
An official website of the United States government
The .gov means it’s official. Federal government websites often end in .gov or .mil. Before sharing sensitive information, make sure you’re on a federal government site.
The site is secure. The https:// ensures that you are connecting to the official website and that any information you provide is encrypted and transmitted securely.
- Publications
- Account settings
Preview improvements coming to the PMC website in October 2024. Learn More or Try it out now .
- Advanced Search
- Journal List
- HHS Author Manuscripts

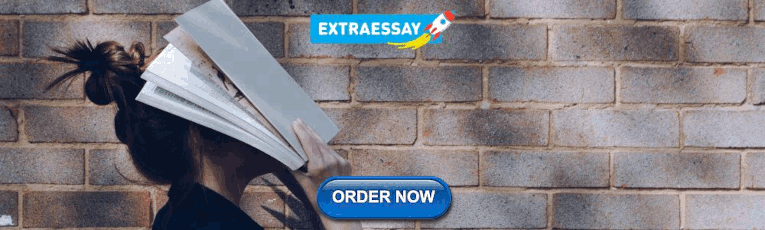
High school dropouts: Interactions between social context, self-perceptions, school engagement, and student dropout ☆
Research suggests that contextual, self-system, and school engagement variables influence dropping out from school. However, it is not clear how different types of contextual and self-system variables interact to affect students’ engagement or contribute to decisions to dropout from high school. The self-system model of motivational development represents a promising theory for understanding this complex phenomenon. The self-system model acknowledges the interactive and iterative roles of social context, self-perceptions, school engagement, and academic achievement as antecedents to the decision to dropout of school. We analyzed data from the Education Longitudinal Study of 2002–2004 in the context of the self-system model, finding that perception of social context (teacher support and parent support) predicts students’ self-perceptions (perception of control and identification with school), which in turn predict students’ academic and behavioral engagement, and academic achievement. Further, students’ academic and behavioral engagement and achievement in 10th grade were associated with decreased likelihood of dropping out of school in 12th grade.
Almost one-third of all public secondary students in the United States each year dropout of school ( Snyder & Dillow, 2010 ; Stillwell, 2010 ). Dropout rates vary across groups and settings, with Hispanic (36.5%) and African American (38.5%) students dropping out at higher rates than Asian (8.6%) and White (19%) students ( Stillwell, 2010 ). High rates of dropout affect individuals, families, and communities ( Dynarski, Gleason, Rangarajan, & Wood, 1998 ; Orfield, 2006 ). Nongraduates are more likely to be unemployed ( Sum, Khatiwada, McLaughlin, & Palma, 2009 ), to earn less when employed ( Levin, Belfield, Muennig, & Rouse, 2007 ), to receive public assistance ( Waldfogel, Garfinkel, & Kelly, 2007 ), to suffer poor health ( Muennig, 2007 ), and to have higher rates of criminal behavior and incarceration ( Moretti, 2007 ). Additionally, children of parents who did not complete high school are more likely to perform poorly in school and eventually dropout, creating an intergenerational dynamic ( Orfield, 2006 ).
Considerable research has addressed factors associated with dropping out of school. Early attempts to identify risk focused on student factors associated with an elevated likelihood of leaving school prior to graduating. This research consistently reports that students from poor or single-parent households, or whose parents did not graduate from high school, are at greater risk of dropping out from school than students from families without these risk factors ( Alexander, Entwisle, & Horsey, 1997 ; Goldschmidt & Wang, 1999 ; Rumberger, 1995 ; Rumberger & Larson, 1998 ; Swanson & Schneider, 1999 ). The earlier research also suggests that students with adult responsibilities ( Cairns, Cairns, & Neckerman, 1989 ; Gleason & Dynarski, 2002 ; Goldschmidt & Wang, 1999 ; Neild & Balfanz, 2006 ), with a sibling who has dropped out ( Teachman, Paasch, & Carver, 1996 ), who have been retained ( Goldschmidt & Wang, 1999 ; Roderick, 1994 ; Roderick, Nagaoka, Bacon, & Easton, 2000 ; Rumberger, 1995 ; Rumberger & Larson, 1998 ), or who have changed schools ( Astone & McLanahan, 1994 ; Rumberger, 1995 ; Rumberger & Larson, 1998 ; Swanson & Schneider, 1999 ) are more likely to dropout of school.
Although this early work centered on person-level characteristics that tend not to be amenable to change, more recent research addresses dynamic factors related to risk status and has led to a growing interest in the construct of engagement ( Appleton, Christenson, Kim, & Reschly, 2006 ; Fredricks, Blumenfeld, & Paris, 2004 ; Sinclair, Christenson, Lehr, & Anderson, 2003 ). School engagement is considered the primary model for understanding and predicting graduation from high school. Conceptualizations of school engagement vary in their details ( Appleton, Christenson, & Furlong, 2008 ; Finn, 1989 ; Fredricks et al., 2004 ; Jimerson, Campos, & Greif, 2003 ). However, they share a premise: that poor school engagement hinders academic achievement ( Caraway, Tucker, Reinke, & Hall, 2003 ; DiPerna, Volpe, & Elliott, 2005 ; Finn & Rock, 1997 ; Wu, Hughes, & Kwok, 2010 ), which, over time, increases the likelihood that students will dropout of school ( Alexander et al., 1997 ; Sinclair et al., 2003 ).
Theories of school dropout ( Appleton et al., 2008 ; Fredricks et al., 2004 ; Rumberger, 2006 ) and a growing body of research also suggests that contextual ( Dotterer & Lowe, 2011 ; Hong & Ho, 2005 ; Patrick, Ryan, & Kaplan, 2007 ; Ryan & Patrick, 2001 ; Wang & Holcombe, 2010 ; You & Sharkey, 2009 ) and self-system ( Caraway et al., 2003 ; Furrer & Skinner, 2003 ; You & Sharkey, 2009 ) variables influence school engagement and dropping out from school. However, it is not clear how aspects of social context influence multiple forms of engagement simultaneously or how different types of contextual and self-system variables interact to affect students’ engagement and lead to decisions to dropout from high school ( Fredricks et al., 2004 ). The self-system model of motivational development (SSMMD) integrates contextual and self-system variables and provides a framework for describing processes that initiate and sustain a decline in student engagement ( Connell & Wellborn, 1991 ; Skinner, Furrer, Marchand, & Kindermann, 2008 ; Skinner, Kindermann, Connell, & Wellborn, 2009 ; Skinner & Wellborn, 1994 ). Using the SSMMD, the central objective of the present study is to empirically test the mechanism involved in the dropout process.
Self-system model of motivational development
SSMMD posits that individuals possess an innate need to connect with others and interact effectively with their environment. It also asserts that the relationship of a given social context (e.g., family support, teacher support, peer support) and an individual’s self-system processes (e.g., perceived identification with school, perceived control) is influenced by the extent to which the social context meets or ignores (fulfills or neglects) these basic needs. Further, self-system profiles differentially influence engagement-related behaviors, which directly contribute to educational outcomes such as student achievement and dropping out. That is, SSMMD suggests that 1) self-systems mediate the relation between a social context and school engagement and that 2) engagement mediates the relation between self-system processes and student outcomes. This model is shown in Fig. 1 .

Self-system model of motivational development applied to dropping out of high school. Dotted lines represent significant indirect effects, and solid lines indicate significant direct effects. Adapted from Connell and Wellborn (1991) ; Skinner et al. (2008) ; and Skinner et al. (2009) .
Studies have provided empirical support for SSMMD, as applied to academic achievement ( Connell, Spencer, &Aber, 1994 ; Skinner et al., 2008 ; Wang & Holcombe, 2010 ). For instance, Connell et al. (1994) conducted path analyses among a sample of 10- to 16-year-old African American youth. Nearly all proposed relations based on SSMMD were significant. In particular, students’ perception of parental involvement predicted self-system processes (a composite measure of perceived competence, perceived relatedness to self, and perceived relatedness to others), which in turn predicted students’ emotional and behavioral engagement. Engagement predicted educational outcomes (a composite measure reflecting the degree of risk for school departure based on attendance, test scores, grade-point average, suspension, and retention). Skinner et al. (2008) found that teacher support and students’ self-system processes (perceived control, autonomy orientation, and sense of relatedness) were significant predictors of behavioral and emotional engagement. Moreover, self-system processes mediated the association between teacher support and student engagement. More recently, Wang and Holcombe (2010) examined the relationships among middle school students’ perception of school environment, engagement, and achievement. Structural equation modeling revealed that students’ perception of school environment in seventh grade (performance goal structure, mastery goal structure, support of autonomy, promotion of discussion, and teacher social support) affected their school engagement (behavioral, emotional, and cognitive engagement) and, in turn, influenced students’ academic achievement in eighth grade.
In contrast, empirical support for SSMMD, as applied to dropping out from high school, is limited. Available evidence, however, suggests that SSMMD can provide an organizing framework for better understanding the role of contextual, self-system, and engagement variables in dropping out from high school. To illustrate, Connell, Halpern-Felsher, Clifford, Cri-chlow, and Usinger (1995) used SSMMD to examine behavioral, psychological, and contextual predictors of staying in high school among a sample of African American adolescents. The authors found that higher levels of support from teachers and adults at home were associated with higher levels of perceived competence, perceived relatedness, and perceived autonomy. These self-perceptions then predicted students’ level of school engagement. School engagement positively predicted males’ staying in school. Among females, the association between engagement and staying in school was not significant. These findings, while promising, are subject to important limitations, notably the unidimensional conceptualization of the engagement construct and the relatively homogenous sample (African American adolescents from an urban school district). There is a need for replication with other populations. There may also be value in applying more nuanced conceptualizations of the engagement construct, including multidimensional models.
Researchers have tended to study the impact of either teacher or parent support on self-system and engagement ( Hong & Ho, 2005 ; Patrick et al., 2007 ; Ryan & Patrick, 2001 ; You & Sharkey, 2009 ). Past research has linked teacher support to student self-system and engagement. Support from teachers enhanced students’ focus on mastery of goals ( Patrick et al., 2007 ), feeling of academic efficacy ( Patrick et al., 2007 ; Ryan & Patrick, 2001 ), and self-regulated learning ( Ryan & Patrick, 2001 ), which in turn facilitated students’ cognitive and behavioral engagement ( Patrick et al., 2007 ; Ryan & Patrick, 2001 ). Although support from teachers is important for student learning and development, support from parents is also related to student self-perception and engagement. Parent support promotes students’ perception of control and perception of self, which in turn promote engagement and benefit student learning ( Hong & Ho, 2005 ; You & Sharkey, 2009 ). However, few studies have examined the impact of both supports in a single study. As a consequence, little has been learned about how parent and teacher support influence and differentially predict students’ self-system and engagement.
Finally, the relationship of engagement and dropping out is understood primarily in terms of student behavior. For example, Finn and Rock (1997) found that behavioral engagement significantly differentiated unsuccessful school completers, successful school completers, and school dropouts among 1803 minority students from low-income backgrounds. Rumberger (1995) , using data from the National Education Longitudinal Study of 1988, found that moderate to high absenteeism, behavior problems, and having no school or outside activities were highly predictive of dropping out. More recently, Ream and Rumberger (2008) investigated the effect of behavioral engagement on school completion and dropout among Mexican American and non-Latino White students, finding that engagement directly influenced high school graduation. Archambault, Janosz, Fallu, and Pagani (2009) used a three-part engagement construct encompassing behavioral, affective, and cognitive dimensions to successfully predict dropout. Although the global measures of engagement predicted school dropout, behavioral engagement was the only unique factor with statistically significant predictive value. In contrast, few studies have examined academic engagement as it relates to dropping out from high school.
Purposes of the present study
The present study addresses limitations in the research on engagement and dropping out where SSMMD provides the theoretical framework. We assess how indicators of social context (e.g., teacher and parent support), self-systems (e.g., perception of control, identification with school), and engagement (e.g., behavioral and academic engagement) relate to academic achievement and dropping out of high school. Fig. 1 depicts the proposed model, which comprises five parts. We hypothesized that higher levels of support from teachers and parents would positively influence students’ perception of self, that positive self-perceptions would positively influence students’ behavioral and academic engagement and academic achievement, and that high levels of behavioral and academic engagement and achievement would decrease the likelihood of dropping out of high school. We further anticipated that self-perceptions would mediate the relations between teacher and parent support and academic and behavioral engagement and that academic and behavioral engagement would mediate the relationship between the two self-perceptions and dropping out of high school.
Participants
Participants in this study were part of ELS: 2002–2004, designed by the National Center for Education Statistics to provide trend data about the experiences of a cohort of high school 10th-graders as they proceeded through high school and into postsecondary education or their careers ( Ingels, Pratt, Rogers, Siegel, & Stutts, 2004 ). The base-year study was carried out in a national probability sample of 752 public, Catholic, and private schools in the spring of the 2001–2002 academic year. In total, 15,362 students completed the base-year questionnaire, as did 13,488 parents, 14,081 teachers, 743 principals, and 718 librarians ( Ingels et al., 2007 ). The first-follow-up survey occurred in 2004, when most sample members were high school seniors—others had dropped out or completed high school early. The second follow-up occurred in 2006, when many sample members were in college for up to their second year of enrollment and others were employed. One additional follow-up is planned for 2012 to document later outcomes, including persistence in higher education or transition into the job market ( Ingels et al., 2007 ). For detailed information about ELS: 2002–2004, please see http://nces.ed.gov/surveys/els2002 .
We used the sample of 14,781 base-year students who participated in the first wave of the study and who were resurveyed in 2004 and identified as either still enrolled in school ( n = 13,995) or dropped out ( n = 786). Of this sample, 49.4% ( n = 7309) were male and 50.6% ( n = 7472) were female. Approximately 57% ( n = 8459) of the participants were White, 14.4% Hispanic ( n = 2126), 13.3% African American ( n = 1962), 9.5% Asian ( n = 1401), and 5.6% ( n = 833) American Indian or of mixed race. Table 1 describes in further detail the demographic characteristics of the sample by dropout status. To generate national population estimates for our analyses, we used the base-year/first-follow-up panel weight.
Demographic characteristics of the sample by dropout status.
Note. N = 14,781.
We drew all data for this study, except dropout status, from the base-year survey, when students were in 10th grade. For dropout status, we used data from the second wave, when most of the students were in 12th grade.
Parent support in 10th grade
Six items from the student questionnaire measured parent support, capturing the frequencies of parent and school communications concerning students’ school problems. On a 3-point scale (never, sometimes, and often), students reported the frequency with which they and their parents spoke about school in general, school-related activities, topics studied in class, and issues that troubled them. The following is a sample item: “In the first semester or term of this school year, how often have you discussed things you’ve studied in class with either or both of your parents or guardians?” Higher scores reflected greater parent support. The construct reliability ( Hancock & Mueller, 2001 ) of this latent variable was .83.
Teacher support in 10th grade
This latent construct represents students’ perceptions of the level of care and support from teachers. The construct included five items, and responses ranged from 1 (strongly agree) to 4 (strongly disagree). The following is a sample item: “In class, I often feel ‘put down’ by my teachers.” Items were coded, so that higher scores represented greater teacher support. The construct reliability was .74.
Perceived control in 10th grade
Perceived control included 4 items from the student questionnaire that assessed the extent to which students believed they were able to produce positive, and prevent negative, outcomes in school. Responses were rated on a 4-point scale (almost never, sometimes, often, and almost always). The following is a sample item: “When I sit myself down to learn something really hard, I can learn it.” Higher scores indicated higher perceived control. The construct reliability was .84.
Perceived identification with school in 10th grade
Identification with school included three items from the student questionnaire that measured students’ interest and satisfaction with school. Two items had response options on a 4-point scale, ranging from 1 (strongly agree) to 4 (strongly disagree). The following is a sample item: “I go to school because I think the subjects I’m taking are interesting and challenging.” The item “How much do you like school?” had response options ranging from 1 (not at all) to 3 (a great deal). Items were coded, so that higher scores indicated higher perceived identification with school. The construct reliability of this scale was .78.
School engagement in 10th grade
The school engagement index consisted of 12 items that measured behavioral and academic dimensions of engagement. The items were coded, so that higher scores reflected higher levels of school engagement. Behavioral engagement included four items from the student questionnaire that measured the extent to which students conformed to classroom norms, such as not skipping school and not getting in trouble. Responses were rated on a 5-point scale (never, 1–2 times, 3–6 times, 7–9 times, and 10 or more times). The following is a sample item: “I got in trouble for not following school rules.” The construct reliability of this latent variable was .69. The academic engagement scale included eight items from a questionnaire that measured English and mathematics teachers’ perception of student effort, persistence, and attention in their classes (for more details, see Table 2 ). Responses for four items were rated on a 2-point scale (yes or no). The following is a sample item: “Does this student usually work hard for good grades in your class?” Responses for another four items were rated on a 5-point scale (never, rarely, some of the time, most of the time, and all of the time). The following is a sample item: “How often does this student complete homework assignments for your class?” The construct reliability of this scale was .80.
Standardized parameter estimates from the confirmatory factor analysis model.
Academic achievement
Academic achievement was estimated as a latent variable using standardized T -scores in math and reading. The standardized T -score provided a norm-referenced measurement of achievement with a mean of 50 and standard deviation of 10.
The construct reliability of this scale was .86.
Student dropout status in 12th grade
To ascertain the impact of school engagement on dropout, we used the ELS: 2002–2004 12th-grade measure of dropout status (1 = enrolled in 12th grade, 0 = identified spring term 2004 dropouts). Dropouts were defined as 10th-grade cohort members who were not enrolled in school during the spring term 2 years later, who had not received a high school diploma or general educational development credentials, and who had missed 4 or more consecutive weeks not due to accident or illness.
Plan for analysis
To answer the research questions, our analysis comprised two steps. First, we assessed the fit of the measurement model, using confirmatory factor analyses (CFAs). Second, we used structural equation modeling to test our hypothesized model of dropping out of high school.
We used bootstrapping to test the indirect effects (see Preacher & Hayes, 2008 ; Shrout & Bolger, 2002 ). We requested the recommended minimum of 500 bootstrap samples ( Cheung & Lau, 2008 ) drawn with replacement from the full dataset of 14,781 cases. Bootstrapping is a recommended method for testing mediation, as it does not require the normality assumption and has greater statistical power and control for Type I error than the widely used three-step multiple regression approach ( Baron & Kenny, 1986 ) or the Sobel ( Sobel, 1982 ) test ( Fairchild & McQuillin, 2010 ; Lau & Cheung, in press ; MacKinnon, Lockwood, Hoffman, West, & Sheets, 2002 ; MacKinnon, Lockwood, & Williams, 2004 ; Preacher & Hayes, 2004 ; Shrout & Bolger, 2002 ). Support for a mediating role is indicated if the bootstrap (bias-corrected) confidence interval does not include zero. In that case, we can conclude that there is a 95% probability that the indirect or mediating effect is significant.
We conducted all statistical analyses with Mplus 5.21 ( Muthén & Muthén, 1993–2010 ). Because most of our measures are categorical, we used robust mean- and variance-adjusted weighted least squares (WLSMV) to estimate our models. The WLSMV estimator produces consistent parameter estimates, unbiased standard errors, and corrects χ 2 when there are categorical variables ( Brown, 2006 ; Muthén & Satorra, 1995 ). WLSMV utilizes all available data without either imputing values or deleting cases, based on the assumption that missing data is missing completely at random ( Little, 1995 ).
To evaluate the fits of the measurement and structural models, we relied on a set of test statistics: the Steiger–Lind root mean square error of approximation (RMSEA; Steiger, 1990 ), the Bentler comparative fit index (CFI; Bentler, 1990 ), and the Tucker–Lewis index (TLI), which are less sensitive to large samples than the more traditional chi-square statistic. We followed the Hu and Bentler (1999) guidelines for evaluating the fit between the target model and the observed data: 1) RMSEA values less than 0.05 indicate excellent fit, and values in the vicinity of 0.08 indicate acceptable fit; 2) CFI and TLI values of .95 or greater indicate an excellent fit, and coefficients of 0.90 indicate a good fit.
Measurement model
The first step in our analyses involved confirming the existence of our hypothesized latent constructs via CFAs. In the first CFA model, we specified a five-factor model to verify the structure of school engagement in terms of behavioral engagement and academic engagement, the structure of self-system processes in terms of perception of control and identification with school, and the structure of academic achievement. As Table 2 suggests, all item parcels loaded significantly onto their respective factors, with standardized loadings ranging from .48 to .67 on behavioral engagement, from .20 to .87 on academic engagement, from .69 to .83 on perception of control, and from .62 to .79 on identification with school. Each of the overall goodness-of-fit indices suggested that the five-factor model fit sample data very well, χ 2 (176) = 2078.808, p > .05, RMSEA = .027, RMSEA C.I. = .026–.028, CFI = .96, TLI = .96.
In the second CFA model, we specified a two-factor model to verify the structure of teacher support and parent support. All item parcels loaded significantly onto their respective factors, with standardized loadings ranging from .42 to .78 on teacher support, and from .55 to .77 on parent support. Each of the overall goodness-of-fit indices suggested that the two-factor model fit data well, χ 2 (43) = 521.202, p > .05, RMSEA = .027, RMSEA C.I. = .025–.030, CFI = .98, TLI = .97.
We next fitted a measurement-only model, which is equivalent to fitting a CFA while simultaneously allowing all factors to correlate with one another. The measurement model showed a good fit to the data, χ 2 (465) = 5541.743, p > .05, RMSEA = 0.027, CFI = 0.97, TLI = 0.96. Table 3 presents the correlations among the latent constructs in the model. All variables appeared to have low to moderate correlations (from −.01 to .61), allowing us to eliminate the problems of multicollinearity ( Kline, 2005 ). In conclusion, the results of CFA supported the measurement component of the proposed model, suggesting that items adequately measured their underlying latent factors.
Intercorrelation among latent and observed variables.
Structural model
We used structural equation modeling to examine how social context, self-perceptions, school engagement, and academic achievement contribute to dropping out of high school. According to the hypothesized model (see Fig. 1 ), student perceptions of teacher and parent support predict students’ perceptions of control and identification with school, which in turn predict students’ behavioral and academic engagement and academic achievement, which in turn predict dropout. The hypothesized model fit the observed data well (fit indices for the model without bootstrap resampling procedure: χ 2 (472) = 8297.830, p > .05, RMSEA = .033, CFI = .96, TLI = .95). SSMMD-related constructs, as a whole, accounted for 36.8% of the variance in dropping out of high school. Fig. 2 is a path diagram showing the fully standardized direct effects. Table 4 shows the specific mediation effects, the bootstrap estimates, and the 95% bias-corrected confidence intervals. For the sake of clarity, we first describe the direct paths within the model and then present the indirect effects.

Standardized coefficients for the self-system model of motivational development applied to dropping out of high school. Only significant direct paths ( p < .05) are shown.
Standardized bootstrap estimates and 95% bias-corrected confidence intervals for indirect effects.
Note. N = 14,781. BC 95% CI = bias-corrected 95% confidence intervals (if does not contain zero, the mediated effect is significant);
PC = perceived control; DO = dropping out from high school; BE = behavioral engagement; AE = academic engagement; IS = identification with school; PS = parent support; TS = teacher support.
Direct effects between social context and self-system processes
Both contextual variables were positively associated with students’ perception of control ( β = .26, p < .05 for teacher support; β = .34, p < .05 for parent support) and identification with school ( β = .51, p < .05 for teacher support; β = .23, p < .05 for parent support). That is, as students’ perception of teacher support and parent support increased, their positive perception of control and of identification with school also increased.
Direct effects between social context and school engagement
We also tested the direct paths from contextual variables to school engagement. The results indicated that both contextual variables significantly contributed to academic ( β = .18, p < .05 for teacher support; β = .16, p < .05 for parent support) and behavioral engagement ( β = .22, p < .05 for teacher support; β = .12, p < .05 for parent support).
Direct effects between self-system processes and school engagement and academic achievement
Perceived control was positively associated with academic engagement ( β = .19, p < .05) and academic achievement ( β = .39, p < .05). Identification with school was positively associated with behavioral ( β = .24, p < .05) and academic ( β = .04, p < .05) engagement and negatively associated with academic achievement ( β = −.25, p < .05). Perceived control was not a significant predictor of behavioral engagement ( β = .03, p > .05).
Direct effects between school engagement and academic achievement
Academic and behavioral engagement were positively associated with achievement ( β = .33, p < .05 and β = .11, p < .05 respectively).
Direct effects between school engagement and achievement in 10th grade and dropping out of school in 12th grade
Behavioral and academic engagement and achievement were associated with decreased likelihood of dropping out of high school ( β = −.30, p < .05, β = −.27, p < .05, and β = −.20, p < .05, respectively).
Mediated effects between self-system processes in 10th grade and dropping out of school in 12th grade
The specific indirect effect of perception of control on dropping out of high school through academic engagement was significant ( β = −.05, BC 95% CI = −.07, −.03) and through behavioral engagement was not significant ( β = −.01, BC 95% CI = −.02, .01). Greater perception of control led to greater academic engagement, which in turn decreased the probability of dropping out of high school. The direct effect of perceived control on dropping out of high school was not significant ( β = −.03, p > .05), implying that academic engagement fully mediated the relations between perceived control and dropping out of high school. Additionally, the specific indirect effect of identification with school on dropping out of high school through behavioral engagement was β = −.07, BC 95% CI = −.10, −.05 and through academic engagement was β = −.01, BC 95% CI = −.02, .00. Greater identification with school led to greater behavioral and academic engagement, which in turn decreased the probability of dropping out of high school. The direct effect of perceived identification with school on dropping out of high school was not significant ( β = .05, p > .05), indicating that behavioral and academic engagement fully mediated the relations between identification with school and dropping out of high school.
Mediated effects between social context and school engagement
Our first outcome variable of interest in these mediation analyses was academic engagement. The specific indirect effect of teacher support on academic engagement through perception of control was β = .05, BC 95% CI = .04, .06 and through identification with school was β = .02, BC 95% CI = .001, .04. That is, greater teacher support led to greater perceived control and identification with school, which in turn increased academic engagement. The specific indirect effect of parent support on academic engagement through perception of control was β = .06, BC 95% CI = .05, .08 and through identification with school was β = .01, BC 95% CI = .001, .02. In other words, greater parent support led to greater perceived control and of identification with school, which in turn increased academic engagement. The direct effect of teacher support on academic engagement was significant ( β = .18, p < .05) and so was the direct effect of parent support on academic engagement ( β = .16, p < .05). These findings indicate that self-system processes partially mediated the relations between the social context and academic engagement.
Our second outcome variable of interest was behavioral engagement. The specific indirect effect of teacher support on behavioral engagement through perception of control was not significant ( β = .01, BC 95% CI = −.01, .02) and through identification with school was significant ( β = .12, BC 95% CI = .10, .14). The specific indirect effect of parent support on behavioral engagement through perception of control was not significant ( β = .01, BC 95% CI =−.01, .02) and through identification with school was significant ( β = .05, BC 95% CI = .04, .07). The direct effect of teacher support on behavioral engagement was significant ( β = .22, p < .05) and so was the direct effect of parent support on behavioral engagement ( β = .12, p < .05). These data indicate that identification with school partially mediated the relation between contextual variables and behavioral engagement.
The purpose of this study was to examine the interdependence of school engagement and dropping out in the context of SSMMD. The self-system model proved to be valid. First, results revealed that contextual factors, including teacher support and parent support, positively influenced students’ self-perceptions (perceived control and identification with school) and school engagement (academic and behavioral). Second, students’ perceived control positively influenced academic engagement and achievement, while identification with school negatively influenced achievement and positively influenced academic and behavioral engagement. Third, as expected, academic and behavioral engagement positively influenced students’ achievement, and academic and behavioral engagement and achievement measured in 10th grade influenced dropping out of school in 12th grade. Fourth, engagement fully mediated the relation between the self-systems and dropping out of high school. Also, self-systems partially mediated the relation between the social context and school engagement. Given these results, the present study contributes to the school engagement and dropout literature in five ways.
First, using data from a nationally representative sample, this study provides empirical support for SSMMD as applied to the important problem of dropping out of high school. Although similar models of school dropout were recently proposed by Appleton et al. (2008) , Fredricks et al. (2004) , and Rumberger (2006) , the authors did not test the underlying process model empirically. The only study that empirically tested the SSMMD as applied to the dropout process was conducted by Connell et al. (1995) . The results of the present study not only confirm Connell el al.’s finding, but also extend it to a nationally representative sample of high school students. Additionally, by measuring engagement as a multidimensional rather than a unidimensional construct we underline the importance of behavioral and academic engagement in the dropout process.
Second, this study provides further support for the role of social context in self-system processes and school engagement. Most research to date has focused on the impact of teachers ( Patrick et al., 2007 ; Ryan & Patrick, 2001 ) or of parents ( Hong & Ho, 2005 ; You & Sharkey, 2009 ) on student self-system processes and school engagement. Very little work has compared the relative impact of the two sources of social support. Results from this study suggest when teachers show interest in students, praise their efforts, and contribute to community building within the school; they directly influence students’ perception of self and nurture students’ levels of school engagement. Similarly, when parents speak frequently with their children about school-related topics, they contribute to students’ sense of identification with school, their general perception of control. As control and identification with school are enhanced, these energizing internal mechanisms motivate students to be academically and behaviorally engaged in school activities.
Third, the findings suggest that students’ self-systems affect their school engagement and academic achievement. This result not only confirms previous findings ( Furrer & Skinner, 2003 ; Legault, Green-Demers, & Pelletier, 2006 ; Skinner et al., 2008 ), but also provides new evidence about the magnitude of the effects. The direct effect of identification with school on behavioral engagement ( β = .24) was twice the magnitude of the direct effect of identification with school on academic engagement ( β = .04). In addition, the effect of perceived control on academic achievement ( β = .39) was about twice the magnitude of the effect on academic engagement ( β = .19). Contrary to our expectation, the effect of perceived control on behavioral engagement was not significant ( β = .03). Hence, our findings suggest that behavioral engagement was more influenced by perceived identification with school, and academic engagement and achievement were more related to perceived control. That is, students who identify with their school are more likely to conform to classroom rules and regulations, and students who believe in their ability to control the outcome of their educational experience are much more likely to work hard, complete homework, be attentive in mathematics and English classes, and score higher on achievement tests.
Fourth, our results suggest that behavioral and academic engagement and academic achievement are key variables to consider when predicting high school dropout. This finding is in line with research that has shown that behavioral disengagement ( Archambault et al., 2009 ; Ekstrom, Goertz, Pollack, & Rock, 1986 ; Finn, 2006 ; Ream & Rumberger, 2008 ) and academic achievement ( Battin-Pearson et al., 2000 ; Hardre & Reeve, 2003 ) are precursors of dropping out of high school. However, the present findings expand this research and provide evidence that academic engagement also is also a significant predictors of dropping out of high school and that it’s utility in predicting dropout is similar to that of behavioral engagement. Educators and policymakers interested in preventing school dropout may want to consider how to implement intervention strategies aimed at increasing students’ academic and behavioral engagement and academic achievement ( Reschly, 2010 ).
Fifth, this study suggests that academic and behavioral engagement are critical mediators between self-system processes and dropping out of high school. This finding suggests that students’ perception of control and identification with school may serve a dynamic purpose by initiating and sustaining a willingness to participate in academic activities and to conform to school rules and regulations, which in turn decrease the likelihood of dropping out of high school.
The current study has several limitations. First, the data were from an extant database; therefore, the measures of parent support and teacher support were limited in scope and design. Parent support is a multidimensional construct ( Epstein, 1995 ; Fan, 2001 ). However, in this study, we examined only one dimension of parent support. More studies employing the multidimensional approach of parent involvement are warranted. Similarly, we focused on only one facet of school context: teacher support. The ELS did not collect data about further aspects of teacher work, including support of autonomy and promotion of performance goals. Future research should investigate these aspects of teachers’ work. Second, we relied mostly on self-report information from students and teachers to assess perception of social context, perception of self, and school engagement. Although self-report measures are appropriate “when the theory or construct involved is attitudinal or perceptual” ( Schmitt, 1994 , p. 393), one could draw a more comprehensive picture by implementing multiple methodologies (e.g., observations). Third, findings are based on two time point. Thus, it is not known how results might vary if studied across multiple time points. Future research with longitudinal data could address this limitation.
In summary, despite the limitations, the findings of the present study are significant for both theory and practice. The study contributes to the literature by explicating the contributions and interactions of social context, self-system processes, and school engagement in predicting dropping out from high school. More specifically the present results highlight the centrality of supportive teachers and parents for promoting positive self-perceptions of control and identification with school and for nurturing student academic and behavioral engagement. Our results also underscore the importance of behavioral and academic engagement and academic achievement in predicting dropping out of high school. Our data offer further evidence that behavioral and academic engagement mediate the link between self-systems and dropping out of high school and that self-perceptions mediate the relations between teacher and parent support and academic and behavioral engagement. Future studies that focus on applying SSMMD to high school dropouts might consider testing this model across genders and ethnic groups.
☆ This research was supported by two grants from theInstitute of Education Sciences, U.S. Department of Education (R3214A100022 and R305F100013). The content is solely the responsibility of the authors and does not necessarily represent the official views of the Institute of Education Sciences or the U.S. Department of Education.
- Alexander KL, Entwisle DR, Horsey CS. From first grade forward: early foundations of high school dropout. Sociology of Education. 1997; 70 (2):87–107. [ Google Scholar ]
- Appleton JJ, Christenson SL, Furlong MJ. Student engagement with school: critical conceptual and methodological issues of the construct. Psychology in the Schools. 2008; 45 (5):369–387. [ Google Scholar ]
- Appleton JJ, Christenson SL, Kim D, Reschly A. Measuring cognitive and psychological engagement: validation of the student engagement instrument. Journal of School Psychology. 2006; 44 (5):427–445. [ Google Scholar ]
- Archambault I, Janosz M, Fallu JS, Pagani LS. Student engagement and its relationship with early high school dropout. Journal of Adolescence. 2009; 32 :651–670. [ PubMed ] [ Google Scholar ]
- Astone NM, McLanahan SS. Family structure, residential mobility, and school dropout: a research note. Demography. 1994; 31 (4):575–584. [ PubMed ] [ Google Scholar ]
- Baron RM, Kenny DA. The moderator-mediator variable distinction in social psychological research: conceptual, strategic, and statistical considerations. Journal of Personality and Social Psychology. 1986; 51 :1173–1182. [ PubMed ] [ Google Scholar ]
- Battin-Pearson S, Newcomb MD, Abbott RD, Hill KG, Catalano RF, Hawkins JD. Predictors of early high school dropout: a test of five theories. Journal of Educational Psychology. 2000; 92 :568–582. [ Google Scholar ]
- Bentler PM. Comparative fit indices in structural models. Psychological Bulletin. 1990; 107 :238–246. [ PubMed ] [ Google Scholar ]
- Brown TA. Confirmatory factor analysis for applied research. New York, NY: Guilford Press; 2006. [ Google Scholar ]
- Cairns RB, Cairns BD, Neckerman HJ. Early school dropout: configurations and determinants. Child Development. 1989; 60 :1437–1452. [ PubMed ] [ Google Scholar ]
- Caraway K, Tucker CM, Reinke WM, Hall C. Self-efficacy, goal orientation, and fear of failure as predictors of school engagement in high school students. Psychology in the Schools. 2003; 40 :417–427. [ Google Scholar ]
- Cheung GW, Lau RS. Testing mediation and suppression effects of latent variables. Organizational Research Methods. 2008; 11 :296–325. [ Google Scholar ]
- Connell JP, Halpern-Felsher B, Clifford E, Crichlow W, Usinger P. Hanging in there: behavioral, psychological, and contextual factors affecting whether African-American adolescents stay in school. Journal of Adolescent Research. 1995; 10 (1):41–63. [ Google Scholar ]
- Connell JP, Spencer MB, Aber JL. Educational risk and resilience in African-American youth: context, self, action, and outcomes in school. Child Development. 1994; 65 :493–506. [ PubMed ] [ Google Scholar ]
- Connell J, Wellborn JG. Competence, autonomy, and relatedness: a motivational analysis of self-system processes. In: Gunnar MR, Sroufe LA, editors. Selfprocess in development: Minnesota symposium on child psychology. Vol. 2. Hillsdale, NJ: Lawrence Erlbaum; 1991. pp. 167–216. [ Google Scholar ]
- DiPerna JC, Volpe RJ, Elliott SN. An examination of academic enablers and achievement in mathematics. Journal of School Psychology. 2005; 43 :379–392. [ Google Scholar ]
- Dotterer AM, Lowe K. Classroom context, school engagement, and academic achievement in early adolescence. Journal of Youth and Adolescence. 2011; 40 (12):1649–1660. [ PubMed ] [ Google Scholar ]
- Dynarski M, Gleason P, Rangarajan A, Wood R. Impacts of dropout prevention programs: Final report. Princeton, NJ: Mathematica Policy Research; 1998. [ Google Scholar ]
- Ekstrom RB, Goertz ME, Pollack JM, Rock DA. Who drops out of high school and why? Findings of a national study. Teachers College Record. 1986; 87 (3):3576–3730. [ Google Scholar ]
- Epstein J. School/family/community partnerships: caring for the children we share. Phi Delta Kappa. 1995; 76 :701–712. [ Google Scholar ]
- Fairchild AJ, McQuillin SD. Evaluating mediation and moderation effects in school psychology: a presentation of methods and review of current practice. Journal of School Psychology. 2010; 48 :53–84. [ PMC free article ] [ PubMed ] [ Google Scholar ]
- Fan X. Parental involvement and students’ academic achievement: a growth modeling analysis. Journal of Experimental Education. 2001; 70 (1):27–61. [ Google Scholar ]
- Finn JD. Withdrawing from school. Review of Educational Research. 1989; 59 (2):117–142. [ Google Scholar ]
- Finn JD. The adult lives of at-risk students: The roles of attainment and engagement in high school (NCES 2006-328) Washington, DC: National Center for Education Statistics; 2006. [ Google Scholar ]
- Finn JD, Rock DA. Academic success among students at risk for school failure. Journal of Applied Psychology. 1997; 82 :221–234. [ PubMed ] [ Google Scholar ]
- Fredricks JA, Blumenfeld PC, Paris AH. School engagement: potential of the concept, state of the evidence. Review of Educational Research. 2004; 74 (1):59–109. [ Google Scholar ]
- Furrer C, Skinner E. Sense of relatedness as a factor in children’s academic engagement and performance. Journal of Educational Psychology. 2003; 95 (1):148–162. [ Google Scholar ]
- Gleason P, Dynarski M. Do we know whom to serve? Issues in using risk factors to identify dropouts. Journal of Education for Students Placed at Risk. 2002; 7 (1):25–41. [ Google Scholar ]
- Goldschmidt P, Wang J. When can schools affect dropout behavior? A longitudinal multilevel analysis. American Educational Research Journal. 1999; 36 (4):715–738. [ Google Scholar ]
- Hancock GR, Mueller RO. Rethinking construct reliability within latent variable systems. In: Cudeck R, du Toit S, Sörbom D, editors. Structural equation modeling: Present and future – A Festschrift in honor of Karl Jöreskog. Lincolnwood, IL: Scientific Software International, Inc; 2001. [ Google Scholar ]
- Hardre P, Reeve J. A motivational model of rural students’ intentions to persist in, versus drop out of, high school. Journal of Educational Psychology. 2003; 95 (2):347–356. [ Google Scholar ]
- Hong S, Ho HZ. Direct and indirect longitudinal effects of parental involvement on student achievement: second order latent growth modeling across ethnic groups. Journal of Educational Psychology. 2005; 97 (1):32–42. [ Google Scholar ]
- Hu L, Bentler PM. Cutoff criteria for fit indexes in covariance structure analysis: conventional criteria versus new alternatives. Structural Equation Modeling. 1999; 6 (1):1–55. [ Google Scholar ]
- Ingels SJ, Pratt DJ, Rogers J, Siegel PH, Stutts ES. Education longitudinal study of 2002: Base-year data file user’s manual (NCES 2004-405) Washington, DC: U.S. Government Printing Office; 2004. http://nces.ed.gov/pubsearch Retrieved from. [ Google Scholar ]
- Ingels SJ, Pratt DJ, Wilson D, Burns LJ, Currivan D, Rogers JE, et al. Education longitudinal study of 2002: Base-year to second follow-up data file documentation (NCES 2008-347) Washington, DC: National Center for Education Statistics; 2007. [ Google Scholar ]
- Jimerson SR, Campos E, Greif JL. Toward an understanding of definitions and measures of school engagement and related terms. California School Psychologists. 2003; 8 :7–27. [ Google Scholar ]
- Kline RB. Principles and practices of structural equation modeling. New York, NY: Guilford Press; 2005. [ Google Scholar ]
- Lau RS, Cheung GW. Estimating and comparing specific mediation effects in complex latent variable models. Organizational Research Methods. in press. [ Google Scholar ]
- Legault L, Green-Demers I, Pelletier LG. Why do high school students lack motivation in the classroom? Toward an understanding of academic motivation and social support. Journal of Educational Psychology. 2006; 98 :567–582. [ Google Scholar ]
- Levin H, Belfield C, Muennig P, Rouse C. The costs and benefits of an excellent education for all of America’s children. New York, NY: Teachers College Press; 2007. [ Google Scholar ]
- Little RJA. Modeling the drop-out mechanism in repeated-measures studies. Journal of the American Statistical Association. 1995; 90 (431):1112–1121. [ Google Scholar ]
- MacKinnon DP, Lockwood CM, Hoffman JM, West SG, Sheets V. A comparison of methods to test mediation and other intervening variable effects. Psychological Methods. 2002; 7 :83–104. [ PMC free article ] [ PubMed ] [ Google Scholar ]
- MacKinnon DP, Lockwood CM, Williams J. Confidence limits for the indirect effect: distribution of the product and resampling methods. Multivariate Behavioral Research. 2004; 39 :99–128. [ PMC free article ] [ PubMed ] [ Google Scholar ]
- Moretti E. Crime and the costs of criminal justice. In: Belfield C, Levin H, editors. The price we pay: Economic and social consequences of inadequate education. Washington, DC: Brookings Institution Press; 2007. pp. 142–159. [ Google Scholar ]
- Muennig P. How education produces health: a hypothetical framework. Teachers College Record. 2007:1–17. [ Google Scholar ]
- Muthén BO, Muthén LK. Mplus statistical analysis with latent variables: User’s guide. 5. Los Angeles, CA: Muthén & Muthén; 1993–2010. [ Google Scholar ]
- Muthén B, Satorra A. Complex sample data in structural equation modeling. In: Marsden PV, editor. Sociological methodology. Washington, DC: American Sociological Association; 1995. pp. 267–316. [ Google Scholar ]
- Neild RC, Balfanz R. Unfulfilled promise: The dimensions and characteristics of Philadelphia’s dropout crisis, 2000–2005. Philadelphia, PA: Phil-adelphia Youth Transitions Collaborative; 2006. [ Google Scholar ]
- Orfield G. Losing our future: minority youth left out. In: Orfield G, editor. Dropouts in America: Confronting the graduation rate crisis. Cambridge, MA: Harvard Educational Press; 2006. [ Google Scholar ]
- Patrick H, Ryan A, Kaplan A. Early adolescents’ perceptions of the classroom social environment, motivational beliefs, and engagement. Journal of Educational Psychology. 2007; 99 :83–98. [ Google Scholar ]
- Preacher KJ, Hayes AF. SPSS and SAS procedures for estimating indirect effects in simple mediation models. Behavior Research Methods, Instruments and Computers. 2004; 36 :717–731. [ PubMed ] [ Google Scholar ]
- Preacher KJ, Hayes AF. Asymptotic and resampling strategies for assessing and comparing indirect effects in multiple mediator models. Behavior Research Methods. 2008; 40 (3):879–891. [ PubMed ] [ Google Scholar ]
- Ream RK, Rumberger RW. Student engagement, peer social capital, and school dropout among Mexican American and non-Latino White students. Sociology of Education. 2008; 81 :109–139. [ Google Scholar ]
- Reschly A. Reading and school completion: critical connections and Matthew effects. Reading and Writing Quarterly. 2010; 26 :1–23. [ Google Scholar ]
- Roderick M. The path to dropping out. Westport, CN: Auburn House; 1994. [ Google Scholar ]
- Roderick M, Nagaoka J, Bacon J, Easton JQ. Update: ending social promotion. 2000 http://ccsr.uchicago.edu/publications/p0g01.pdf Retrieved from.
- Rumberger RW. Dropping out of middle school: a multilevel analysis of students and schools. American Educational Research Journal. 1995; 32 (3):583–625. [ Google Scholar ]
- Rumberger RW. Why students drop out of school. In: Orfield G, editor. Dropouts in America: Confronting the graduation rate crisis. Cambridge, MA: Harvard Educational Press; 2006. [ Google Scholar ]
- Rumberger RW, Larson KA. Student mobility and the increased risk of high school dropout. American Journal of Education. 1998; 107 :1–35. [ Google Scholar ]
- Ryan AM, Patrick H. The classroom social environment and changes in adolescents’ motivation and engagement during middle school. American Educational Research Journal. 2001; 38 :437–460. [ Google Scholar ]
- Schmitt N. Method bias: the importance of theory and measurement. Journal of Organizational Behavior. 1994; 15 :393–398. [ Google Scholar ]
- Shrout PE, Bolger N. Mediation in experimental and nonexperimental studies: new procedures and recommendations. Psychological Methods. 2002; 7 (4):422–445. [ PubMed ] [ Google Scholar ]
- Sinclair MF, Christenson SL, Lehr CA, Anderson AR. Facilitating student engagement: lessons learned from check & connect longitudinal studies. The California School Psychologist. 2003; 8 :29–42. [ Google Scholar ]
- Skinner EA, Furrer C, Marchand G, Kindermann T. Engagement and disaffection in the classroom: part of a larger motivational dynamic? Journal of Educational Psychology. 2008; 100 (4):765–781. [ Google Scholar ]
- Skinner EA, Kindermann TA, Connell JP, Wellborn JG. Engagement as an organizational construct in the dynamics of motivational development. In: Wentzel K, Wigfield A, editors. Handbook of motivation in school. Mahwah, NJ: Erlbaum; 2009. pp. 223–245. [ Google Scholar ]
- Skinner EA, Wellborn JG. Coping during childhood and adolescence: a motivational perspective. In: Featherman D, Lerner R, Perlmutter M, editors. Life-span development and behavior. Vol. 12. Hillsdale, NJ: Erlbaum; 1994. pp. 91–133. [ Google Scholar ]
- Snyder TD, Dillow SA. Digest of education statistics 2009 (NCES 2010-013) Washington, DC: National Center for Education Statistics; 2010. [ Google Scholar ]
- Sobel ME. Asymptotic confidence intervals for indirect effects in structural equation models. In: Leinhardt S, editor. Sociological methodology. Washington, DC: American Sociological Association; 1982. pp. 290–312. [ Google Scholar ]
- Steiger JH. Structural model evaluation and modification: an interval estimation approach. Multivariate Behavioral Research. 1990; 25 :173–180. [ PubMed ] [ Google Scholar ]
- Stillwell R. Public school graduates and dropouts from the common core of data: School year 2007–08 (NCES 2010-341) Washington, DC: National Center for Education Statistics; 2010. http://nces.ed.gov/pubsearch/pubsinfo.asp?pubid=2010341 Retrieved from. [ Google Scholar ]
- Sum A, Khatiwada I, McLaughlin J, Palma S. The consequences of dropping out of high school. Boston, MA: Center for Labor Market Studies; 2009. [ Google Scholar ]
- Swanson CB, Schneider B. Students on the move: Residential and educational mobility in America’s schools. Sociology of Education. 1999; 72 :54–67. [ Google Scholar ]
- Teachman J, Paasch K, Carver K. Social capital and dropping out of school early. Journal of Marriage and the Family. 1996; 58 :773–783. [ Google Scholar ]
- Waldfogel J, Garfinkel I, Kelly B. Public assistance programs: how much could be saved with improved education? In: Belfield C, Levin HM, editors. The price we pay. Washington DC: Brookings Institution Press; 2007. pp. 160–176. [ Google Scholar ]
- Wang M, Holcombe R. Adolescents’ perceptions of school environment, engagement, and academic achievement in middle school. American Educational Research Journal. 2010; 47 :633–662. [ Google Scholar ]
- Wu JY, Hughes JN, Kwok OM. Teacher–student relationship quality type in elementary grades: effects on trajectories for achievement and engagement. Journal of School Psychology. 2010; 48 :357–387. [ PMC free article ] [ PubMed ] [ Google Scholar ]
- You S, Sharkey J. Testing a developmental–ecological model of student engagement: a multilevel latent growth curve analysis. Educational Psychology: An International Journal of Experimental Educational Psychology. 2009; 29 (6):659–684. [ Google Scholar ]
Academia.edu no longer supports Internet Explorer.
To browse Academia.edu and the wider internet faster and more securely, please take a few seconds to upgrade your browser .
Enter the email address you signed up with and we'll email you a reset link.
- We're Hiring!
- Help Center
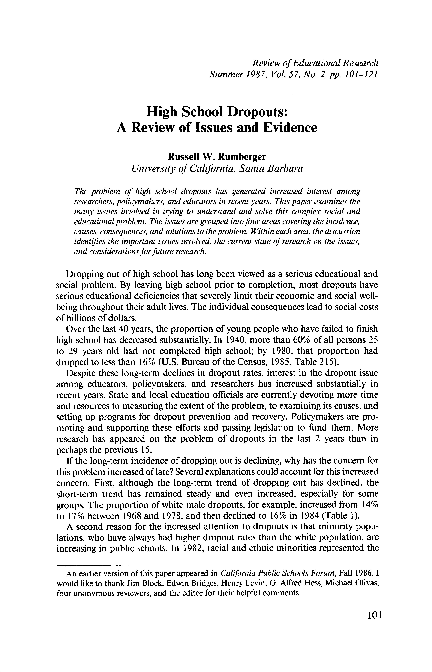
High school dropouts: A review of issues and evidence

1987, Review of educational research
Related Papers
Aaron Pallas
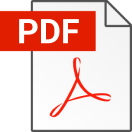
Steven Meeker , Stacey Edmonson
This study attempts to answer the following research question: What are the factors that prevent students from completing high school? The participants in this study included 228 current and recent students from General Educational Development (GED) programs across the state of Texas. In an effort to gain a clearer picture of circumstances faced by current high school students, only responses from participants in their teens or twenties were considered for the research concerning dropout factors. There were 158 respondents in this category. Data for this qualitative study were collected by means of surveys containing open-ended questions, focus groups, and semi-structured interviews. The significant findings of this study are as follows: (1) More than a quarter of the 158 participants in this study reported that pregnancy and parenting prevented them from graduating high school; (2) More than one-sixth reported that conflicts with school personnel as well as overall school dysfuncti...
Rodi Satriawan
Todd Fennimore
Youth & Society
Elizabeth Stearns
National Center for Education Statistics
Elizabeth Glennie
This report presents information about selected characteristics and experiences of high school sophomores in 2002 who subsequently dropped out of school. It also presents comparative data about late high school dropouts in the years 1982, 1992, and 2004. Three data sources ...
Dean Ramser, Ed.D.
Where and when does progress begin with our high school dropout problem? Is it simply bringing new technology to the class room as Morrell (2009) and Mahiri (2011) suggest? Is it tracking and detecting the potential dropout, and implementing intervention strategies as Heppen & Bowles (2008) suggest? If the ERO (20100 study was correct, and if 0.09% improvement is what can be hoped through Enhanced Reading Opportunities, what can be done with greater improvement? The Governor’s Report (2010) suggest that high school dropout behavior is predictable, why isn’t an intervention program in place now? Berliner (2008) saw an increase in high school graduation from those who reenrolled, so is that the strategy? Push to reenroll? Princotta & Harris (2009) suggest extended hours and extended days. Will that work, and if so, why is it not in place now? Hammond (2009) looks at teacher training institutions as the solution, yet it leans on Apple’s (2002; 2009; 2010) contention that the social structure of a hegemonic society is the obstacle to open discussions. Deli-Amen (2011) and Durkheim (1951) emphasize academic integration and social integration as effective models at addressing the achievement gap, and so does Rumberger (2011). As salient as it may be, the fissure of perceived inequality based on racial differences, will not be resolved through hierarchical mediation. That strategy reinforces the Hammond model of employing teaching graduates from the privileged institutions, and thereby purveyors of the pedagogical ideology of those institutions, which may not reflect the student population, like that of Paulo Freire’s conscientização. If the objective of learning is to develop higher level critical thinking skills (Dewey), then focusing on achieving an in-depth understanding of the world, allowing for the perception and exposure of perceived social and political contradictions, including the inequalities in the social stratification of ethnicities in our schools, will naturally lead to a form of critical consciousness that necessitates taking action against the oppressive elements in one's life that are illuminated by that understanding. The solution may rest in the uncharted territory of historical consciousness of the collective achievement gap carved out of our humanity, with a bit of 21st century technology to articulate the lighted path in the abyss between those two eternities of darkness.
Kate Sirota
Cynthia Kelly
RELATED PAPERS
cristian torres
DANIELA FLOREA
Emmanuel Flora
Ni patrie ni frontières
Yves F Coleman
International Journal of Engineering Research and Technology (IJERT)
IJERT Journal
Frontiers in Bioengineering and Biotechnology
Elisabeth Moyano
Hacia La Promocion De La Salud
Jose Enrique Vanegas
Clinical Nursing Studies
Optics Letters
Alexei Sokolov
Sains Malaysiana
Maizura Abu Sin
Analytical and Bioanalytical Electrochemistry
Jean-paul Chopart
Theranostics
Nikola Obradović
Thanasinh phunnavat
Jonas Petry
SN Comprehensive Clinical Medicine
mohammadali vakili
Baltic Journal of Modern Computing
Peteris Daugulis
Gene technology
Mohammad Al-Haggar
Journal of Bioprocessing & Biotechniques
Mugur Balan
The Journal of Thoracic and Cardiovascular Surgery
ANDREW COCHRANE
Frontiers in Earth Science
Ashfaq Ahmad Shah
Biotechnology Techniques
Rik Beeftink
aspek hukum
Syifa Aullia , IkhsanAli Sutarya
RELATED TOPICS
- We're Hiring!
- Help Center
- Find new research papers in:
- Health Sciences
- Earth Sciences
- Cognitive Science
- Mathematics
- Computer Science
- Academia ©2024

High School Dropout, Graduation, and Completion Rates: Better Data, Better Measures, Better Decisions (2011)
Chapter: 1 introduction, 1 introduction.
H igh school graduation and dropout rates have long been used as a central indicator of education system productivity and effectiveness and of social and economic well-being. Today, interest in the accuracy and usefulness of these statistics is particularly acute owing to a confluence of circumstances, including changing demographics, new legislative mandates, and heightened political pressures to reduce the incidence of dropping out. The population of American school-age children is shifting from native whites toward minorities and immigrants, populations that have a higher risk of dropping out; the new regime of educational accountability, especially the movement toward testing for promotion and graduation, has raised fears of a secondary effect on school dropout rates. In other words, students who are unable to pass these assessments may simply leave school before graduating. In addition, the No Child Left Behind (NCLB) Act of 2002 specifically requires an indicator of educational progress other than test scores at the high school level. Timely high school graduation appears to be the indicator of choice.
HIGH SCHOOL DROPOUT AND GRADUATION RATES
Despite the strong need for sound and reliable measures of high school dropout and completion, there has been widespread disagreement among researchers, statisticians, and policy analysts about the “true” rates, how they are best measured, and what trends are evident over time. Recently, a number of analysts have argued that the growing importance of alternative high school credentials, combined with various technical problems and political pressures,
has led to serious overreporting of “official” high school graduation rates. Their analyses produce national graduation rates of about 70 percent overall and 50 percent for minorities, numbers that are lower than those reported on the basis of official government sources (e.g., Education Week , 2009; Greene and Winters, 2002; Warren, 2004). Some researchers also contend that this problem of overreporting the graduation rate has been getting worse over time (Heckman and LaFontaine, 2008, 2010). Others (e.g., Mishel and Roy, 2006) counter that these analyses are incorrect and that the graduation rate, while still unacceptably low, has been accurately reported in national government surveys and has not changed appreciably over the past 20 years. Similar discrepancies, depending on data sources and the analyses conducted, exist in dropout and graduation estimates at state and local levels. At a time when policy makers are vitally interested in tracking the incidence of dropping out of school, they are faced with choosing among substantially discrepant estimates that would lead them to different conclusions regarding both the size of the dropout problem and how it has changed in recent years.
DATA SOURCES USED FOR ESTIMATES
Estimates of these rates are derived from a variety of sources using a variety of procedures. National estimates are derived from both cross-sectional and longitudinal sample surveys. The Current Population Survey (CPS) conducted by the U.S. Census Bureau is a nationally representative cross-sectional household survey that asks detailed questions about educational enrollment and experiences in October of each year. The National Center for Education Statistics (NCES) and the Bureau of Labor Statistics periodically conduct longitudinal surveys that track representative samples of youth through the usual high school years and beyond.
School administrative records on enrollments, dropouts, and diplomas have typically been used by states and school districts for reporting these rates. These data are reported annually to NCES as part of the Common Core of Data (CCD) collection of information on public schools in the country and have also been used to generate national, state, and district estimates of dropout and completion rates. Many states and school districts now have longitudinal unit-record administrative data systems that allow them to track the progress of individual students over time. However, decisions about ways to handle specific groups of students (e.g., students who transfer or who leave school but obtain a high school equivalency credential, like the General Educational Development [GED]) can affect the statistics that are calculated, even when the same formulas are used to calculate the rates.
Each data source brings with it a unique set of issues that can substantially affect the quality and usefulness of dropout rate statistics. Rates derived from sample-based surveys (both cross-sectional and longitudinal) have
been criticized because they rely on respondent self-reports (Heckman and LaFontaine, 2008, 2010), and some have questioned the degree to which longitudinal data accurately track disadvantaged populations (see National Research Council, 2010). Rates estimated from aggregated counts in administrative data systems have been questioned when adjustments are not made to control for repeating ninth graders or to account for transfer students (Warren, 2005). The ways that states and local school districts classify students as dropouts, graduates, or completers can significantly affect the rates that are calculated.
Whatever the data source, there are also major questions in defining both an appropriate numerator and a denominator in calculating these rates. For example, should it include private school enrollees? Recent émigrés enrolled in U.S. schools but who spent most of their education outside the U.S. education system? GED recipients? Special education students? “On-time” graduates only? Obviously, these choices should be driven by the policy questions being addressed as well as the availability of the desired data. However, until recently, no standard conventions for data inclusion or exclusion have been widely accepted in the education research and policy community. Efforts by the National Governors Association represent some progress toward standardizing methods for estimating graduation rates (National Governors Association Task Force on State High School Graduation Data, 2005). Nevertheless, there remains a lack of understanding about which calculation methods and which data are most appropriate for different policy questions, and often the best data sources may not be available for the calculations.
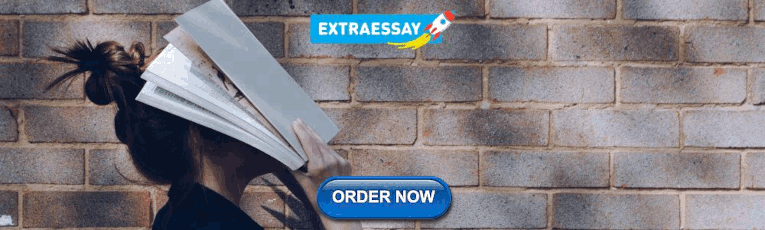
COMMITTEE CHARGE
The Committee for Improved Measurement of High School Dropout and Completion Rates was formed to convene a workshop and to make recommendations about these issues. Specifically, the steering committee was asked to address the following questions:
What are the available measures of dropout and completion rates, how are they determined, and what are their strengths and limitations?
To what extent do current and proposed measures attain the necessary levels of accuracy, given the types of policy decision that they inform?
What is the state of the art with respect to constructing longitudinal student accounting systems for measuring dropout and completion rates? What is the feasibility and desirability of moving to such systems? What are some of the issues that need to be considered when designing these data systems?
In what ways can the analysis of data from current and proposed systems for measuring dropout and completion rates be used to help understand changes in the rates?
How can this information be used to improve practice at the local level and improve public policies at the state and national levels?
In response to the charge, the committee organized a workshop designed to explore the strengths and weaknesses of various kinds of rates, the policy decisions based on them, and the kinds of data required to inform those policy decisions. The committee began this task by conducting a review of the literature. The topic addressed by this project—dropping out of high school—is one that has been studied in great depth, and the literature base is quite expansive. A review of the entire literature base was beyond the scope and resources of this study. The committee therefore focused its review on research explicitly related to its charge: studies on the calculation of dropout and completion rates, the information needed to calculate them, and the policy uses of these rates. The committee also conducted a limited review of research on the relationships between education attainment and social and economic outcomes. This review was designed to provide context for the work and to document the value of reporting dropout and completion rates, but it was not intended to be an exhaustive review of the literature on this topic. Based on this review, the committee identified the researchers who have been actively pursuing this line of study and invited a subset of them to participate in the workshop. The committee also recruited a set of policy makers, practitioners, and stakeholders to discuss these issues during the workshop.
The workshop was held on October 23 and 24, 2008, and consisted of four panels of speakers. The first panel focused on policy uses of these rates, and panelists represented different administrative levels of the education system in this country (i.e., national, state, district, and school). The second panel made presentations about methods for calculating the rates, including discussion of the decisions required and the strengths and weaknesses of the methods. The third panel focused on development of longitudinal databases and included representatives from state and local school districts, who talked about their work to develop these systems. The final panel addressed the issue of how these data systems can be used to improve policy and practice. This panel focused specifically on early indicators of students at risk of dropping out and how this research could be used to better inform policy and practice. The workshop agenda appears in Appendix A , along with a list of workshop participants and guests. The papers and presentations from this workshop, the research that the presenters referenced, and the information that the committee gathered as part of its own literature review served as the basis for this report and the committee’s recommendations.
IMPORTANT TERMS
Throughout this report, we use several terms that warrant clarification. We use the term “graduate” to refer to a student who earns a regular high school diploma and “graduation rate” as an indicator of the percentage of students in a given population who earned a regular high school diploma. We note, however, that the definition of “regular diploma” may vary as well as the time allowed to complete it. We use the term “completer” as the all-encompassing term to refer to a student who finished high school via one of multiple ways, such as by earning a regular high school diploma, a GED, or another type of certificate (a certificate of attendance, certificate of completion, etc.). Likewise, “completion rate” indicates the percentage of students in a given population who finished high school in any of these ways. We use the term “dropout” to refer to a student who did not complete high school and “dropout rate” as an indicator of the percentage of students in a given population who did not complete high school. Dropouts may include those who earn a GED or an alternative credential (depending on the specific indicator or the purpose of the indicator), but the group does not include students still enrolled in school after they were expected to complete. There are a number of policy definitions of these terms that further specify them (e.g., NCLB specifies that the graduation rate should include only on-time diploma earners, and it classifies GED recipients with dropouts). Unless otherwise specified in the report, we use the terms in their most general sense.
There are four general categories of dropout/completion indicators, which are defined below.
Individual cohort rate: a rate derived from longitudinal data on a population of individuals who share a common characteristic at one point in time, such as entering high school. The rate is based on tracking the students over the 4 years of high school or more to determine which of them graduated and which of them dropped out.
Aggregate cohort rate: a rate designed to approximate an individual cohort rate when longitudinal data are not available by using aggregate counts of students (e.g., number of ninth graders in a given year, number of graduates in a given year). For instance, an aggregate cohort rate might compare the number of students who graduate in one year with the number of students who entered high school 4 years earlier.
Status rate: a rate that represents the fraction of a population that falls into a certain category at a given point of time (e.g., the percentage of the total U.S. population that does not have a high school diploma).
Event rate: a rate that is the fraction of a population that experiences a particular event over a given time interval. For instance, the event dropout rate indicates the percentage of students who exit school during a specific academic year without having earned a diploma.
ORGANIZATION OF THE REPORT
This report summarizes the proceedings from the workshop. Following this introduction, Chapter 2 draws on the presentations from the first panel and explains why these rates are important and how they are used for policy purposes. Based on information presented during the second panel discussion, Chapter 3 discusses the decisions that must be made in calculating these rates, and Chapter 4 explores the different types of rates and their uses. An important use of dropout and completion rates is to identify which students are likely to drop out and when they are most at risk in order to implement programs and/or interventions aimed at keeping students in school. Chapter 5 draws from several of the workshop presentations and discusses the research on early indicators of dropping out as well as on building data systems that incorporate these indicators to enable early identification of at-risk students. Chapter 6 continues the discussion of database development and summarizes the presentations made by state and district representatives participating in the third panel. Chapter 7 lays out ways the data systems can be used to improve policy and practice. The committee’s conclusions and recommendations are presented at the end of each chapter and are summarized in Chapter 8 . The workshop agenda appears in Appendix A , along with a list of workshop participants and guests. Biographical sketches of committee members and staff appear in Appendix B .
High school graduation and dropout rates have long been used as indicators of educational system productivity and effectiveness and of social and economic well being. While determining these rates may seem like a straightforward task, their calculation is in fact quite complicated. How does one count a student who leaves a regular high school but later completes a GED? How does one count a student who spends most of his/her high school years at one school and then transfers to another? If the student graduates, which school should receive credit? If the student drops out, which school should take responsibility?
High School Dropout, Graduation, and Completion Rates addresses these issues and to examine (1) the strengths, limitations, accuracy, and utility of the available dropout and completion measures; (2) the state of the art with respect to longitudinal data systems; and (3) ways that dropout and completion rates can be used to improve policy and practice.
READ FREE ONLINE
Welcome to OpenBook!
You're looking at OpenBook, NAP.edu's online reading room since 1999. Based on feedback from you, our users, we've made some improvements that make it easier than ever to read thousands of publications on our website.
Do you want to take a quick tour of the OpenBook's features?
Show this book's table of contents , where you can jump to any chapter by name.
...or use these buttons to go back to the previous chapter or skip to the next one.
Jump up to the previous page or down to the next one. Also, you can type in a page number and press Enter to go directly to that page in the book.
Switch between the Original Pages , where you can read the report as it appeared in print, and Text Pages for the web version, where you can highlight and search the text.
To search the entire text of this book, type in your search term here and press Enter .
Share a link to this book page on your preferred social network or via email.
View our suggested citation for this chapter.
Ready to take your reading offline? Click here to buy this book in print or download it as a free PDF, if available.
Get Email Updates
Do you enjoy reading reports from the Academies online for free ? Sign up for email notifications and we'll let you know about new publications in your areas of interest when they're released.
Exploring statistical approaches for predicting student dropout in education: a systematic review and meta-analysis
- Survey Article
- Published: 29 November 2023
Cite this article
- Raghul Gandhi Venkatesan ORCID: orcid.org/0000-0001-8624-8282 1 ,
- Dhivya Karmegam ORCID: orcid.org/0000-0003-3307-8704 2 &
- Bagavandas Mappillairaju ORCID: orcid.org/0000-0003-4794-6250 3
325 Accesses
Explore all metrics
Student dropout is non-attendance from school or college for an extended period for no apparent cause. Tending to this issue necessitates a careful comprehension of the basic issues as well as an appropriate intervention strategy. Statistical approaches have acquired much importance in recent years in resolving the issue of student dropout. This is due to the fact that statistical techniques can efficiently and effectively identify children at risk and plan interventions at the right time. Thirty-six studies in total were reviewed to compile, arrange, and combine current information about statistical techniques applied to predict student dropout from various academic databases between 2000 and 2023. Our findings revealed that the Random Forest in 23 studies and the Decision Tree in 16 studies were among the most widely adopted statistical techniques. Accuracy and Area Under the Curve were the frequently used evaluation metrics that are available in existing studies. However, it is notable that the majority of these techniques have been developed and tested within the context of developed nations, raising questions about their applicability in different global settings. Moreover, our meta-analysis estimated a pooled proportion of overall dropouts of 0.2061 (95% confidence interval: 0.1845–0.2278), revealing significant heterogeneity among the selected studies. As a result, this systematic review and meta-analysis provide a brief overview of statistical techniques focusing on strategies for predicting student dropout. In addition, this review highlights unsolved problems like data imbalance, interpretability, and geographic disparities that might lead to new research in the future.
This is a preview of subscription content, log in via an institution to check access.
Access this article
Price includes VAT (Russian Federation)
Instant access to the full article PDF.
Rent this article via DeepDyve
Institutional subscriptions
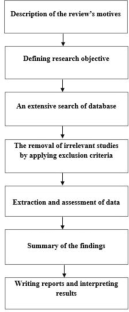
Similar content being viewed by others
Machine Learning Models for Predicting Student Dropout—a Review
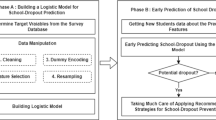
On predicting school dropouts in Egypt: A machine learning approach
Design, Development, and Evaluation of a Predictive Model for Regular School Dropout in the Chilean Educational System
Data availability.
All relevant data are within the manuscript and its supplementary information files.
Yukselturk, E., Ozekes, S., & Turel, Y. K. (2014). Predicting dropout student: An application of data mining methods in an online education program. European Journal of Open, Distance and E-Learning., 17 (1), 118–133.
Article Google Scholar
Lin, J. J. J., Imbrie ,P. K., & Reid, K. J. (2009). Student retention modelling: An evaluation of different methods and their impact on prediction results. In 2009 Research in Engineering Education Symposium REES 2009 (January).
Hu, Y.-H., Lo, C.-L., & Shih, S.-P. (2014). Developing early warning systems to predict students’ online learning performance. Computers in Human Behavior, 36 , 469–478.
Jia, P., & Maloney, T. (2015). Using predictive modelling to identify students at risk of poor university outcomes. Higher Education, 70 (1), 127–149.
Chun-Teck, L. (2010). Predicting preuniversity students’ mathematics achievement (published conference proceedings style). In: International conference on mathematics education research, multimedia university , Malaysia (pp. 299–306).
Adhatrao, K., Gaykar, A., Dhawan, A., Jha, R., & Honrao, V. (2013). Predicting students’ performance using ID3 and C4.5 classification algorithms. arXiv Preprint http://arxiv.org/abs/1310.2071
Durairaj, M., & Vijitha, C. (2014). Educational data mining for prediction of student performance using clustering algorithms. International Journal of Computer Science and Information Technologies, 5 (4), 5987–5991.
Google Scholar
Chen, J.-F., Hsieh, H.-N., & Do, Q. H. (2014). Predicting student academic performance: A comparison of two meta-heuristic algorithms inspired by cuckoo birds for training neural networks. Algorithms, 7 (4), 538–553.
Sales, A., Balby, L., & Cajueiro, A. (2016). Exploiting academic records for predicting student drop out: A case study in Brazilian higher education. Journal of Data, Information and Management, 7 (2), 166.
Ameri, S., Fard, M. J., Chinnam, R. B., & Reddy, C. K. (2016). Survival analysis based framework for early prediction of student dropouts. In International conference on information and knowledge management , 24–28 October (pp. 903–12).
Kitchenham, B., & Charters, S. (2007). Guidelines for performing Systematic Literature reviews in SoftwareEngineering Version 2.3. Engineering , 45 (4), 1051.
Okoli, C., & Schabram, K. (2012). A Guide to Conducting a Systematic Literature Review of Information Systems Research. SSRN Electron J [Internet]. . https://doi.org/10.2139/ssrn.1954824 .
Liberati, A., Altman, D. G., Tetzlaff, J., Mulrow, C., Gøtzsche, P. C., Ioannidis, J. P. A., et al. (2009). The PRISMA statement for reporting systematic reviews and meta-analyses of studies that evaluate health care interventions: Explanation and elaboration. Journal of Clinical Epidemiology, 62 (10), e1-34.
Moola, S. (2017). Checklist for analytical cross sectional studies. Joanna Briggs Institute Rev Man. (pp. 1–7). http://joannabriggs.org/research/critical-appraisal-tools .
Karmegam, D., Ramamoorthy, T., & Mappillairajan, B. (2019). A systematic review of techniques employed for determining mental health using social media in psychological surveillance during disasters. Disaster Medicine and Public Health Preparedness, 14 (2), 265–272.
Lamote, C., Van Damme, J., Van Den Noortgate, W., Speybroeck, S., Boonen, T., & de Bilde, J. (2013). Dropout in secondary education: An application of a multilevel discrete-time hazard model accounting for school changes. Quality & Quantity, 47 (5), 2425–2446.
Márquez-Vera, C., Romero Morales, C., & Ventura, S. S. (2013). Predicting school failure and dropout by using data mining techniques. Revista Iberoamericana de Tecnologias del Aprendizaje, 8 (1), 7–14.
Şara, N. B., Halland, R., Igel, C., & Alstrup, S. (2015). High-school dropout prediction using machine learning: A Danish large-scale study. In 23rd European symposium on artificial neural networks, computational intelligence and machine learning ESANN 2015—Proc eedings 2015 (pp. 319–324).
Costa, E. B., Fonseca, B., Santana, M. A., de Araújo, F. F., & Rego, J. (2017). Evaluating the effectiveness of educational data mining techniques for early prediction of students’ academic failure in introductory programming courses. Computers in Human Behavior, 73 , 247–256.
Aulck, L., Velagapudi, N., Blumenstock, J., & West, J. (2016). Predicting student dropout in higher education. http://arxiv.org/abs/1606.06364
Weybright, E. H., Caldwell, L. L., Xie, H., Wegner, L., & Smith, E. A. (2017). Predicting secondary school dropout among South African adolescents: A survival analysis approach. South African Journal of Education, 37 (2), 1–11.
Adelman, M., Haimovich, F., Ham, A., & Vazquez, E. (2018). Predicting school dropout with administrative data: New evidence from Guatemala and Honduras. Education Economics, 26 (4), 356–372. https://doi.org/10.1080/09645292.2018.1433127
Berens, J., Schneider, K., Görtz, S., Oster, S., & Burghoff, J. (2021). Early detection of students at risk—Predicting student dropouts using administrative student data and machine learning methods. SSRN Electronic Journal, 11 (3), 1–41.
Hegde, V., & Prageeth, P. P. (2018). Higher education student dropout prediction and analysis through educational data mining. In: 2018 2nd international conference on inventive systems and control (ICISC) . IEEE [cited 2021 Oct 14]. https://ieeexplore.ieee.org/document/8398887/
Nagy, M., & Molontay, R. (2018). Predicting dropout in higher education based on secondary school performance. In 2018 IEEE 22nd international conference on intelligent engineering systems (INES) . IEEE [cited 2021 Oct 14]. https://ieeexplore.ieee.org/document/8523888/
Lee S, Chung JY. The machine learning-based dropout early warning system for improving the performance of dropout prediction. Appl Sci. 2019;9(15).
da Silva, P. M., Lima, M. N. C. A., Soares, W. L., Silva, I. R. R., de Fagundes, R. A. A., de Souza, F. F. (2019). Ensemble regression models applied to dropout in higher education. In 2019 8th Brazilian conference on intelligent systems (BRACIS) . IEEE [cited 2021 Oct 14]. https://ieeexplore.ieee.org/document/8923655/
Al-Shabandar, R., Hussain, A. J., Liatsis, P., & Keight, R. (2019). Detecting at-risk students with early interventions using machine learning techniques. IEEE Access., 7 , 149464–149478.
Barros, T. M., Neto, P. A. S., Silva, I., & Guedes, L. A. (2019). Predictive models for imbalanced data: A school dropout perspective. Education Sciences, 9 (4), 275.
Biswas, A. A., Majumder, A., Mia, M. J., Nowrin, I., & Ritu, N. A. (2019). Predicting the enrollment and dropout of students in the post-graduation degree using machine learning classifier. International Journal of Innovative Technology and Exploring Engineering, 8 (11), 3083–3088.
Del Bonifro, F., Gabbrielli, M., Lisanti, G., & Zingaro, S. P. (2020). Student dropout prediction. Vol. 12163 LNAI, Lecture Notes in Computer Science (including subseries Lecture Notes in Artificial Intelligence and Lecture Notes in Bioinformatics) . Springer International Publishing (pp. 129–140). https://doi.org/10.1007/978-3-030-52237-7_11
Tenpipat, W., & Akkarajitsakul, K. (2020). Student dropout prediction: A KMUTT case study. In 2020 1st international conference on big data analytics and practices (IBDAP) . IEEE [cited 2021 Oct 14]. https://ieeexplore.ieee.org/document/9245457/
Patacsil, F. F. (2020). Survival analysis approach for early prediction of student dropout using enrollment student data and ensemble models. Universal Journal of Educational Research, 8 (9), 4036–4047.
Baranyi, M., Nagy, M., & Molontay, R. (2020). Interpretable deep learning for university dropout prediction. In SIGITE 2020—Proceedings 21st annual conference on information technology education (pp. 13–9).
Nangia, S., Anurag, J., & Gambhir, I. (2020). A machine learning approach to identity the students at the risk of dropping out of secondary education in India. Advances in Intelligent Systems and Computing . https://doi.org/10.1007/978-981-15-2475-2_51
Lottering, R., Hans, R., & Lall, M. (2020). A machine learning approach to identifying students at risk of dropout: A case study. International Journal of Advanced Computer Science and Applications, 11 (10), 417–422.
Freitas, F. A. D., Vasconcelos, F. F. X., Peixoto, S. A., Hassan, M. M., Dewan, M. A. A., de Albuquerque, V. H. C., et al. (2020). IoT system for school dropout prediction using machine learning techniques based on socioeconomic data. Electronics, 9 (10), 1613.
Maldonado, S., Miranda, J., Olaya, D., Vásquez, J., & Verbeke, W. (2021). Redefining profit metrics for boosting student retention in higher education. Decision Support Systems, 143 (August 2020), 113493.
Opazo, D., Moreno, S., Álvarez-Miranda, E., & Pereira, J. (2021). Analysis of first-year university student dropout through machine learning models: A comparison between universities. Mathematics., 9 (20), 1–27.
Fernandez-Garcia, A. J., Preciado, J. C., Melchor, F., Rodriguez-Echeverria, R., Conejero, J. M., & Sanchez-Figueroa, F. (2021). A real-life machine learning experience for predicting university dropout at different stages using academic data. IEEE Access., 9 , 133076–133090.
Queiroga, E. M., Batista Machado, M. F., Paragarino, V. R., Primo, T. T., & Cechinel, C. (2022). Early prediction of at-risk students in secondary education: A countrywide K-12 learning analytics initiative in Uruguay. Information, 13 (9), 1–25.
Segura, M., Mello, J., & Hernandez, A. (2022). Machine learning prediction of university student dropout: Does preference play a key role? Mathematics., 10 (18), 3359.
Moreira da Silva, D. E., Solteiro Pires, E. J., Reis, A., de Moura Oliveira, P. B., & Barroso, J. (2022). Forecasting students dropout: A UTAD university study. Future Internet., 14 (3), 1–14.
Mnyawami, Y. N., Maziku, H. H., & Mushi, J. C. (2022). Comparative study of AutoML approach, conventional ensemble learning method, and KNearest Oracle-AutoML model for predicting student dropouts in Sub-Saharan African countries. Applied Artificial Intelligence, 36 (1), 2145632.
Dake, D. K., & Buabeng-Andoh, C. (2022). Using machine learning techniques to predict learner drop-out rate in higher educational institutions. Mobile Information Systems, 2022 , 1–9.
Niyogisubizo, J., Liao, L., Nziyumva, E., Murwanashyaka, E., & Nshimyumukiza, P. C. (2022). Predicting student’s dropout in university classes using two-layer ensemble machine learning approach: A novel stacked generalization. Computers and Education: Artificial Intelligence., 3 (March), 100066. https://doi.org/10.1016/j.caeai.2022.100066
Flores, V., Heras, S., & Julian, V. (2022). Comparison of predictive models with balanced classes using the SMOTE method for the forecast of student dropout in higher education. Electronics, 11 (3), 457.
Garcia-Zanabria, G., Gutierrez-Pachas, D. A., Camara-Chavez, G., Poco, J., & Gomez-Nieto, E. (2022). SDA-Vis: A visualization system for student dropout analysis based on counterfactual exploration. Applied Sciences, 12 (12), 5785.
Selim, K. S., & Rezk, S. S. (2023). On predicting school dropouts in Egypt: A machine learning approach. Education and Information Technologies, 28 , 9235–9266. https://doi.org/10.1007/s10639-022-11571-x
Song, Z. H., Sung, S. H., Park, D., & Park, B. K. (2023). All-year dropout prediction modeling and analysis for university students. Applied Sciences, 13 (2), 1143.
Wang, N. (2016). How to conduct a meta-analysis of proportions in R: A comprehensive tutorial . John Jay College Criminal Justice (June):1–63.
Zheng, Z., Cai, Y., & Li, Y. (2015). Oversampling method for imbalanced classification. Computer Informatics., 34 (5), 1017–1037.
Learned-Miller, E. G. (2014). Introduction to Supervised Learning (p. 3). Amherst, MA, USA: Department of Computer Science, University of Massachusetts. https://people.cs.umass.edu/~elm/Teaching/Docs/supervised2014a.pdf
Kumar, M., Singh, A. J., & Handa, D. (2017). Literature survey on educational dropout prediction. International Journal of Education and Management Engineering, 7 (2), 8.
Shahiri, A. M., Husain, W., & Rashid, N. A. (2015). A review on predicting student’s performance using data mining techniques. Procedia Computer Science., 72 , 414–422. https://doi.org/10.1016/j.procs.2015.12.157
Gray, G., McGuinness, C., & Owende, P. (2014). An application of classification models to predict learner progression in tertiary education. In 2014 IEEE international advance computing conference (IACC) . IEEE (pp. 549–554).
Arsad, P. M., & Buniyamin, N. (2013). A neural network students’ performance prediction model (NNSPPM). In 2013 IEEE international conference on smart instrumentation, measurement and applications (ICSIMA) . IEEE (pp. 1–5).
Sathya, R., & Abraham, A. (2013). Comparison of supervised and unsupervised learning algorithms for pattern classification. International Journal of Advanced Research in Artificial Intelligence, 2 (2), 34–38.
Natek, S., & Zwilling, M. (2014). Student data mining solution-knowledge management system related to higher education institutions. Expert Systems with Applications, 41 (14), 6400–6407.
Kartal, O. O. (2015). Using survival analysis to investigate the persistence of students in an introductory information technology course at METU . Middle East Technical University.
Li, Y., Yang, T., Zhou, J., & Ye, J. (2018). A multi-task learning formulation for survival analysis. Proceedings of SIGKDD . https://doi.org/10.1137/1.9781611975321.33
Bani, M. J., & Haji, M. (2017). College student retention: When do we losing them? arXiv Preprint http://arxiv.org/abs/1707.06210
Zohair, L. M. A. (2019). Prediction of student’s performance by modelling small dataset size. International Journal of Educational Technology in Higher Education, 16 (1), 1–18.
Hellas, A., Ihantola, P., Petersen, A., Ajanovski, V. V., Gutica, M., Hynninen, T., Knutas, A., Leinonen, J., Messom, C., & Liao, S. N. Predicting academic performance: A systematic literature review. In Proceedings companion of the 23rd annual ACM conference on innovation and technology in computer science education (pp. 175–99).
Kaliannan, M., & Chandran, S. D. (2012). Empowering Students through Outcome-Based Education (OBE). Res Educ[Internet]. , 87 (1), 50–63.
Arroway, P., Morgan, G., O’Keefe, M., & Yanosky, R. (2016). Learning analytics in higher education . Research report. ECAR, Louisville, CO.
Rajak, A., Shrivastava, A. K., & Shrivastava, D. P. (2018). Automating outcome based education for the attainment of course and program outcomes. In 2018 Fifth HCT Information Technology Trends (ITT) . IEEE (pp. 373–376).
Namoun, A., & Alshanqiti, A. (2021). Predicting student performance using data mining and learning analytics techniques: A systematic literature review. Applied Sciences, 11 (1), 1–28.
Chen, J., Fang, B., Zhang, H., & Xue, X. (2022). A systematic review for MOOC dropout prediction from the perspective of machine learning. Interactive Learning Environments . https://doi.org/10.1080/10494820.2022.2124425
Manjarres, A. V., Sandoval, L. G. M., & Suárez, M. S. (2018). Data mining techniques applied in educational environments: Literature review. Digital Education Review, 33 , 235–266.
Romero, C., & Ventura, S. (2020). Educational data mining and learning analytics: An updated survey. Wiley Interdisciplinary Reviews: Data Mining and Knowledge Discovery, 10 (3), e1355.
Shmueli, G. (2010). To explain or to predict? Statistical Science, 25 (3), 289–310.
Thammasiri, D., Delen, D., Meesad, P., & Kasap, N. (2014). A critical assessment of imbalanced class distribution problem: The case of predicting freshmen student attrition. Expert Systems with Applications, 41 (2), 321–330.
Kaur, H., Pannu, H. S., & Malhi, A. K. (2019). A systematic review on imbalanced data challenges in machine learning: Applications and solutions. ACM Computing Surveys, 52 (4), 1–36.
Mduma, N. (2023). Data balancing techniques for predicting student dropout using machine learning. Data, 8 (3), 49.
Mgala, M., & Mbogho, A. (2015). Data-driven intervention-level prediction modeling for academic performance. In Proceedings of the seventh international conference on information and communication technologies and development (pp. 1–8).
Adadi, A., & Berrada, M. (2020). Peeking inside the black-box: A survey on explainable artificial intelligence (XAI). IEEE Access., 2018 (6), 52138–52160.
Sghir, N., Adadi, A., & Lahmer, M. (2023). Recent advances in predictive learning analytics: A decade systematic review (2012–2022). Education and Information Technologies, 28 , 8299–8333. https://doi.org/10.1007/s10639-022-11536-0
Lakkaraju, H., Aguiar, E., Shan, C., Miller, D., Bhanpuri, N., Ghani, R., & Addison, K. L. A machine learning framework to identify students at risk of adverse academic outcomes. In Proceedings of the 21th ACM SIGKDD international conference on knowledge discovery and data mining (pp. 1909–1918).
Mduma, N., Kalegele, K., & Machuve, D. (2019). A survey of machine learning approaches and techniques for student dropout prediction. Data Science Journal, 18 (1), 1–10.
Download references
Acknowledgements
We thank Mr. Naman Gupta, Research Assistant, Department of Ophthalmology, Visual, and Anatomical Sciences, Wayne State University, Detroit, Michigan for his valuable support for database access throughout the process. We also thank Ms. Supriya Sathish Kumar, Research Scholar, Translational Medicine and Research, SRM Institute of Science and Technology, and reviewers for comments that greatly improved the manuscript.
Author information
Authors and affiliations.
Department of Mathematics, SRM Institute of Science and Technology, Kattankulathur, Tamil Nadu, 603203, India
Raghul Gandhi Venkatesan
School of Public Health, SRM Institute of Science and Technology, Kattankulathur, Tamil Nadu, 603203, India
Dhivya Karmegam
Centre for Statistics, SRM Institute of Science and Technology, Kattankulathur, Tamil Nadu, 603203, India
Bagavandas Mappillairaju
You can also search for this author in PubMed Google Scholar
Corresponding author
Correspondence to Bagavandas Mappillairaju .
Ethics declarations
Conflict of interest.
On behalf of all authors, the corresponding author states that there is no conflict of interest.
Additional information
Publisher's note.
Springer Nature remains neutral with regard to jurisdictional claims in published maps and institutional affiliations.
Supplementary Information
Below is the link to the electronic supplementary material.
Supplementary file1 (DOCX 14 KB)
Supplementary file2 (docx 25 kb), supplementary file3 (docx 14 kb), supplementary file4 (docx 33 kb), supplementary file5 (docx 15 kb), supplementary file6 (docx 19 kb), supplementary file7 (docx 778 kb), rights and permissions.
Springer Nature or its licensor (e.g. a society or other partner) holds exclusive rights to this article under a publishing agreement with the author(s) or other rightsholder(s); author self-archiving of the accepted manuscript version of this article is solely governed by the terms of such publishing agreement and applicable law.
Reprints and permissions
About this article
Venkatesan, R.G., Karmegam, D. & Mappillairaju, B. Exploring statistical approaches for predicting student dropout in education: a systematic review and meta-analysis. J Comput Soc Sc (2023). https://doi.org/10.1007/s42001-023-00231-w
Download citation
Received : 17 June 2023
Accepted : 23 October 2023
Published : 29 November 2023
DOI : https://doi.org/10.1007/s42001-023-00231-w
Share this article
Anyone you share the following link with will be able to read this content:
Sorry, a shareable link is not currently available for this article.
Provided by the Springer Nature SharedIt content-sharing initiative
- Dropout prediction
- Supervised learning
- Unsupervised learning
- Meta-analysis
- Find a journal
- Publish with us
- Track your research
High School Dropout Research Paper
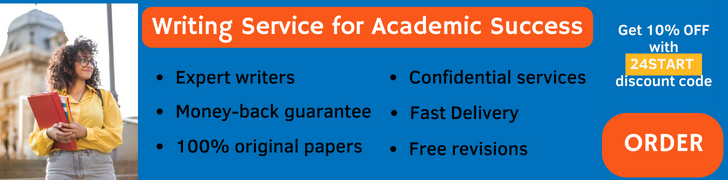
Sample High School Dropout Research Paper. Browse other research paper examples and check the list of research paper topics for more inspiration. If you need a research paper written according to all the academic standards, you can always turn to our experienced writers for help. This is how your paper can get an A! Feel free to contact our custom research paper writing service for professional assistance. We offer high-quality assignments for reasonable rates.
Dropping out of high school, that is, leaving school without graduating, is a central educational issue in almost all industrialized countries, although large differences between educational systems make cross-national or cross-cultural comparisons problematic. Nevertheless, there is broad international agreement that dropping out increases the risk of subsequent criminal behavior and drug abuse, lower occupational and economic prospects, lower lifetime earnings, and becoming a member of the underclass. Furthermore, dropping out increases the probability of lower levels of mental and physical health. Studies investigating the consequences of high school dropout at a macrolevel have shown that higher dropout rates result in lower tax revenues and increased expenditure for governmental assistance programs. Against the backdrop of all these negative consequences, sociological, educational, and psychological researchers have investigated the reasons for dropping out. The reasons identified can be classified as school-related, family-based, or personal. Researchers agree that the risk of dropping out begins to develop early in a student’s life and that intervention programs to prevent dropout should therefore be initiated at an early age.
Academic Writing, Editing, Proofreading, And Problem Solving Services
Get 10% off with 24start discount code, 1. problems in calculating the dropout rate.
A major challenge facing any study of high school dropout is how to define and identify the dropout population. There is some agreement, among American researchers at least, that students who were enrolled in grades 10, 11, or 12, but left school without graduating are to be defined as dropouts. However, this definition is often not viable in other countries. In international comparison, school systems not only differ with respect to the organization of primary and secondary schools, forms of ability grouping, and curricula, they also differ in terms of the age at which students are allowed to leave school. While students in the UK and most states of the USA are legally obliged to attend school until the age of 16, for example, students in other countries (e.g., Germany) are not allowed to leave school until they are 18. As a consequence of the different lengths of compulsory schooling, the probability that a student will drop out between the ages of 16 and 18 varies from country to country. Furthermore, there is some evidence that high schools tend to overestimate the percentage of dropout (e.g., Rumberger et al. 1990), and it has been argued that ‘dropout data are probably the least reliable information available today regarding the reality of schools’ (Frymier 1996, p. 4).
Beyond these problems of definition, it is characteristic of almost all industrialized countries that school participation rates increased continuously during the twentieth century. In the USA, for example, the proportion of 14to 17-year-olds enrolled in high school increased from 11 percent in 1900 to 94 percent in 1978. However, dropout rates have been relatively stable since the beginning of the 1970s (between 5 and 10 percent in industrialized countries).
2. Factors Influencing High School Dropout
Three major directions of research into the antecedents of high school dropout can be distinguished, although there is substantial theoretical overlap between the three approaches. While the first two types of research concentrate on family background and school variables from the sociological perspective, the third— more psychological—approach focuses on individual characteristics in terms of achievement and motivation.
2.1 Family Background And High School Dropout
Where family background is concerned, the determinants found to be of importance for academic achievement have also been considered with respect to dropout (e.g., Rumberger 1983, Rumberger and Thomas 2000). Parents’ level of education represents a potential predictor of dropout, that is, better educated parents spend more time with their children, thus supporting their accomplishments and increasing the likelihood that they will remain in school. Furthermore, these parents influence the academic performance of their children by imparting the values, aspirations, and motivation needed to succeed and remain in school. With regard to parental income, children from poor families probably feel more pressure to contribute to the family income, and thus leave school to seek work at an earlier stage. Family structures are also important, in that children from broken homes where one or both parents are absent are less likely to receive the support and encouragement needed to stay in school. The absence of parents, however, is often combined with financial problems that might explain the dropout. In a more detailed analysis of family influences, Rumberger et al. (1990) investigated the interaction styles between parents and children and the consequences for dropout. Dropouts were more likely to live in households characterized by a permissive or an authoritarian parenting style, their parents were more likely to use extrinsic punishments as a reaction to poor grades, and reacted with more negative emotions to both good and bad school grades. Furthermore, dropout students reported that their parents were less involved in their education and gave them less help with their homework.
In the USA, in particular, relations between ethnic group membership and high school dropout have been investigated, and higher percentages of dropout have been reported for Hispanic and African-American students. In a study investigating primary reasons for dropout, Rumberger (1983) found out that African-American females most often cited marriage and pregnancy as reasons for leaving school. However, if SES and individual achievement are held constant, the relationship between ethnic background and dropout diminishes or reverses, thus suggesting that the association is mainly mediated by family background and individual levels of achievement (e.g., McNeal 1995).
2.2 School Characteristics And High School Dropout
School characteristics have been investigated in research on school effectiveness. Authors agree that not only academic achievement, but dropping out of school is a reliable indicator of school effectiveness. Rumberger and Thomas (2000) identify school resources, structural characteristics of schools, and school processes as factors, which can influence high school dropout. With regard to the financial resources of schools, research shows that the student-teacher ratio is positively related to high school dropout. Furthermore, there is some evidence that a higher percentage of teachers with advanced degrees increase the likelihood that students will remain in high school.
Structural characteristics such as school location (urban, suburban, rural), size, and type (state vs. private) also seem to influence dropout, even when important individual and family characteristics are controlled. Research by Pittman and Haughtwout (1987) revealed a positive correlation between high school size and dropout rate. Mediation analyses demonstrated that this effect was mediated primarily by the social climate (sense of cohesion, level of student participation in school activities, interaction with faculty, the magnitude of certain problems at the school), and indicated that smaller schools had a better social climate and consequently lower dropout rates. Private schools usually have lower dropout rates than state schools (e.g., Rumberger and Thomas 2000), and students attending urban schools are more likely to drop out than their counterparts in suburban schools (e.g., Frymier 1996).
Research on school processes has identified a number of school-related variables that influence dropout rates. A higher level of cooperation between teachers and students seems to reduce dropout rates and, with regard to the academic climate, Bryk and Thum (1989) found that dropout rates were lower in schools in which students took more advanced courses and reported doing more homework. Bryk and Thum also reported that high schools with serious staffing problems had higher dropout rates.
Rumberger and Thomas (2000) suggested that because schools are anxious to show that their students’ test scores are improving, they actively reduce the membership of low-achieving students through a process of discharge. This process affects not only low achieving students, but also hard-to-educate students. Since both variables are confounded with SES and ethnicity, these school processes usually lead to higher dropout rates for students with low SES, and for Hispanic and African-American students.
2.3 Individual Determinants Of High School Dropout
Although many background and school variables are important antecedents of dropout, a more psychological view of the problem suggests that the concrete decision of whether or not to carry on or leave school is guided by individual characteristics such as attitudes and motivational states. Schools, parents, and teachers provide an academic environment, and students’ perceptions of this environment determine their achievement motivation, their behavioral intentions, and their academic behavior and choices. In one of the few psychological studies on reasons for dropout, Vallerand et al. (1997) proposed a model assuming self-determined academic motivation to be an important factor influencing behavioral intentions and dropout behavior. Their study suggests that students who perceive their parents, teachers, and school administrations as supportive develop a higher level of perceived competence and academic autonomy, and that this leads to higher intrinsic academic motivation and a lower likelihood of leaving school without graduating.
Expectancy-value models (e.g., Wigfield and Eccles 2000) suggest that academic choices are based mainly on expectancies of success in the domain of choice, and on how students value the domain. Both expectancies and values are based on family background, school and class variables, and previous individual achievement-related experiences. Low expectancies and values with respect to the future school career increase the probability of dropping out.
3. Prevention Of High School Dropout
Low levels of academic achievement (e.g., Caliste 1984) and high absenteeism (e.g., Bryk and Thum 1989) are the strongest behavioral predictors of high school dropout. Several authors (e.g., Simner and Barnes 1991) have pointed out that the earlier a student with low achievement levels and high absenteeism can be identified, the more likely is it that dropout prevention will be successful. An effective 12- week prevention program proposed by Caliste (1984) first identifies at-risk students (grade-point averages below 1.0 on a 4.0 scale). These students receive extra tuition from their teachers and participate in group counseling sessions. Topics discussed during the weekly counseling sessions include motivation, relevance of school, academic problems, career goals, and study habits. In the tutoring sessions, students are primarily assisted with current coursework and given some remedial instruction.
Participation in out-of-school activities, particularly sports clubs, can also prevent dropout. McNeal (1995) argues that higher social integration in both school and in non-academic clubs influences whether or not students remain in school. His analyses show that for low-achieving students (one standard deviation below the population mean) not involved in sports clubs, the probability of dropout is p=0.25, while for members of sports clubs the probability is only p=0.17.
Finally, authors agree that children from disadvantaged family backgrounds need particular social and financial support. Females, especially African-Americans, often leave school without graduating because of marriage and pregnancy. Day-care programs are viable measures to prevent drop out here.
Bibliography:
- Bryk A S, Thum Y M 1989 The effects of high school organization on dropping out: An exploratory investigation. American Educational Research Journal 26: 353–83
- Caliste E R 1984 The effect of a twelve week dropout intervention program. Adolescence 19: 649–57
- Frymier J 1996 Determining dropout rates in large city school districts: Problems and accomplishments. The High School Journal 80(1): 1–10
- McNeal R B Jr 1995 Extracurricular activities and high school dropouts. Sociology of Education 68: 62–81
- Pittman B P, Haughtwout P 1987 Influence of high school size on dropout rate. Educational Evaluation and Policy Analysis 9: 337–43
- Rumberger R W 1983 Dropping out of high school: The influence of race, sex, and family background. American Educational Research Journal 20: 199–220
- Rumberger R W, Ghatak R, Poulos G, Ritter P L, Dornbusch S M 1990 Family influences on dropout behavior in one California high school. Sociology of Education 63: 283–99
- Rumberger R W, Thomas S L 2000 The distribution of dropout and turnover rates among urban and suburban high schools. Sociology of Education 73: 39–67
- Simner M L, Barnes M J 1991 Relationships between first-grade marks and the high school dropout problem. The Journal of School Psychology 29: 331–5
- Vallerand R J, Fortier M S, Guay F 1997 Self-determination and persistence in a real-life setting: Toward a motivational model of high school dropout. Journal of Personality and Social Psychology 72: 1161–76
- Wigfield A, Eccles J S 2000 Expectancy-value theory of achievement motivation. Contemporary Educational Psychology 25: 68–81
ORDER HIGH QUALITY CUSTOM PAPER

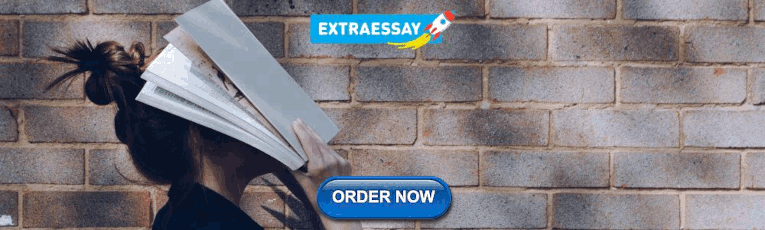
IMAGES
VIDEO
COMMENTS
The first nationally representative study to address reasons for high school dropout was the EEO study of 1955. It was a private study of 35,472 high school sophomores and seniors conducted by Educational Testing Services from a National Science Foundation grant (Eckland, 1972; Griffin & Alexander, 1978). It included 35,472 high school ...
show that 3.4% of students dropped out that year. Alternatively, status dropout is the percentage. of 16- to 24-year-olds who are not enrolled in school and have not earned a high school ...
California Dropout Research Project Report #15 October 2008 By ... high school dropout and graduation. The paper first reviews the theoretical literature on student ... persistence.1 Most models have attempted to explain why students drop out of high school. Some have attempted to explain engagement, an important precursor to dropping out. ...
been a significant change in the high school dropout rate in Wisconsin since the 1970's. In the 1969-1970 school year, the dropout rate was 4.68%. In the 2002-2003 school year it was at 8.17%. It has fluctuated over the years but the best school year for Wisconsin in terms of the least amount of dropouts was the 1970-1 97 1 when the dropout ...
I dedicate this paper to the memory of my loving brother, Robbie. 4 ... Department of Education's High School Dropout and Completion Rates 2007 Compendium ... there is a need for dropout research that investigates more than graduation outcome resultant of such alternative programs, and instead considers the
The aim of this study is to determine the risk level of high school students for dropout. The sample of the research in the descriptive survey model consisted of 578 students studying in public high schools in the central districts of Mersin. The "School Dropout Risk Scale" was used as a data collection tool in the study.
School dropout is a major concern in many societies. In Western countries in particular, a large proportion of youth quit school before obtaining a high school diploma (Eurostat, 2017; Statistics Canada, 2017; U.S. Department of Commerce, 2017).Many youth who drop out face important setbacks upon entering adulthood: compared to high school graduates, they rely more on social assistance ...
Almost one-third of all public secondary students in the United States each year dropout of school (Snyder & Dillow, 2010; Stillwell, 2010).Dropout rates vary across groups and settings, with Hispanic (36.5%) and African American (38.5%) students dropping out at higher rates than Asian (8.6%) and White (19%) students (Stillwell, 2010).High rates of dropout affect individuals, families, and ...
1 Background and Context. F ailure to complete high school has been recognized as a social problem in the United States for decades and, as discussed below, the individual and social costs of dropping out are considerable. Social scientists, policy makers, journalists, and the public have pondered questions about why students drop out, how many drop out, what happens to dropouts, and how young ...
student who eventually drop out of high school. If so, attendance patterns could be used as an indicator for students at risk or dropping out. Using attendance data, the study investigated these absences. This study examined the elementary attendance records of 42 high school dropouts. All of these students attended
A Bleak Prospect. High school dropouts earn $9,200 less per year on average than those who graduate. Over the course of their lifetimes, they will earn an average of $375,000 less than high school graduates, and roughly $1 million less than college graduates (Center for Labor Market Studies, 2007).
Different research studies conducted on the dropout rate in high schools have taken it as a complex process, ... which probably not up to the mark presently causing a high rate of school dropout students." ... Rumberger R. W. (1987). High school dropouts: A review of issues and evidence. Review of Educational Research, 57(2), 101-121.
High School Dropout, Graduation, and Completion Rates addresses these issues and to examine (1) the strengths, limitations, accuracy, and utility of the available dropout and completion measures; (2) the state of the art with respect to longitudinal data systems; and (3) ways that dropout and completion rates can be used to improve policy and ...
Overall, dropout programs (152 studies; 317 independent samples) were effective in reducing school dropout (or increasing school completion). The random effects weighted mean odds ratio for the ...
Academia.edu is a platform for academics to share research papers. High school dropouts: A review of issues and evidence ... for example, dropout rates for the 63 Chicago high schools range from 10% to 62% (Toles, Schulz, & Rice, 1986, Table 1). ... The demographic factors associated with dropping out are well-known and were TABLE 3 Primary ...
High school graduation and dropout rates have long been used as a central indicator of education system productivity and effectiveness and of social and economic well-being.Today, interest in the accuracy and usefulness of these statistics is particularly acute owing to a confluence of circumstances, including changing demographics, new legislative mandates, and heightened political pressures ...
Student dropout is non-attendance from school or college for an extended period for no apparent cause. Tending to this issue necessitates a careful comprehension of the basic issues as well as an appropriate intervention strategy. Statistical approaches have acquired much importance in recent years in resolving the issue of student dropout. This is due to the fact that statistical techniques ...
Amsterdam School of Economics, Uni versity of Amsterdam, Roeterstraat 11 , 1017 LW Amsterdam. April 2013. Abstract. This paper reviews the growing literature on early school leaving. We clarify ...
High School Dropout Research Paper Example - Free download as PDF File (.pdf), Text File (.txt) or read online for free. high school dropout research paper example
Students who dropped out of the HRE Online master's degree program at the University of Illinois at Urbana-Champaign were the focus of this study. Students were admitted to the HRE Online degree program in cohorts of approximately 30 students. Data were collected from the first three cohorts of students.
Good Example Of Research Paper On High Dropout Rates Reduce Academic And Economic Opportunities For Latino Students. The graduation rate for Hispanic students has risen, but the dropout rate for Hispanic students is still the highest among their African-American, Asian, and White peers. This results in too few Hispanics enrolling in college or ...
In the USA, for example, the proportion of 14to 17-year-olds enrolled in high school increased from 11 percent in 1900 to 94 percent in 1978. However, dropout rates have been relatively stable since the beginning of the 1970s (between 5 and 10 percent in industrialized countries). 2.
A learner. can be called a dropout when the learner fails to enroll in school despite having reached the mandatory. school age. This paper aims to identify the root causes of school dropouts in ...