayo Clinic Press
Check out these best-sellers and special offers on books and newsletters from Mayo Clinic Press .
- Mayo Clinic on Incontinence - Mayo Clinic Press Mayo Clinic on Incontinence
- The Essential Diabetes Book - Mayo Clinic Press The Essential Diabetes Book
- Mayo Clinic on Hearing and Balance - Mayo Clinic Press Mayo Clinic on Hearing and Balance
- FREE Mayo Clinic Diet Assessment - Mayo Clinic Press FREE Mayo Clinic Diet Assessment
- Mayo Clinic Health Letter - FREE book - Mayo Clinic Press Mayo Clinic Health Letter - FREE book
- Healthy Lifestyle
- Exercise for weight loss Calories burned in 1 hour
Your gift holds great power – donate today!
Make your tax-deductible gift and be a part of the cutting-edge research and care that's changing medicine.
Analytics transformation in wealth management
The wealth management industry is typically seen as embodying old-fashioned values and providing discrete, tailored services. These attributes remain valuable parts of the business, but for many clients, they are no longer sufficient. In a highly connected world, people want faster and more convenient offerings and a cutting-edge digital experience. Amid rising competition, established wealth managers need to keep pace with new offerings as they retain the values that set them apart.
About the authors
This article is a collaborative effort by Anutosh Banerjee , Fumiaki Katsuki , Vishal Kaushik, Aditya Saxena, Sanchit Suneja, and Renny Thomas .
Wealth managers are unlikely to be able to serve modern clients effectively without a digitized operating model. This will support advisory and non-advisory activities and service everchanging investment preferences. Some leading managers are building modular data and IT architectures, which enable smart decision-making, personalization at scale, and more extensive product offerings. 1 For an in-depth look at how some of these elements are being developed in an overall banking context, see our collection “ Building the AI bank of the future ,” May 2021, mckinsey.com. The changes are also helping them meet their regulatory obligations, boosting the productivity of relationship managers (RMs), and lifting compressed margins.
For wealth managers interested in pursuing these benefits, this article lays out the potential of deploying advanced analytics and offers a playbook of measures that wealth managers should consider including in a digital transformation.
The case for advanced analytics
Meeting the needs of today’s customers requires a business model that is at the same time efficient and adaptable to individual clients. Wealth managers are finding success with two approaches:
- Serve clients across the wealth continuum on a flat-fee advisory basis. Instead of the still-prevalent product-focused model, wealth managers need to build in pricing flexibility aligned to clients’ needs at every stage of their lives. An increasingly common pricing model is for clients to negotiate a flat fee based on the value of their investments. To maintain revenues with this model, wealth managers need to create new efficiencies and ensure RMs are more productive, which means spending more time with clients.
- Embrace personalization aligned to client life stages and goals. Today’s customers are increasingly dissatisfied with a one-size-fits-all service model, so wealth managers should consider transitioning to needs-based personalization. This requires RMs to get comfortable with a wider range of solutions, from the simplest products to complex higher-yielding investments (private markets, venture capital, pre-IPO, and structured products). In addition, RMs must be equipped to help clients make complex investment decisions, supported by analytics.
In today’s context, each of these goals is achievable only with advanced capabilities in data and analytics, especially targeting relationship management.
Focus on relationship management
Modernization can be game changing when it targets the role of RMs. Based on conversations with industry participants, we estimate that RMs typically spend 60 to 70 percent of their time on non-revenue-generating activities, amid rising regulatory and compliance obligations (Exhibit 1). One reason is that most still work with legacy IT systems or even spreadsheets. As clients demand more engagement and remote channel options, that needs to change.
A few leading wealth managers are using technology to provide RMs with the tools to serve clients more efficiently and effectively. Some have taken a zero-based approach, rebuilding their tech stacks and embracing advanced analytics to inform more personalized services. By providing targeted solutions, these firms have been able to boost revenues and reduce operational costs.
Clear benefits of being more client focused
The benefits of digitization are relevant in most markets, but the potential to leverage digitization to achieve a significant performance uplift is especially great in regions where wealth managers have not yet seized the opportunity. In Asia, for example, many wealth managers still need to fully embrace digital ways of working (Exhibit 2). We estimate that IT-based transformations could create some $40 billion to $45 billion of incremental value for wealth managers serving high-net-worth individuals in Asia, equating to roughly 25 basis points on a wealth pool of $17 trillion. 2 Wealth management penetration in the region is 35 to 40 percent, but for the purposes of the calculation, we assume 100 percent of high-networth individuals’ personal financial assets (investable assets of more than $1 million).
Would you like to learn more about our Financial Services Practice ?
Drilling down in the potential gains from data and analytics, we see benefits in three key areas: acquisition and onboarding, engagement and deepening of client relationships, and servicing and retention.
Acquisition and onboarding. Basic acquisition and onboarding applications include client discovery, risk profiling, account opening, and onboarding. RMs and investment teams can use analytics for lead generation, share-of-wallet modeling, and automated proposals. There are also multiple applications in investment management, risk, and compliance, including social-profile checking, anti-money-laundering and know your customer, and fraud protection.
How analytics creates sustainable impact: Two examples from Asia
One leading Asian wealth manager deployed an analytics-led program to produce granular client insights that enabled it to offer responsive, timely, and personalized services to client microsegments. The bank leveraged personalization at scale to boost assets under management by 30 to 40 percent per client in six to eight months.
A second wealth manager created the position of chief investment officer to inform a bankwide view of asset classes and geographies. The CIO used analytics to link product selection to the “house view,” ensuring consistency across model portfolios.
Engagement and deepening. Client-focused applications include personalized research, portfolio management, and notifications. RMs and investment teams can implement client clustering, propensity modeling, recommendation engines, and digital performance management (see sidebar “How analytics creates sustainable impact: Two examples from Asia”). In investment management, risk, and compliance, there are opportunities to de-bias investment decisions, data analysis, and trade execution.
Servicing and retention. Client-related applications include portfolio simulations and optimization, as well as self-execution of trades. RMs can leverage applications such as churn predictors and work planners, while investment management, risk, and compliance can scale up portfolio planning and trade surveillance.
A playbook for analytics-driven wealth management
Early success stories are encouraging, but they are the exception rather than the rule. More often, firms have started the transformation journey but have faltered along the way. Common reasons include a lack of ownership at senior levels and budgetary or strategic restraints that prevent project teams from executing effectively.
The challenges of transforming service models are significant but not insurmountable. Indeed, as analytics use cases become more pervasive, implementation at scale becomes more achievable. In the following paragraphs, we present five ingredients of an analytics-based transformation (Exhibit 3). These can be supported by strong leadership, a rigorous focus on outcomes, and a willingness to embrace new ways of working. Indeed, managers who execute effectively will get ahead of the competition and be much more adept in meeting client needs.
Set a leadership vision
Analytics-driven transformations are often restricted to narrow silos occupied by a few committed experts. As a result, applications fail to pick up enough momentum to make a real difference to performance. Conversely, if support for change programs comes from the top and is guided by an outcomes-driven approach, the business can break away from entrenched operating norms and reset for structural change. With that in mind, executive teams should communicate a vision that can be cascaded through the business. They should also create a safe environment, or sandbox, for business lines to experiment before scaling.
Plot the change journey
Wealth managers have applied advanced analytics to achieving different objectives. Some have found that the application of advanced analytics to business problems delivers significant value and enables them to make better decisions faster and more consistently. Others are using data and advanced analytics to improve sales and marketing, inform investment decision-making, and boost RM productivity.
Any plan for data-driven change must fit the organization’s business model. Implementation will vary based on the technical feasibility, data accuracy and accessibility, time to impact, scalability, and availability of funds. The first few use cases will set the mood and direction, so careful thought is required ahead of action.
One common impediment to scaling is the lack of a single metric to describe impact, which makes it hard for tech teams to communicate benefits. Still, there are workarounds. Financial key performance indicators (KPIs) can show flows across key mandates or volumes of advisory, rather than execution-driven assets under management. Nonfinancial metrics can focus on cross-sell ratios, increased client retention, number of RMs trained, or adoption rates for solutions. Other helpful evaluations include customer satisfaction scores, new trust-based RM-client relationships, time to market, and cultural shifts. Progress on these measures will boost organizational conviction that transformation is beneficial.

The value of personal advice: Wealth management through the pandemic
Build a strong foundation, leading with technology.
Data and technology together form the backbone that supports analytics-led transformation. A strong analytics backbone requires a rigorous standard of data management, coupled with informed decisions about the IT applications and systems to employ.
A digital approach to client-centric servicing
A leading bank created a digital and analytics-powered application that ingests internal and external data points, enabling it to identify “hidden affluence” among its clients.
Another bank combined demographic data with information from client conversations to generate real-time product recommendations and facilitate cross-selling. To continuously train the recommendation engine, the bank built a central data lake—consolidated, centralized storage for raw, unstructured, semistructured, and structured data from multiple sources—so the system has an ever-growing set of data to work from. It then pushed product recommendations through multiple client channels.
A leading investment bank continuously scrapes more than 2,000 financial news sources and more than 800 blogs, stock message sites, and social-media platforms. This exercise helped to enrich the data used by the analytics engine to assess sentiment and inform insights on stocks, bonds, commodities, countries, currencies, and cryptocurrencies.
Wealth managers are routinely in touch with their clients offline. These interactions elicit significant information about client preferences and requirements, but the information is often stored on paper or in RMs’ heads. To mine this knowledge fully, wealth managers must capture it digitally and convert it into a structured format that can be processed to create insights and personalized services (see sidebar “A digital approach to client-centric servicing”). In doing so, they need to put systems in place to ingest, store, and organize the data in line with regulatory obligations while ensuring the data are accurate, available, and accessible.
On the technology side, some leading wealth managers use natural-language processing to analyze text and voice data and identify personalized triggers and insights. Others are building feedback loops across channels to train artificial intelligence algorithms. Technologies can also be applied to processes: robotic process automation, for example, can replace routine manual labor and mental processing in regulatory compliance, risk assessment, reporting, and query management.
Deployment of data-driven decision-making requires scalable, adaptable, and resilient core technology components—a unified data and technology stack that connects across IT activities. 3 Sven Blumberg, Rich Isenberg, Dave Kerr, Milan Mitra, and Renny Thomas, “ Beyond digital transformations: Modernizing core technology for the AI bank of the future ,” April 2021, McKinsey.com. This will enable managers to adopt a tech-first approach to designing customer journeys.
In building data and IT architecture, wealth managers require a basic tool kit with four key components:
- a rationalized IT stack to create a common front-and back-end platform and a unified resource for mobile and web applications
- a scalable data platform with modular data pipelines and application-programming-interface (API)-based microservices for building and deploying analytics solutions at scale
- a semi-autonomous lab environment to enable experimentation, coupled with an at-scale factory environment for production of analytics solutions
- a highly scalable distributed network on the cloud to respond to variable demand for data storage and processing
In parallel to assembling these components, banks must consolidate data from across geographies and business lines. This will enable analysts to elicit insights based on the maximum amount of information. Some leading players first experiment in a sandbox environment and work with external partners to acquire the necessary skills, after which they scale up incrementally.
Build the team and prioritize change management
It is not easy to scale and sustain analytics impact. Organizational silos and cultural resistance are common inhibiting factors, while the vital role that RMs play in forming and maintaining relationships must be adapted to the new environment. Indeed, RMs must be front and center of the transformation process. For this, organizations need effective team building and change management.
Team building. A productive approach to team building is to create cross-functional squads with a range of talents (Exhibit 4). Product owners and designers should be responsible for ensuring that the team meets the needs of its clients (RMs or end clients) and stays focused on delivering value. Data scientists and data engineers implement use cases and check that insights are generated as data are ingested—a minimal-viable-product (MVP) approach. IT architects and software engineers, meanwhile, build the slick interfaces and back-end systems that deliver insights to clients across channels.
How three Asian wealth managers engaged clients and boosted RM productivity
A leading private bank deployed machine learning to generate next-best conversation ideas. It built propensity models and analyzed customer clusters to identify anchor clients and learn from transaction patterns.
Another private bank built a digital workbench that enables RMs to serve clients via a single platform. The workbench was integrated with a centrally hosted recommendation engine that provides personalized recommendations based on life events and transaction data.
A third private bank used explanatory and predictive modeling to identify “moments of truth.” These informed RM coverage and outreach strategy, which helped the bank develop initiatives to support growth and focus RMs on high-value activities.
A core objective should be to explore analytics and AI use cases that boost RM productivity (see sidebar “How three Asian wealth managers engaged clients and boosted RM productivity”). To that end, the squad should embed business and channel management teams so that ideas are aligned with RM client services. Several firms have found that involving RMs and other domain experts in squads leads to significant improvements in data interpretation and modeling.
In many cases, assembling productive squads will require new talent. In particular, banks will need data scientists to be responsible for building analytics software and data engineers to scope and build data pipelines and data architecture. Translators, who act as conduits between the business and technology teams, will be critical for ensuring that squads understand business needs. Finally, squads need IT skill sets to ensure that analytics and digital solutions are compatible with core data and technology stacks.
The best approach to talent acquisition is to take baby steps: get one squad right, foster RM adoption, and then gradually expand capabilities as use cases multiply and are scaled up. Some of the required skill sets are in high demand, so outsourcing may be a realistic early option. In the longer term, however, it makes sense to build internally.
Change management. Relationship managers should be encouraged to embrace analytics and convinced that new applications lead to better services and higher levels of performance. Change management strategies can help. Examples include creating teams of “influencers,” running capability-building sessions, developing change narratives that generate widespread excitement, redefining roles, and aligning performance with financial or nonfinancial awards.
Institutionalize new ways of working
Analytics-driven transformation at scale should be predicated on collaboration, team self-steering, and an iterative approach to problem-solving—elements of the so-called agile approach, which originated in software development. In running agile sprints, it pays to keep business needs in sight, accepting that failure is part of the process. Two-week sprints are usually sufficient to get pilots up and running, and the aim should be to produce an MVP with every sprint.
Wealth managers can apply these basic principles via four process disciplines:
- Inspect and adapt. Daily check-ins will ensure that teams identify roadblocks, such as product backlogs, and maintain their focus on goals.
- Engage end users. Sprint reviews with end users, stakeholders, and sponsors enable teams to gather feedback and bake in recommendations.
- Embed a sense of unity and purpose. Teams should hold retrospectives to incorporate learnings.
- Institutionalize support infrastructure. Agile tooling (for example, Confluence, Jira, and Zeplin) will facilitate experimentation and support remote working where necessary.
Organizations using agile operating models must embrace flexible learning. This is a departure from traditional waterfall-based approaches, in which decision-making occurs at the beginning of each project. In agile, capability building and a relentless focus on change management will be vital elements of optimizing the program. To cement the relationship between innovation and growth, leading firms also assign KPIs to application rollouts, and they reward decision makers based on the value created.
Most wealth managers would say they have already embarked on an analytics journey; many have begun deploying digital applications in various aspects of their businesses. Often, however, the whole system is less than the sum of its parts, and people remain attached to established ways of working. To make a leap forward, wealth managers should commit to bold agendas that will support the scaling up of analytics-driven approaches.
Anutosh Banerjee is a partner in McKinsey’s Singapore office, where Vishal Kaushik and Aditya Saxena are associate partners; Fumiaki Katsuki is a partner in the Hanoi office; and Sanchit Suneja is an associate partner in the Mumbai office, where Renny Thomas is a senior partner.
The authors wish to thank Tiffany Kwok and Charu Singhal for their contributions to this article.
Explore a career with us
Related articles.

Building the AI bank of the future
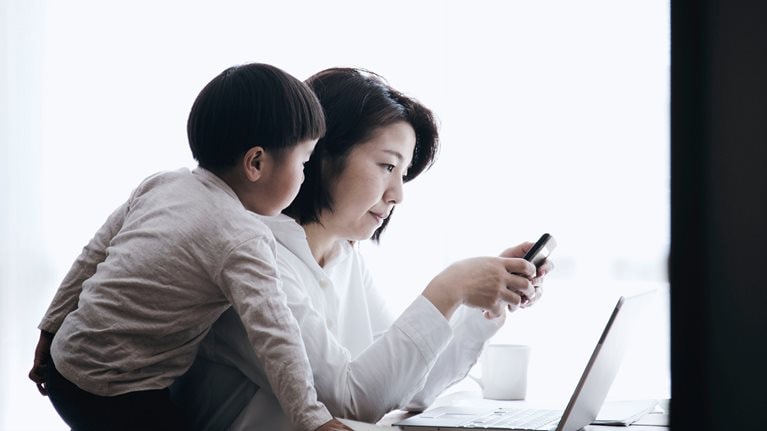
Asia wealth management post-COVID-19: Adapting and thriving in an uncertain world

Clinical Research Coordinator 2 -Neurosurgery (Hybrid)
🔍 school of medicine, stanford, california, united states.
Cancer Clinical Research Coordinator 2 – Neurosurgery (Hybrid)
The Stanford Cancer Institute (SCI) is one of an elite number of National Cancer Institute-Designated Comprehensive Cancer Centers in the country, and is a prominent, dynamic, and complex Institute within the Stanford University School of Medicine. The SCI actively works to build synergies and collaborations among faculty with cancer-relevant expertise from four Schools and over 30 departments across Stanford University. We seek a Clinical Research Coordinator 2 – Neurosurgery to help us enact our mission to reduce cancer mortality through comprehensive programs of cancer research, treatment, education, and outreach. Given the SCI’s mission, breadth, and depth, it employs over 320 staff members in a fast-paced, team-oriented, and forward-thinking environment with tremendous opportunities for personal and professional growth. The Stanford Cancer Institute’s Clinical Trials Office (SCI-CTO) enables our adult and pediatric cancer centers to translate research from the laboratory into the clinical setting and is integral to the mission of the Stanford Cancer Institute. You will be working with an unparalleled leading-edge community of faculty and staff who are fundamentally changing the world of health care in the cancer arena.
Reporting to the Neurosurgery Clinical Research Manager, the Clinical Research Coordinator 2 – Neurosurgery will be conversant in the goals, mission, and priorities of the Institute, and utilize this knowledge to oversee complex clinical research trials. We are seeking candidates with excellent people and time management skills. Our staff run toward challenges, and you will have a demonstrated history of doing the same with a high degree of professionalism, initiative, and flexibility. Responsibilities include trial oversight and ensuring accrual targets are met.
Duties include*:
- Oversee subject recruitment and study enrollment goals. Determine effective strategies for promoting/recruiting research participants and retaining participants in long-term clinical trials.
- Oversee data management for research projects. Develop and manage systems to organize, collect, report, and monitor data collection. Extract, analyze, and interpret data.
- Develop project schedules, targets, measurements, and accountabilities, as assigned. Lead team meetings and prepare/approve minutes.
- Formally supervise, train, and/or mentor new staff or students, as assigned, potentially including hiring, preparing, or assisting with the preparation of performance evaluations, and performing related duties, in addition to instruction on project work.
- Audit operations, including laboratory procedures, to ensure compliance with applicable regulations; provide leadership in identifying and implementing corrective actions/processes. Monitor Institutional Review Board submissions and respond to requests and questions.
- Collaborate with principal investigators and study sponsors, monitor, and report serious adverse events, and resolve study queries.
- Provide leadership in determining, recommending, and implementing improvements to policies/processes; define best practices.
- Develop study budget with staff and principal investigator, identifying standard of care versus study procedures. Track patient and study specific milestones, and invoice sponsors according to study contract.
- Ensure regulatory compliance. Regularly inspect study document to ensure ongoing regulatory compliance.
- Work with principal investigator to ensure Investigational New Drug applications are submitted to the FDA when applicable. Ensure Institutional Review Board renewals are completed.
*- Other duties may also be assigned.
DESIRED QUALIFICATIONS:
- Oncology clinical trial coordination experience.
- Investigator Initiated clinical trial coordination experience.
EDUCATION & EXPERIENCE (REQUIRED):
Bachelor's degree in a related field and two years of experience in clinical research, or an equivalent combination of education and relevant experience.
KNOWLEDGE, SKILLS, AND ABILITIES (REQUIRED):
- Strong interpersonal skills.
- Proficiency with Microsoft Office and database applications.
- Experience with research protocols and regulatory or governing bodies, which include HIPAA and FDA regulations, Institutional Review Board requirements, and Good Clinical Practices.
- Knowledge of medical terminology.
CERTIFICATIONS & LICENSES:
Society of Clinical Research Associates or Association of Clinical Research Professionals certification is preferred. May require a valid California Driver’s License.
PHYSICAL REQUIREMENTS*:
- Frequently stand, walk, twist, bend, stoop, squat and use fine light/fine grasping.
- Occasionally sit, reach above shoulders, perform desk-based computer tasks, use a telephone and write by hand, lift, carry, push, and pull objects that weigh up to 40 pounds.
- Rarely kneel, crawl, climb ladders, grasp forcefully, sort and file paperwork or parts, rarely lift, carry, push, and pull objects that weigh 40 pounds or more.
* - Consistent with its obligations under the law, the University will provide reasonable accommodation to any employee with a disability who requires accommodation to perform the essential functions of his or her job.
WORKING CONDITIONS:
- Position may at times require the employee to work with or be in areas where hazardous materials and/or exposure to chemicals, blood, body fluid or tissues and risk of exposure to contagious diseases and infections.
- May require extended or unusual work hours based on research requirements and business needs.
WORK STANDARDS:
- Interpersonal Skills: Demonstrates the ability to work well with Stanford colleagues and clients and with external organizations.
- Promote Culture of Safety: Demonstrates commitment to personal responsibility and value for safety; communicates safety concerns; uses and promotes safe behaviors based on training and lessons learned.
- Subject to and expected to comply with all applicable University policies and procedures, including but not limited to the personnel policies and other policies found in the University's Administrative Guide, http://adminguide.stanford.edu .
The expected pay range for this position is $69,100 to $92,000 per annum.
Stanford University provides pay ranges representing its good faith estimate of what the university reasonably expects to pay for a position. The pay offered to a selected candidate will be determined based on factors such as (but not limited to) the scope and responsibilities of the position, the qualifications of the selected candidate, departmental budget availability, internal equity, geographic location and external market pay for comparable jobs.
At Stanford University, base pay represents only one aspect of the comprehensive rewards package. The Cardinal at Work website ( https://cardinalatwork.stanford.edu/benefits-rewards ) provides detailed information on Stanford’s extensive range of benefits and rewards offered to employees. Specifics about the rewards package for this position may be discussed during the hiring process.
Consistent with its obligations under the law, the University will provide reasonable accommodations to applicants and employees with disabilities. Applicants requiring a reasonable accommodation for any part of the application or hiring process should contact Stanford University Human Resources by submitting a contact form .
Stanford is an equal employment opportunity and affirmative action employer. All qualified applicants will receive consideration for employment without regard to race, color, religion, sex, sexual orientation, gender identity, national origin, disability, protected veteran status, or any other characteristic protected by law.
The job duties listed are typical examples of work performed by positions in this job classification and are not designed to contain or be interpreted as a comprehensive inventory of all duties, tasks, and responsibilities. Specific duties and responsibilities may vary depending on department or program needs without changing the general nature and scope of the job or level of responsibility. Employees may also perform other duties as assigned.
- Schedule: Full-time
- Job Code: 4923
- Employee Status: Regular
- Requisition ID: 103160
- Work Arrangement : Hybrid Eligible
My Submissions
Track your opportunities.
Similar Listings
School of Medicine, Stanford, California, United States
📁 Research
Post Date: 2 days ago
Post Date: Jan 29, 2024
Post Date: Feb 15, 2023
Global Impact We believe in having a global impact
Climate and sustainability.
Stanford's deep commitment to sustainability practices has earned us a Platinum rating and inspired a new school aimed at tackling climate change.
Medical Innovations
Stanford's Innovative Medicines Accelerator is currently focused entirely on helping faculty generate and test new medicines that can slow the spread of COVID-19.
From Google and PayPal to Netflix and Snapchat, Stanford has housed some of the most celebrated innovations in Silicon Valley.
Advancing Education
Through rigorous research, model training programs and partnerships with educators worldwide, Stanford is pursuing equitable, accessible and effective learning for all.
Working Here We believe you matter as much as the work
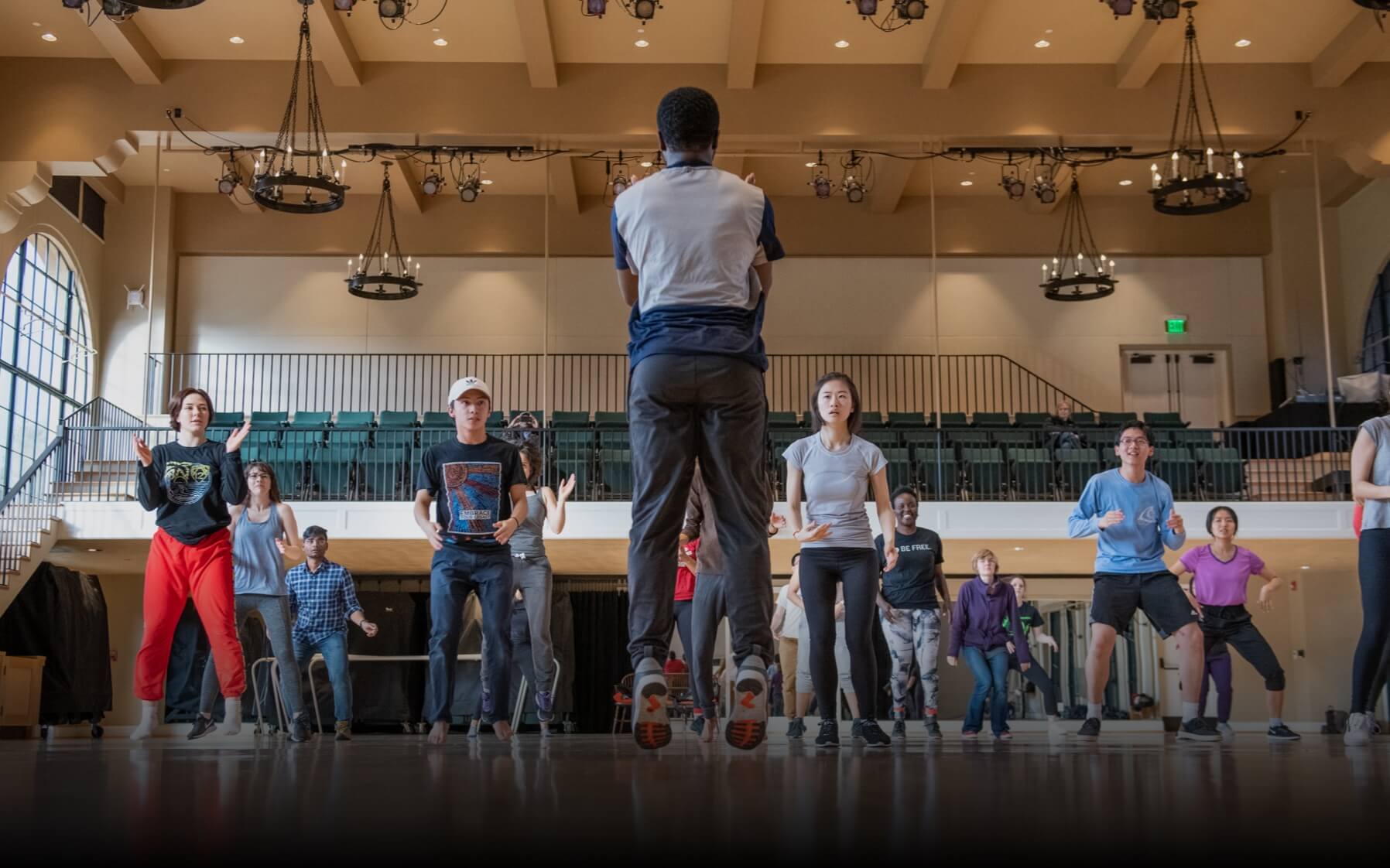
I love that Stanford is supportive of learning, and as an education institution, that pursuit of knowledge extends to staff members through professional development, wellness, financial planning and staff affinity groups.
School of Engineering
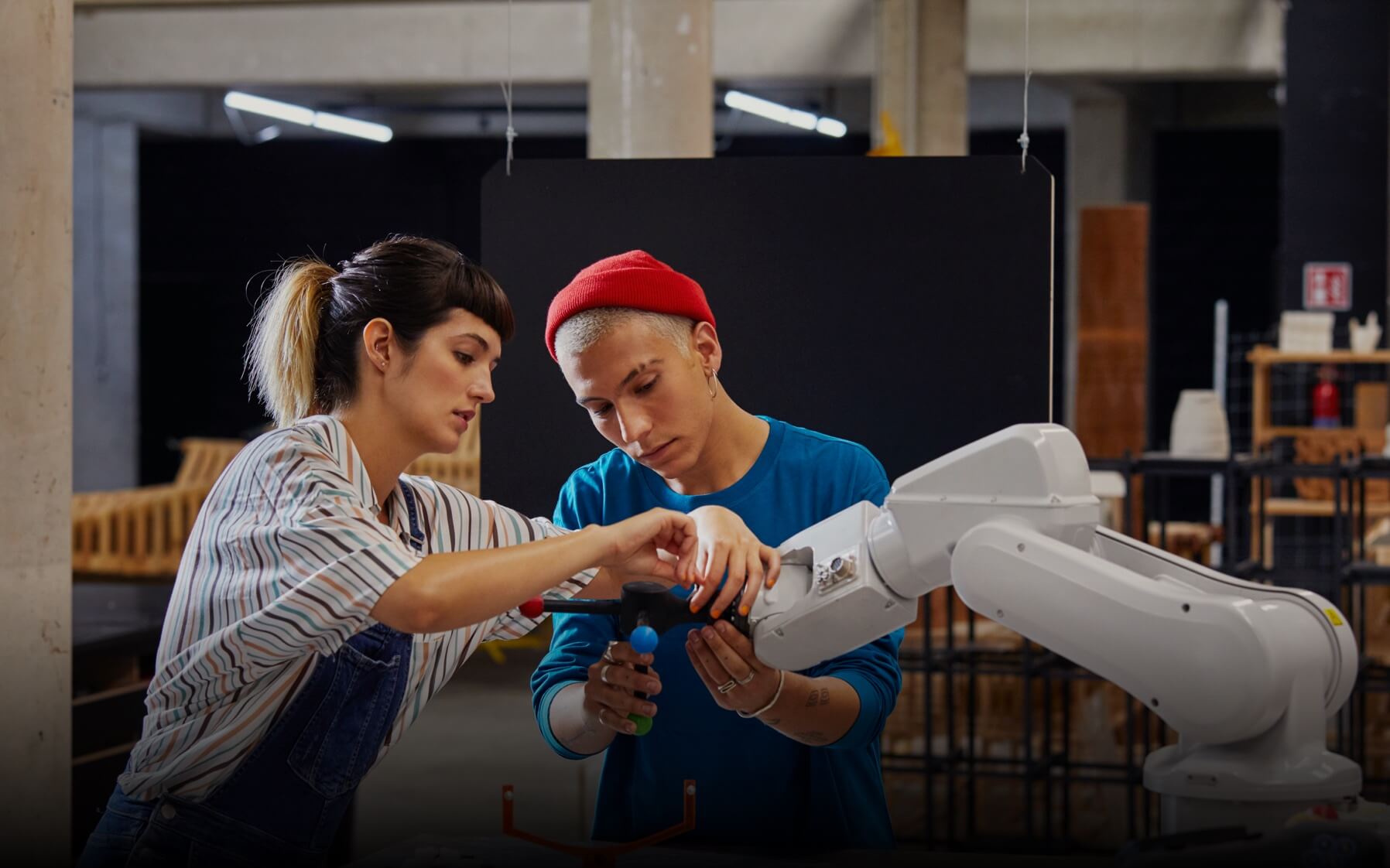
I get to apply my real-world experiences in a setting that welcomes diversity in thinking and offers support in applying new methods. In my short time at Stanford, I've been able to streamline processes that provide better and faster information to our students.
Phillip Cheng
Office of the Vice Provost for Student Affairs
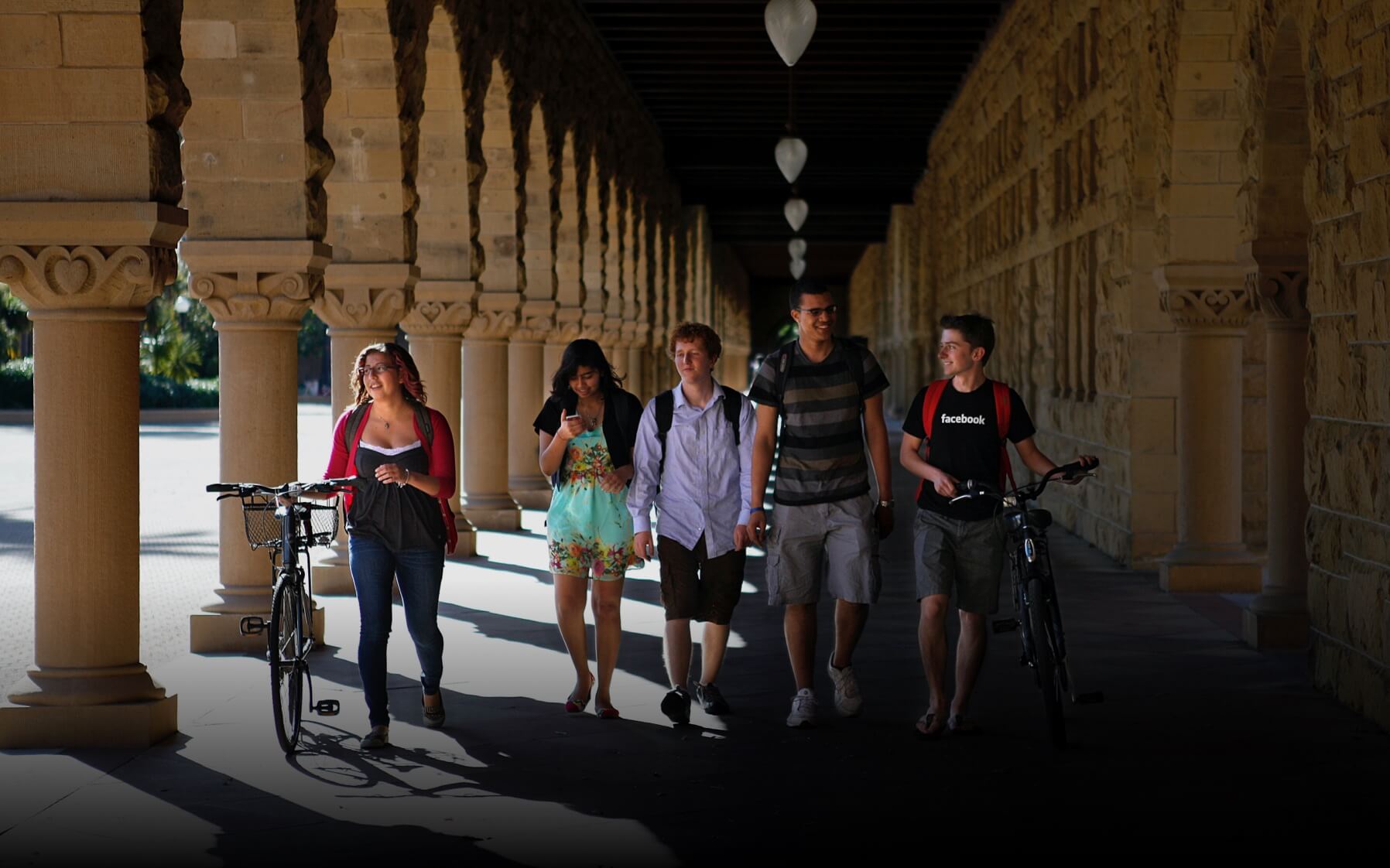
Besides its contributions to science, health, and medicine, Stanford is also the home of pioneers across disciplines. Joining Stanford has been a great way to contribute to our society by supporting emerging leaders.
Denisha Clark
School of Medicine
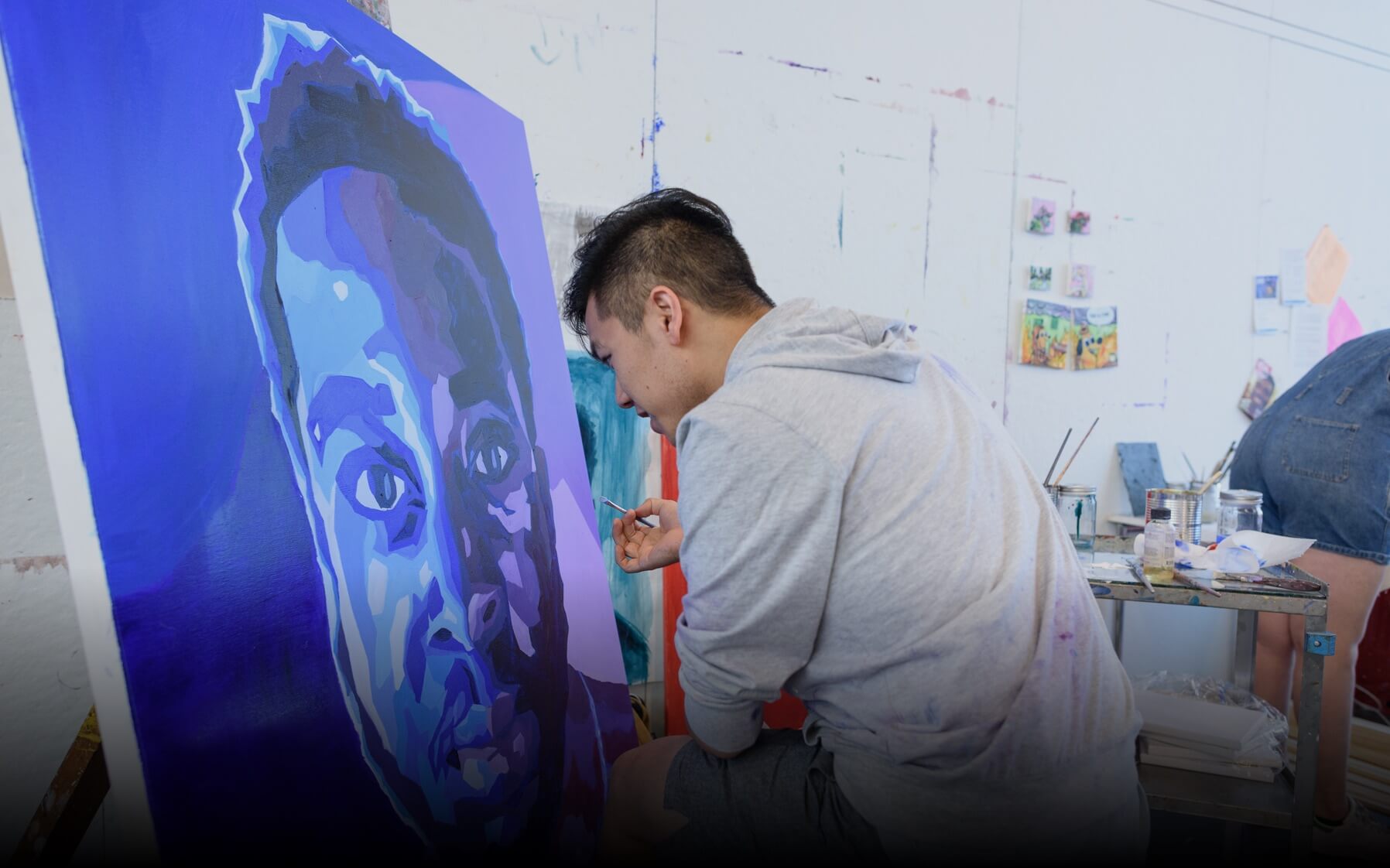
I like working in a place where ideas matter. Working at Stanford means being part of a vibrant, international culture in addition to getting to do meaningful work.
Office of the President and Provost
Getting Started We believe that you can love your job
Join Stanford in shaping a better tomorrow for your community, humanity and the planet we call home.
- 4.2 Review Ratings
- 81% Recommend to a Friend
View All Jobs
We couldn’t find any results matching your search.
Please try using other words for your search or explore other sections of the website for relevant information.
We’re sorry, we are currently experiencing some issues, please try again later.
Our team is working diligently to resolve the issue. Thank you for your patience and understanding.
News & Insights

‘Jump on the Bandwagon,’ Says Bank of America About These 2 Stock Picks

May 08, 2024 — 08:56 pm EDT
Written by Michael Marcus for TipRanks ->

This year started with a bang, saw a fizzle in April – but now appears to be regaining its stride. As we saw last year, the gains continue to be dominated by the ‘Magnificent 7’ tech stocks. This group of mega-cap companies collectively experienced a 48% year-over-year earnings increase in the recent first-quarter 2024 releases, in contrast to a 2% collective decline across the other S&P companies.
Going forward, however, expectations are that the remaining 493 companies on the S&P will close that gap, and Bank of America strategist Ritesh Samadhiya sets out a case for the Mag 7 stocks to realize a 15% earnings share gain in Q4 while the rest of the index increases its share to 14%.
That forecast implies a hefty broadening of the base, opening more opportunities for investors. The combination of a broader base and the current improved investor sentiment has Samadhiya’s team feeling bullish – and their colleagues among the Bank of America stock analysts are running with it, telling investors that it’s time to take advantage of the potential for wider gains and to jump on the bandwagon for two stocks in particular.
A look into the TipRanks data shows that these 2 stock picks from BofA offer widely divergent potentialities – but Bank of America’s analysts are predicting solid upsides for both. Here are the details.
Cisco Systems ( CSCO )
Cisco Systems is a well-known name in the world of networking technology, with a wide and varied set of product lines on the market. The company’s product offerings include switches, routers, cloud and network management, interfaces and modules, outdoor and industrial wireless access points, wireless controllers, firewalls, and secure endpoints. The list is long and includes product lines for data centers, data analytics, video, IoT, and software. There are few areas involved in online networking and security that Cisco isn’t involved in.
In a move designed to bolster its strong position in software and data analytics, Cisco acquired the analytic software company Splunk in a transaction worth approximately $28 billion. The deal brings Splunk’s capabilities in data searching, monitoring, and analysis into Cisco’s stable of product offerings, making Cisco one of the world’s largest software companies. New features for Cisco, derived from the acquisition, include security and observability solutions. The transaction was completed on March 18.
Cisco has multiple opportunities on tap for the near future, including the movement of AI systems to Ethernet networks. This will bring the ‘shiny new thing,’ gen AI, directly into one of Cisco’s core competencies. In addition, Cisco’s strength in optical networks will open up opportunities for the company to move into the hyperscaler ecosystem.
Despite Cisco’s solid reputation and strong market position, the company’s stock has underperformed in recent months. The company reported its fiscal 2Q24 results in February, and while the financial results surpassed the forecasts, the company’s guidance failed to impress.
But the Q2 results do bear a closer look. The company brought in $12.8 billion in revenue, down 6% year-over-year but $100 million better than had been expected. At the bottom line, Cisco’s non-GAAP EPS of 87 cents was 3 cents per share over the estimates. Looking forward, management gave fiscal Q324 revenue guidance in the range between $12.1 billion and $12.3 billion – but the consensus had looked for $13.1 billion. The EPS forecast of 84 of 86 cents per share was also lower than the 92 cents analysts had hoped to see.
At least one analyst, however, is not fazed by Cisco’s guidance. Tal Liani, 5-star analyst from Bank of America, believes that the upside potential here – coming from Cisco’s strengths in the AI and Security segments, as well as the Splunk acquisition – simply outweigh the weaknesses.
“We expect Networking to start normalizing and see renewed growth driven by Cisco’s share gains in Ethernet-based AI buildouts of hyperscalers. We expect Security growth to accelerate with the help of firewall stabilization and recent new product launches. Lastly, we see great growth synergies from Splunk’s acquisition. While the next two quarters may remain pressured, we believe this weakness is fully reflected in Street expectations and management guidance is adequately conservative,” Liani opined.
Liani complements his positive stance with a Buy rating on CSCO, and a $60 price target that points toward a 25% upside on the one-year time horizon. (To watch Liani’s track record, click here )
The bullish Bank of America viewpoint here is something of an outlier; this stock has a Hold rating from the analyst consensus, based on 17 recent recommendations that include 4 Buys and 13 Holds. The shares have a $53.67 average price target, suggesting a ~12% one-year gain from the current share price of $48. (See CSCO stock forecast )
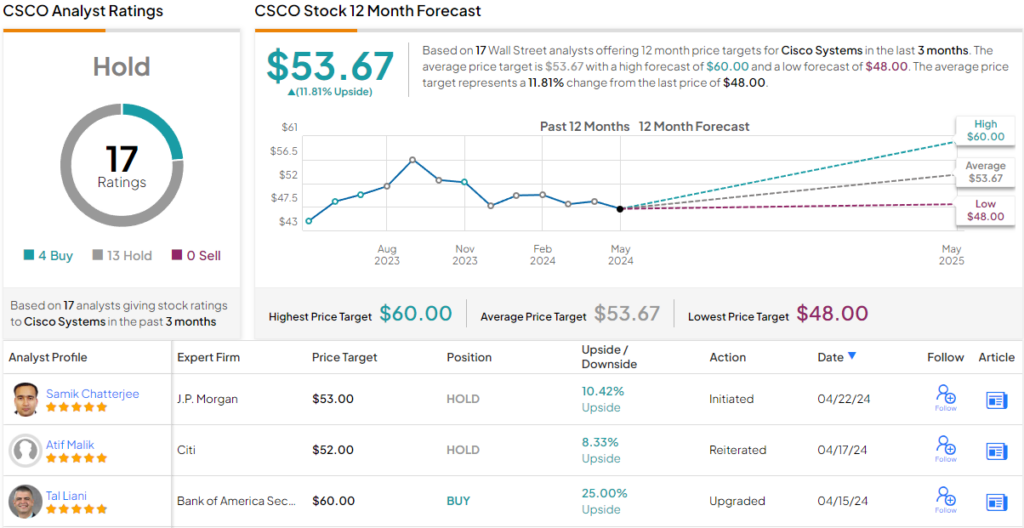
89bio, Inc. ( ETNB )
The second Bank of America pick we’ll look at is 89bio, a research-oriented biopharmaceutical company with a focus on finding, developing, and commercializing new therapeutic agents for the treatment of liver and cardio-metabolic diseases. The company’s research pipeline is organized around its advanced drug candidate BIO89-100, and includes clinical studies in the treatment of NASH (nonalcoholic steatohepatitis, sometimes now referred to as MASH, or metabolic dysfunction-associated steatohepatitis) and SHTG (severe hypertriglyceridemia).
The drug candidate BIO89-100, also known as pegozafermin, was engineered specifically to target the underlying metabolic issues that cause NASH and SHTG. The drug is a glycoPEGylated analog of FGF21, or fibroblast growth factor 21. FGF21 is a liver hormone known to act as a master metabolic regulator with broad effects, particularly on the glucose and lipid metabolism. Pegozafermin was developed to increase the half-life of the FGF21 hormone.
89bio has recently posted updates from three trials, all targeting the NASH indication. In March, the company initiated a Phase 3 trial, dubbed ENLIGHTEN, for non-cirrhotic (F2-F3) and cirrhotic (F4) patients. In addition, the company has also released two data sets from the Phase 2b ENLIVEN trial, in patients with advanced NASH and with cirrhotic NASH. The data sets demonstrated statistically significant improvements in key markers of liver health. On the SHTG side, the company is continuing to enroll patients in the Phase 3 ENTRUST trial, to evaluate the efficacy, safety and tolerability of pegozafermin in the treatment of SHTG. Data from this trial is expected for release in 2025.
The solid research program on pegozafermin and its long-term commercial potential caught the attention of analyst Alexandria Hammond, who writes in her coverage for Bank of America.
“Beyond macro uncertainties, we suspect concerns over competition in the fatty liver disease space (aka MASH) and commercial unknowns ahead of the first-to-market MASH launch have weighed sentiment. We acknowledge the uncertainties of the MASH commercial landscape, but, in our view, 89Bio’s FGF21 analogue pegozafermin offers intriguing upside as a next—and possibly best-in-class— MASH agent given a more favorable safety/tolerability profile (projected launch 2027) in a particularly attractive and less crowded market sub-segment,” Hammond opined.
Hammond adds, laying out a clear case for investors to buy in now, “With pegozafermin well-positioned to compete for the space’s meaningful—and addressable potential—along with the limited downside, we think the current risk/reward is particularly attractive.”
These comments support the analyst’s Buy rating on ETNB, while her $30 price target implies a robust 232% one-year upside potential. (To watch Hammond’s track record, click here )
Overall, this stock is clear winner in Wall Street’s eyes, boasting a Strong Buy consensus rating from the analysts based on 5 recent ‘Buy’ calls against a single ‘Hold.’ The stock is selling for $9.03 and its $29.80 average price target suggests that it will gain 230% over the course of the next 12 months. (See ETNB stock forecast )
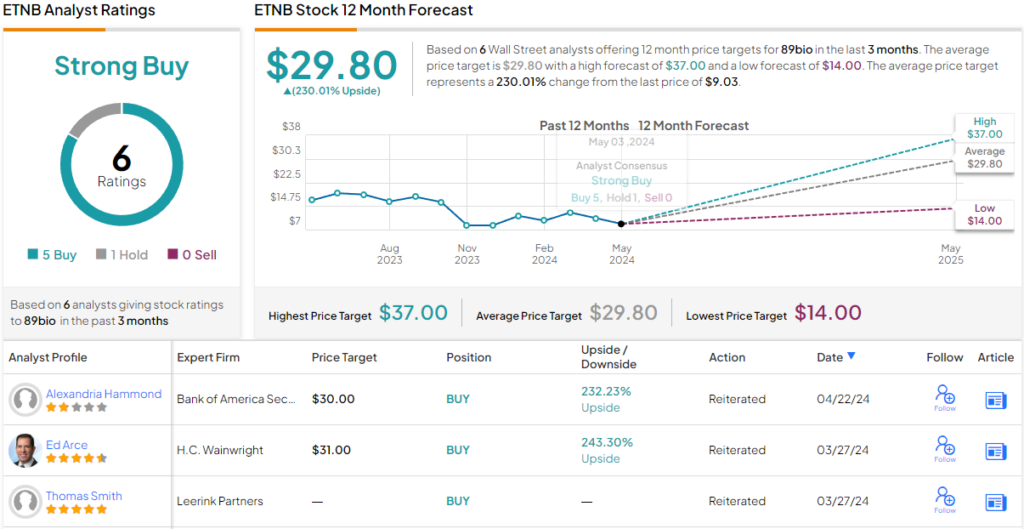
To find good ideas for stocks trading at attractive valuations, visit TipRanks’ Best Stocks to Buy , a tool that unites all of TipRanks’ equity insights.
Disclaimer: The opinions expressed in this article are solely those of the featured analysts. The content is intended to be used for informational purposes only. It is very important to do your own analysis before making any investment.
The views and opinions expressed herein are the views and opinions of the author and do not necessarily reflect those of Nasdaq, Inc.

Stocks mentioned
More related articles.
This data feed is not available at this time.
Sign up for the TradeTalks newsletter to receive your weekly dose of trading news, trends and education. Delivered Wednesdays.
- Type a symbol or company name. When the symbol you want to add appears, add it to My Quotes by selecting it and pressing Enter/Return.
These symbols will be available throughout the site during your session.
Your symbols have been updated
Edit watchlist.
- Type a symbol or company name. When the symbol you want to add appears, add it to Watchlist by selecting it and pressing Enter/Return.
Opt in to Smart Portfolio
Smart Portfolio is supported by our partner TipRanks. By connecting my portfolio to TipRanks Smart Portfolio I agree to their Terms of Use .
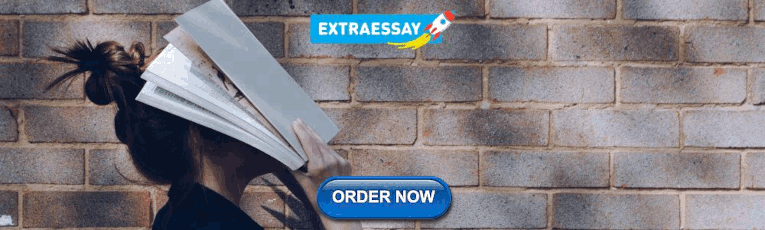
COMMENTS
Time Management Is About More Than Life Hacks. by. Erich C. Dierdorff. January 29, 2020. Maurizio Cigognetti/Getty Images. Summary. There is certainly no shortage of advice — books and blogs ...
A critical gap in time management research is the question of whether time management works [28, 29]. For instance, studies on the relationship between time management and job performance reveal mixed findings [30, 31].
Time management can be defined as clusters of behavioral skills that are important in the organization of study and course load (Lay & Schouwenburg, 1993).Empirical evidence suggests that effective time management is associated with greater academic achievement (McKenzie & Gow, 2004; Trueman & Hartley, 1996) as students learn coping strategies that allow them to negotiate competing demands.
The principles below are derived from research on time management, motivation theory and much experience working with university students. Think of time management techniques as tools to help you do what you value the most. Make these tools into an expression of your values—what's most important to you—not just a schedule to get more stuff done....
Does time management work? We conducted a meta-analysis to assess the impact of time management on performance and well-being. Results show that time management is moderately related to job performance, academic achievement, and wellbeing. Time management also shows a moderate, negative relationship with distress. Interestingly, individual differences and contextual factors have a much weaker ...
The World Management Survey at 18: Lessons and the Way Forward. by Daniela Scur, Raffaella Sadun, John Van Reenen, Renata Lemos, and Nicholas Bloom. With a dataset of 13,000 firms and 4,000 schools and hospitals spanning more than 35 countries, the World Management Survey provides a systematic measure of management practices used in organizations.
Abstract. Purpose - The purpose of this article is to provide an overview for those interested in the current. state-of-the-art in time management research. Design/methodology/approach - This ...
Time management seems to have more con- structures and time norms, two key concepts in the sistent effects on performance defined as behaviors sociology of time often overlooked in time research compared to performance defined as results or out- in the management and psychology literatures. Time comes.
Time management has been defined as "the self-controlled attempt to use time in a subjectively efficient way to achieve outcomes" (Koch and Kleinmann 2002, p. 201) and as "achieving an effective use of time while performing certain goal-directed activities" (Claessens et al. 2007, p. 262).Although there is no consensus on its major components, theorists typically portray time ...
Time management is defined as "a form of decision making used by individuals to struc-ture, protect, and adapt their time to changing conditions" [18]. This means time manage- ... A critical gap in time management research is the question of whether time management works [28, 29]. For instance, studies on the relationship between time ...
Time management has helped people organize their professional lives for centuries. The existing literature, however, reveals mixed findings and lack of clarity as to whether, when, how, and why time management leads to critical outcomes such as well-being and job performance. Furthermore, insights relevant to time management are scattered across various disciplines, including sociology ...
The Basics of Time Management. The key steps for successful time management are as follows: 1) set realistic goals, 2) get organized, 3) delegate, 4) relax and recharge, and 5) stop feeling guilty. There are two major time management stumbling blocks: procrastination and perfectionism.
However, research suggests that study skills (time management) are also significant factors affecting academic achievement in medical schools.[8,21,22,23,24,25] Study skills are one of the more reliable predictors of first-semester total grades. The predictive strength of first-semester final average is accounted for by scores on time management,
The Impact of Time Management on Students' Academic Achievement. S N A M Razali 1, M S Rusiman 1, W S Gan 1 and N Arbin 2. Published under licence by IOP Publishing Ltd Journal of Physics: Conference Series, Volume 995, International Seminar on Mathematics and Physics in Sciences and Technology 2017 (ISMAP 2017) 28-29 October 2017, Hotel Katerina, Malaysia Citation S N A M Razali et al 2018 ...
Due to the clear link between time management and academic performance, researchers have turned their attention toward understanding the specific processes involved in time management (Wolters and Brady, 2021). The purpose of the present study was to investigate one process related to time management: time estimation bias.
In this conceptual article, we review three decades of research on time and meaning in consumer research and psychology to identify key themes that have emerged, build frameworks that integrate past research, and reveal areas of potential for future empirical exploration. We begin by carving out a conceptual understanding of meaning in life and ...
Task Managers - Prioritize and organize tasks to improve time management. Habit Developers - Create healthy habits to encourage time management. 4. Get Organized. Disorganization leads to poor time management. Research has shown that clutter has a strong negative impact on perceived well-being (Roster, 2016).
Time management is a. valuable area, this has been highlighted with the help of a case study of a college student who is. undergoing four classes; college graduate course, MBA entrance coaching ...
Time mana gement pla ys a vital role in improving studen t's academic perfor mance and achievements. Each and. every student should have time management ability which includes setting goals ...
Time management is regarded as an important prerequisite for effective and efficient learning in higher education. However, university students' time management frequently proves to be deficient, especially with freshman students, who can therefore benefit from appropriate time management interventions. The aim of this study was to compare the effects of an intervention focused on imparting ...
Time management for surgeons can be broken down into two major phases: the daily schedule and our overall career goals. Thankfully, being efficient on a daily basis can have profound implications on your overall productivity and satisfaction, even beyond 1 day. Prioritize your daily goals and create a list.
The definition of time management has been offered as "the process of skilfully applying time to finish and perfect a specific activity within time constraint" (Harris, 2008, p.22). However, there is no single definition of time that fully captures all aspects of the concept. Becker and Mustric (2008) divide time into two categories ...
First, research requires a significant amount of time, and effective time management ensures that this time is used efficiently. Poor time management can result in missed deadlines, incomplete projects, and wasted resources. Second, time management can help researchers achieve a healthy work-life balance, which is essential for maintaining well ...
Mastering Time Management ; Accessing Academic Resources ; Reflecting on Your Progress ; Celebrating Achievements ; Self-Care ; Overcoming Test Anxiety; Faculty Feedback; Time Management; Reading Comprehension; Note Taking; Support Services
Good time management skills are a must. ... Our Research Expert. Natasha Etzel. Natasha is a freelance writer who specializes in personal finance, credit card, credit card rewards, and travel ...
Working time around the world. Flexible working hours can benefit work-life balance, businesses and productivity. According to a new ILO report, innovative working time arrangements, such as those introduced during the COVID-19 crisis, can bring benefits for economies, businesses and workers, including greater productivity and improved work-life balance.
Exercising this much may help with weight loss or keeping off lost weight. But even small amounts of physical activity can be helpful. Being active for short periods of time during the day can add up and give you great health benefits. Strength training. Do strength training exercises for all major muscle groups at least two times a week.
Focus on relationship management. Modernization can be game changing when it targets the role of RMs. Based on conversations with industry participants, we estimate that RMs typically spend 60 to 70 percent of their time on non-revenue-generating activities, amid rising regulatory and compliance obligations (Exhibit 1).
Reporting to the Neurosurgery Clinical Research Manager, the Clinical Research Coordinator 2 - Neurosurgery will be conversant in the goals, mission, and priorities of the Institute, and utilize this knowledge to oversee complex clinical research trials. We are seeking candidates with excellent people and time management skills.
Looking forward, management gave fiscal Q324 revenue guidance in the range between $12.1 billion and $12.3 billion - but the consensus had looked for $13.1 billion.